SIMD Processor Extensions Houffaneh Osman halio 029uottawa ca
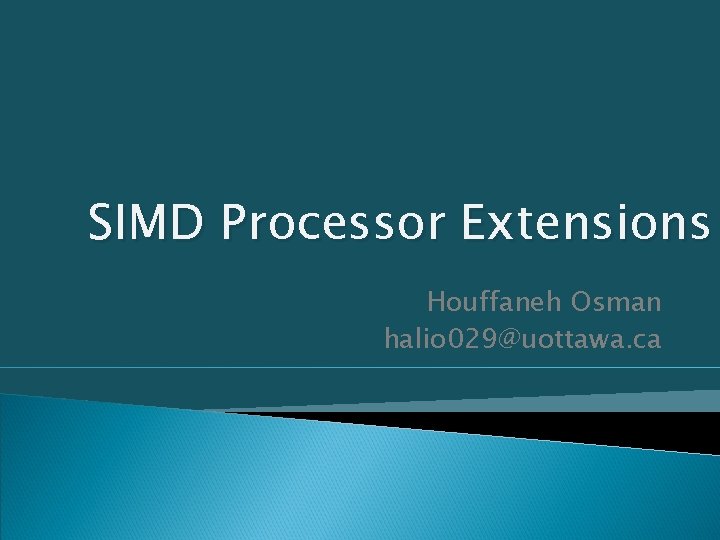
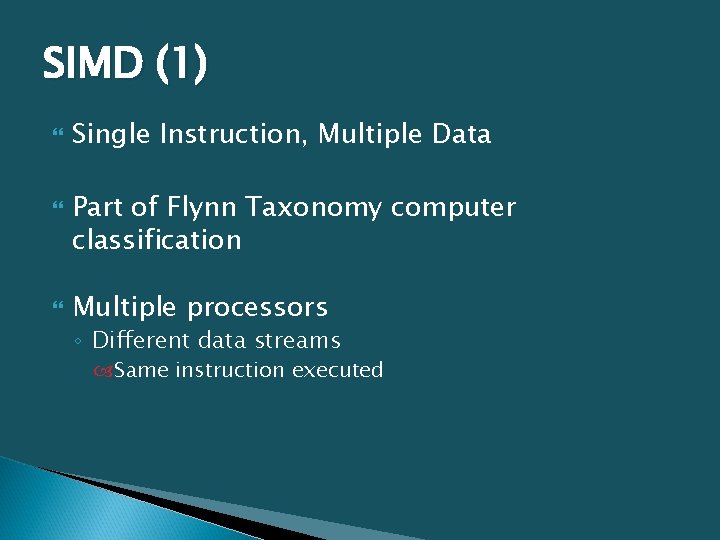
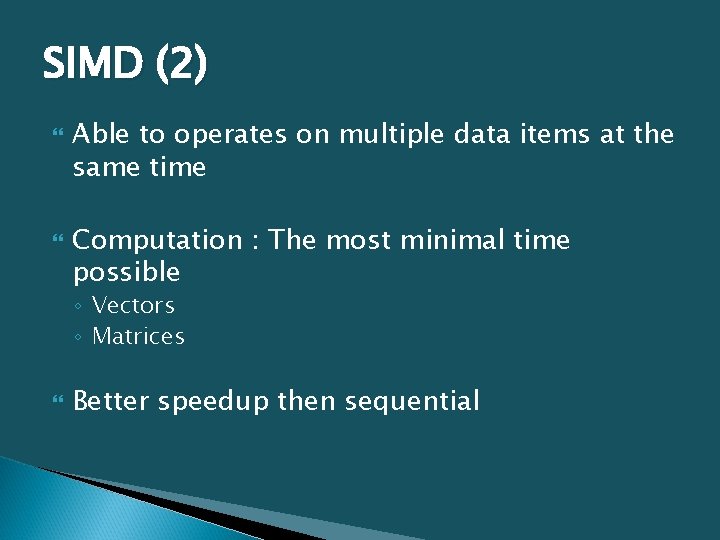
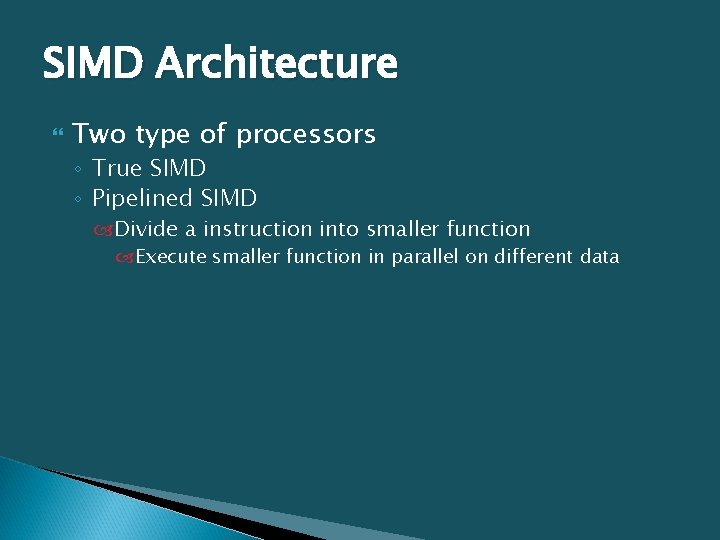
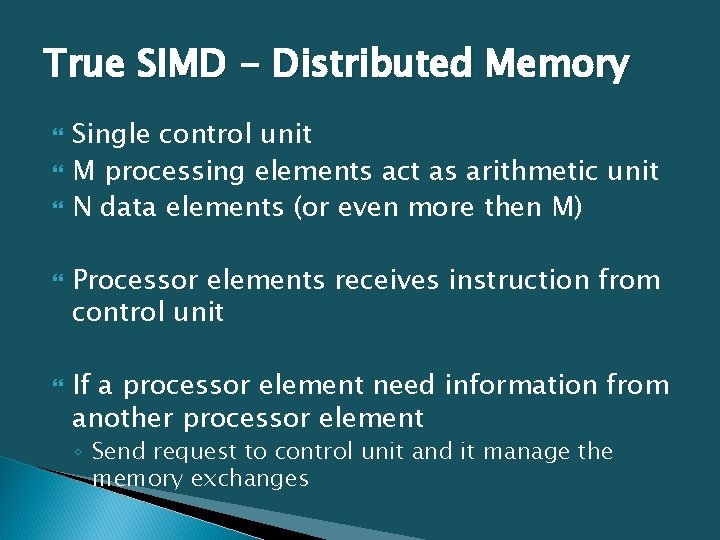
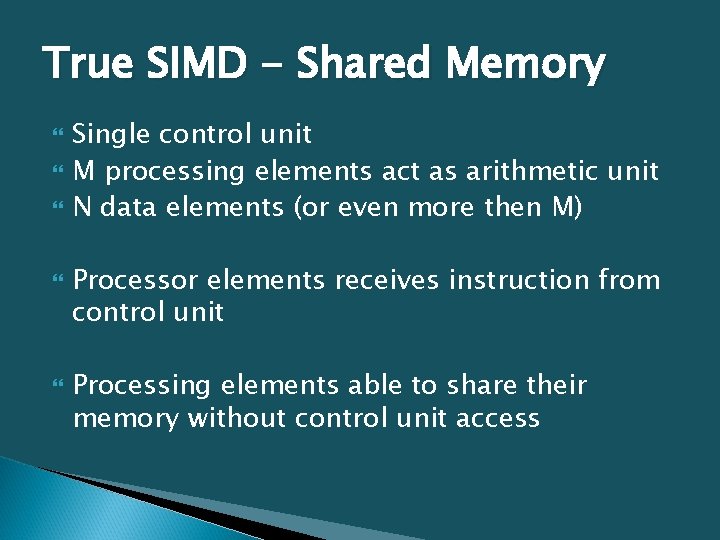
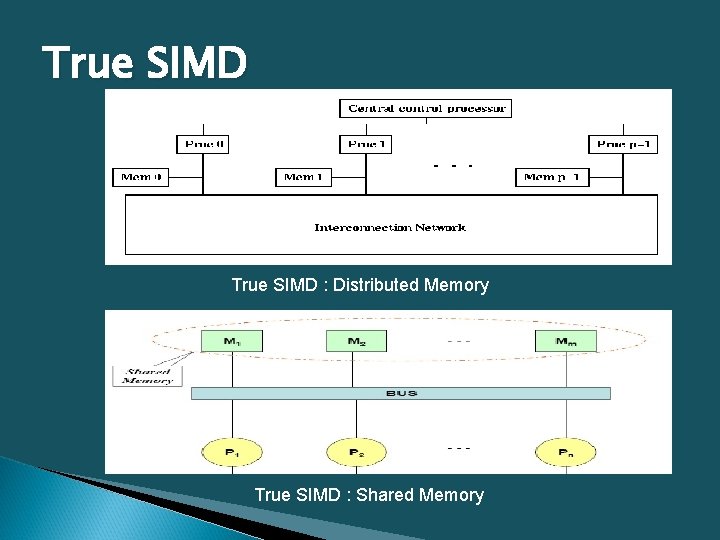
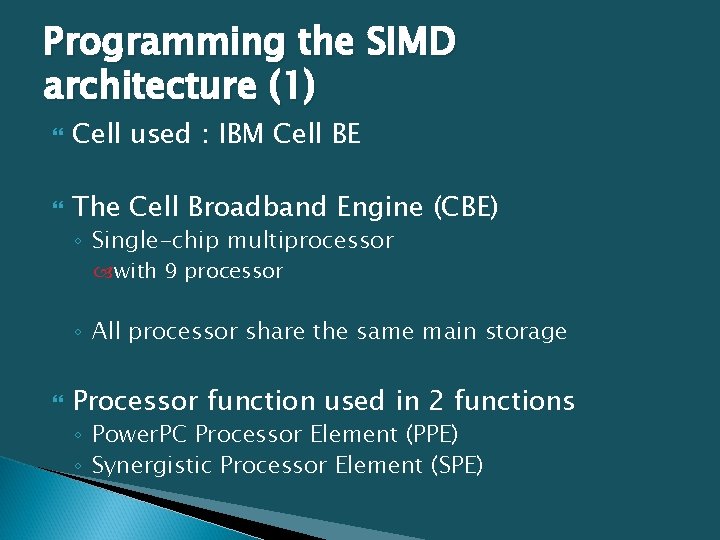
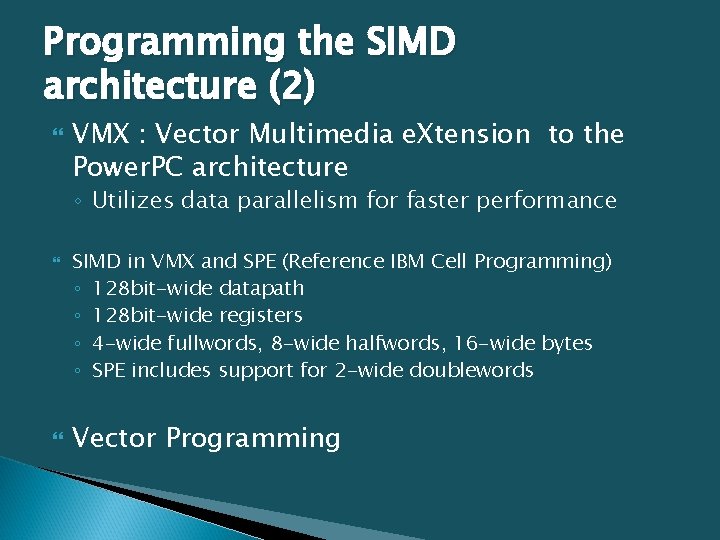
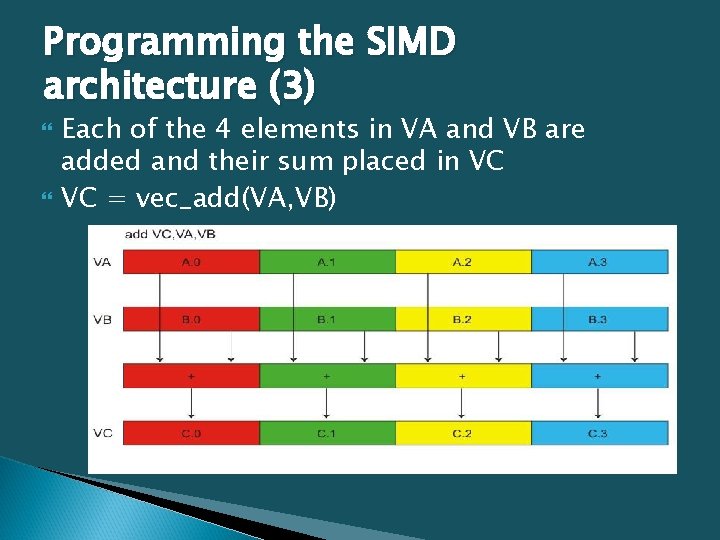
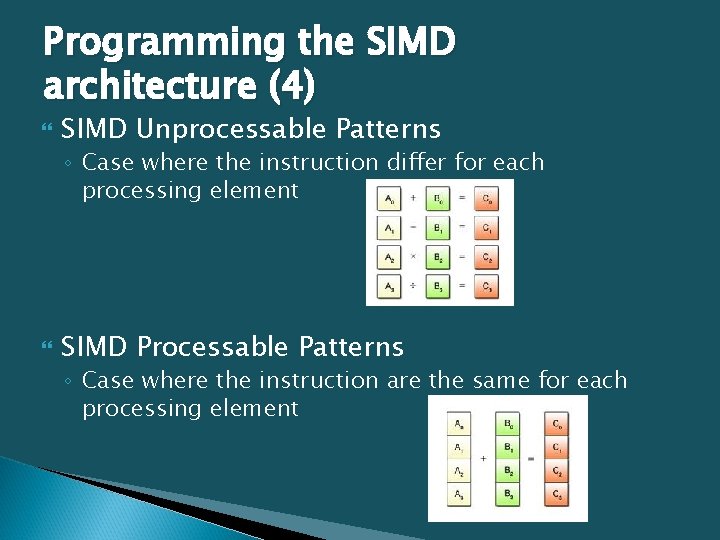
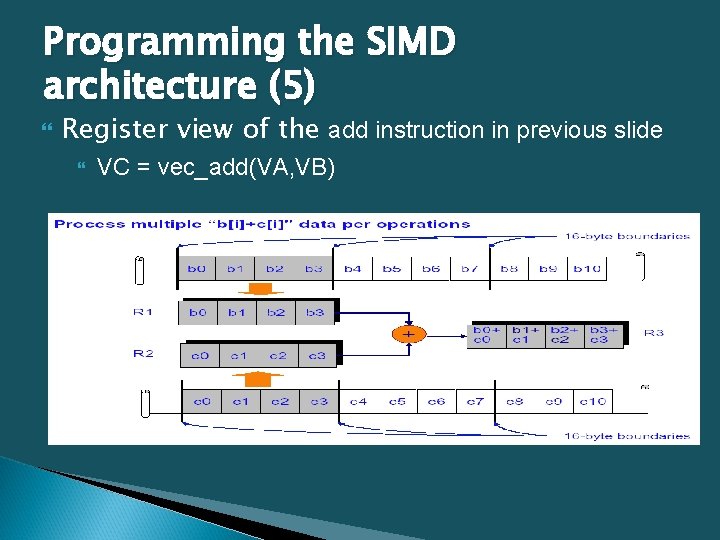
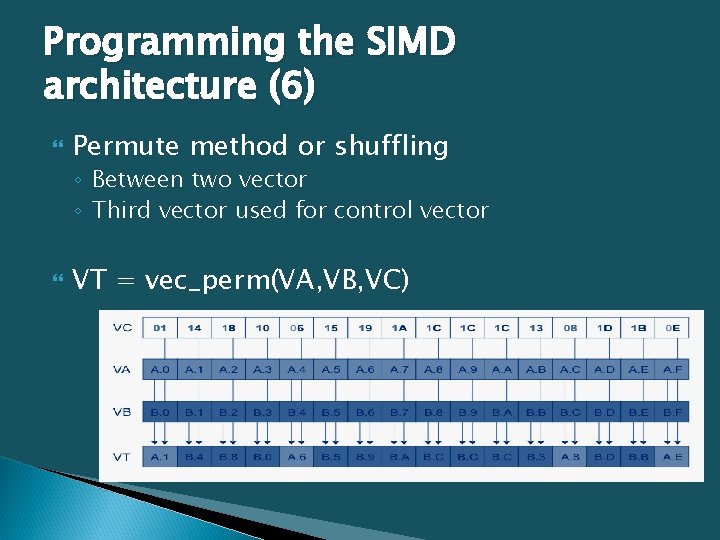
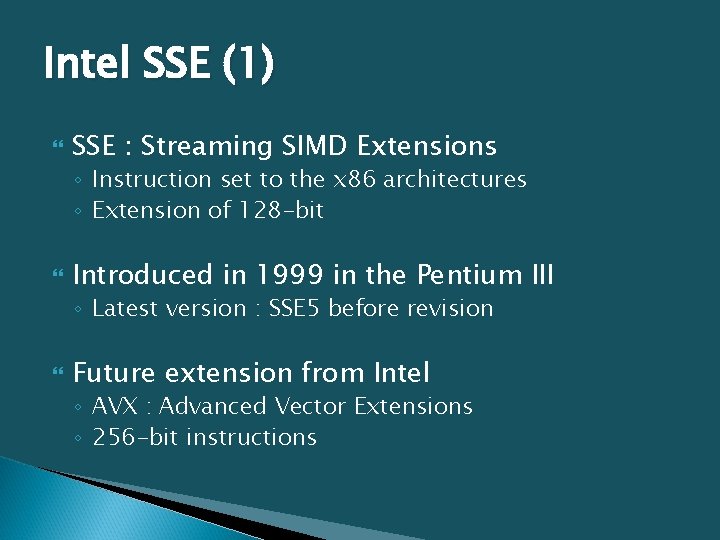
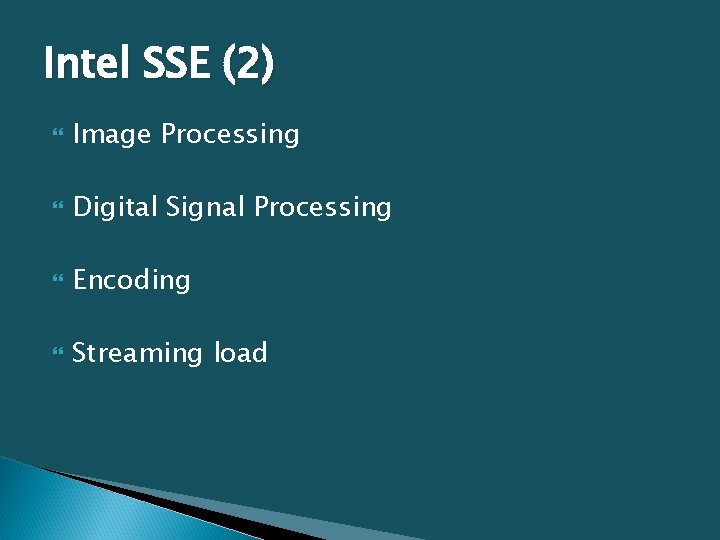
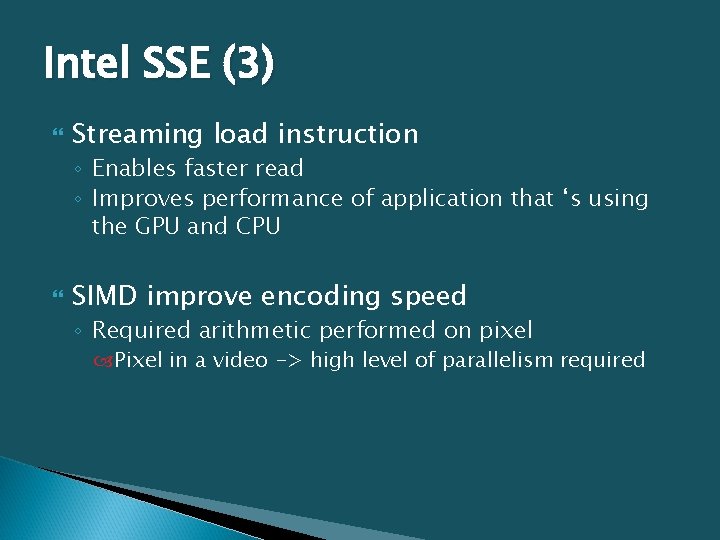
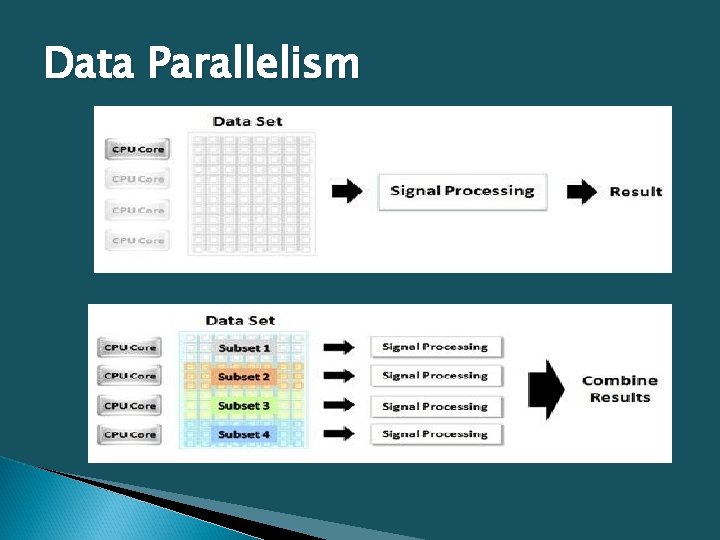
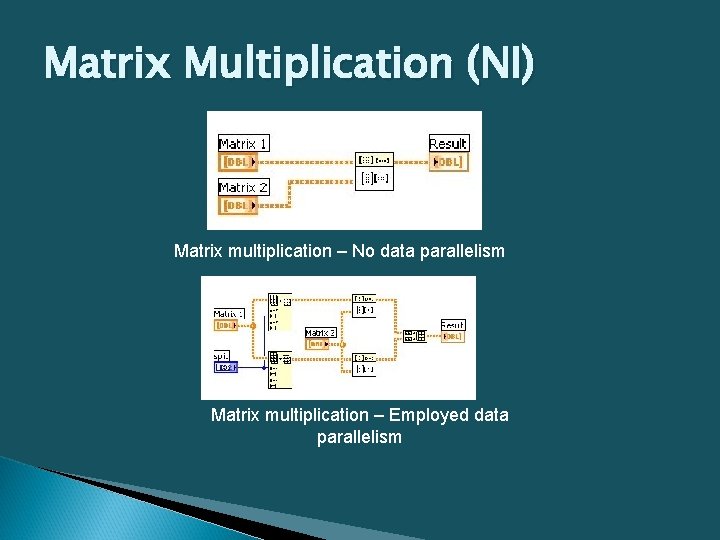
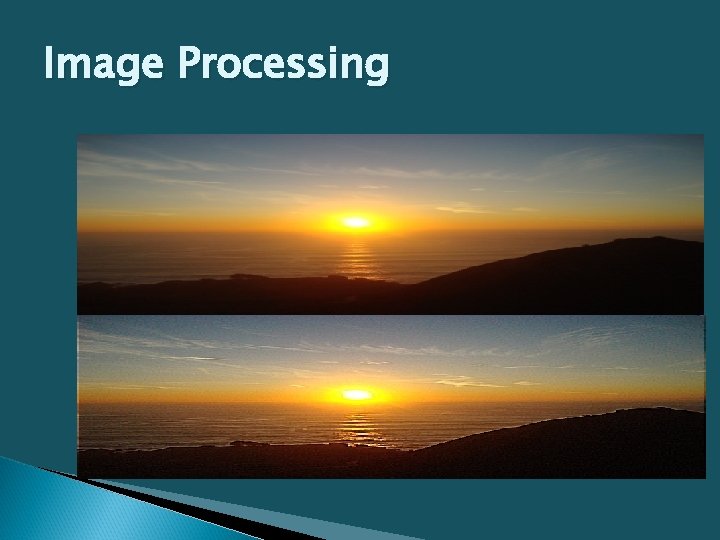
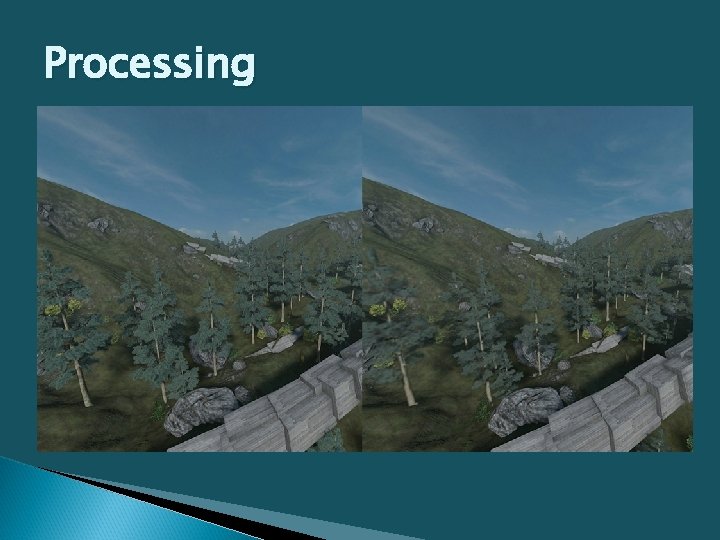
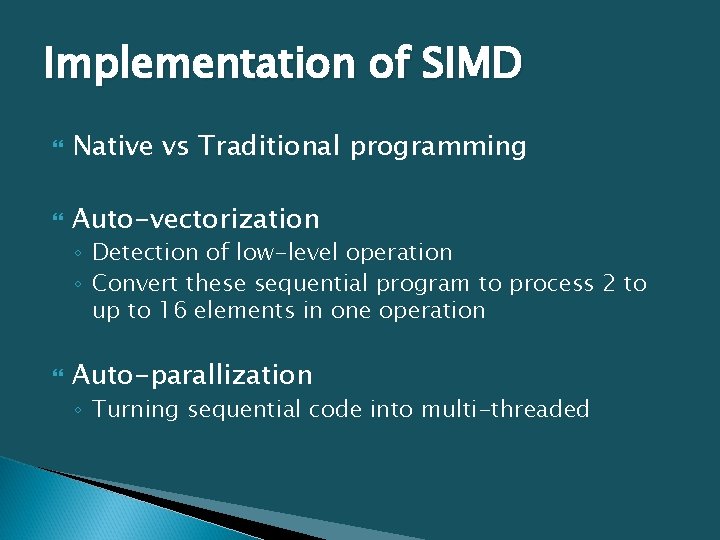
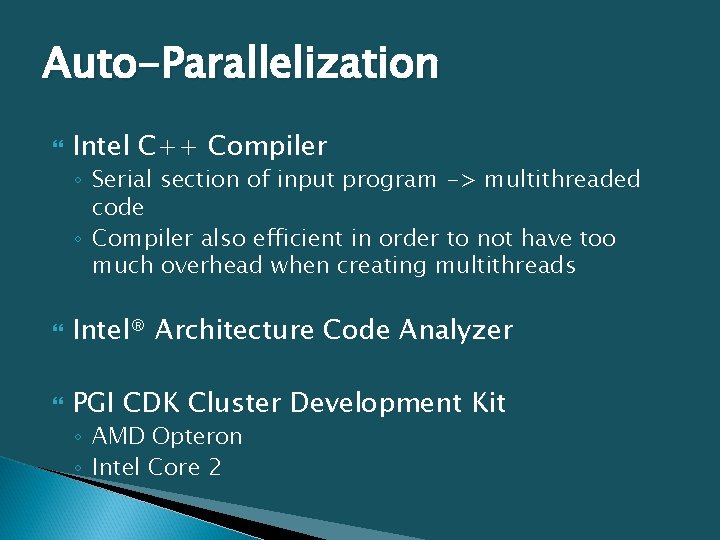
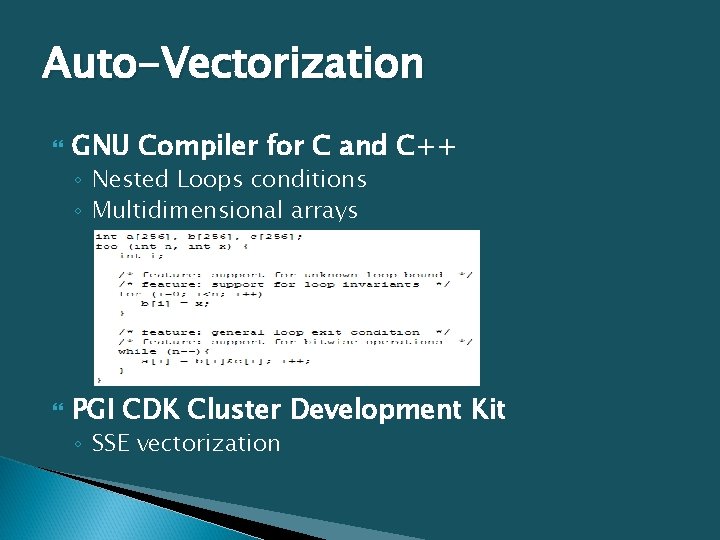
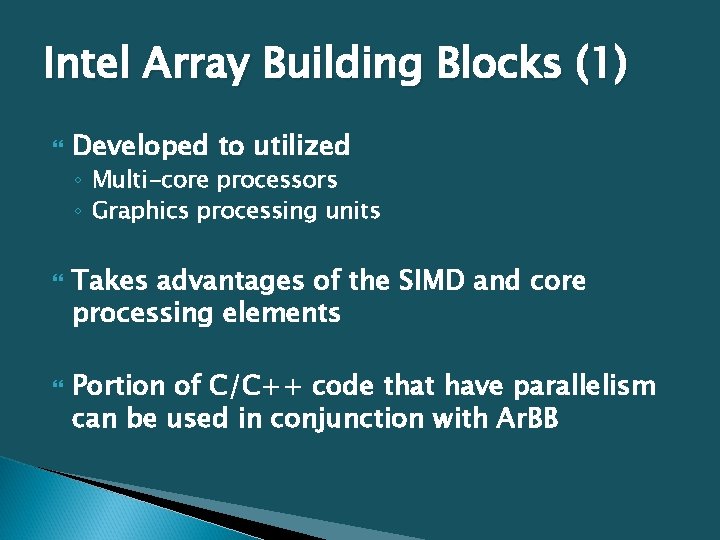
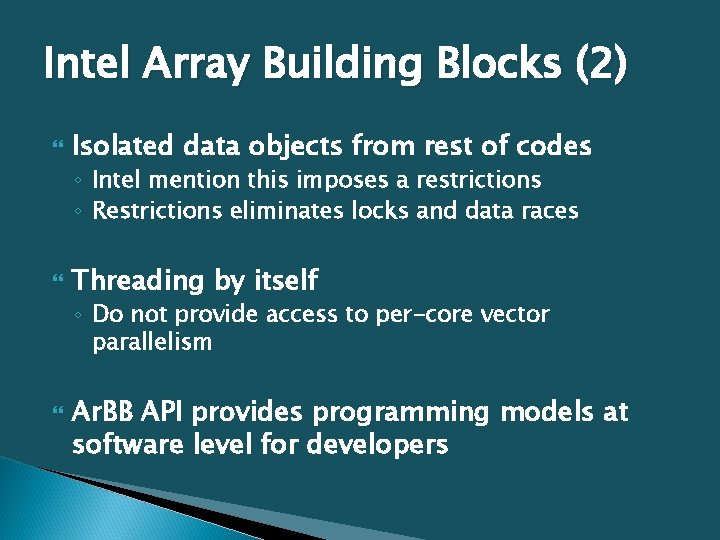
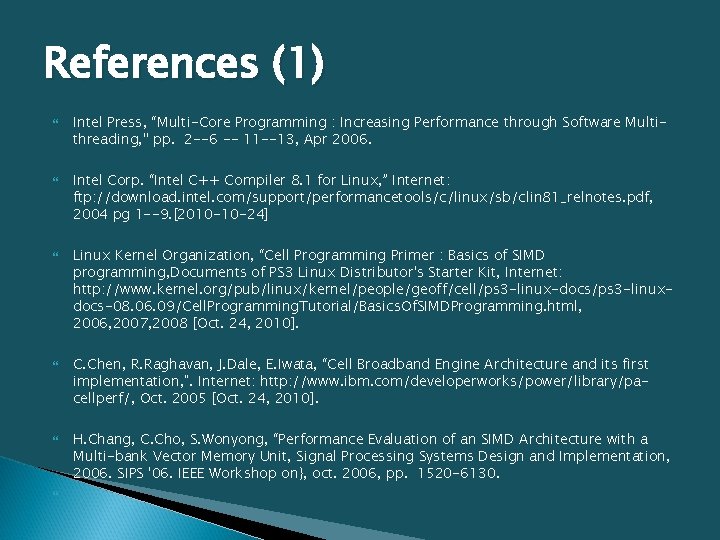
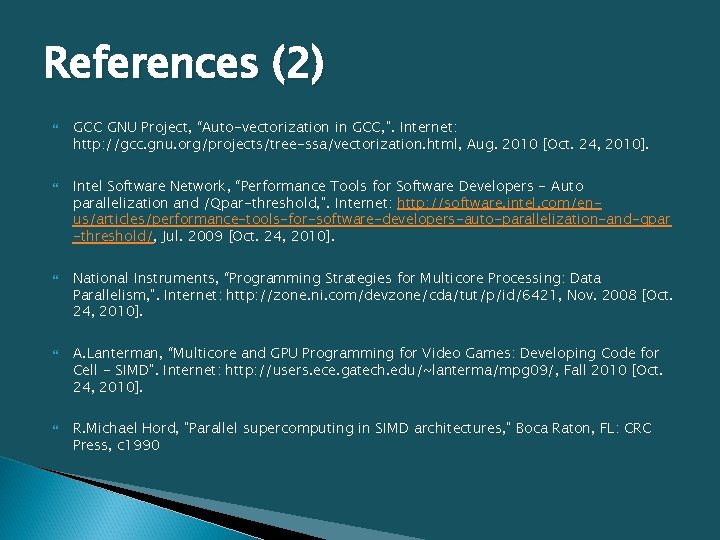
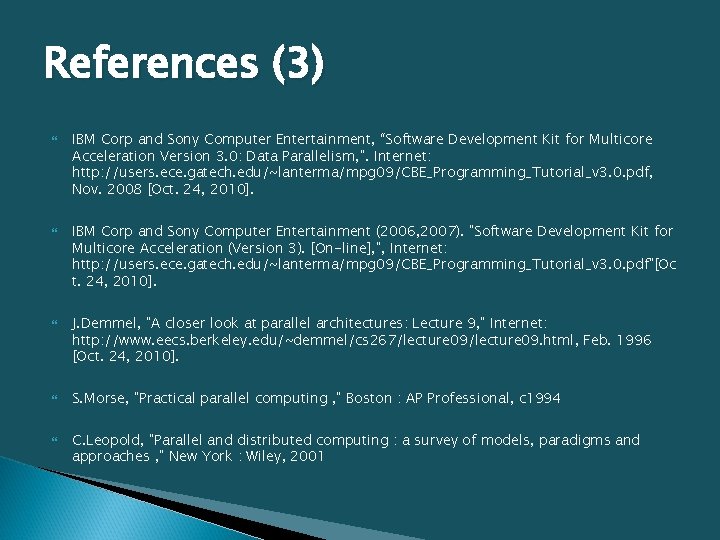
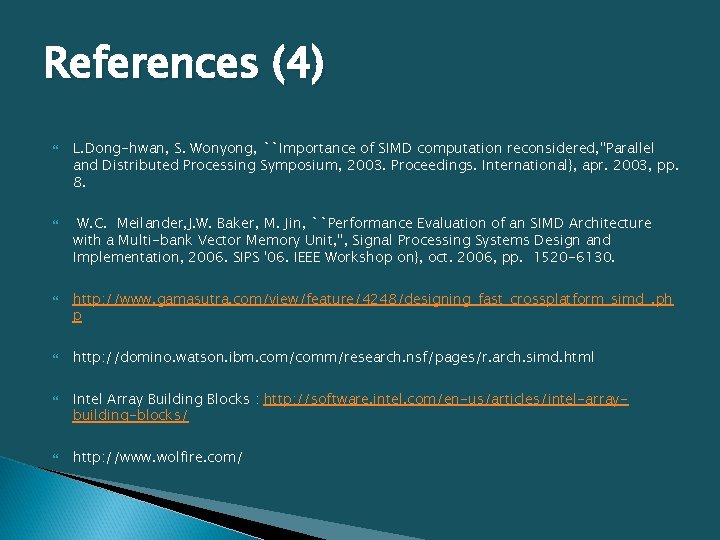
- Slides: 29
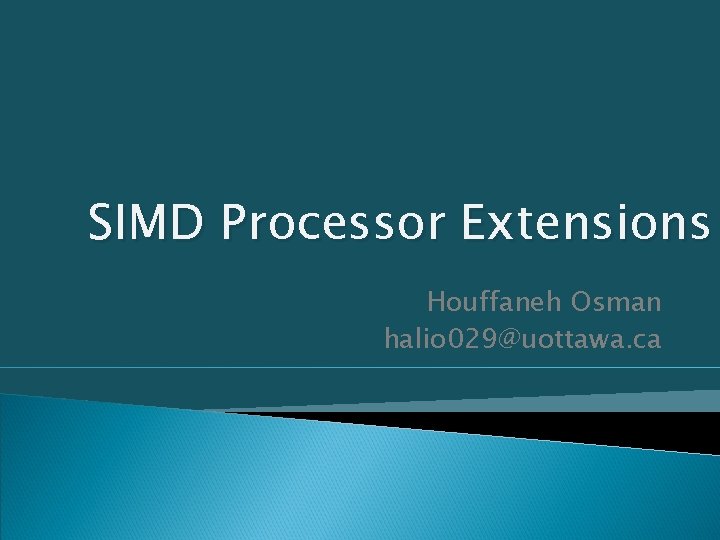
SIMD Processor Extensions Houffaneh Osman halio 029@uottawa. ca
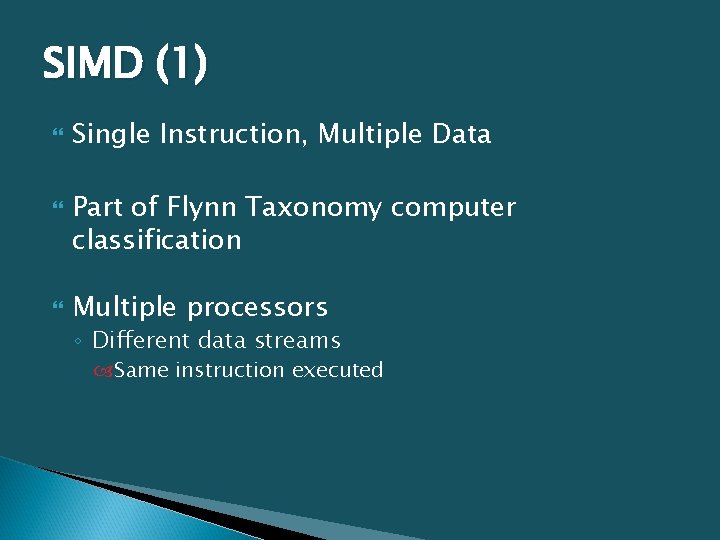
SIMD (1) Single Instruction, Multiple Data Part of Flynn Taxonomy computer classification Multiple processors ◦ Different data streams Same instruction executed
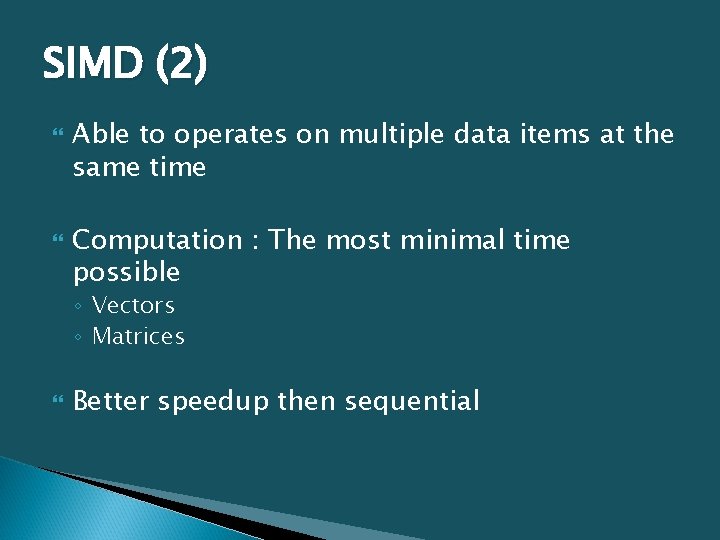
SIMD (2) Able to operates on multiple data items at the same time Computation : The most minimal time possible ◦ Vectors ◦ Matrices Better speedup then sequential
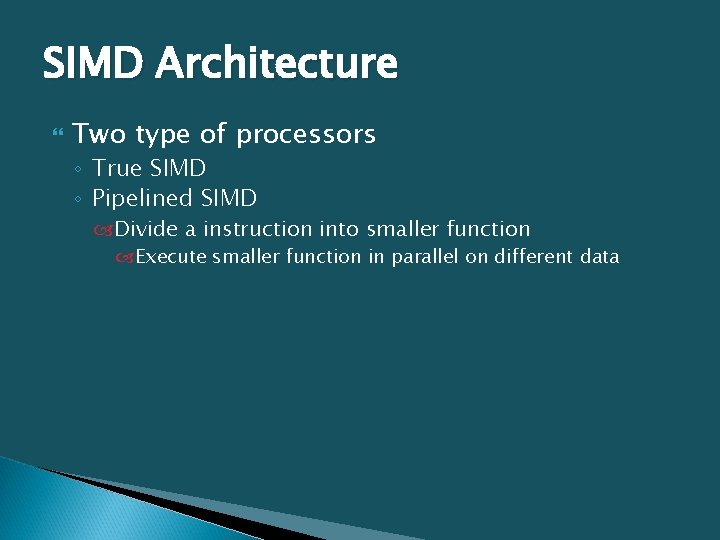
SIMD Architecture Two type of processors ◦ True SIMD ◦ Pipelined SIMD Divide a instruction into smaller function Execute smaller function in parallel on different data
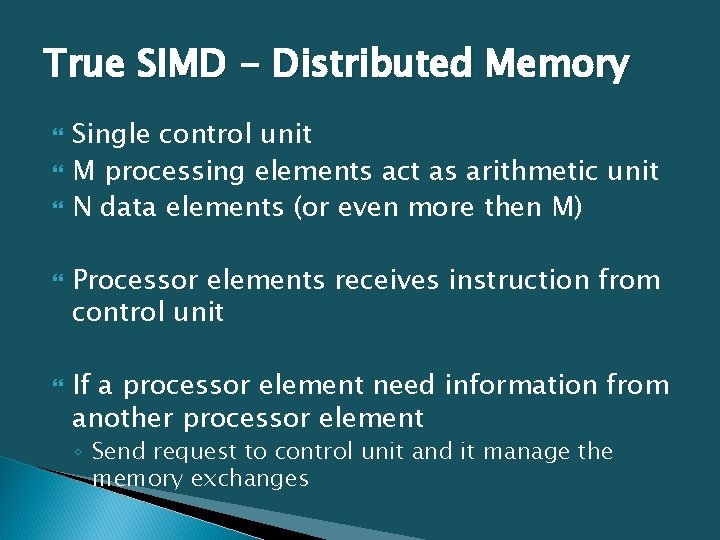
True SIMD - Distributed Memory Single control unit M processing elements act as arithmetic unit N data elements (or even more then M) Processor elements receives instruction from control unit If a processor element need information from another processor element ◦ Send request to control unit and it manage the memory exchanges
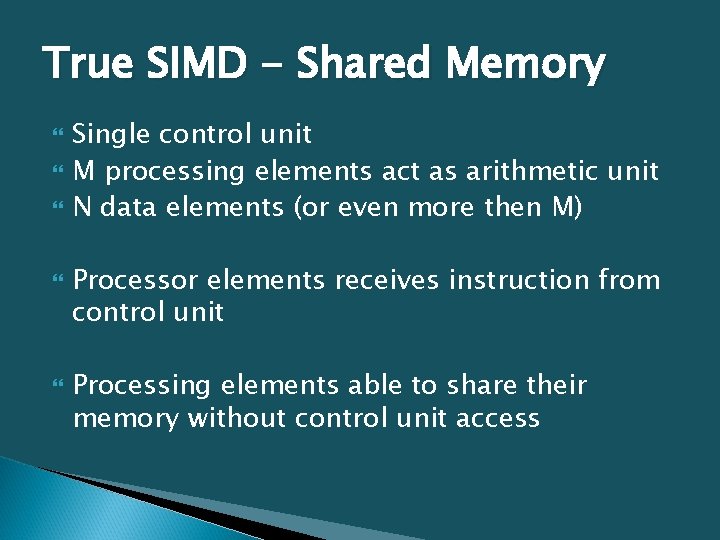
True SIMD - Shared Memory Single control unit M processing elements act as arithmetic unit N data elements (or even more then M) Processor elements receives instruction from control unit Processing elements able to share their memory without control unit access
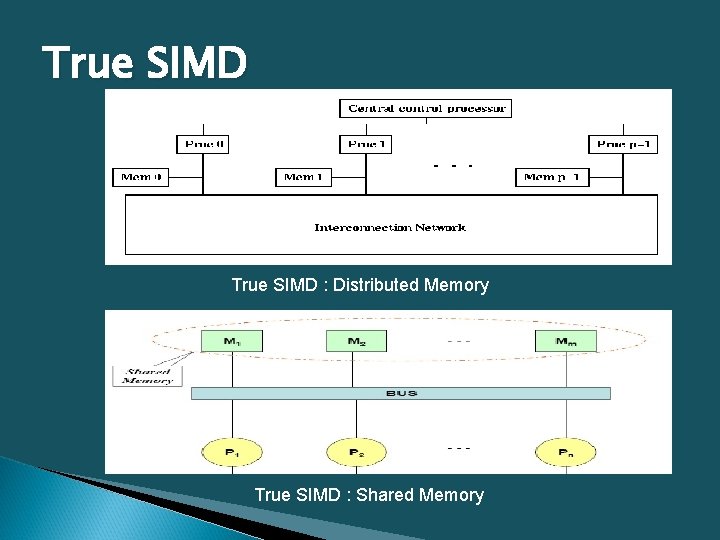
True SIMD : Distributed Memory True SIMD : Shared Memory
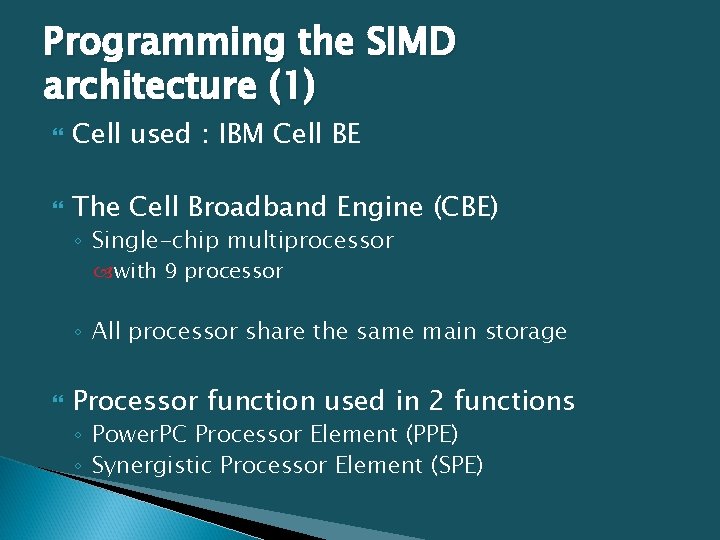
Programming the SIMD architecture (1) Cell used : IBM Cell BE The Cell Broadband Engine (CBE) ◦ Single-chip multiprocessor with 9 processor ◦ All processor share the same main storage Processor function used in 2 functions ◦ Power. PC Processor Element (PPE) ◦ Synergistic Processor Element (SPE)
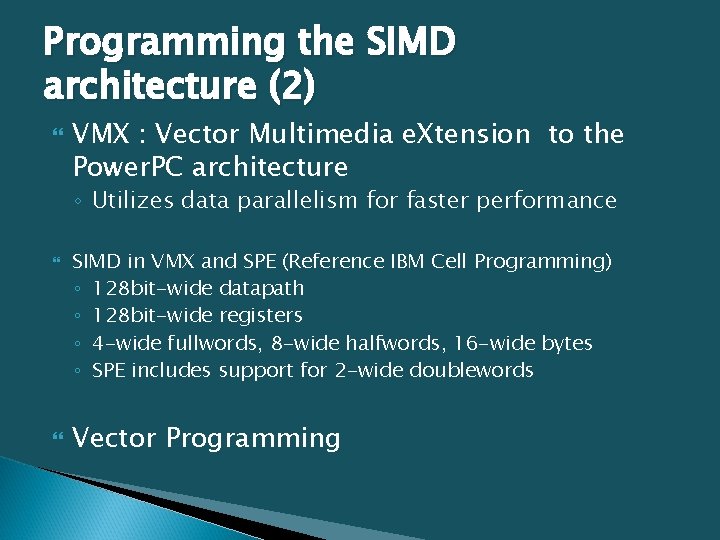
Programming the SIMD architecture (2) VMX : Vector Multimedia e. Xtension to the Power. PC architecture ◦ Utilizes data parallelism for faster performance SIMD in VMX and SPE (Reference IBM Cell Programming) ◦ 128 bit-wide datapath ◦ 128 bit-wide registers ◦ 4 -wide fullwords, 8 -wide halfwords, 16 -wide bytes ◦ SPE includes support for 2 -wide doublewords Vector Programming
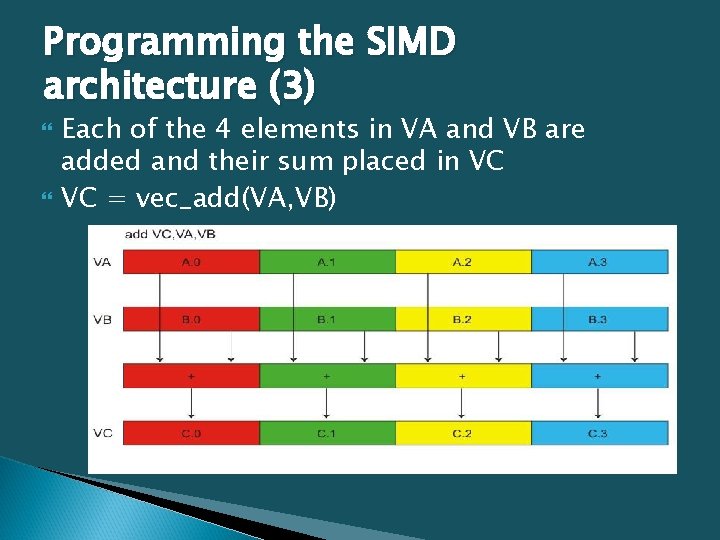
Programming the SIMD architecture (3) Each of the 4 elements in VA and VB are added and their sum placed in VC VC = vec_add(VA, VB)
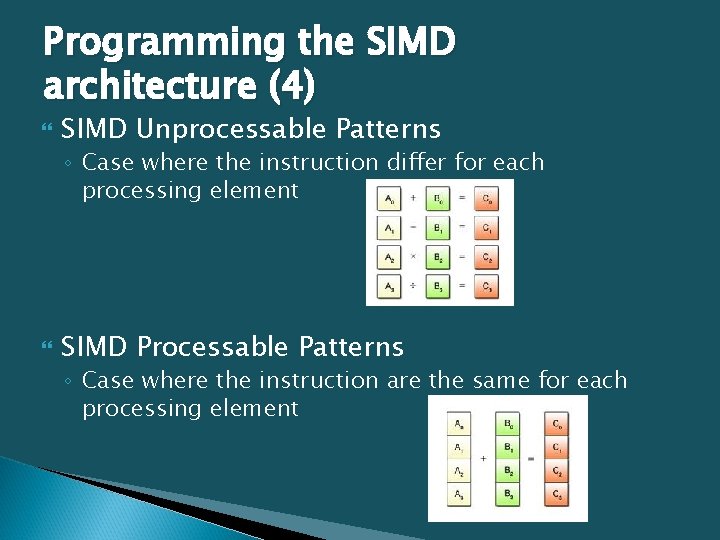
Programming the SIMD architecture (4) SIMD Unprocessable Patterns ◦ Case where the instruction differ for each processing element SIMD Processable Patterns ◦ Case where the instruction are the same for each processing element
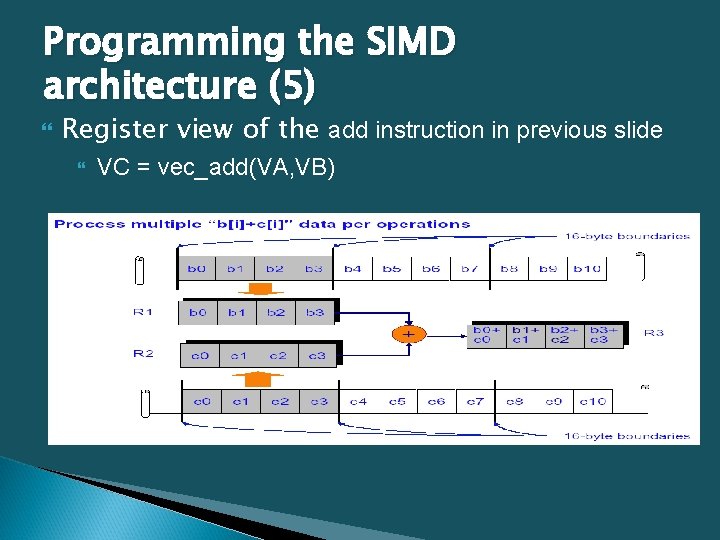
Programming the SIMD architecture (5) Register view of the add instruction in previous slide VC = vec_add(VA, VB)
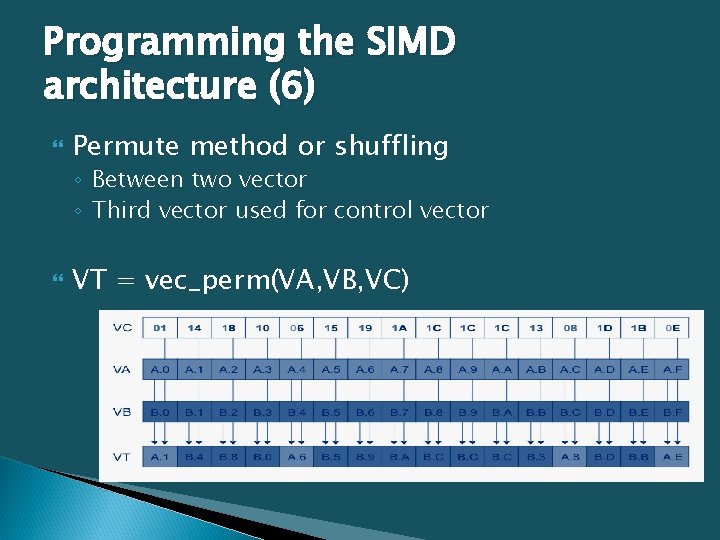
Programming the SIMD architecture (6) Permute method or shuffling ◦ Between two vector ◦ Third vector used for control vector VT = vec_perm(VA, VB, VC)
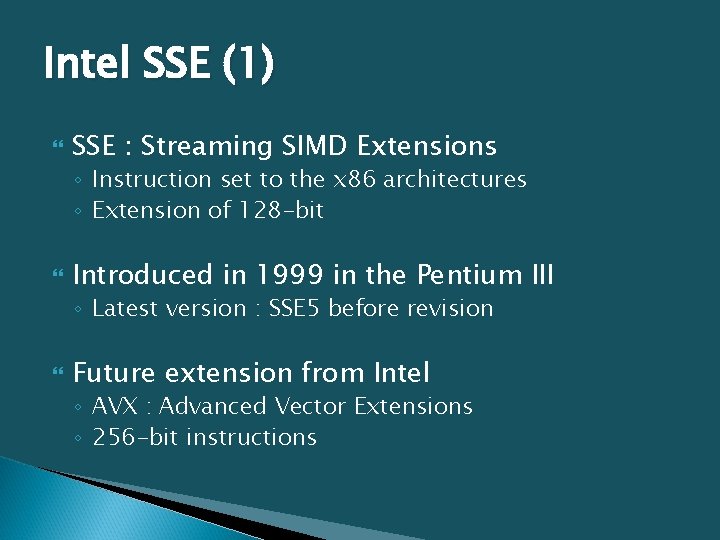
Intel SSE (1) SSE : Streaming SIMD Extensions ◦ Instruction set to the x 86 architectures ◦ Extension of 128 -bit Introduced in 1999 in the Pentium III ◦ Latest version : SSE 5 before revision Future extension from Intel ◦ AVX : Advanced Vector Extensions ◦ 256 -bit instructions
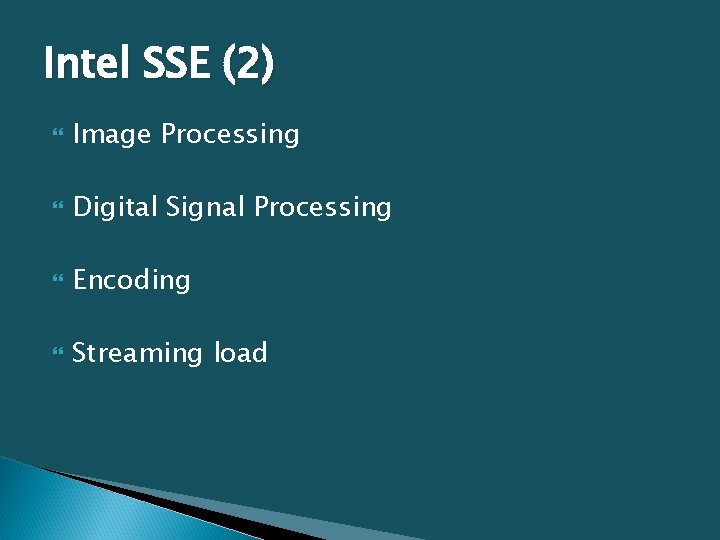
Intel SSE (2) Image Processing Digital Signal Processing Encoding Streaming load
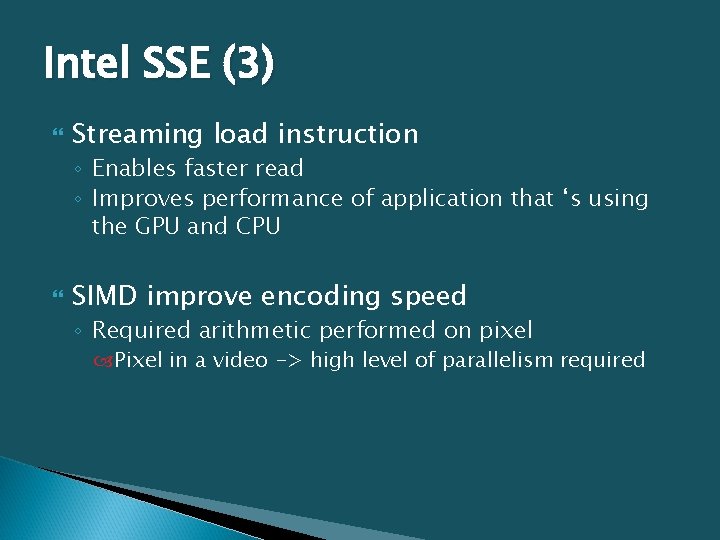
Intel SSE (3) Streaming load instruction ◦ Enables faster read ◦ Improves performance of application that ‘s using the GPU and CPU SIMD improve encoding speed ◦ Required arithmetic performed on pixel Pixel in a video -> high level of parallelism required
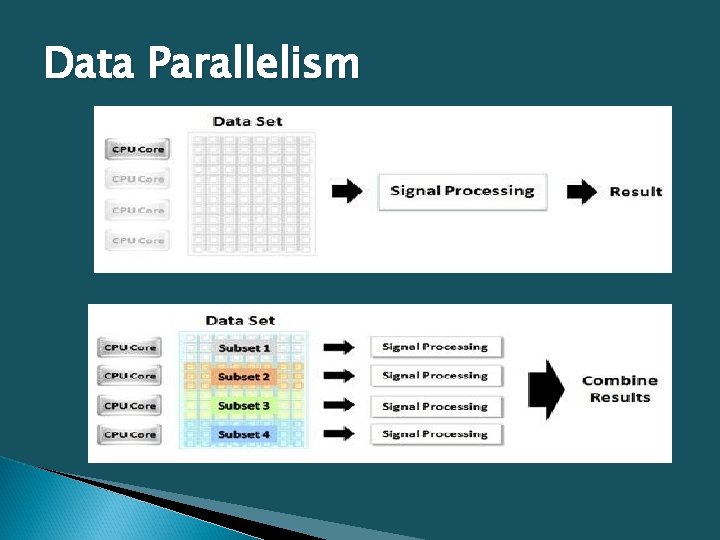
Data Parallelism
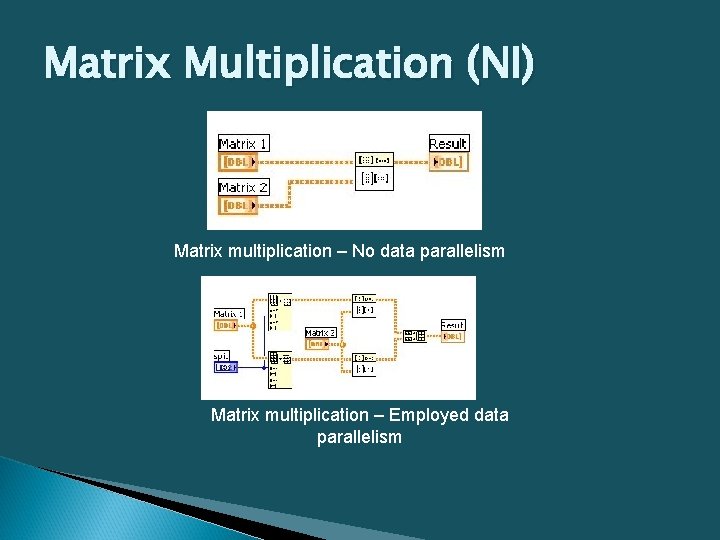
Matrix Multiplication (NI) Matrix multiplication – No data parallelism Matrix multiplication – Employed data parallelism
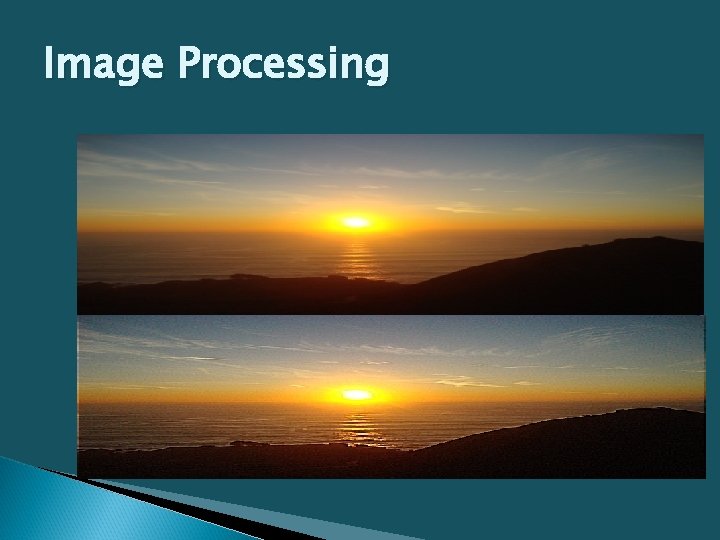
Image Processing
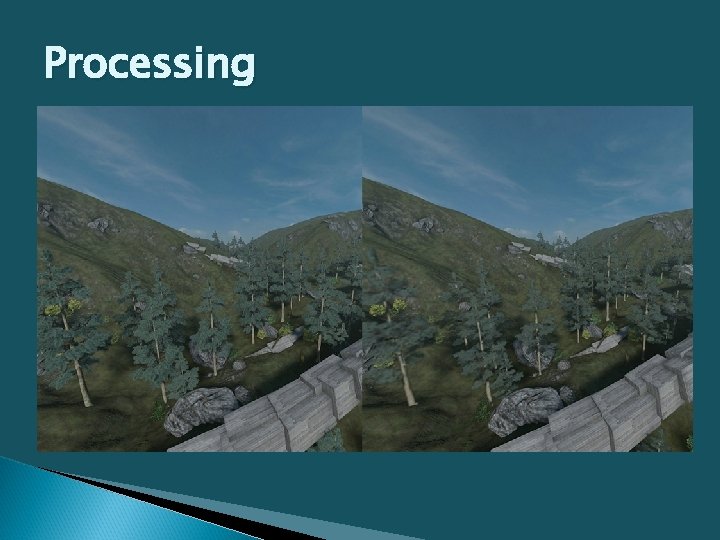
Processing
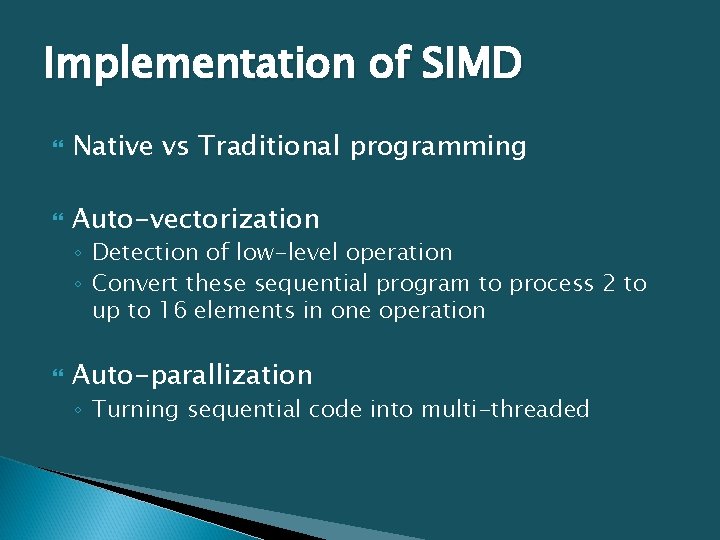
Implementation of SIMD Native vs Traditional programming Auto-vectorization ◦ Detection of low-level operation ◦ Convert these sequential program to process 2 to up to 16 elements in one operation Auto-parallization ◦ Turning sequential code into multi-threaded
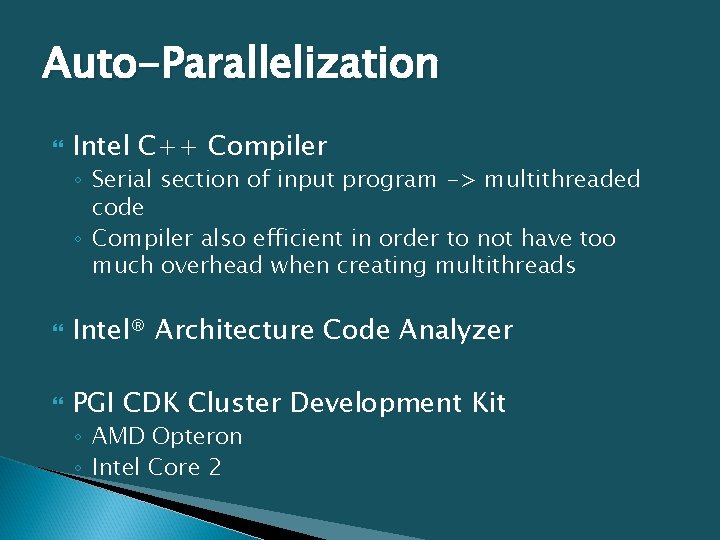
Auto-Parallelization Intel C++ Compiler ◦ Serial section of input program -> multithreaded code ◦ Compiler also efficient in order to not have too much overhead when creating multithreads Intel® Architecture Code Analyzer PGI CDK Cluster Development Kit ◦ AMD Opteron ◦ Intel Core 2
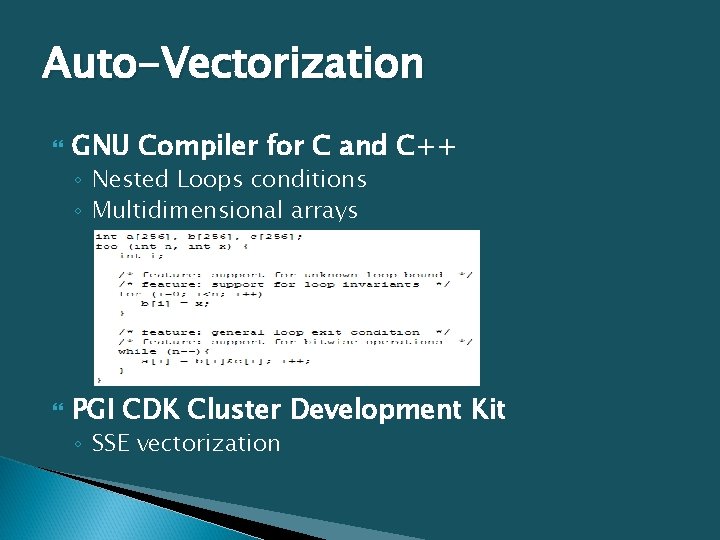
Auto-Vectorization GNU Compiler for C and C++ ◦ Nested Loops conditions ◦ Multidimensional arrays PGI CDK Cluster Development Kit ◦ SSE vectorization
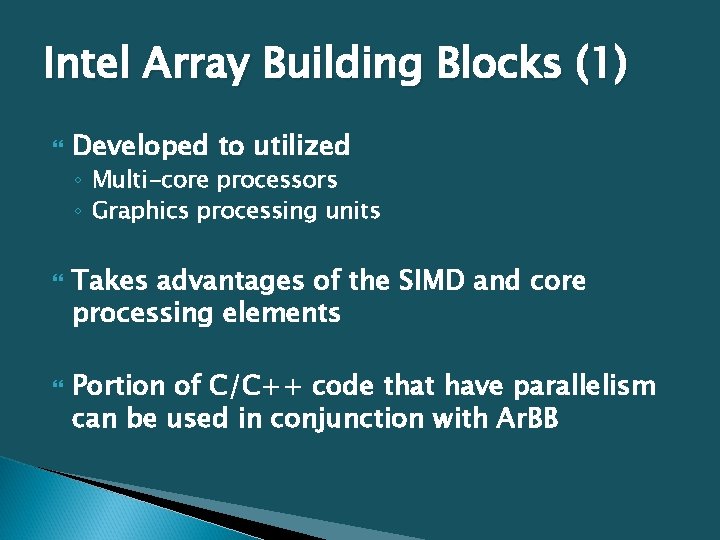
Intel Array Building Blocks (1) Developed to utilized ◦ Multi-core processors ◦ Graphics processing units Takes advantages of the SIMD and core processing elements Portion of C/C++ code that have parallelism can be used in conjunction with Ar. BB
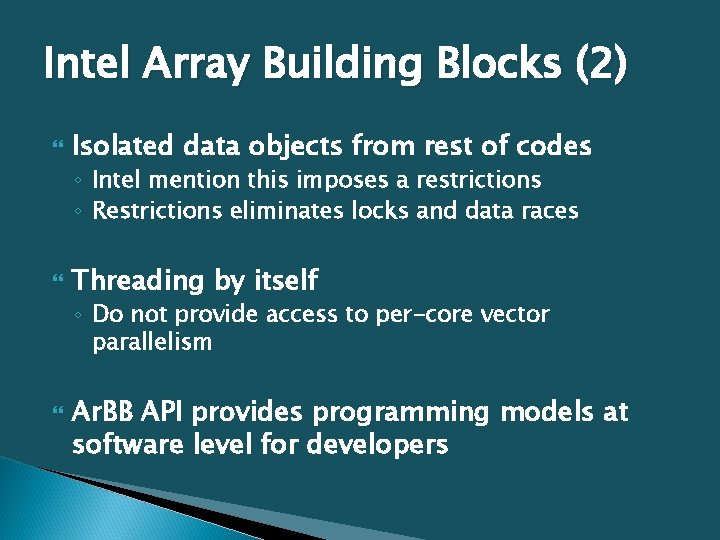
Intel Array Building Blocks (2) Isolated data objects from rest of codes ◦ Intel mention this imposes a restrictions ◦ Restrictions eliminates locks and data races Threading by itself ◦ Do not provide access to per-core vector parallelism Ar. BB API provides programming models at software level for developers
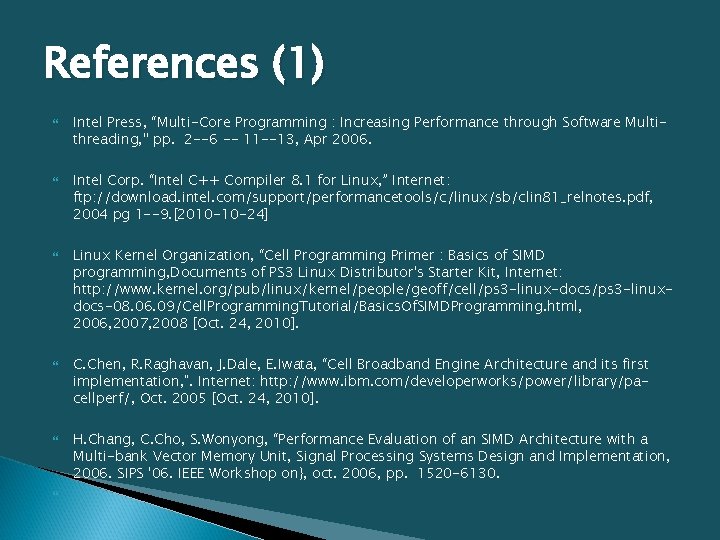
References (1) Intel Press, “Multi-Core Programming : Increasing Performance through Software Multithreading, '' pp. 2 --6 -- 11 --13, Apr 2006. Intel Corp. “Intel C++ Compiler 8. 1 for Linux, ” Internet: ftp: //download. intel. com/support/performancetools/c/linux/sb/clin 81_relnotes. pdf, 2004 pg 1 --9. [2010 -10 -24] Linux Kernel Organization, “Cell Programming Primer : Basics of SIMD programming, Documents of PS 3 Linux Distributor's Starter Kit, Internet: http: //www. kernel. org/pub/linux/kernel/people/geoff/cell/ps 3 -linux-docs/ps 3 -linuxdocs-08. 06. 09/Cell. Programming. Tutorial/Basics. Of. SIMDProgramming. html, 2006, 2007, 2008 [Oct. 24, 2010]. C. Chen, R. Raghavan, J. Dale, E. Iwata, “Cell Broadband Engine Architecture and its first implementation, ". Internet: http: //www. ibm. com/developerworks/power/library/pacellperf/, Oct. 2005 [Oct. 24, 2010]. H. Chang, C. Cho, S. Wonyong, “Performance Evaluation of an SIMD Architecture with a Multi-bank Vector Memory Unit, Signal Processing Systems Design and Implementation, 2006. SIPS '06. IEEE Workshop on}, oct. 2006, pp. 1520 -6130.
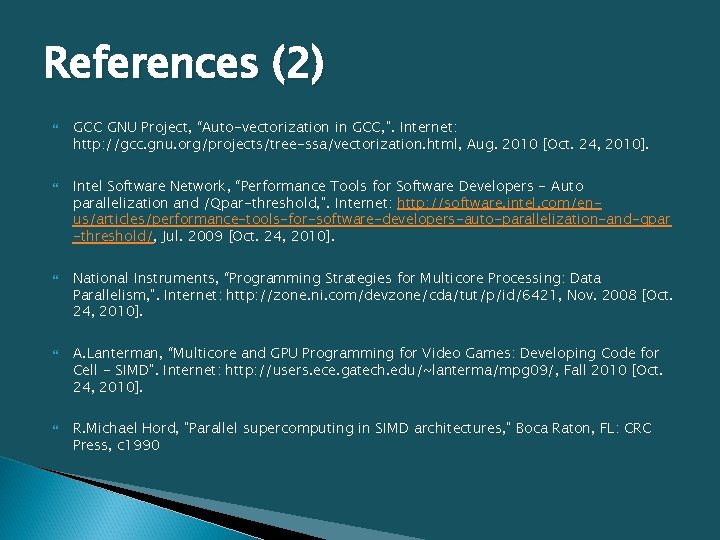
References (2) GCC GNU Project, “Auto-vectorization in GCC, ". Internet: http: //gcc. gnu. org/projects/tree-ssa/vectorization. html, Aug. 2010 [Oct. 24, 2010]. Intel Software Network, “Performance Tools for Software Developers - Auto parallelization and /Qpar-threshold, ". Internet: http: //software. intel. com/enus/articles/performance-tools-for-software-developers-auto-parallelization-and-qpar -threshold/, Jul. 2009 [Oct. 24, 2010]. National Instruments, “Programming Strategies for Multicore Processing: Data Parallelism, ". Internet: http: //zone. ni. com/devzone/cda/tut/p/id/6421, Nov. 2008 [Oct. 24, 2010]. A. Lanterman, “Multicore and GPU Programming for Video Games: Developing Code for Cell - SIMD". Internet: http: //users. ece. gatech. edu/~lanterma/mpg 09/, Fall 2010 [Oct. 24, 2010]. R. Michael Hord, "Parallel supercomputing in SIMD architectures, " Boca Raton, FL: CRC Press, c 1990
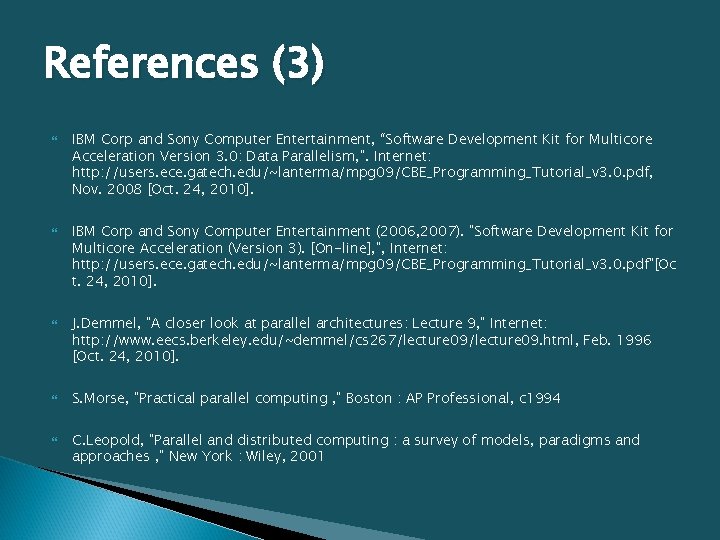
References (3) IBM Corp and Sony Computer Entertainment, “Software Development Kit for Multicore Acceleration Version 3. 0: Data Parallelism, ". Internet: http: //users. ece. gatech. edu/~lanterma/mpg 09/CBE_Programming_Tutorial_v 3. 0. pdf, Nov. 2008 [Oct. 24, 2010]. IBM Corp and Sony Computer Entertainment (2006, 2007). "Software Development Kit for Multicore Acceleration (Version 3). [On-line], ", Internet: http: //users. ece. gatech. edu/~lanterma/mpg 09/CBE_Programming_Tutorial_v 3. 0. pdf"[Oc t. 24, 2010]. J. Demmel, "A closer look at parallel architectures: Lecture 9, " Internet: http: //www. eecs. berkeley. edu/~demmel/cs 267/lecture 09. html, Feb. 1996 [Oct. 24, 2010]. S. Morse, "Practical parallel computing , " Boston : AP Professional, c 1994 C. Leopold, "Parallel and distributed computing : a survey of models, paradigms and approaches , " New York : Wiley, 2001
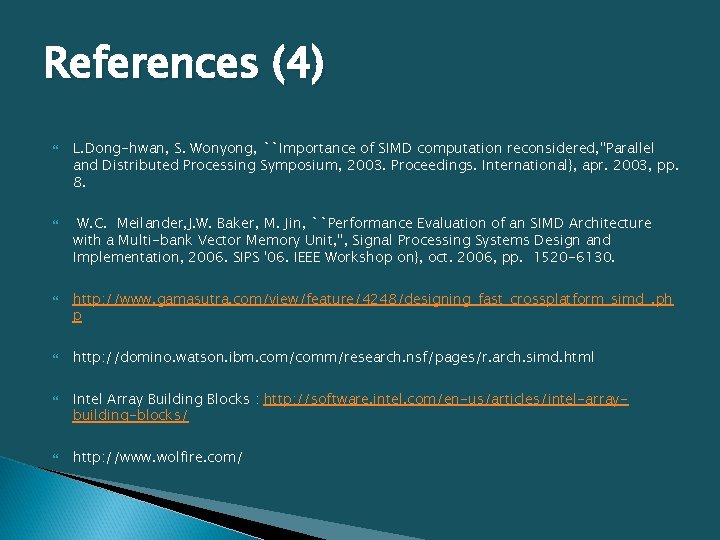
References (4) L. Dong-hwan, S. Wonyong, ``Importance of SIMD computation reconsidered, ''Parallel and Distributed Processing Symposium, 2003. Proceedings. International}, apr. 2003, pp. 8. W. C. Meilander, J. W. Baker, M. Jin, ``Performance Evaluation of an SIMD Architecture with a Multi-bank Vector Memory Unit, '', Signal Processing Systems Design and Implementation, 2006. SIPS '06. IEEE Workshop on}, oct. 2006, pp. 1520 -6130. http: //www. gamasutra. com/view/feature/4248/designing_fast_crossplatform_simd_. ph p http: //domino. watson. ibm. com/comm/research. nsf/pages/r. arch. simd. html Intel Array Building Blocks : http: //software. intel. com/en-us/articles/intel-arraybuilding-blocks/ http: //www. wolfire. com/
Simd extensions
_mm_add_ss
Simd vs gpu
Intel simd instruction set
Associative array processing in parallel computing
Flynn’s classification
Simd in computer architecture
Sisd processor
Intel simd
Simd architecture ppt
Systolic array vs simd
Simd units
Simd.scot
Ytl osman kimdir
Osman öğünçlü
Osman parlaktuna
Ergün önal biyoloji öğretmeni
Osman imperia
Bandung4d
Rhyme time house of games examples
Osman keysan
Komik mani
Osman edin
Gay osman ifşa
Ali osman alaca
Turkcell arge
Hassibe osman dewitt
Dr nicolas osman
Tez2yok
Osman fırat turan