Overview Contrast in f MRI v contrast in
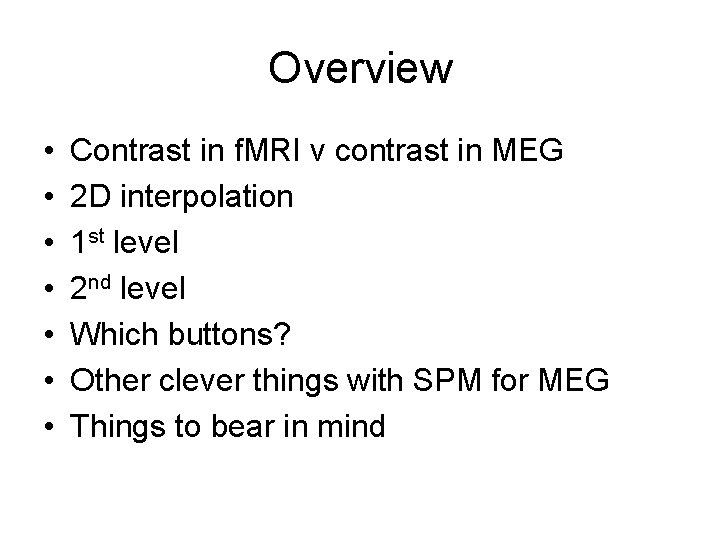
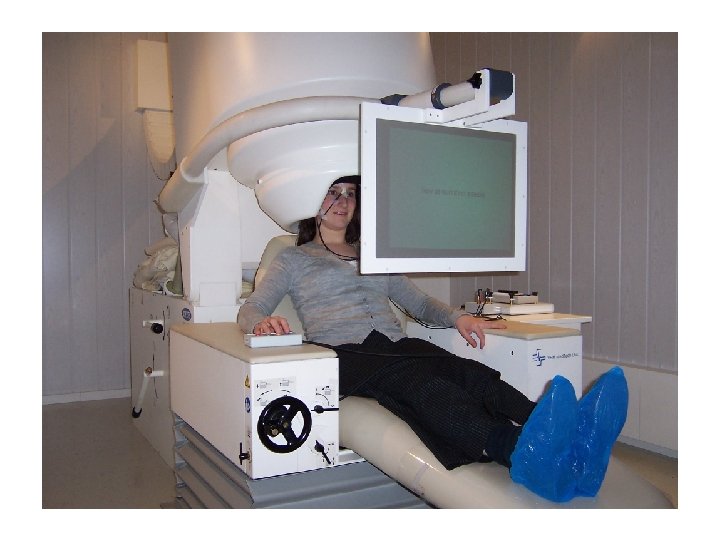
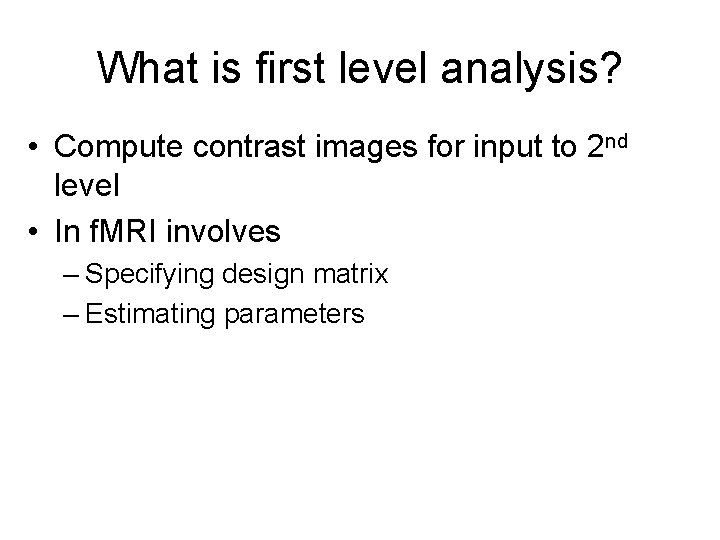
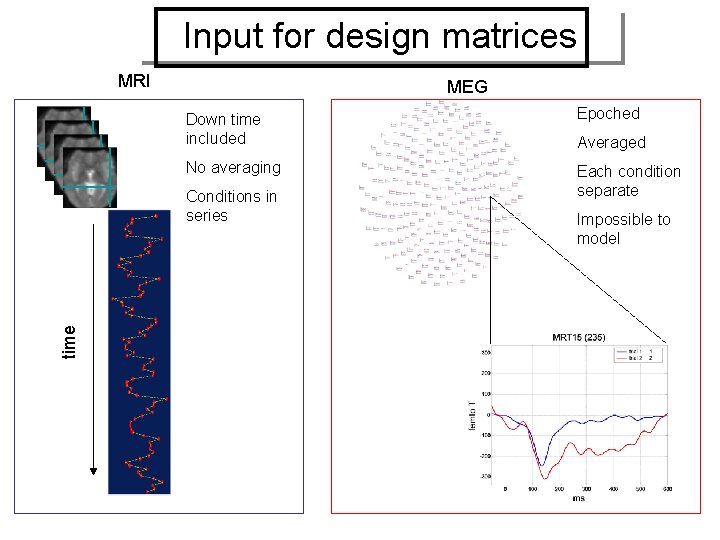
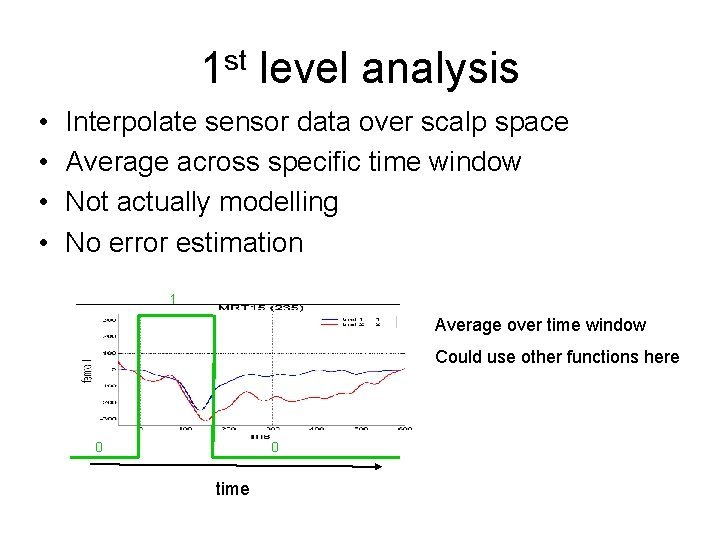
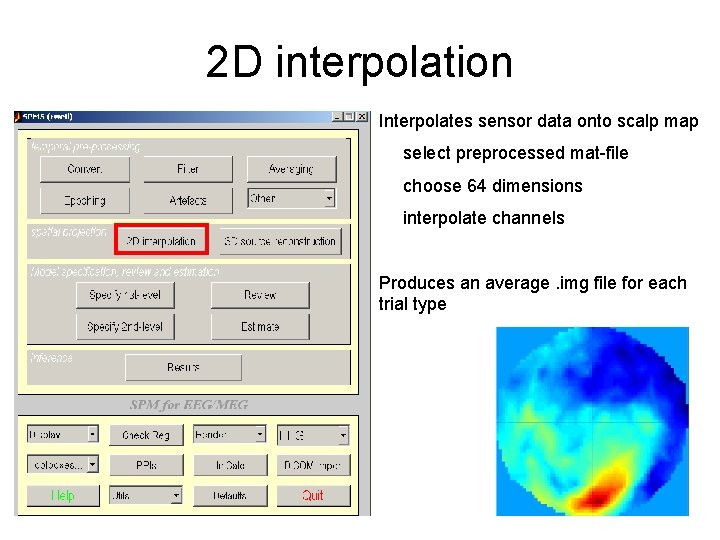
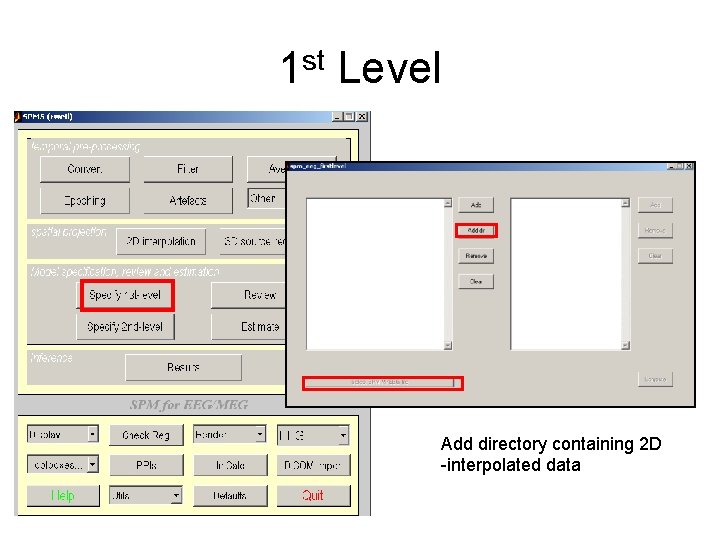
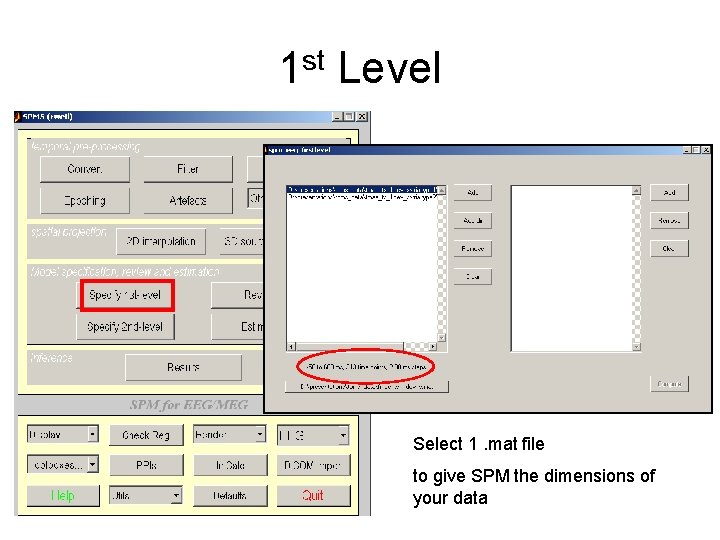
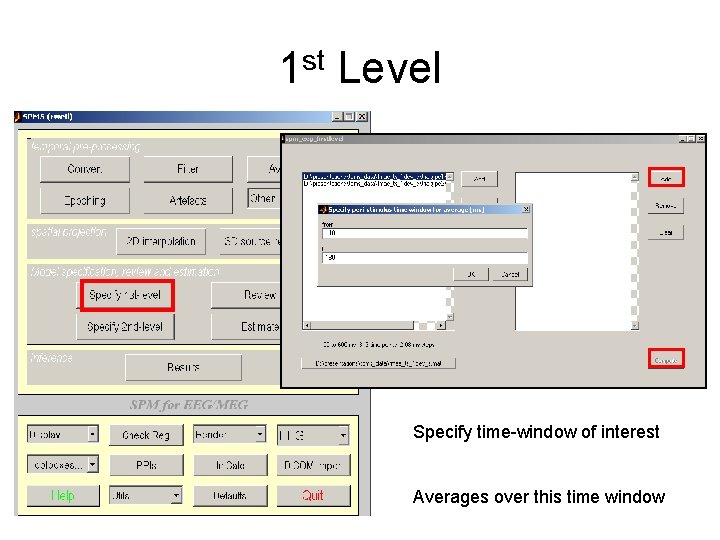
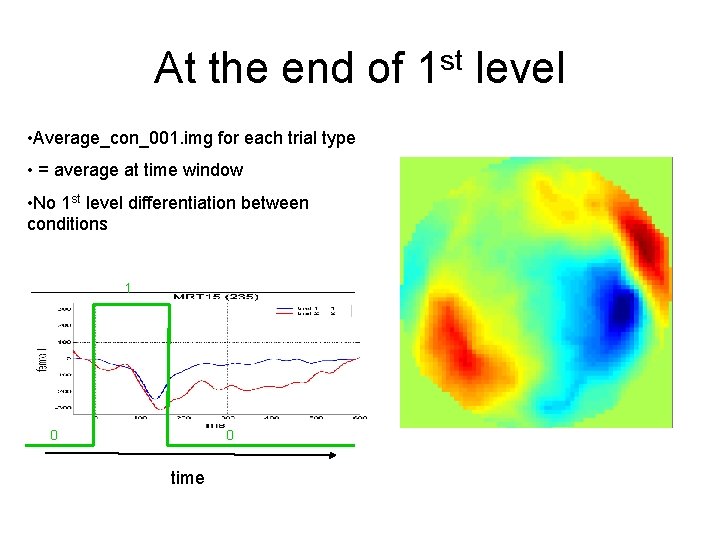
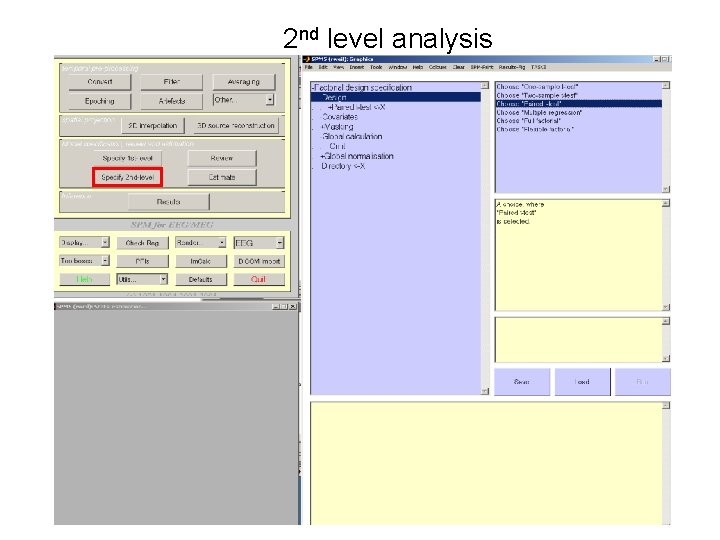
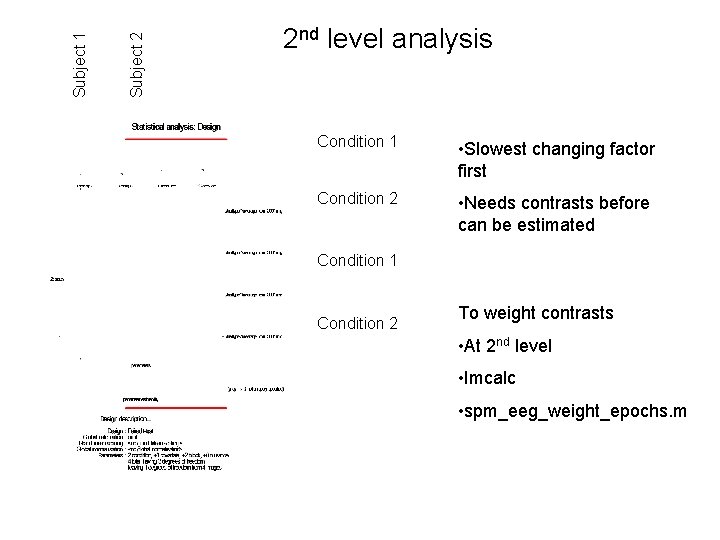
![2 nd level analysis Contrast vector = [0 0 1 -1] 2 nd level analysis Contrast vector = [0 0 1 -1]](https://slidetodoc.com/presentation_image_h2/3d82bdd7b0b2935553a58b53f3653adf/image-13.jpg)
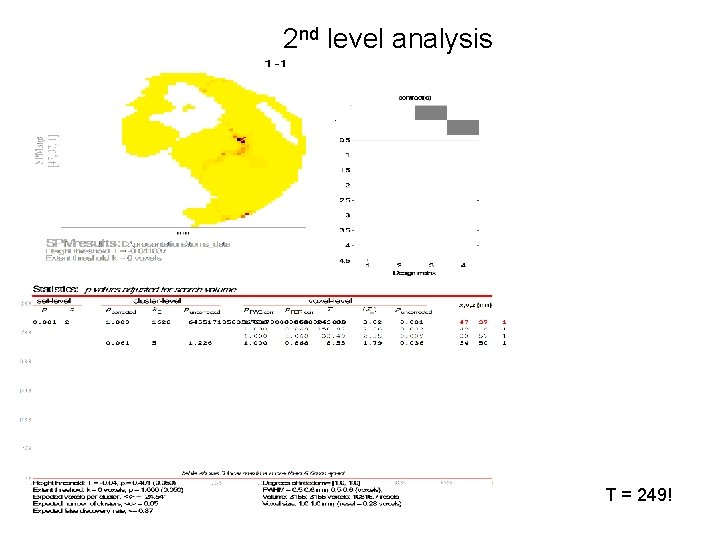
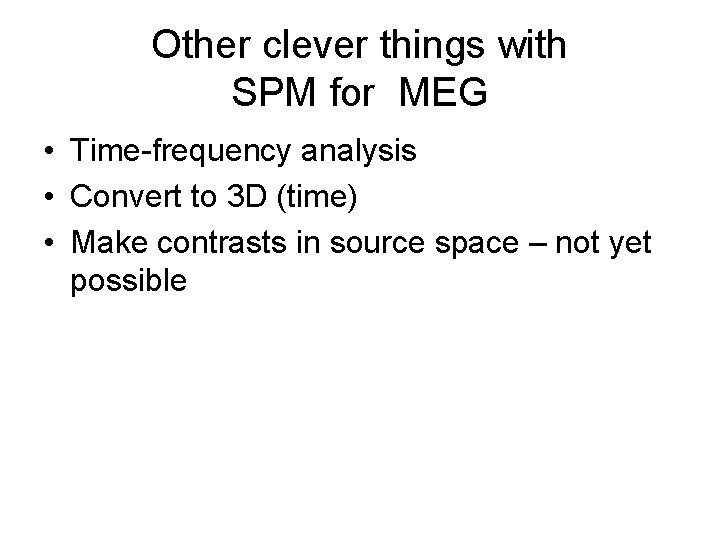
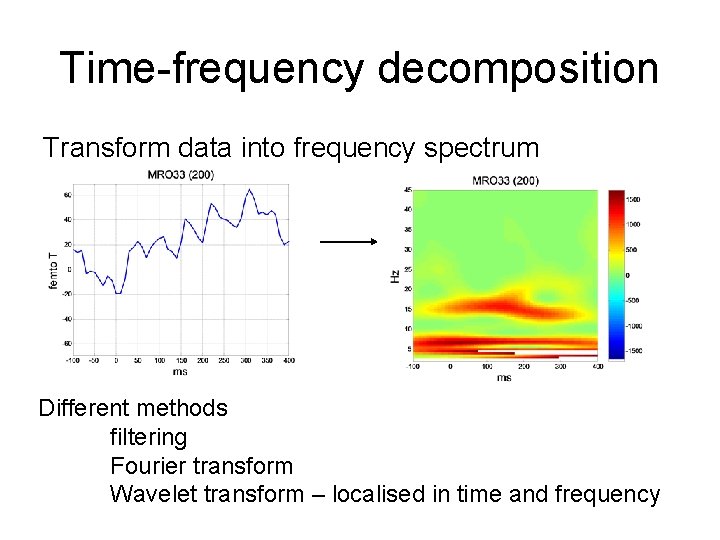
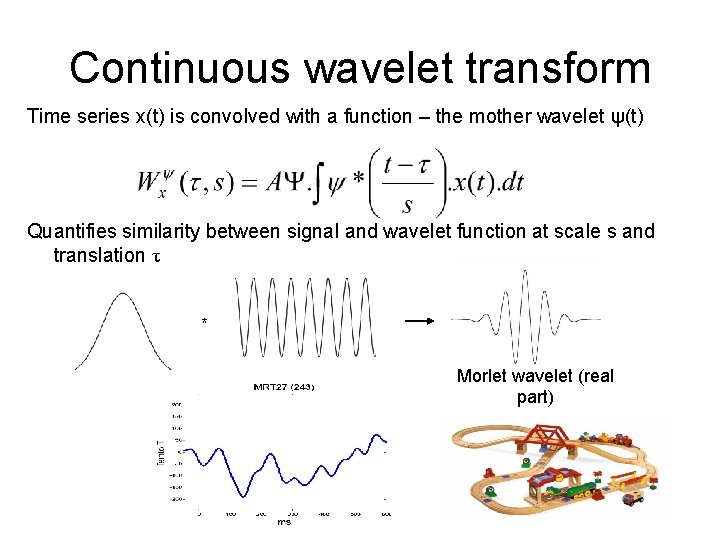
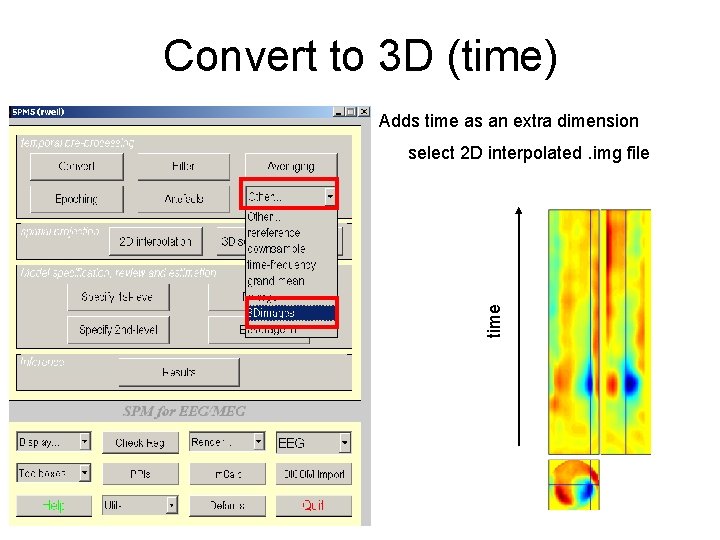
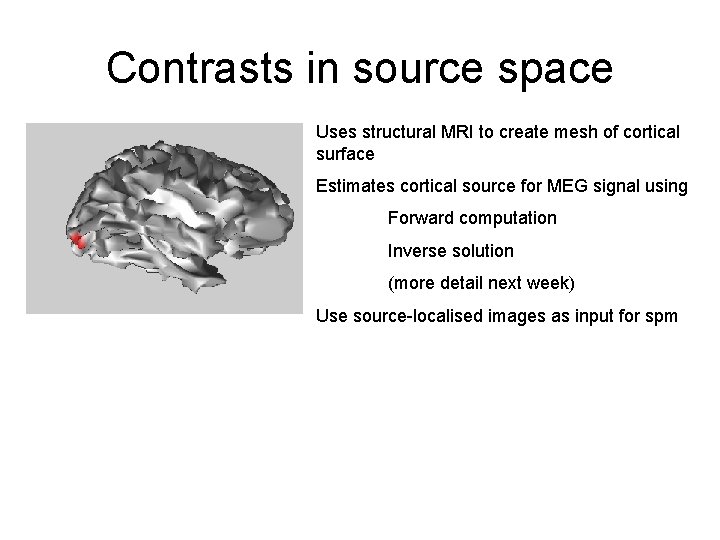
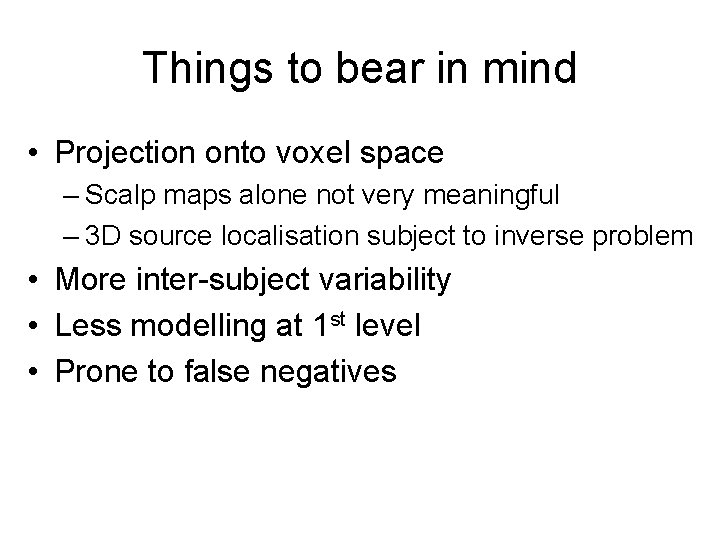
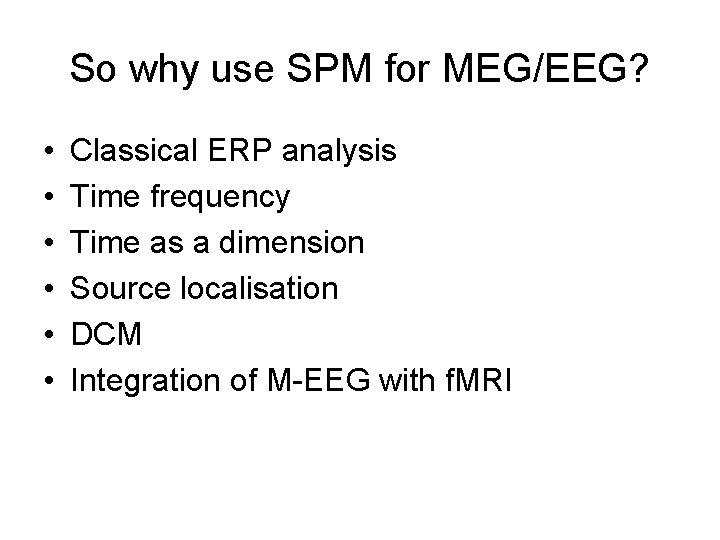
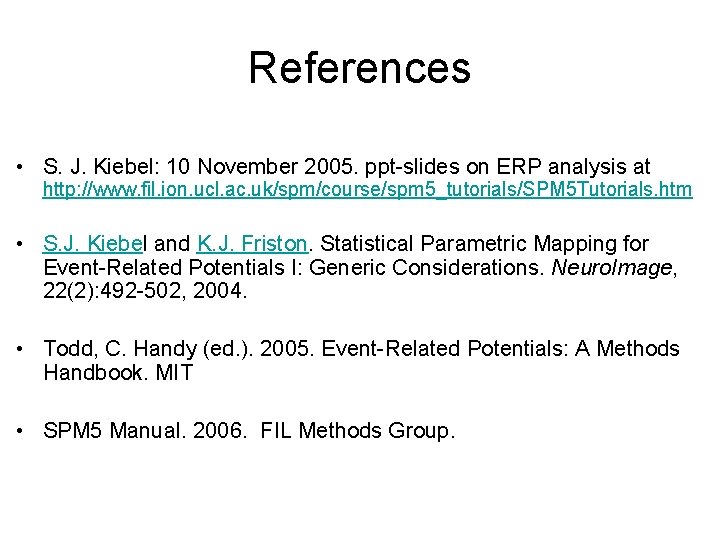
- Slides: 22
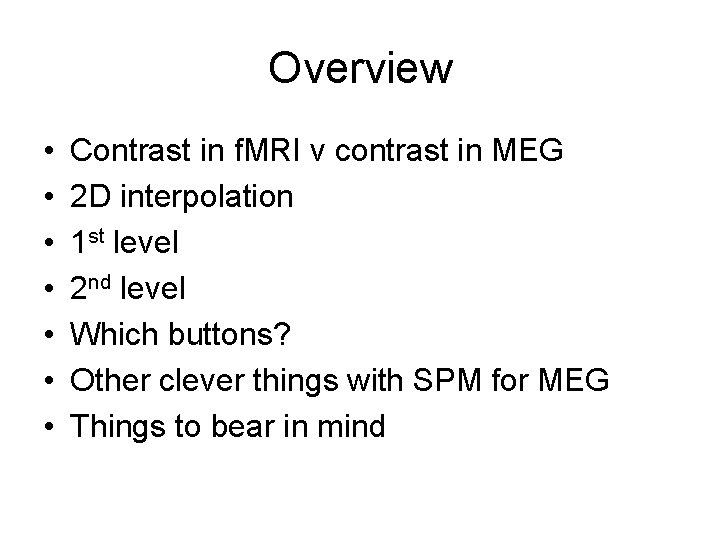
Overview • • Contrast in f. MRI v contrast in MEG 2 D interpolation 1 st level 2 nd level Which buttons? Other clever things with SPM for MEG Things to bear in mind
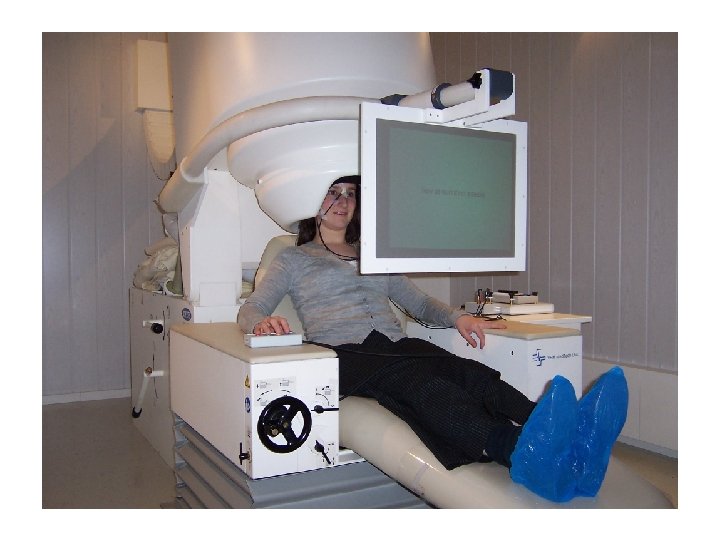
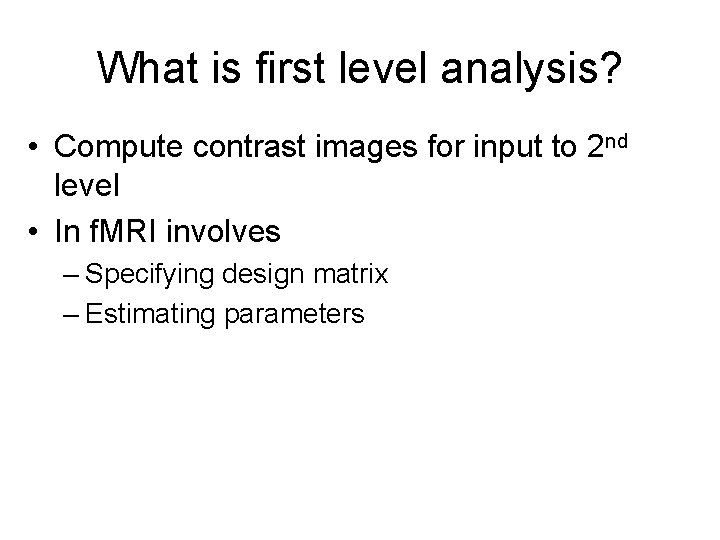
What is first level analysis? • Compute contrast images for input to 2 nd level • In f. MRI involves – Specifying design matrix – Estimating parameters
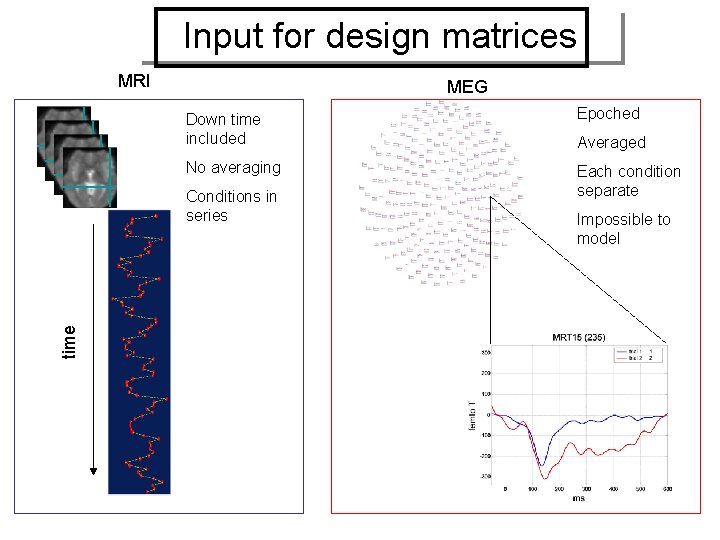
Input for design matrices MRI MEG Down time included Epoched No averaging Each condition separate time Conditions in series Averaged Impossible to model
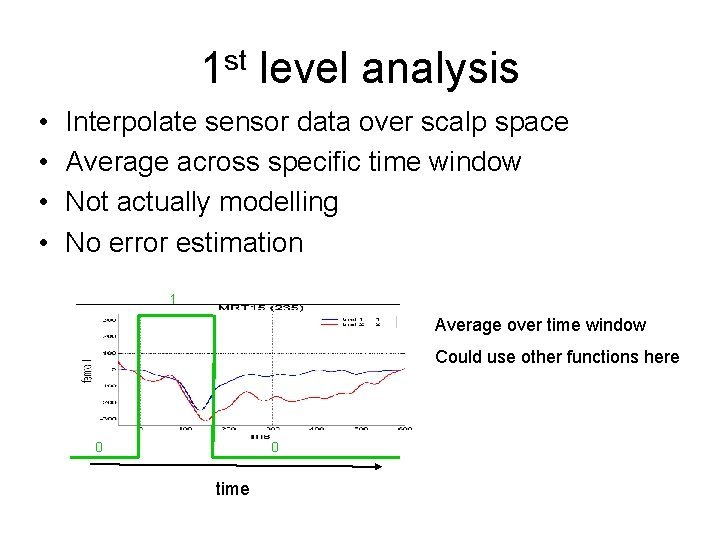
1 st level analysis • • Interpolate sensor data over scalp space Average across specific time window Not actually modelling No error estimation 1 Average over time window Could use other functions here 0 0 time
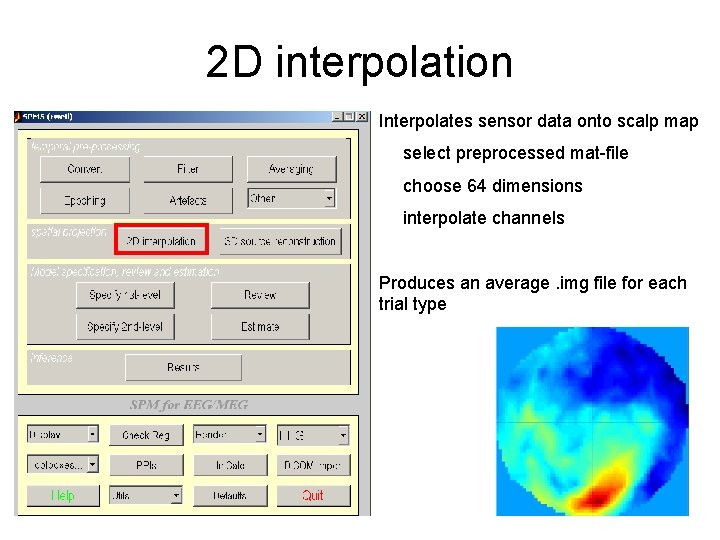
2 D interpolation Interpolates sensor data onto scalp map select preprocessed mat-file choose 64 dimensions interpolate channels Produces an average. img file for each trial type
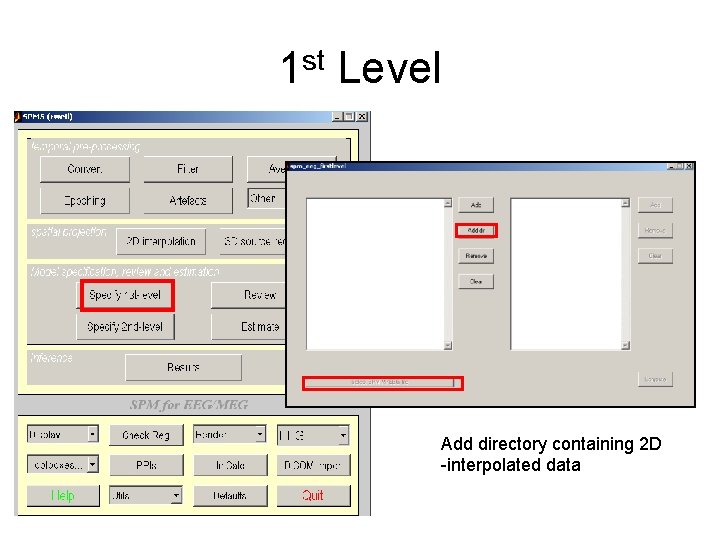
1 st Level Add directory containing 2 D -interpolated data
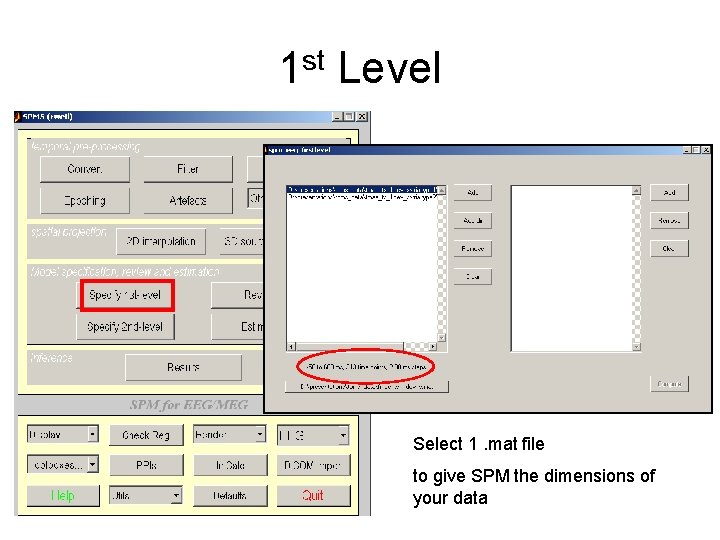
1 st Level Select 1. mat file to give SPM the dimensions of your data
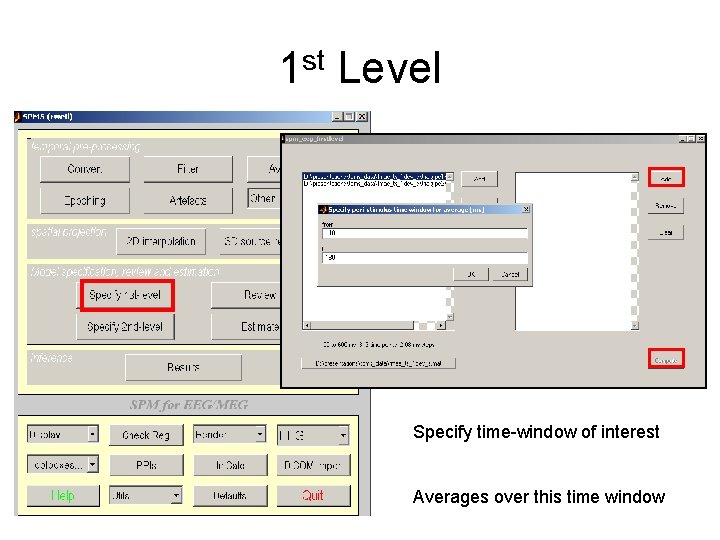
1 st Level Specify time-window of interest Averages over this time window
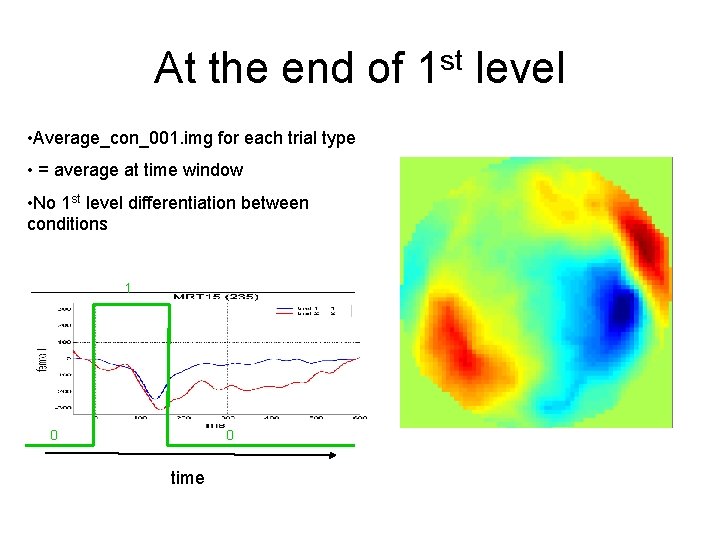
At the end of 1 st level • Average_con_001. img for each trial type • = average at time window • No 1 st level differentiation between conditions 1 0 0 time
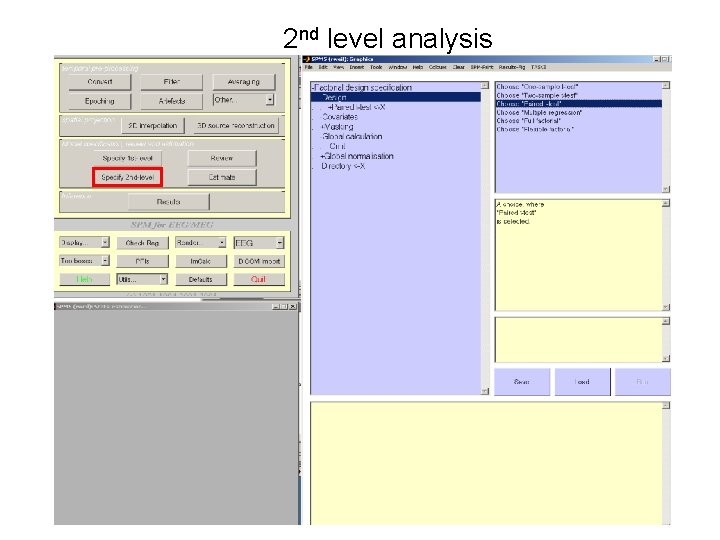
2 nd level analysis
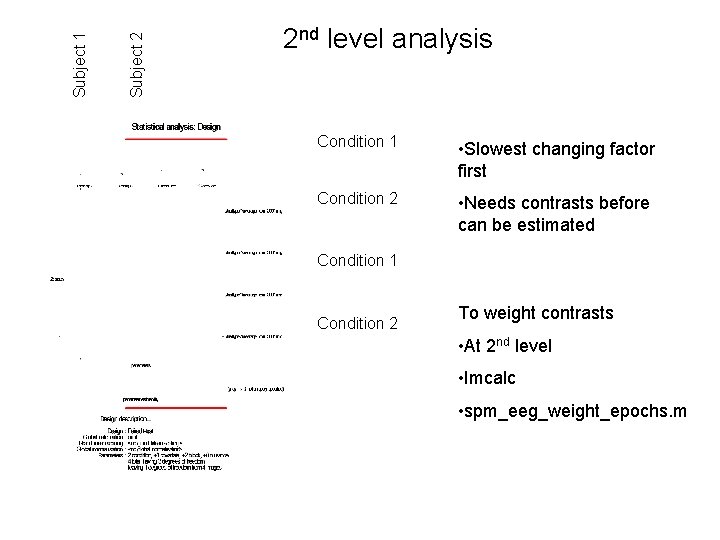
Subject 2 Subject 1 2 nd level analysis Condition 1 • Slowest changing factor first Condition 2 • Needs contrasts before can be estimated Condition 1 Condition 2 To weight contrasts • At 2 nd level • Imcalc • spm_eeg_weight_epochs. m
![2 nd level analysis Contrast vector 0 0 1 1 2 nd level analysis Contrast vector = [0 0 1 -1]](https://slidetodoc.com/presentation_image_h2/3d82bdd7b0b2935553a58b53f3653adf/image-13.jpg)
2 nd level analysis Contrast vector = [0 0 1 -1]
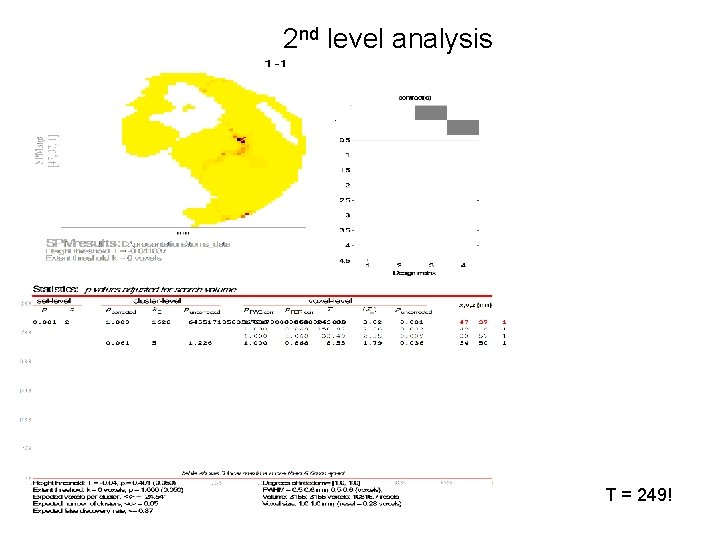
2 nd level analysis T = 249!
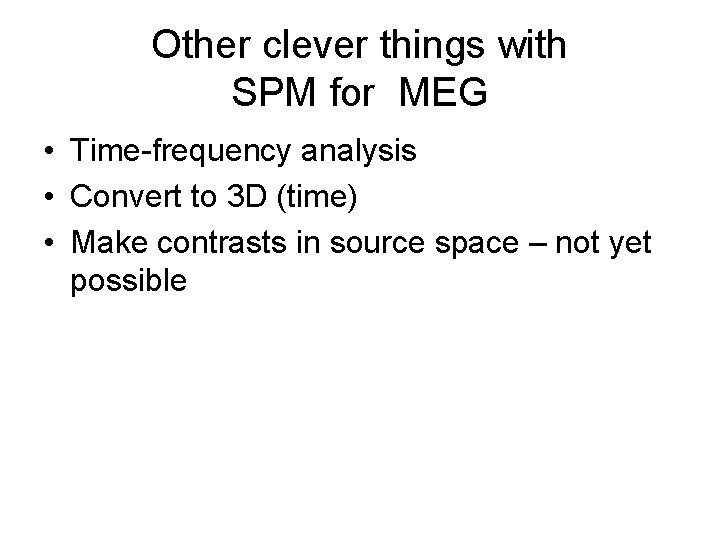
Other clever things with SPM for MEG • Time-frequency analysis • Convert to 3 D (time) • Make contrasts in source space – not yet possible
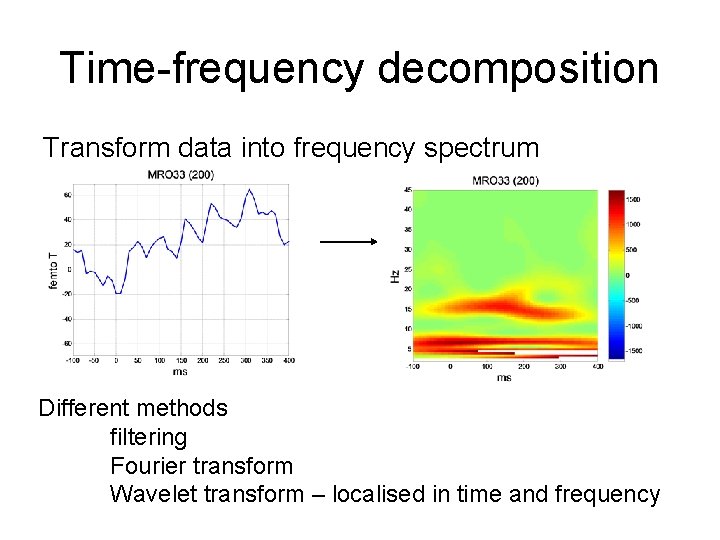
Time-frequency decomposition Transform data into frequency spectrum Different methods filtering Fourier transform Wavelet transform – localised in time and frequency
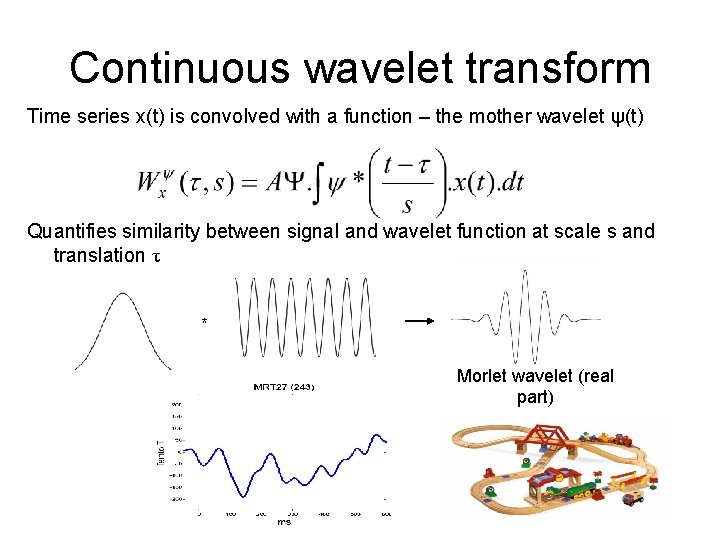
Continuous wavelet transform Time series x(t) is convolved with a function – the mother wavelet ψ(t) Quantifies similarity between signal and wavelet function at scale s and translation τ * Morlet wavelet (real part)
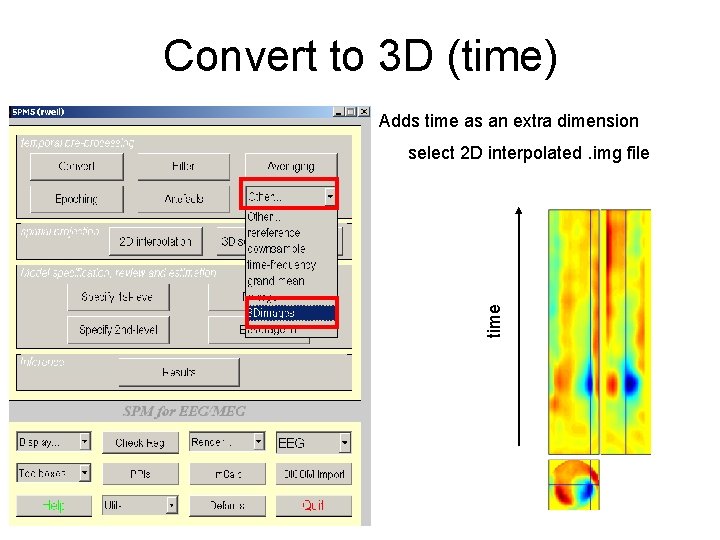
Convert to 3 D (time) Adds time as an extra dimension time select 2 D interpolated. img file
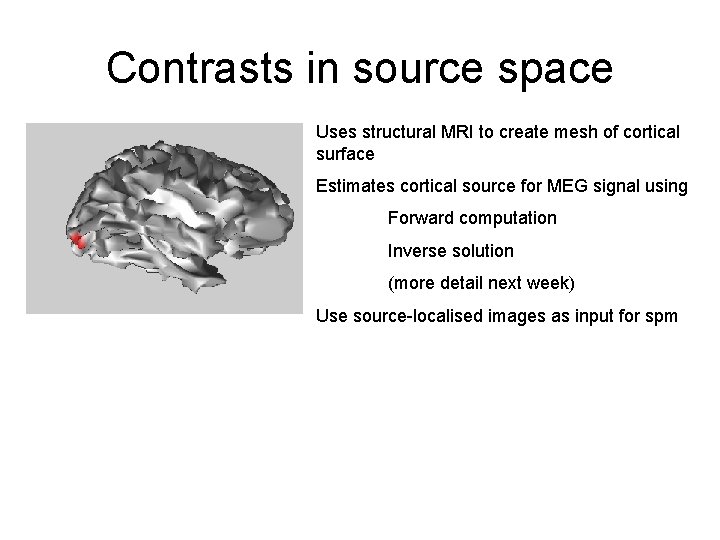
Contrasts in source space Uses structural MRI to create mesh of cortical surface Estimates cortical source for MEG signal using Forward computation Inverse solution (more detail next week) Use source-localised images as input for spm
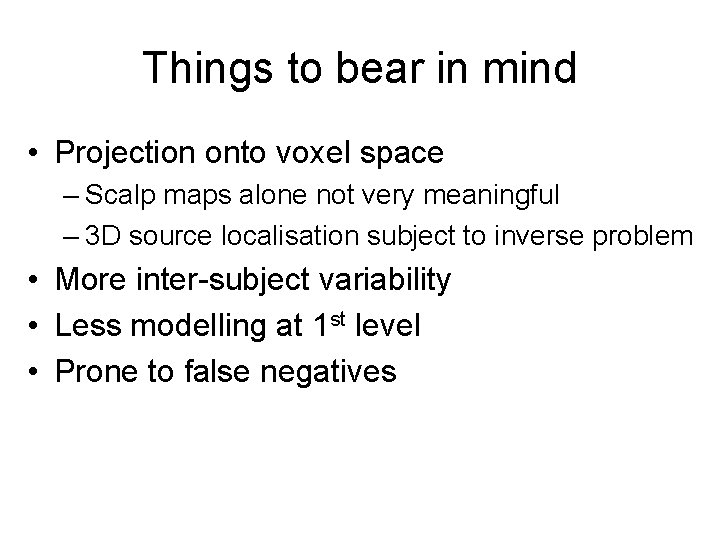
Things to bear in mind • Projection onto voxel space – Scalp maps alone not very meaningful – 3 D source localisation subject to inverse problem • More inter-subject variability • Less modelling at 1 st level • Prone to false negatives
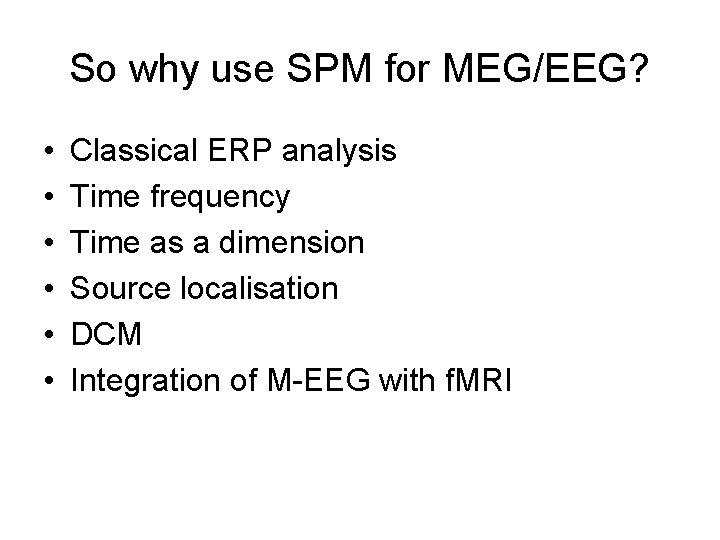
So why use SPM for MEG/EEG? • • • Classical ERP analysis Time frequency Time as a dimension Source localisation DCM Integration of M-EEG with f. MRI
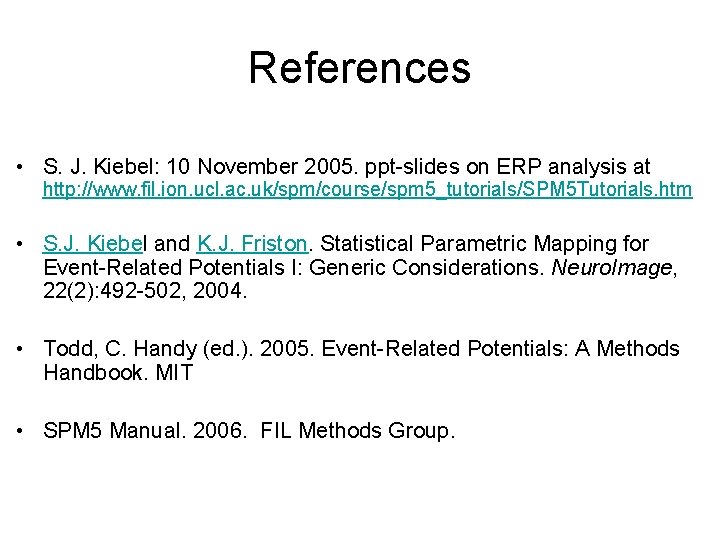
References • S. J. Kiebel: 10 November 2005. ppt-slides on ERP analysis at http: //www. fil. ion. ucl. ac. uk/spm/course/spm 5_tutorials/SPM 5 Tutorials. htm • S. J. Kiebel and K. J. Friston. Statistical Parametric Mapping for Event-Related Potentials I: Generic Considerations. Neuro. Image, 22(2): 492 -502, 2004. • Todd, C. Handy (ed. ). 2005. Event-Related Potentials: A Methods Handbook. MIT • SPM 5 Manual. 2006. FIL Methods Group.
Double contrast vs single contrast
Thoracic mri images
Nomics mri
Mri scanner
Baby mri face
Mri k space
Mri hydrogen atoms
Musculoskeletal mri anatomy
Gracilis muscle mri
Geometric distortion mri
Dog mri hancock county
Mrinella
Mri pulse sequence
Mri scoliosis protocol
How mri works
Mri full form
Romberg test positive causes
Hta diagnoosi
Function of putamen
Mri knee gp
Mri position
Viva quad xt
Pons mri