Professorship Personalised Digital Health Working on self management
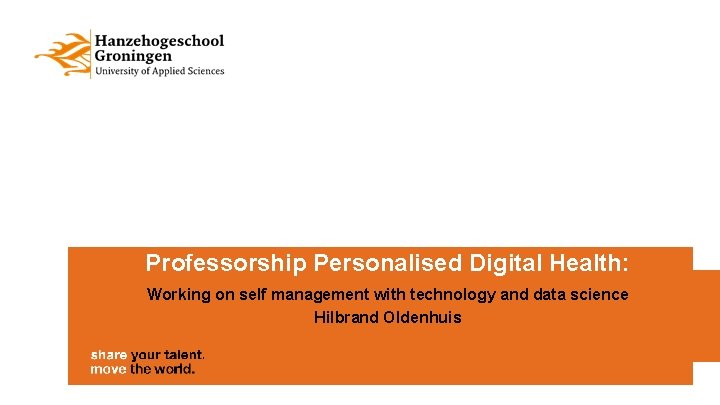
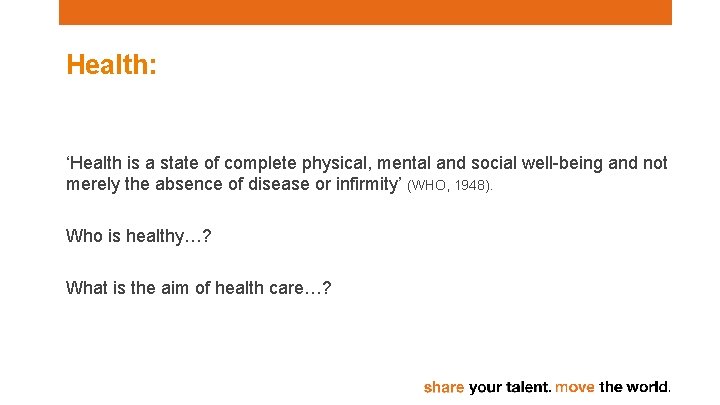
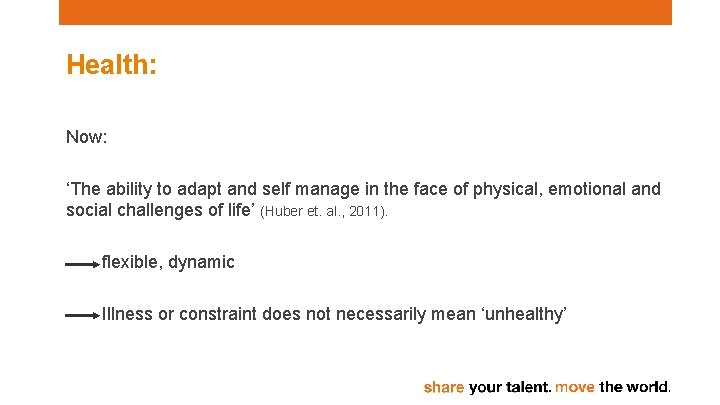
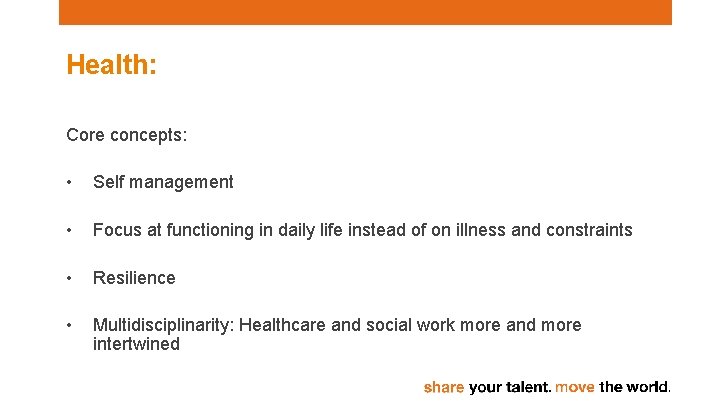
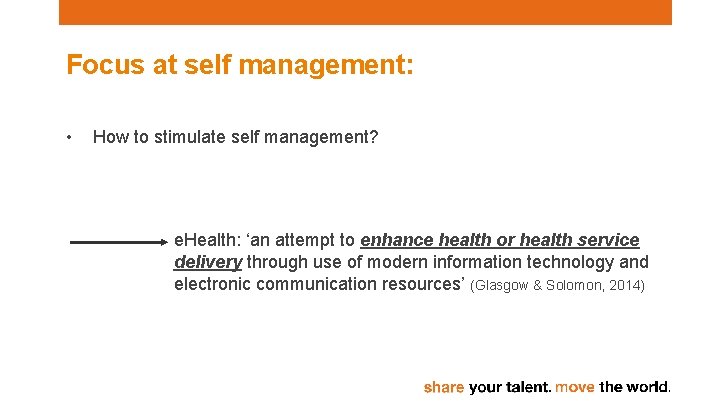
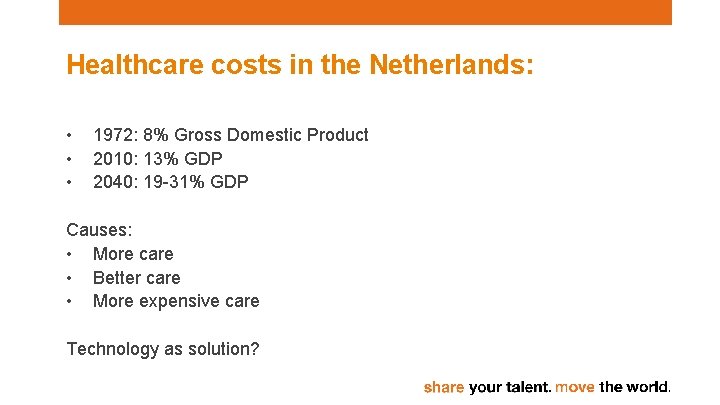
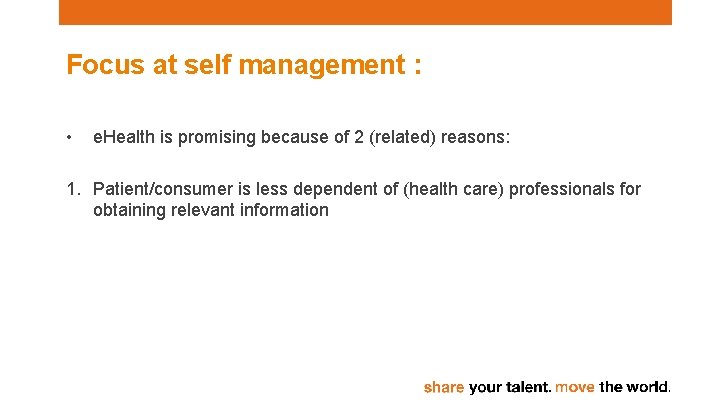
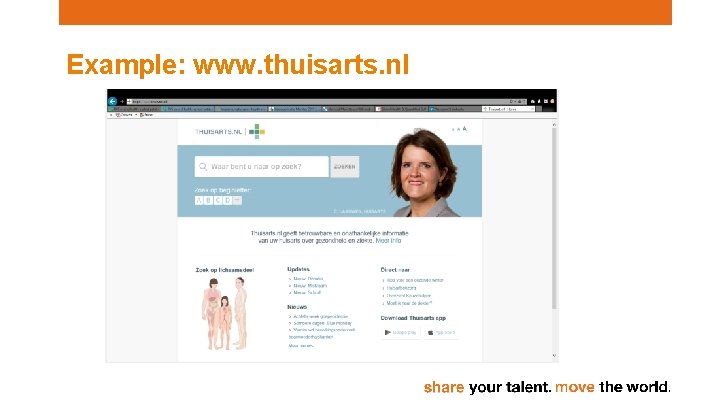
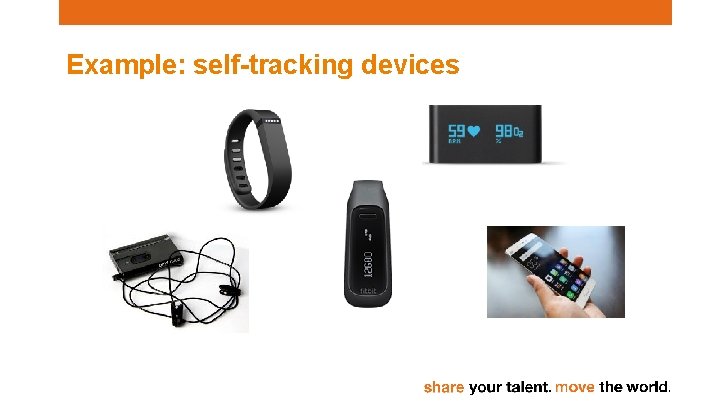
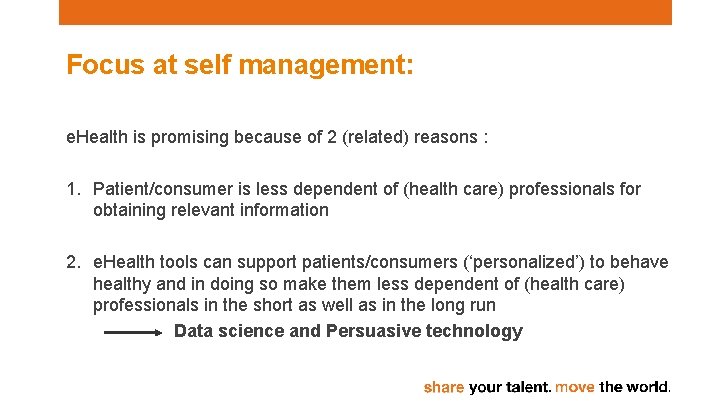
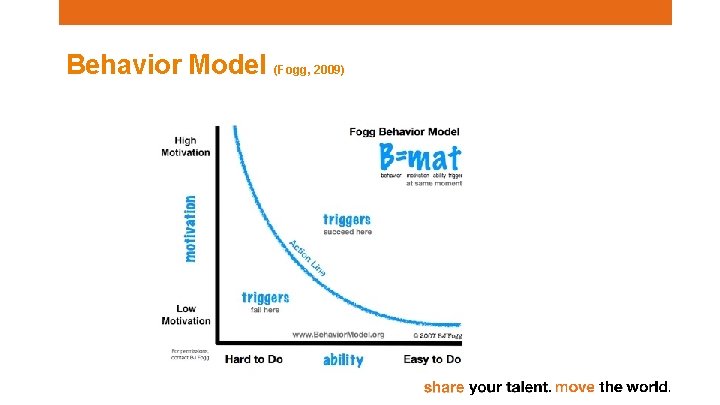
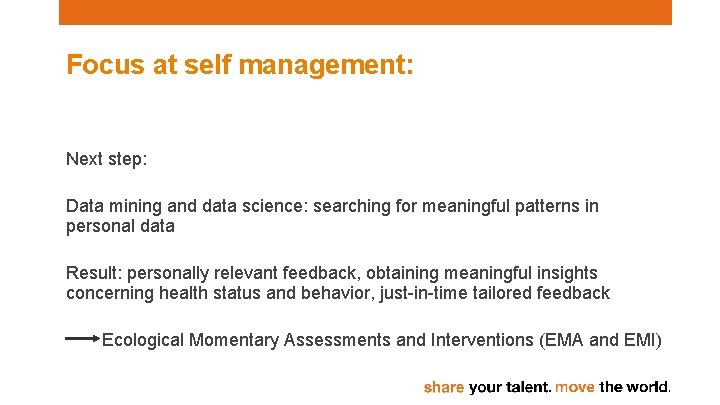
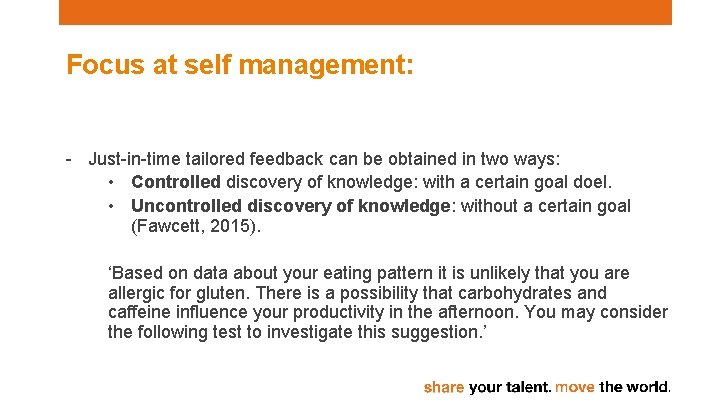
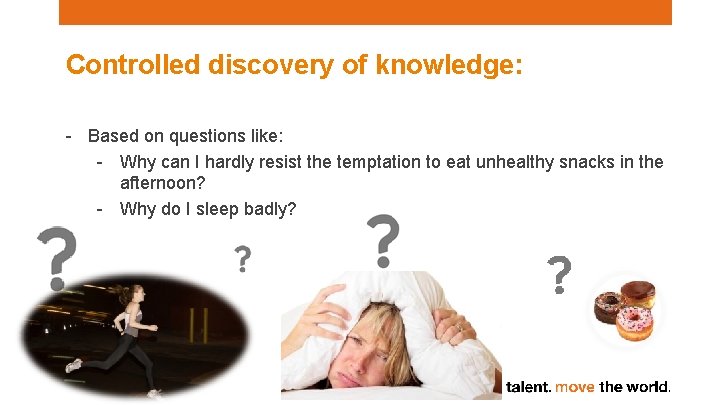
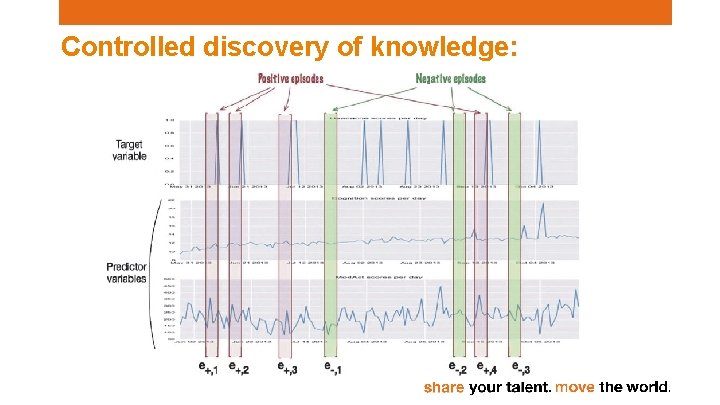
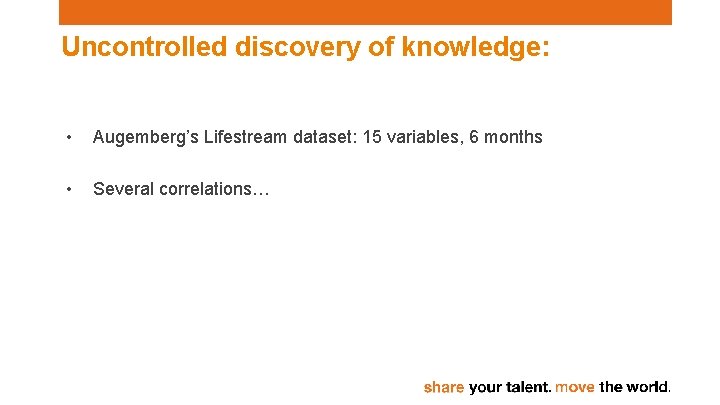
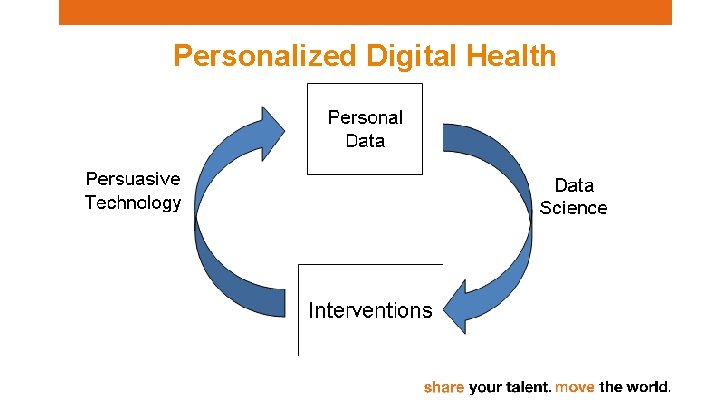
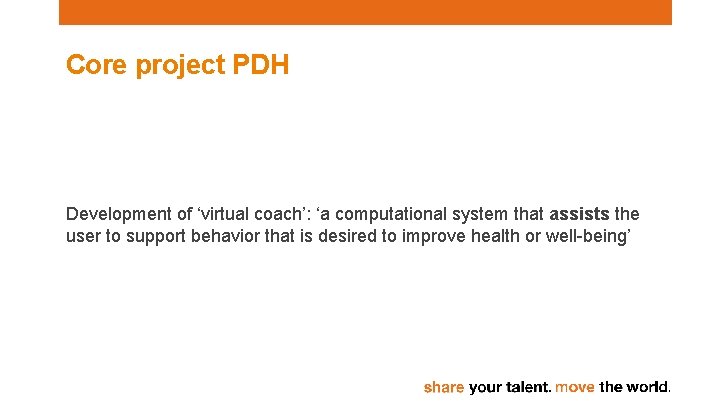
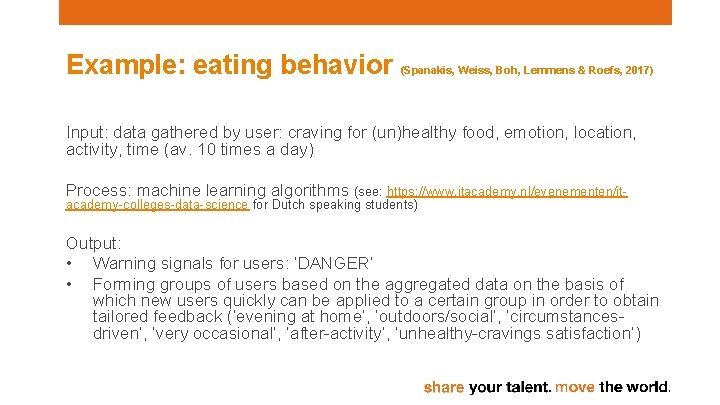
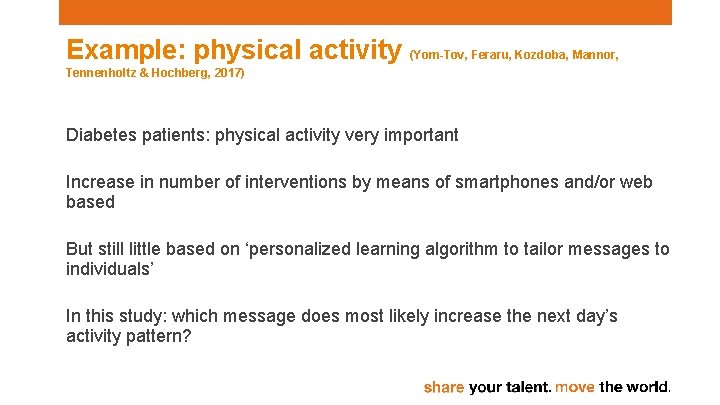
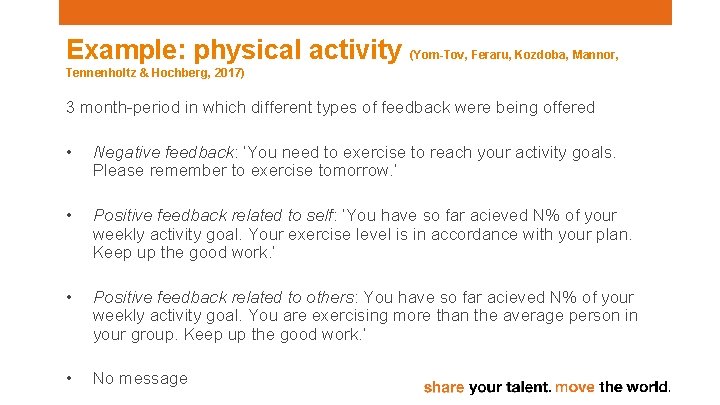
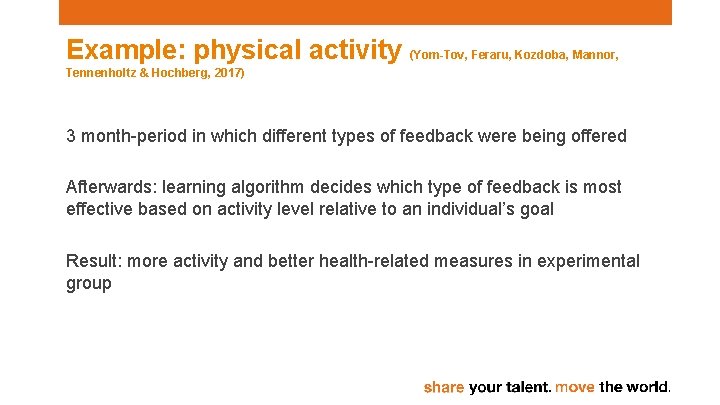
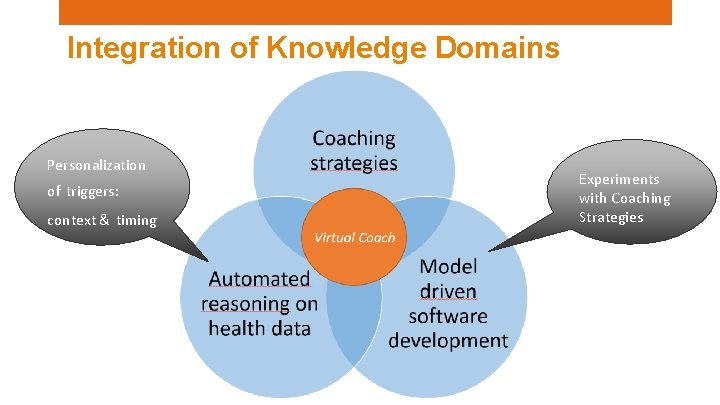
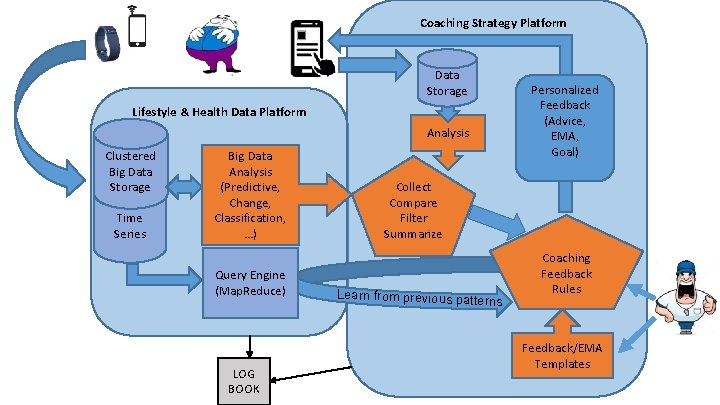
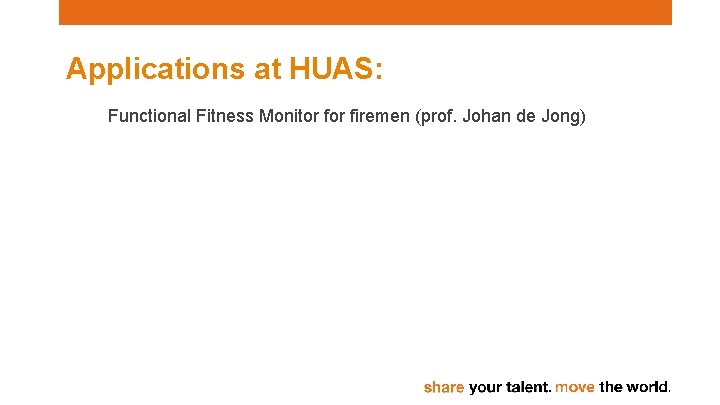
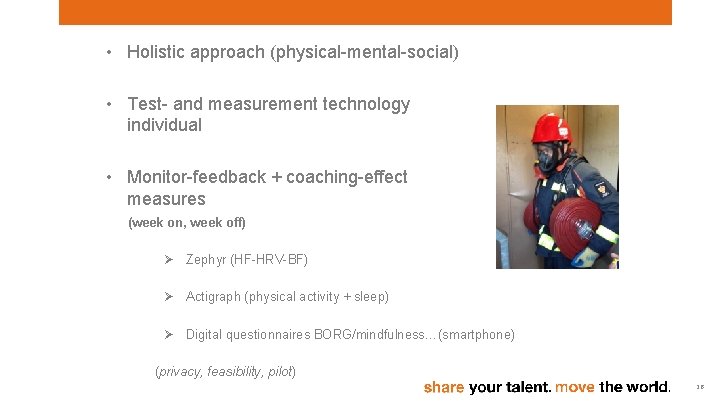
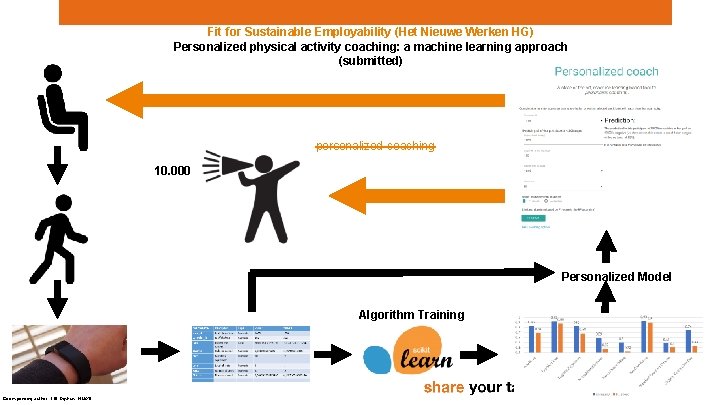
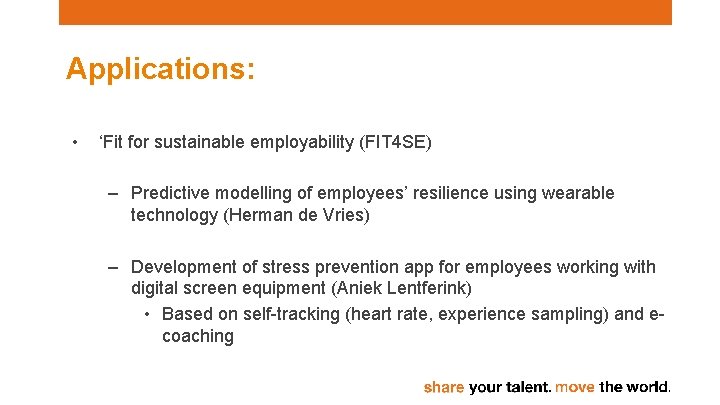
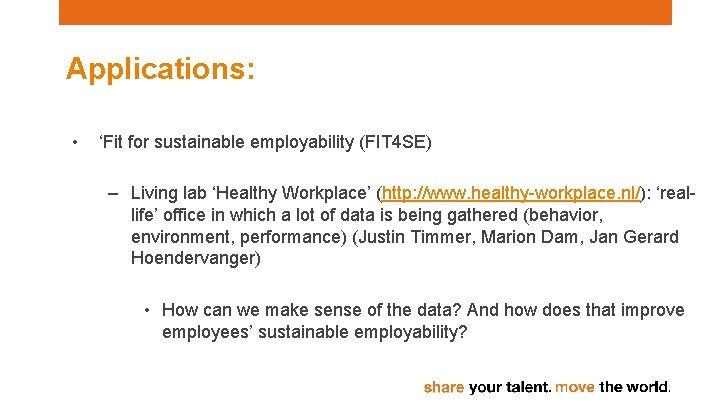
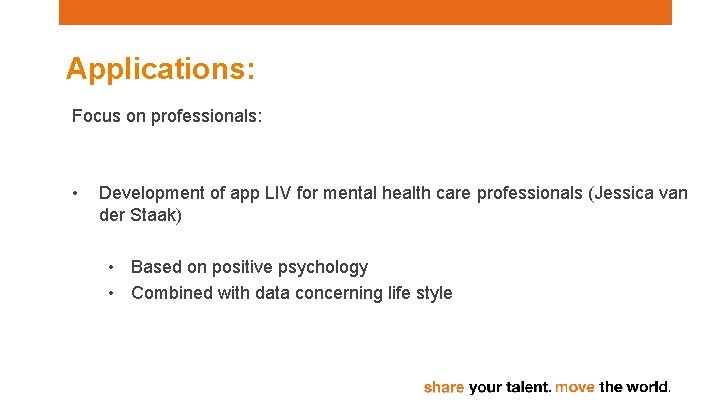
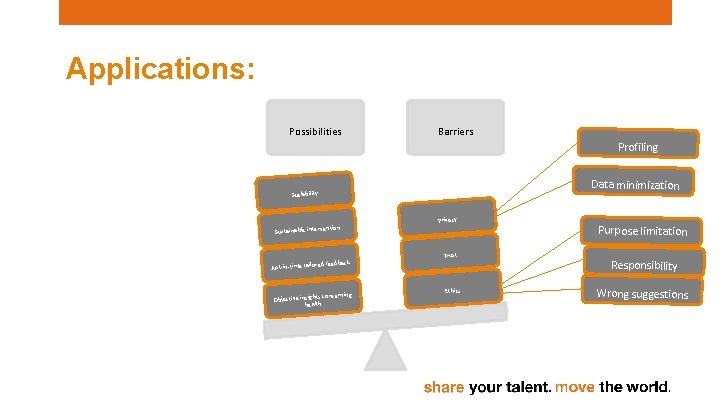
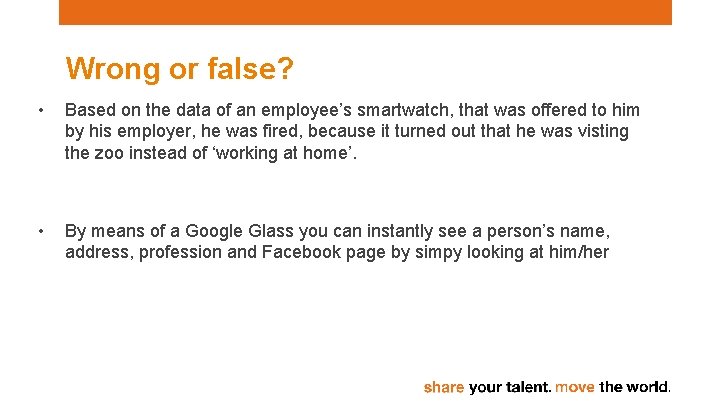
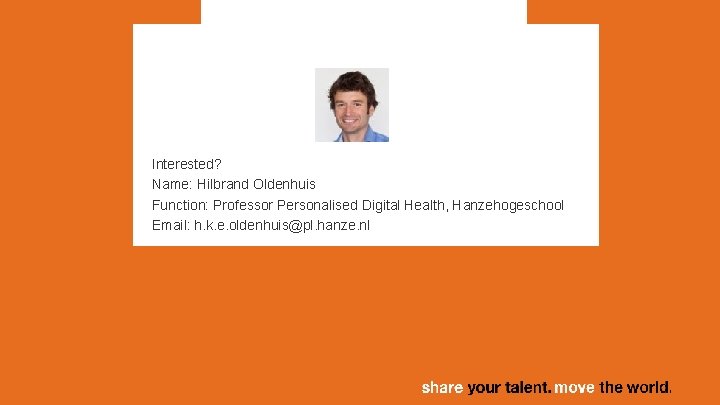
- Slides: 33
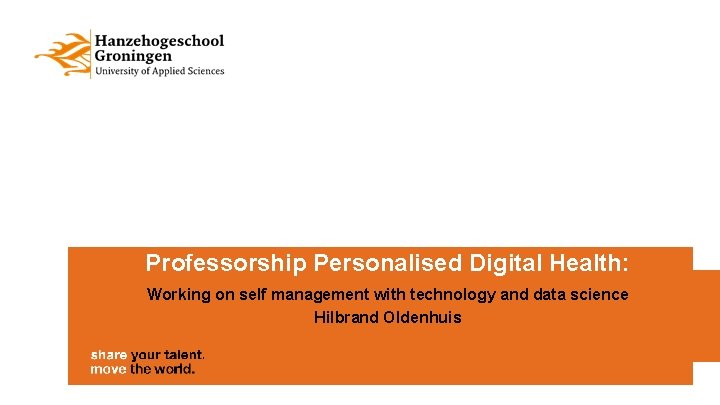
Professorship Personalised Digital Health: Working on self management with technology and data science Hilbrand Oldenhuis
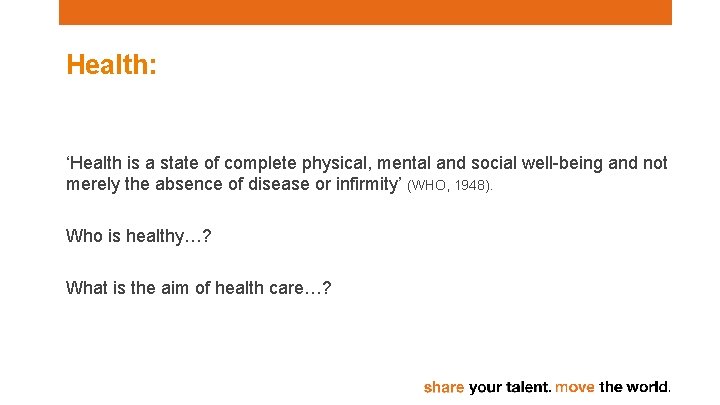
Health: ‘Health is a state of complete physical, mental and social well-being and not merely the absence of disease or infirmity’ (WHO, 1948). Who is healthy…? What is the aim of health care…?
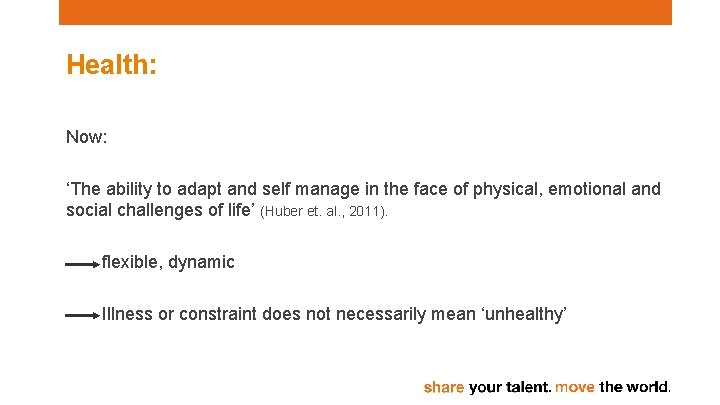
Health: Now: ‘The ability to adapt and self manage in the face of physical, emotional and social challenges of life’ (Huber et. al. , 2011). flexible, dynamic Illness or constraint does not necessarily mean ‘unhealthy’
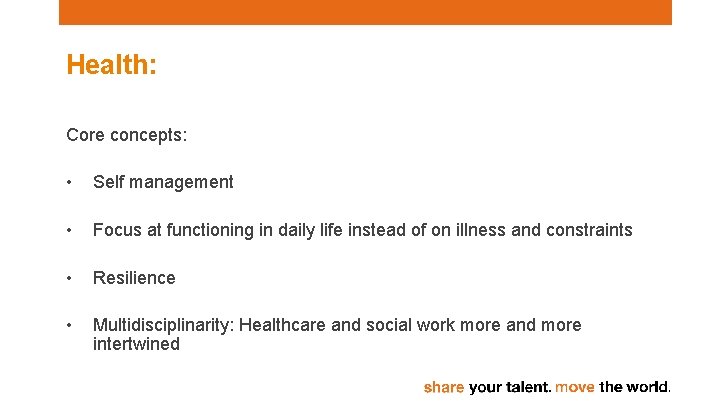
Health: Core concepts: • Self management • Focus at functioning in daily life instead of on illness and constraints • Resilience • Multidisciplinarity: Healthcare and social work more and more intertwined
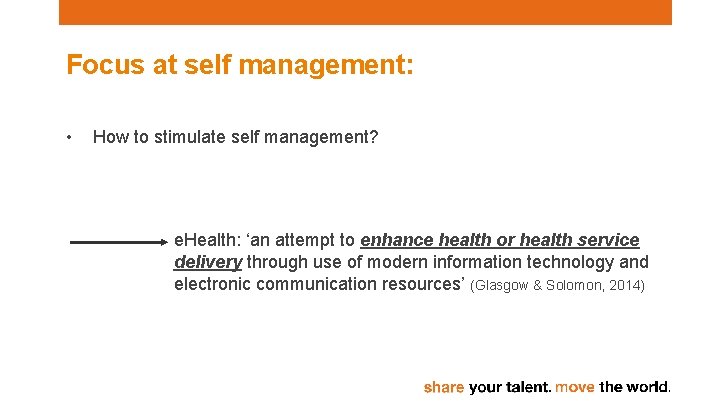
Focus at self management: • How to stimulate self management? e. Health: ‘an attempt to enhance health or health service delivery through use of modern information technology and electronic communication resources’ (Glasgow & Solomon, 2014)
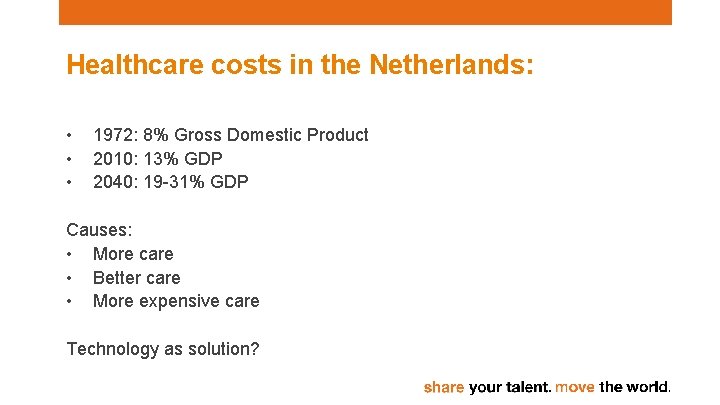
Healthcare costs in the Netherlands: • • • 1972: 8% Gross Domestic Product 2010: 13% GDP 2040: 19 -31% GDP Causes: • More care • Better care • More expensive care Technology as solution?
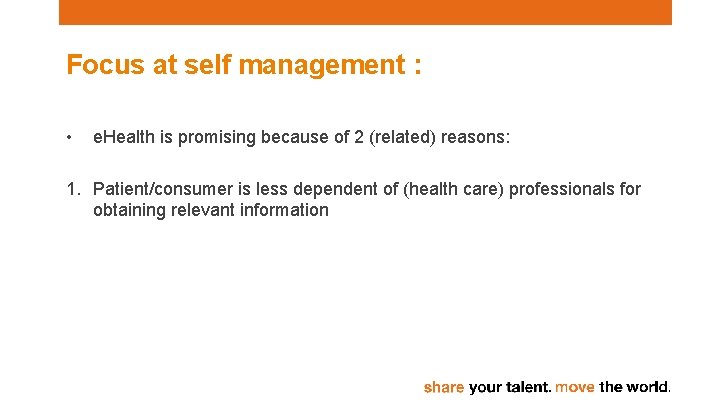
Focus at self management : • e. Health is promising because of 2 (related) reasons: 1. Patient/consumer is less dependent of (health care) professionals for obtaining relevant information
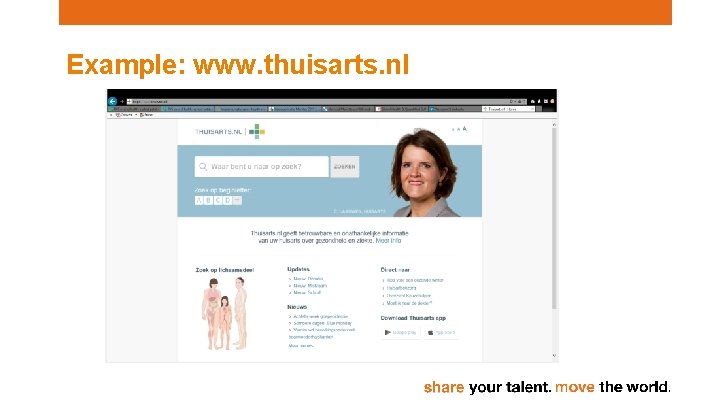
Example: www. thuisarts. nl
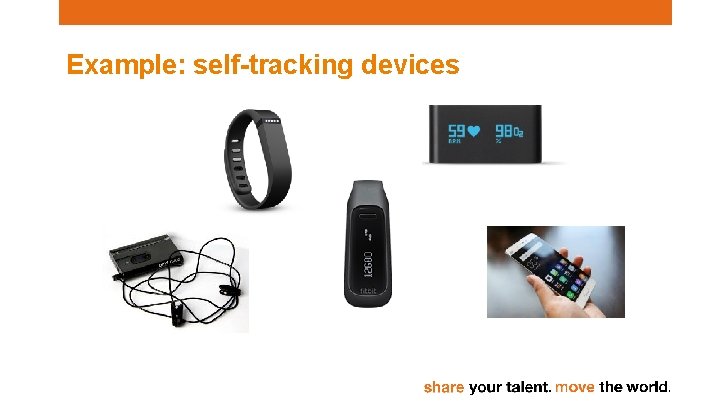
Example: self-tracking devices
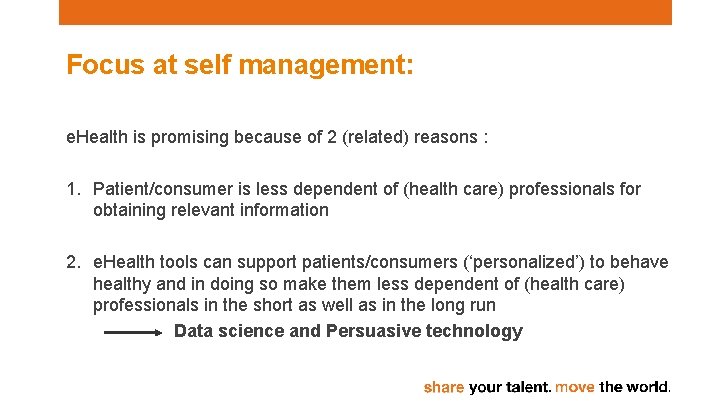
Focus at self management: e. Health is promising because of 2 (related) reasons : 1. Patient/consumer is less dependent of (health care) professionals for obtaining relevant information 2. e. Health tools can support patients/consumers (‘personalized’) to behave healthy and in doing so make them less dependent of (health care) professionals in the short as well as in the long run Data science and Persuasive technology
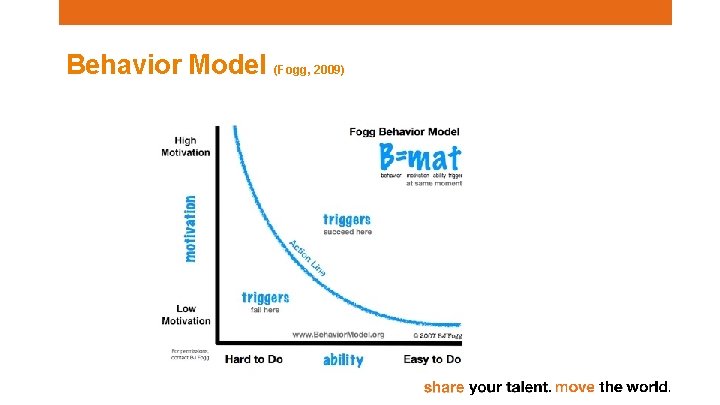
Behavior Model (Fogg, 2009)
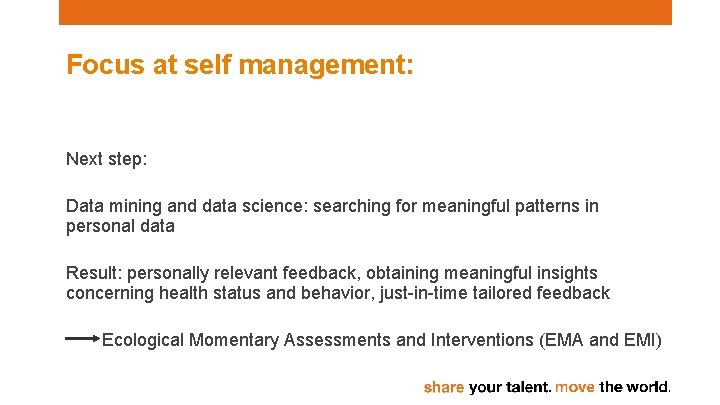
Focus at self management: Next step: Data mining and data science: searching for meaningful patterns in personal data Result: personally relevant feedback, obtaining meaningful insights concerning health status and behavior, just-in-time tailored feedback Ecological Momentary Assessments and Interventions (EMA and EMI)
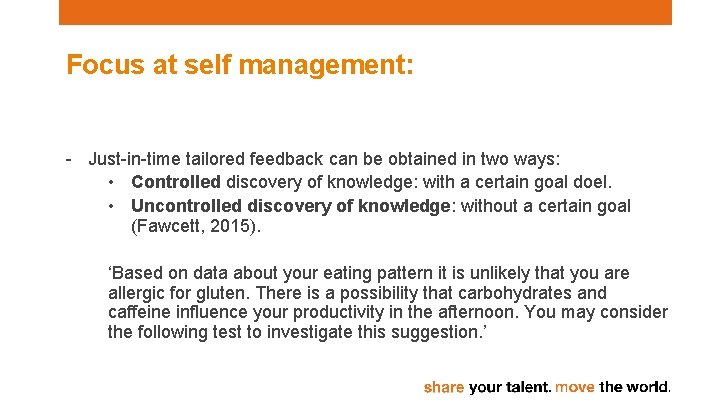
Focus at self management: - Just-in-time tailored feedback can be obtained in two ways: • Controlled discovery of knowledge: with a certain goal doel. • Uncontrolled discovery of knowledge: without a certain goal (Fawcett, 2015). ‘Based on data about your eating pattern it is unlikely that you are allergic for gluten. There is a possibility that carbohydrates and caffeine influence your productivity in the afternoon. You may consider the following test to investigate this suggestion. ’
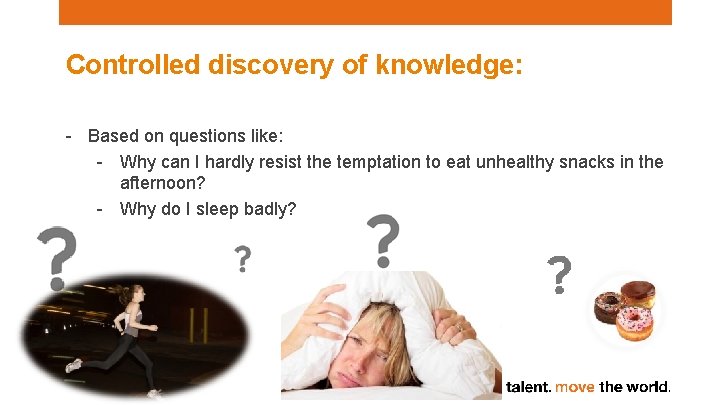
Controlled discovery of knowledge: - Based on questions like: - Why can I hardly resist the temptation to eat unhealthy snacks in the afternoon? - Why do I sleep badly?
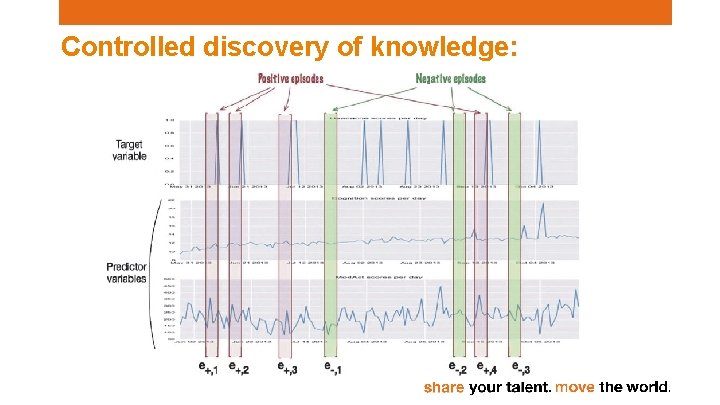
Controlled discovery of knowledge:
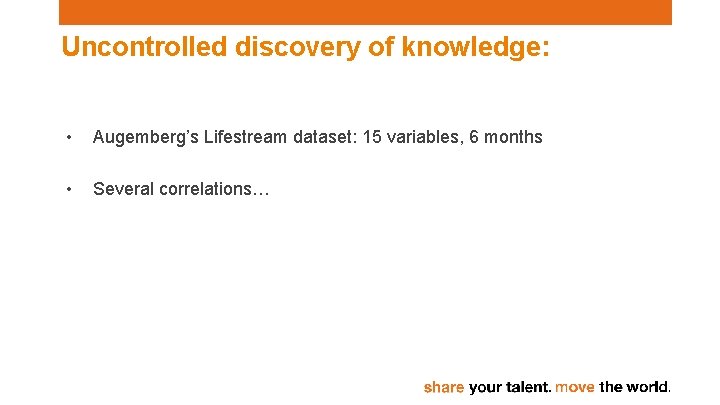
Uncontrolled discovery of knowledge: • Augemberg’s Lifestream dataset: 15 variables, 6 months • Several correlations…
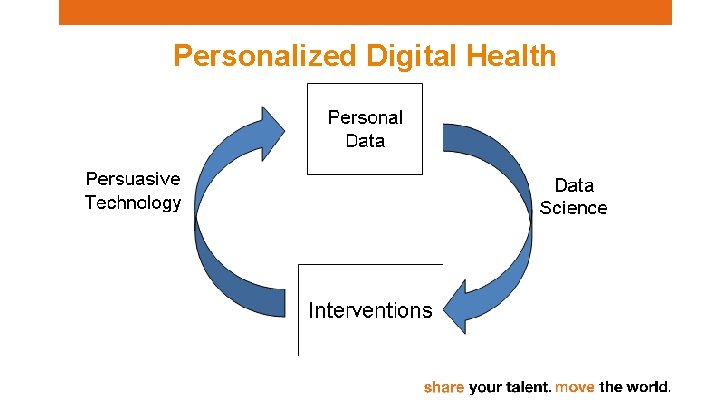
Personalized Digital Health
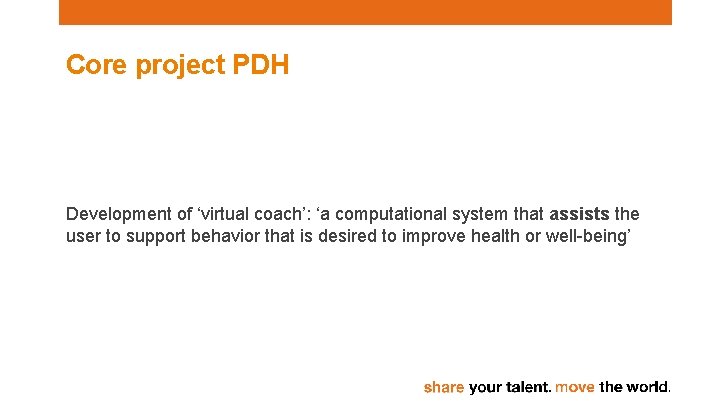
Core project PDH Development of ‘virtual coach’: ‘a computational system that assists the user to support behavior that is desired to improve health or well-being’
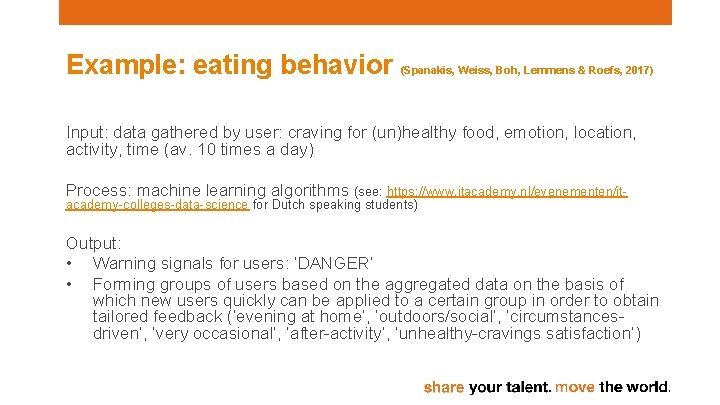
Example: eating behavior (Spanakis, Weiss, Boh, Lemmens & Roefs, 2017) Input: data gathered by user: craving for (un)healthy food, emotion, location, activity, time (av. 10 times a day) Process: machine learning algorithms (see: https: //www. itacademy. nl/evenementen/itacademy-colleges-data-science for Dutch speaking students) Output: • Warning signals for users: ‘DANGER’ • Forming groups of users based on the aggregated data on the basis of which new users quickly can be applied to a certain group in order to obtain tailored feedback (‘evening at home’, ‘outdoors/social’, ‘circumstancesdriven’, ‘very occasional’, ‘after-activity’, ‘unhealthy-cravings satisfaction’)
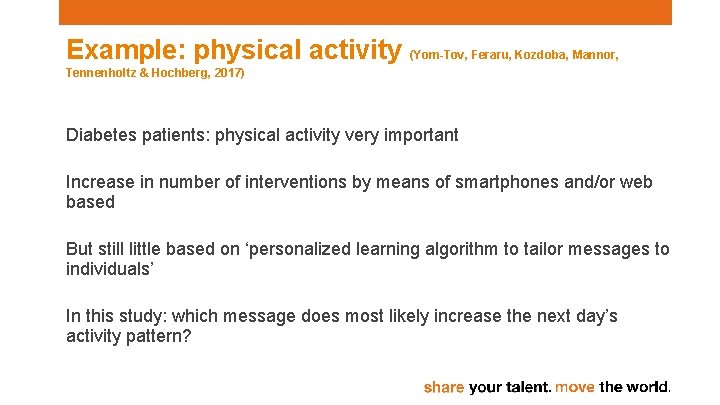
Example: physical activity (Yom-Tov, Feraru, Kozdoba, Mannor, Tennenholtz & Hochberg, 2017) Diabetes patients: physical activity very important Increase in number of interventions by means of smartphones and/or web based But still little based on ‘personalized learning algorithm to tailor messages to individuals’ In this study: which message does most likely increase the next day’s activity pattern?
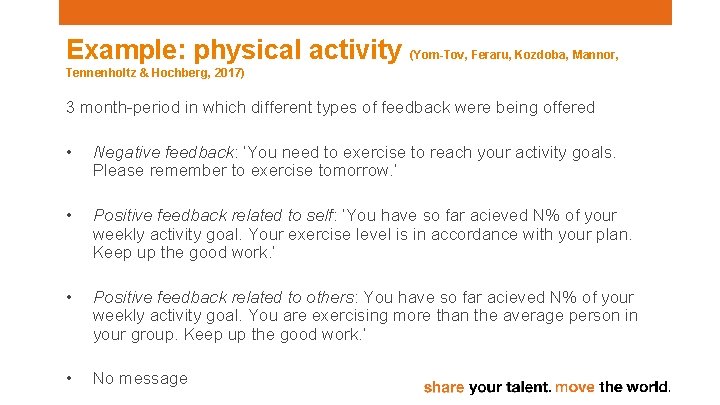
Example: physical activity (Yom-Tov, Feraru, Kozdoba, Mannor, Tennenholtz & Hochberg, 2017) 3 month-period in which different types of feedback were being offered • Negative feedback: ‘You need to exercise to reach your activity goals. Please remember to exercise tomorrow. ’ • Positive feedback related to self: ‘You have so far acieved N% of your weekly activity goal. Your exercise level is in accordance with your plan. Keep up the good work. ’ • Positive feedback related to others: You have so far acieved N% of your weekly activity goal. You are exercising more than the average person in your group. Keep up the good work. ’ • No message
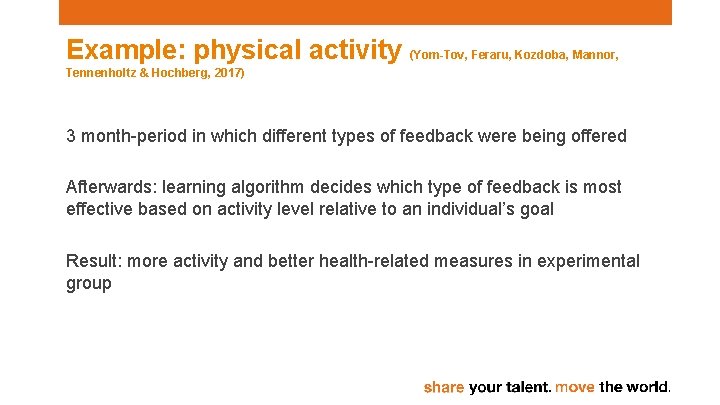
Example: physical activity (Yom-Tov, Feraru, Kozdoba, Mannor, Tennenholtz & Hochberg, 2017) 3 month-period in which different types of feedback were being offered Afterwards: learning algorithm decides which type of feedback is most effective based on activity level relative to an individual’s goal Result: more activity and better health-related measures in experimental group
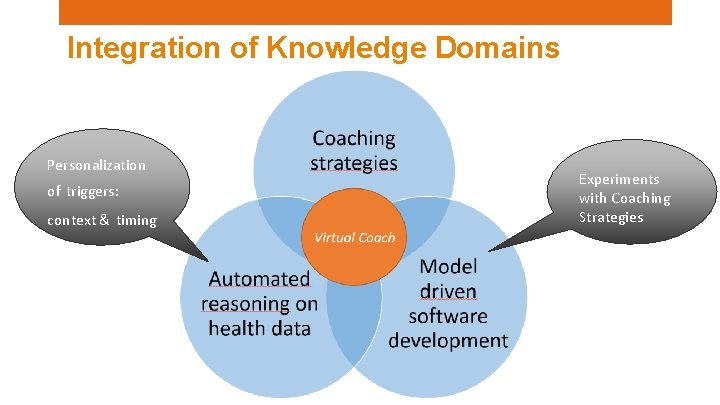
Integration of Knowledge Domains Personalization of triggers: context & timing Experiments with Coaching Strategies
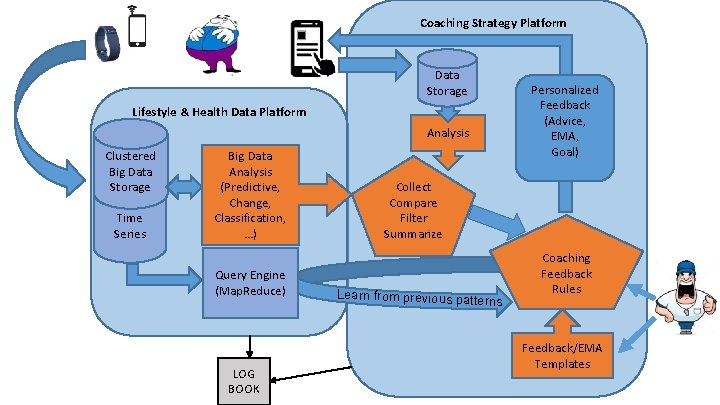
Coaching Strategy Platform Data Storage Personalized Feedback (Advice, EMA, Goal) Lifestyle & Health Data Platform Analysis Clustered Big Data Storage Time Series Big Data Analysis (Predictive, Change, Classification, …) Query Engine (Map. Reduce) LOG BOOK Collect Compare Filter Summarize Learn from previous patte rns Coaching Feedback Rules Feedback/EMA Templates
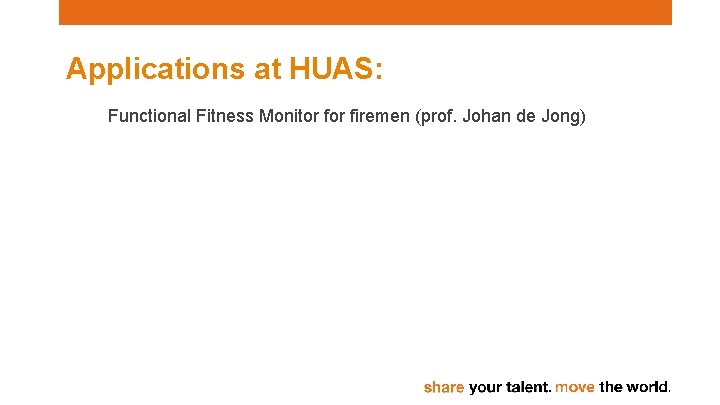
Applications at HUAS: Functional Fitness Monitor firemen (prof. Johan de Jong)
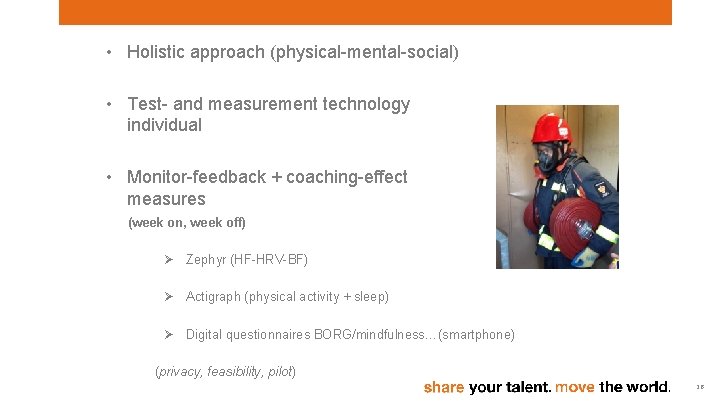
• Holistic approach (physical-mental-social) • Test- and measurement technology individual • Monitor-feedback + coaching-effect measures (week on, week off) Ø Zephyr (HF-HRV-BF) Ø Actigraph (physical activity + sleep) Ø Digital questionnaires BORG/mindfulness…(smartphone) (privacy, feasibility, pilot) 26
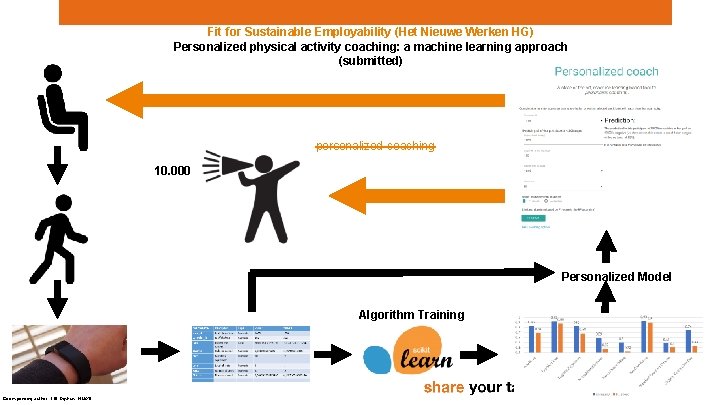
Fit for Sustainable Employability (Het Nieuwe Werken HG) Personalized physical activity coaching: a machine learning approach (submitted) personalized-coaching 10. 000 Personalized Model Algorithm Training Corresponding author: T. B. Dijkhuis, HUAS
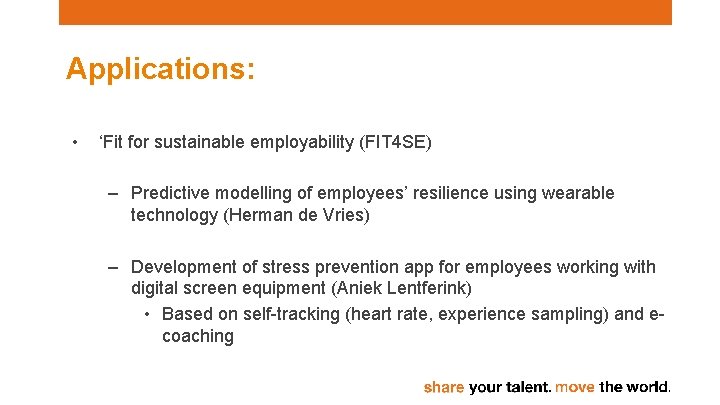
Applications: • ‘Fit for sustainable employability (FIT 4 SE) – Predictive modelling of employees’ resilience using wearable technology (Herman de Vries) – Development of stress prevention app for employees working with digital screen equipment (Aniek Lentferink) • Based on self-tracking (heart rate, experience sampling) and ecoaching
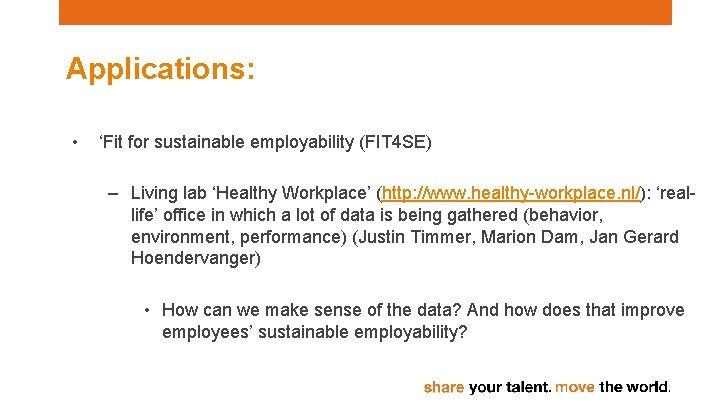
Applications: • ‘Fit for sustainable employability (FIT 4 SE) – Living lab ‘Healthy Workplace’ (http: //www. healthy-workplace. nl/): ‘reallife’ office in which a lot of data is being gathered (behavior, environment, performance) (Justin Timmer, Marion Dam, Jan Gerard Hoendervanger) • How can we make sense of the data? And how does that improve employees’ sustainable employability?
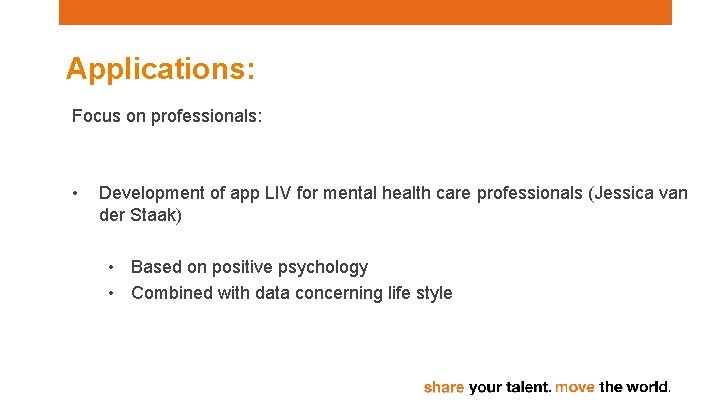
Applications: Focus on professionals: • Development of app LIV for mental health care professionals (Jessica van der Staak) • Based on positive psychology • Combined with data concerning life style
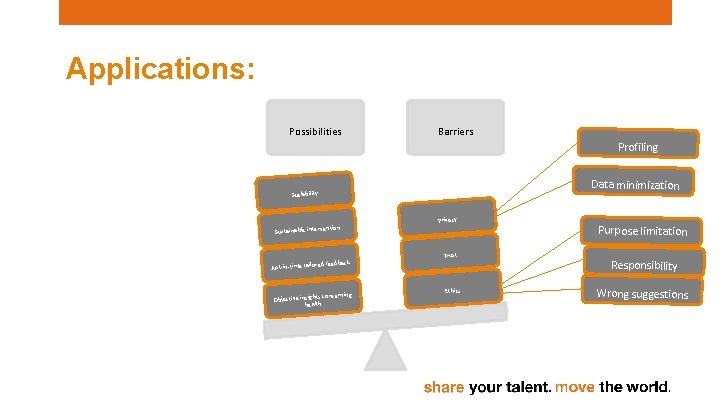
Applications: Possibilities Barriers Profiling Data minimization Scalability ention Sustainable interv d feedback Just-in-time tailore Objective insights health concerning Privacy Trust Ethics Purpose limitation Responsibility Wrong suggestions
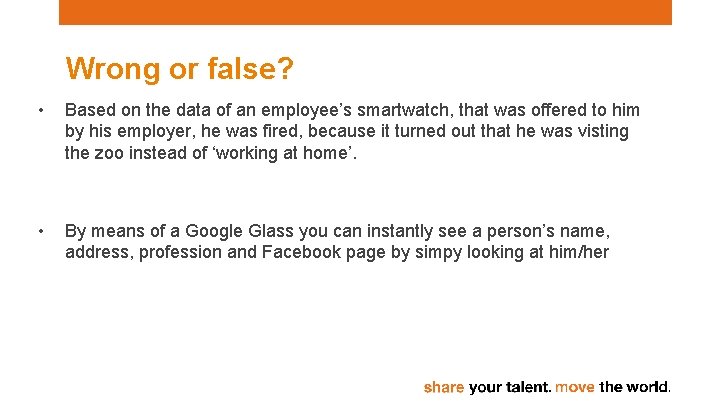
Wrong or false? • Based on the data of an employee’s smartwatch, that was offered to him by his employer, he was fired, because it turned out that he was visting the zoo instead of ‘working at home’. • By means of a Google Glass you can instantly see a person’s name, address, profession and Facebook page by simpy looking at him/her
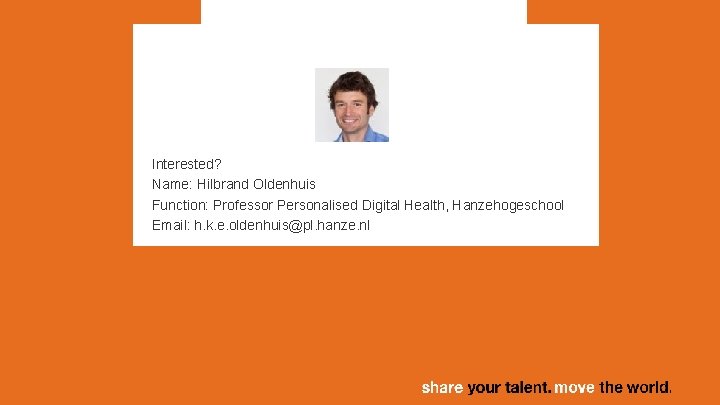
Interested? Name: Hilbrand Oldenhuis Function: Professor Personalised Digital Health, Hanzehogeschool Email: h. k. e. oldenhuis@pl. hanze. nl
Prof mark ferguson
Personalised mobile search engine
Comprehensive model of personalised care
Comprehensive personalised care model
Comprehensive model of personalised care
Personalised fortification
General motors
James sanderson nhs
Real self vs ideal self poster
Smart vs hard working
Hot and cold working process
Hot working and cold working difference
Differentiate between hot working and cold working
Prinsip dasar proses pengerjaan panas yang benar adalah
Spectrum/self install.net
Pfms java settings
Digital light processing introduction
Health and social care unit 2
Unit 2 working in health and social care
Ashwg
Self image vs self perception
Self concept vs self esteem
Self concept vs self esteem
Self concept vs self esteem
An idealized image that we have developed over time
I self and me self difference
Contoh ideal self dan real self
Procedural self-knowledge includes ________.
Eyfs self confidence and self awareness
Me myself and i self concept
Konsep warga digital
Unique features of digital markets
Digital data digital signals
Data encoding and transmission