Inferential Statistics Comparison of Group Means A Research
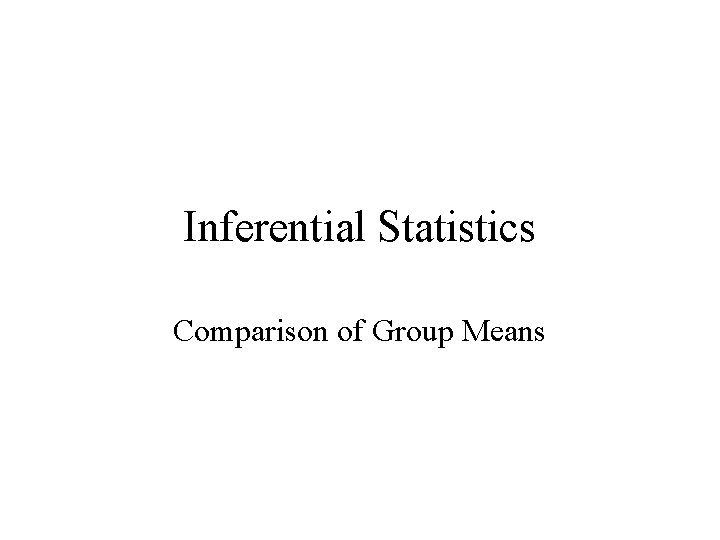
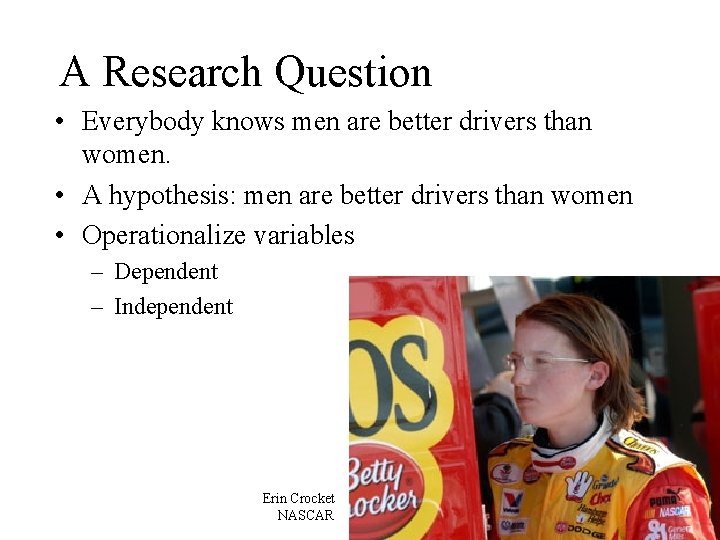
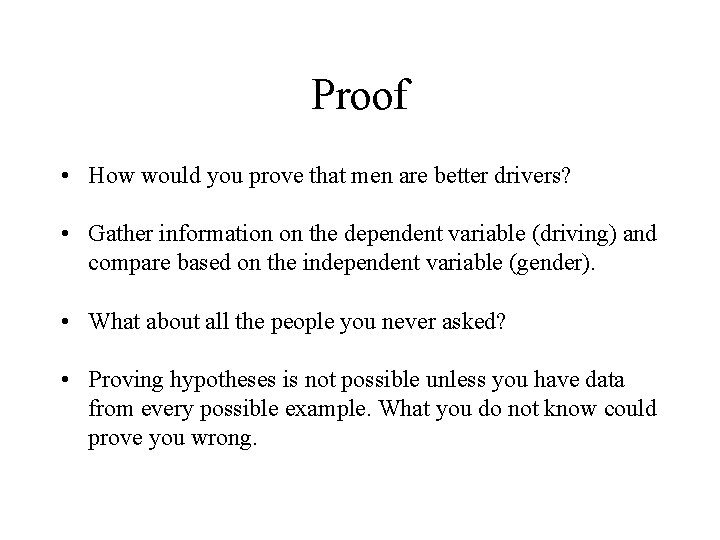
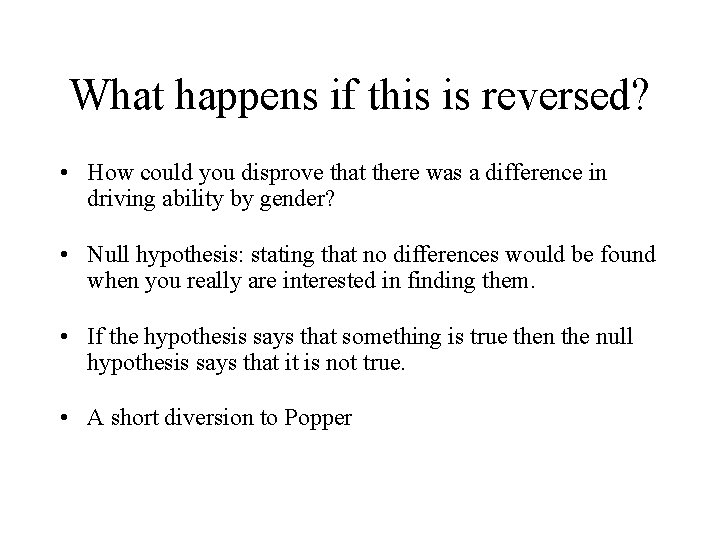
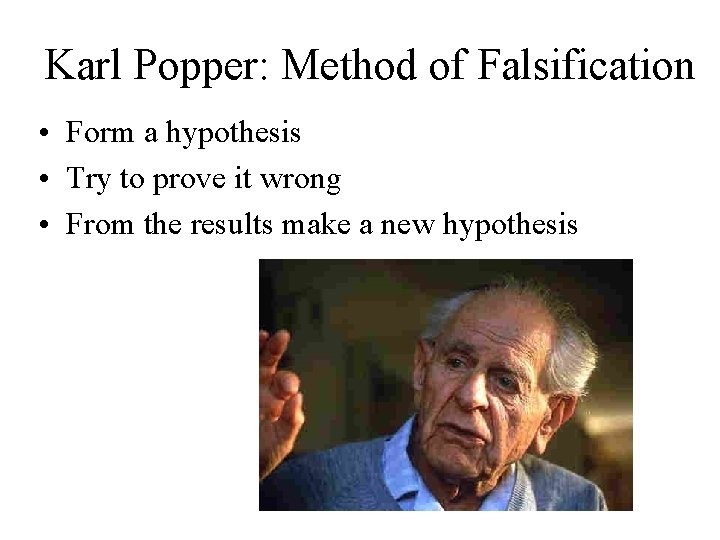
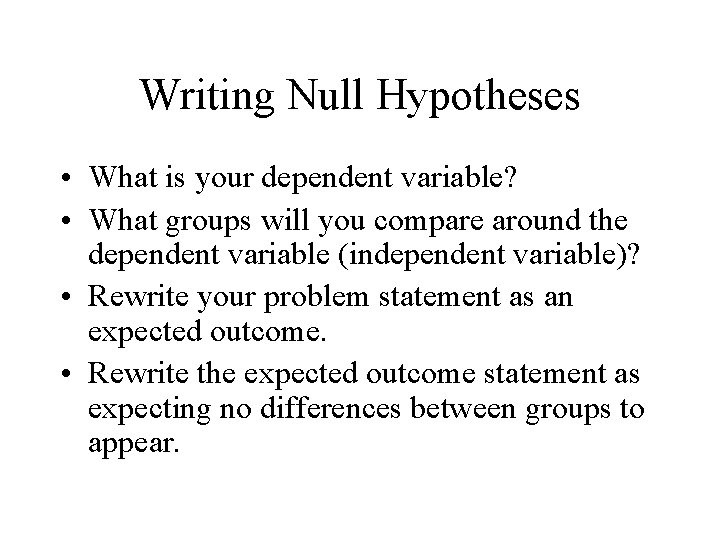
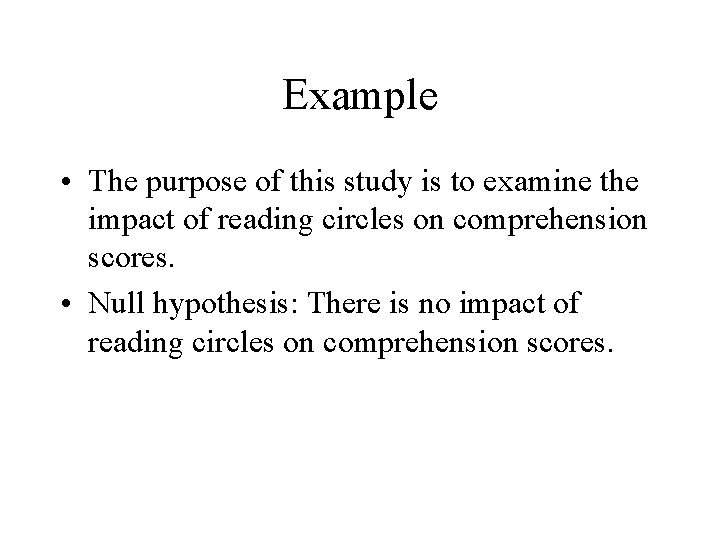
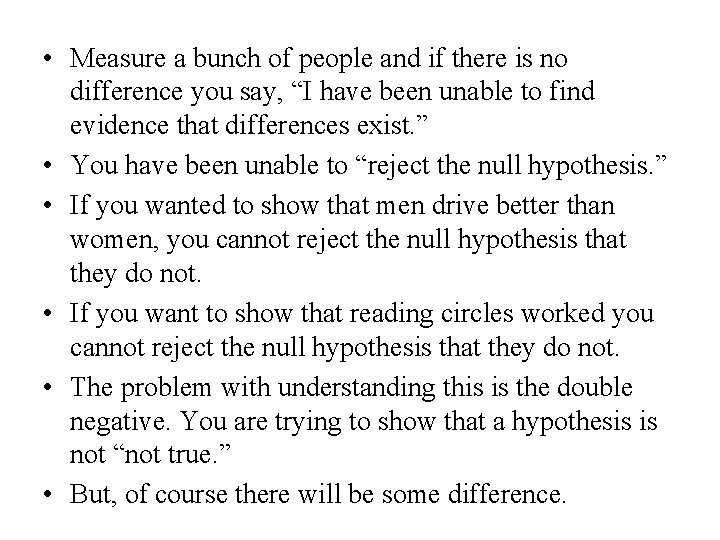
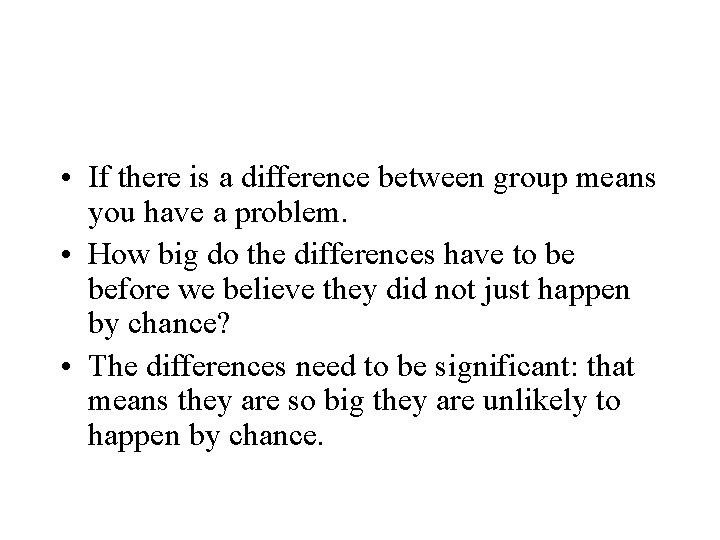
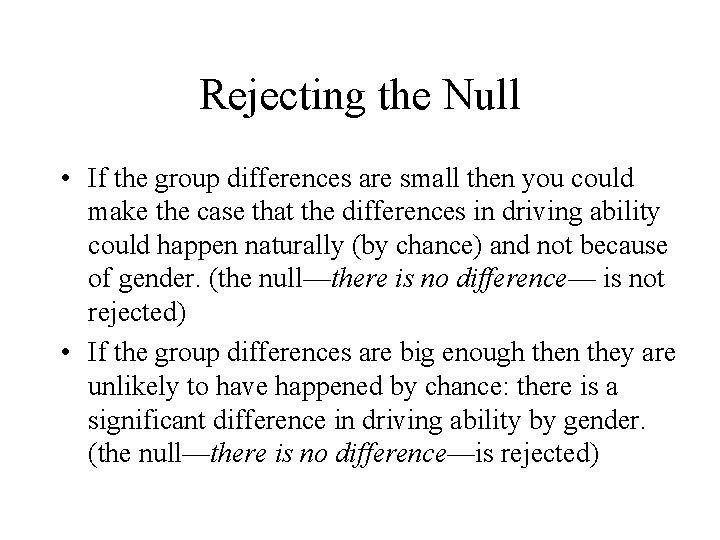
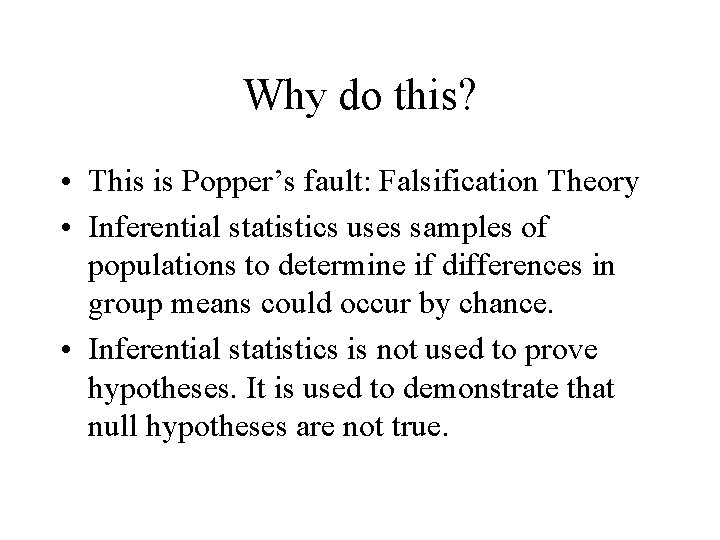
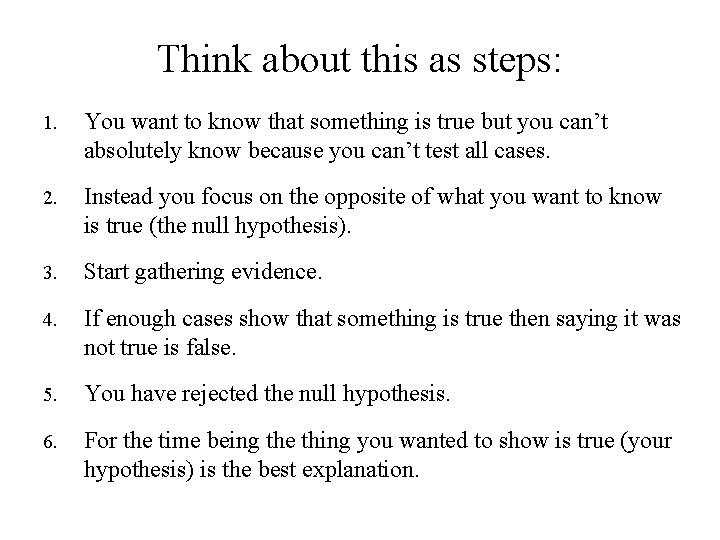
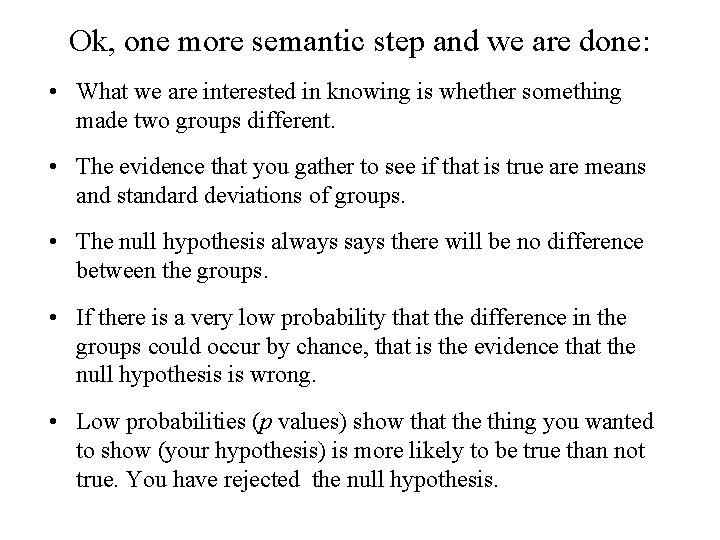
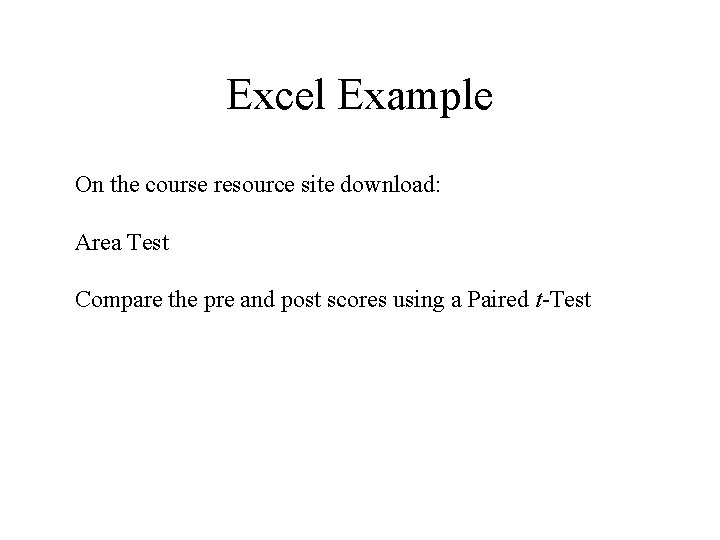
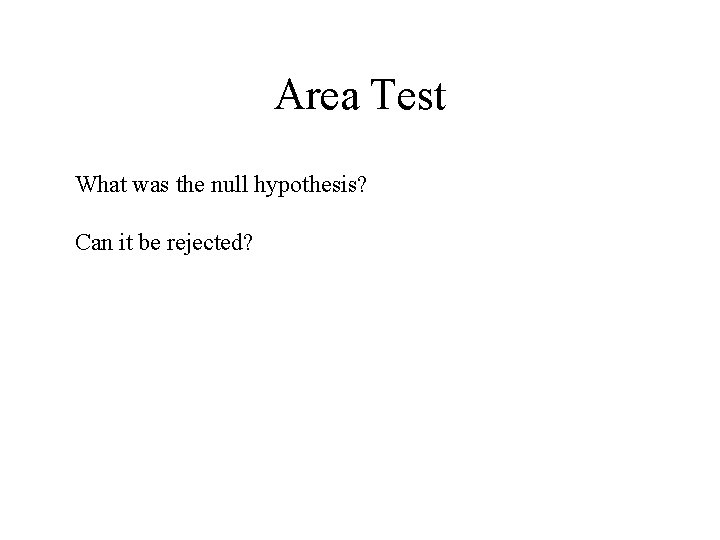
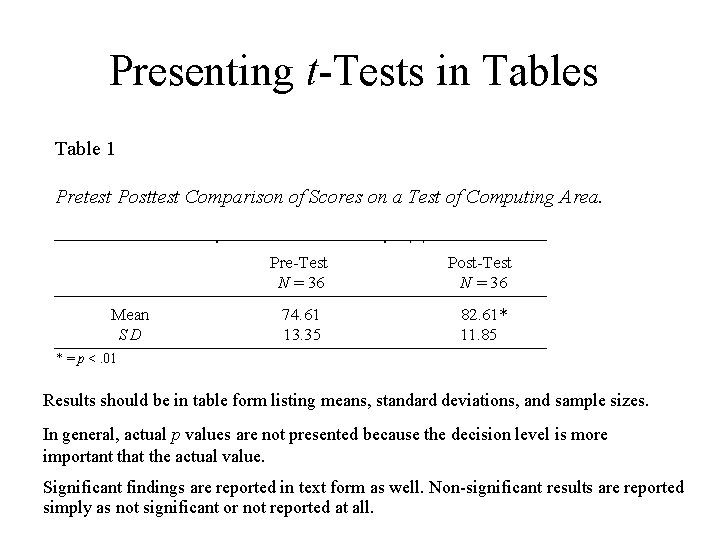
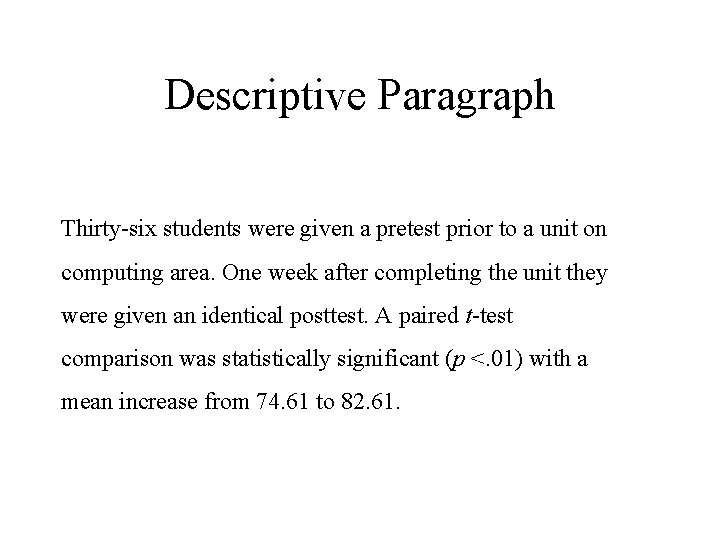
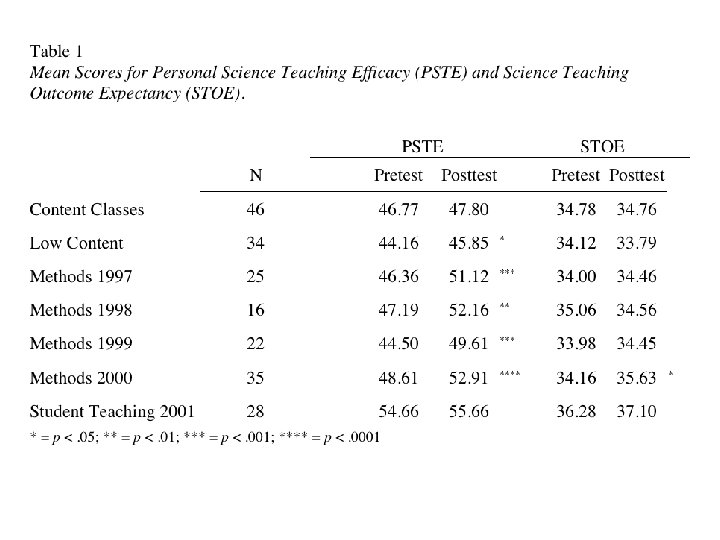
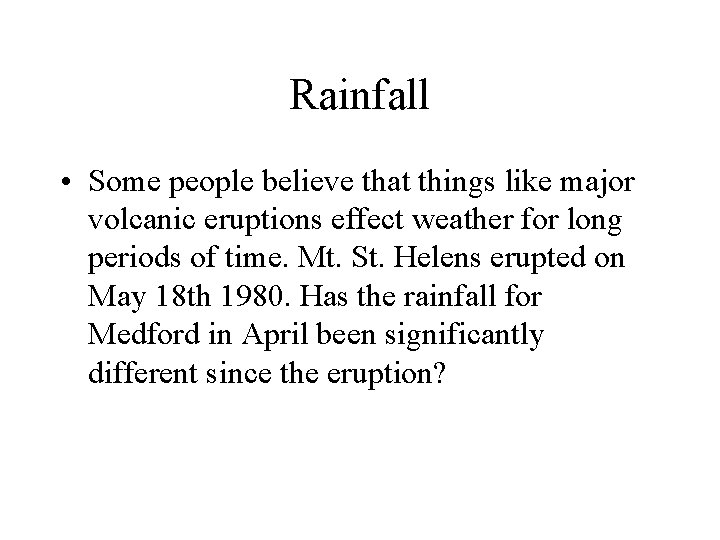
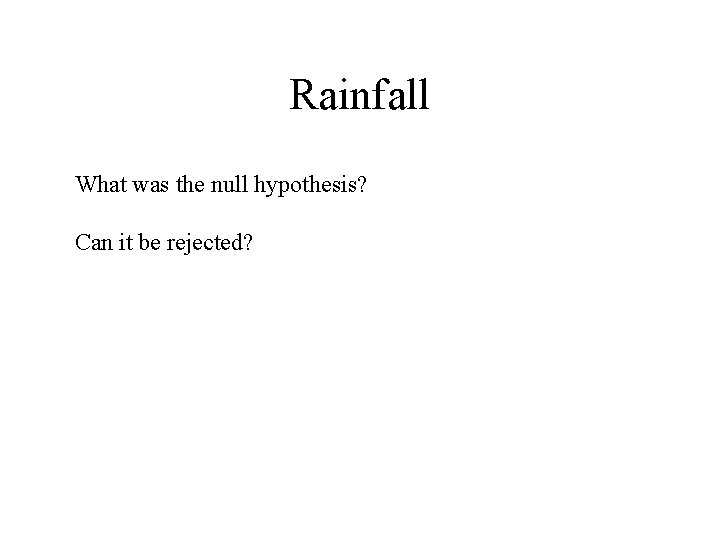
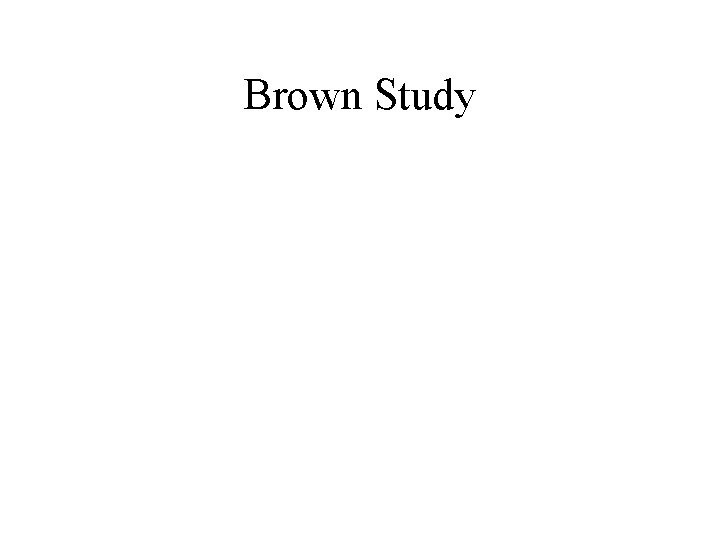
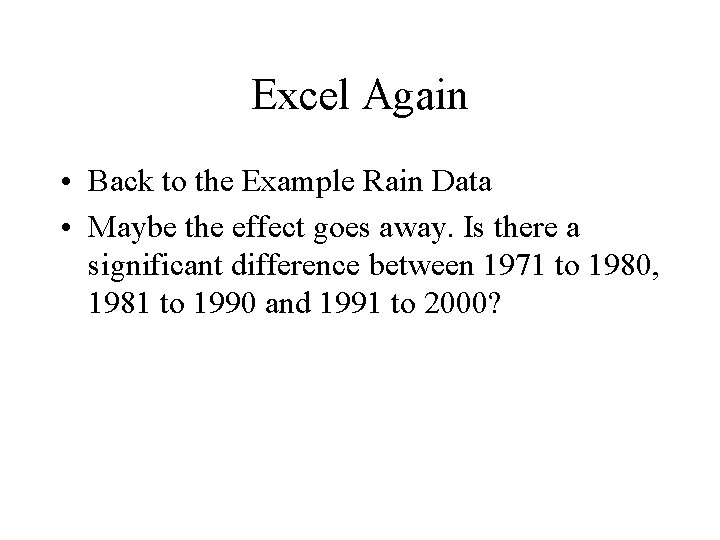
- Slides: 22
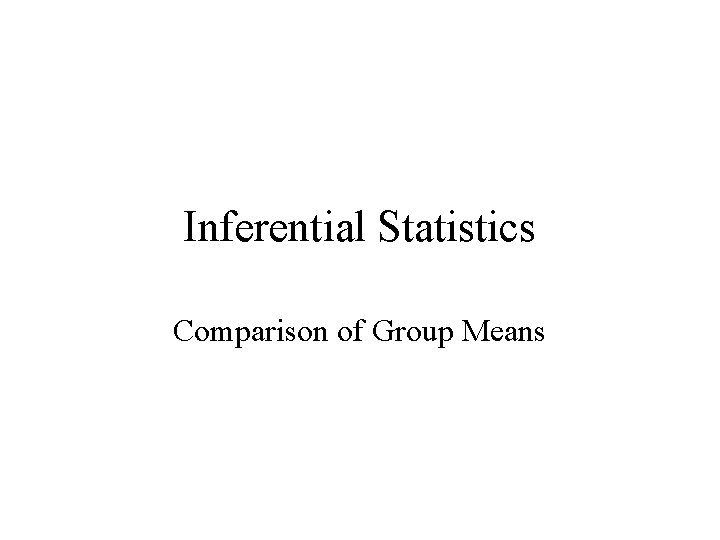
Inferential Statistics Comparison of Group Means
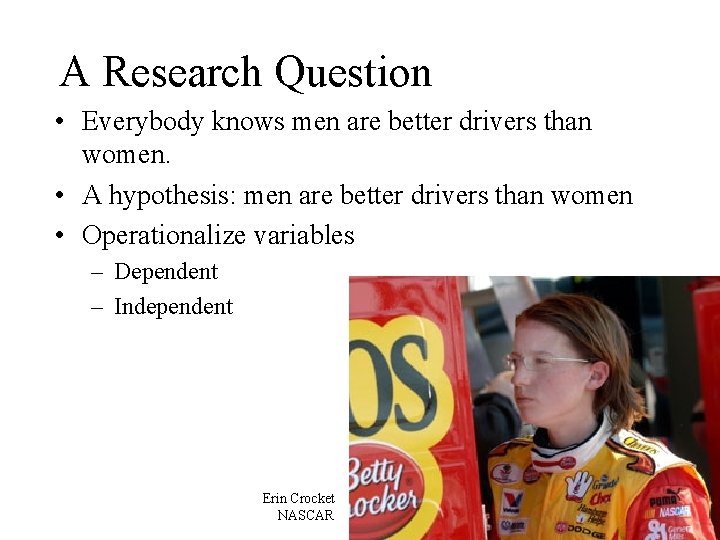
A Research Question • Everybody knows men are better drivers than women. • A hypothesis: men are better drivers than women • Operationalize variables – Dependent – Independent Erin Crocket NASCAR
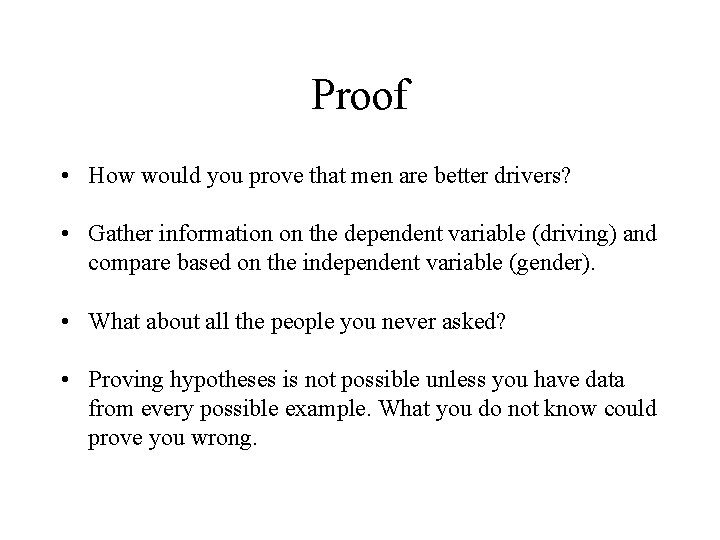
Proof • How would you prove that men are better drivers? • Gather information on the dependent variable (driving) and compare based on the independent variable (gender). • What about all the people you never asked? • Proving hypotheses is not possible unless you have data from every possible example. What you do not know could prove you wrong.
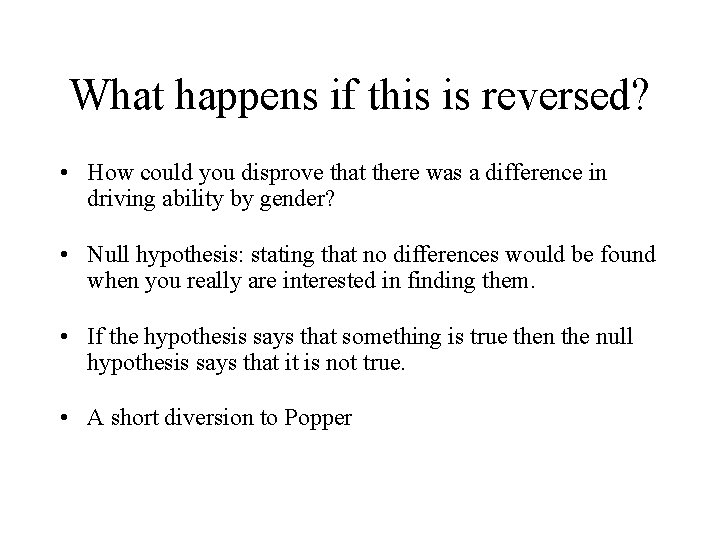
What happens if this is reversed? • How could you disprove that there was a difference in driving ability by gender? • Null hypothesis: stating that no differences would be found when you really are interested in finding them. • If the hypothesis says that something is true then the null hypothesis says that it is not true. • A short diversion to Popper
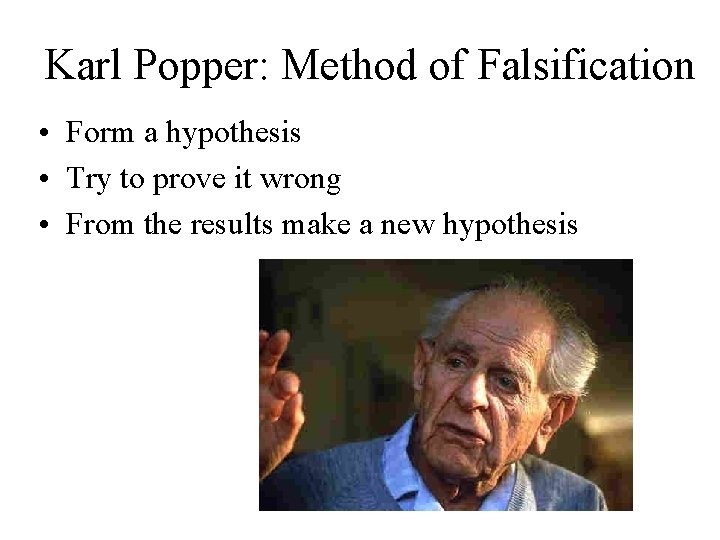
Karl Popper: Method of Falsification • Form a hypothesis • Try to prove it wrong • From the results make a new hypothesis
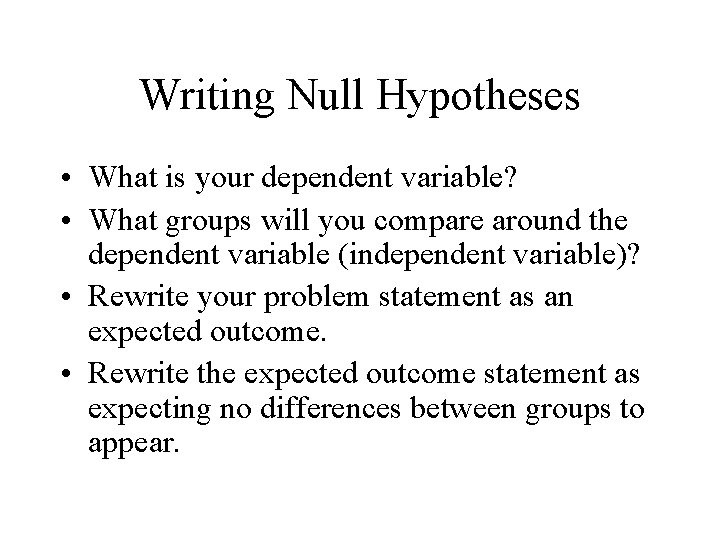
Writing Null Hypotheses • What is your dependent variable? • What groups will you compare around the dependent variable (independent variable)? • Rewrite your problem statement as an expected outcome. • Rewrite the expected outcome statement as expecting no differences between groups to appear.
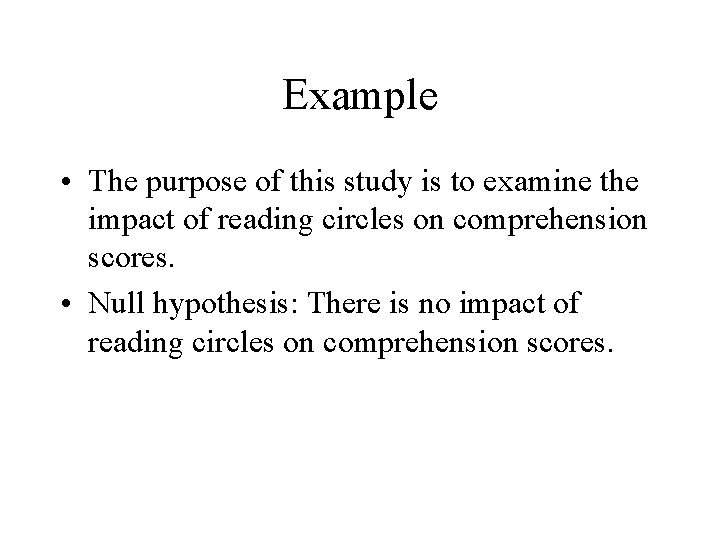
Example • The purpose of this study is to examine the impact of reading circles on comprehension scores. • Null hypothesis: There is no impact of reading circles on comprehension scores.
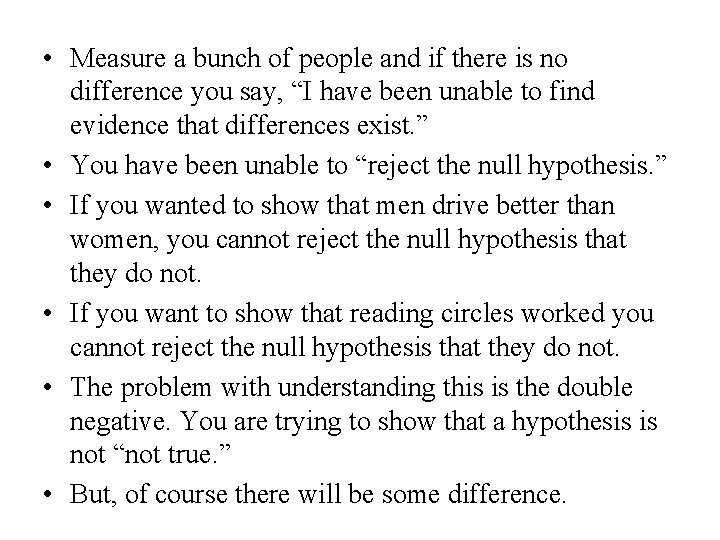
• Measure a bunch of people and if there is no difference you say, “I have been unable to find evidence that differences exist. ” • You have been unable to “reject the null hypothesis. ” • If you wanted to show that men drive better than women, you cannot reject the null hypothesis that they do not. • If you want to show that reading circles worked you cannot reject the null hypothesis that they do not. • The problem with understanding this is the double negative. You are trying to show that a hypothesis is not “not true. ” • But, of course there will be some difference.
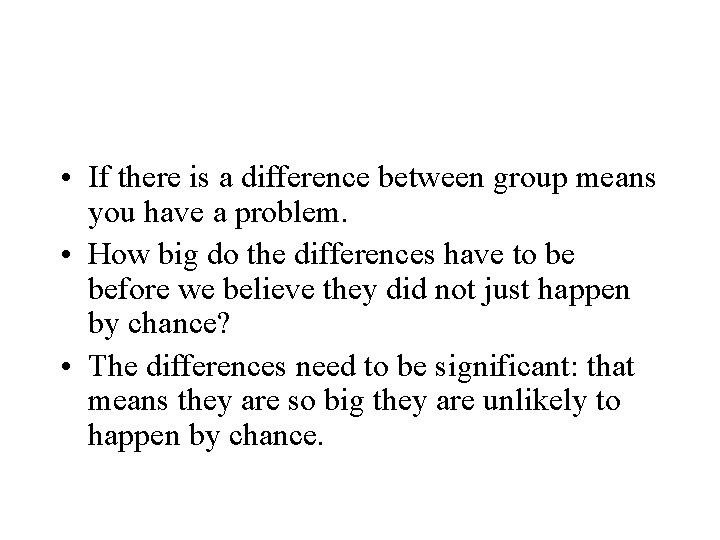
• If there is a difference between group means you have a problem. • How big do the differences have to be before we believe they did not just happen by chance? • The differences need to be significant: that means they are so big they are unlikely to happen by chance.
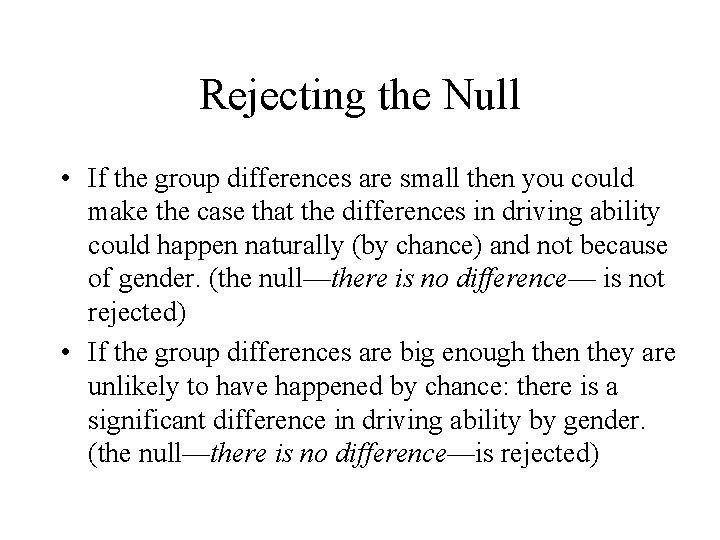
Rejecting the Null • If the group differences are small then you could make the case that the differences in driving ability could happen naturally (by chance) and not because of gender. (the null—there is no difference— is not rejected) • If the group differences are big enough then they are unlikely to have happened by chance: there is a significant difference in driving ability by gender. (the null—there is no difference—is rejected)
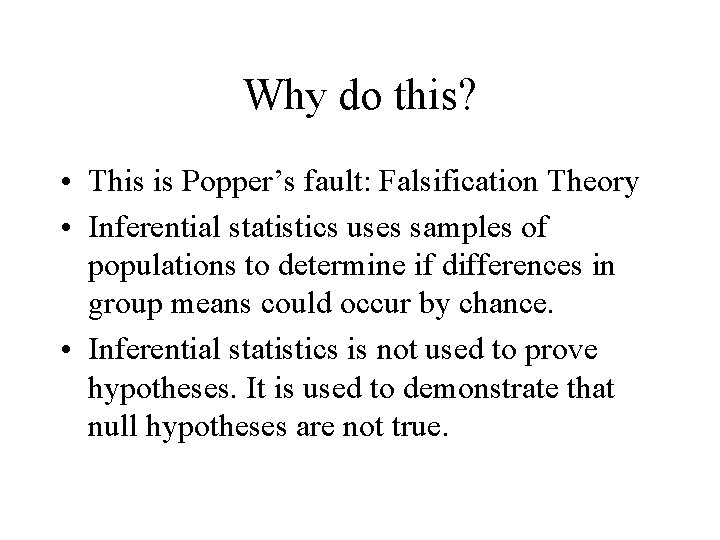
Why do this? • This is Popper’s fault: Falsification Theory • Inferential statistics uses samples of populations to determine if differences in group means could occur by chance. • Inferential statistics is not used to prove hypotheses. It is used to demonstrate that null hypotheses are not true.
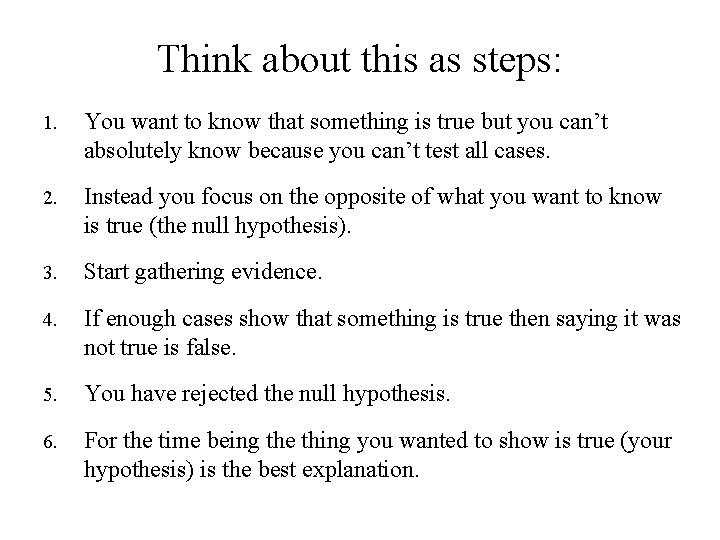
Think about this as steps: 1. You want to know that something is true but you can’t absolutely know because you can’t test all cases. 2. Instead you focus on the opposite of what you want to know is true (the null hypothesis). 3. Start gathering evidence. 4. If enough cases show that something is true then saying it was not true is false. 5. You have rejected the null hypothesis. 6. For the time being the thing you wanted to show is true (your hypothesis) is the best explanation.
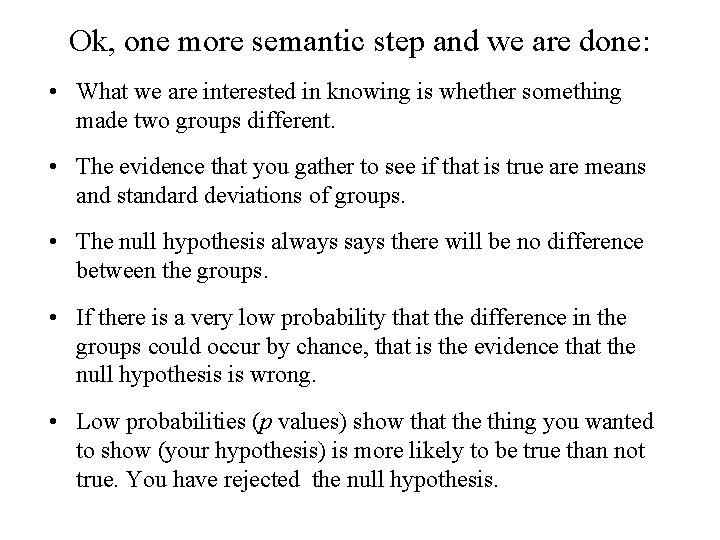
Ok, one more semantic step and we are done: • What we are interested in knowing is whether something made two groups different. • The evidence that you gather to see if that is true are means and standard deviations of groups. • The null hypothesis always says there will be no difference between the groups. • If there is a very low probability that the difference in the groups could occur by chance, that is the evidence that the null hypothesis is wrong. • Low probabilities (p values) show that the thing you wanted to show (your hypothesis) is more likely to be true than not true. You have rejected the null hypothesis.
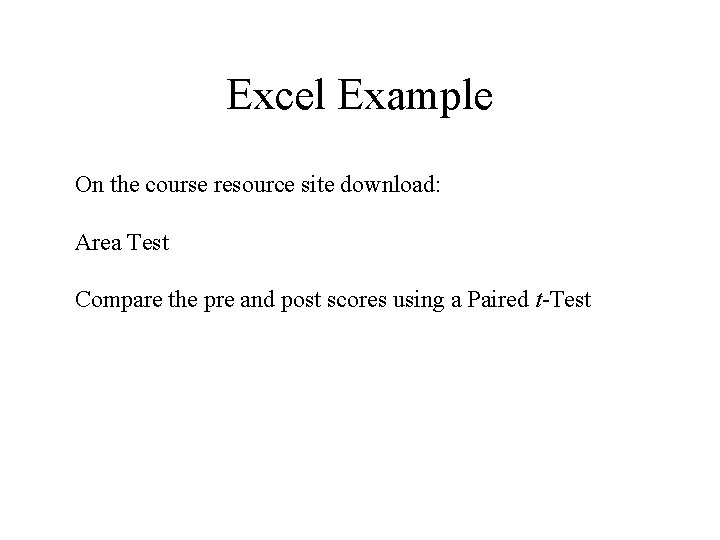
Excel Example On the course resource site download: Area Test Compare the pre and post scores using a Paired t-Test
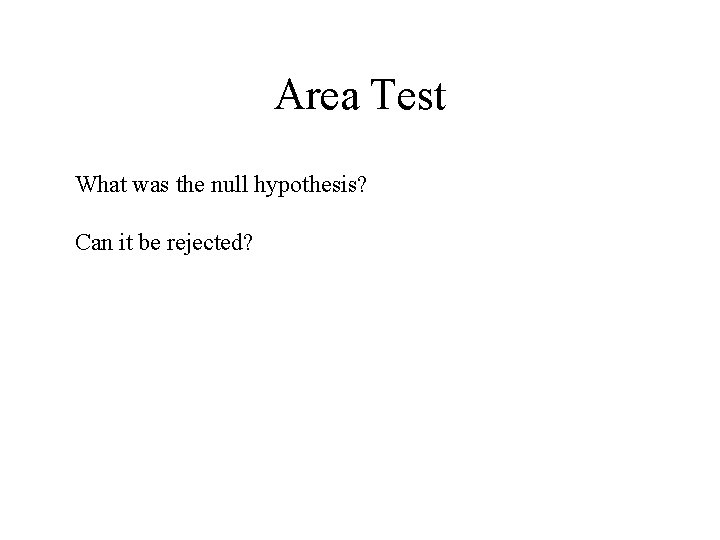
Area Test What was the null hypothesis? Can it be rejected?
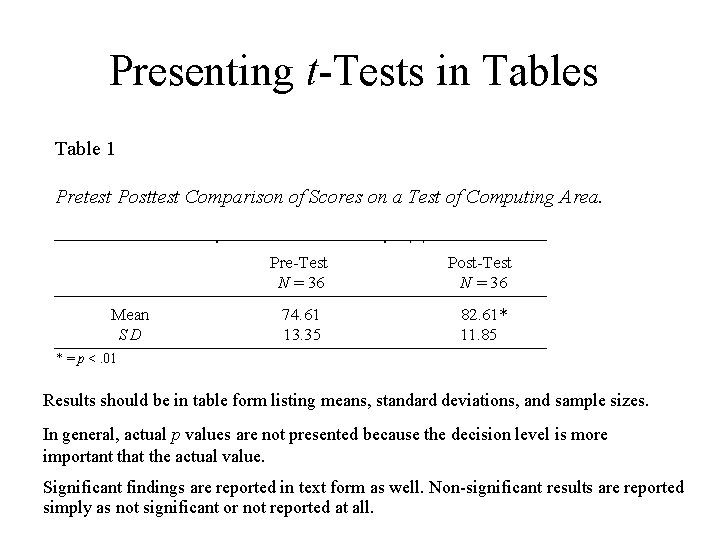
Presenting t-Tests in Tables Table 1 Pretest Posttest Comparison of Scores on a Test of Computing Area. Mean SD Pre-Test N = 36 Post-Test N = 36 74. 61 13. 35 82. 61* 11. 85 * = p <. 01 Results should be in table form listing means, standard deviations, and sample sizes. In general, actual p values are not presented because the decision level is more important that the actual value. Significant findings are reported in text form as well. Non-significant results are reported simply as not significant or not reported at all.
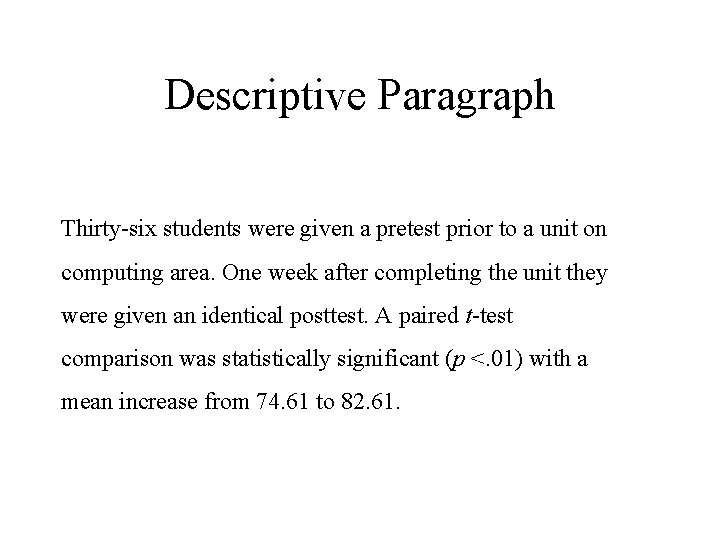
Descriptive Paragraph Thirty-six students were given a pretest prior to a unit on computing area. One week after completing the unit they were given an identical posttest. A paired t-test comparison was statistically significant (p <. 01) with a mean increase from 74. 61 to 82. 61.
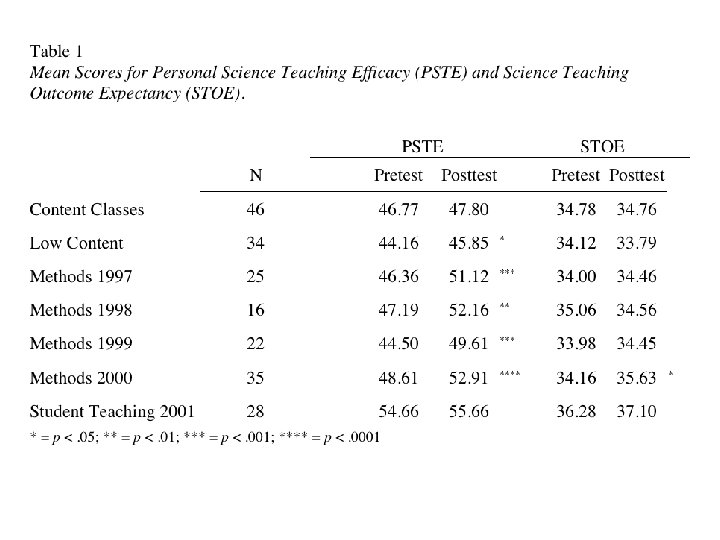
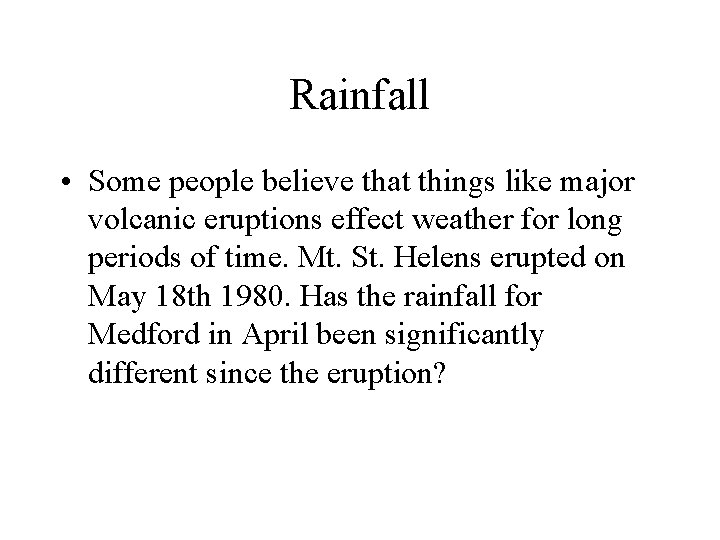
Rainfall • Some people believe that things like major volcanic eruptions effect weather for long periods of time. Mt. St. Helens erupted on May 18 th 1980. Has the rainfall for Medford in April been significantly different since the eruption?
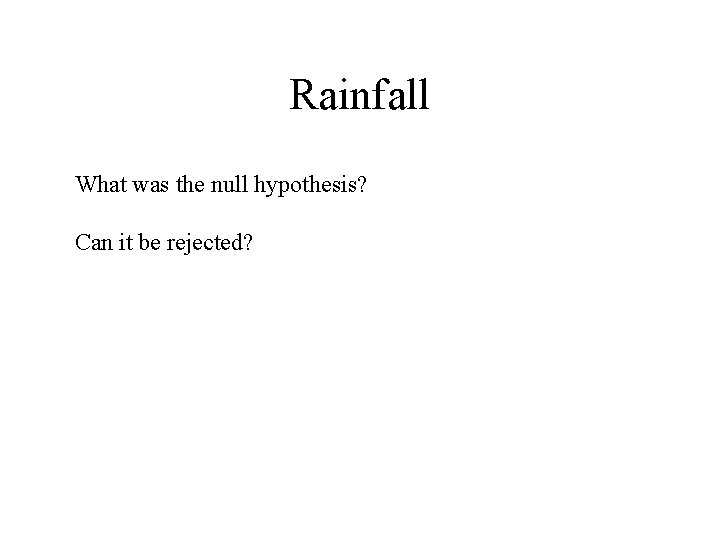
Rainfall What was the null hypothesis? Can it be rejected?
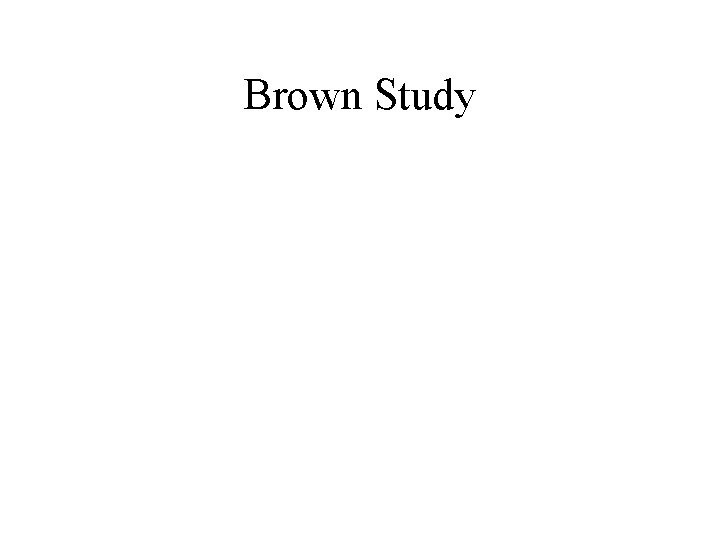
Brown Study
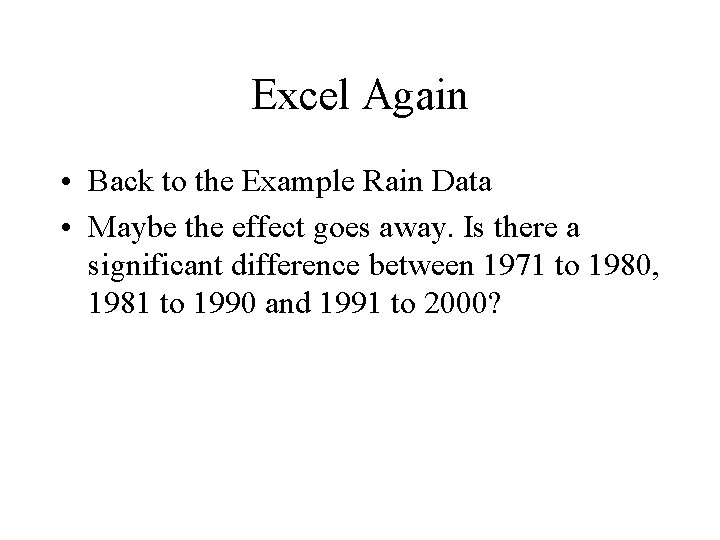
Excel Again • Back to the Example Rain Data • Maybe the effect goes away. Is there a significant difference between 1971 to 1980, 1981 to 1990 and 1991 to 2000?
Does qualitative research have hypothesis
Inferential statistics test
Empirical method probability
Inferential statistics in psychology
Inferential generalization
Hypothesis setting
Rare event rule for inferential statistics
Inferential statistics
Inferential statistics
Inferential testing
Inferential statistics correlation
Advantages of inferential statistics
Characteristics of inferential statistics
Inferential statistics ap psychology
Types of statistics
Characteristics of inferential statistics
Characteristics of inferential statistics
Class width
Inferential statistics definition
Inferential statistics examples
Inferential statement of the problem example
Define inferential statistics
Compairson test