Language Speech Technology Arjan van Hessen Franciska de
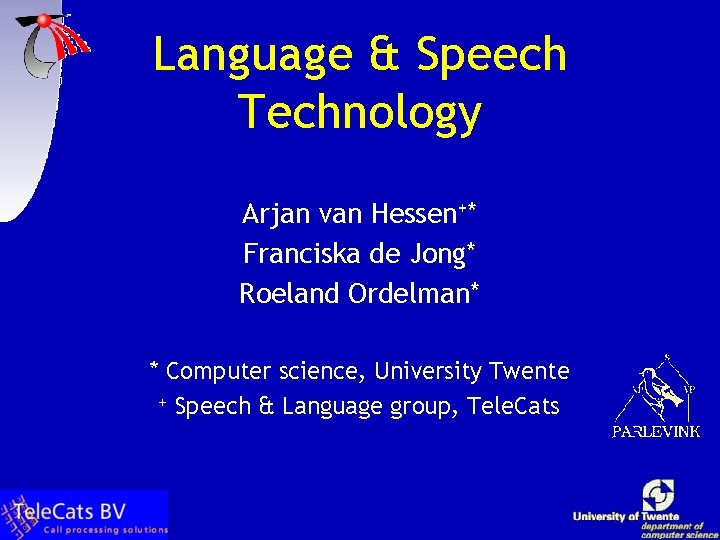
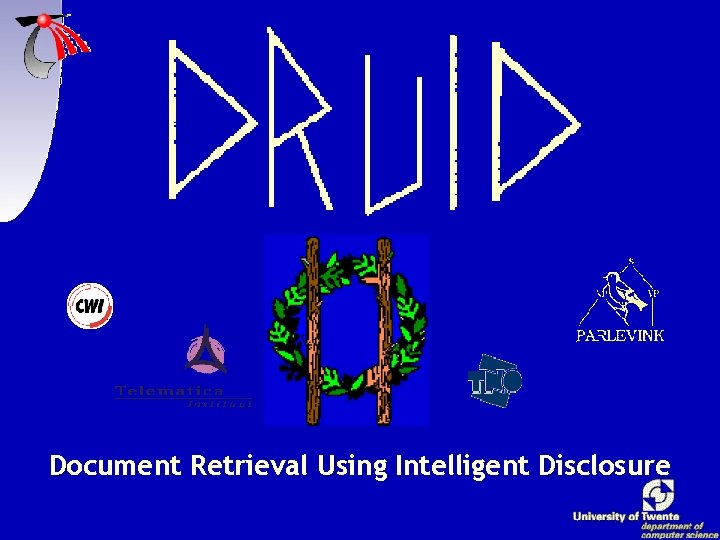
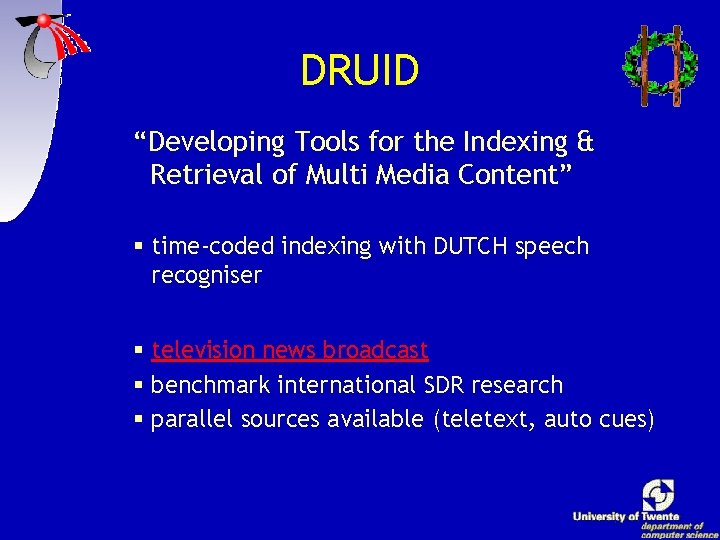
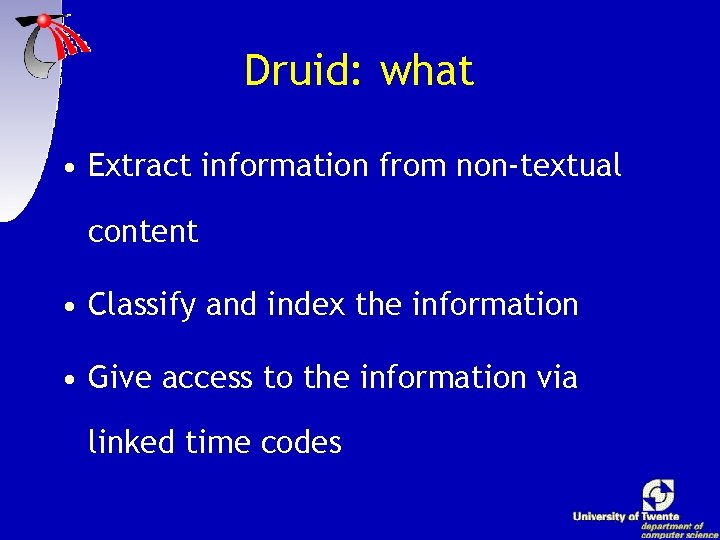
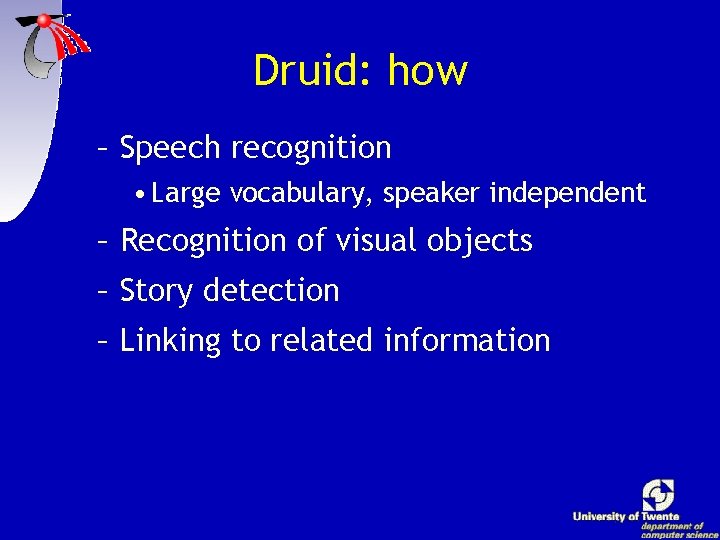
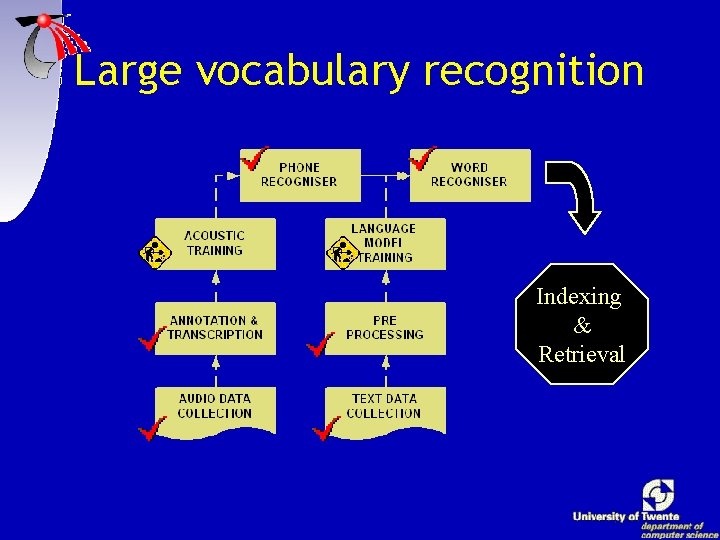
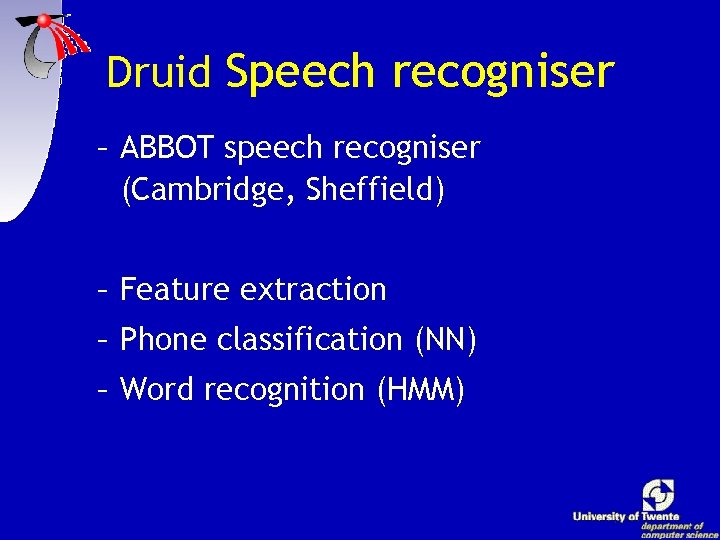
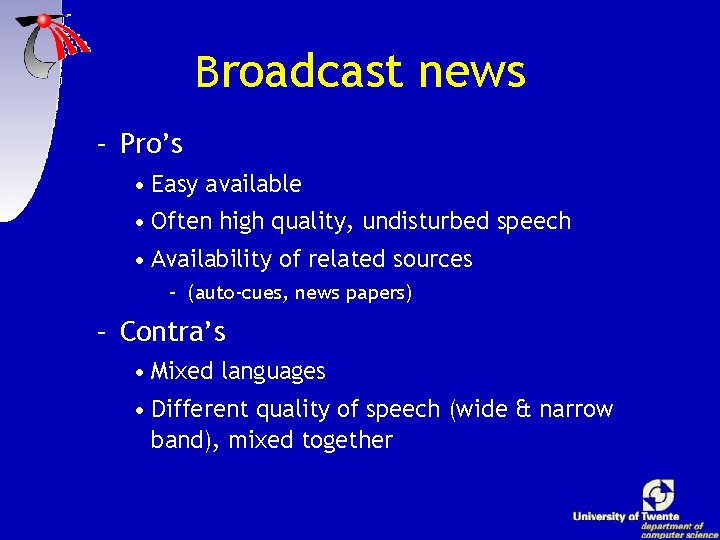
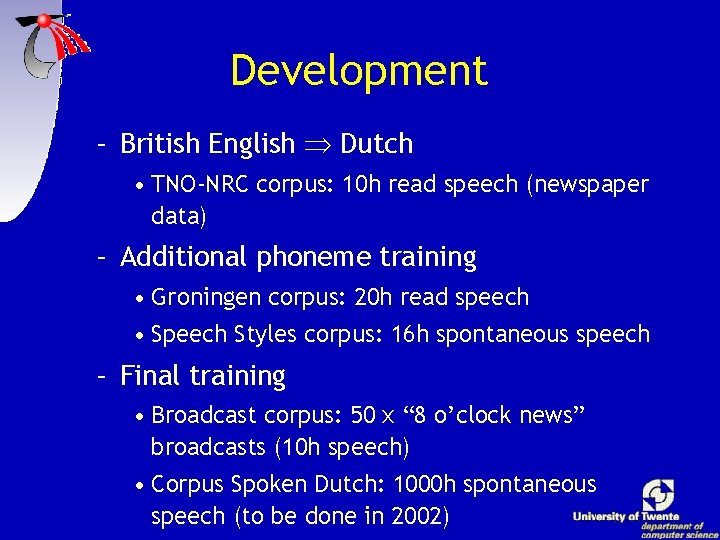
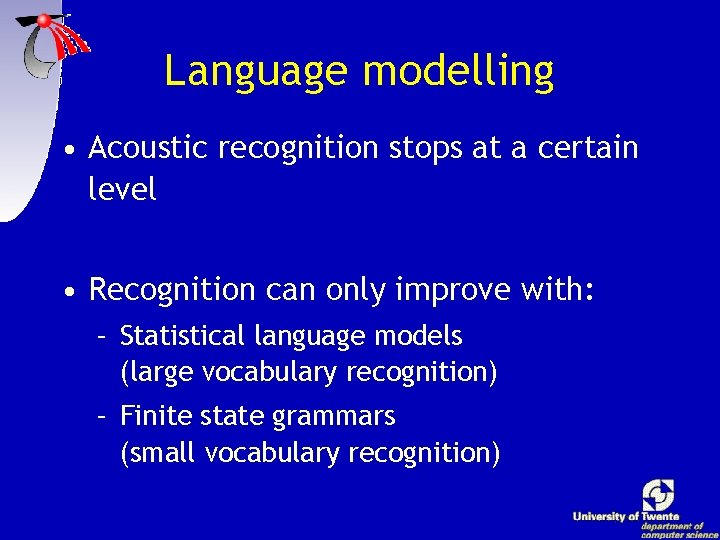
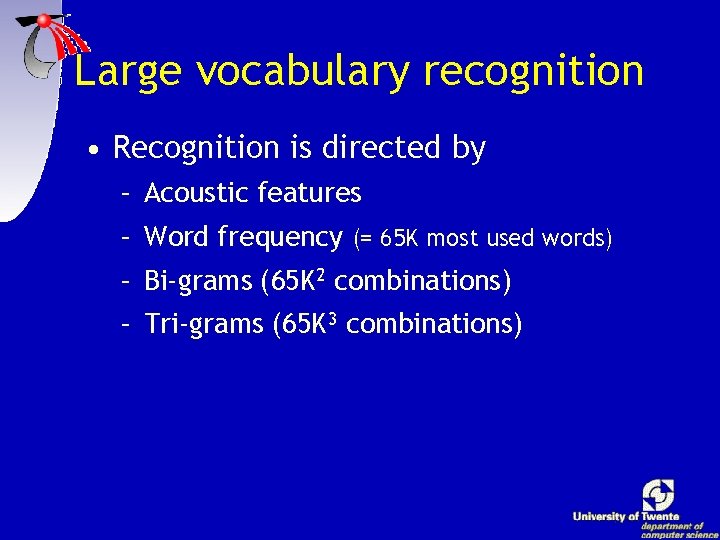
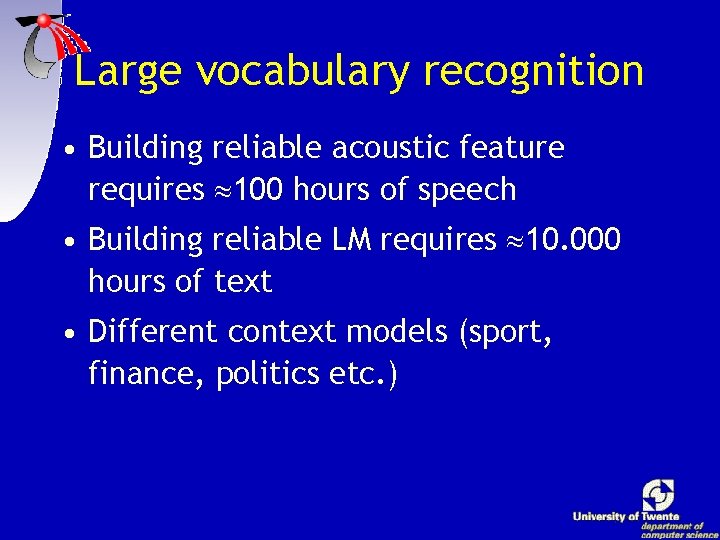
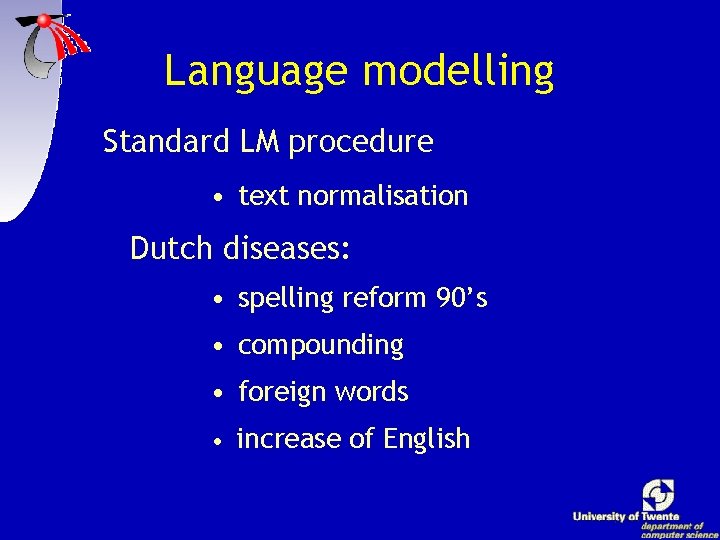
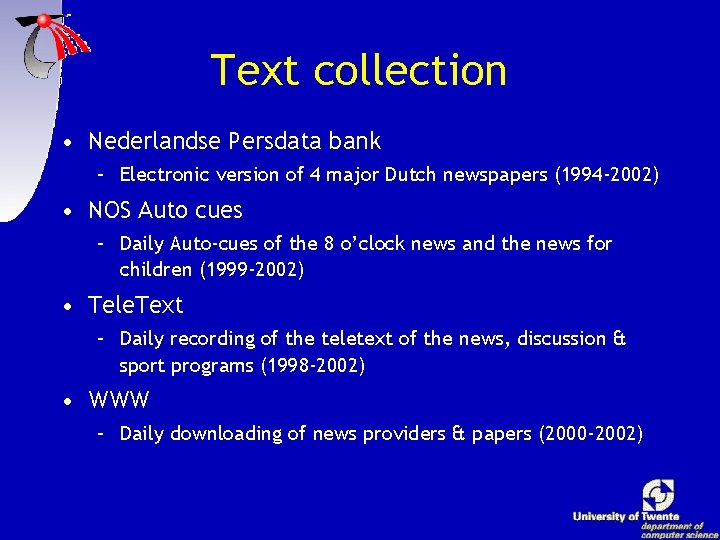
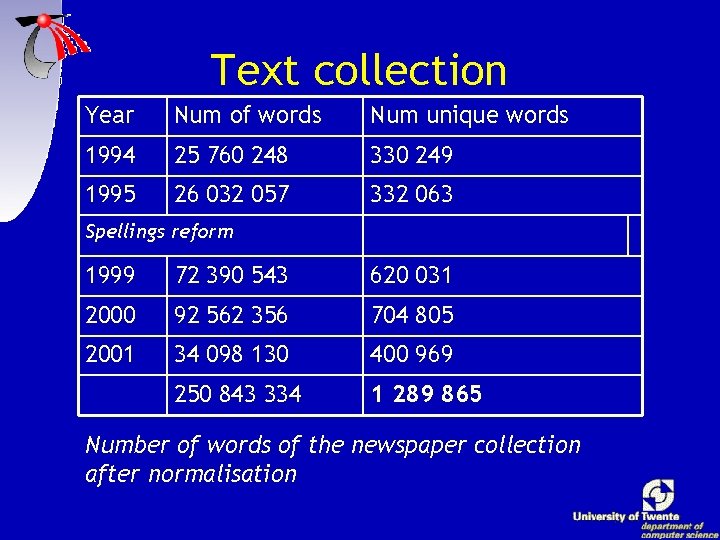
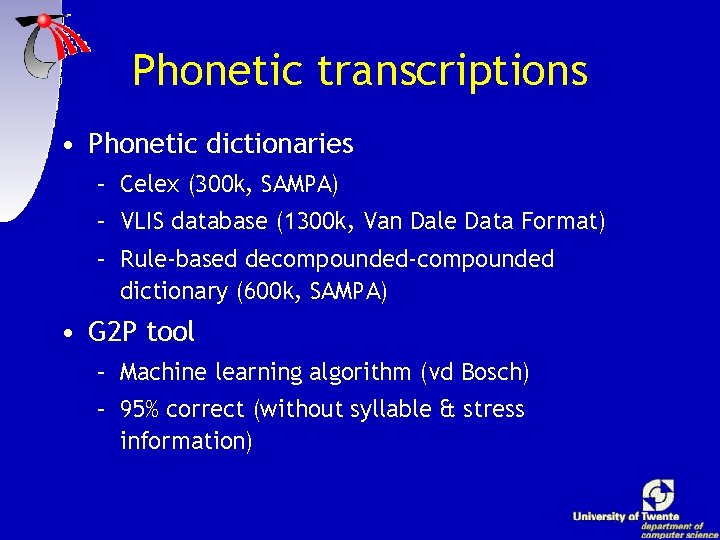
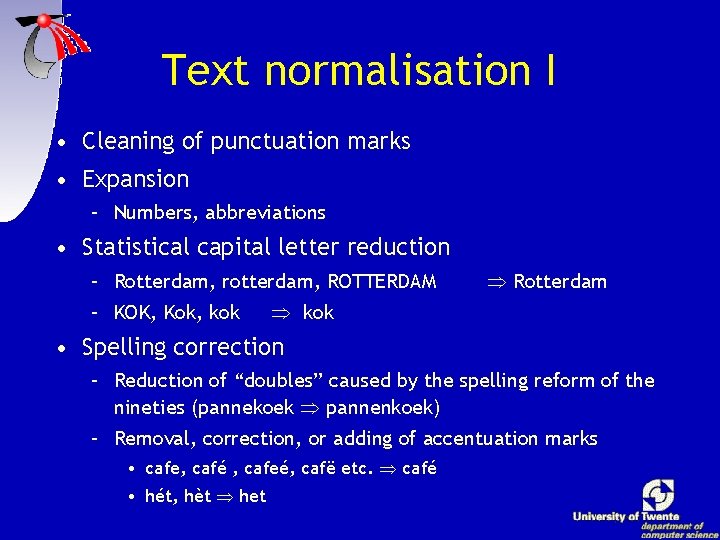
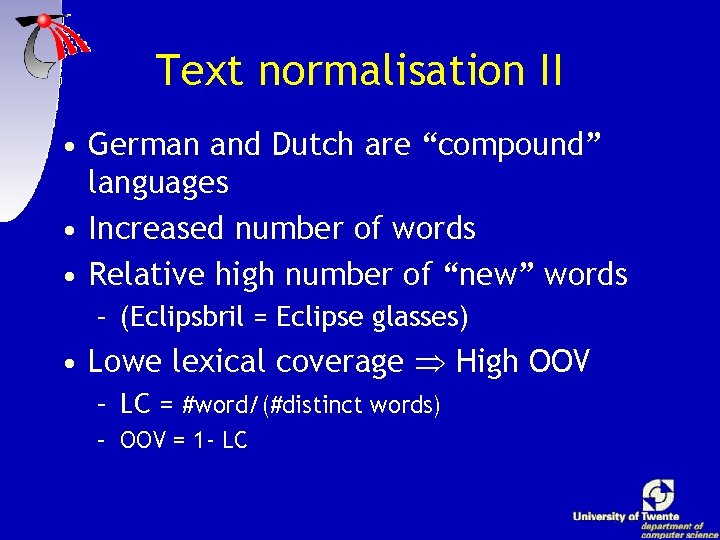
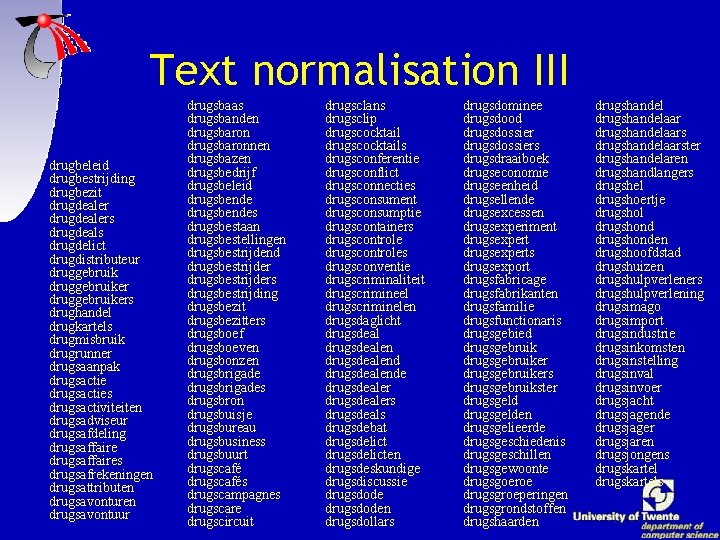
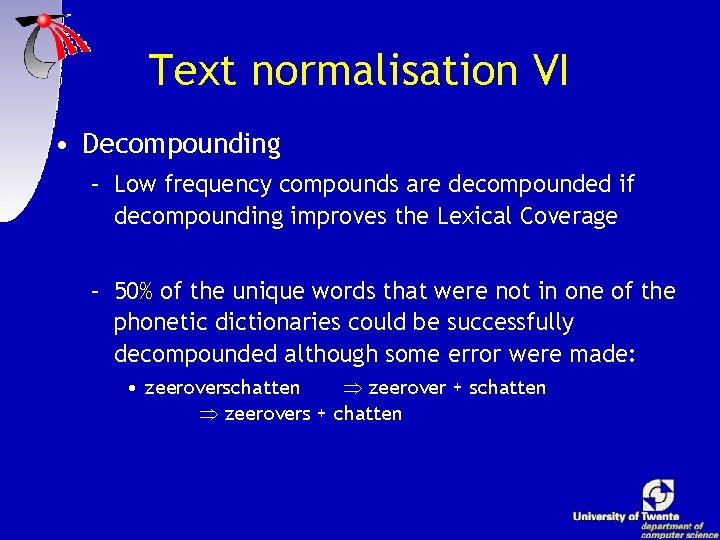
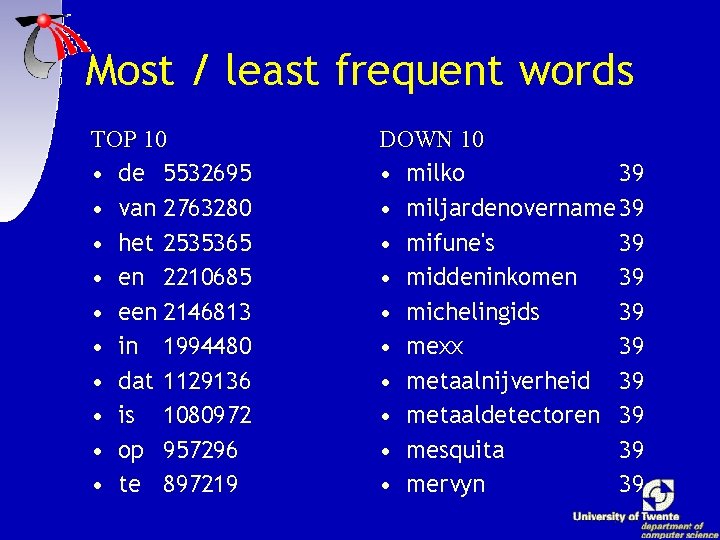
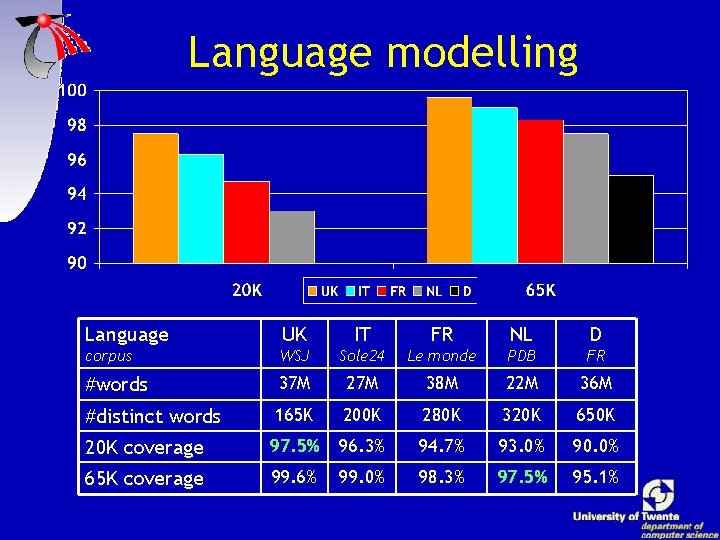
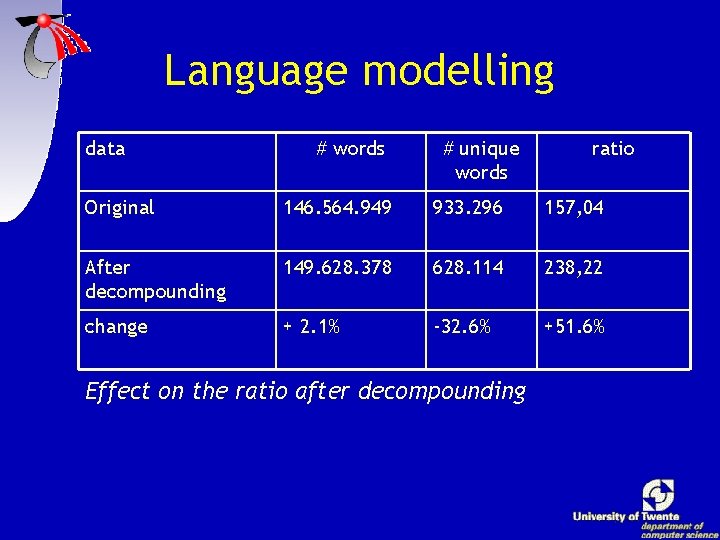
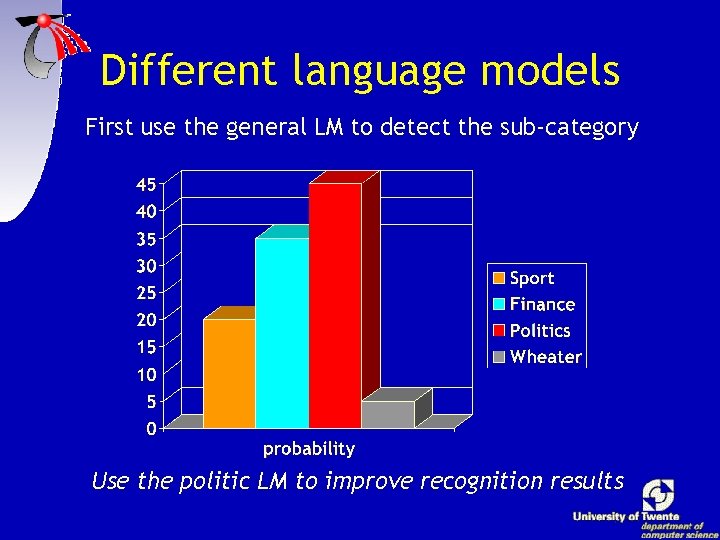
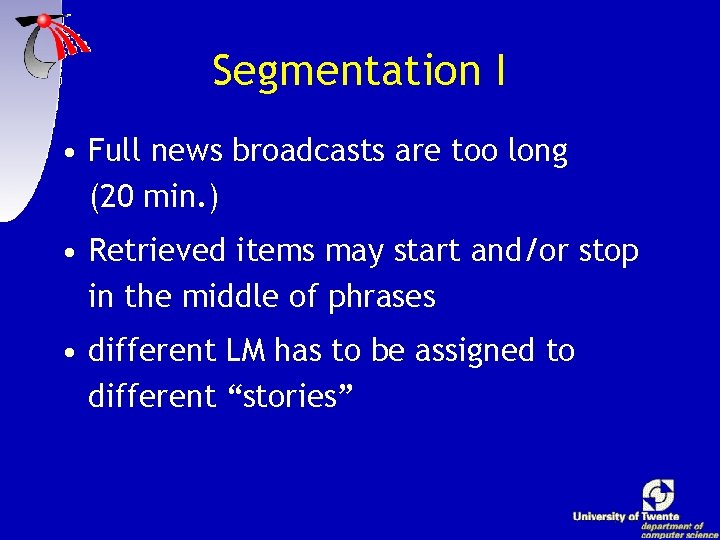
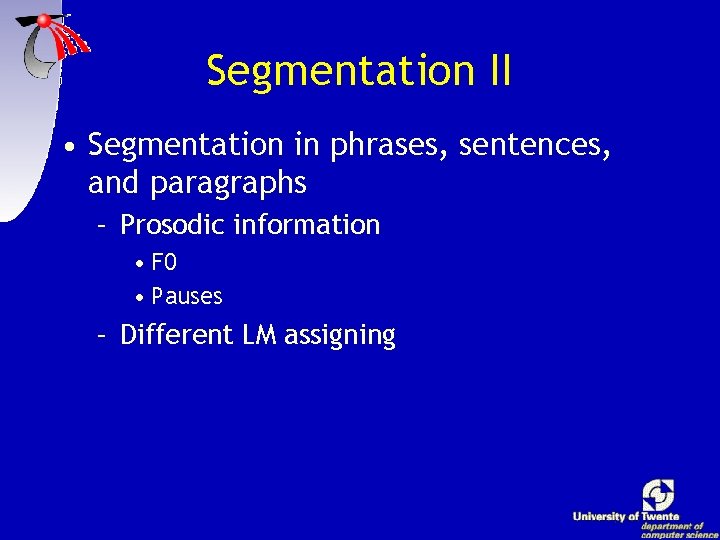
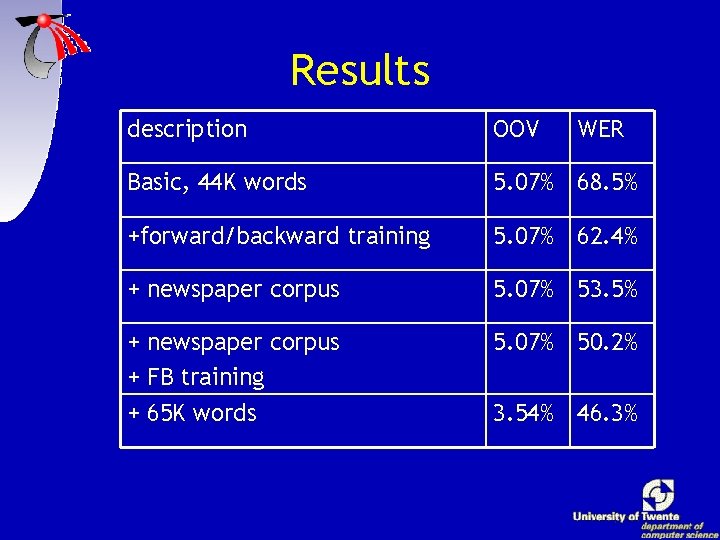
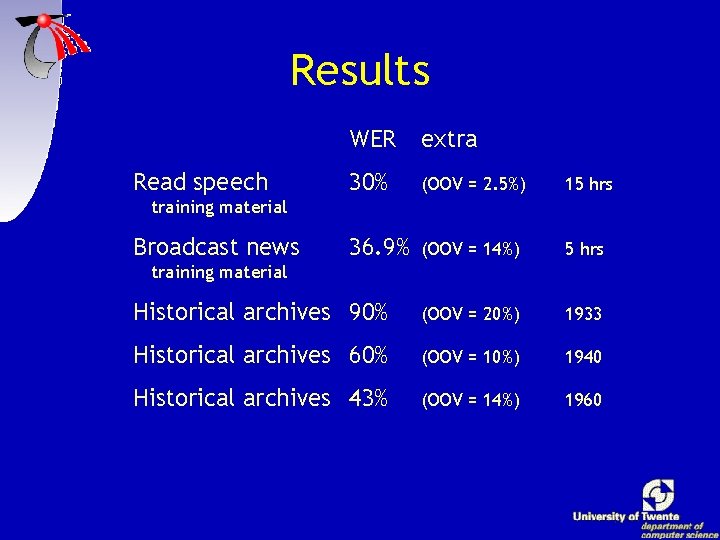
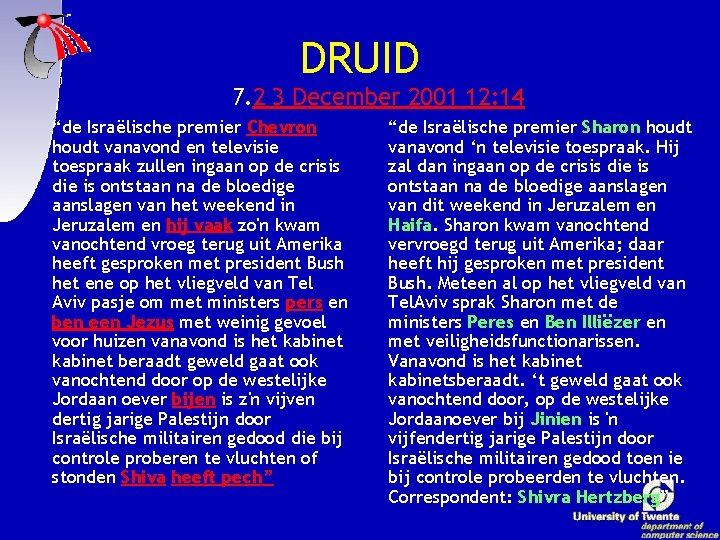
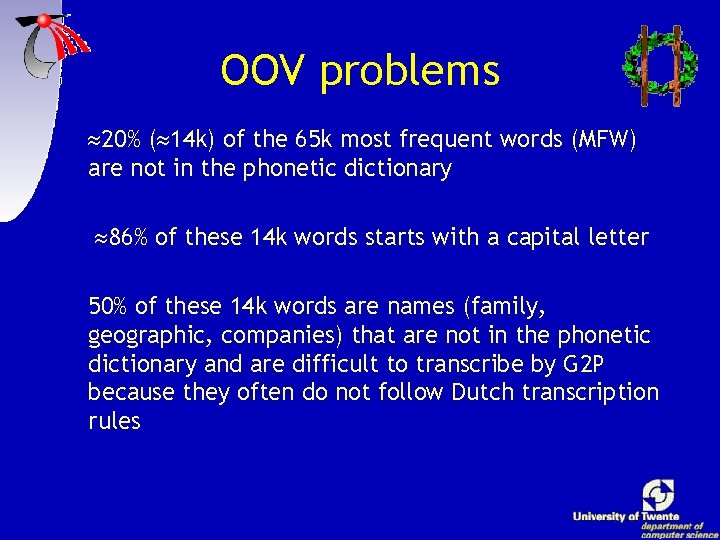
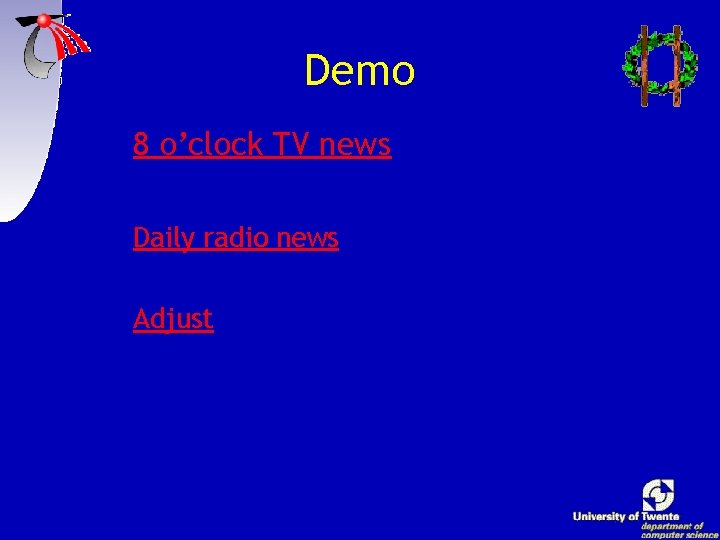
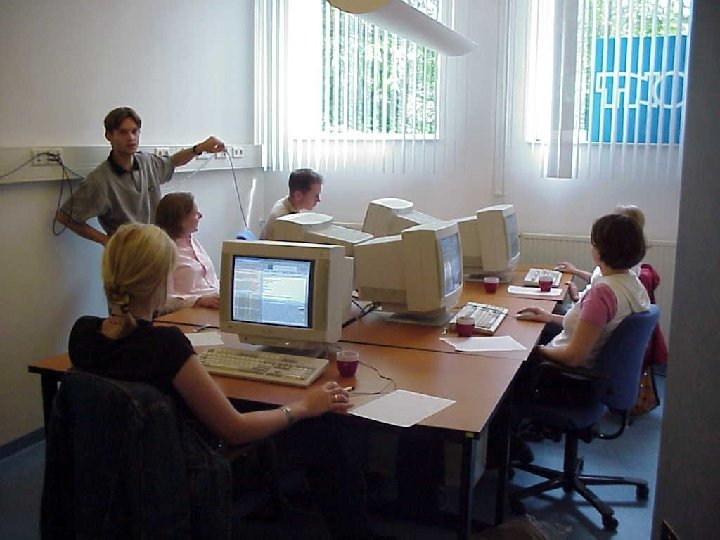
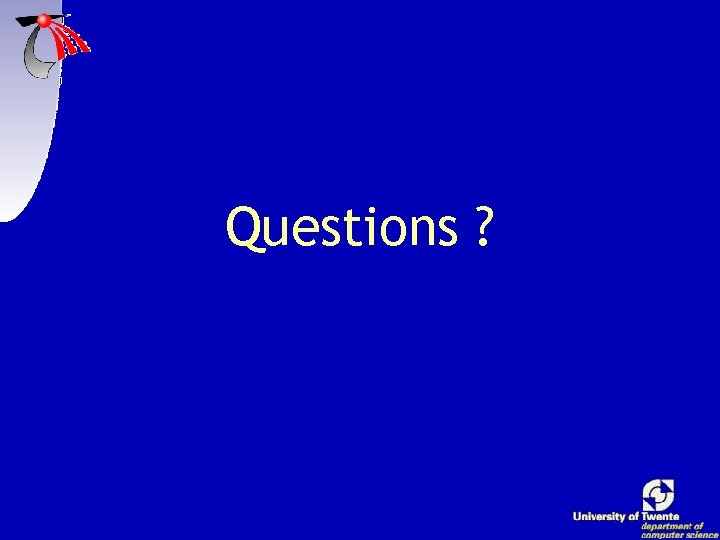
- Slides: 33
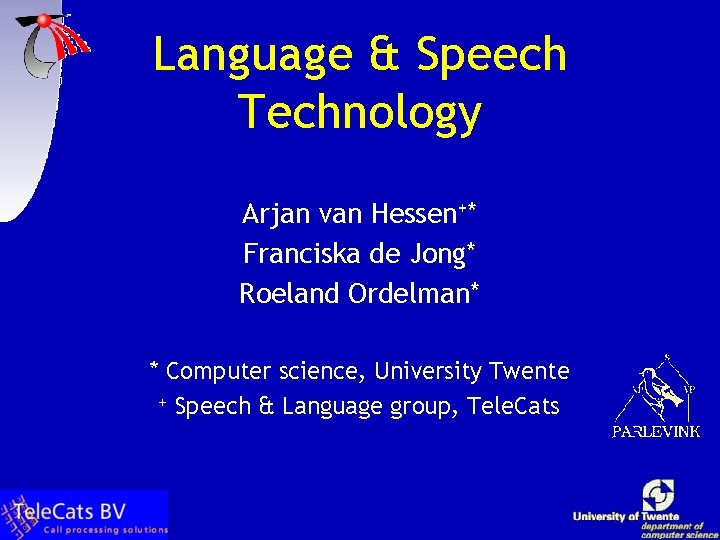
Language & Speech Technology Arjan van Hessen+* Franciska de Jong* Roeland Ordelman* * Computer science, University Twente + Speech & Language group, Tele. Cats
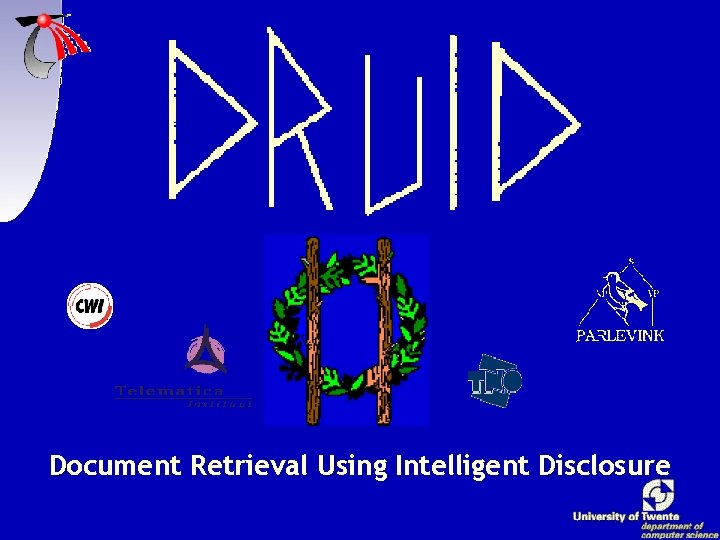
Document Retrieval Using Intelligent Disclosure
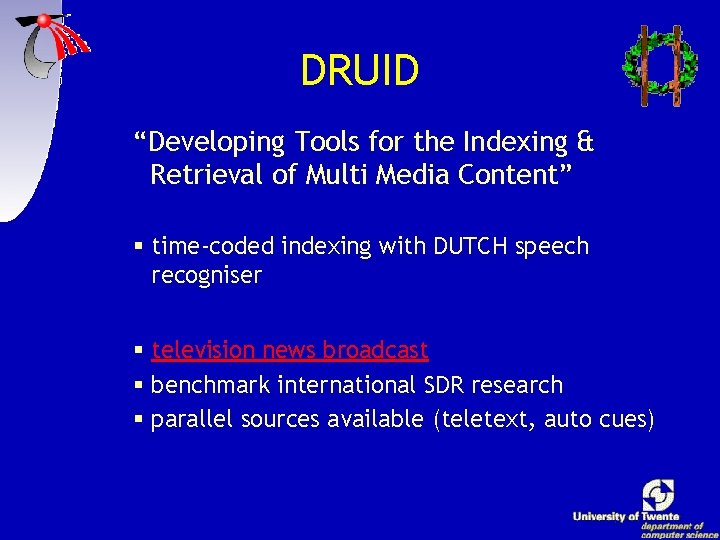
DRUID “Developing Tools for the Indexing & Retrieval of Multi Media Content” § time-coded indexing with DUTCH speech recogniser § television news broadcast § benchmark international SDR research § parallel sources available (teletext, auto cues)
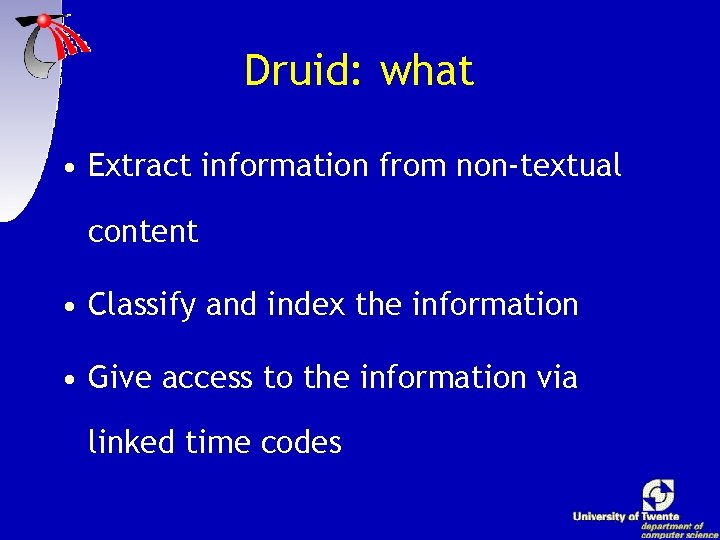
Druid: what • Extract information from non-textual content • Classify and index the information • Give access to the information via linked time codes
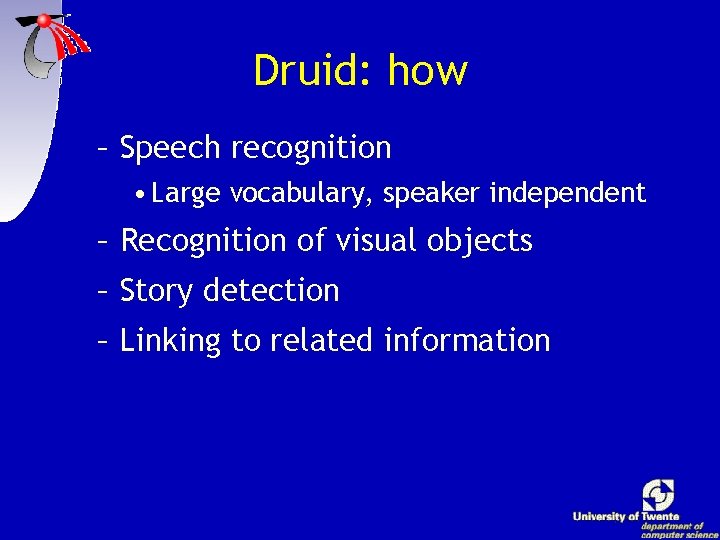
Druid: how – Speech recognition • Large vocabulary, speaker independent – Recognition of visual objects – Story detection – Linking to related information
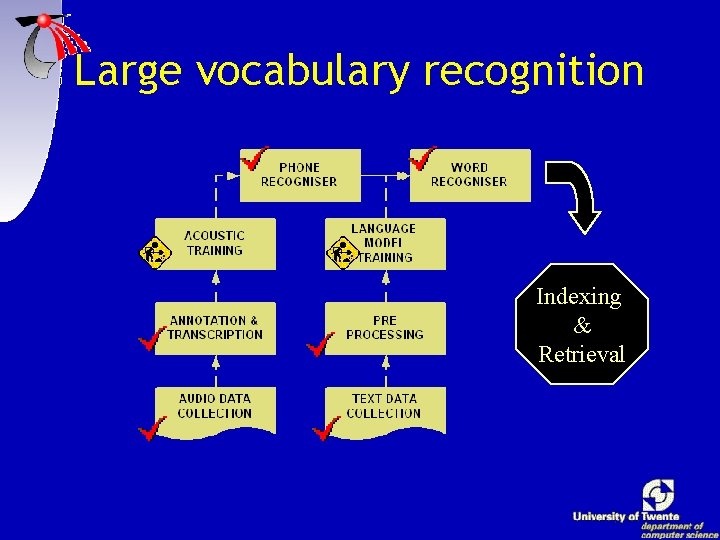
Large vocabulary recognition Indexing & Retrieval
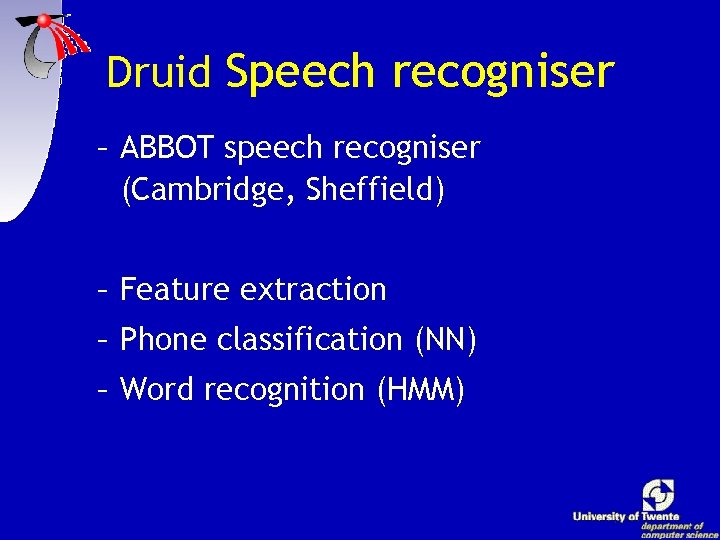
Druid Speech recogniser – ABBOT speech recogniser (Cambridge, Sheffield) – Feature extraction – Phone classification (NN) – Word recognition (HMM)
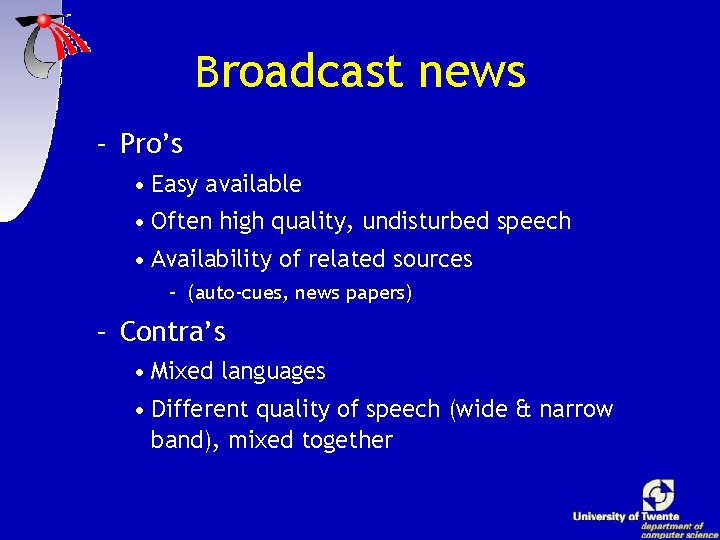
Broadcast news – Pro’s • Easy available • Often high quality, undisturbed speech • Availability of related sources – (auto-cues, news papers) – Contra’s • Mixed languages • Different quality of speech (wide & narrow band), mixed together
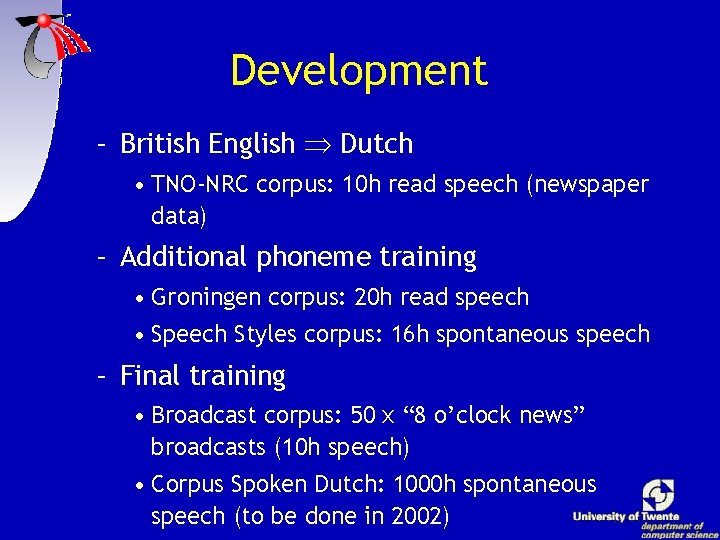
Development – British English Dutch • TNO-NRC corpus: 10 h read speech (newspaper data) – Additional phoneme training • Groningen corpus: 20 h read speech • Speech Styles corpus: 16 h spontaneous speech – Final training • Broadcast corpus: 50 x “ 8 o’clock news” broadcasts (10 h speech) • Corpus Spoken Dutch: 1000 h spontaneous speech (to be done in 2002)
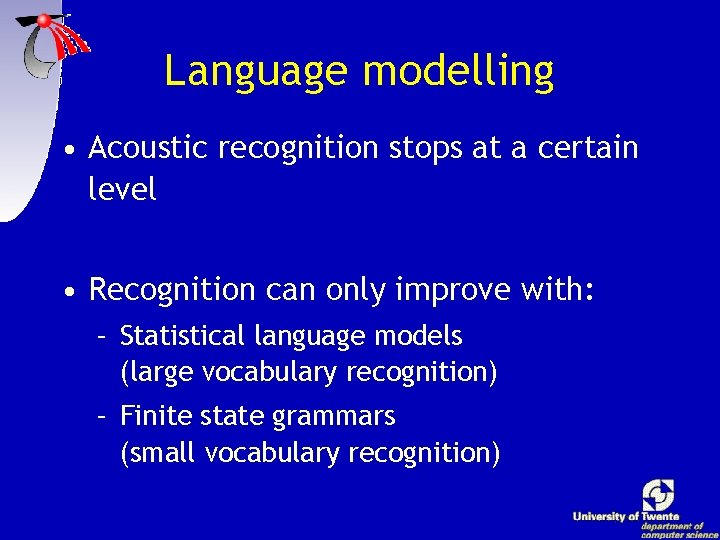
Language modelling • Acoustic recognition stops at a certain level • Recognition can only improve with: – Statistical language models (large vocabulary recognition) – Finite state grammars (small vocabulary recognition)
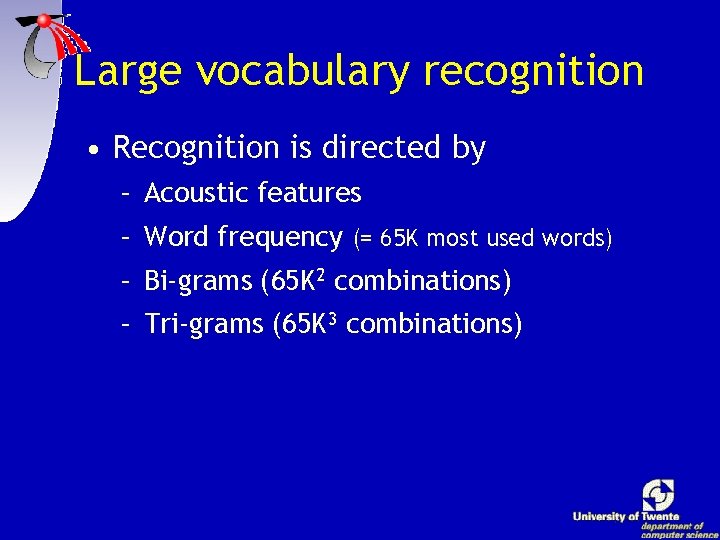
Large vocabulary recognition • Recognition is directed by – Acoustic features – Word frequency (= 65 K most used words) – Bi-grams (65 K 2 combinations) – Tri-grams (65 K 3 combinations)
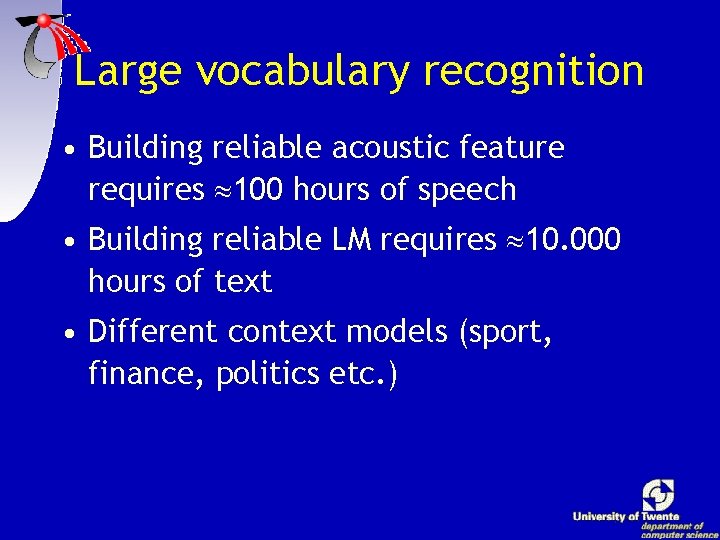
Large vocabulary recognition • Building reliable acoustic feature requires 100 hours of speech • Building reliable LM requires 10. 000 hours of text • Different context models (sport, finance, politics etc. )
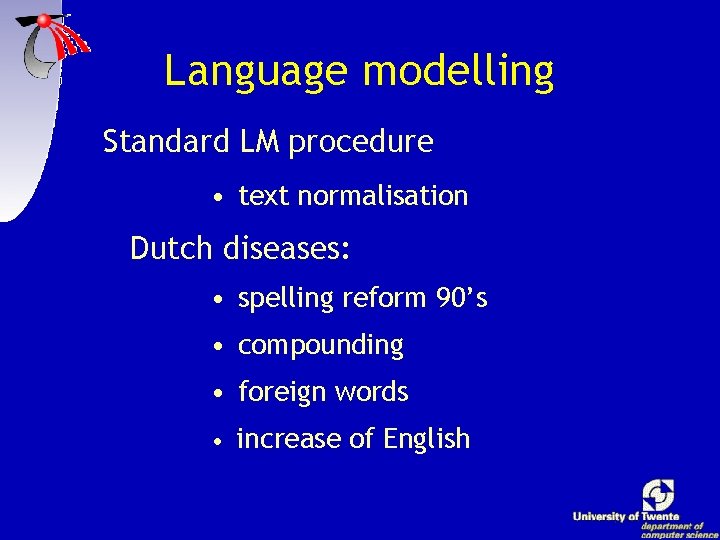
Language modelling Standard LM procedure • text normalisation Dutch diseases: • spelling reform 90’s • compounding • foreign words • increase of English
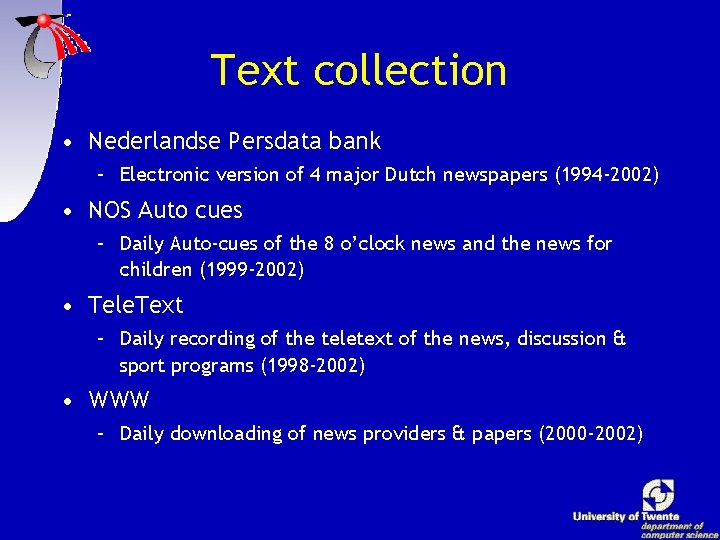
Text collection • Nederlandse Persdata bank – Electronic version of 4 major Dutch newspapers (1994 -2002) • NOS Auto cues – Daily Auto-cues of the 8 o’clock news and the news for children (1999 -2002) • Tele. Text – Daily recording of the teletext of the news, discussion & sport programs (1998 -2002) • WWW – Daily downloading of news providers & papers (2000 -2002)
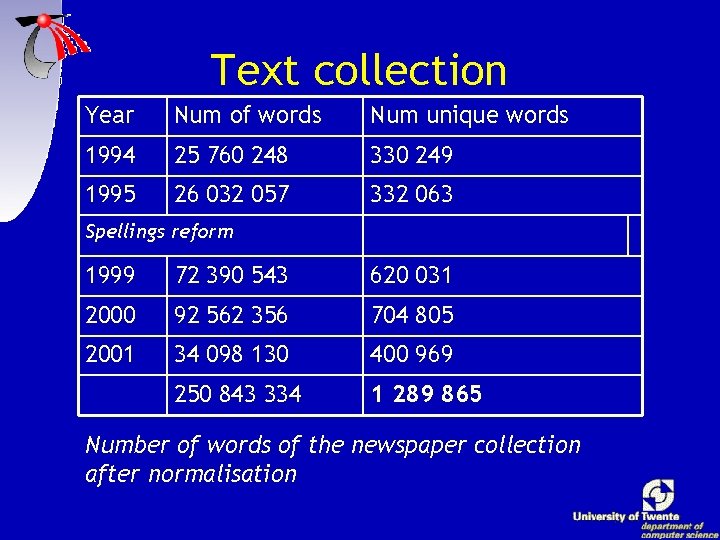
Text collection Year Num of words Num unique words 1994 25 760 248 330 249 1995 26 032 057 332 063 Spellings reform 1999 72 390 543 620 031 2000 92 562 356 704 805 2001 34 098 130 400 969 250 843 334 1 289 865 Number of words of the newspaper collection after normalisation
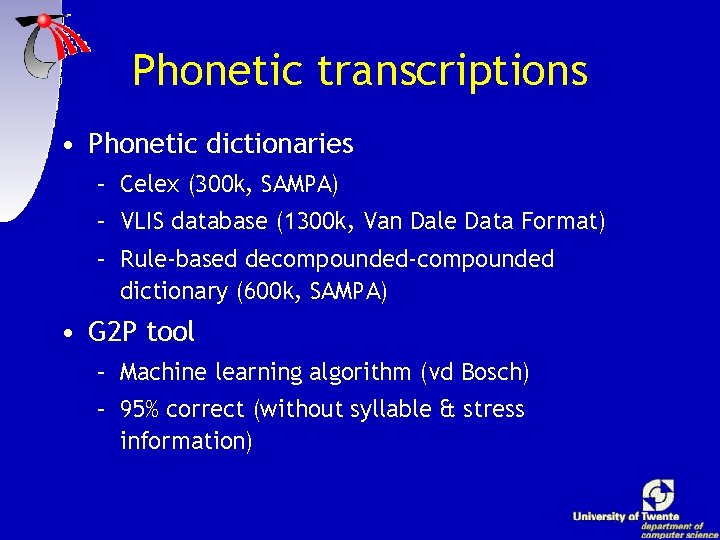
Phonetic transcriptions • Phonetic dictionaries – Celex (300 k, SAMPA) – VLIS database (1300 k, Van Dale Data Format) – Rule-based decompounded-compounded dictionary (600 k, SAMPA) • G 2 P tool – Machine learning algorithm (vd Bosch) – 95% correct (without syllable & stress information)
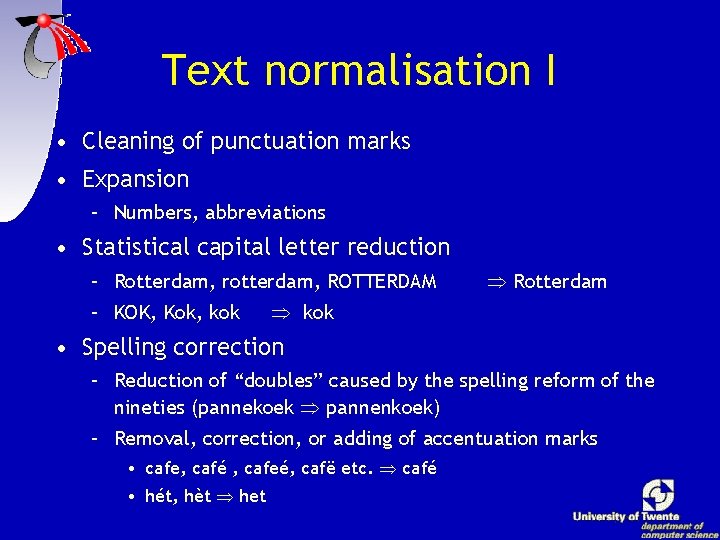
Text normalisation I • Cleaning of punctuation marks • Expansion – Numbers, abbreviations • Statistical capital letter reduction – Rotterdam, rotterdam, ROTTERDAM – KOK, Kok, kok Rotterdam kok • Spelling correction – Reduction of “doubles” caused by the spelling reform of the nineties (pannekoek pannenkoek) – Removal, correction, or adding of accentuation marks • cafe, café , cafeé, cafë etc. café • hét, hèt het
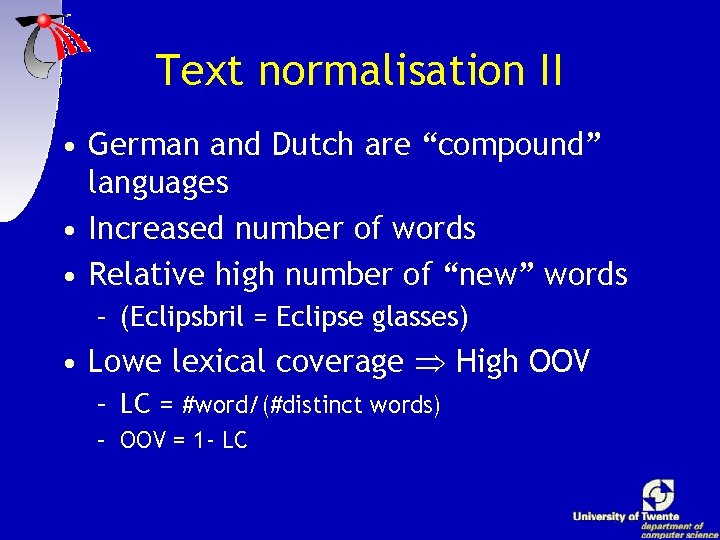
Text normalisation II • German and Dutch are “compound” languages • Increased number of words • Relative high number of “new” words – (Eclipsbril = Eclipse glasses) • Lowe lexical coverage High OOV – LC = #word/(#distinct words) – OOV = 1 - LC
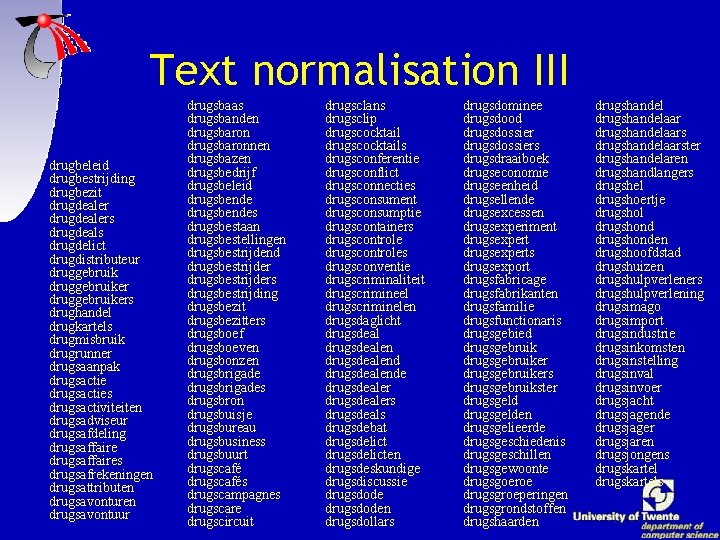
Text normalisation III drugbeleid drugbestrijding drugbezit drugdealers drugdeals drugdelict drugdistributeur druggebruikers drughandel drugkartels drugmisbruik drugrunner drugsaanpak drugsacties drugsactiviteiten drugsadviseur drugsafdeling drugsaffaires drugsafrekeningen drugsattributen drugsavonturen drugsavontuur drugsbaas drugsbanden drugsbaronnen drugsbazen drugsbedrijf drugsbeleid drugsbendes drugsbestaan drugsbestellingen drugsbestrijdend drugsbestrijders drugsbestrijding drugsbezitters drugsboef drugsboeven drugsbonzen drugsbrigades drugsbron drugsbuisje drugsbureau drugsbusiness drugsbuurt drugscafés drugscampagnes drugscare drugscircuit drugsclans drugsclip drugscocktails drugsconferentie drugsconflict drugsconnecties drugsconsument drugsconsumptie drugscontainers drugscontroles drugsconventie drugscriminaliteit drugscrimineel drugscriminelen drugsdaglicht drugsdealende drugsdealers drugsdeals drugsdebat drugsdelicten drugsdeskundige drugsdiscussie drugsdoden drugsdollars drugsdominee drugsdood drugsdossiers drugsdraaiboek drugseconomie drugseenheid drugsellende drugsexcessen drugsexperiment drugsexperts drugsexport drugsfabricage drugsfabrikanten drugsfamilie drugsfunctionaris drugsgebied drugsgebruikers drugsgebruikster drugsgelden drugsgelieerde drugsgeschiedenis drugsgeschillen drugsgewoonte drugsgoeroe drugsgroeperingen drugsgrondstoffen drugshaarden drugshandelaarster drugshandelaren drugshandlangers drugshel drugshoertje drugshol drugshonden drugshoofdstad drugshuizen drugshulpverleners drugshulpverlening drugsimago drugsimport drugsindustrie drugsinkomsten drugsinstelling drugsinval drugsinvoer drugsjacht drugsjagende drugsjager drugsjaren drugsjongens drugskartels
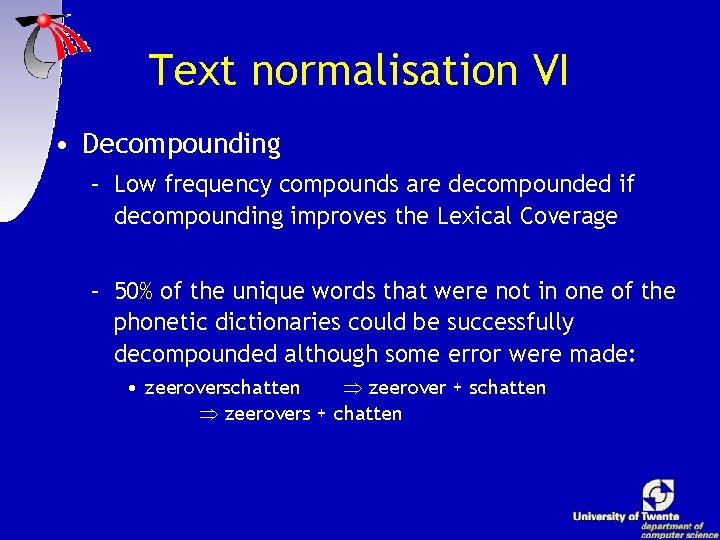
Text normalisation VI • Decompounding – Low frequency compounds are decompounded if decompounding improves the Lexical Coverage – 50% of the unique words that were not in one of the phonetic dictionaries could be successfully decompounded although some error were made: • zeeroverschatten zeerover + schatten zeerovers + chatten
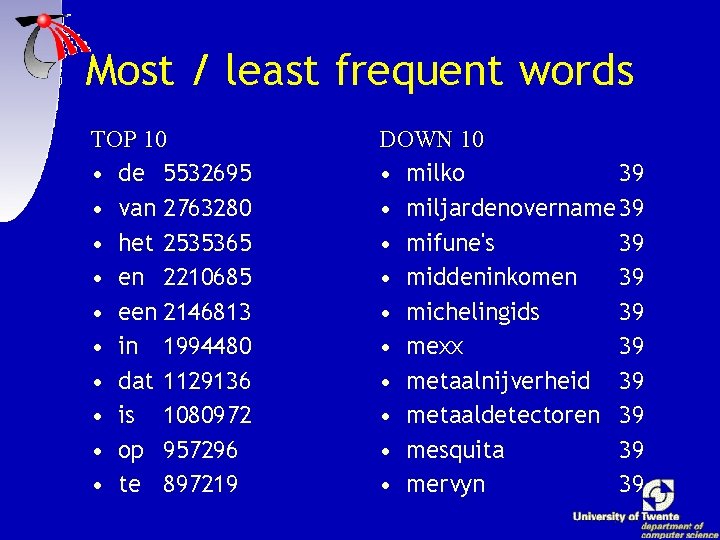
Most / least frequent words TOP 10 • de 5532695 • van 2763280 • het 2535365 • en 2210685 • een 2146813 • in 1994480 • dat 1129136 • is 1080972 • op 957296 • te 897219 DOWN 10 • milko 39 • miljardenovername 39 • mifune's 39 • middeninkomen 39 • michelingids 39 • mexx 39 • metaalnijverheid 39 • metaaldetectoren 39 • mesquita 39 • mervyn 39
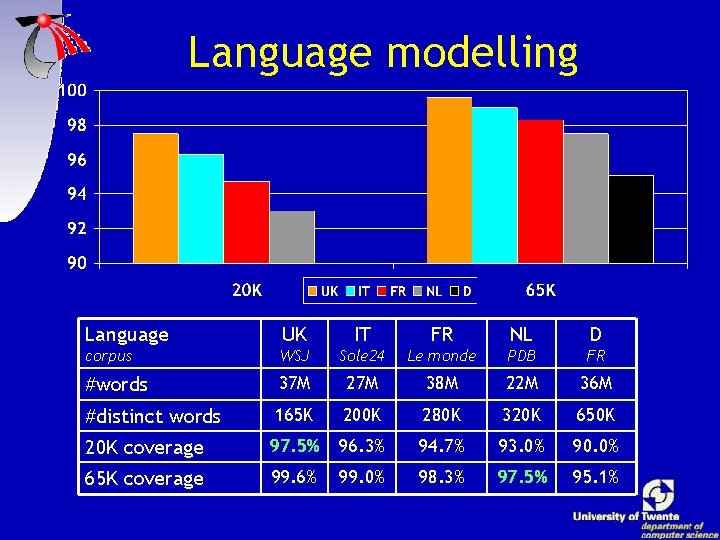
Language modelling Language UK IT FR NL D corpus WSJ Sole 24 Le monde PDB FR #words 37 M 27 M 38 M 22 M 36 M #distinct words 165 K 200 K 280 K 320 K 650 K 20 K coverage 97. 5% 96. 3% 94. 7% 93. 0% 90. 0% 65 K coverage 99. 6% 99. 0% 98. 3% 97. 5% 95. 1%
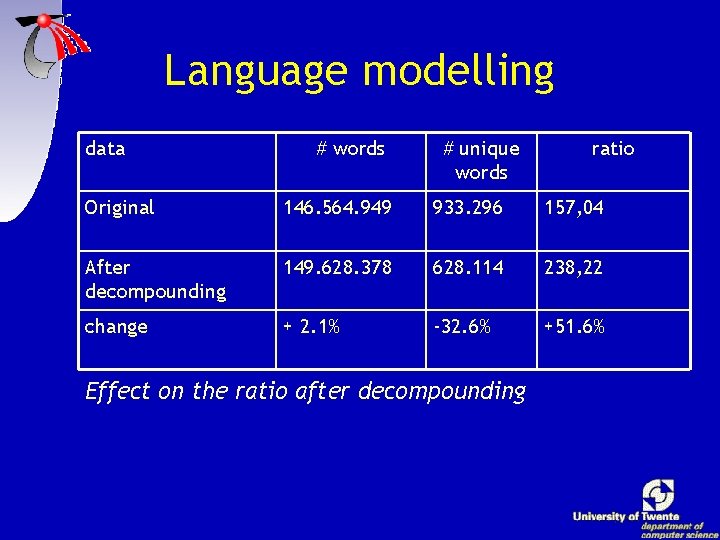
Language modelling data # words # unique words ratio Original 146. 564. 949 933. 296 157, 04 After decompounding 149. 628. 378 628. 114 238, 22 change + 2. 1% -32. 6% +51. 6% Effect on the ratio after decompounding
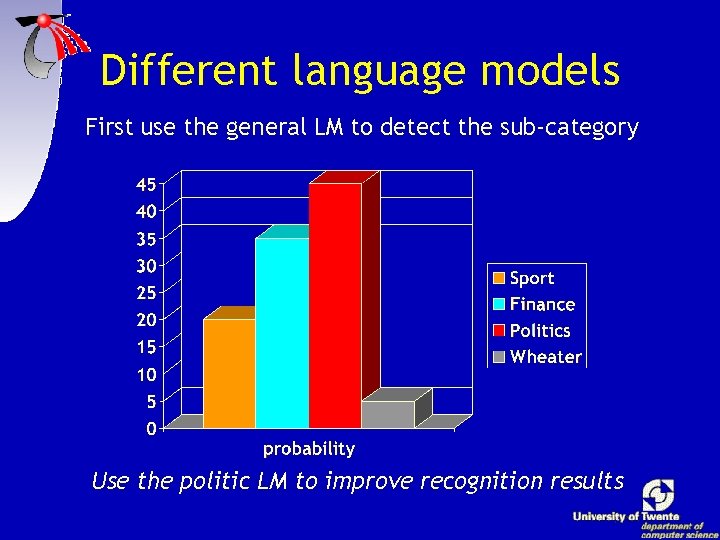
Different language models First use the general LM to detect the sub-category Use the politic LM to improve recognition results
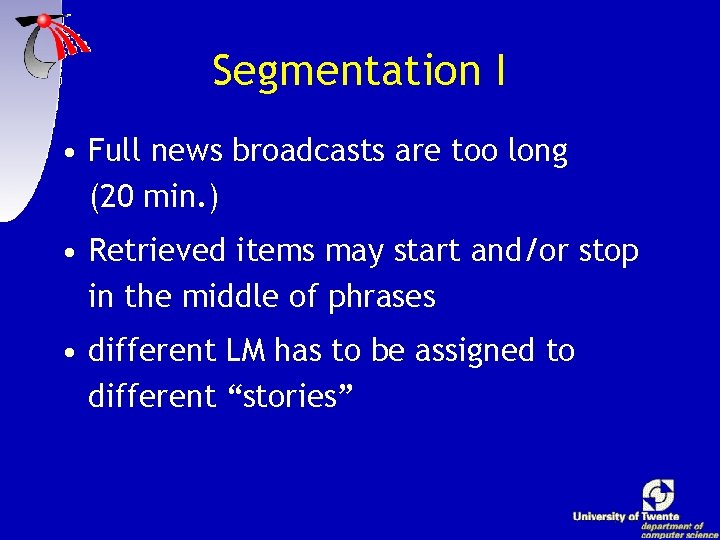
Segmentation I • Full news broadcasts are too long (20 min. ) • Retrieved items may start and/or stop in the middle of phrases • different LM has to be assigned to different “stories”
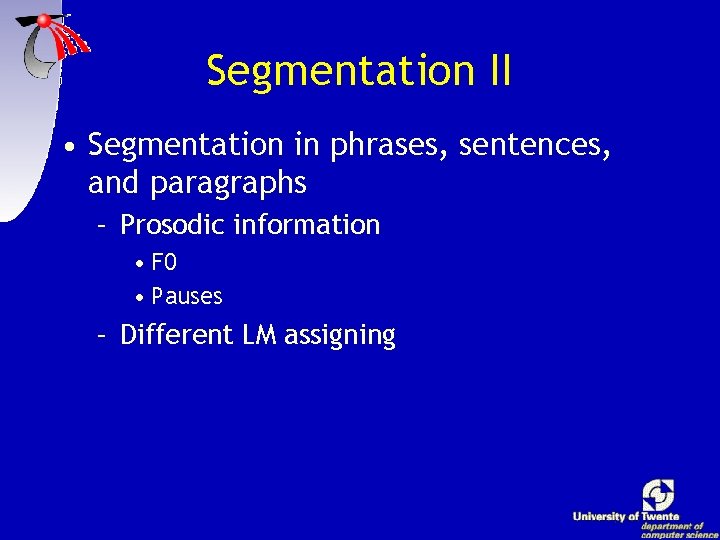
Segmentation II • Segmentation in phrases, sentences, and paragraphs – Prosodic information • F 0 • Pauses – Different LM assigning
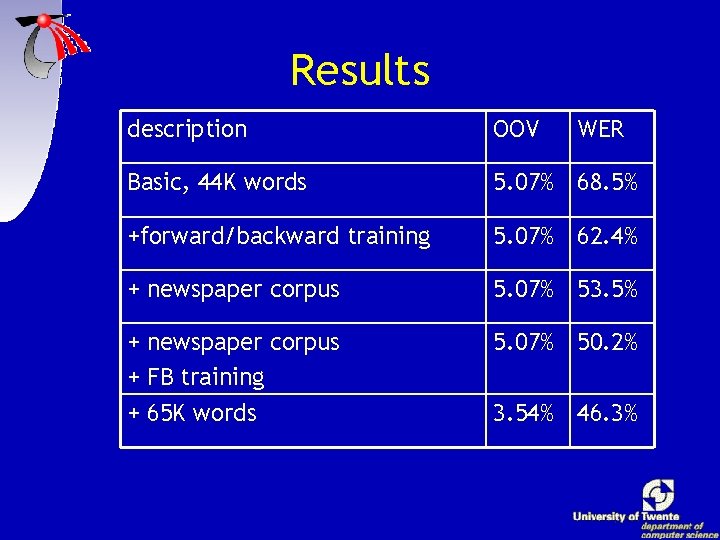
Results description OOV WER Basic, 44 K words 5. 07% 68. 5% +forward/backward training 5. 07% 62. 4% + newspaper corpus 5. 07% 53. 5% + newspaper corpus + FB training + 65 K words 5. 07% 50. 2% 3. 54% 46. 3%
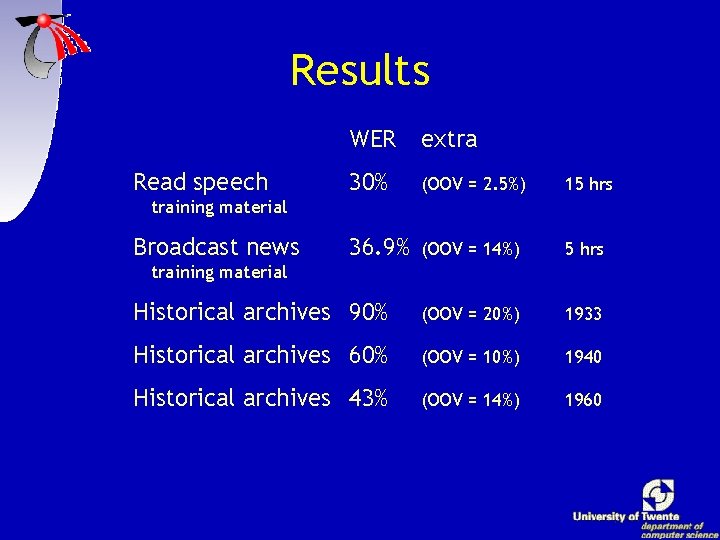
Results WER extra 30% (OOV = 2. 5%) 15 hrs 36. 9% (OOV = 14%) 5 hrs Historical archives 90% (OOV = 20%) 1933 Historical archives 60% (OOV = 10%) 1940 Historical archives 43% (OOV = 14%) 1960 Read speech training material Broadcast news training material
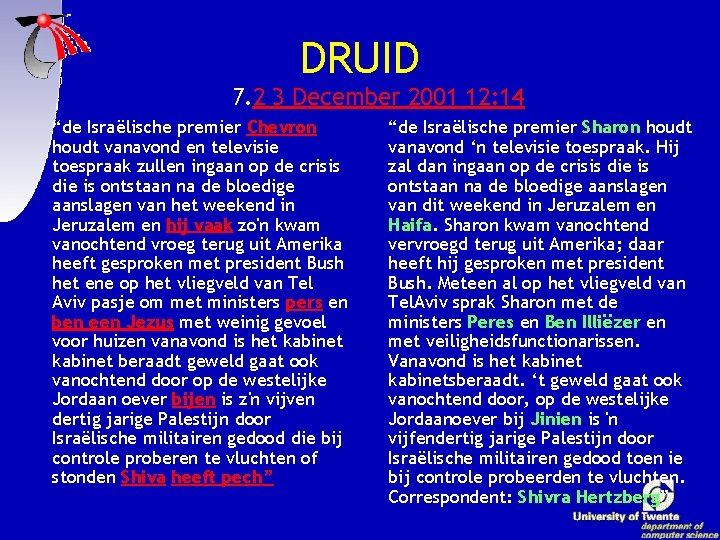
DRUID 7. 2 3 December 2001 12: 14 “de Israëlische premier Chevron houdt vanavond en televisie toespraak zullen ingaan op de crisis die is ontstaan na de bloedige aanslagen van het weekend in Jeruzalem en hij vaak zo'n kwam vanochtend vroeg terug uit Amerika heeft gesproken met president Bush het ene op het vliegveld van Tel Aviv pasje om met ministers pers en ben een Jezus met weinig gevoel voor huizen vanavond is het kabinet beraadt geweld gaat ook vanochtend door op de westelijke Jordaan oever bijen is z'n vijven dertig jarige Palestijn door Israëlische militairen gedood die bij controle proberen te vluchten of stonden Shiva heeft pech” “de Israëlische premier Sharon houdt vanavond ‘n televisie toespraak. Hij zal dan ingaan op de crisis die is ontstaan na de bloedige aanslagen van dit weekend in Jeruzalem en Haifa. Sharon kwam vanochtend vervroegd terug uit Amerika; daar heeft hij gesproken met president Bush. Meteen al op het vliegveld van Tel. Aviv sprak Sharon met de ministers Peres en Ben Illiëzer en met veiligheidsfunctionarissen. Vanavond is het kabinetsberaadt. ‘t geweld gaat ook vanochtend door, op de westelijke Jordaanoever bij Jinien is 'n vijfendertig jarige Palestijn door Israëlische militairen gedood toen ie bij controle probeerden te vluchten. Correspondent: Shivra Hertzberg”
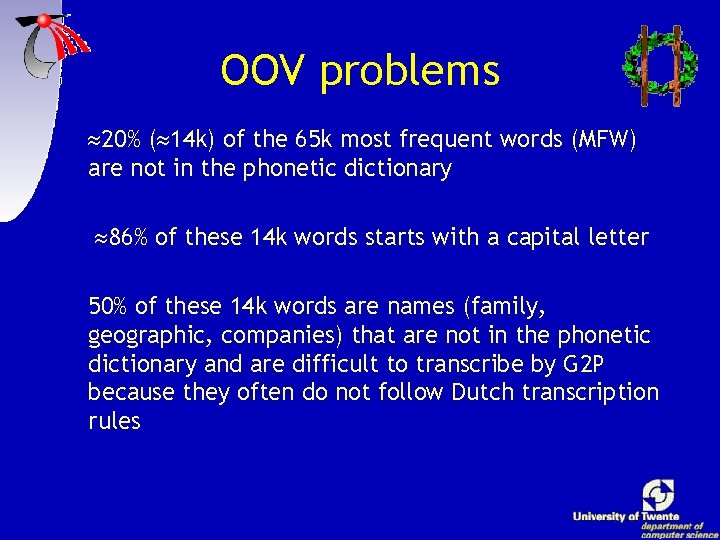
OOV problems 20% ( 14 k) of the 65 k most frequent words (MFW) are not in the phonetic dictionary 86% of these 14 k words starts with a capital letter 50% of these 14 k words are names (family, geographic, companies) that are not in the phonetic dictionary and are difficult to transcribe by G 2 P because they often do not follow Dutch transcription rules
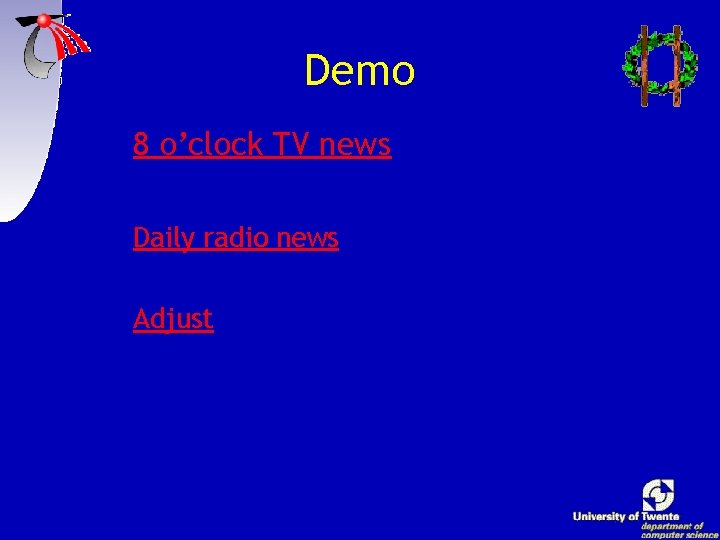
Demo 8 o’clock TV news Daily radio news Adjust
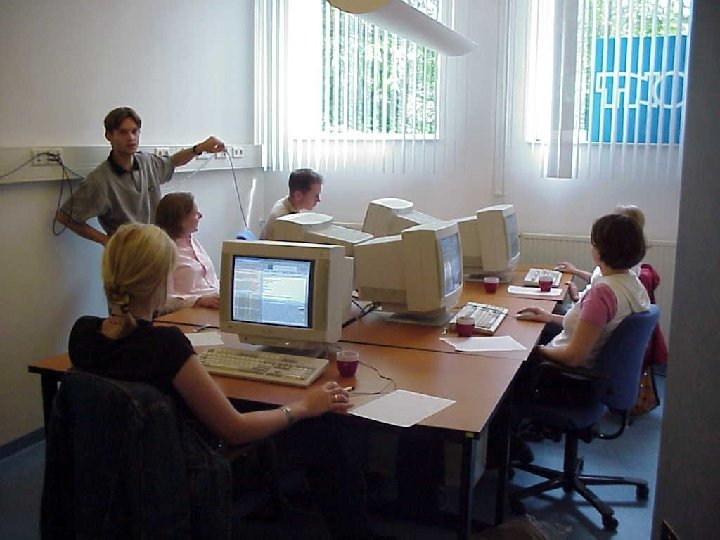
DRUID • Evaluation – A time consuming, boring, but necessary process!!
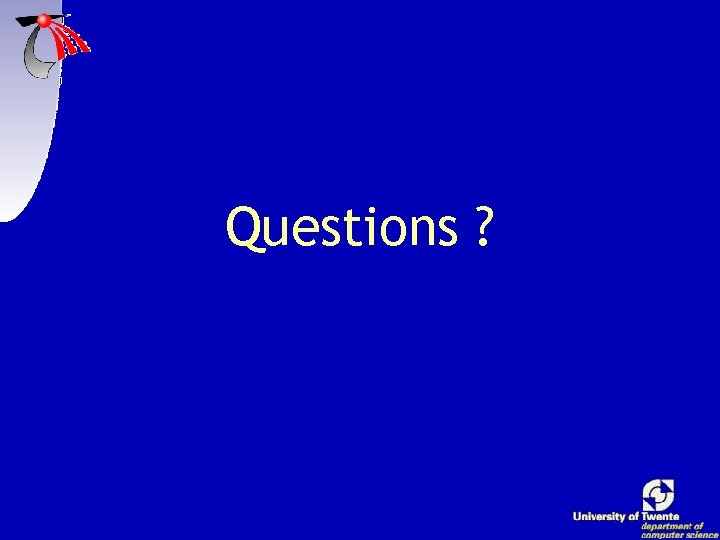
Questions ?
Arjan van hessen
Arjan van hessen
Arjan van der horst
Dr tizedes franciska debrecen
Franciska de jong
Franciska jamies
Arjan hogewoning
Arjan koning
Arjan baan
Arjan verweij
Arjan veerman
Arjan verweij
Rettungsfähigkeit lehrer hessen
Hessisches amt für versorgung und soziales gießen
Jorge hessen
Kerncurriculum hessen geschichte
Präsentationsprüfung realschule hessen
Feuerwehrdienstvorschriften hessen
Governikus communicator download
Minderleistungen abitur hessen
Hessen fläche
Www.mauswiesel.de
Mittelständische beteiligungsgesellschaft hessen
Kultusministerium hessen fachoberschule
Lehrplan englisch hessen
Kleines schulbudget hessen
Vernunftkraft hessen
Fehlerindex hessen oberstufe
Mdk hessen kassel
Hessen matriisi
Technology part of speech
Match the tenses in direct and reported speech
Pure speech definition
Speach to the young