Research Done Differently Comparative Effectiveness Research PatientCentered Outcomes
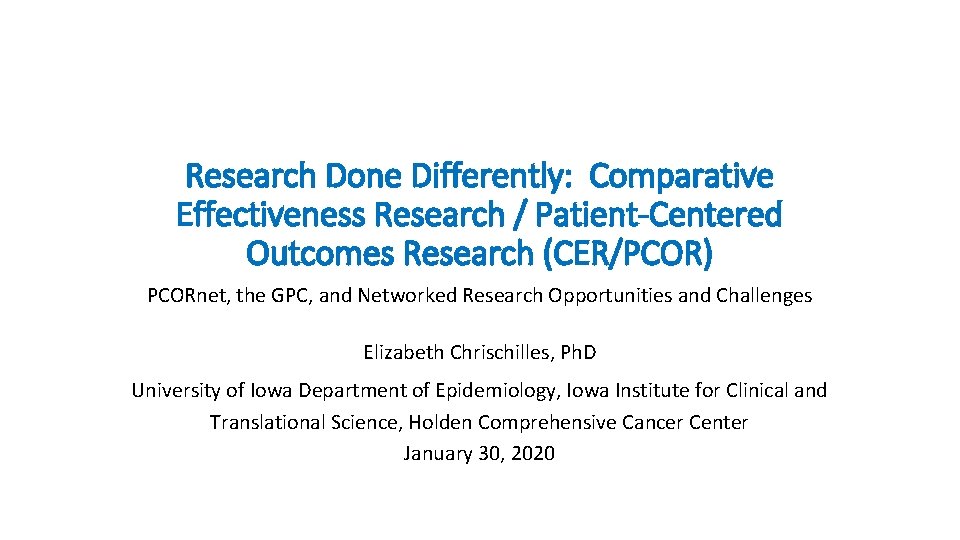
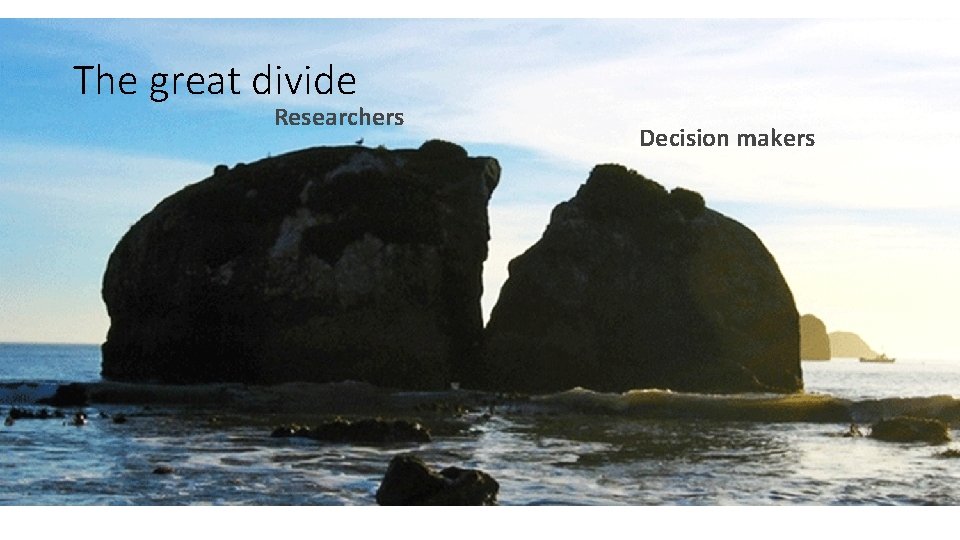
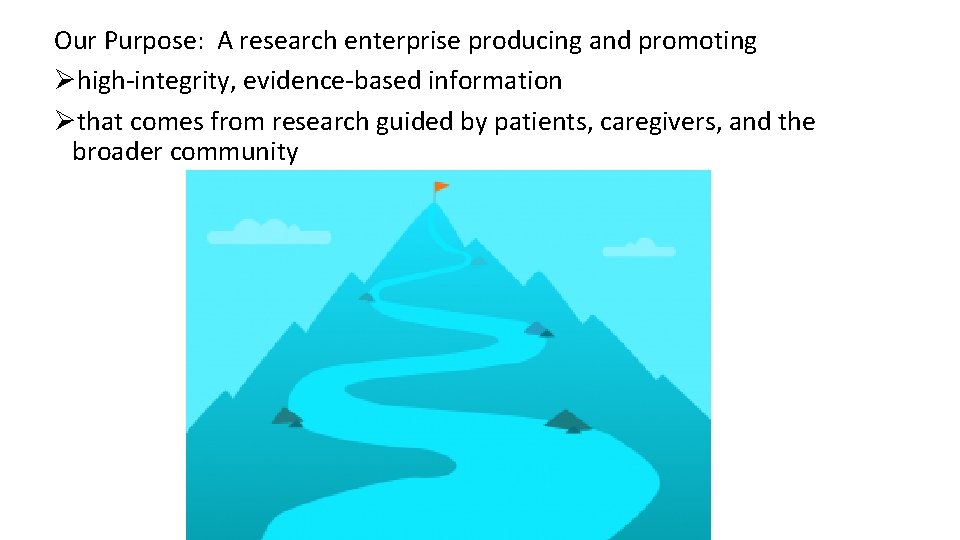
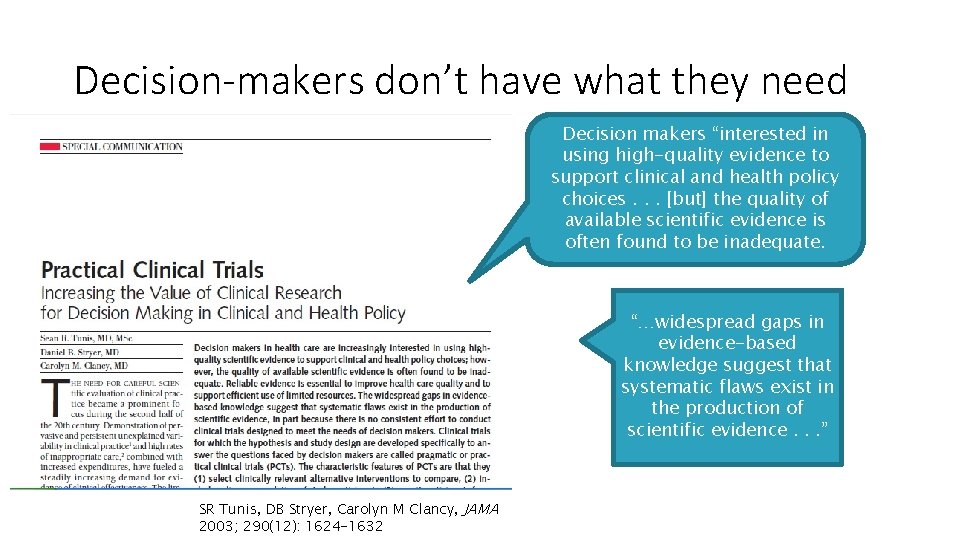
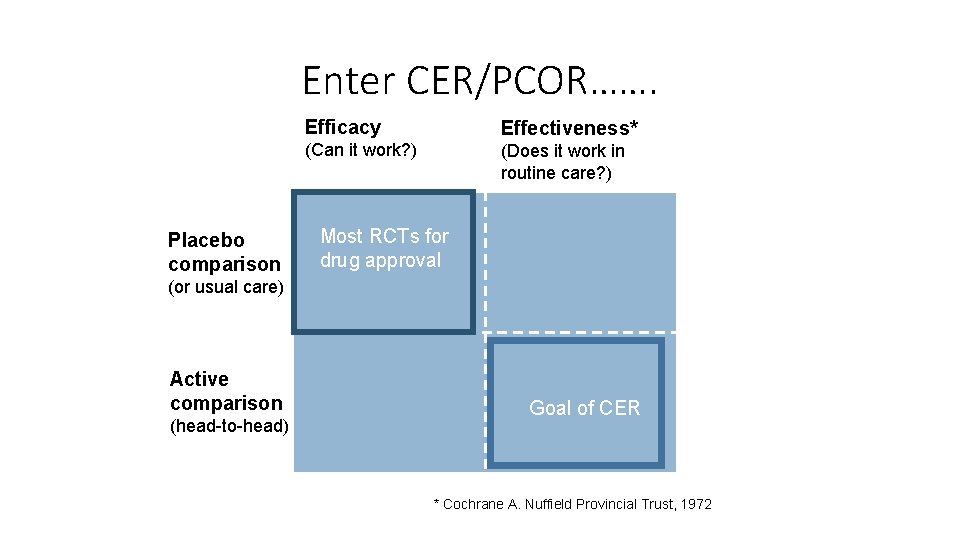
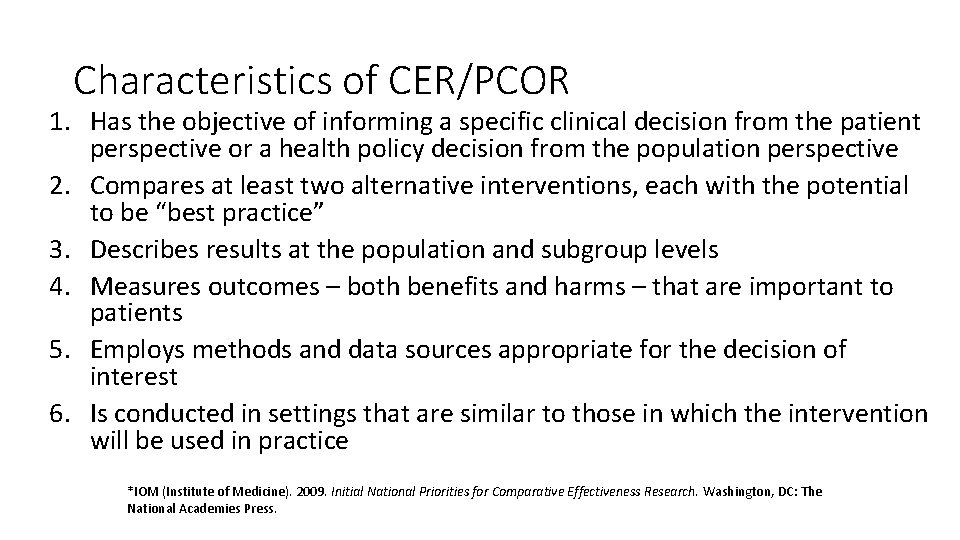
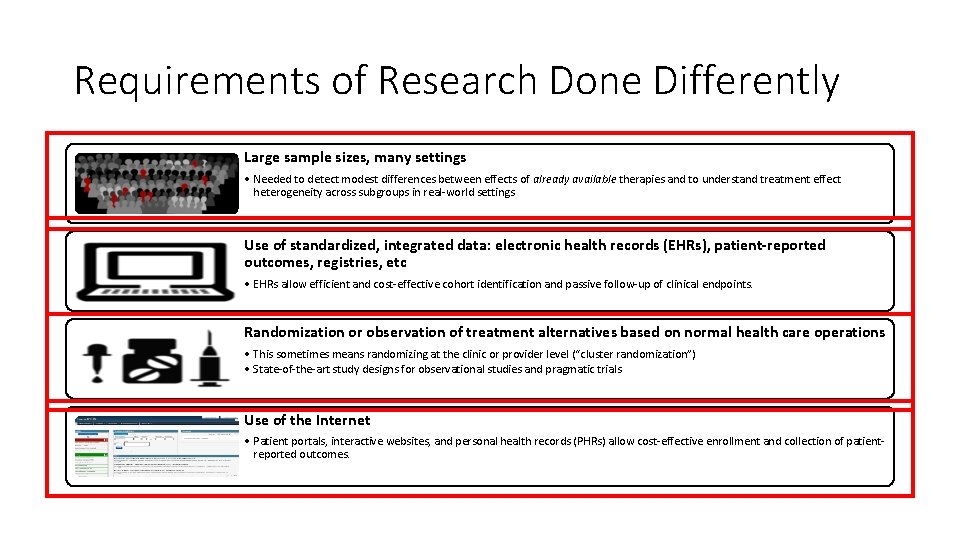
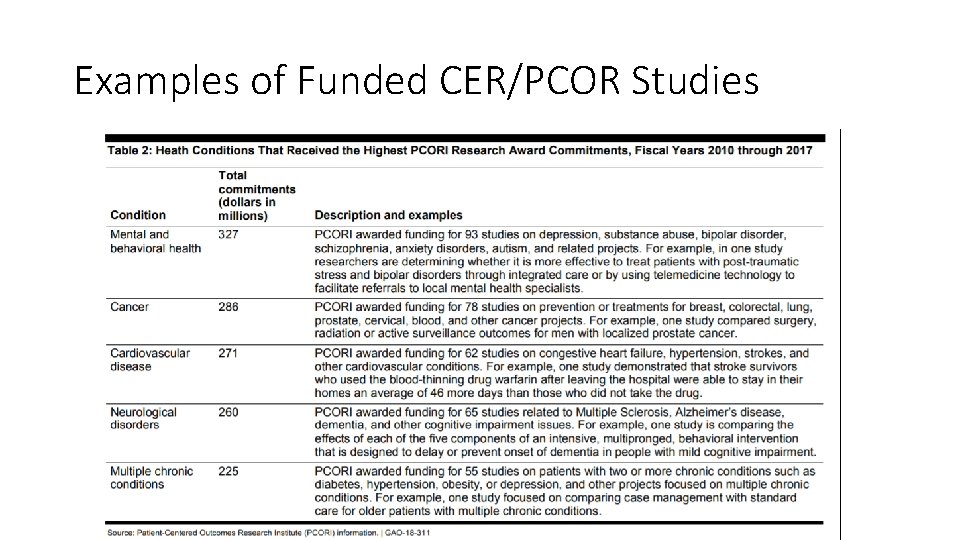
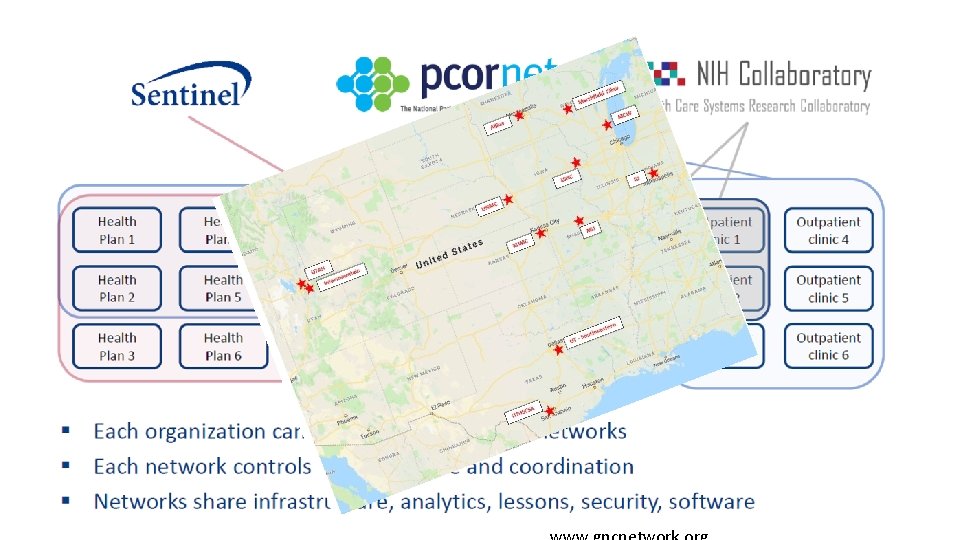
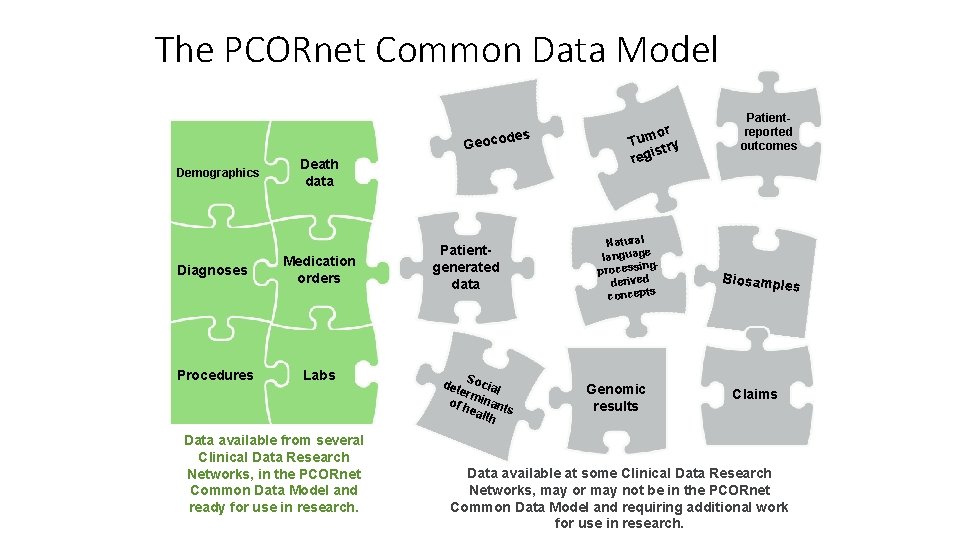
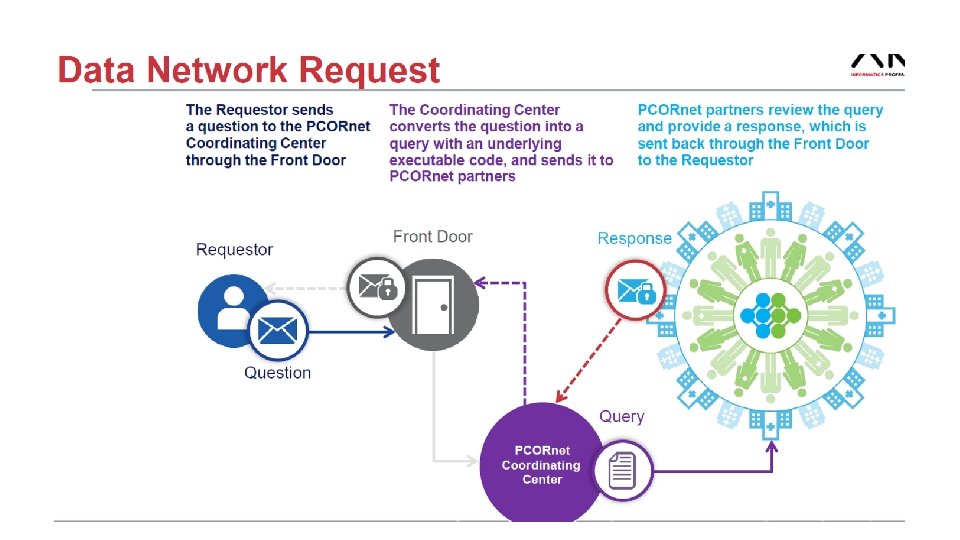
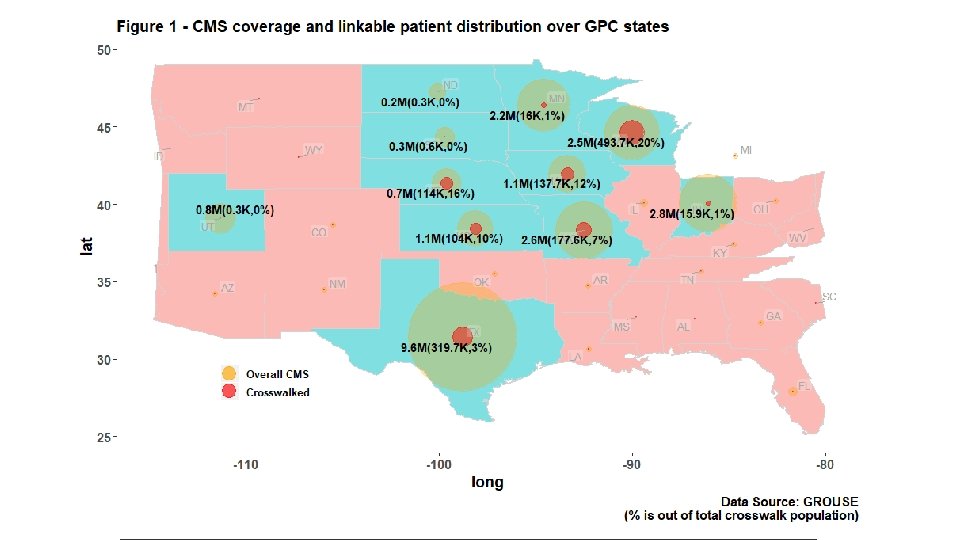
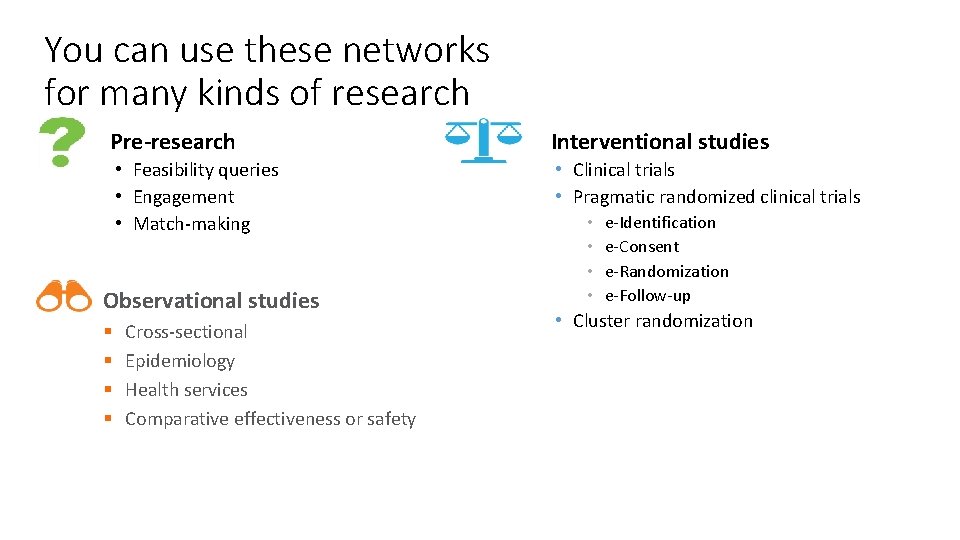
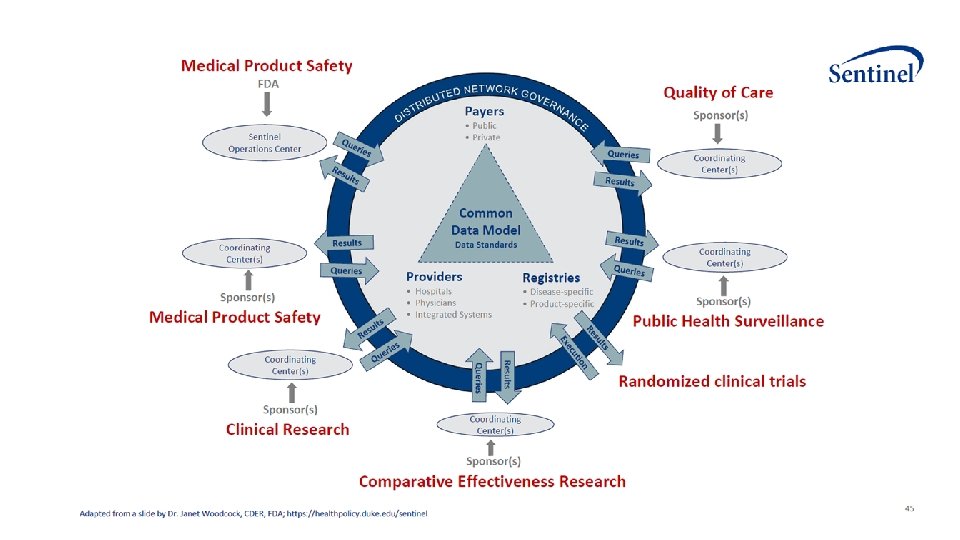
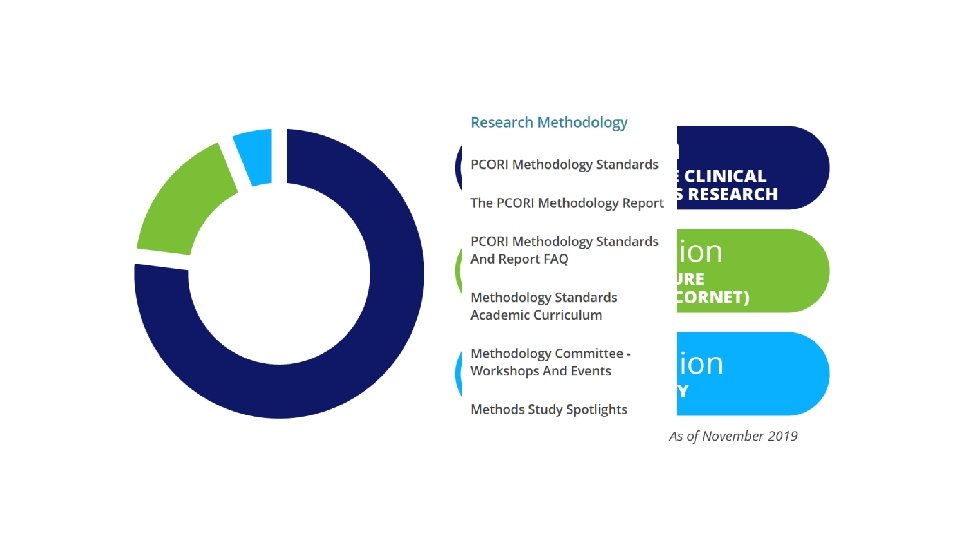
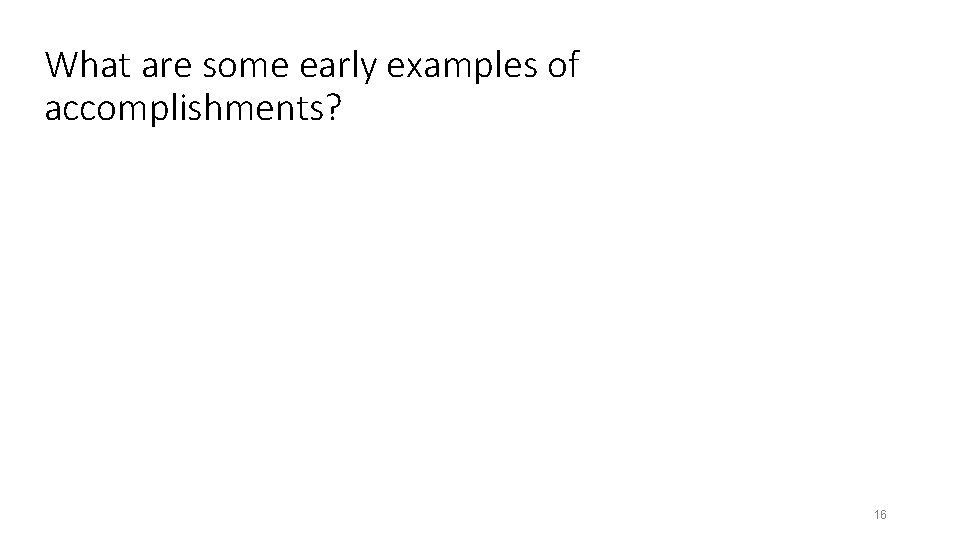
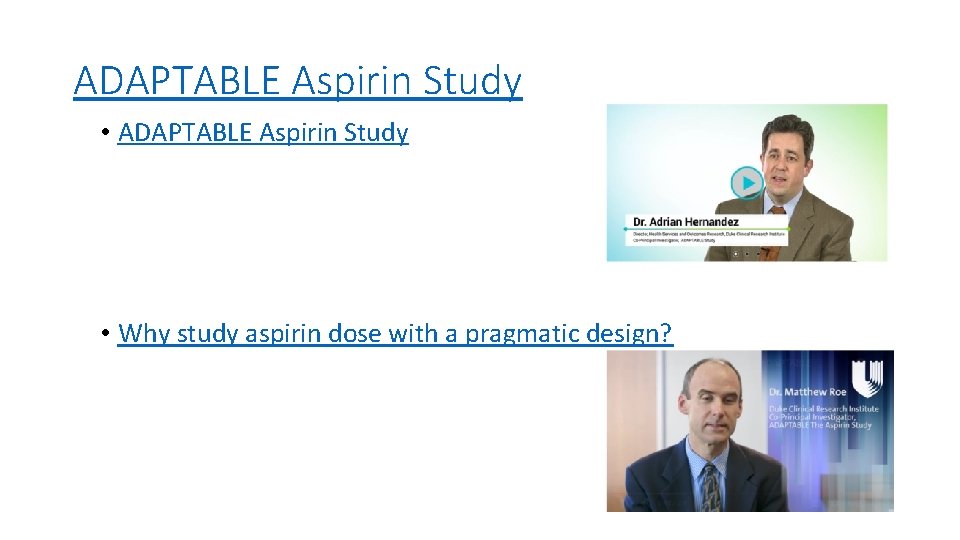
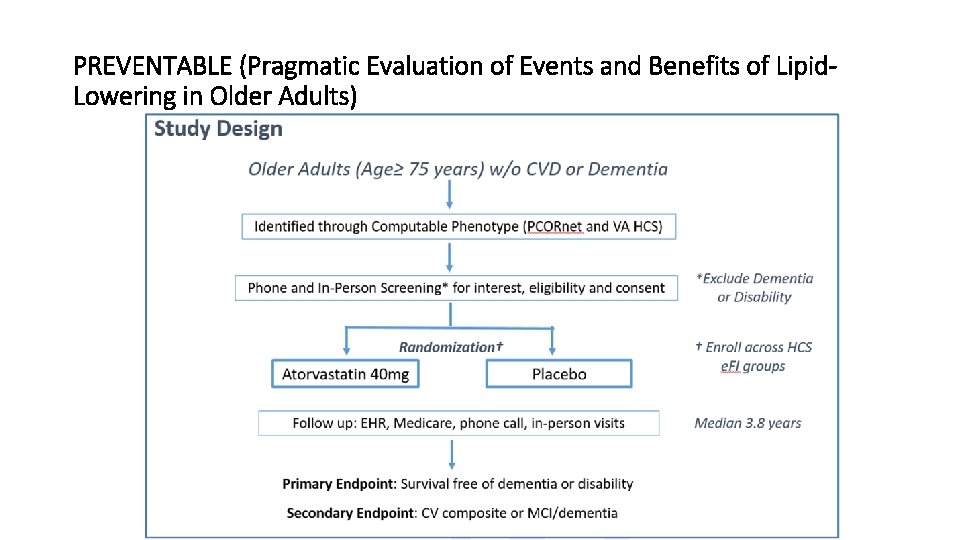
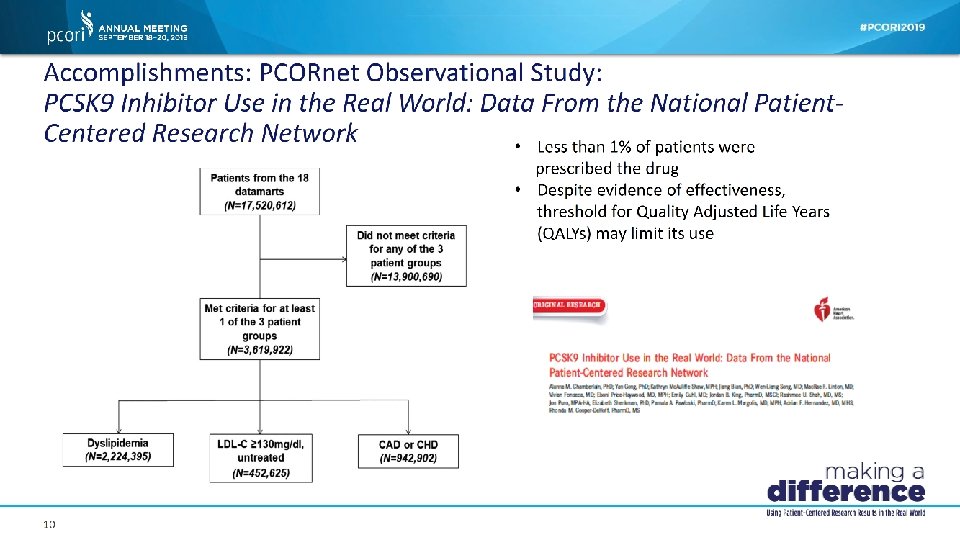
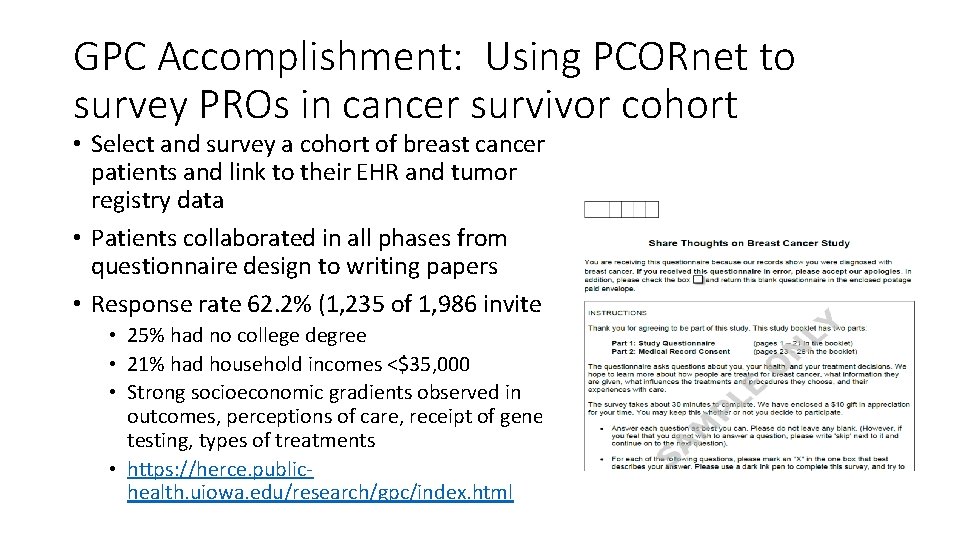
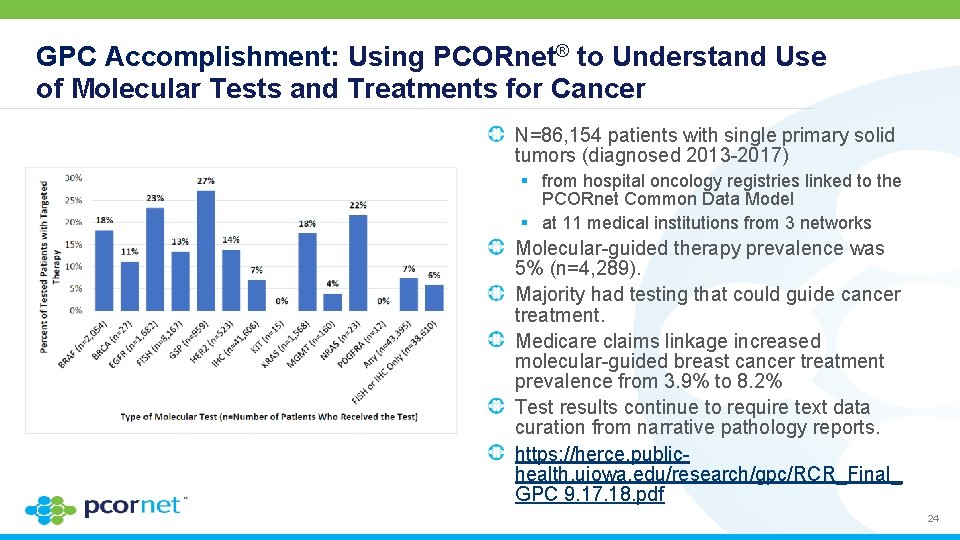
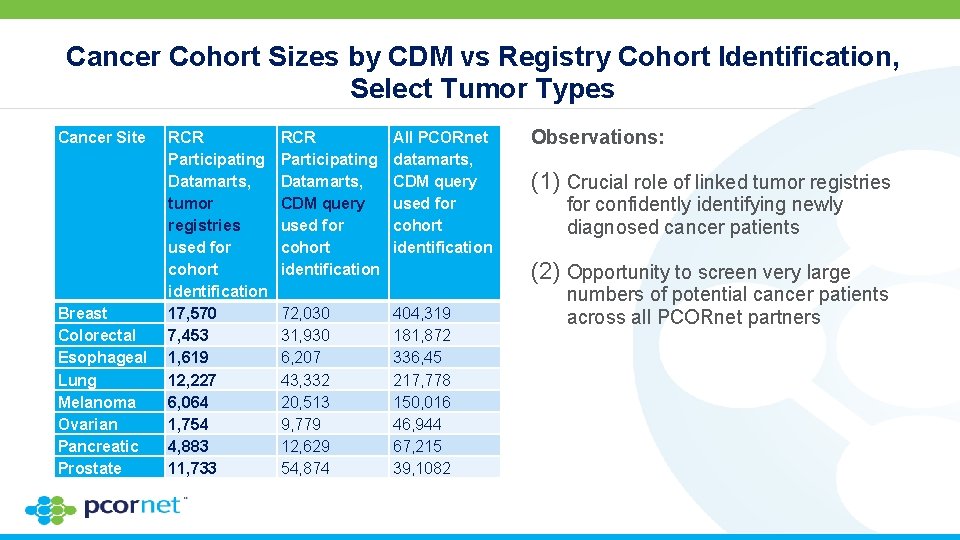
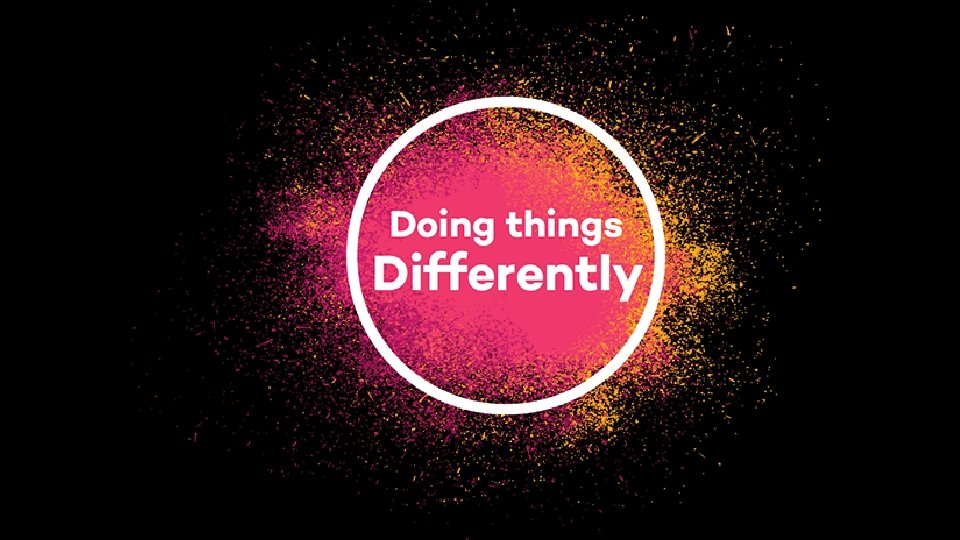
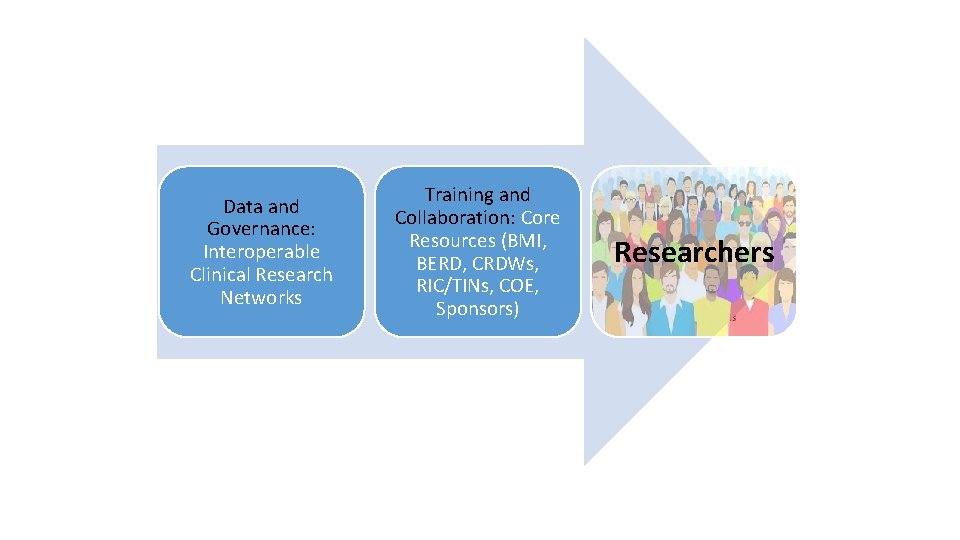
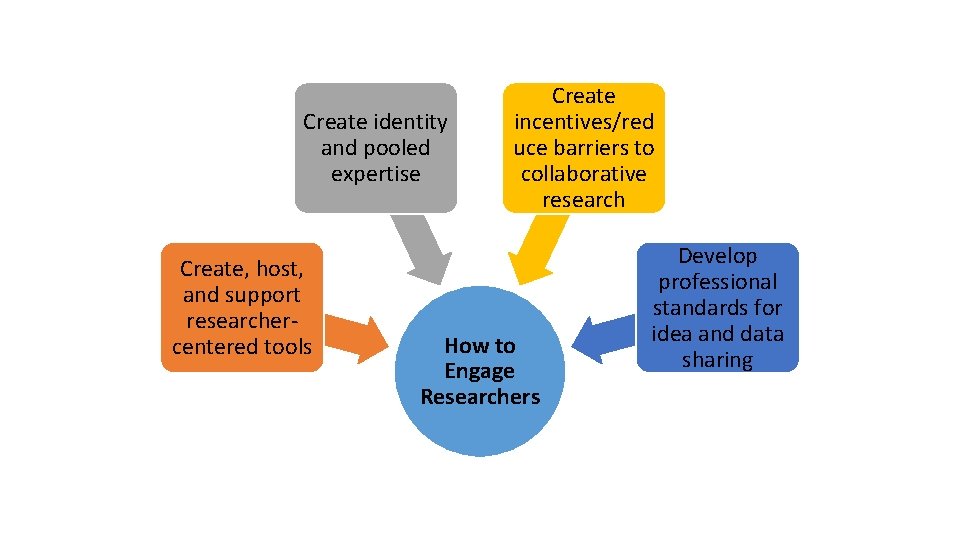
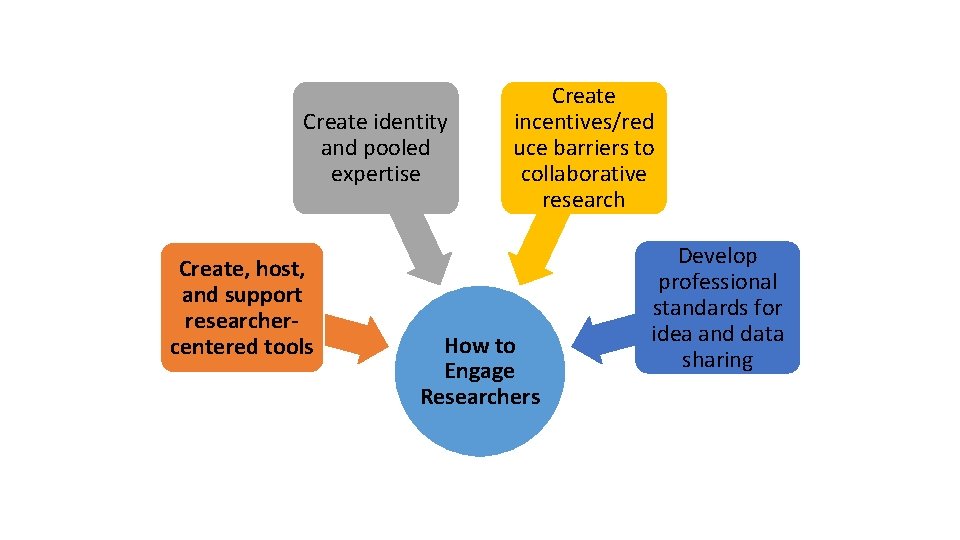
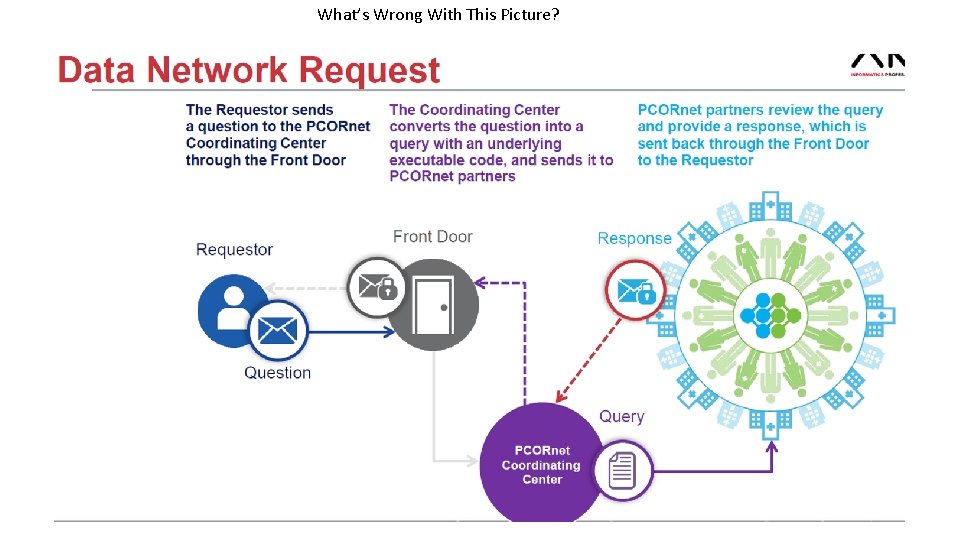
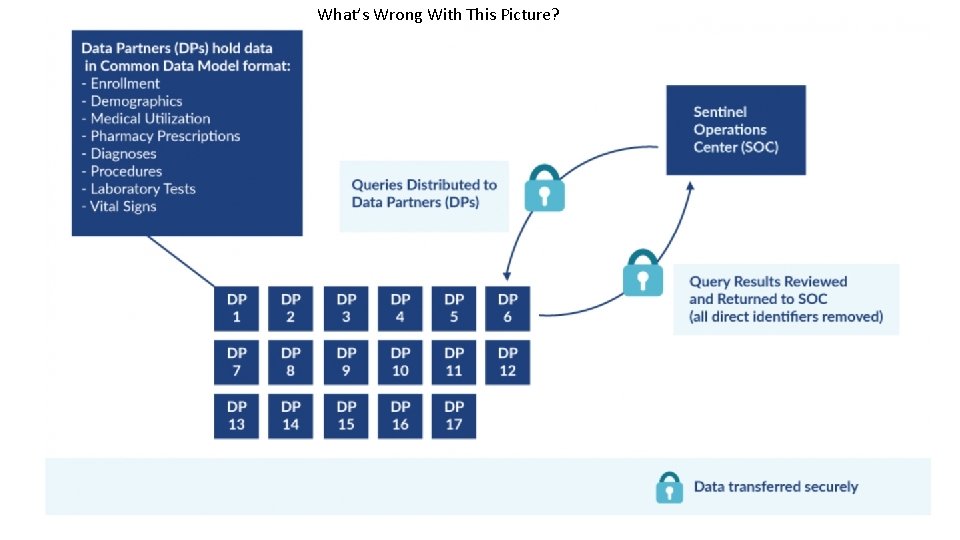
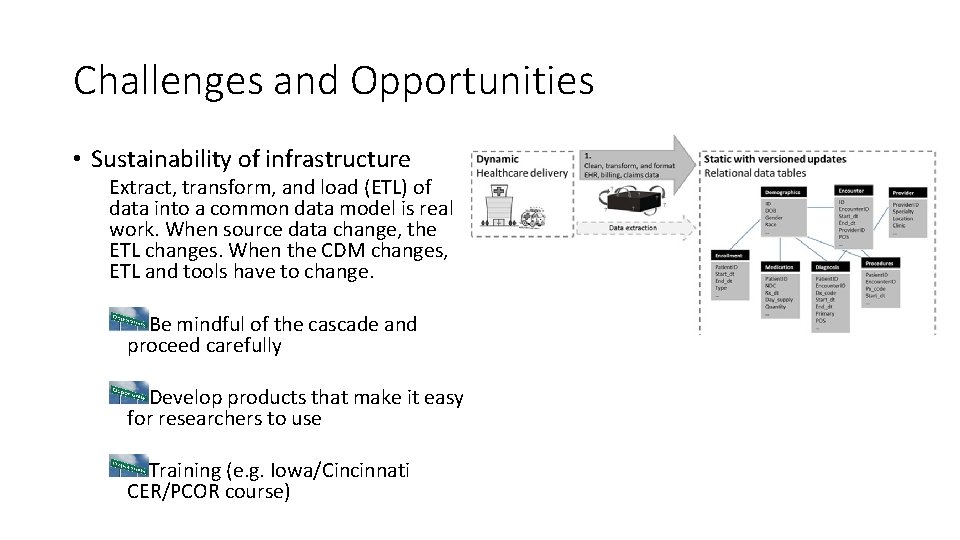
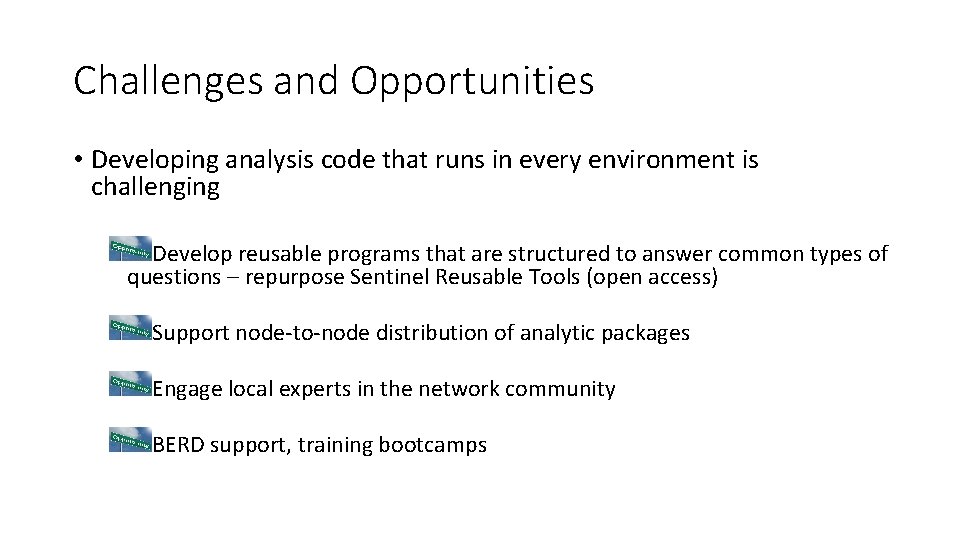
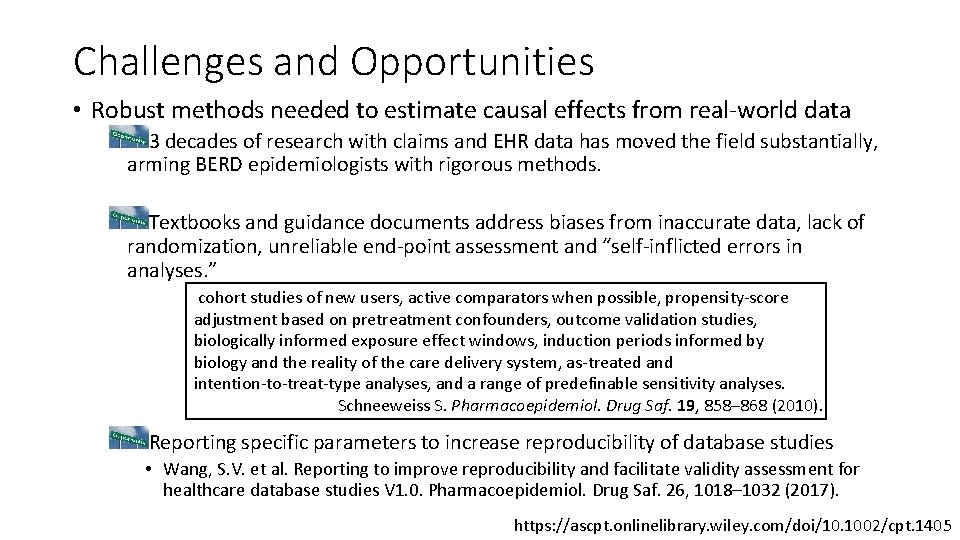
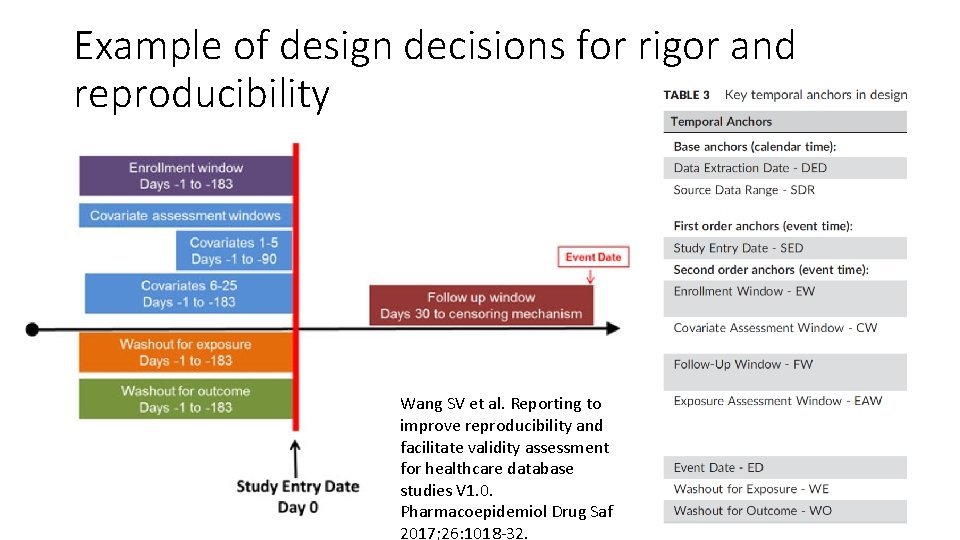
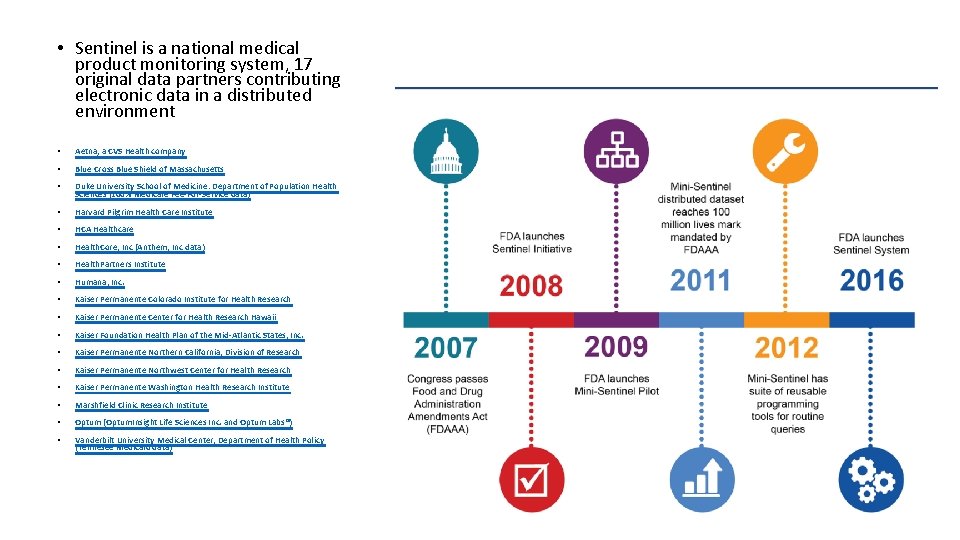
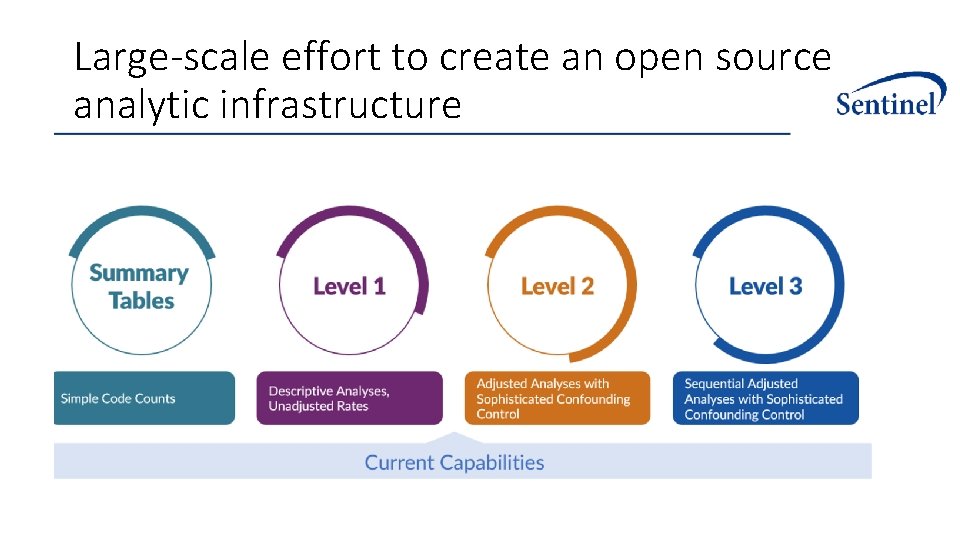
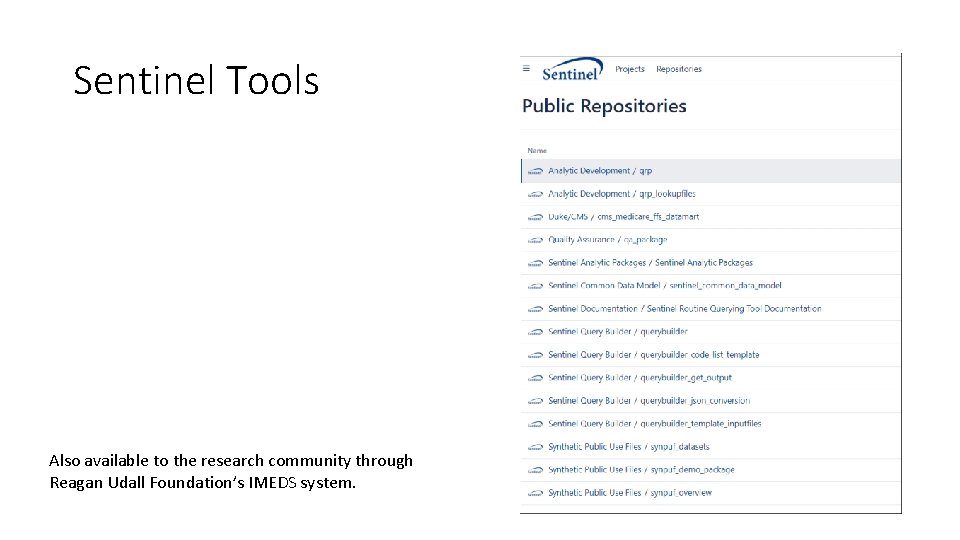
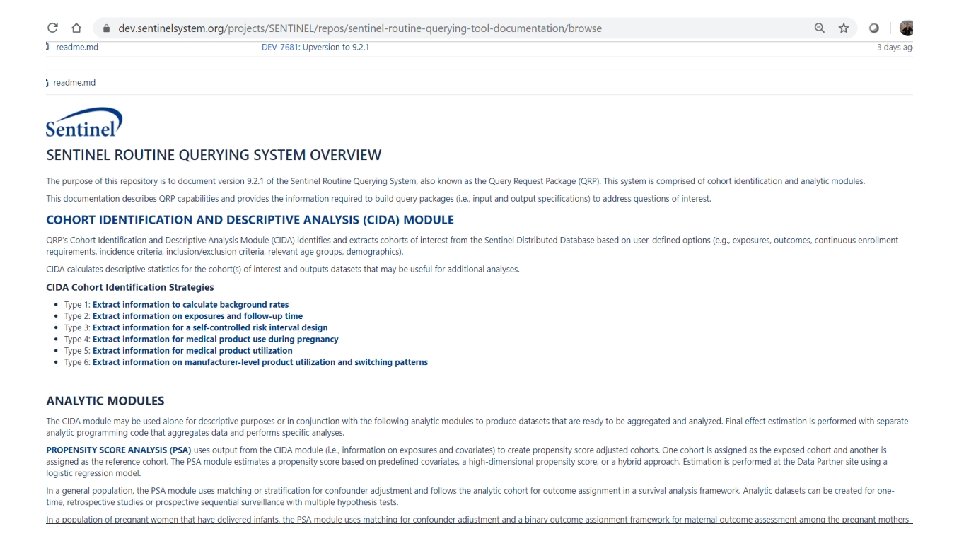
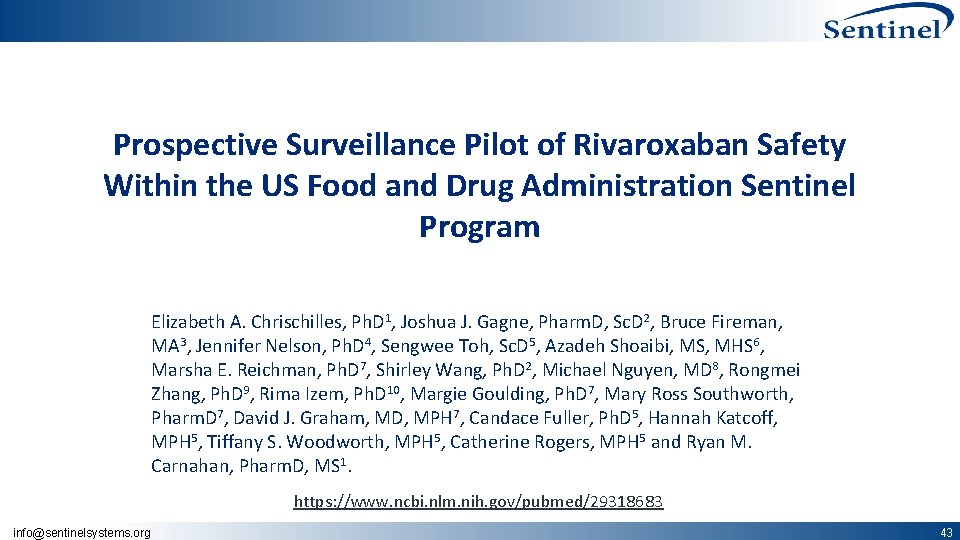
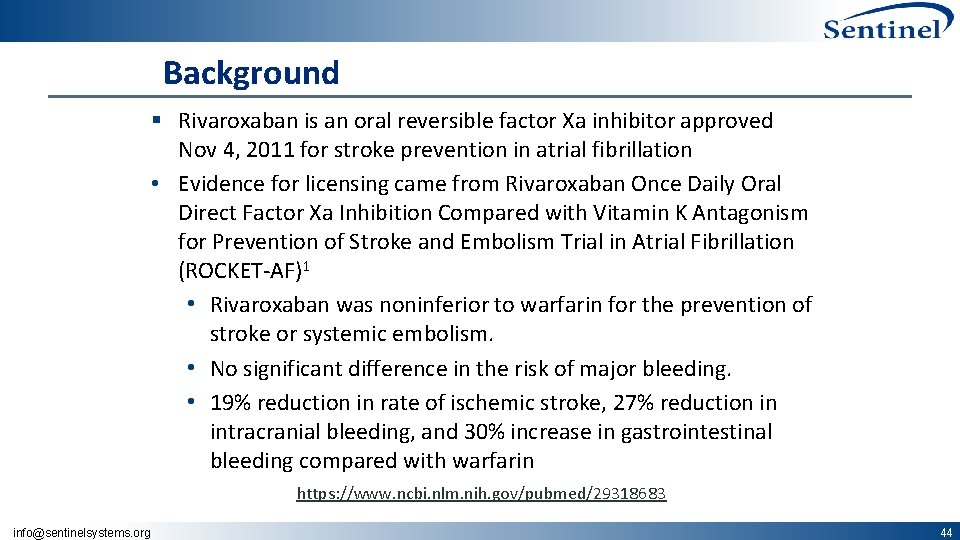
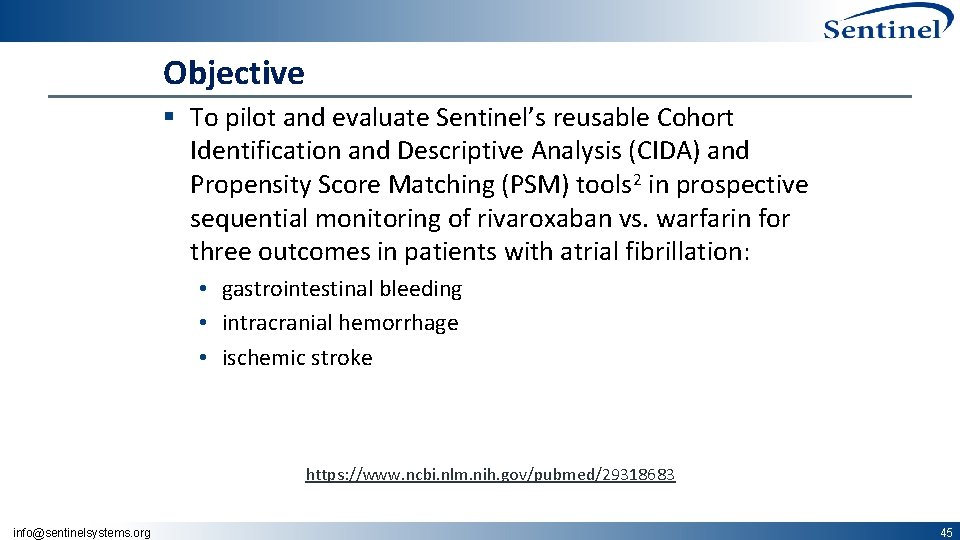
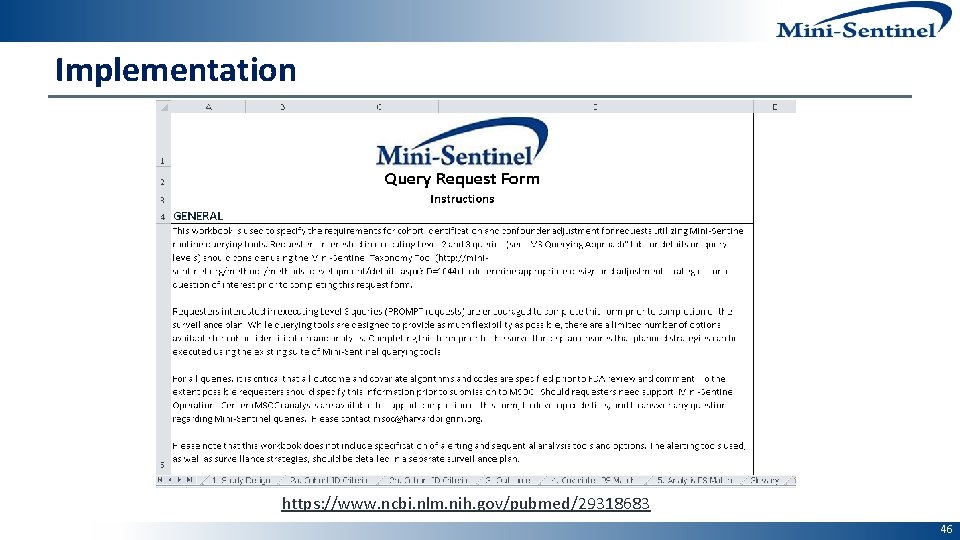
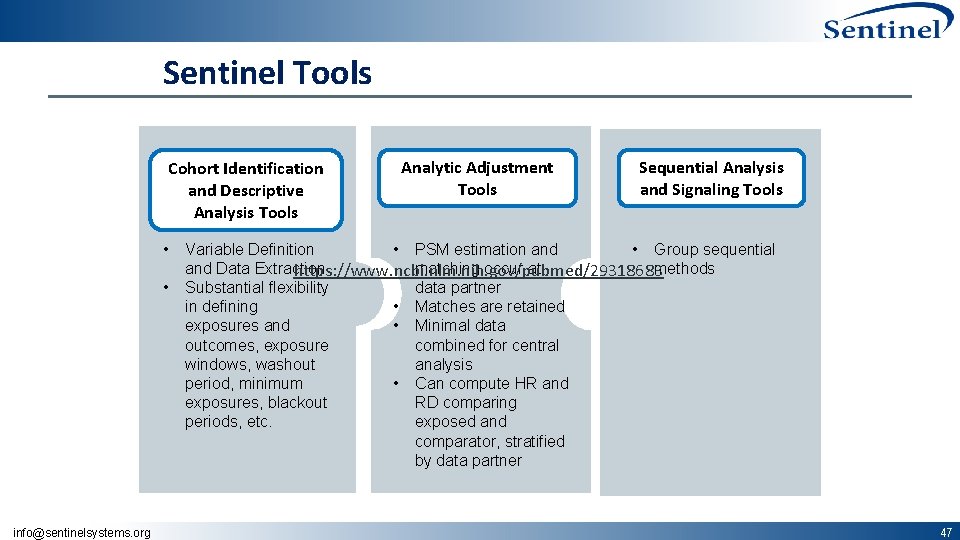
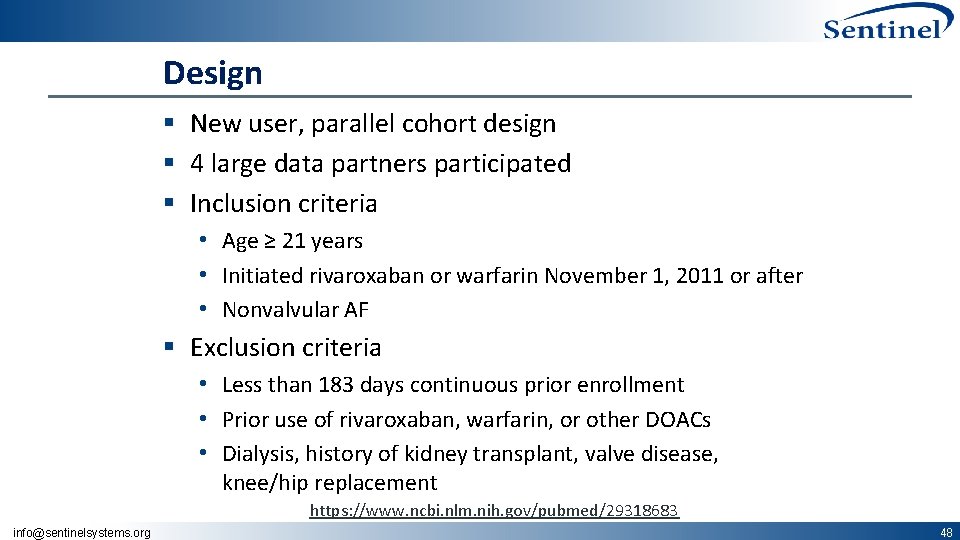
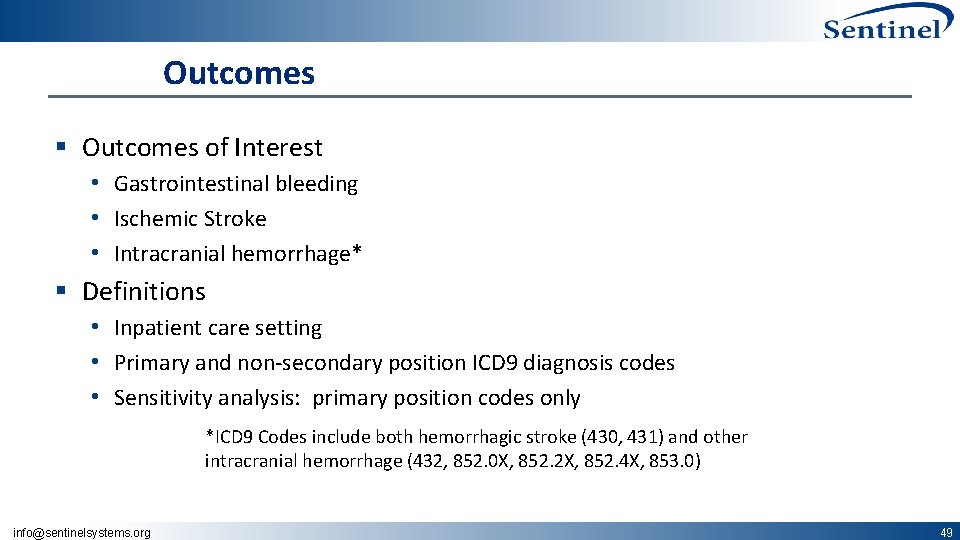
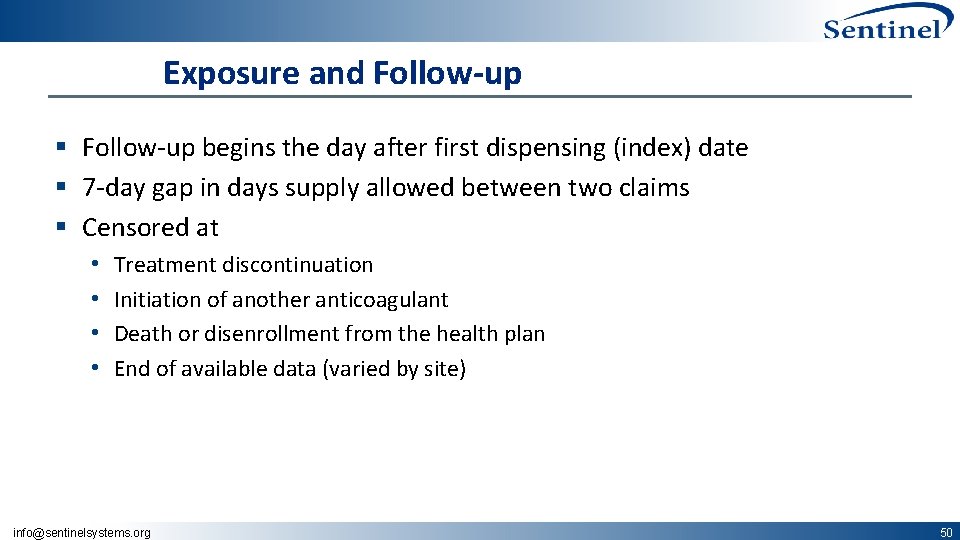
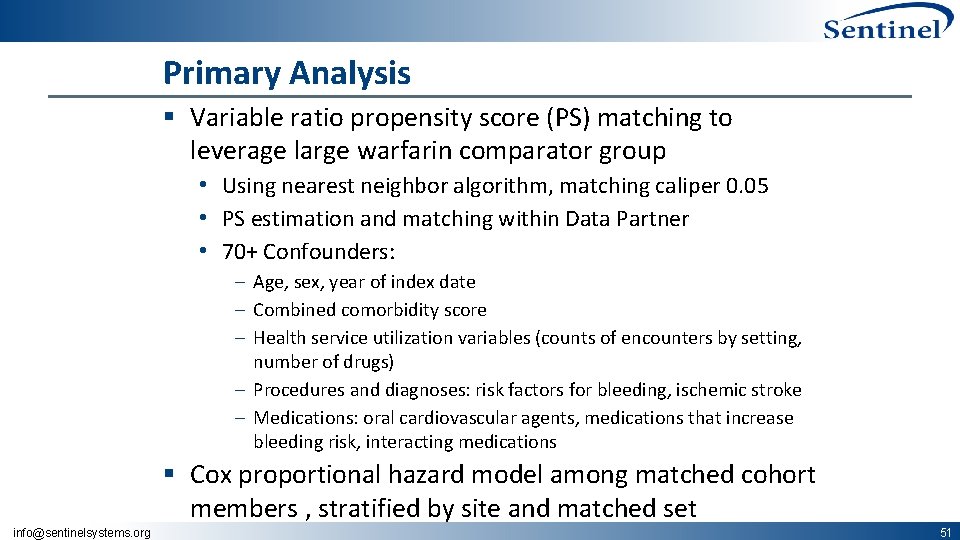
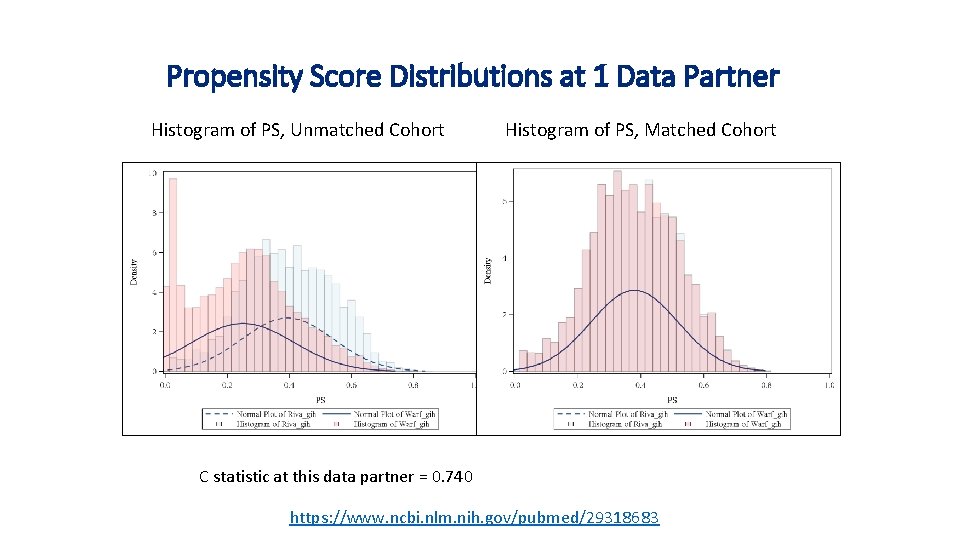
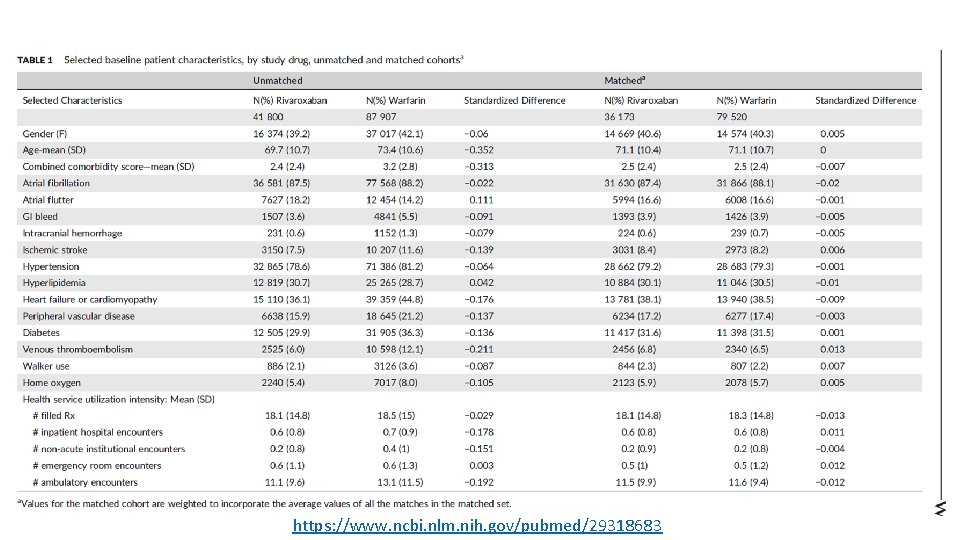
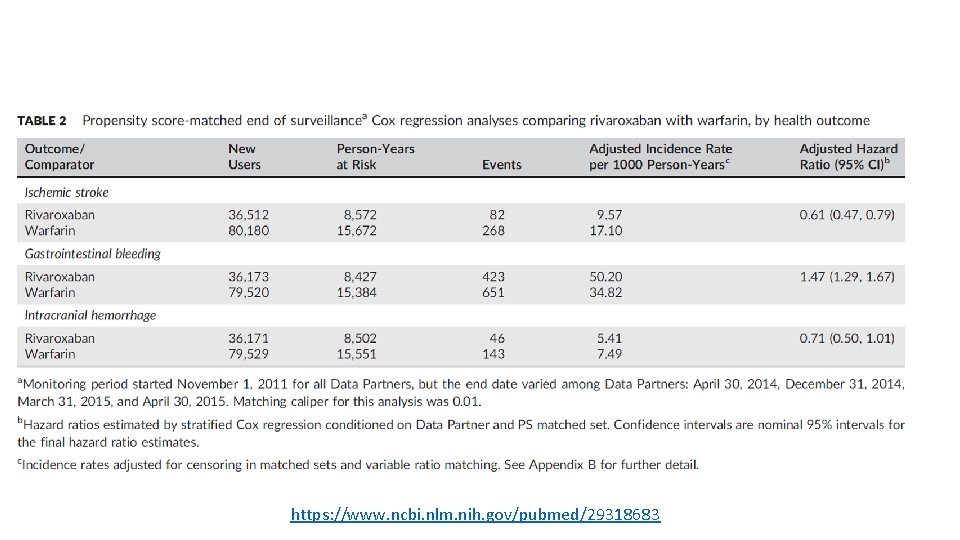
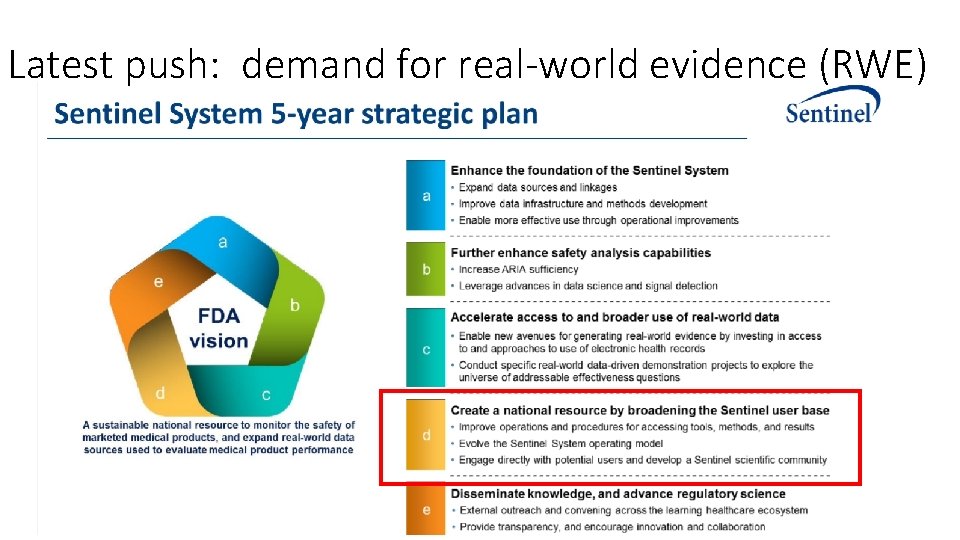
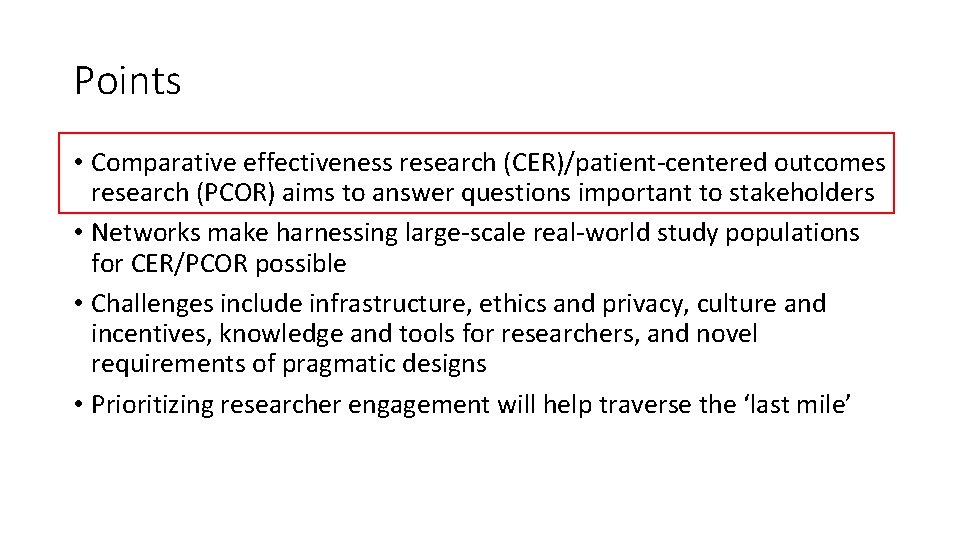
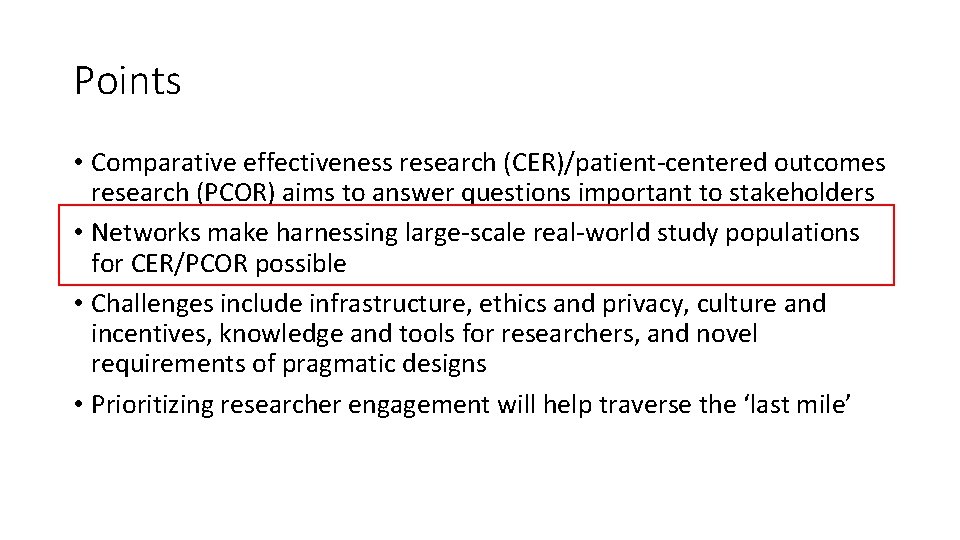
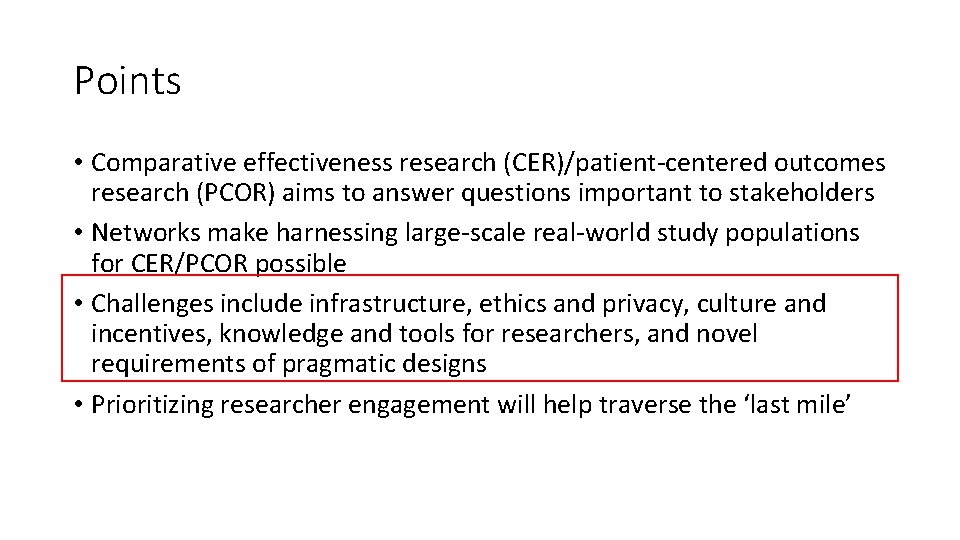
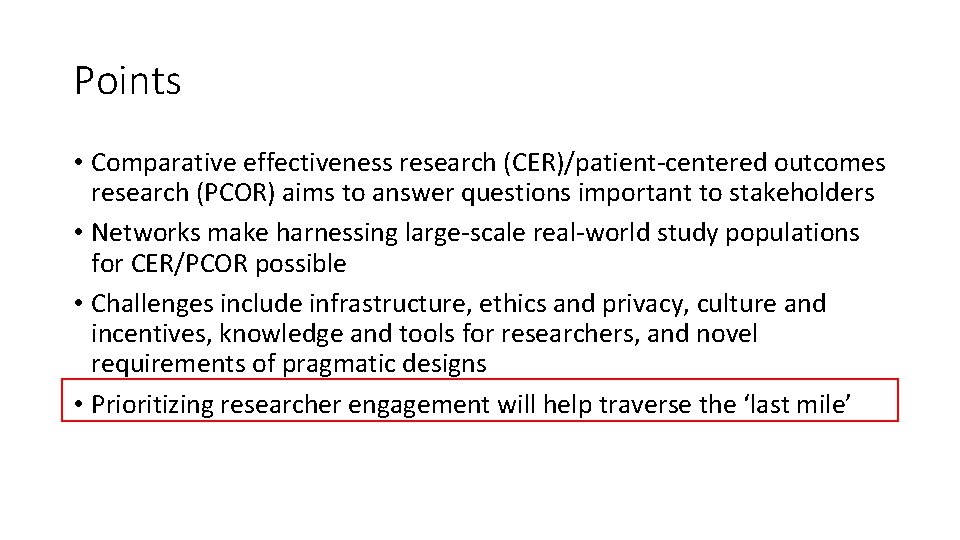
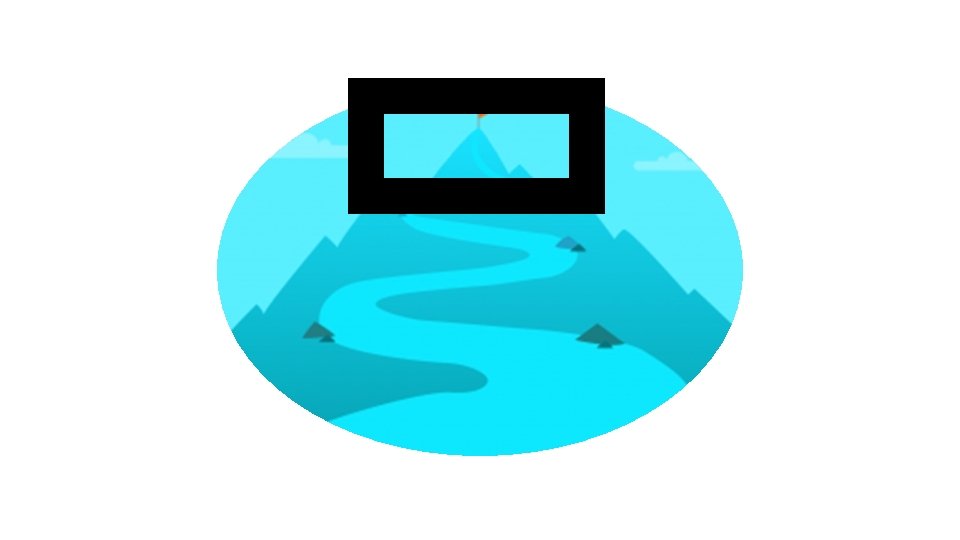
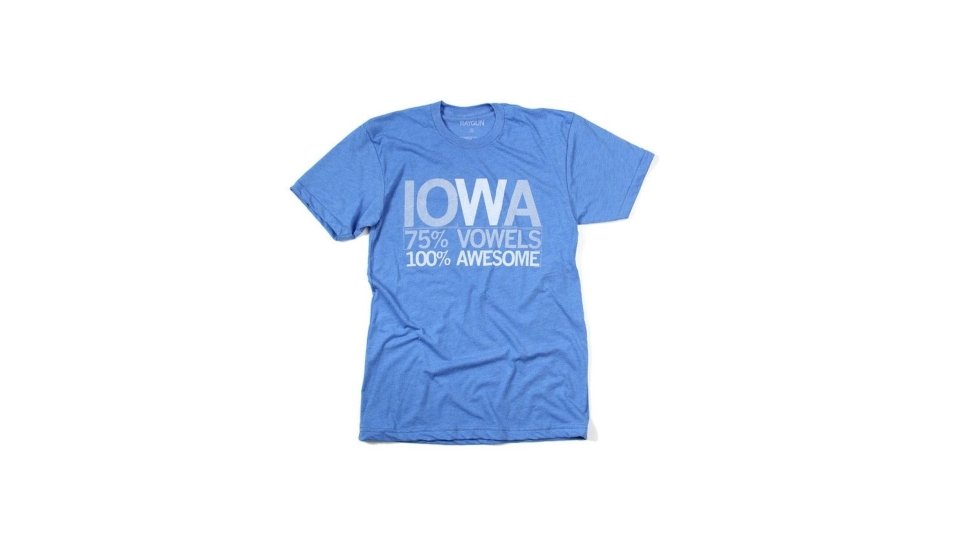
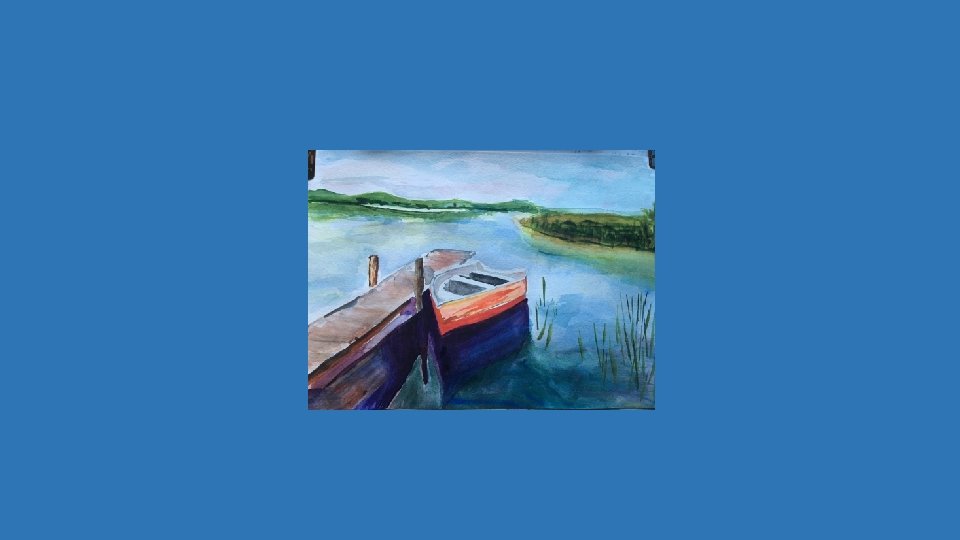
- Slides: 56
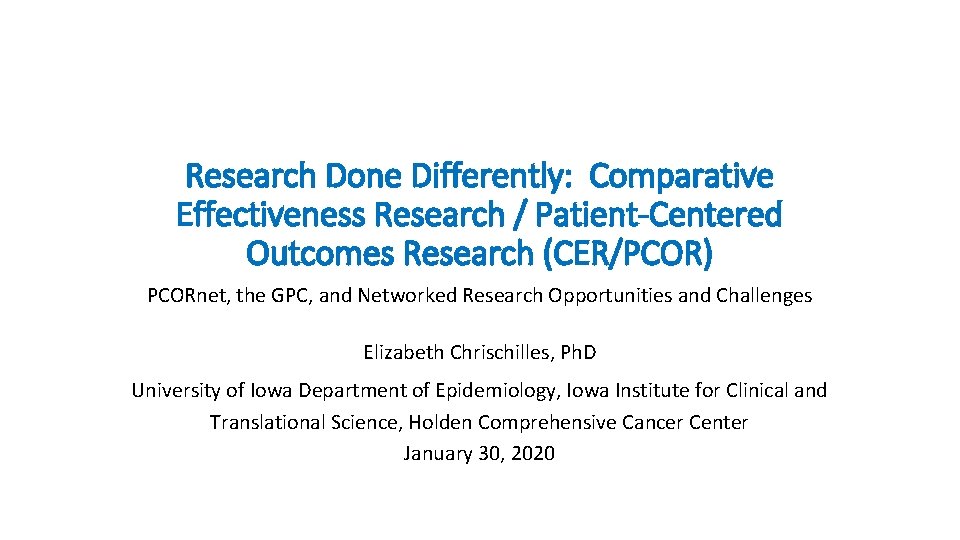
Research Done Differently: Comparative Effectiveness Research / Patient-Centered Outcomes Research (CER/PCOR) PCORnet, the GPC, and Networked Research Opportunities and Challenges Elizabeth Chrischilles, Ph. D University of Iowa Department of Epidemiology, Iowa Institute for Clinical and Translational Science, Holden Comprehensive Cancer Center January 30, 2020
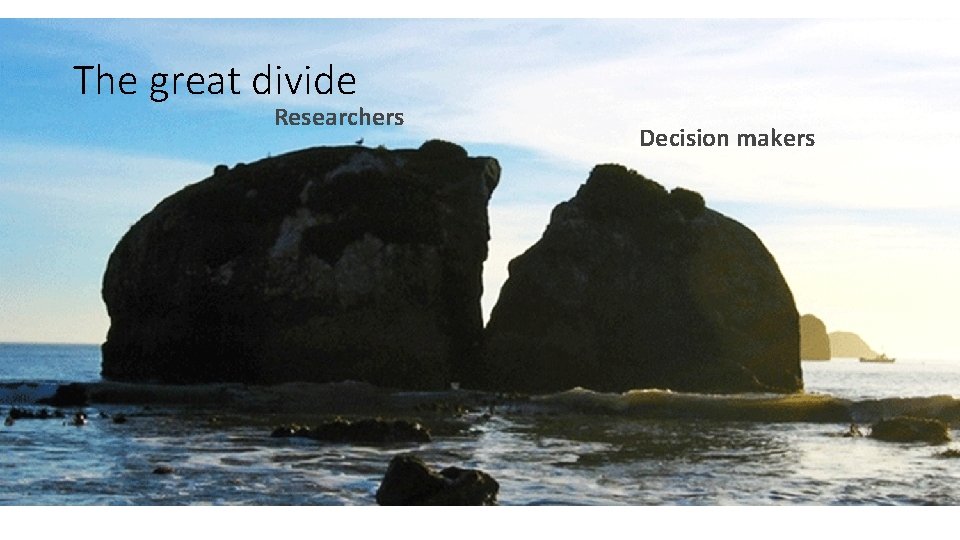
The great divide Researchers Decision makers
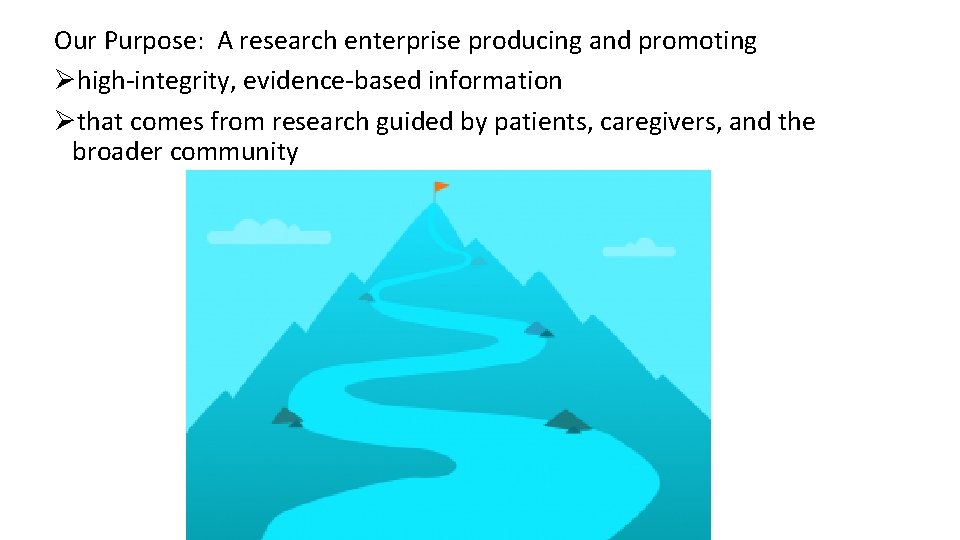
Our Purpose: A research enterprise producing and promoting Øhigh‐integrity, evidence‐based information Øthat comes from research guided by patients, caregivers, and the broader community
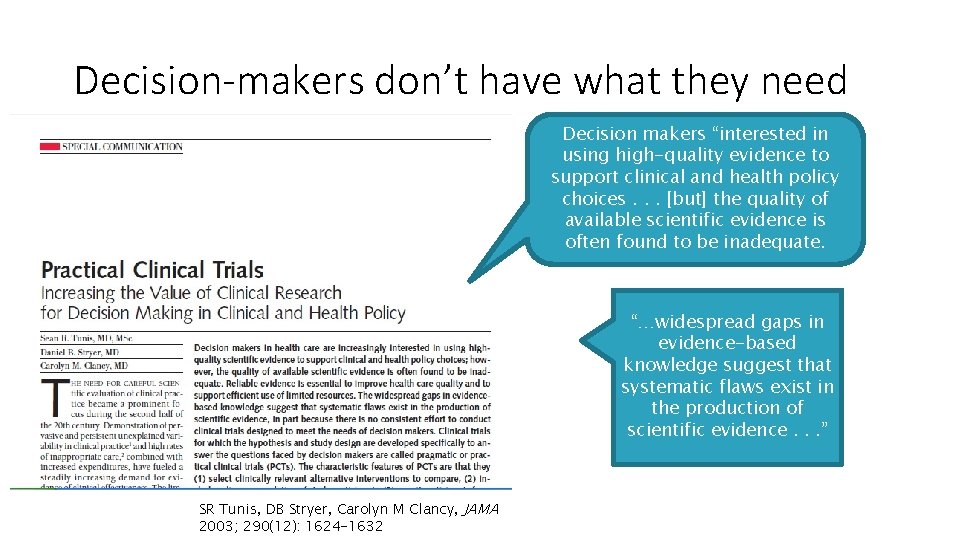
Decision-makers don’t have what they need Decision makers “interested in using high-quality evidence to support clinical and health policy choices. . . [but] the quality of available scientific evidence is often found to be inadequate. “…widespread gaps in evidence-based knowledge suggest that systematic flaws exist in the production of scientific evidence. . . ” SR Tunis, DB Stryer, Carolyn M Clancy, JAMA 2003; 290(12): 1624 -1632
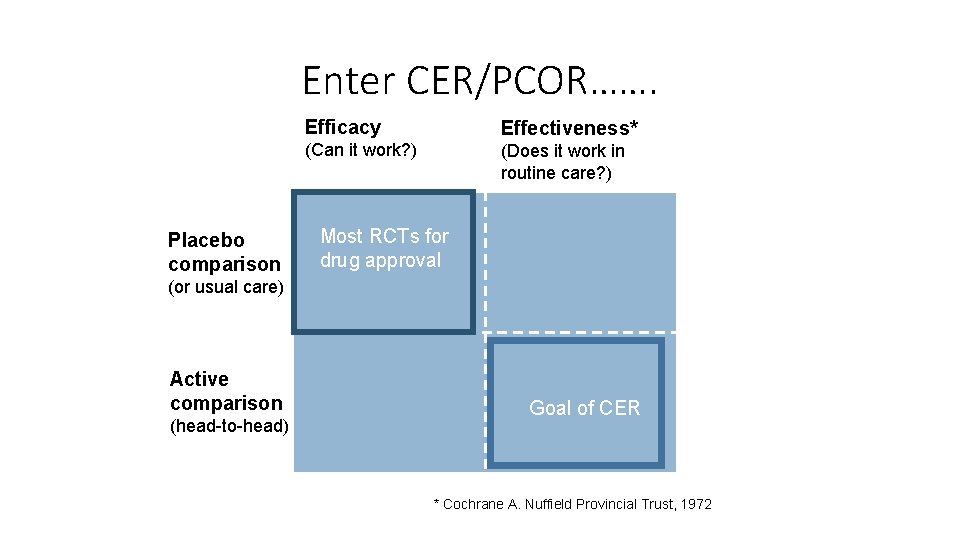
Enter CER/PCOR……. Placebo comparison Efficacy Effectiveness* (Can it work? ) (Does it work in routine care? ) Most RCTs for drug approval (or usual care) Active comparison (head-to-head) Goal of CER * Cochrane A. Nuffield Provincial Trust, 1972
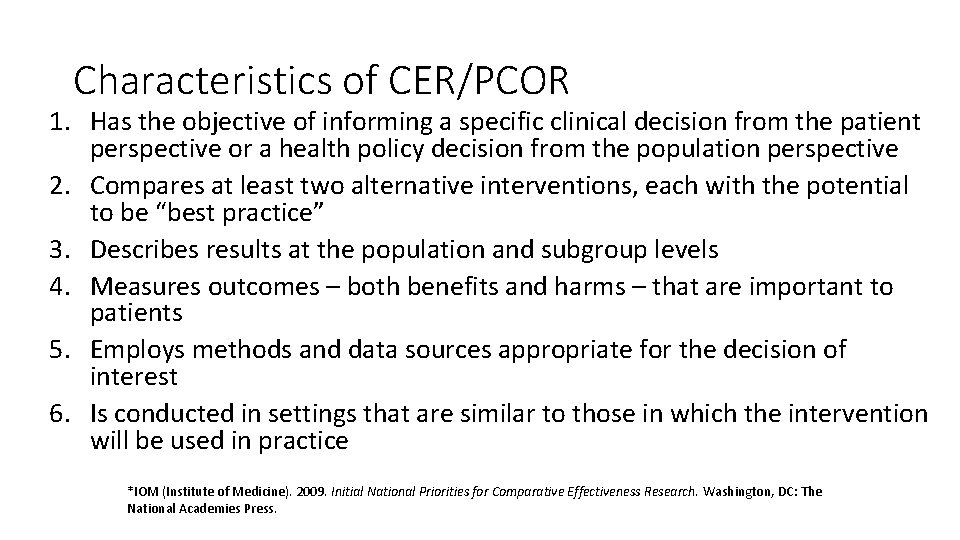
Characteristics of CER/PCOR 1. Has the objective of informing a specific clinical decision from the patient perspective or a health policy decision from the population perspective 2. Compares at least two alternative interventions, each with the potential to be “best practice” 3. Describes results at the population and subgroup levels 4. Measures outcomes – both benefits and harms – that are important to patients 5. Employs methods and data sources appropriate for the decision of interest 6. Is conducted in settings that are similar to those in which the intervention will be used in practice *IOM (Institute of Medicine). 2009. Initial National Priorities for Comparative Effectiveness Research. Washington, DC: The National Academies Press.
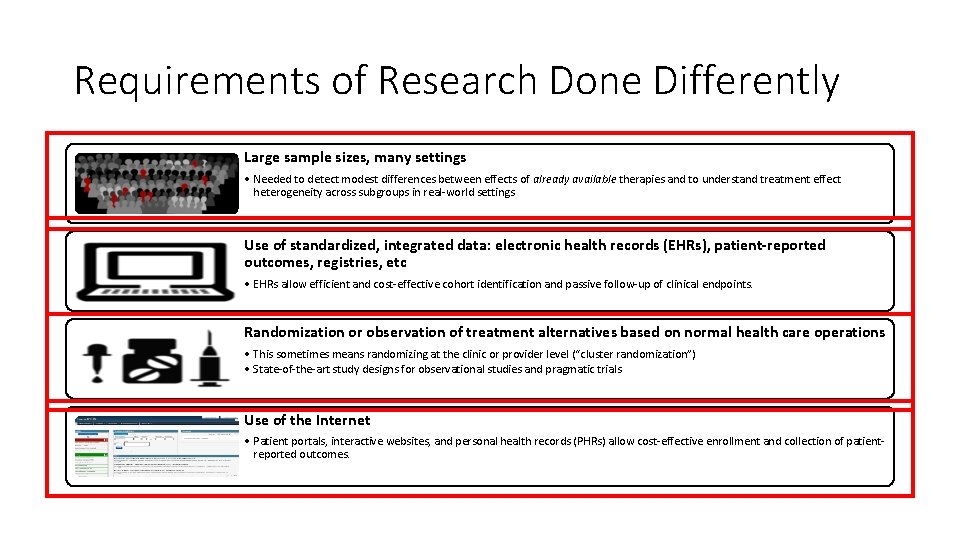
Requirements of Research Done Differently Large sample sizes, many settings • Needed to detect modest differences between effects of already available therapies and to understand treatment effect heterogeneity across subgroups in real‐world settings Use of standardized, integrated data: electronic health records (EHRs), patient-reported outcomes, registries, etc • EHRs allow efficient and cost‐effective cohort identification and passive follow‐up of clinical endpoints. Randomization or observation of treatment alternatives based on normal health care operations • This sometimes means randomizing at the clinic or provider level (“cluster randomization”) • State‐of‐the‐art study designs for observational studies and pragmatic trials Use of the Internet • Patient portals, interactive websites, and personal health records (PHRs) allow cost‐effective enrollment and collection of patient‐ reported outcomes.
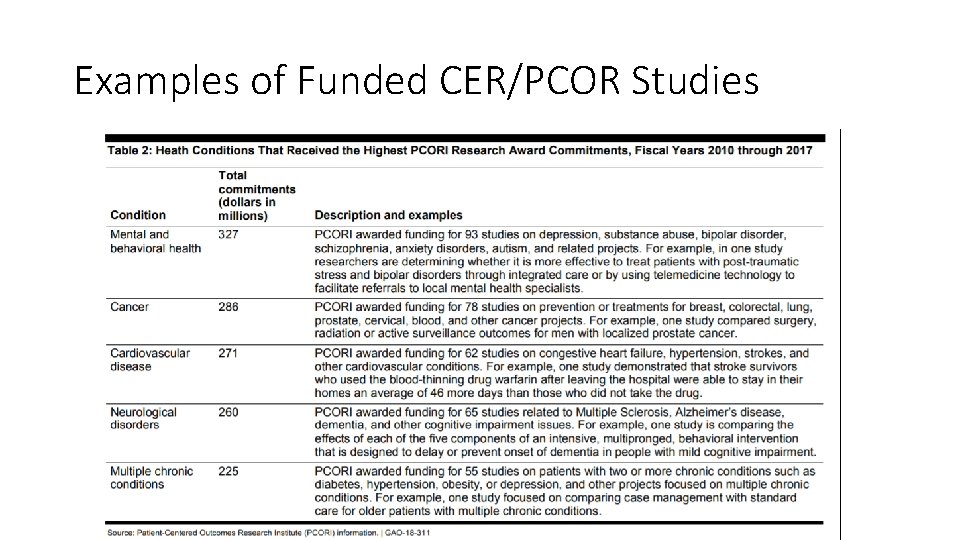
Examples of Funded CER/PCOR Studies
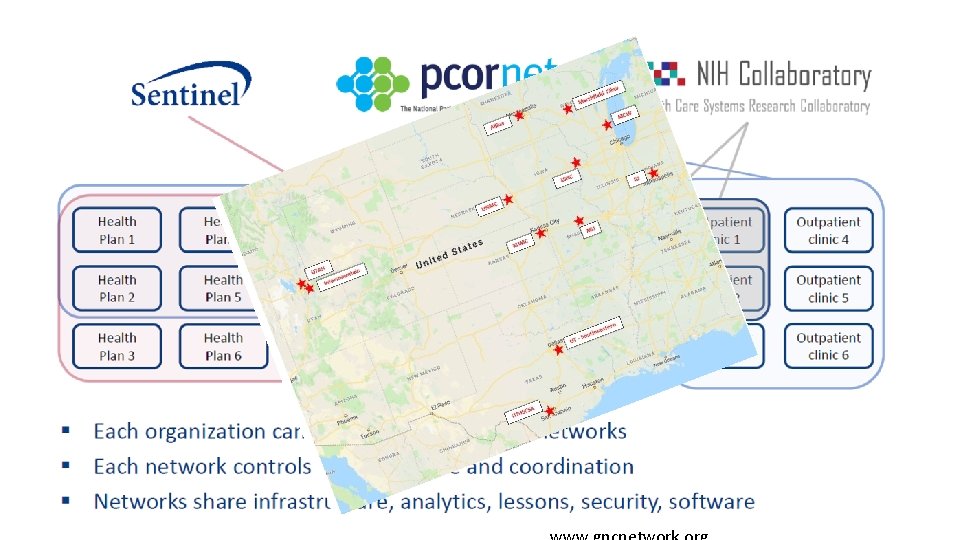
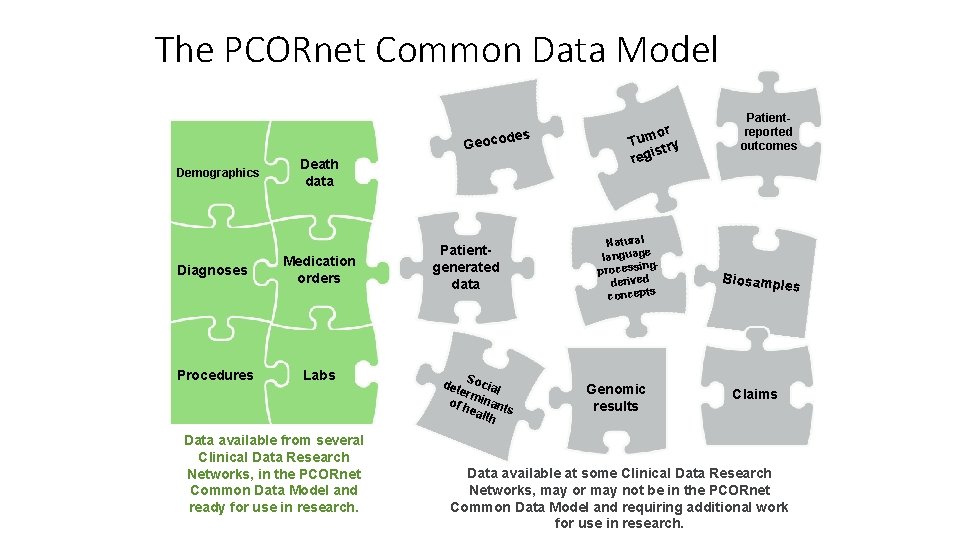
The PCORnet Common Data Model des Geoco Demographics Death data Diagnoses Medication orders Procedures Labs Data available from several Clinical Data Research Networks, in the PCORnet Common Data Model and ready for use in research. Patientgenerated data det Social erm of h inant s eal th or Tum try is reg Natural language gprocessin derived concepts Genomic results Patientreported outcomes Biosamp les Claims Data available at some Clinical Data Research Networks, may or may not be in the PCORnet Common Data Model and requiring additional work for use in research.
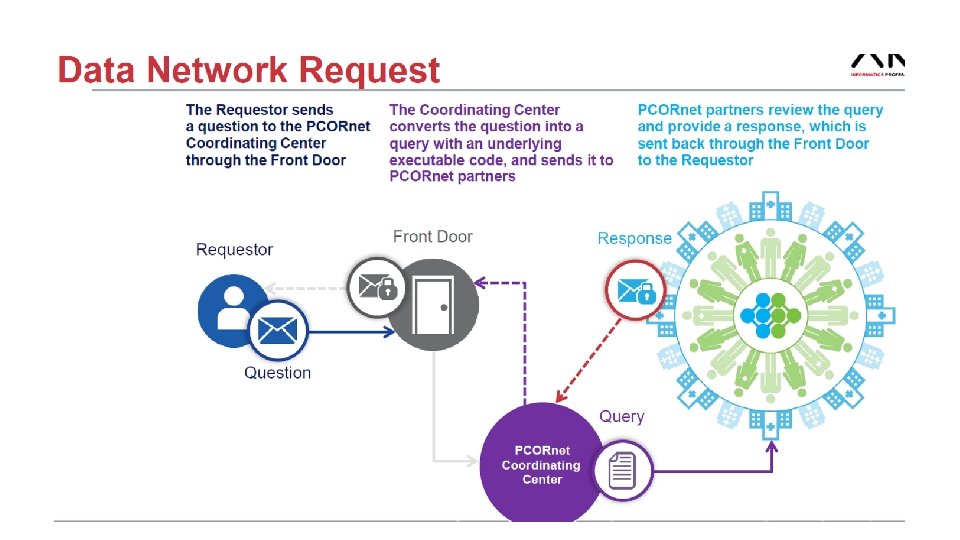
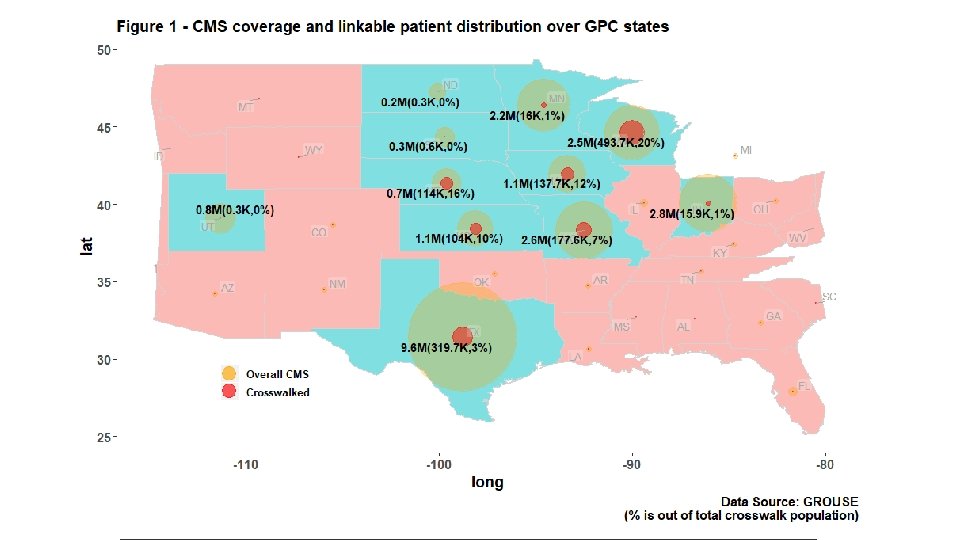
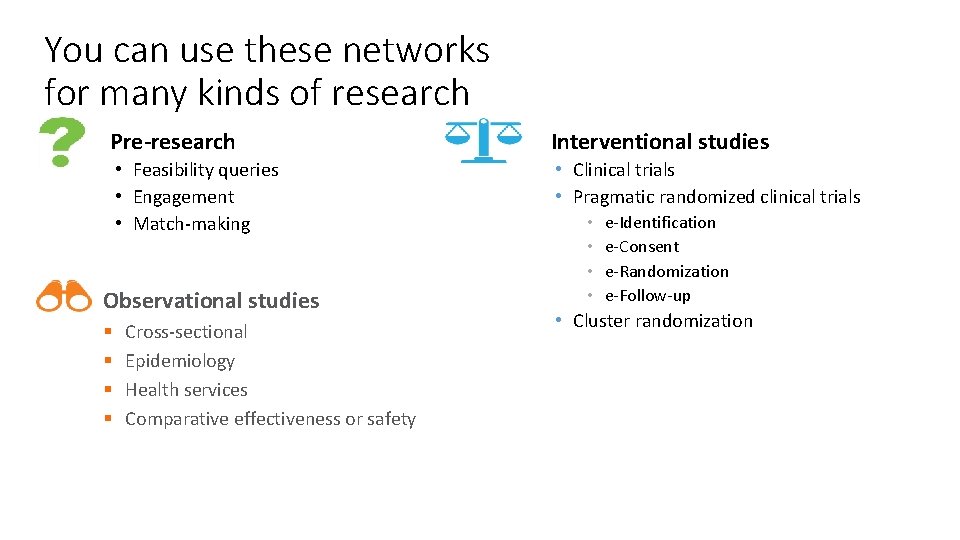
You can use these networks for many kinds of research Pre-research Interventional studies • Feasibility queries • Engagement • Match‐making • Clinical trials • Pragmatic randomized clinical trials Observational studies § § Cross‐sectional Epidemiology Health services Comparative effectiveness or safety • • e‐Identification e‐Consent e‐Randomization e‐Follow‐up • Cluster randomization
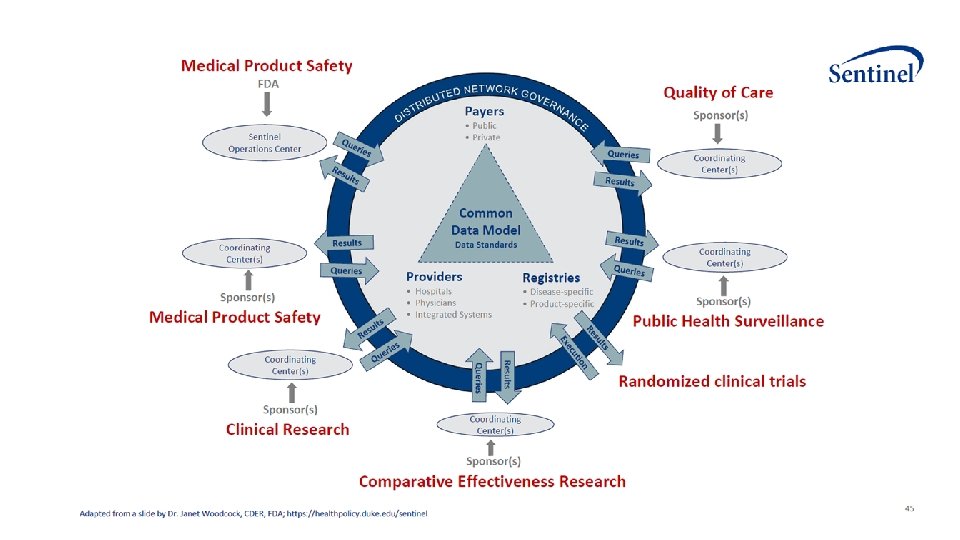
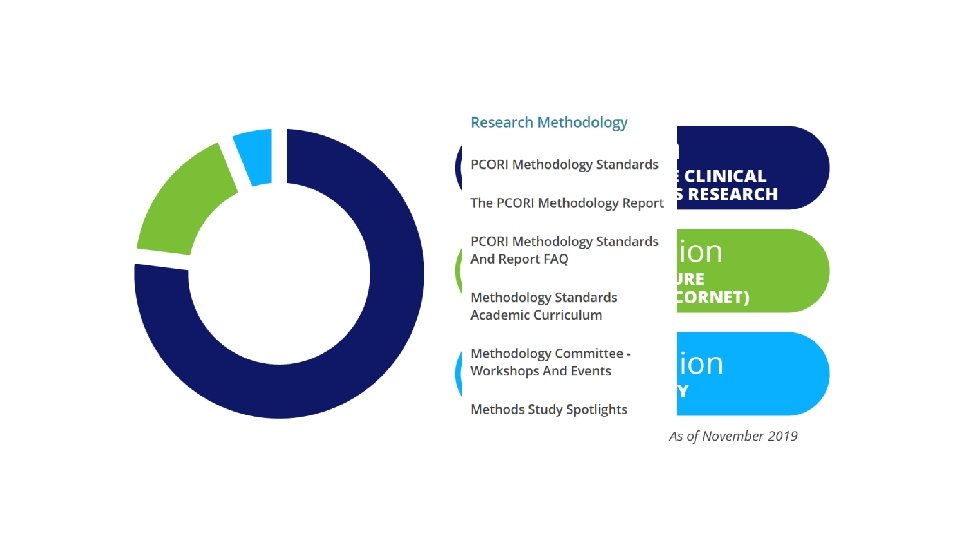
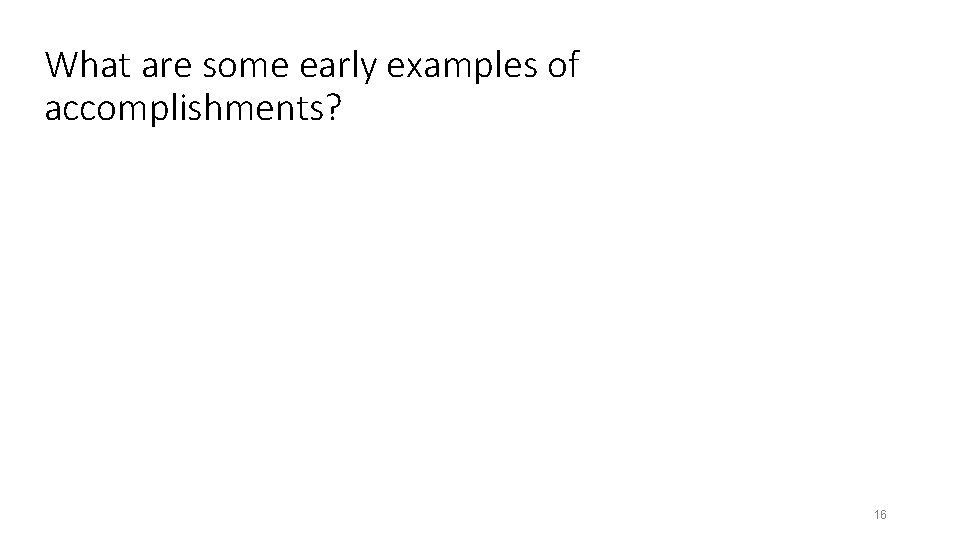
What are some early examples of accomplishments? 16
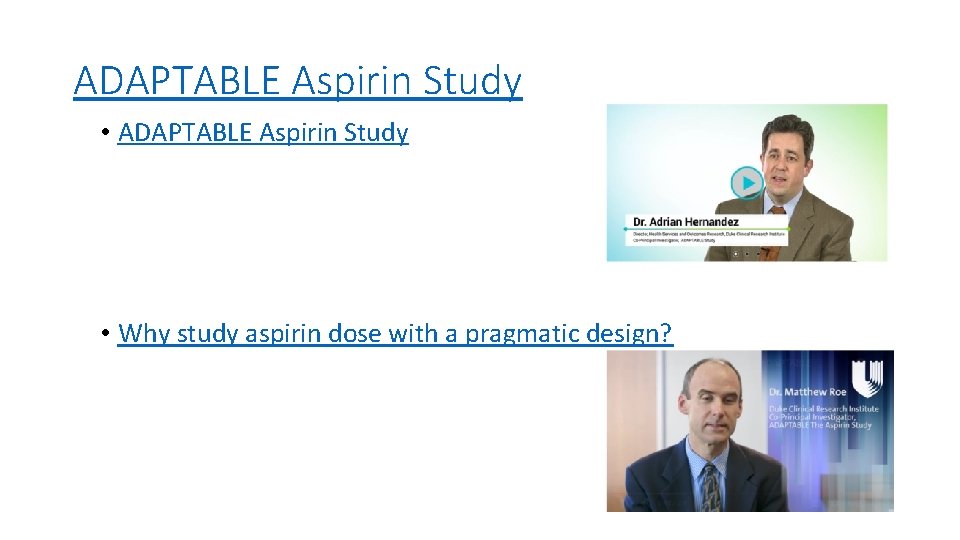
ADAPTABLE Aspirin Study • ADAPTABLE Aspirin Study • Why study aspirin dose with a pragmatic design?
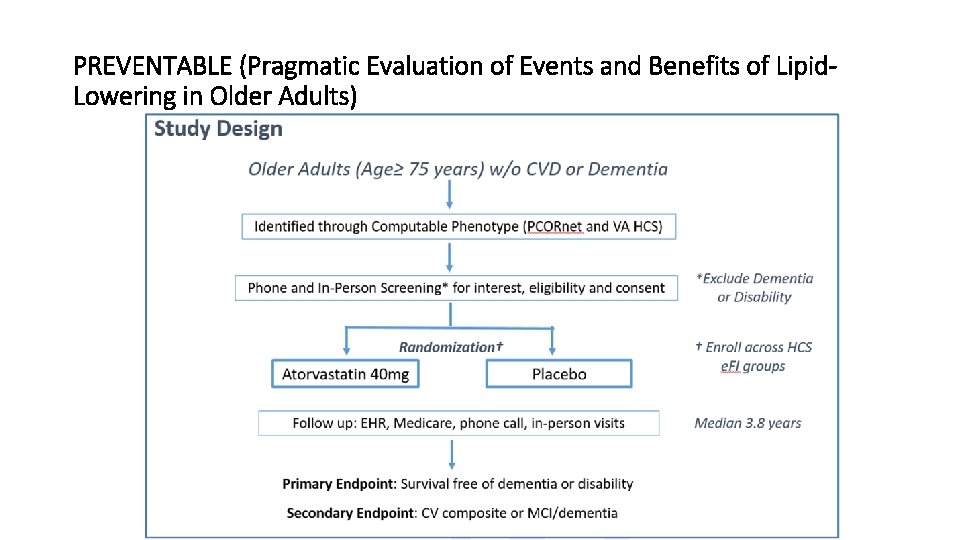
PREVENTABLE (Pragmatic Evaluation of Events and Benefits of Lipid. Lowering in Older Adults)
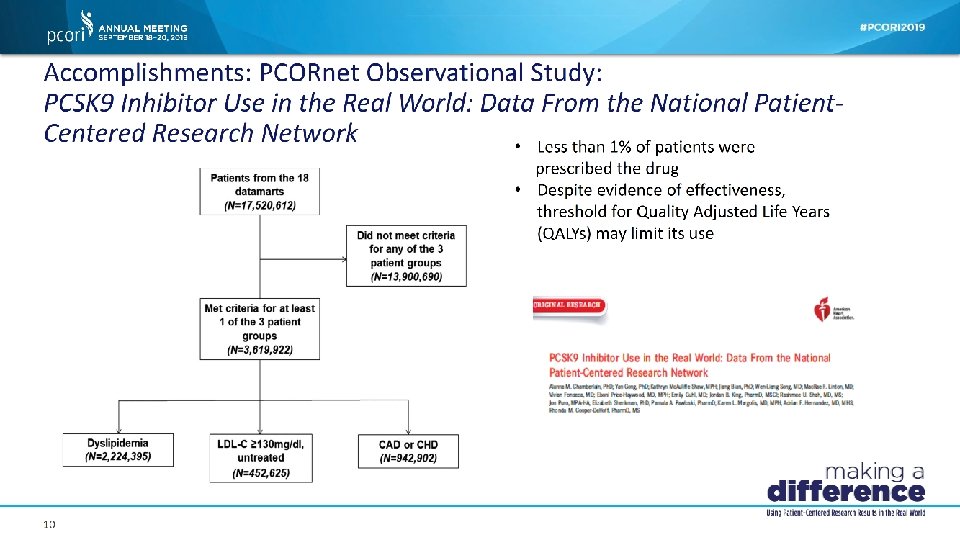
21
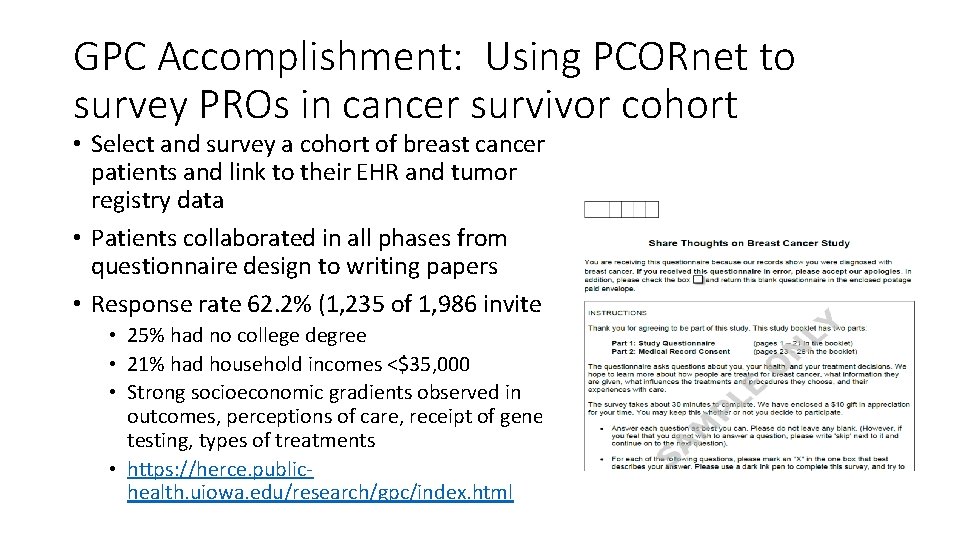
GPC Accomplishment: Using PCORnet to survey PROs in cancer survivor cohort • Select and survey a cohort of breast cancer patients and link to their EHR and tumor registry data • Patients collaborated in all phases from questionnaire design to writing papers • Response rate 62. 2% (1, 235 of 1, 986 invited) • 25% had no college degree • 21% had household incomes <$35, 000 • Strong socioeconomic gradients observed in outcomes, perceptions of care, receipt of genetic testing, types of treatments • https: //herce. public‐ health. uiowa. edu/research/gpc/index. html
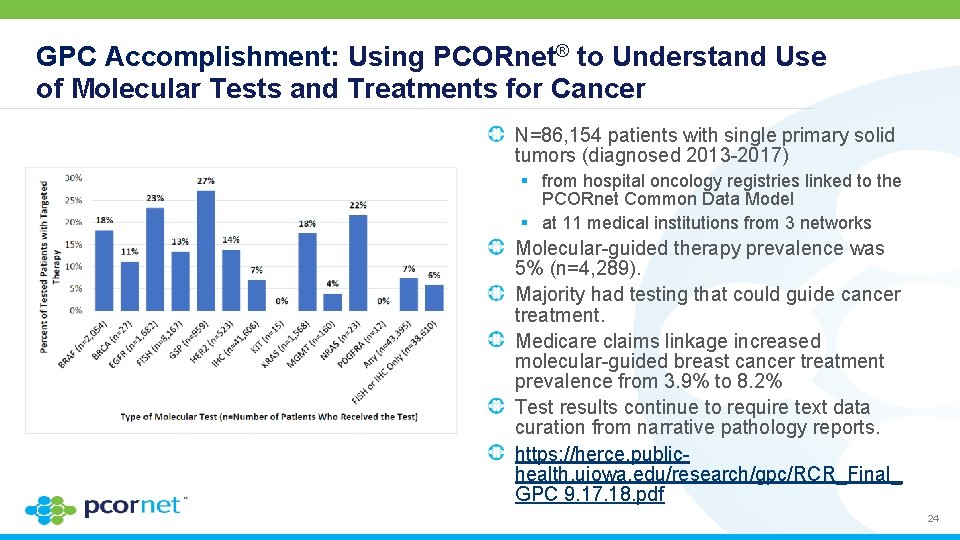
GPC Accomplishment: Using PCORnet® to Understand Use of Molecular Tests and Treatments for Cancer N=86, 154 patients with single primary solid tumors (diagnosed 2013 -2017) § from hospital oncology registries linked to the PCORnet Common Data Model § at 11 medical institutions from 3 networks Molecular-guided therapy prevalence was 5% (n=4, 289). Majority had testing that could guide cancer treatment. Medicare claims linkage increased molecular-guided breast cancer treatment prevalence from 3. 9% to 8. 2% Test results continue to require text data curation from narrative pathology reports. https: //herce. publichealth. uiowa. edu/research/gpc/RCR_Final_ GPC 9. 17. 18. pdf 24
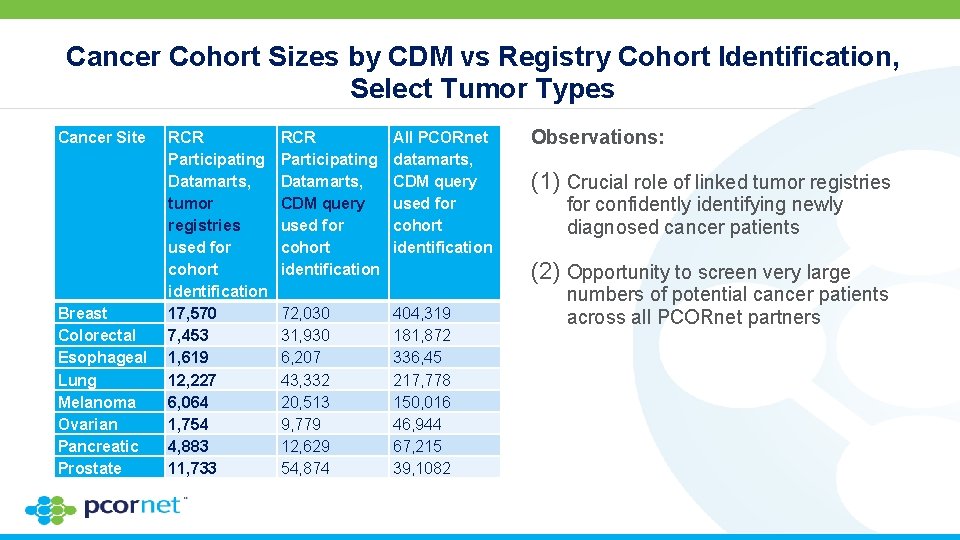
Cancer Cohort Sizes by CDM vs Registry Cohort Identification, Select Tumor Types Cancer Site Breast Colorectal Esophageal Lung Melanoma Ovarian Pancreatic Prostate RCR Participating Datamarts, tumor registries used for cohort identification 17, 570 7, 453 1, 619 12, 227 6, 064 1, 754 4, 883 11, 733 RCR Participating Datamarts, CDM query used for cohort identification 72, 030 31, 930 6, 207 43, 332 20, 513 9, 779 12, 629 54, 874 All PCORnet datamarts, CDM query used for cohort identification 404, 319 181, 872 336, 45 217, 778 150, 016 46, 944 67, 215 39, 1082 Observations: (1) Crucial role of linked tumor registries for confidently identifying newly diagnosed cancer patients (2) Opportunity to screen very large numbers of potential cancer patients across all PCORnet partners
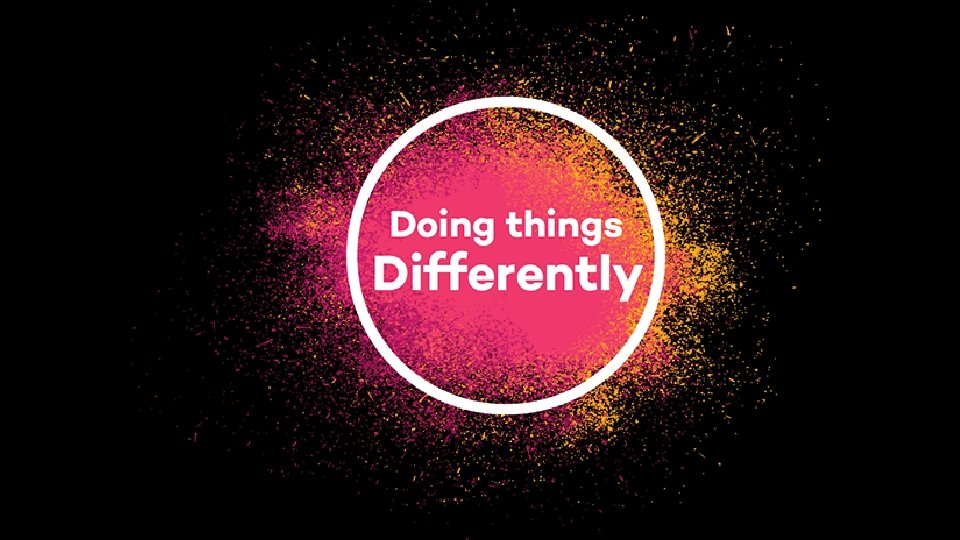
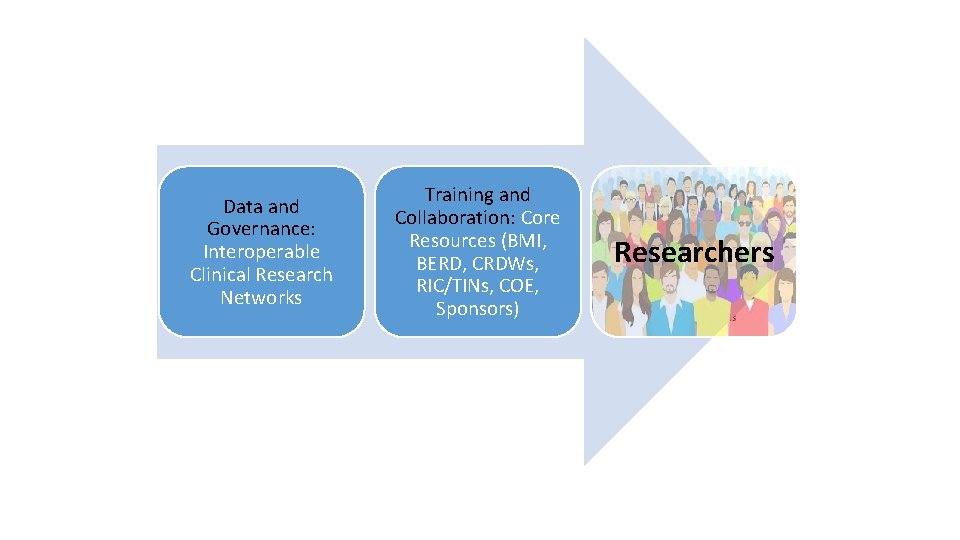
Data and Governance: Interoperable Clinical Research Networks Training and Collaboration: Core Resources (BMI, BERD, CRDWs, RIC/TINs, COE, Sponsors) Researchers
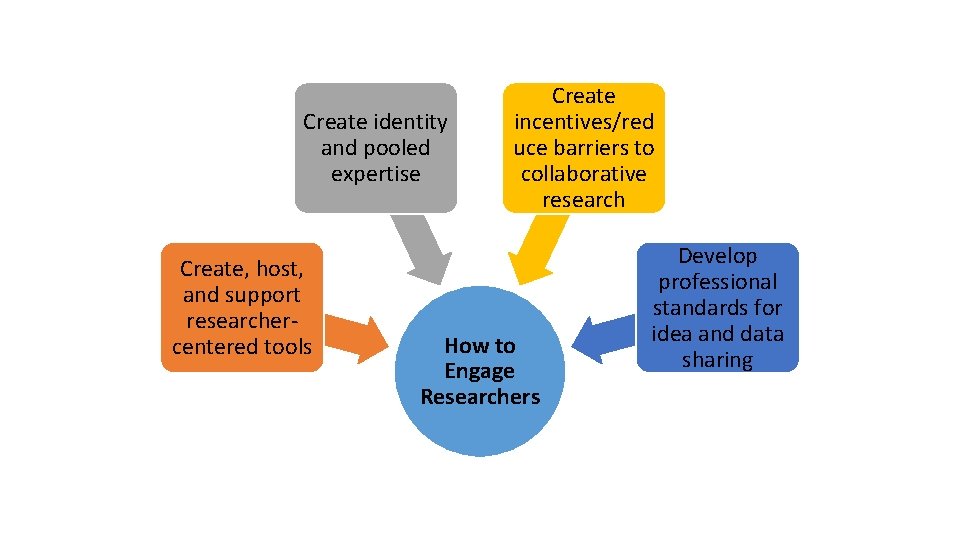
Create identity and pooled expertise Create, host, and support researcher‐ centered tools Create incentives/red uce barriers to collaborative research How to Engage Researchers Develop professional standards for idea and data sharing
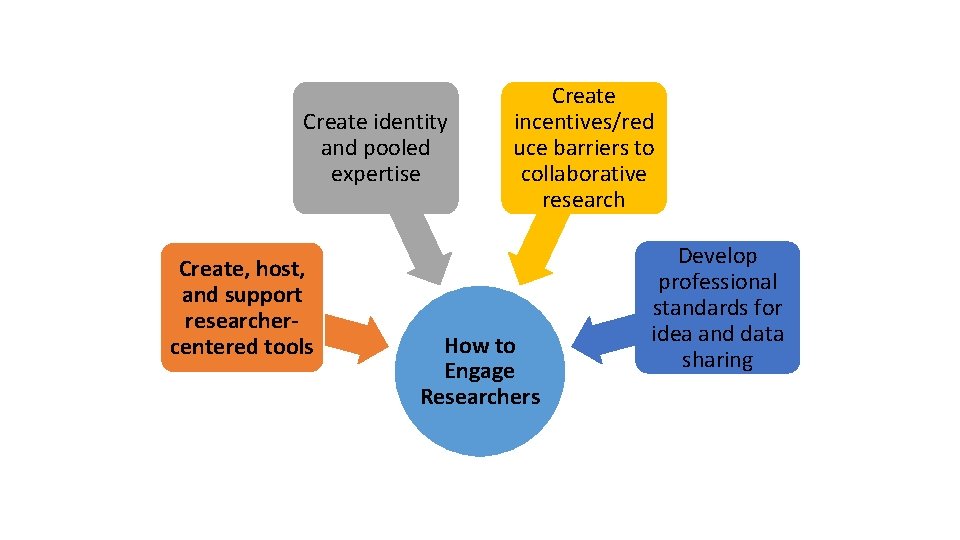
Create identity and pooled expertise Create, host, and support researchercentered tools Create incentives/red uce barriers to collaborative research How to Engage Researchers Develop professional standards for idea and data sharing
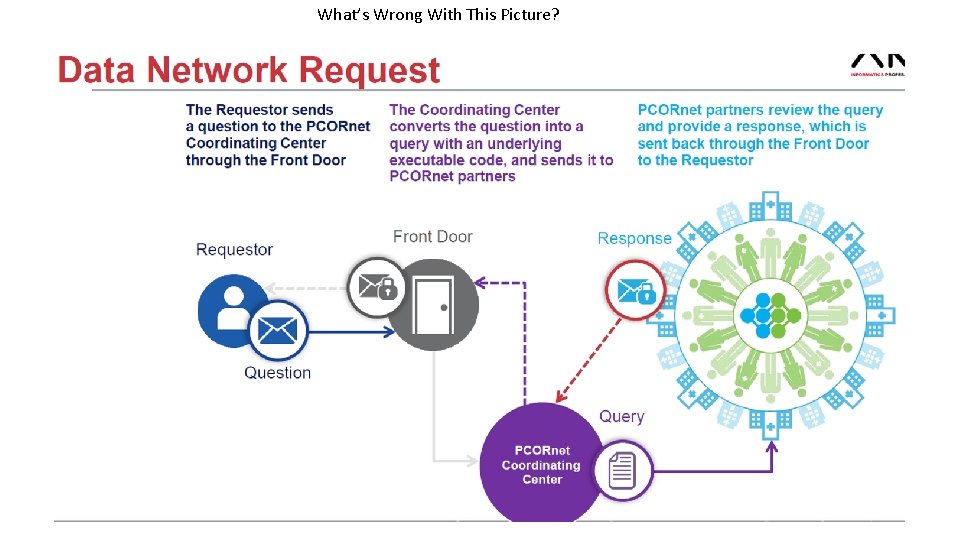
What’s Wrong With This Picture?
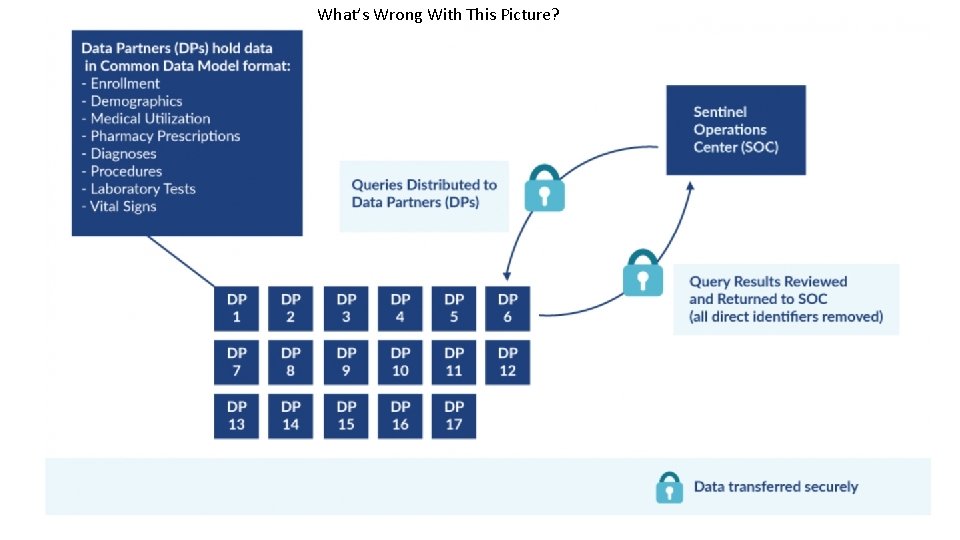
What’s Wrong With This Picture?
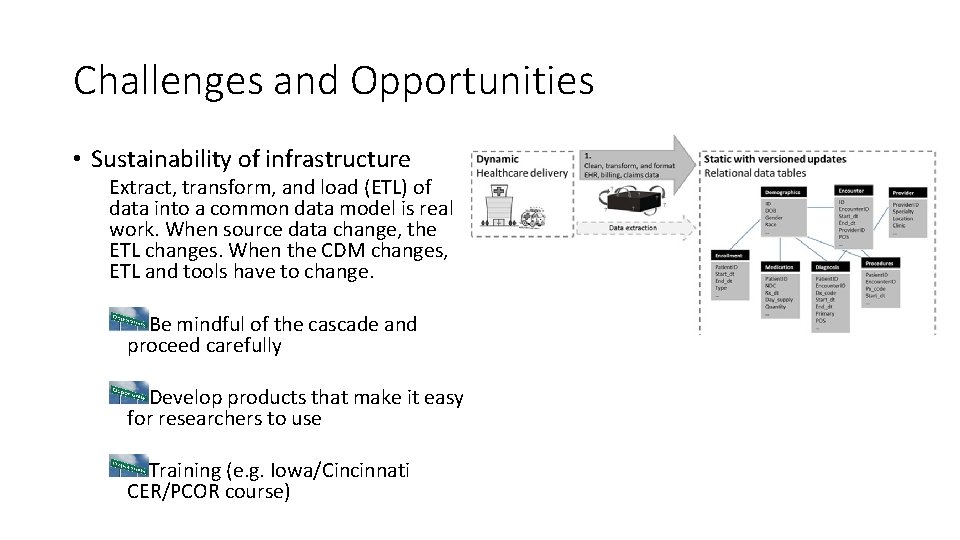
Challenges and Opportunities • Sustainability of infrastructure Extract, transform, and load (ETL) of data into a common data model is real work. When source data change, the ETL changes. When the CDM changes, ETL and tools have to change. Be mindful of the cascade and proceed carefully Develop products that make it easy for researchers to use Training (e. g. Iowa/Cincinnati CER/PCOR course)
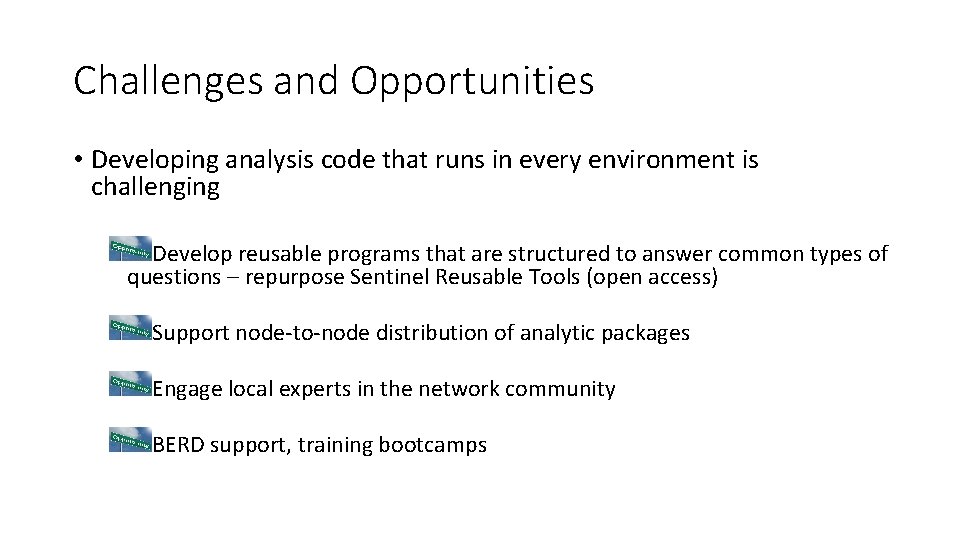
Challenges and Opportunities • Developing analysis code that runs in every environment is challenging Develop reusable programs that are structured to answer common types of questions – repurpose Sentinel Reusable Tools (open access) Support node‐to‐node distribution of analytic packages Engage local experts in the network community BERD support, training bootcamps
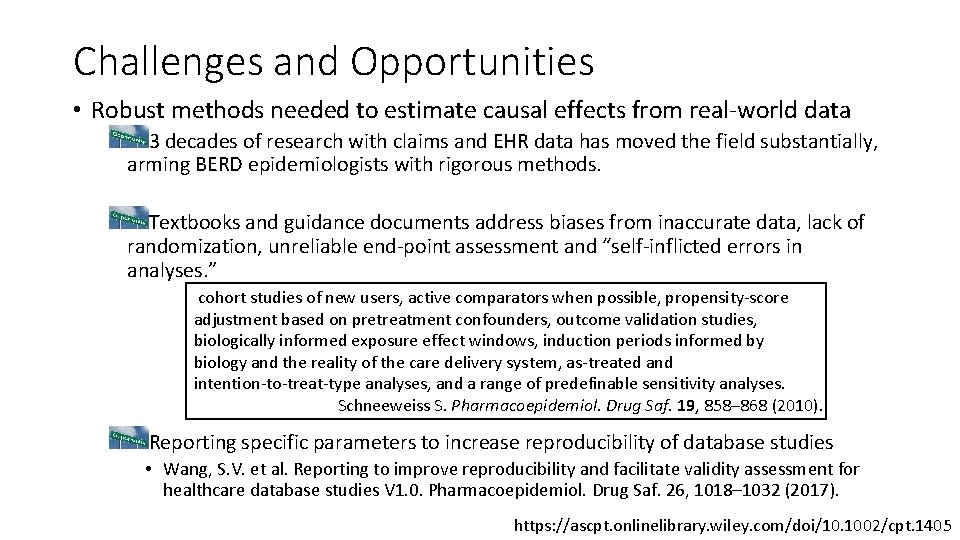
Challenges and Opportunities • Robust methods needed to estimate causal effects from real‐world data 3 decades of research with claims and EHR data has moved the field substantially, arming BERD epidemiologists with rigorous methods. Textbooks and guidance documents address biases from inaccurate data, lack of randomization, unreliable end‐point assessment and “self‐inflicted errors in analyses. ” cohort studies of new users, active comparators when possible, propensity‐score adjustment based on pretreatment confounders, outcome validation studies, biologically informed exposure effect windows, induction periods informed by biology and the reality of the care delivery system, as‐treated and intention‐to‐treat‐type analyses, and a range of predefinable sensitivity analyses. Schneeweiss S. Pharmacoepidemiol. Drug Saf. 19, 858– 868 (2010). Reporting specific parameters to increase reproducibility of database studies • Wang, S. V. et al. Reporting to improve reproducibility and facilitate validity assessment for healthcare database studies V 1. 0. Pharmacoepidemiol. Drug Saf. 26, 1018– 1032 (2017). https: //ascpt. onlinelibrary. wiley. com/doi/10. 1002/cpt. 1405
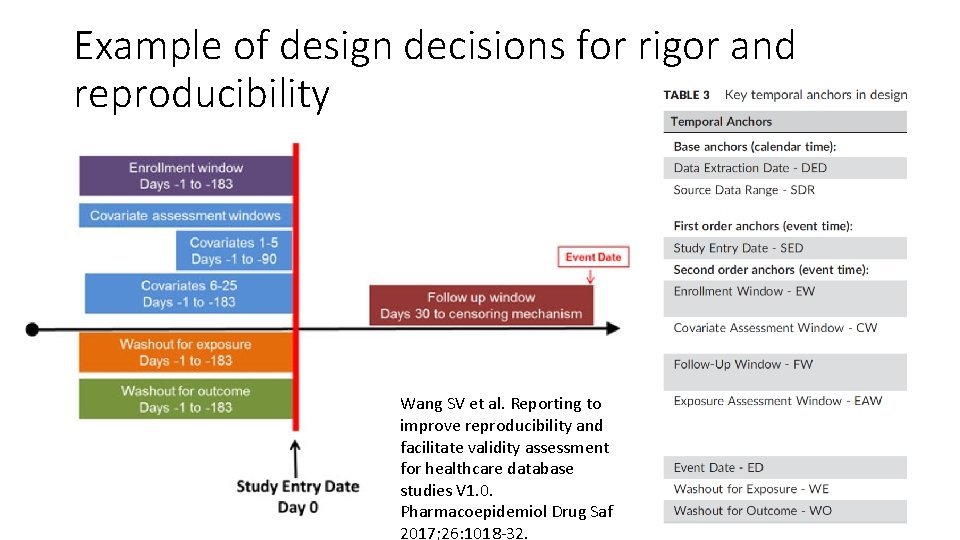
Example of design decisions for rigor and reproducibility Wang SV et al. Reporting to improve reproducibility and facilitate validity assessment for healthcare database studies V 1. 0. Pharmacoepidemiol Drug Saf 2017; 26: 1018‐ 32.
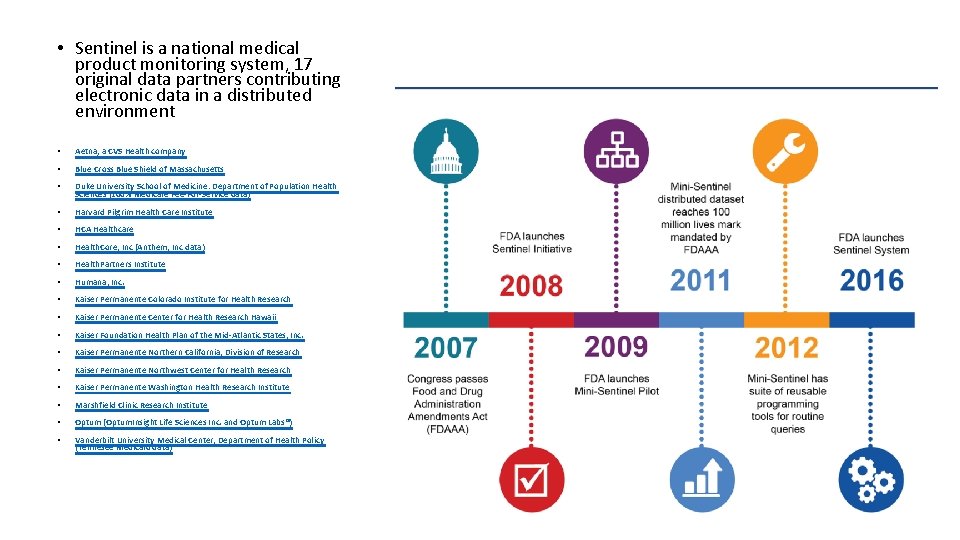
• Sentinel is a national medical product monitoring system, 17 original data partners contributing electronic data in a distributed environment • Aetna, a CVS Health company • Blue Cross Blue Shield of Massachusetts • Duke University School of Medicine: Department of Population Health Sciences (100% Medicare Fee-For-Service data) • Harvard Pilgrim Health Care Institute • HCA Healthcare • Health. Core, Inc (Anthem, Inc data) • Health. Partners Institute • Humana, Inc. • Kaiser Permanente Colorado Institute for Health Research • Kaiser Permanente Center for Health Research Hawaii • Kaiser Foundation Health Plan of the Mid-Atlantic States, Inc. • Kaiser Permanente Northern California, Division of Research • Kaiser Permanente Northwest Center for Health Research • Kaiser Permanente Washington Health Research Institute • Marshfield Clinic Research Institute • Optum (Optum. Insight Life Sciences Inc. and Optum Labs®) • Vanderbilt University Medical Center, Department of Health Policy (Tennesee Medicaid data)
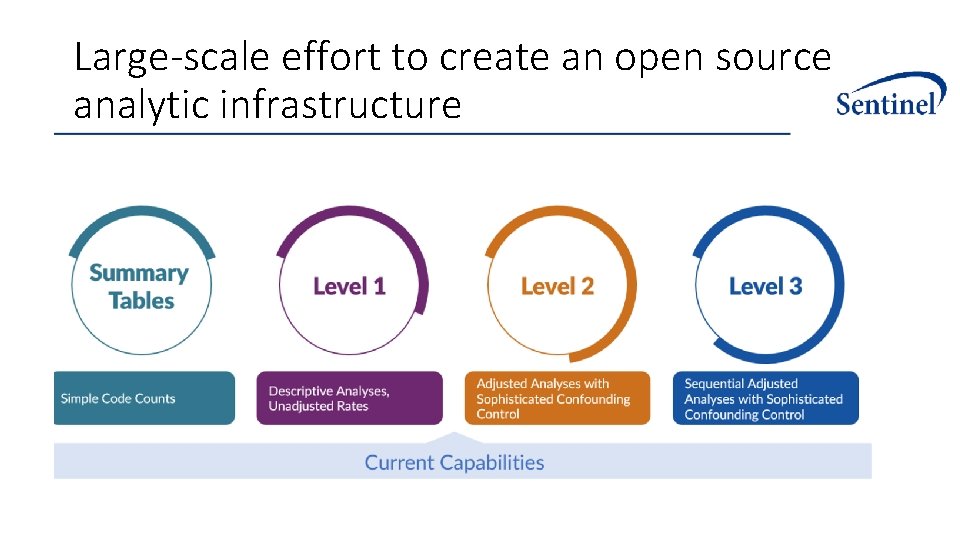
Large-scale effort to create an open source analytic infrastructure
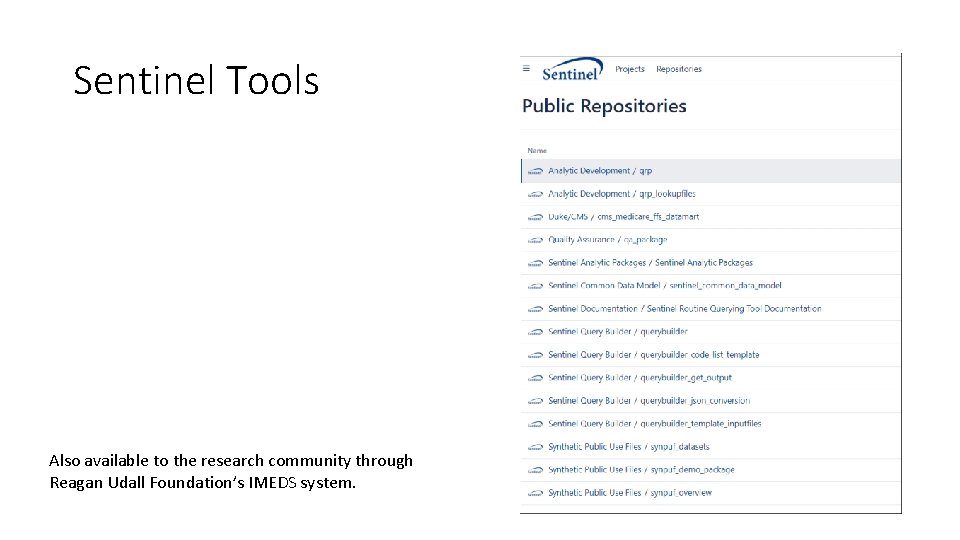
Sentinel Tools Also available to the research community through Reagan Udall Foundation’s IMEDS system.
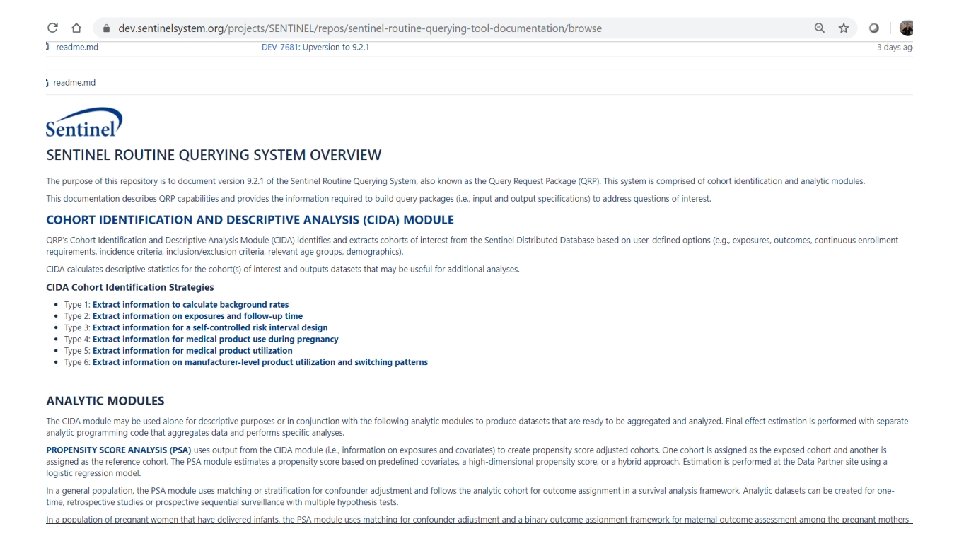
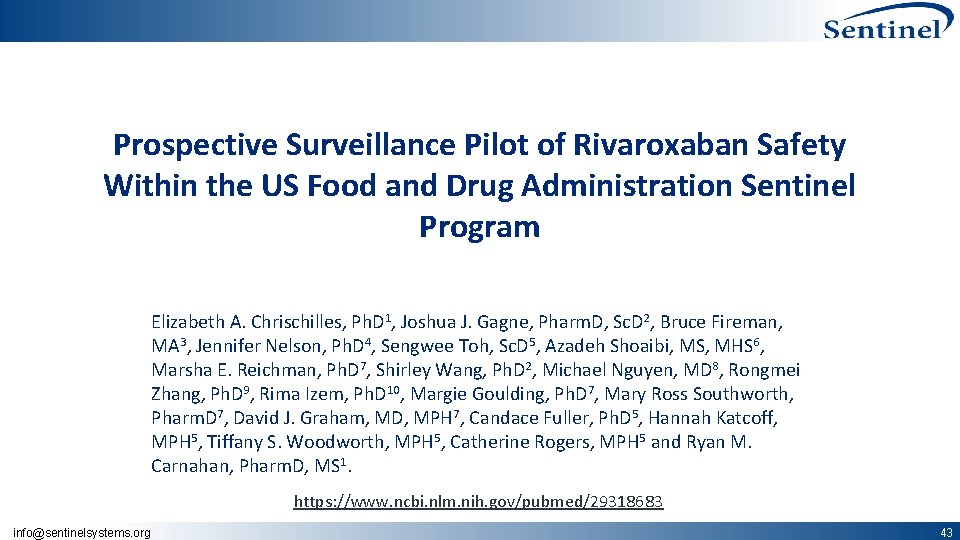
Prospective Surveillance Pilot of Rivaroxaban Safety Within the US Food and Drug Administration Sentinel Program Elizabeth A. Chrischilles, Ph. D 1, Joshua J. Gagne, Pharm. D, Sc. D 2, Bruce Fireman, MA 3, Jennifer Nelson, Ph. D 4, Sengwee Toh, Sc. D 5, Azadeh Shoaibi, MS, MHS 6, Marsha E. Reichman, Ph. D 7, Shirley Wang, Ph. D 2, Michael Nguyen, MD 8, Rongmei Zhang, Ph. D 9, Rima Izem, Ph. D 10, Margie Goulding, Ph. D 7, Mary Ross Southworth, Pharm. D 7, David J. Graham, MD, MPH 7, Candace Fuller, Ph. D 5, Hannah Katcoff, MPH 5, Tiffany S. Woodworth, MPH 5, Catherine Rogers, MPH 5 and Ryan M. Carnahan, Pharm. D, MS 1. https: //www. ncbi. nlm. nih. gov/pubmed/29318683 info@sentinelsystems. org 43
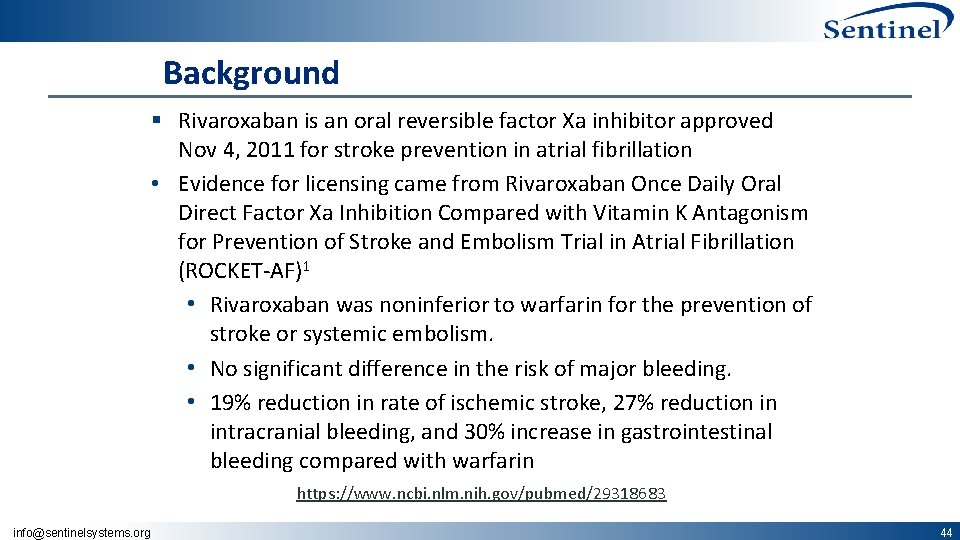
Background § Rivaroxaban is an oral reversible factor Xa inhibitor approved Nov 4, 2011 for stroke prevention in atrial fibrillation • Evidence for licensing came from Rivaroxaban Once Daily Oral Direct Factor Xa Inhibition Compared with Vitamin K Antagonism for Prevention of Stroke and Embolism Trial in Atrial Fibrillation (ROCKET‐AF)1 • Rivaroxaban was noninferior to warfarin for the prevention of stroke or systemic embolism. • No significant difference in the risk of major bleeding. • 19% reduction in rate of ischemic stroke, 27% reduction in intracranial bleeding, and 30% increase in gastrointestinal bleeding compared with warfarin https: //www. ncbi. nlm. nih. gov/pubmed/29318683 info@sentinelsystems. org 44
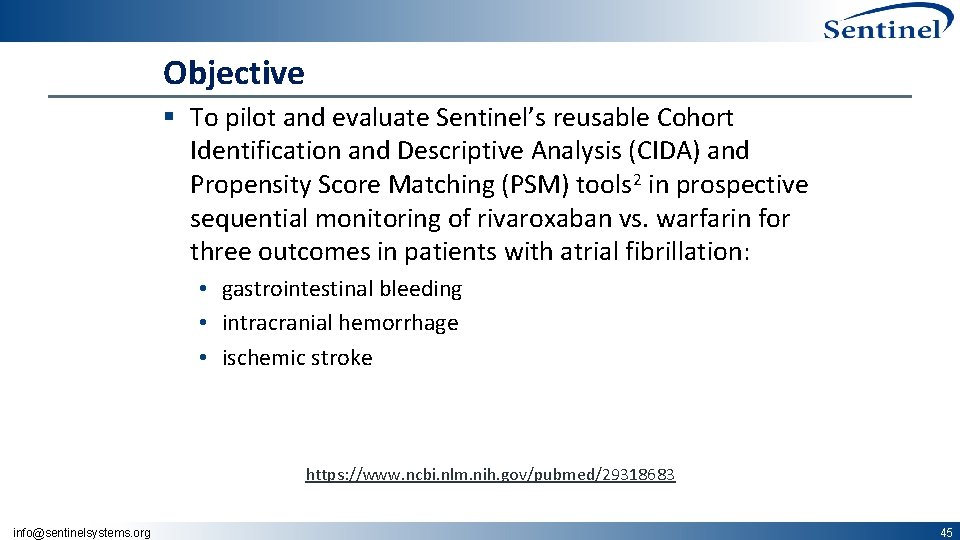
Objective § To pilot and evaluate Sentinel’s reusable Cohort Identification and Descriptive Analysis (CIDA) and Propensity Score Matching (PSM) tools 2 in prospective sequential monitoring of rivaroxaban vs. warfarin for three outcomes in patients with atrial fibrillation: • gastrointestinal bleeding • intracranial hemorrhage • ischemic stroke https: //www. ncbi. nlm. nih. gov/pubmed/29318683 info@sentinelsystems. org 45
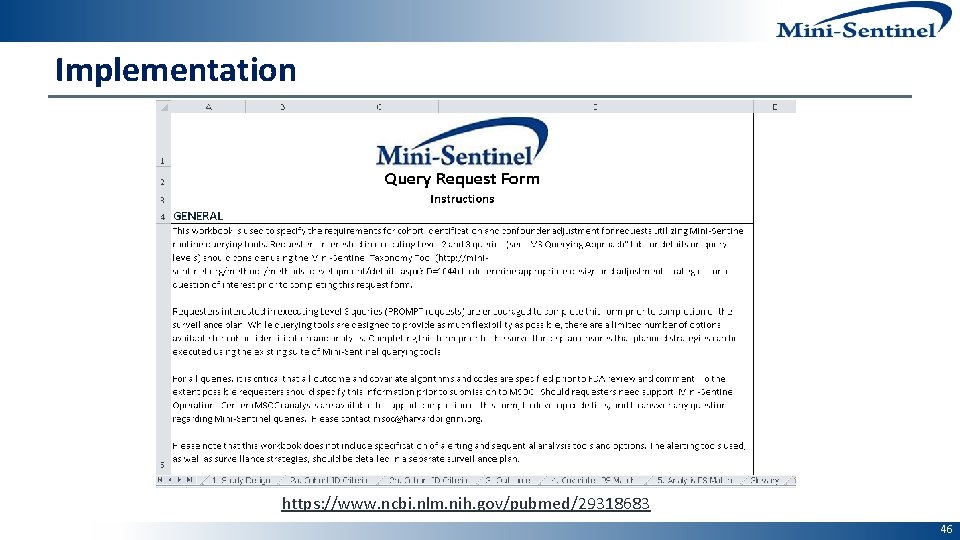
Implementation https: //www. ncbi. nlm. nih. gov/pubmed/29318683 46
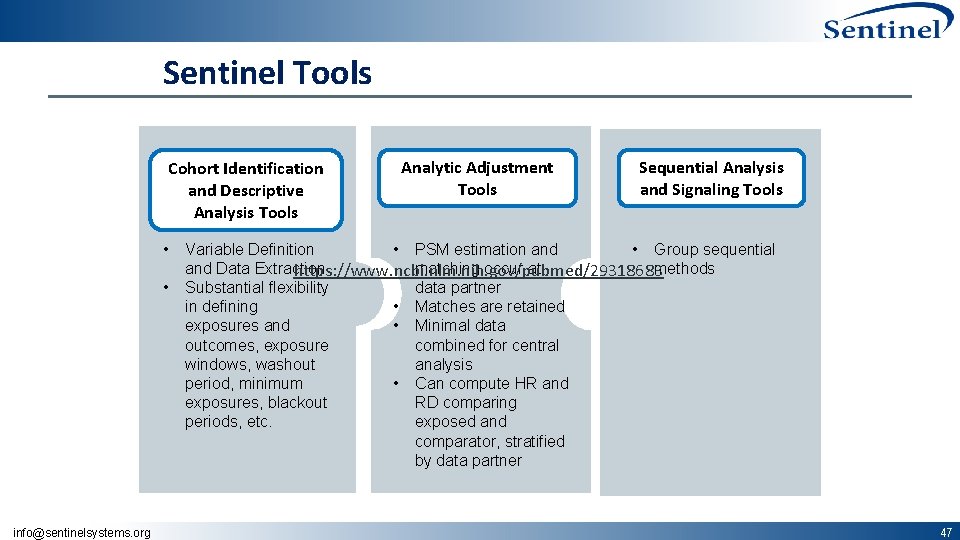
Sentinel Tools Cohort Identification and Descriptive Analysis Tools • • info@sentinelsystems. org Analytic Adjustment Tools Sequential Analysis and Signaling Tools Variable Definition • PSM estimation and • Group sequential and Data Extraction matching occur at methods https: //www. ncbi. nlm. nih. gov/pubmed/29318683 Substantial flexibility data partner in defining • Matches are retained exposures and • Minimal data outcomes, exposure combined for central windows, washout analysis period, minimum • Can compute HR and exposures, blackout RD comparing periods, etc. exposed and comparator, stratified by data partner 47
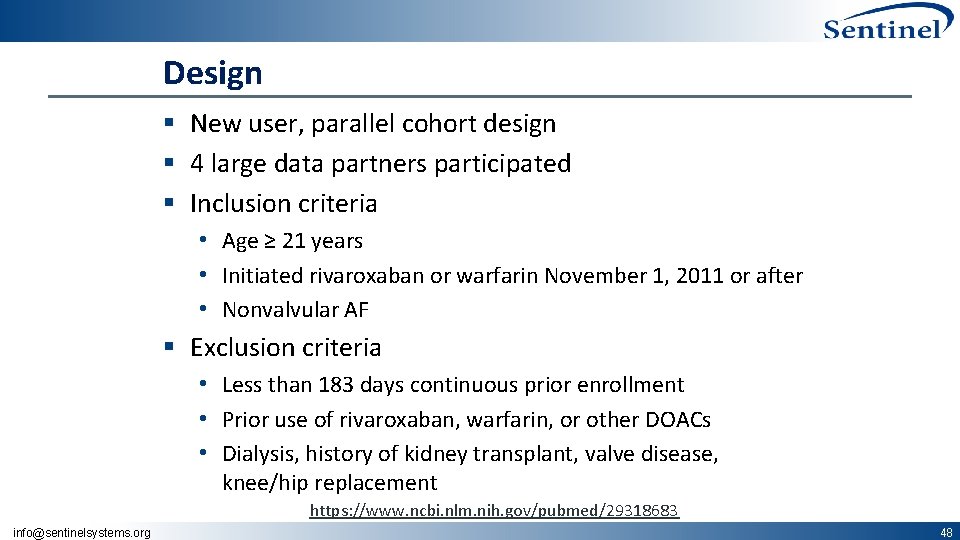
Design § New user, parallel cohort design § 4 large data partners participated § Inclusion criteria • Age ≥ 21 years • Initiated rivaroxaban or warfarin November 1, 2011 or after • Nonvalvular AF § Exclusion criteria • Less than 183 days continuous prior enrollment • Prior use of rivaroxaban, warfarin, or other DOACs • Dialysis, history of kidney transplant, valve disease, knee/hip replacement https: //www. ncbi. nlm. nih. gov/pubmed/29318683 info@sentinelsystems. org 48
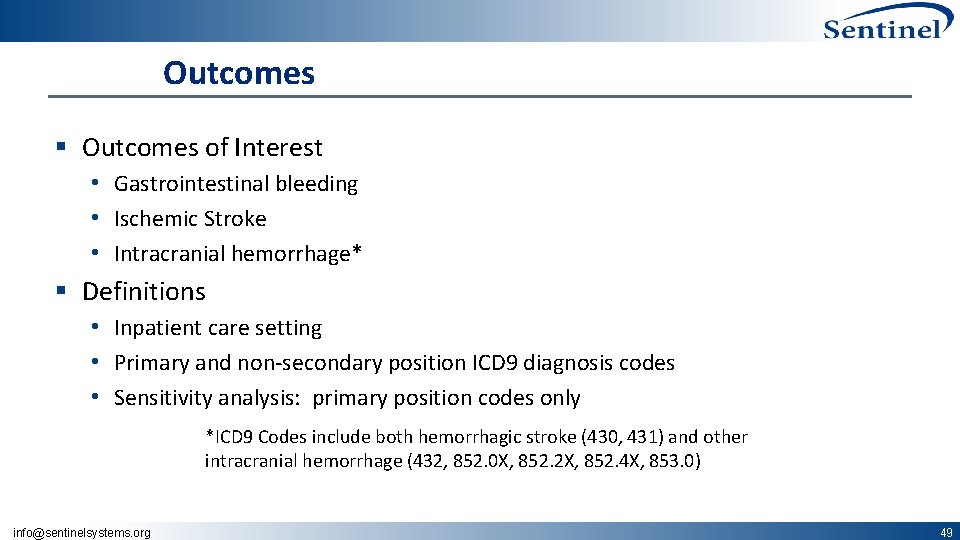
Outcomes § Outcomes of Interest • Gastrointestinal bleeding • Ischemic Stroke • Intracranial hemorrhage* § Definitions • Inpatient care setting • Primary and non‐secondary position ICD 9 diagnosis codes • Sensitivity analysis: primary position codes only *ICD 9 Codes include both hemorrhagic stroke (430, 431) and other intracranial hemorrhage (432, 852. 0 X, 852. 2 X, 852. 4 X, 853. 0) info@sentinelsystems. org 49
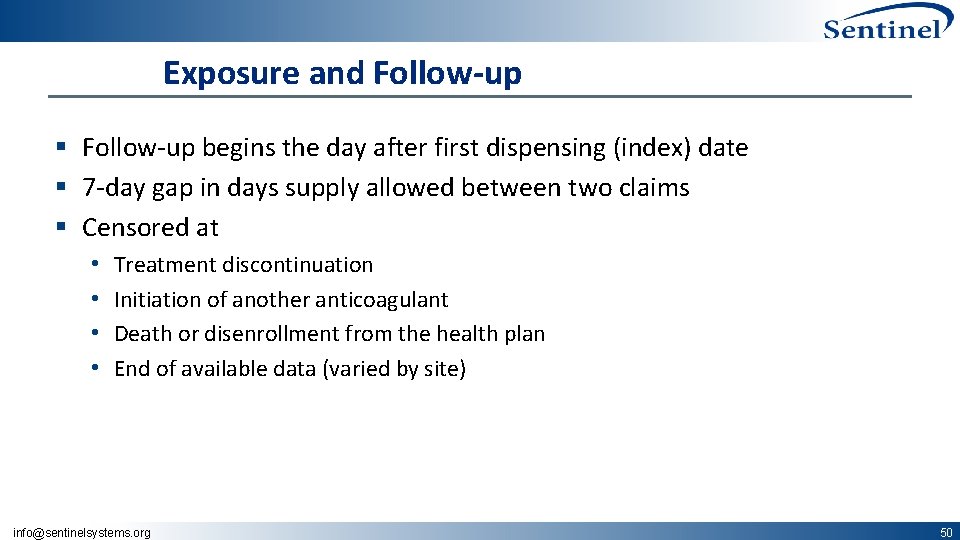
Exposure and Follow-up § Follow‐up begins the day after first dispensing (index) date § 7‐day gap in days supply allowed between two claims § Censored at • • Treatment discontinuation Initiation of another anticoagulant Death or disenrollment from the health plan End of available data (varied by site) info@sentinelsystems. org 50
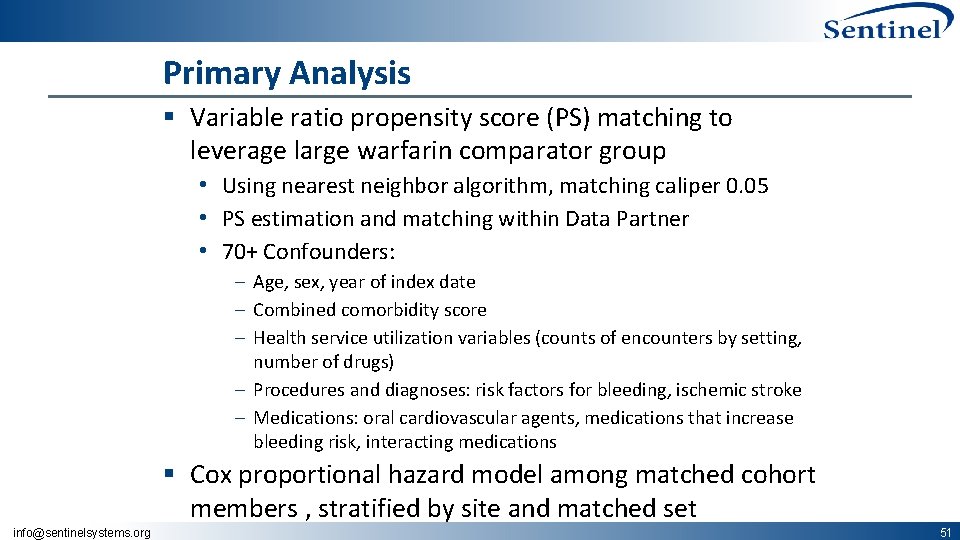
Primary Analysis § Variable ratio propensity score (PS) matching to leverage large warfarin comparator group • Using nearest neighbor algorithm, matching caliper 0. 05 • PS estimation and matching within Data Partner • 70+ Confounders: – Age, sex, year of index date – Combined comorbidity score – Health service utilization variables (counts of encounters by setting, number of drugs) – Procedures and diagnoses: risk factors for bleeding, ischemic stroke – Medications: oral cardiovascular agents, medications that increase bleeding risk, interacting medications § Cox proportional hazard model among matched cohort members , stratified by site and matched set info@sentinelsystems. org 51
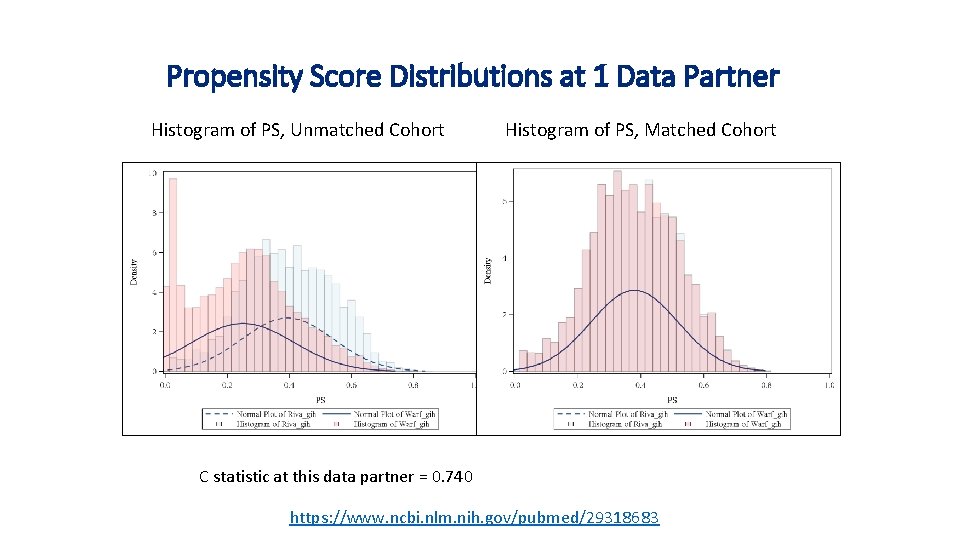
Propensity Score Distributions at 1 Data Partner Histogram of PS, Unmatched Cohort Histogram of PS, Matched Cohort C statistic at this data partner = 0. 740 https: //www. ncbi. nlm. nih. gov/pubmed/29318683
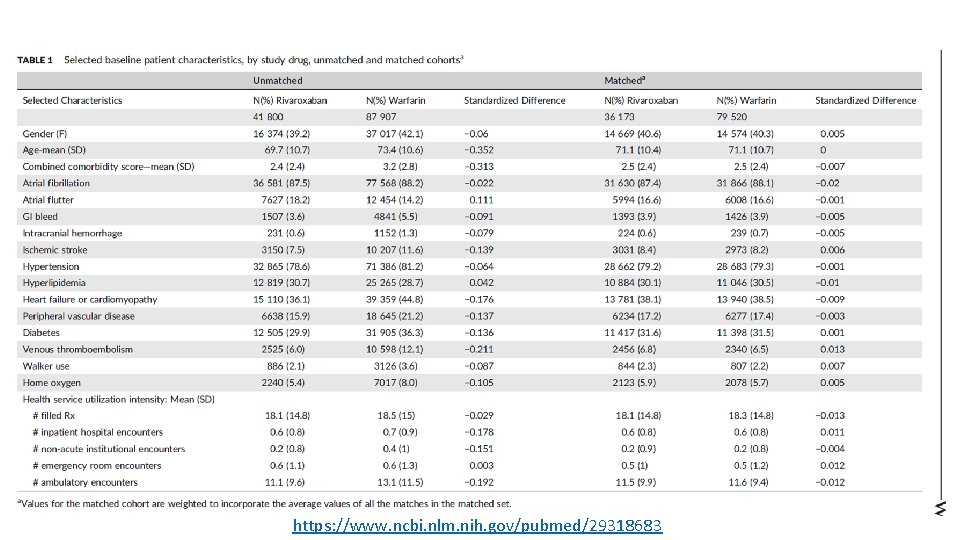
https: //www. ncbi. nlm. nih. gov/pubmed/29318683
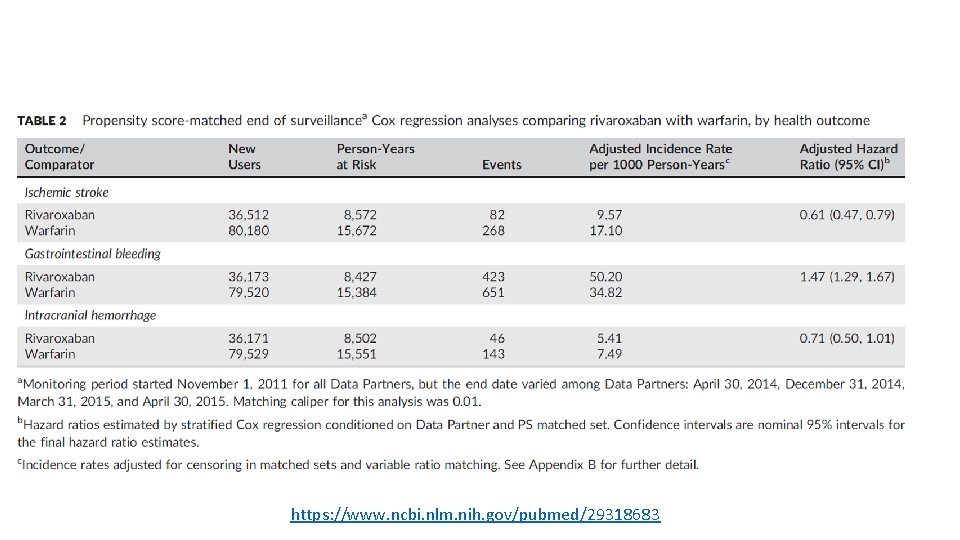
https: //www. ncbi. nlm. nih. gov/pubmed/29318683
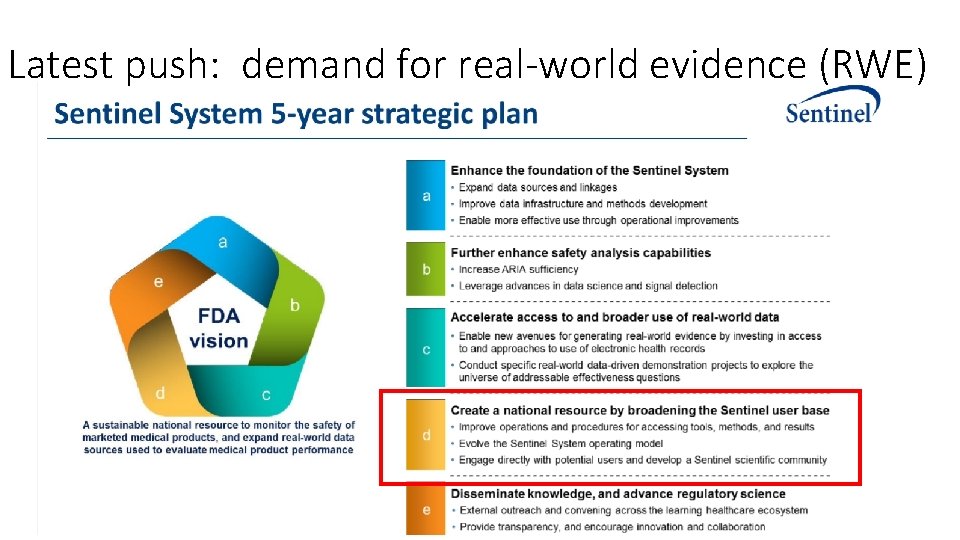
Latest push: demand for real-world evidence (RWE)
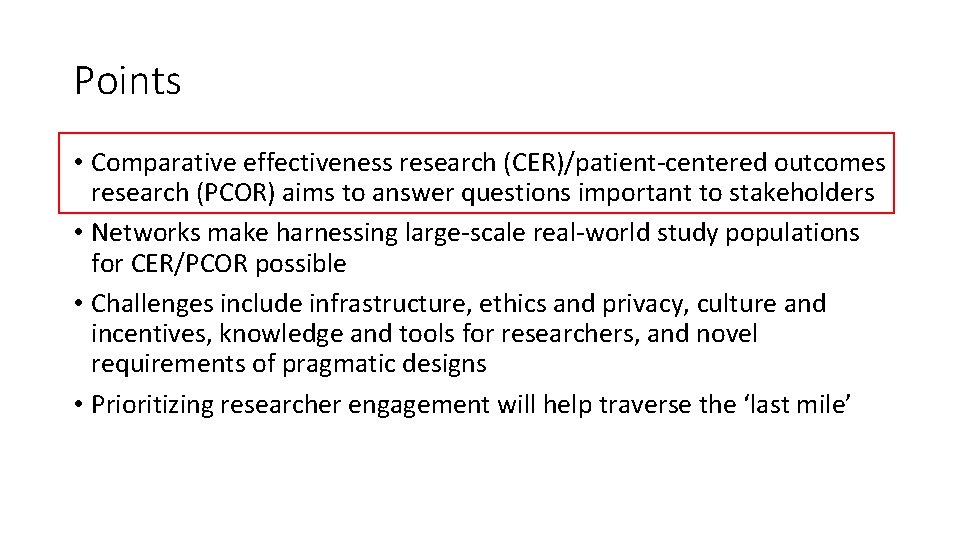
Points • Comparative effectiveness research (CER)/patient‐centered outcomes research (PCOR) aims to answer questions important to stakeholders • Networks make harnessing large‐scale real‐world study populations for CER/PCOR possible • Challenges include infrastructure, ethics and privacy, culture and incentives, knowledge and tools for researchers, and novel requirements of pragmatic designs • Prioritizing researcher engagement will help traverse the ‘last mile’
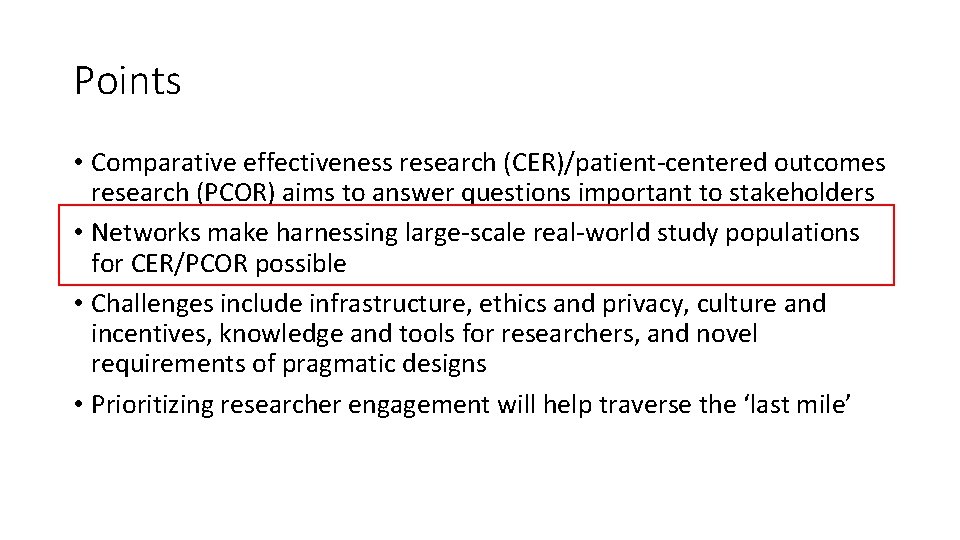
Points • Comparative effectiveness research (CER)/patient‐centered outcomes research (PCOR) aims to answer questions important to stakeholders • Networks make harnessing large‐scale real‐world study populations for CER/PCOR possible • Challenges include infrastructure, ethics and privacy, culture and incentives, knowledge and tools for researchers, and novel requirements of pragmatic designs • Prioritizing researcher engagement will help traverse the ‘last mile’
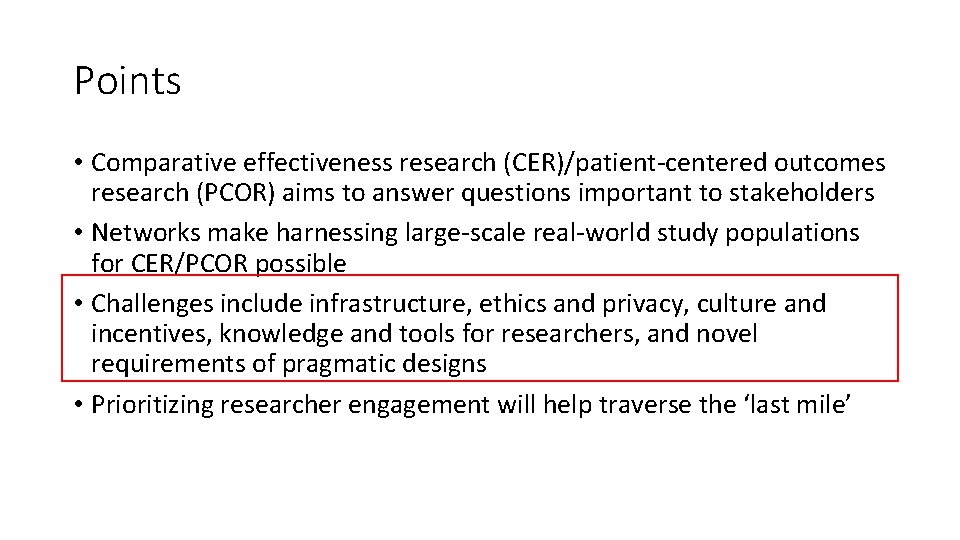
Points • Comparative effectiveness research (CER)/patient‐centered outcomes research (PCOR) aims to answer questions important to stakeholders • Networks make harnessing large‐scale real‐world study populations for CER/PCOR possible • Challenges include infrastructure, ethics and privacy, culture and incentives, knowledge and tools for researchers, and novel requirements of pragmatic designs • Prioritizing researcher engagement will help traverse the ‘last mile’
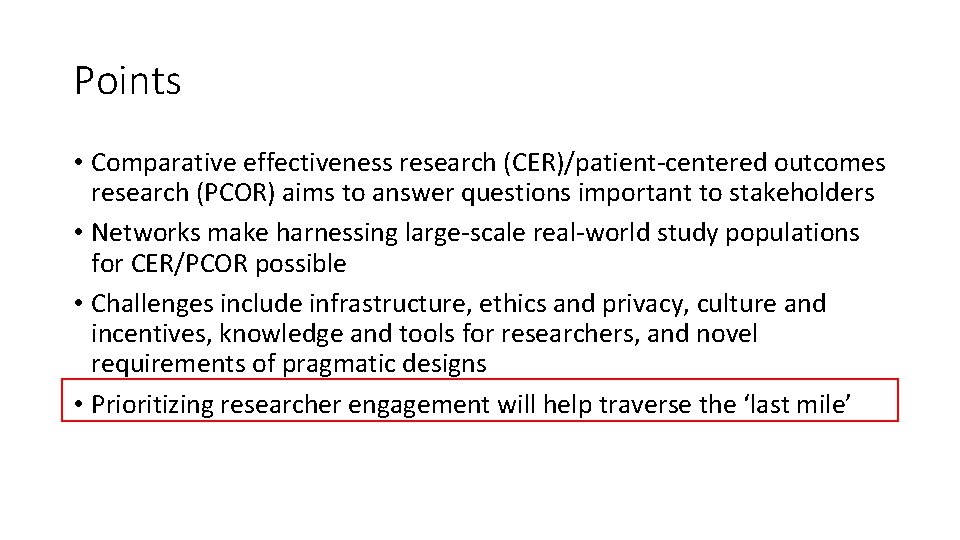
Points • Comparative effectiveness research (CER)/patient‐centered outcomes research (PCOR) aims to answer questions important to stakeholders • Networks make harnessing large‐scale real‐world study populations for CER/PCOR possible • Challenges include infrastructure, ethics and privacy, culture and incentives, knowledge and tools for researchers, and novel requirements of pragmatic designs • Prioritizing researcher engagement will help traverse the ‘last mile’
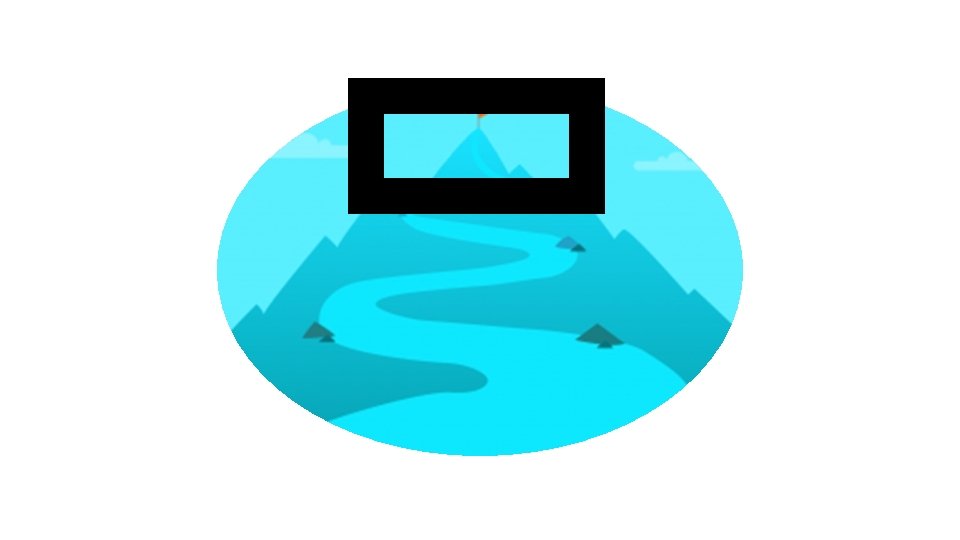
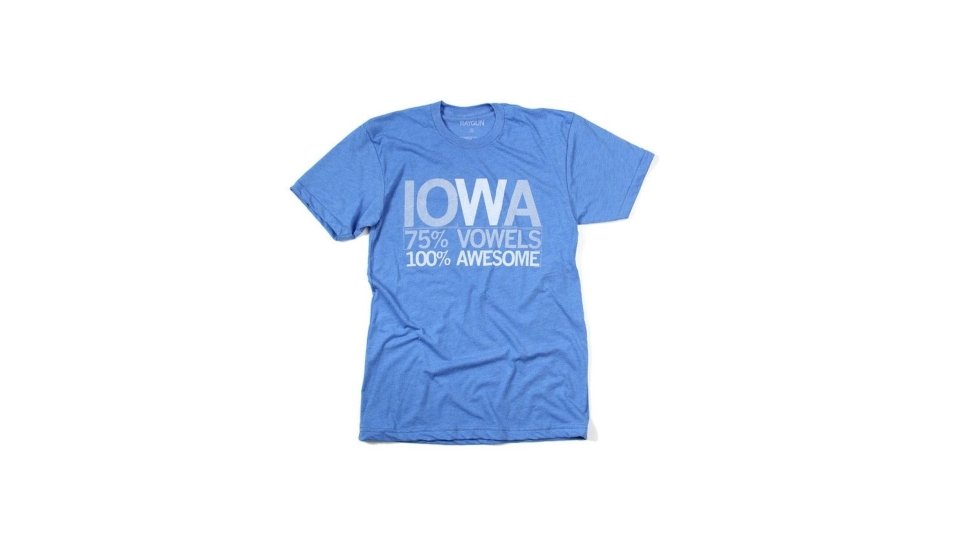
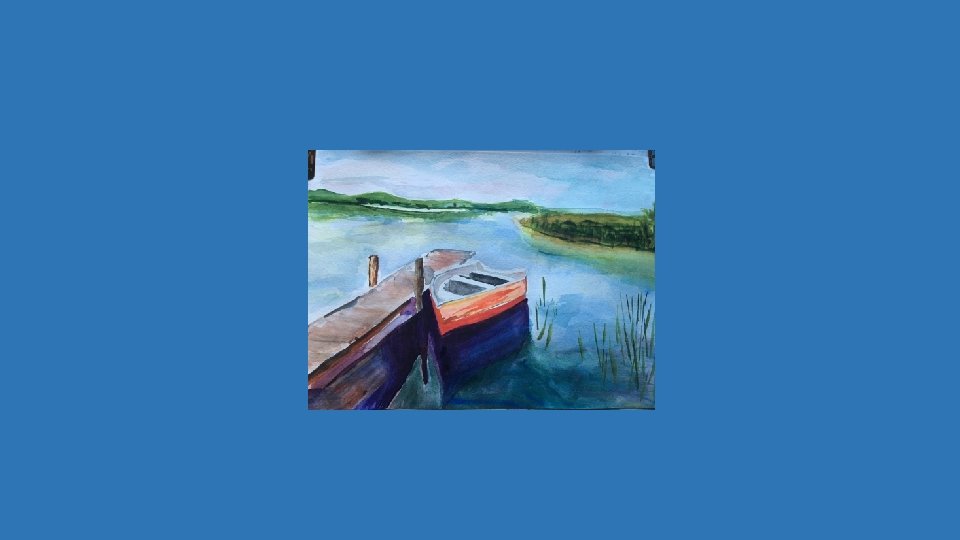
Unit 42 passive 1 is done was done
Hammer mill principle
Sen bir koyun olsan bende bir kuzu sözleri
Words that are spelled the same but pronounced differently
Properties of light
Translucent object
C 2 =121
Why water behaves differently
What chapter does rasheed make mariam eat rocks
Everyone learns
Through grandpa's eyes questions and answers
We all think differently
Magnipros see things differently
Why do irr and npv rank the two projects differently?
Choose the word which is stressed differently from the rest
Boys and girls brains
Expected results research proposal example
Expected outcomes in research examples
Causal comparative research meaning
Criminology unit 4 crime and punishment
The effectiveness of online and blended learning
Steiner's model of group productivity
Definition of personal resources
Ntu in heat exchanger
It is arrangement of people in an organization
Efficiency and effectiveness examples
Cath lab cost effectiveness
Higher history britain essays
Volumetric efficiency formula
Parallel plate heat exchanger
Enhancing personal effectiveness
Slip, slop, slap campaign effectiveness
Team effectiveness model robbins
It means making use of all the personal resources
Certificate of personal effectiveness
Pear audit
Goals of interpersonal effectiveness
Instructional effectiveness
Efficiency and effectiveness in higher education
Effectiveness algoritma
Managing productivity and marketing effectiveness
Measuring the effectiveness of promotional program
Advancing education effectiveness
Leadership effectiveness inventory
Constraint in accounting
Ppg examples for educator effectiveness
Sales effectiveness framework
Overall equipment effectiveness
Personal effectiveness framework
Enthalpy wheel effectiveness calculation
Graphic language definition
Measuring coaching effectiveness
Principles of effectiveness
Nebidos
Happy mid term break
Sales force effectiveness ppt
Pear process effectiveness assessment report