Introduction to Data Mining Why Mine Data Commercial
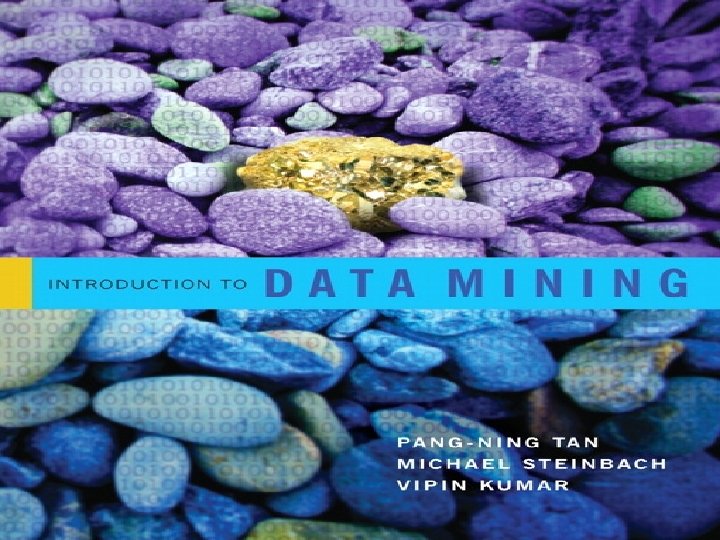
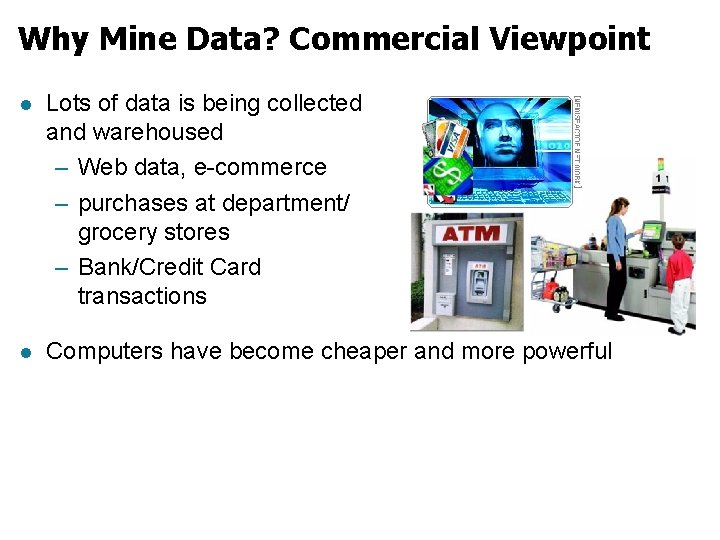
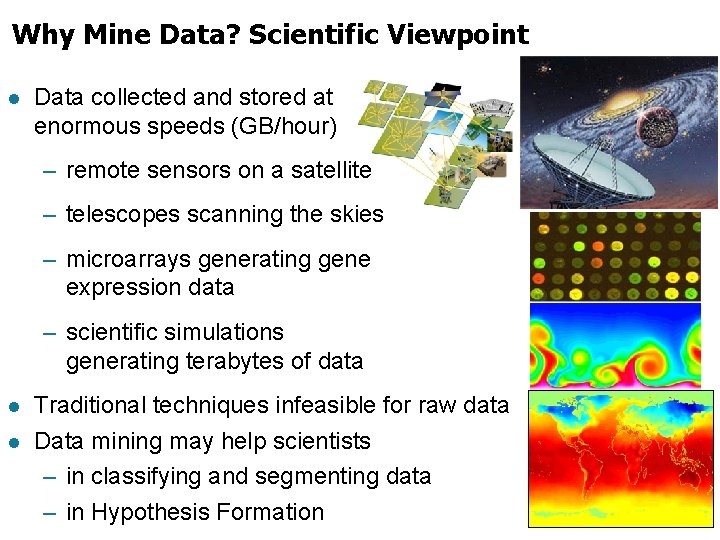
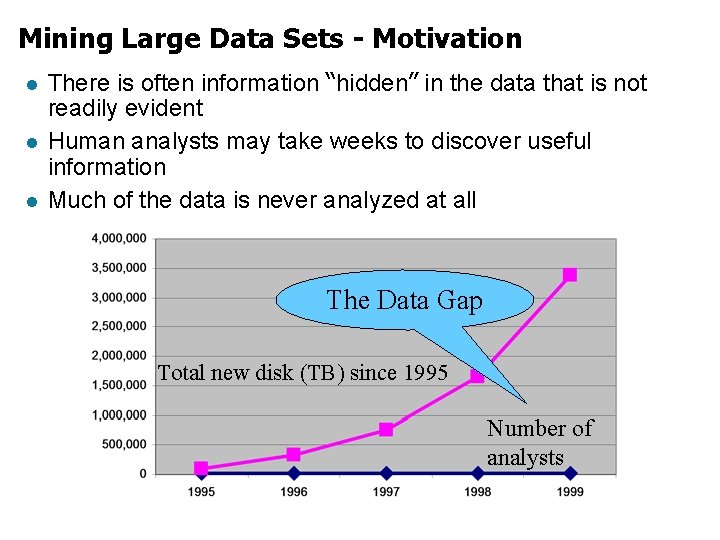
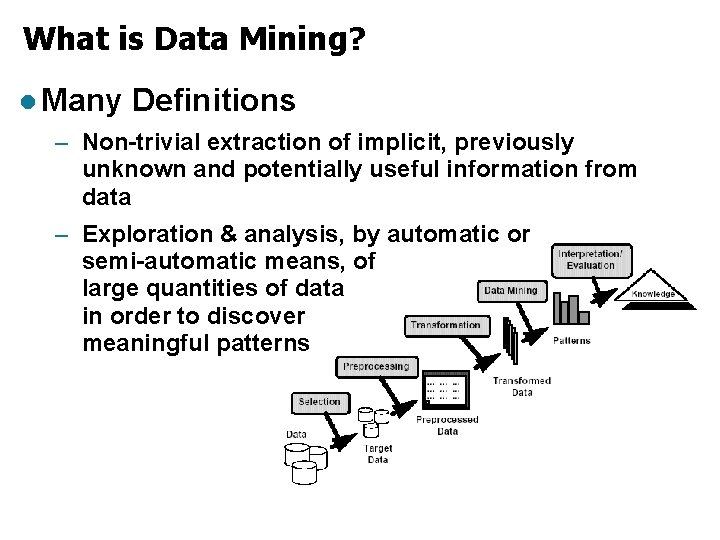
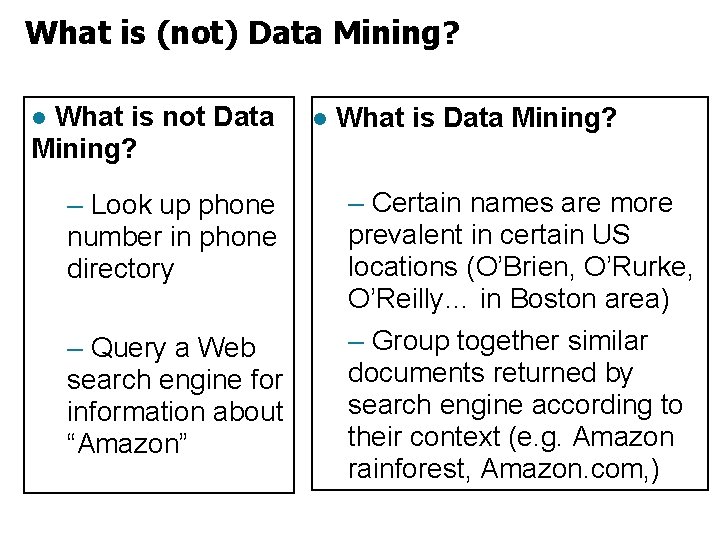
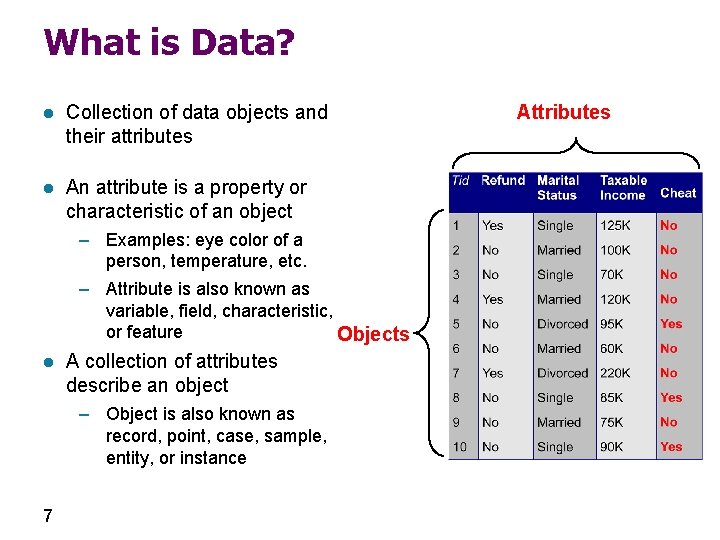
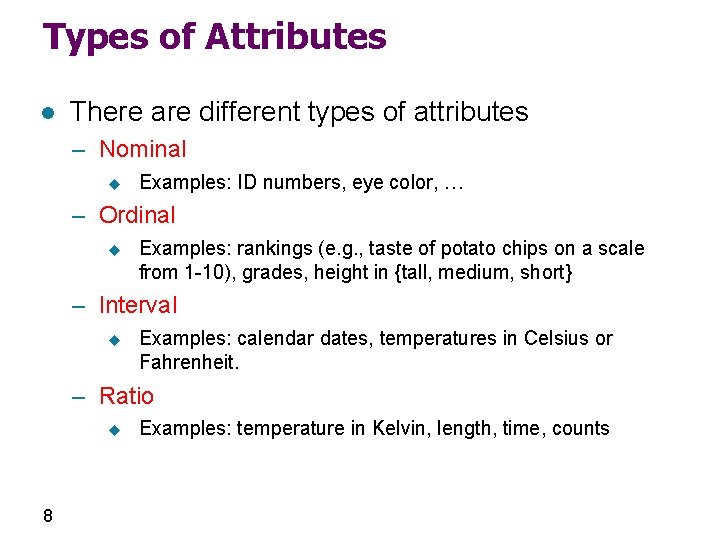
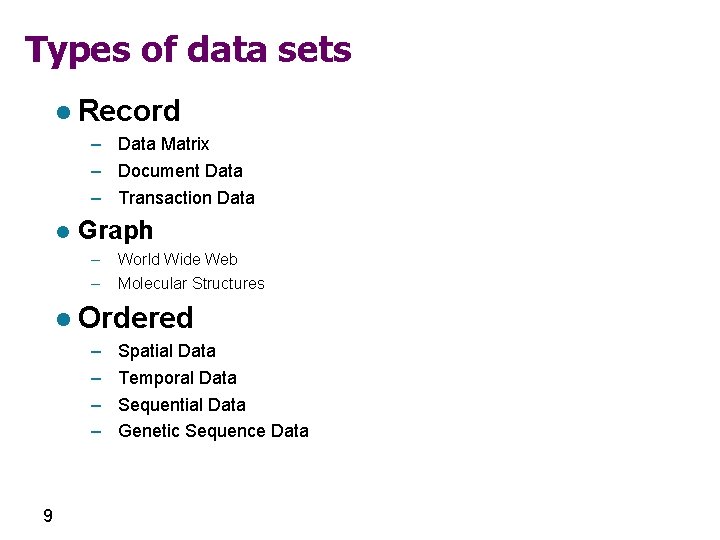
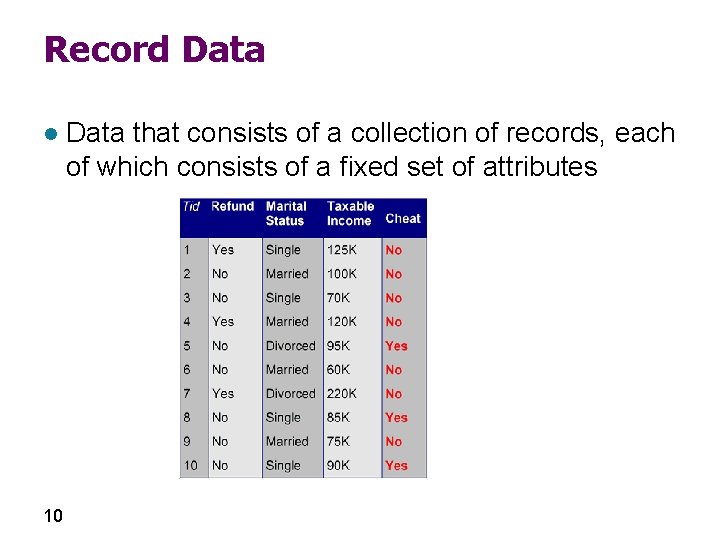
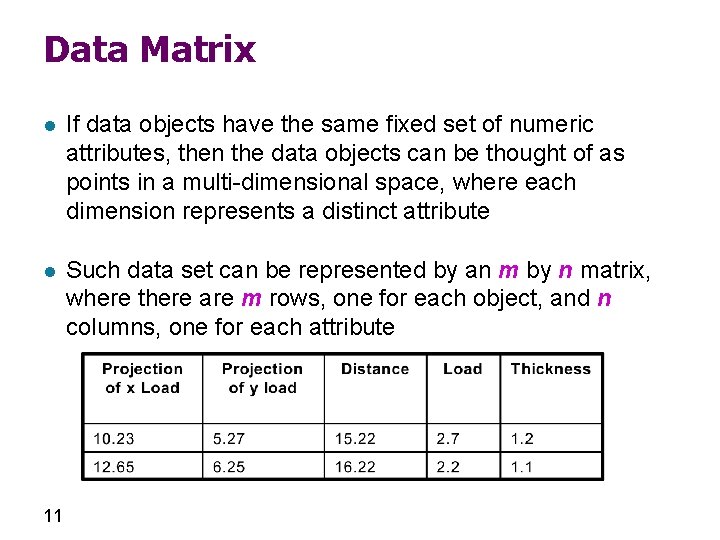
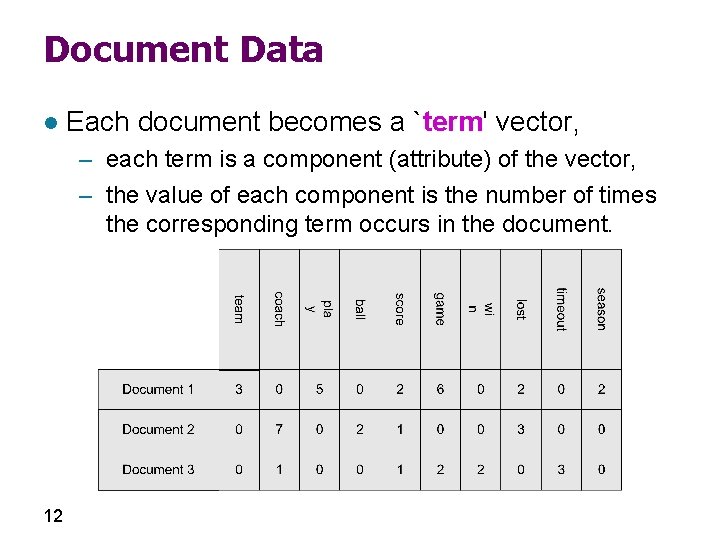
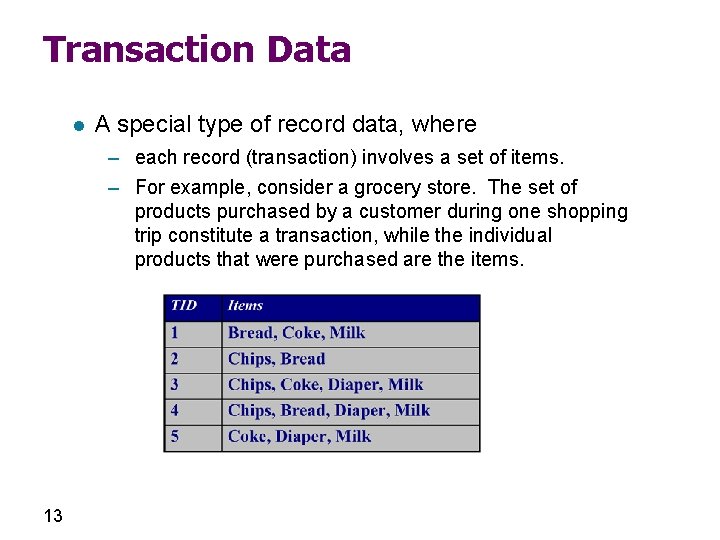
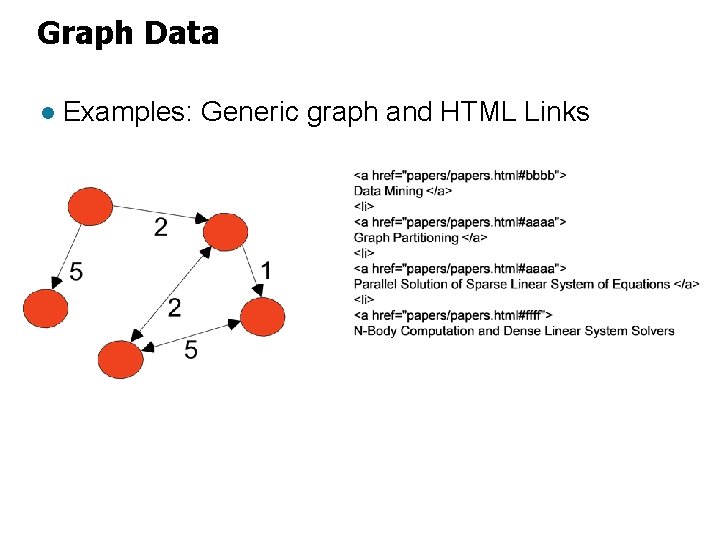
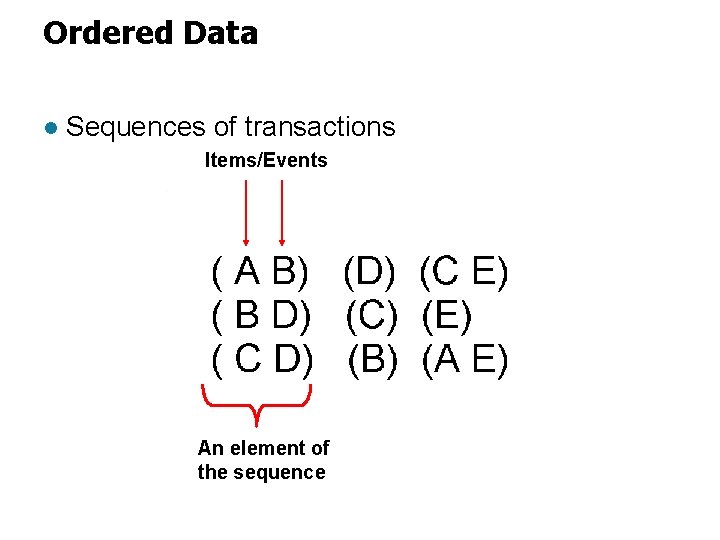
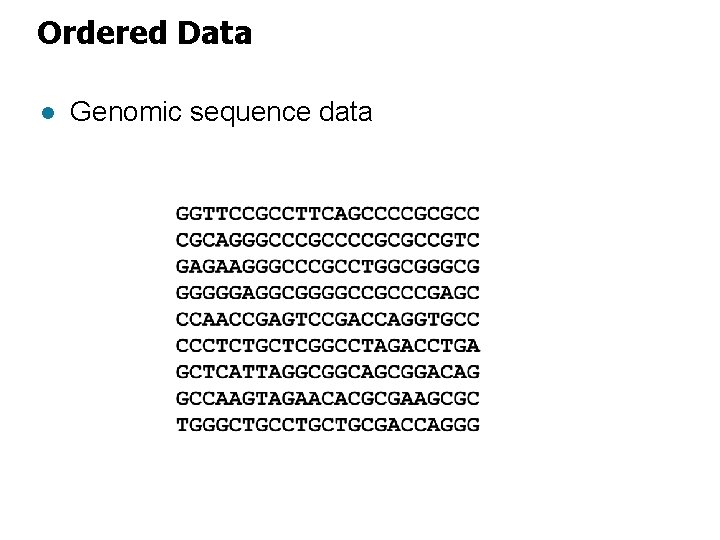
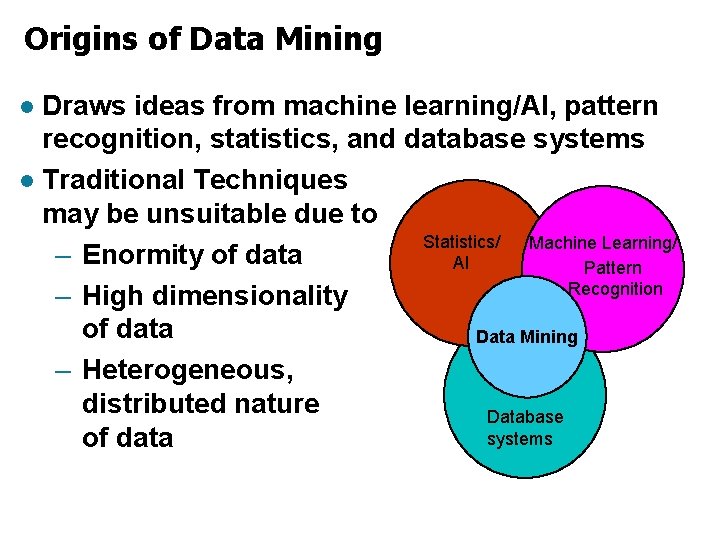
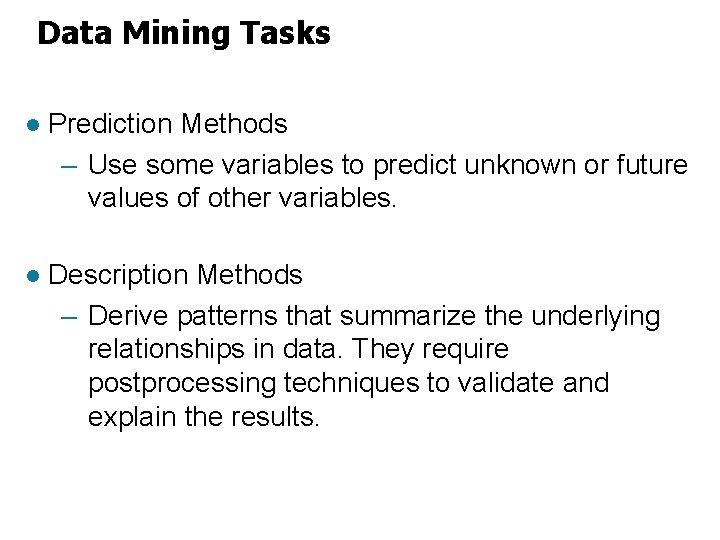
![Data Mining Tasks. . . Classification [Predictive] l Clustering [Descriptive] l Association Rule Discovery Data Mining Tasks. . . Classification [Predictive] l Clustering [Descriptive] l Association Rule Discovery](https://slidetodoc.com/presentation_image_h2/982aeaa0fde931c358ab9648e472bf78/image-19.jpg)
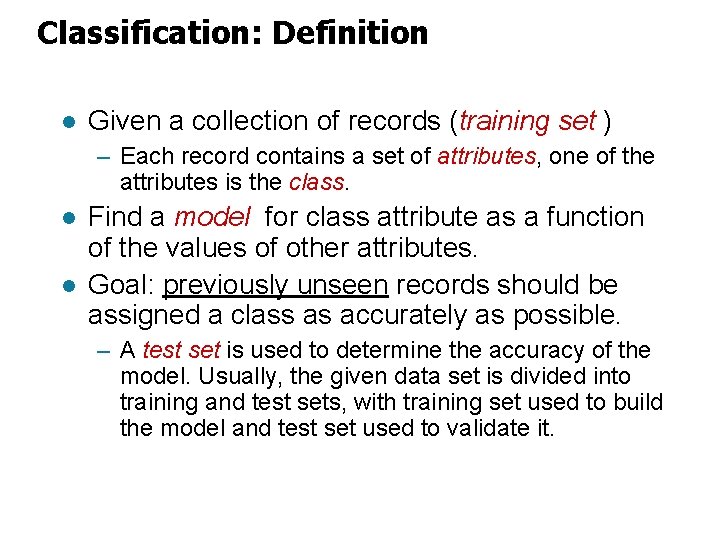
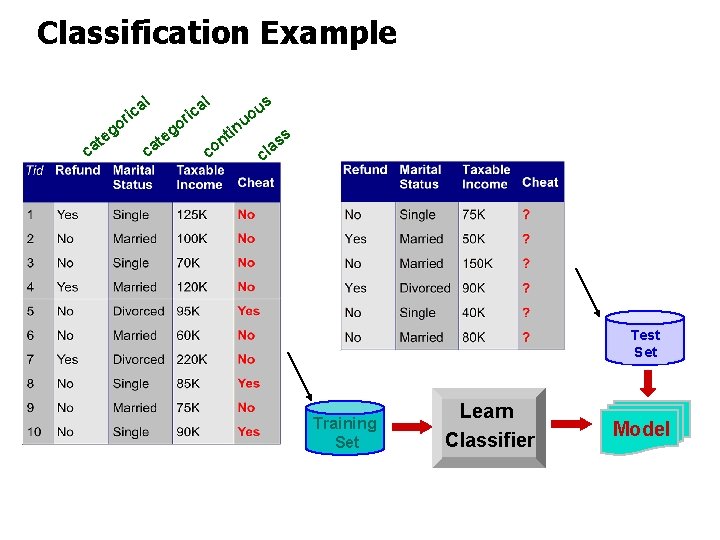
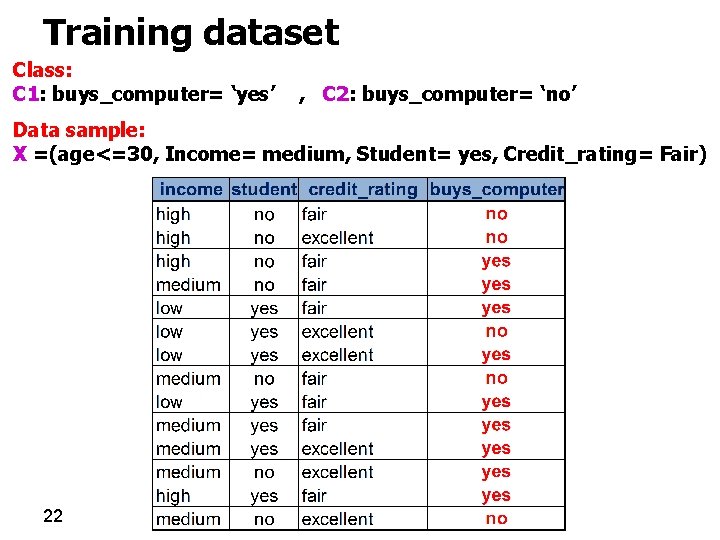
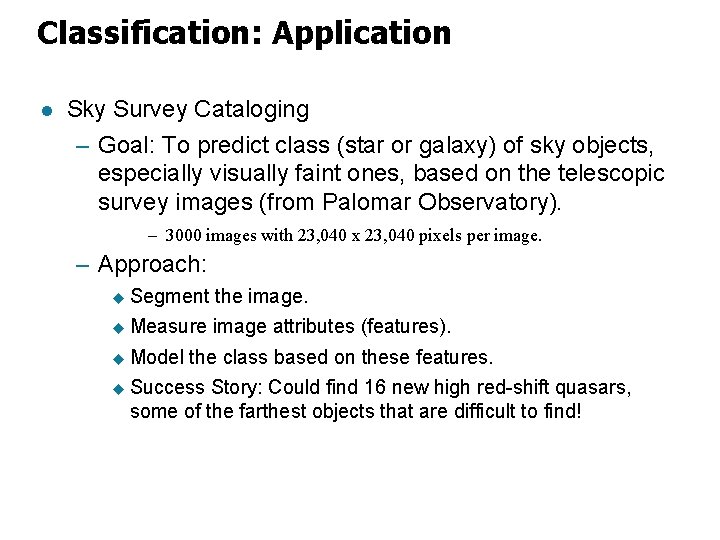
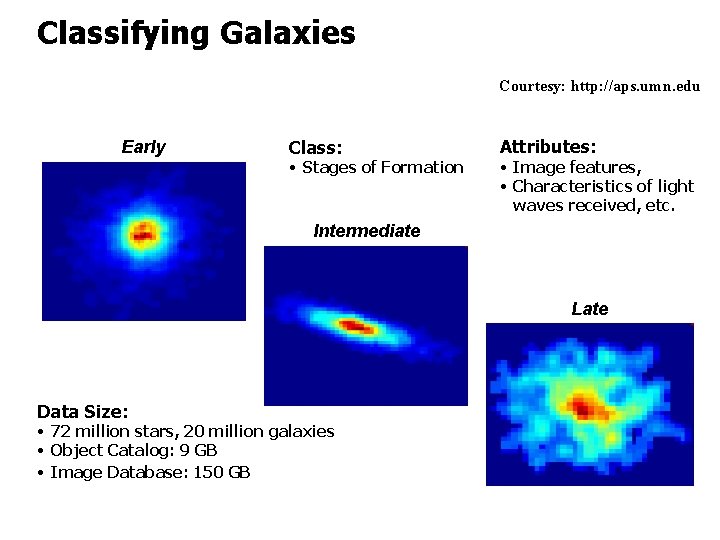
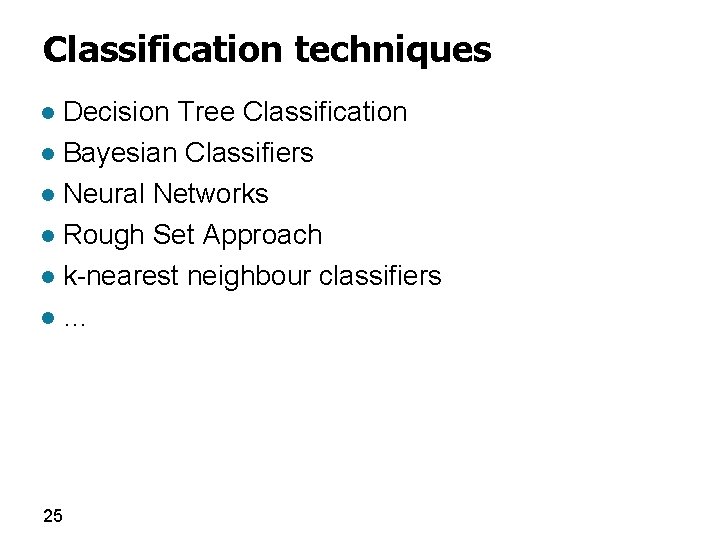
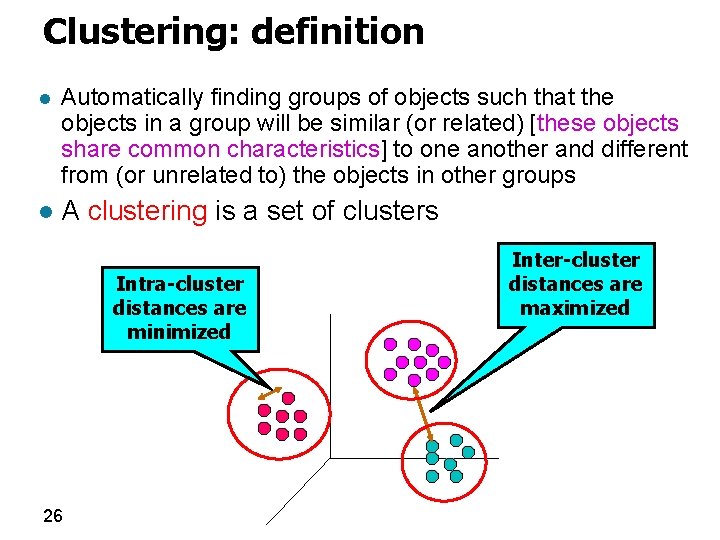
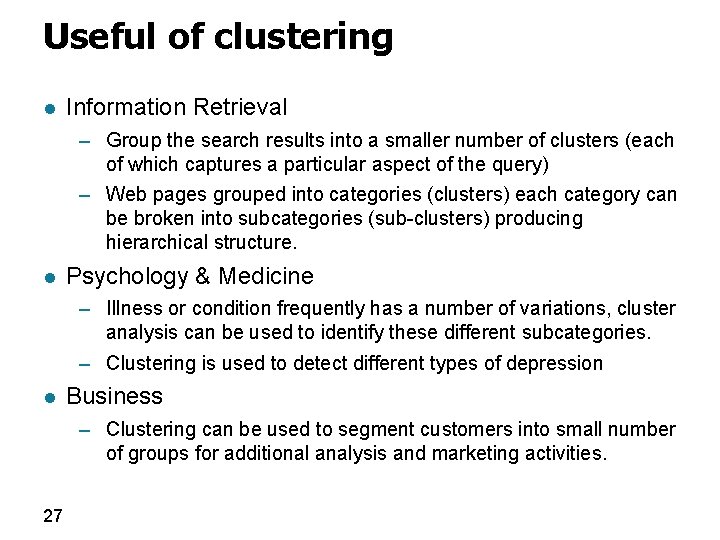
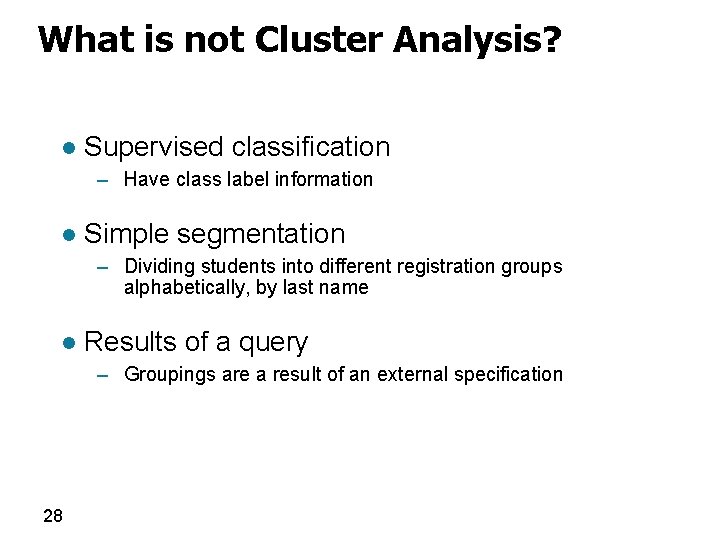
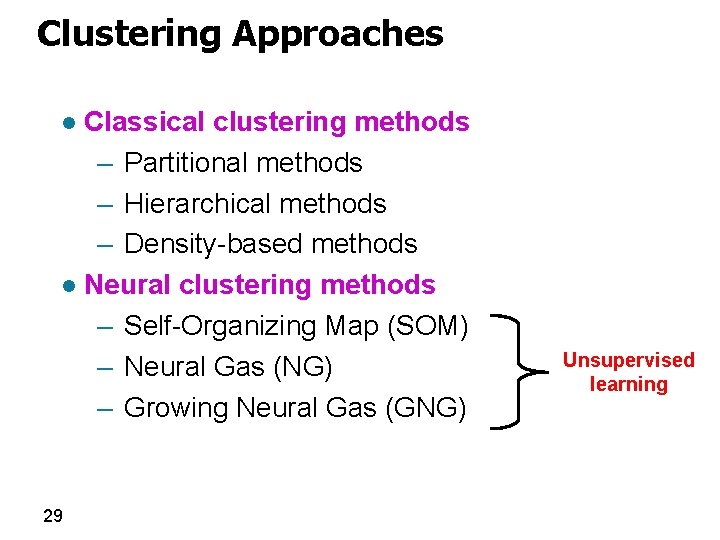
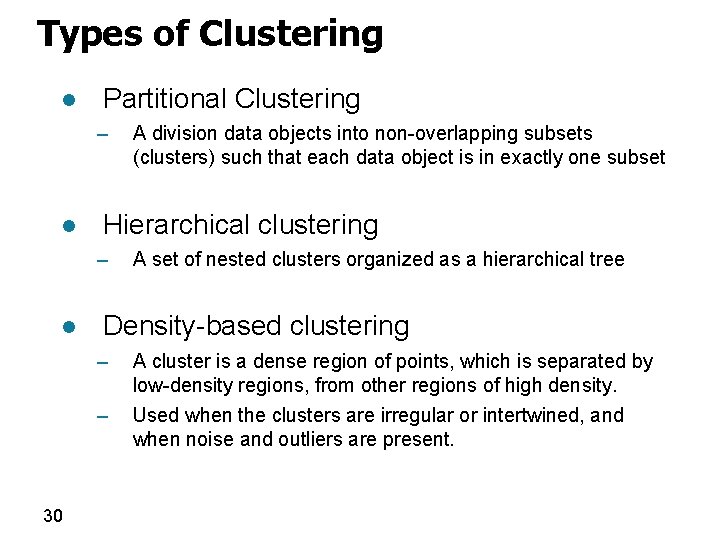
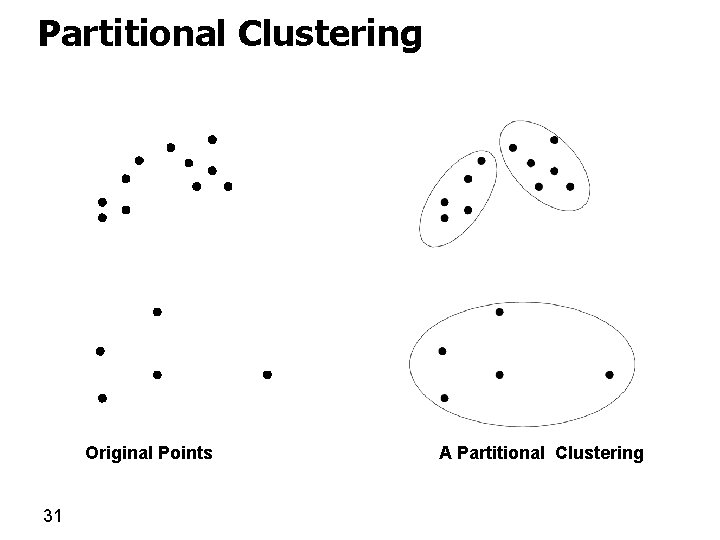
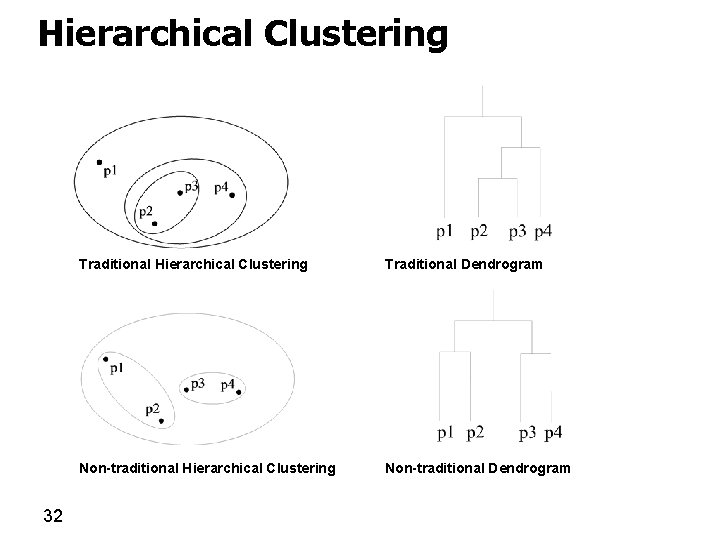
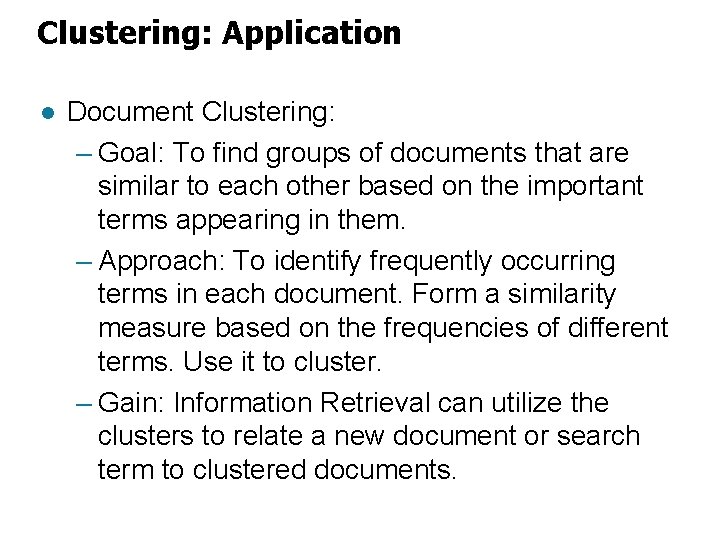
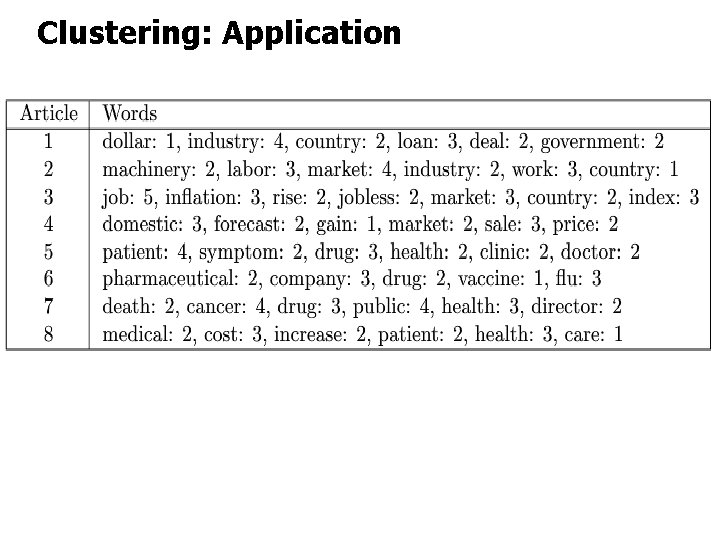
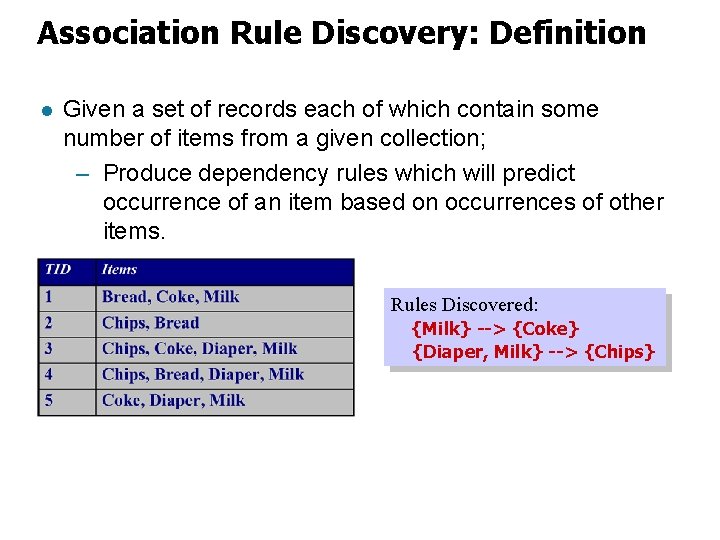
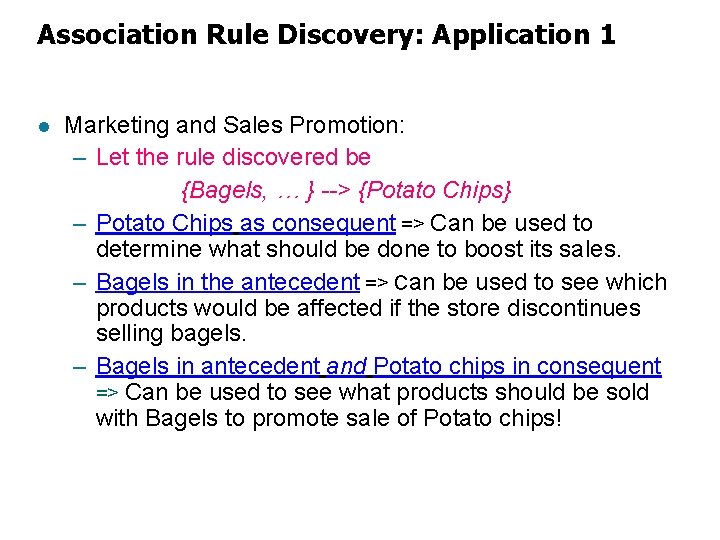
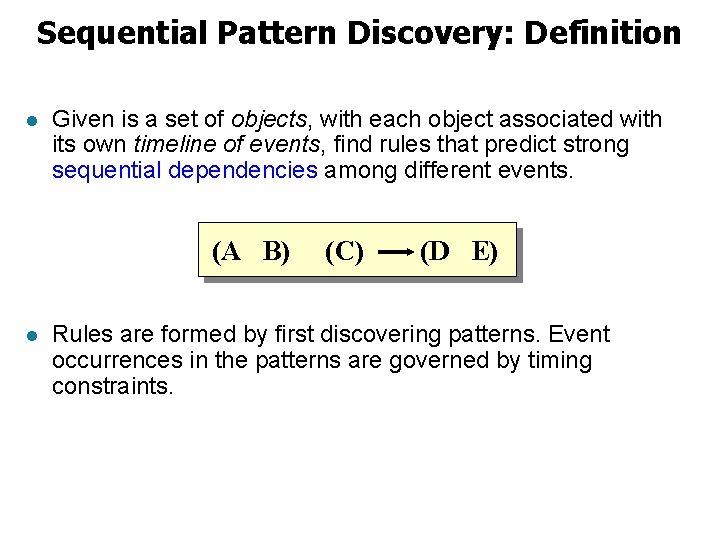
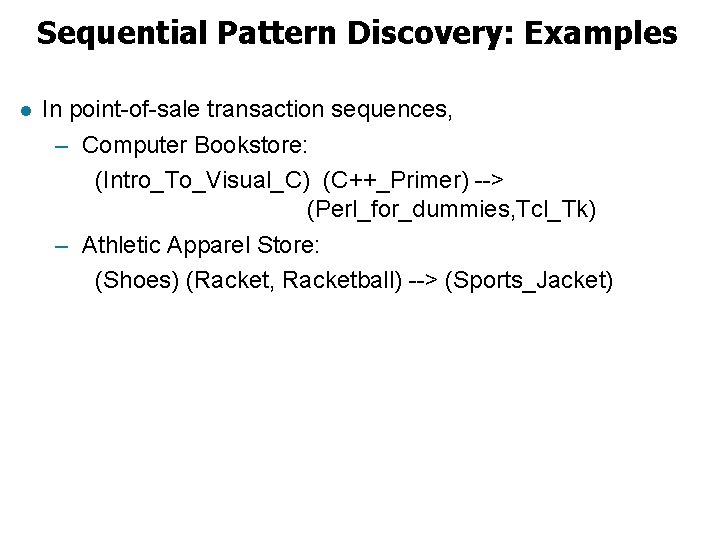
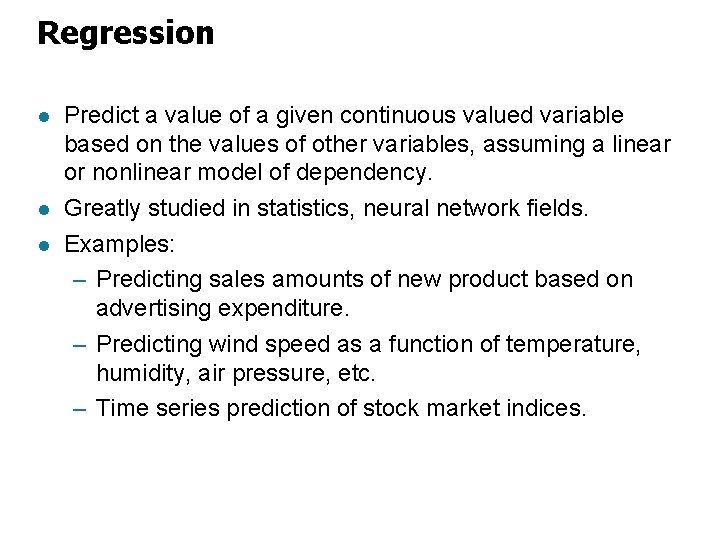
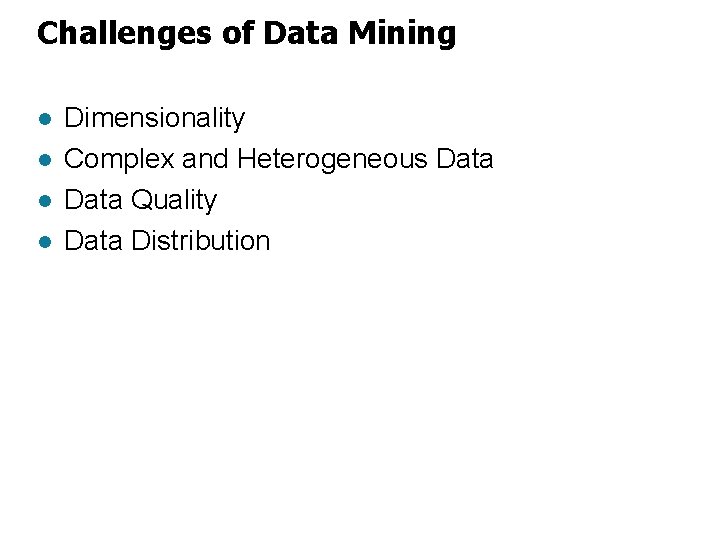
- Slides: 40
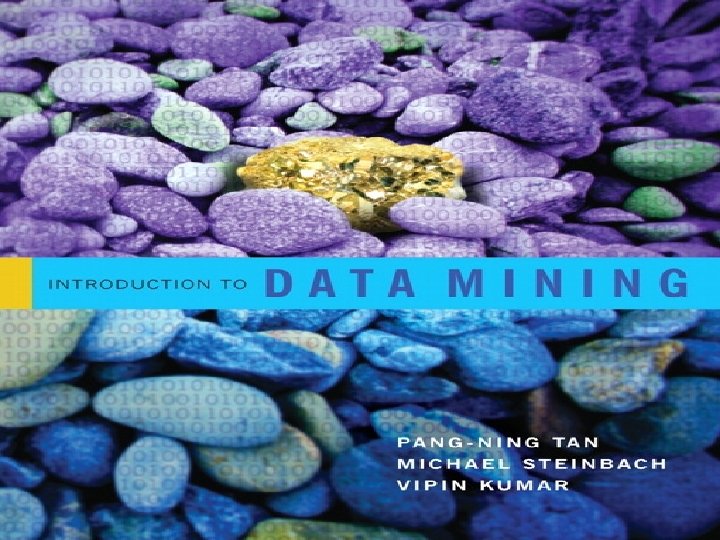
Introduction to Data Mining
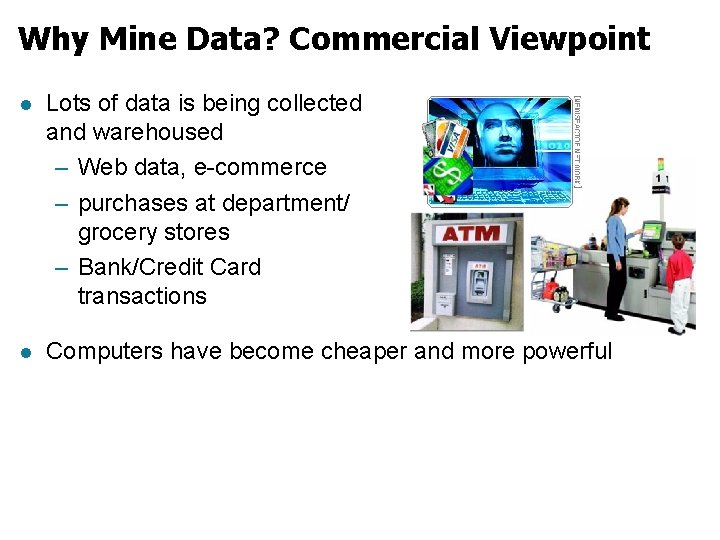
Why Mine Data? Commercial Viewpoint l Lots of data is being collected and warehoused – Web data, e-commerce – purchases at department/ grocery stores – Bank/Credit Card transactions l Computers have become cheaper and more powerful
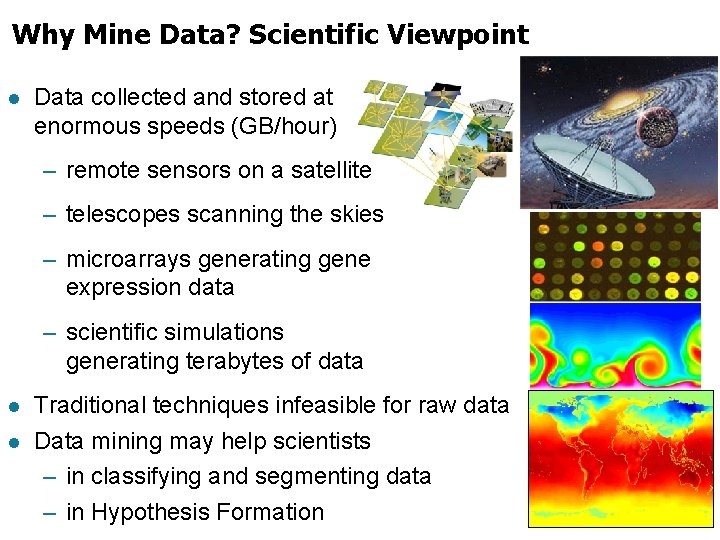
Why Mine Data? Scientific Viewpoint l Data collected and stored at enormous speeds (GB/hour) – remote sensors on a satellite – telescopes scanning the skies – microarrays generating gene expression data – scientific simulations generating terabytes of data l l Traditional techniques infeasible for raw data Data mining may help scientists – in classifying and segmenting data – in Hypothesis Formation
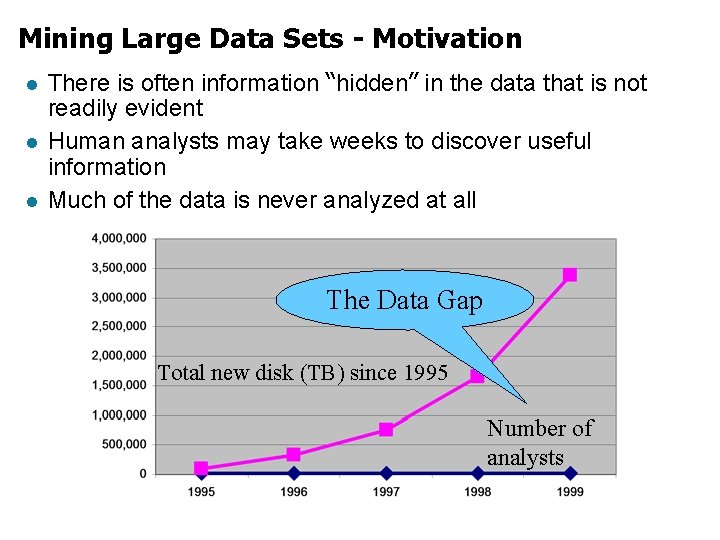
Mining Large Data Sets - Motivation l l l There is often information “hidden” in the data that is not readily evident Human analysts may take weeks to discover useful information Much of the data is never analyzed at all The Data Gap Total new disk (TB) since 1995 Number of analysts
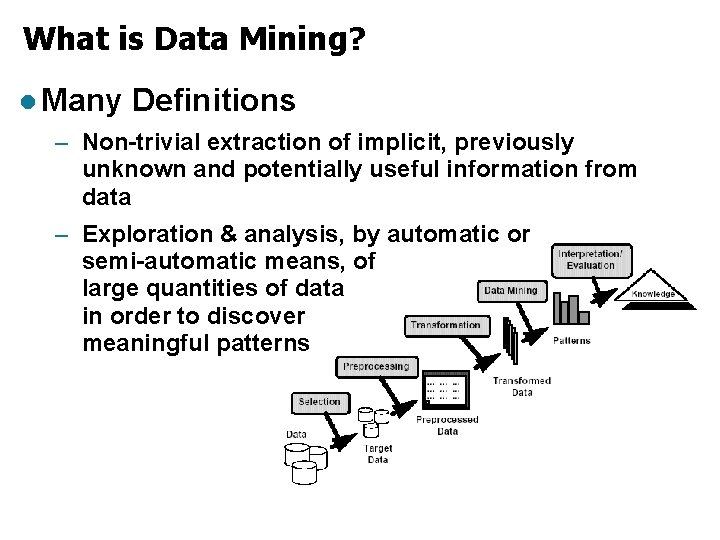
What is Data Mining? l Many Definitions – Non-trivial extraction of implicit, previously unknown and potentially useful information from data – Exploration & analysis, by automatic or semi-automatic means, of large quantities of data in order to discover meaningful patterns
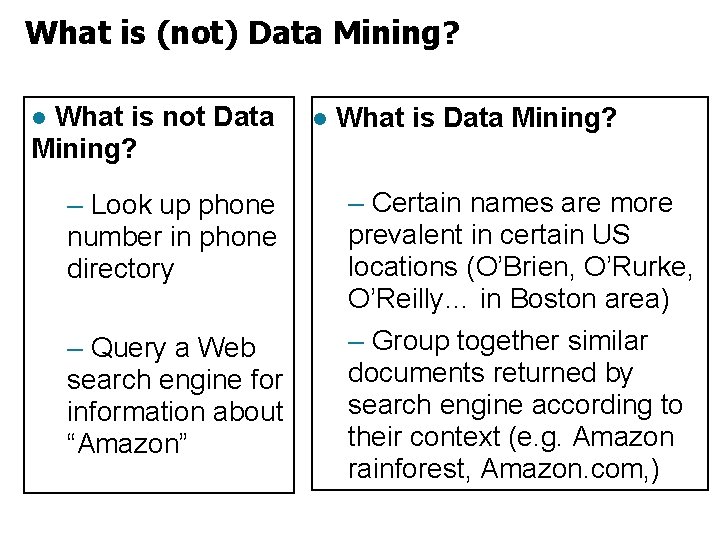
What is (not) Data Mining? What is not Data Mining? l – Look up phone number in phone directory – Query a Web search engine for information about “Amazon” l What is Data Mining? – Certain names are more prevalent in certain US locations (O’Brien, O’Rurke, O’Reilly… in Boston area) – Group together similar documents returned by search engine according to their context (e. g. Amazon rainforest, Amazon. com, )
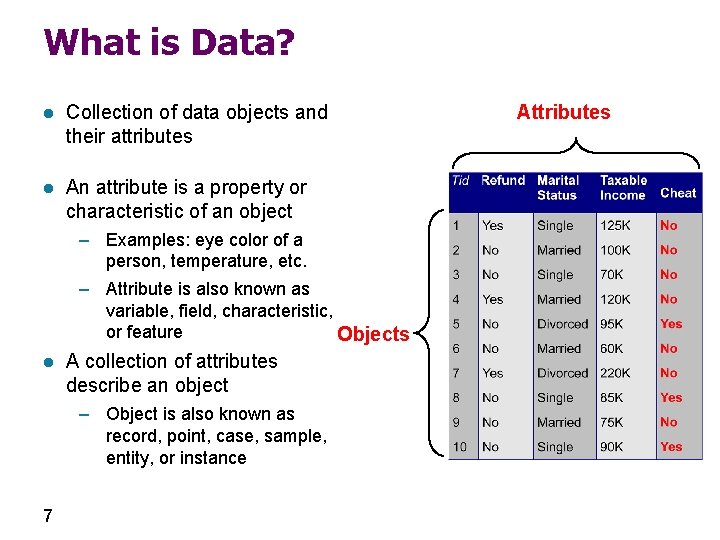
What is Data? l Collection of data objects and their attributes l An attribute is a property or characteristic of an object – Examples: eye color of a person, temperature, etc. – Attribute is also known as variable, field, characteristic, or feature Objects l A collection of attributes describe an object – Object is also known as record, point, case, sample, entity, or instance 7 Attributes
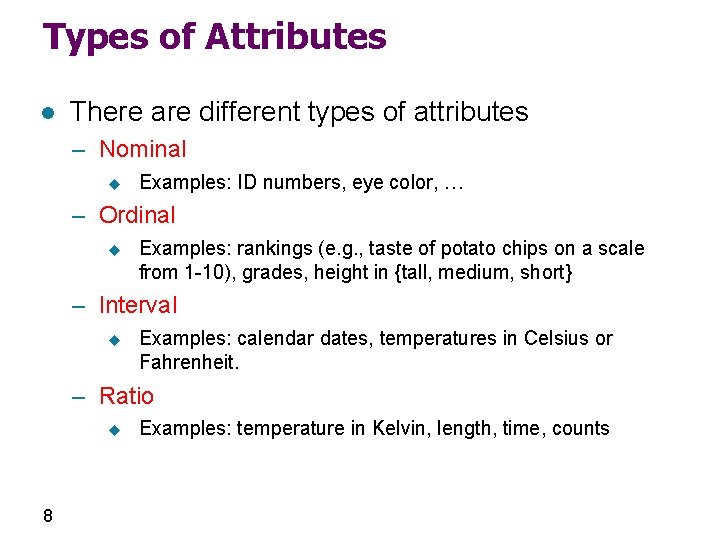
Types of Attributes l There are different types of attributes – Nominal u Examples: ID numbers, eye color, … – Ordinal u Examples: rankings (e. g. , taste of potato chips on a scale from 1 -10), grades, height in {tall, medium, short} – Interval u Examples: calendar dates, temperatures in Celsius or Fahrenheit. – Ratio u 8 Examples: temperature in Kelvin, length, time, counts
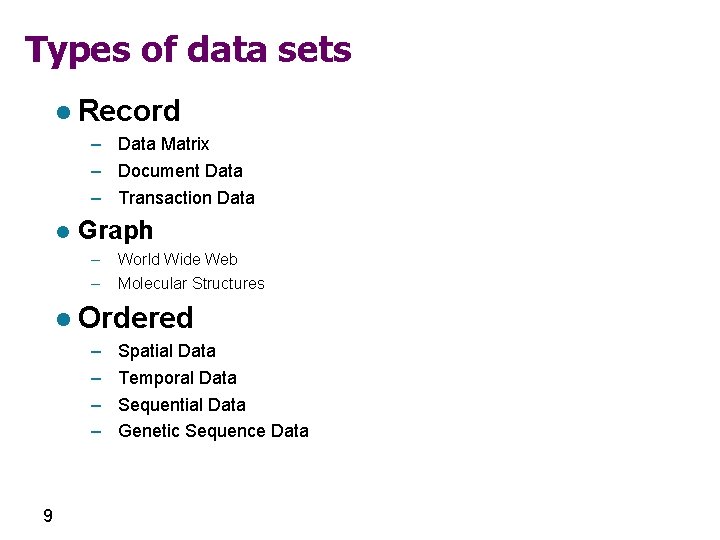
Types of data sets l Record – Data Matrix – Document Data – Transaction Data l Graph – – World Wide Web Molecular Structures l Ordered – – 9 Spatial Data Temporal Data Sequential Data Genetic Sequence Data
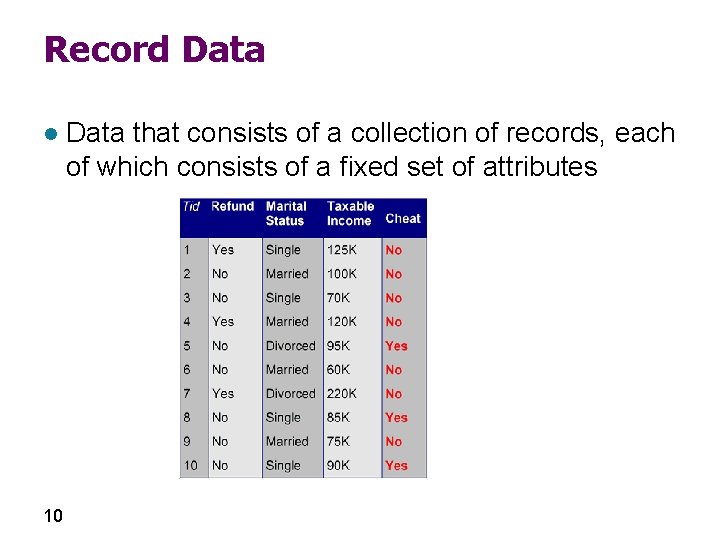
Record Data l 10 Data that consists of a collection of records, each of which consists of a fixed set of attributes
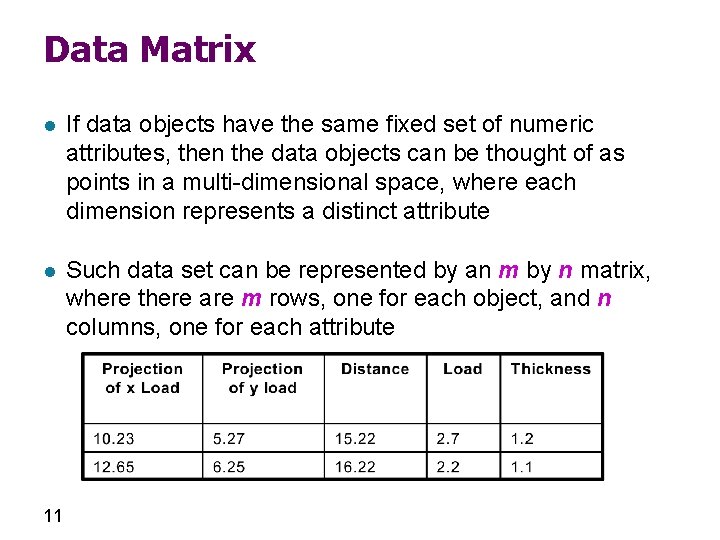
Data Matrix l If data objects have the same fixed set of numeric attributes, then the data objects can be thought of as points in a multi-dimensional space, where each dimension represents a distinct attribute l Such data set can be represented by an m by n matrix, where there are m rows, one for each object, and n columns, one for each attribute 11
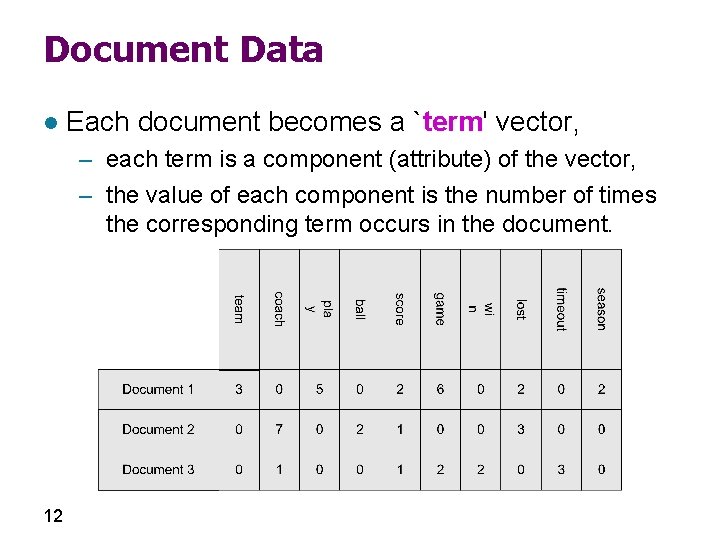
Document Data l Each document becomes a `term' vector, – each term is a component (attribute) of the vector, – the value of each component is the number of times the corresponding term occurs in the document. 12
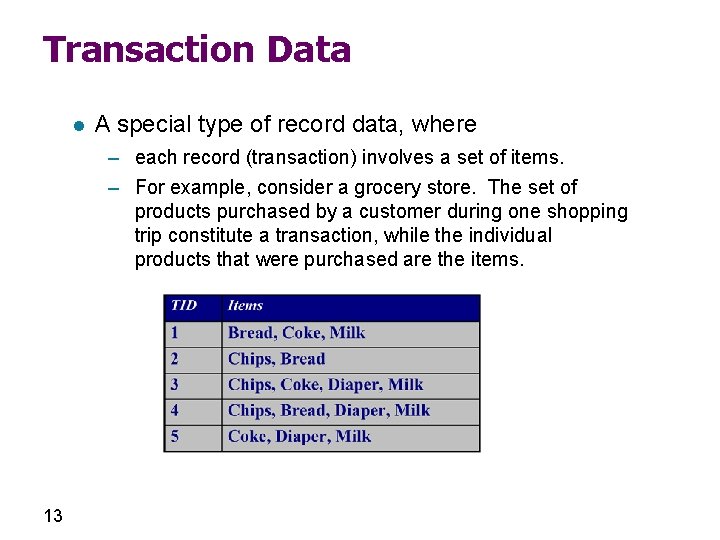
Transaction Data l A special type of record data, where – each record (transaction) involves a set of items. – For example, consider a grocery store. The set of products purchased by a customer during one shopping trip constitute a transaction, while the individual products that were purchased are the items. 13
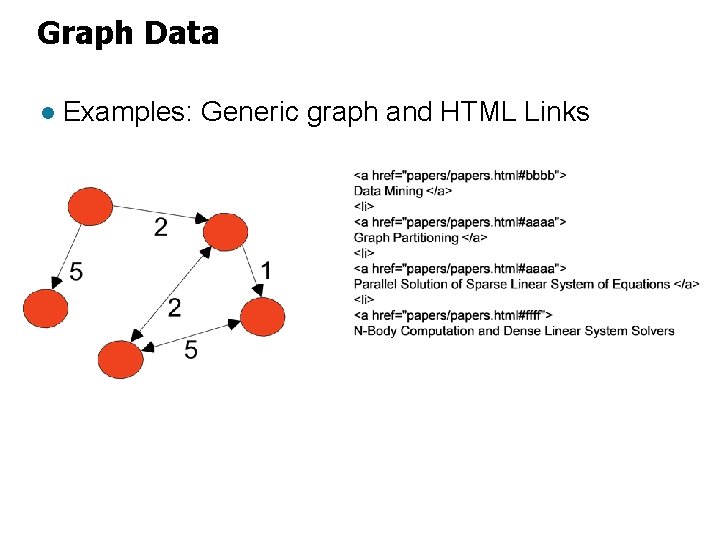
Graph Data l Examples: Generic graph and HTML Links
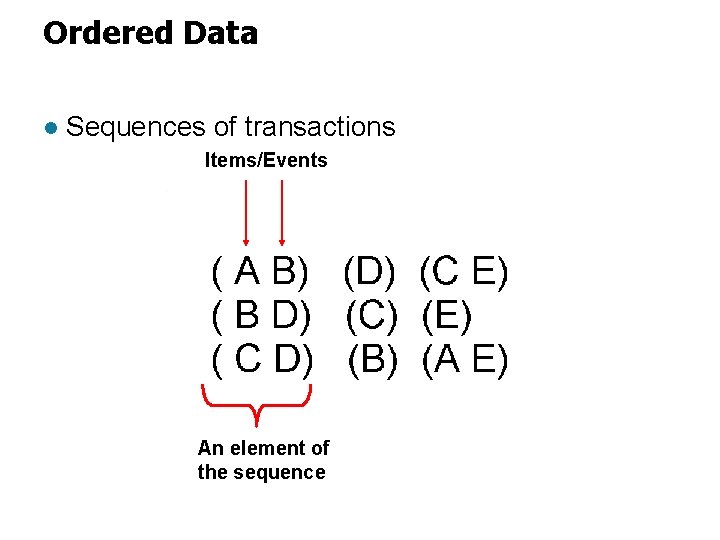
Ordered Data l Sequences of transactions Items/Events An element of the sequence
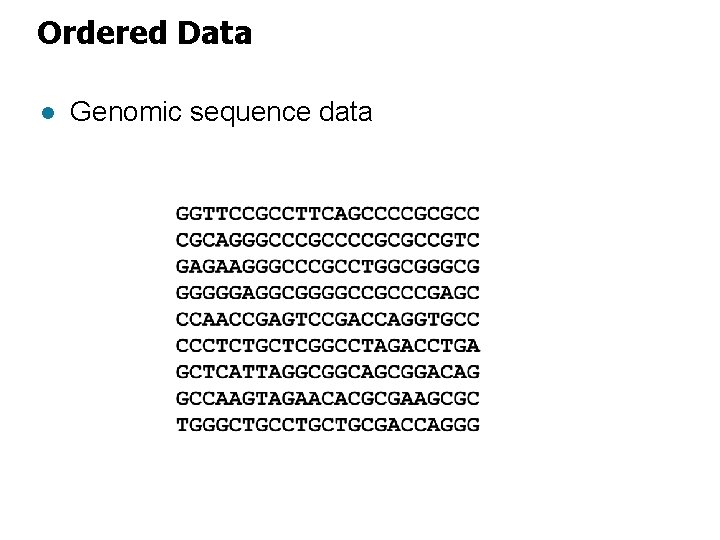
Ordered Data l Genomic sequence data
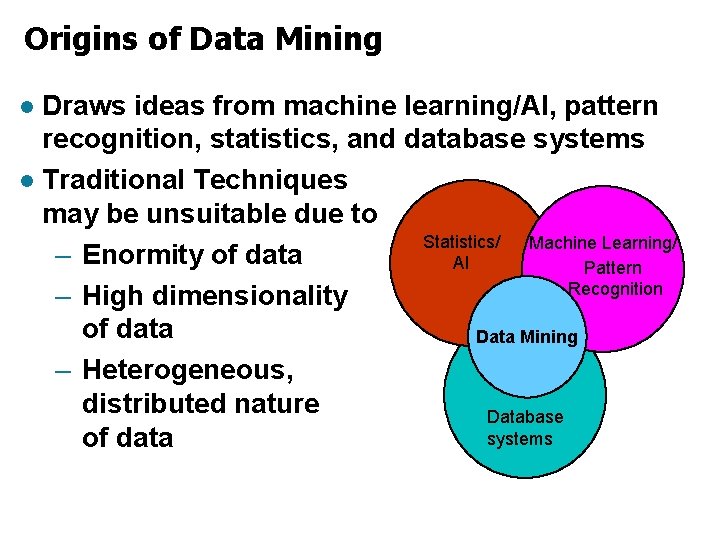
Origins of Data Mining Draws ideas from machine learning/AI, pattern recognition, statistics, and database systems l Traditional Techniques may be unsuitable due to Statistics/ Machine Learning/ – Enormity of data AI Pattern Recognition – High dimensionality of data Data Mining – Heterogeneous, distributed nature Database systems of data l
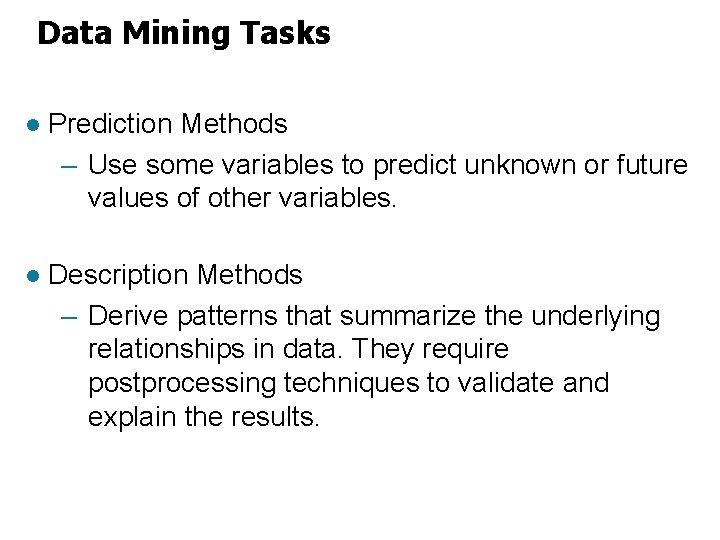
Data Mining Tasks l Prediction Methods – Use some variables to predict unknown or future values of other variables. l Description Methods – Derive patterns that summarize the underlying relationships in data. They require postprocessing techniques to validate and explain the results.
![Data Mining Tasks Classification Predictive l Clustering Descriptive l Association Rule Discovery Data Mining Tasks. . . Classification [Predictive] l Clustering [Descriptive] l Association Rule Discovery](https://slidetodoc.com/presentation_image_h2/982aeaa0fde931c358ab9648e472bf78/image-19.jpg)
Data Mining Tasks. . . Classification [Predictive] l Clustering [Descriptive] l Association Rule Discovery [Descriptive] l Sequential Pattern Discovery [Descriptive] l Regression [Predictive] l
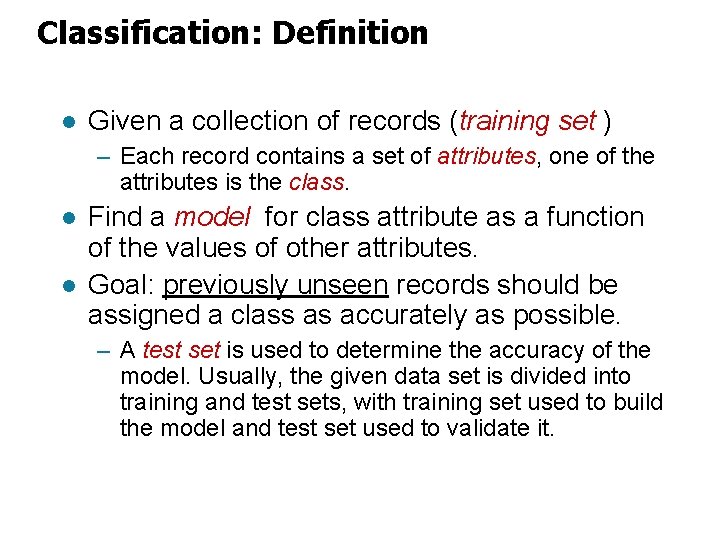
Classification: Definition l Given a collection of records (training set ) – Each record contains a set of attributes, one of the attributes is the class. l l Find a model for class attribute as a function of the values of other attributes. Goal: previously unseen records should be assigned a class as accurately as possible. – A test set is used to determine the accuracy of the model. Usually, the given data set is divided into training and test sets, with training set used to build the model and test set used to validate it.
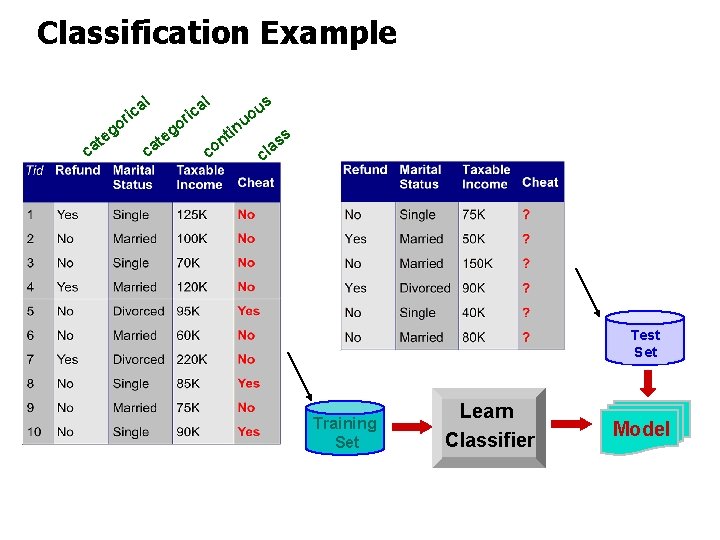
Classification Example l l a c i r o ca g te a c i r c in t on u uo s s as l c Test Set Training Set Learn Classifier Model
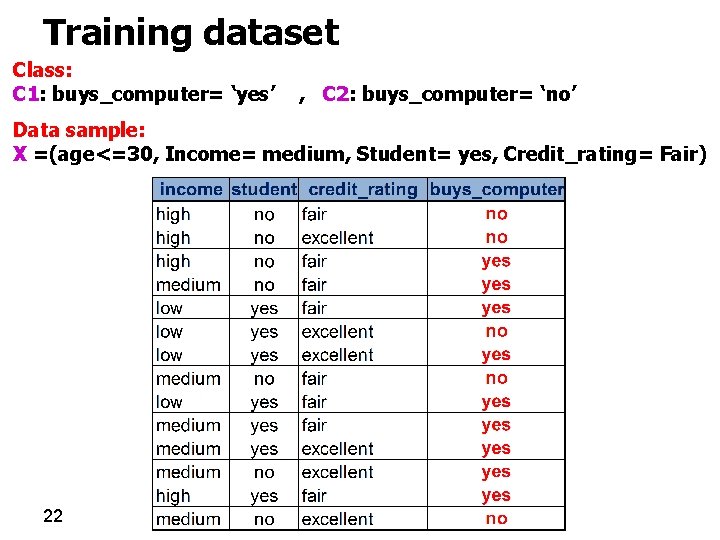
Training dataset Class: C 1: buys_computer= ‘yes’ , C 2: buys_computer= ‘no’ Data sample: X =(age<=30, Income= medium, Student= yes, Credit_rating= Fair) 22
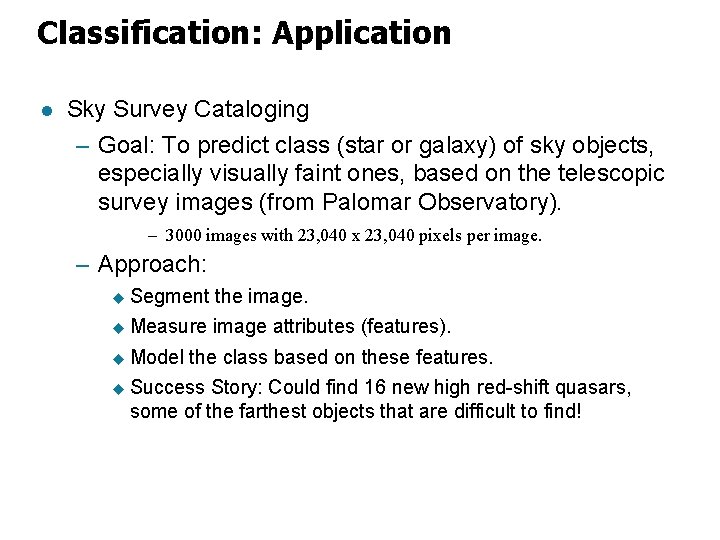
Classification: Application l Sky Survey Cataloging – Goal: To predict class (star or galaxy) of sky objects, especially visually faint ones, based on the telescopic survey images (from Palomar Observatory). – 3000 images with 23, 040 x 23, 040 pixels per image. – Approach: u Segment the image. u Measure image attributes (features). u Model the class based on these features. u Success Story: Could find 16 new high red-shift quasars, some of the farthest objects that are difficult to find!
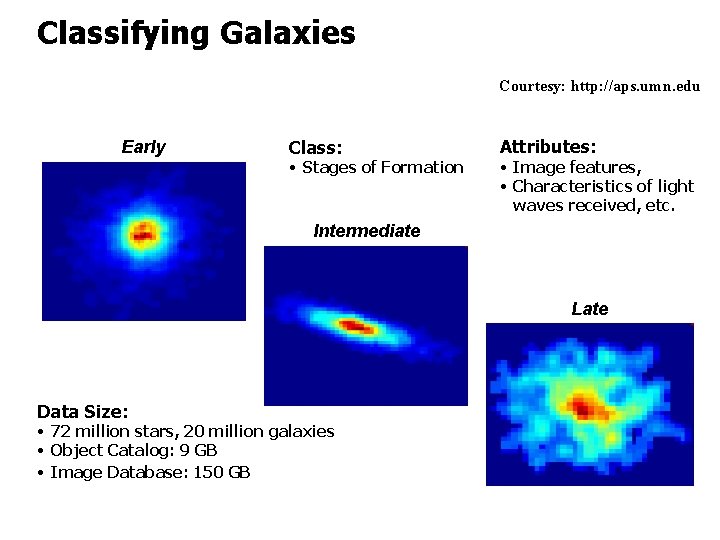
Classifying Galaxies Courtesy: http: //aps. umn. edu Early Class: • Stages of Formation Attributes: • Image features, • Characteristics of light waves received, etc. Intermediate Late Data Size: • 72 million stars, 20 million galaxies • Object Catalog: 9 GB • Image Database: 150 GB
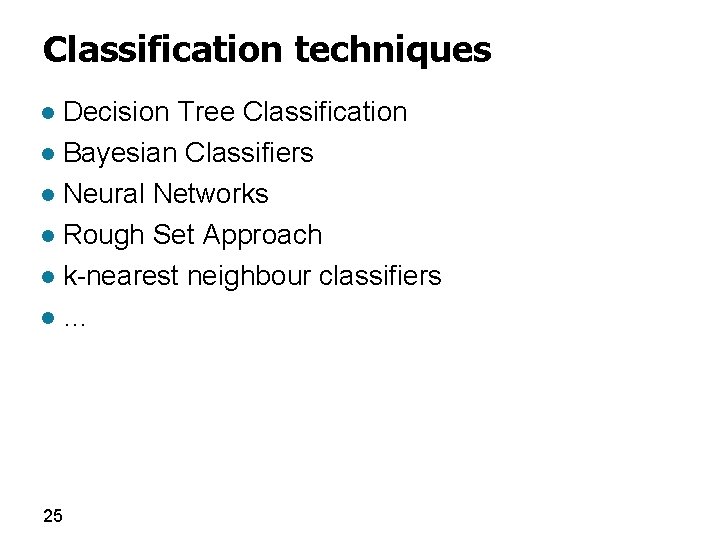
Classification techniques Decision Tree Classification l Bayesian Classifiers l Neural Networks l Rough Set Approach l k-nearest neighbour classifiers l… l 25
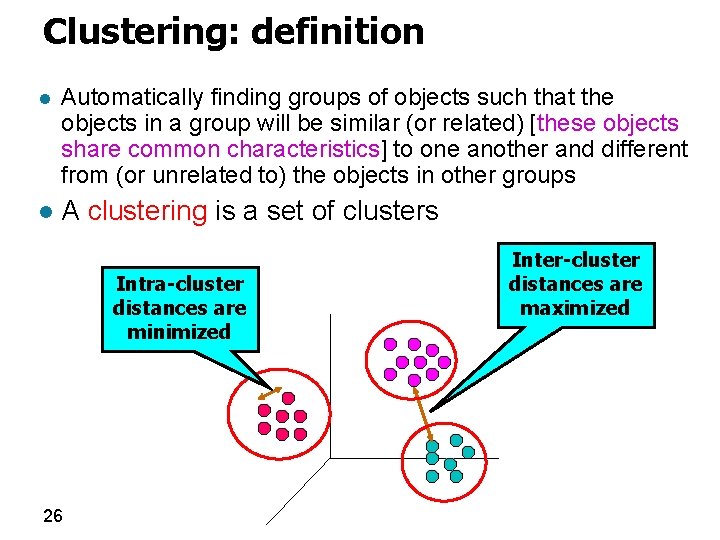
Clustering: definition l Automatically finding groups of objects such that the objects in a group will be similar (or related) [these objects share common characteristics] to one another and different from (or unrelated to) the objects in other groups l A clustering is a set of clusters Intra-cluster distances are minimized 26 Inter-cluster distances are maximized
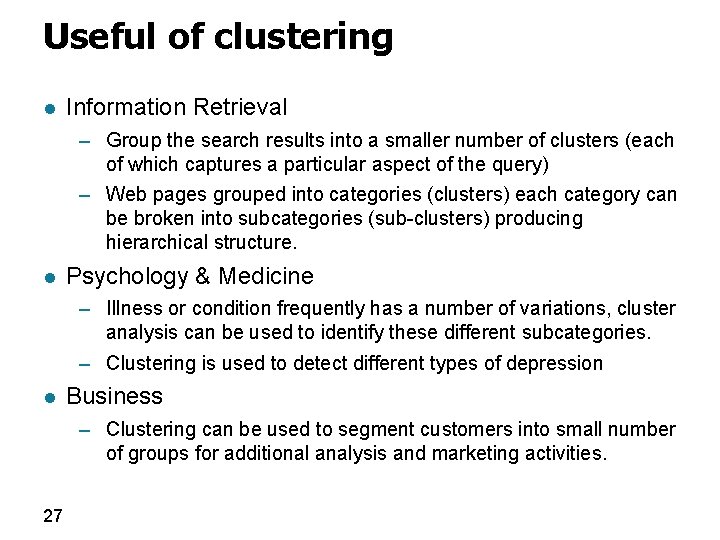
Useful of clustering l Information Retrieval – Group the search results into a smaller number of clusters (each of which captures a particular aspect of the query) – Web pages grouped into categories (clusters) each category can be broken into subcategories (sub-clusters) producing hierarchical structure. l Psychology & Medicine – Illness or condition frequently has a number of variations, cluster analysis can be used to identify these different subcategories. – Clustering is used to detect different types of depression l Business – Clustering can be used to segment customers into small number of groups for additional analysis and marketing activities. 27
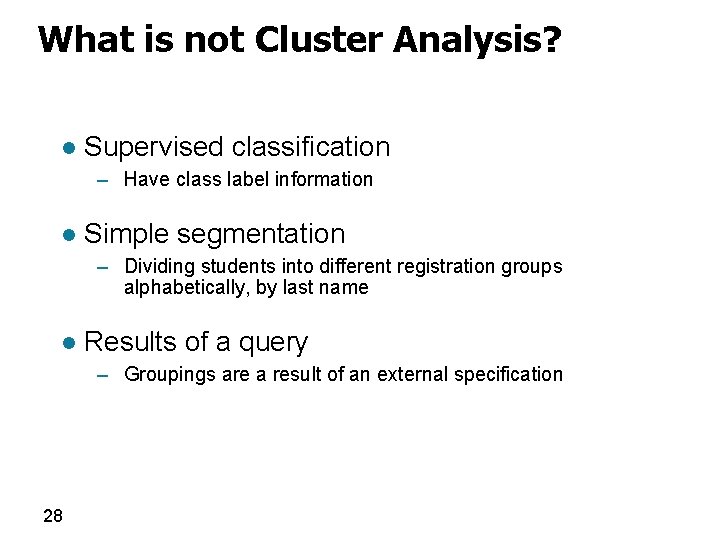
What is not Cluster Analysis? l Supervised classification – Have class label information l Simple segmentation – Dividing students into different registration groups alphabetically, by last name l Results of a query – Groupings are a result of an external specification 28
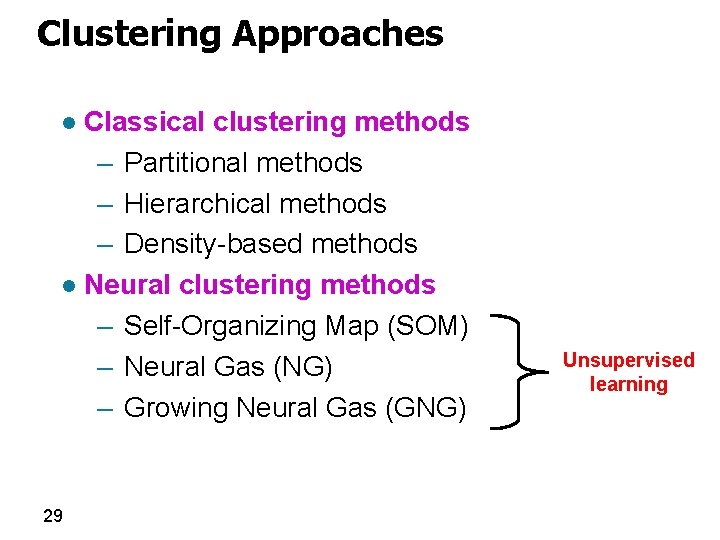
Clustering Approaches Classical clustering methods – Partitional methods – Hierarchical methods – Density-based methods l Neural clustering methods – Self-Organizing Map (SOM) – Neural Gas (NG) – Growing Neural Gas (GNG) l 29 Unsupervised learning
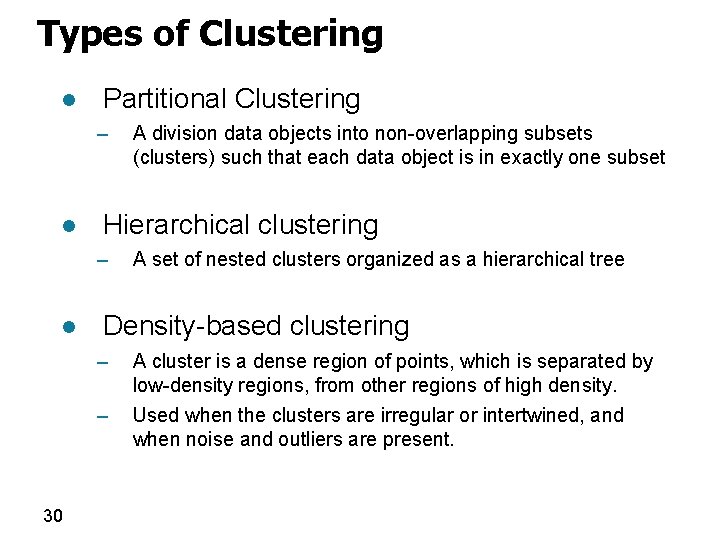
Types of Clustering l Partitional Clustering – l Hierarchical clustering – l 30 A division data objects into non-overlapping subsets (clusters) such that each data object is in exactly one subset A set of nested clusters organized as a hierarchical tree Density-based clustering – A cluster is a dense region of points, which is separated by low-density regions, from other regions of high density. – Used when the clusters are irregular or intertwined, and when noise and outliers are present.
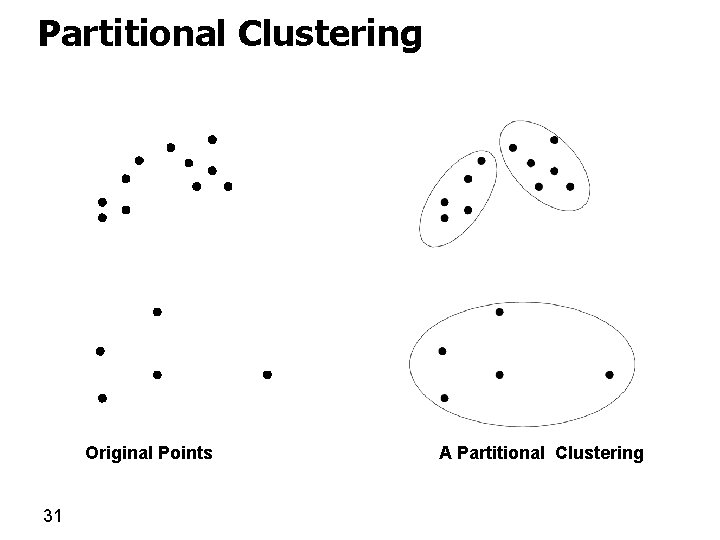
Partitional Clustering Original Points 31 A Partitional Clustering
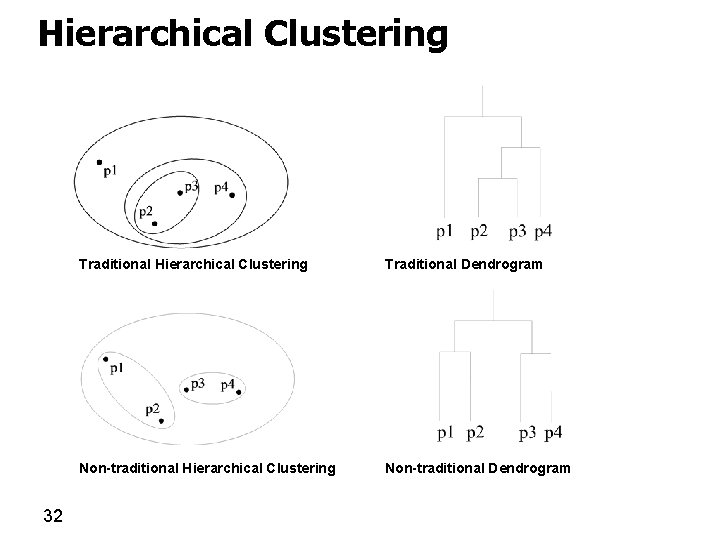
Hierarchical Clustering 32 Traditional Hierarchical Clustering Traditional Dendrogram Non-traditional Hierarchical Clustering Non-traditional Dendrogram
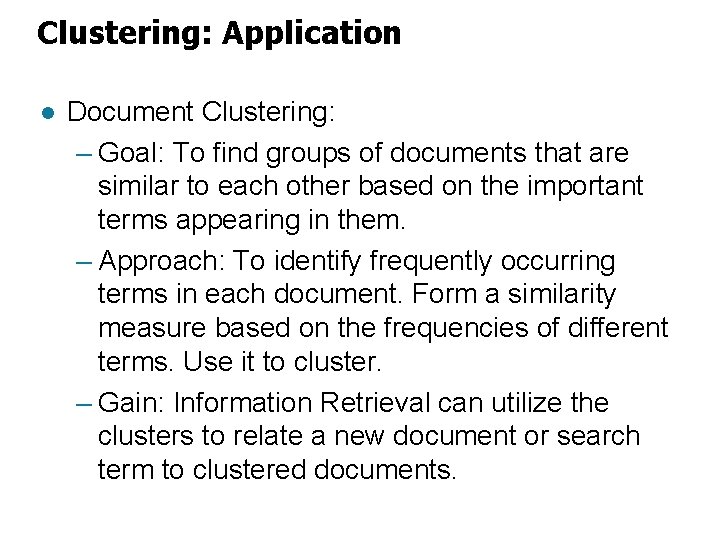
Clustering: Application l Document Clustering: – Goal: To find groups of documents that are similar to each other based on the important terms appearing in them. – Approach: To identify frequently occurring terms in each document. Form a similarity measure based on the frequencies of different terms. Use it to cluster. – Gain: Information Retrieval can utilize the clusters to relate a new document or search term to clustered documents.
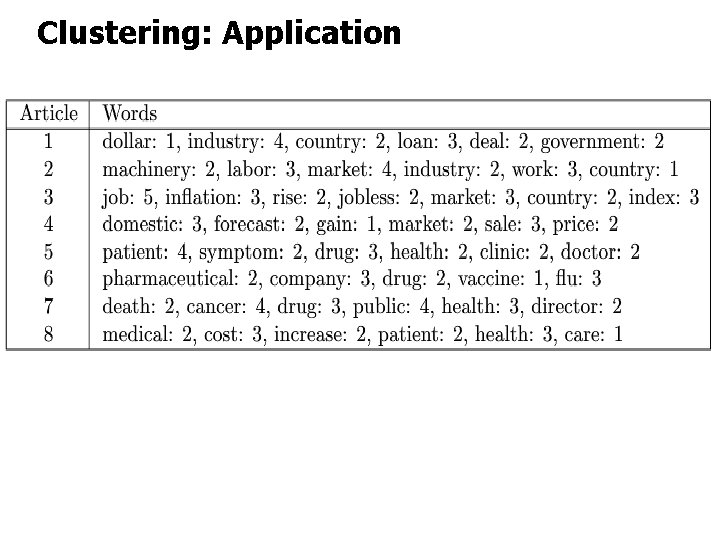
Clustering: Application
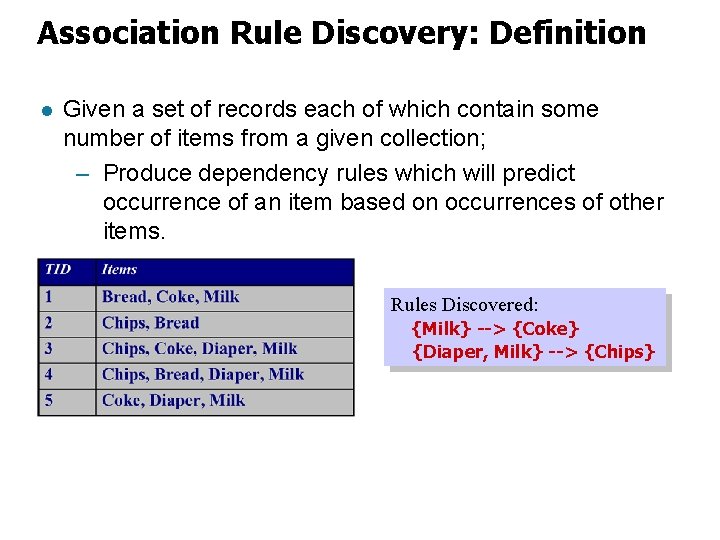
Association Rule Discovery: Definition l Given a set of records each of which contain some number of items from a given collection; – Produce dependency rules which will predict occurrence of an item based on occurrences of other items. Rules Discovered: {Milk} --> {Coke} {Diaper, Milk} --> {Chips}
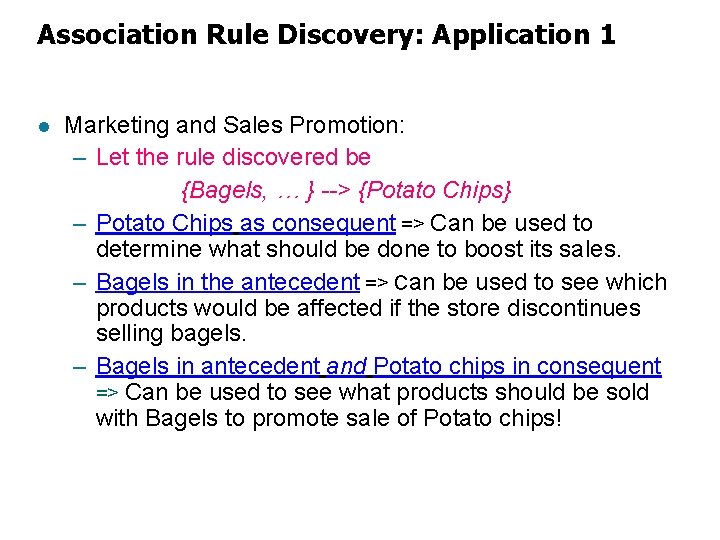
Association Rule Discovery: Application 1 l Marketing and Sales Promotion: – Let the rule discovered be {Bagels, … } --> {Potato Chips} – Potato Chips as consequent => Can be used to determine what should be done to boost its sales. – Bagels in the antecedent => Can be used to see which products would be affected if the store discontinues selling bagels. – Bagels in antecedent and Potato chips in consequent => Can be used to see what products should be sold with Bagels to promote sale of Potato chips!
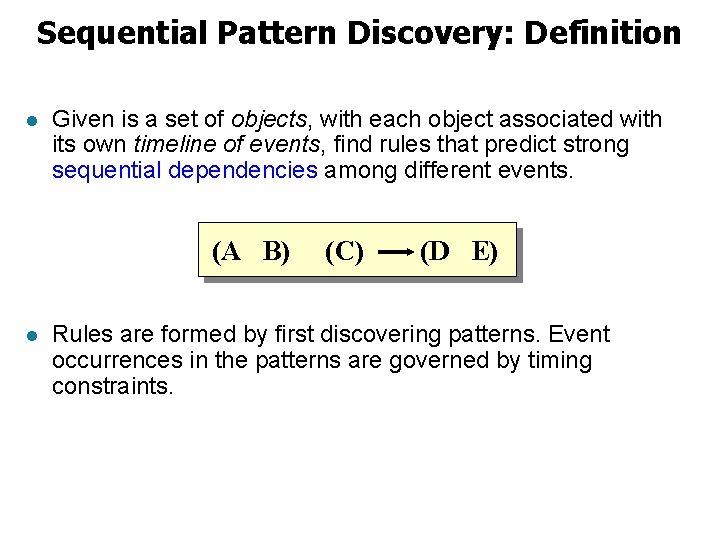
Sequential Pattern Discovery: Definition l Given is a set of objects, with each object associated with its own timeline of events, find rules that predict strong sequential dependencies among different events. (A B) l (C) (D E) Rules are formed by first discovering patterns. Event occurrences in the patterns are governed by timing constraints.
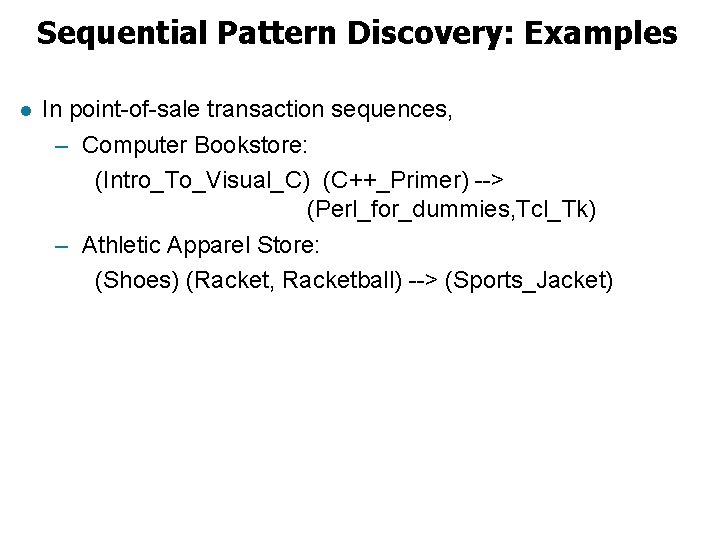
Sequential Pattern Discovery: Examples l In point-of-sale transaction sequences, – Computer Bookstore: (Intro_To_Visual_C) (C++_Primer) --> (Perl_for_dummies, Tcl_Tk) – Athletic Apparel Store: (Shoes) (Racket, Racketball) --> (Sports_Jacket)
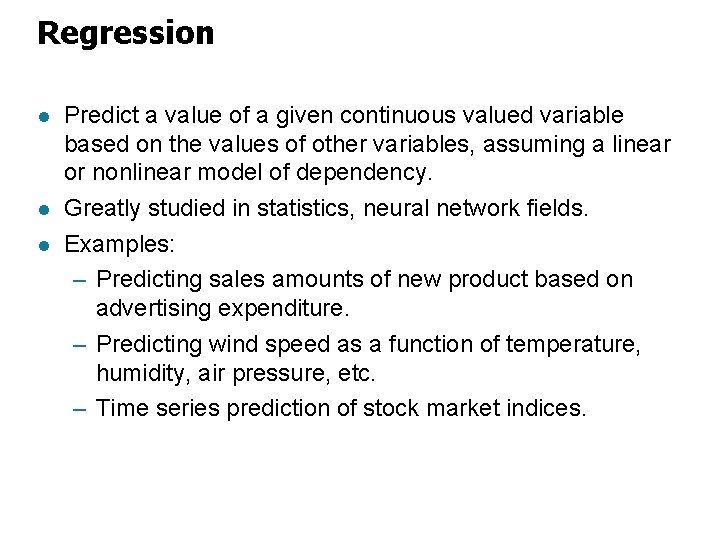
Regression l l l Predict a value of a given continuous valued variable based on the values of other variables, assuming a linear or nonlinear model of dependency. Greatly studied in statistics, neural network fields. Examples: – Predicting sales amounts of new product based on advertising expenditure. – Predicting wind speed as a function of temperature, humidity, air pressure, etc. – Time series prediction of stock market indices.
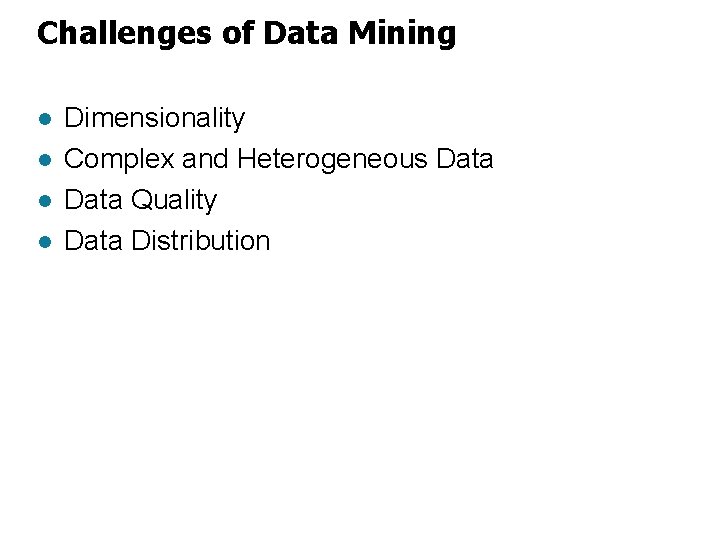
Challenges of Data Mining l l Dimensionality Complex and Heterogeneous Data Quality Data Distribution
Why mine data commercial viewpoint
Mining complex types of data in data mining
That moment she was mine mine fair
Multimedia data mining
Andreas carlsson bye bye bye
Introduction to data warehousing and data mining
Noncommercial food service
Strip mining vs open pit mining
Strip mining before and after
Difference between strip mining and open pit mining
Difference between text mining and web mining
Introduction to data mining and knowledge discovery
Complex data types in data mining
Azure data mining
Dont ask
Data reduction in data mining
Data mining in data warehouse
What is missing data in data mining
Concept hierarchy generation for nominal data
Data reduction in data mining
Data reduction in data mining
Data cube technology in data mining
Data reduction in data mining
Data warehouse dan data mining
Data mining dan data warehouse
Data warehousing and data mining in crm
Descriptive mining of complex data objects
Olap data mining
Noisy data in data mining
Data warehouse 3 tier architecture
Data preparation for data mining
Data compression in data mining
Data warehouse dan data mining
Commercial audit meaning
What is your favorite commercial
Introduction to commercial law
Evolution of commercial bank
What is commercial data processing
Primary function of commercial bank
Commercial data warehouse
Unsupervised learning in data mining