Genome analysis and annotation Genome Annotation Which sequences
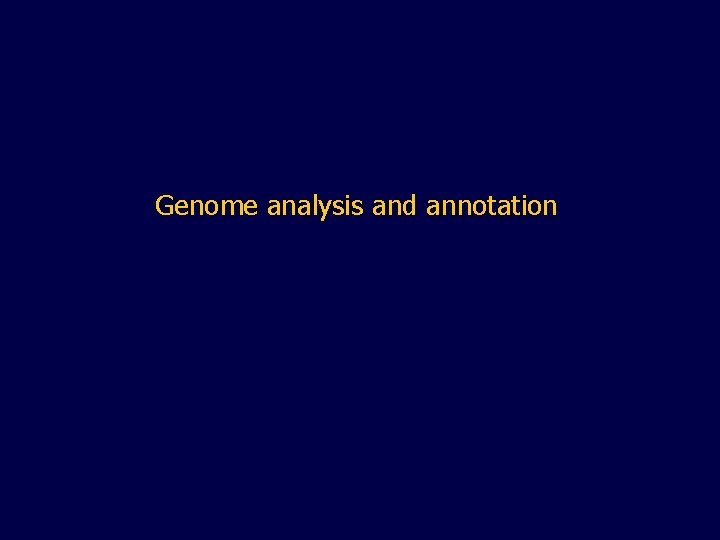
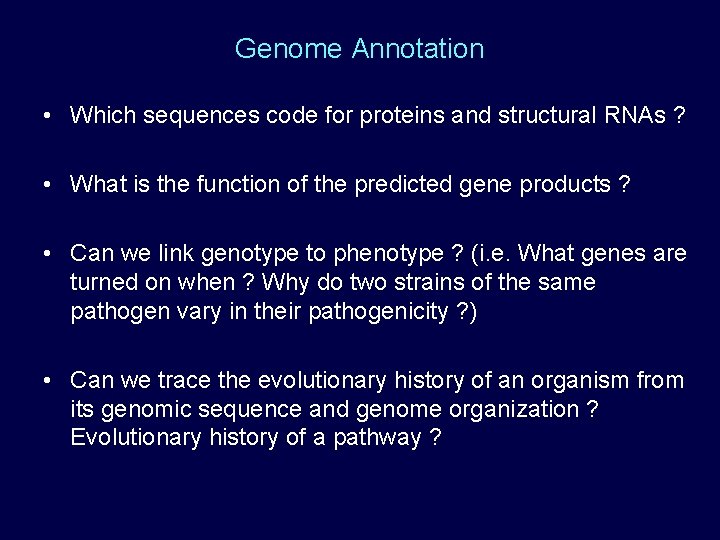
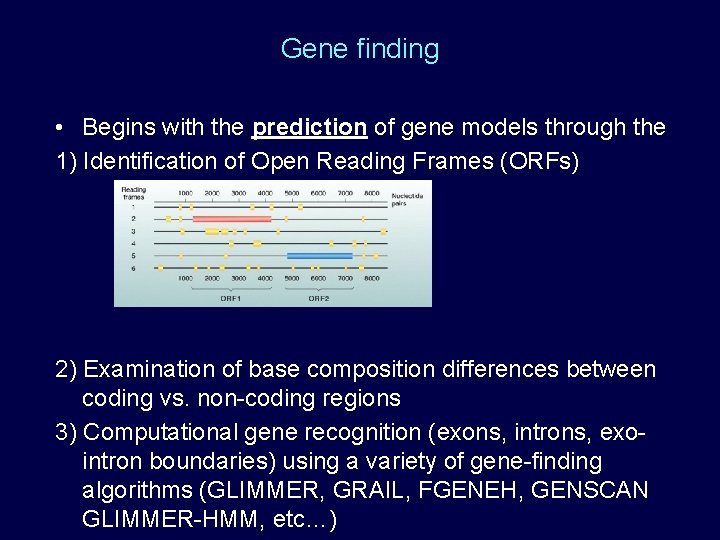
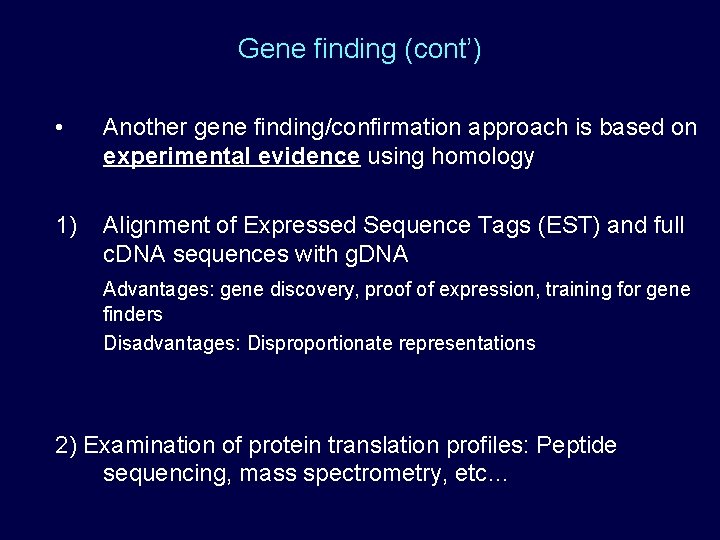
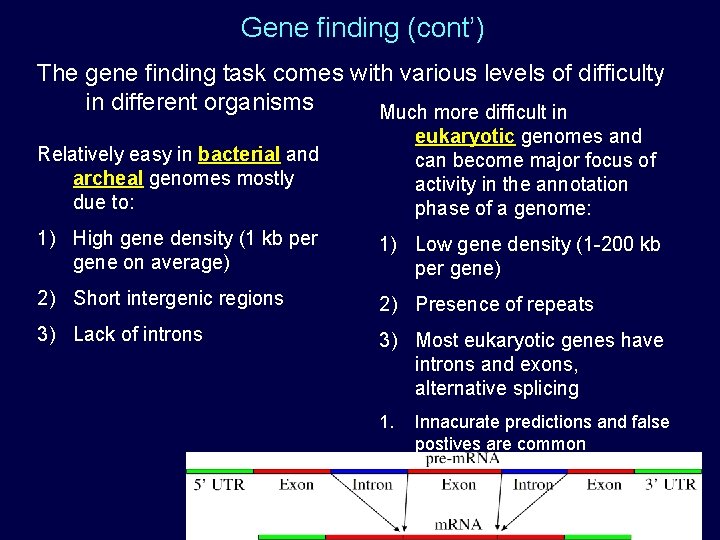
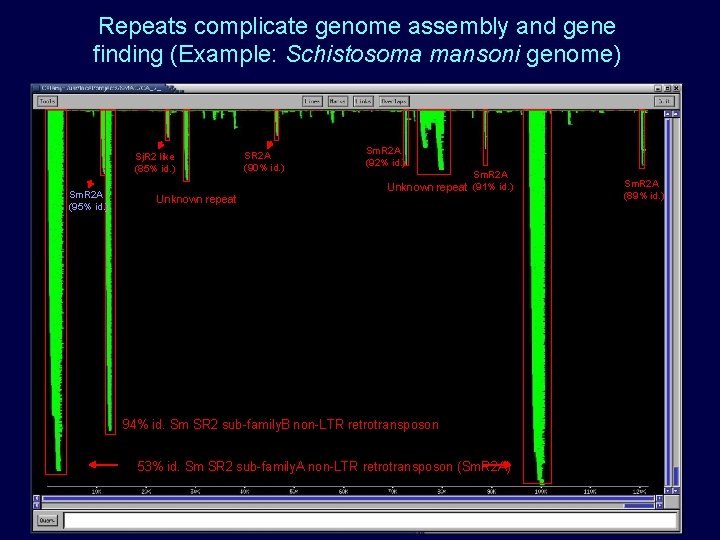
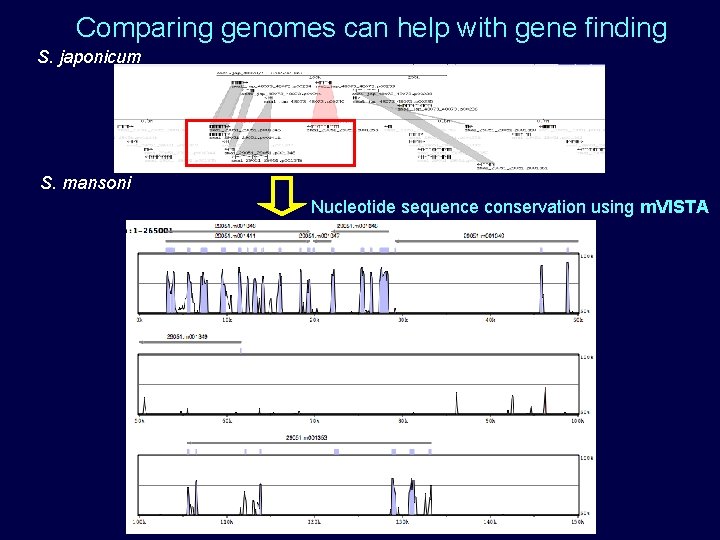
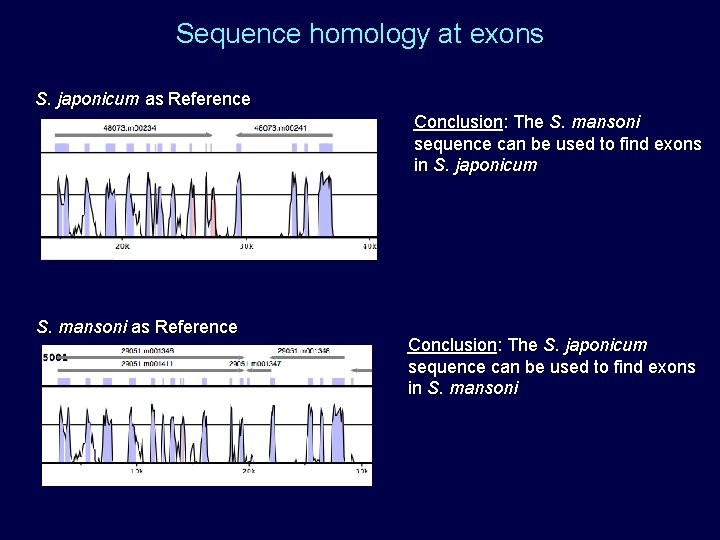
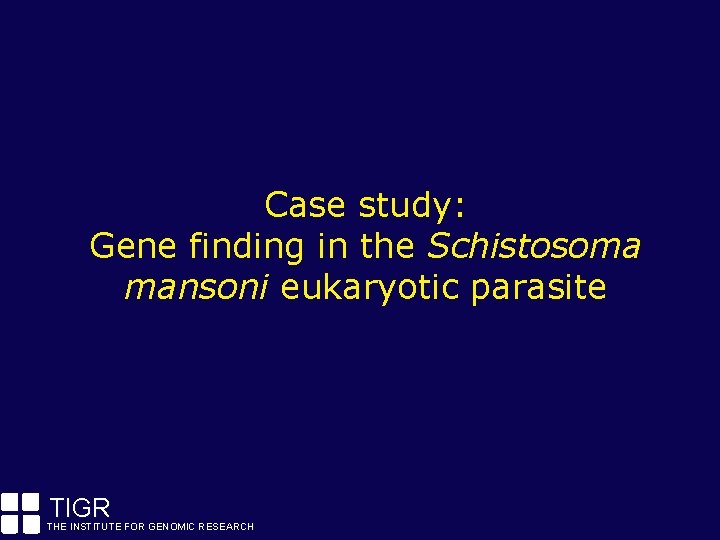
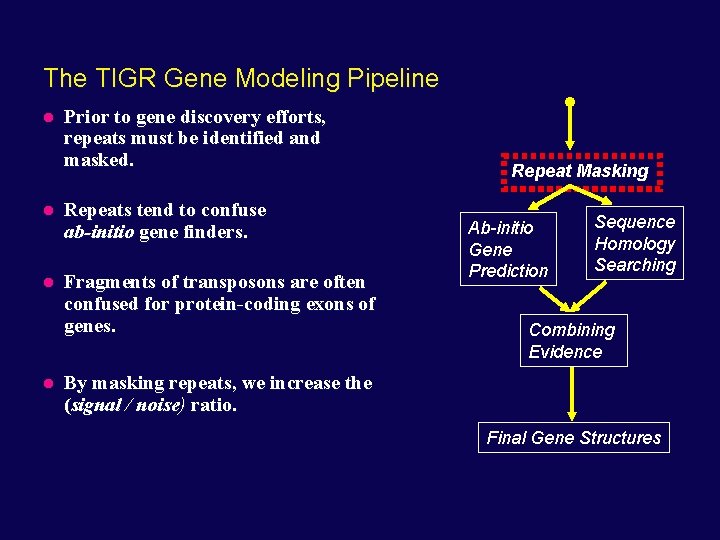
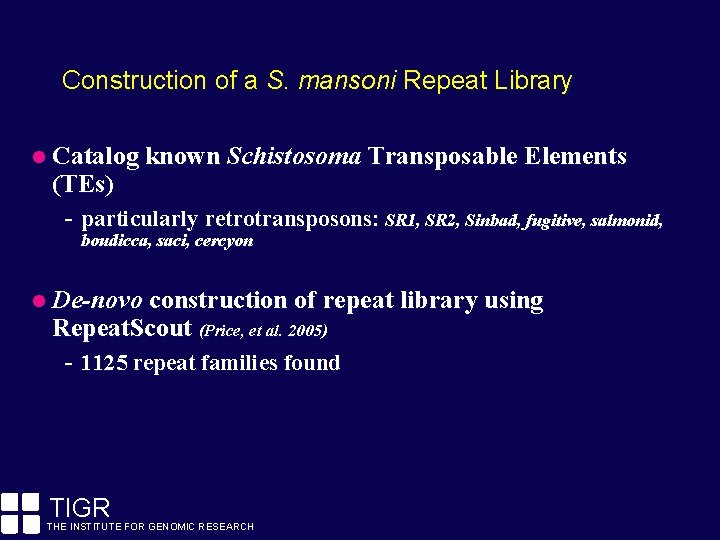
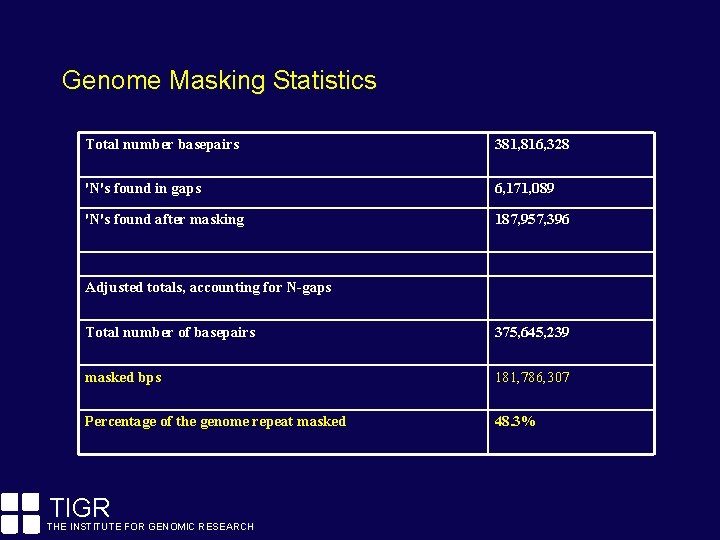
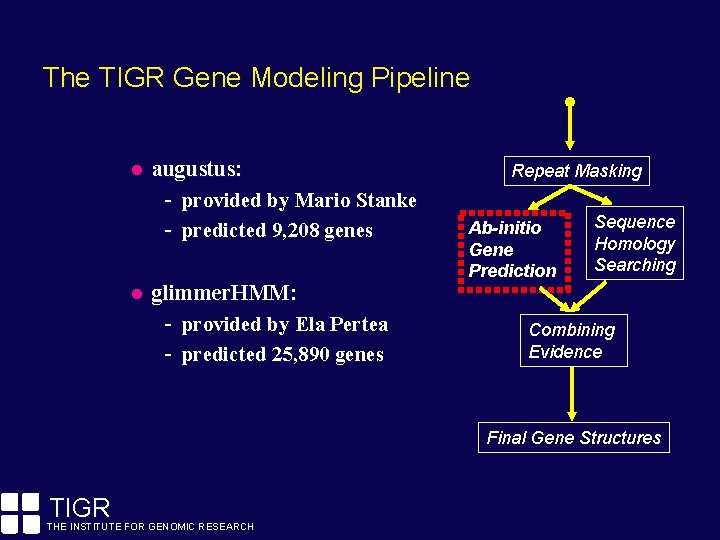
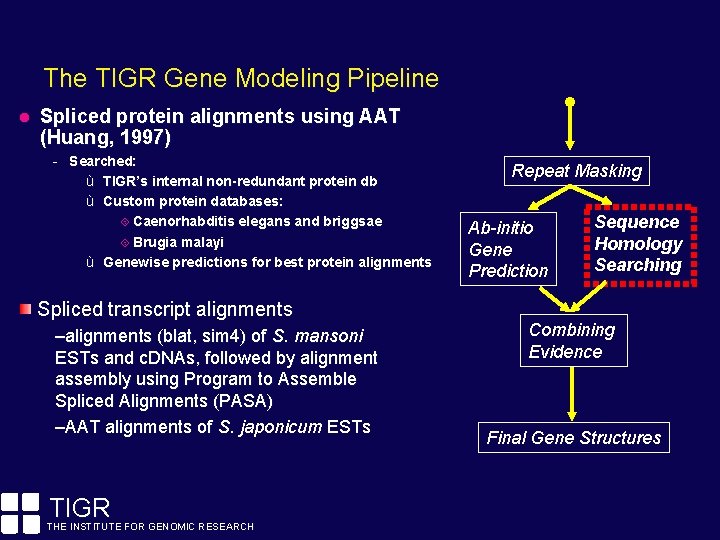
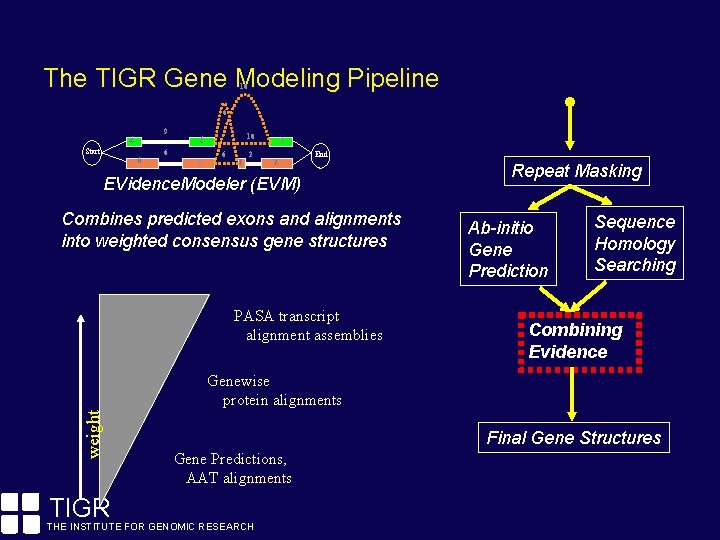
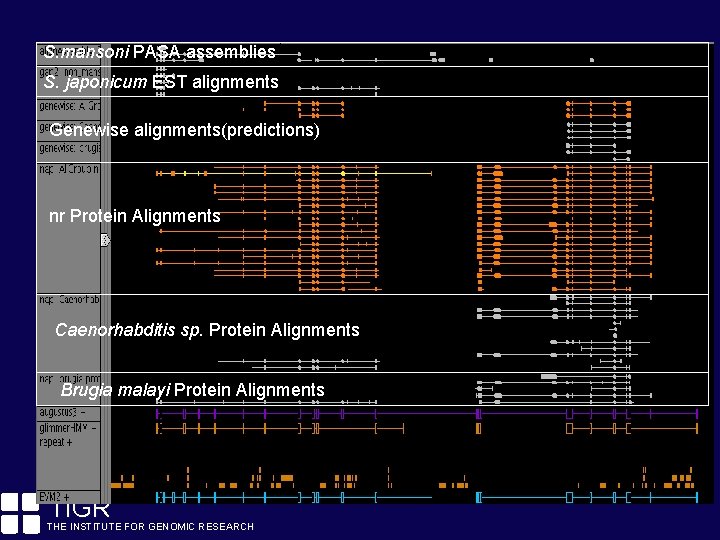
- Slides: 16
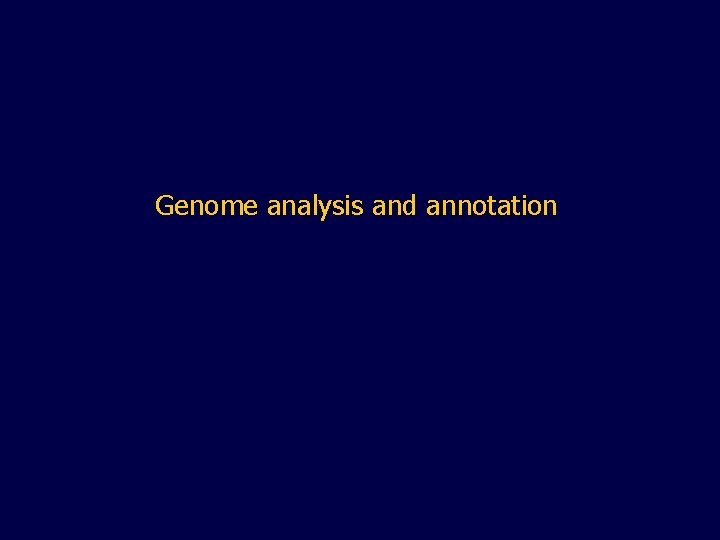
Genome analysis and annotation
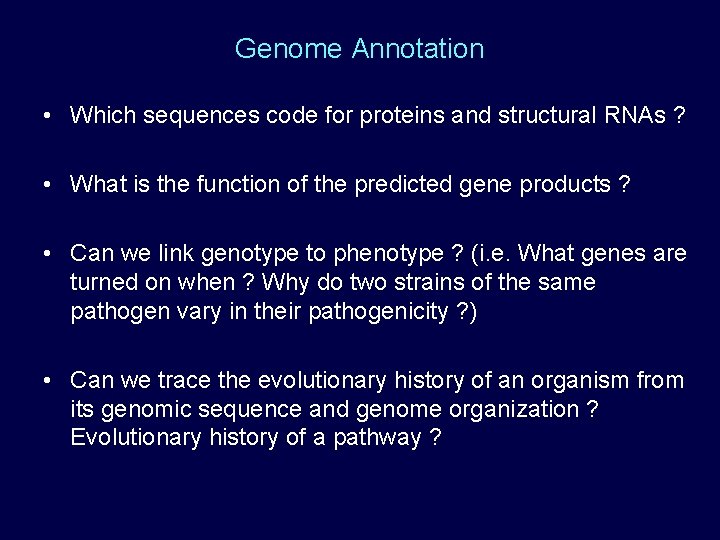
Genome Annotation • Which sequences code for proteins and structural RNAs ? • What is the function of the predicted gene products ? • Can we link genotype to phenotype ? (i. e. What genes are turned on when ? Why do two strains of the same pathogen vary in their pathogenicity ? ) • Can we trace the evolutionary history of an organism from its genomic sequence and genome organization ? Evolutionary history of a pathway ?
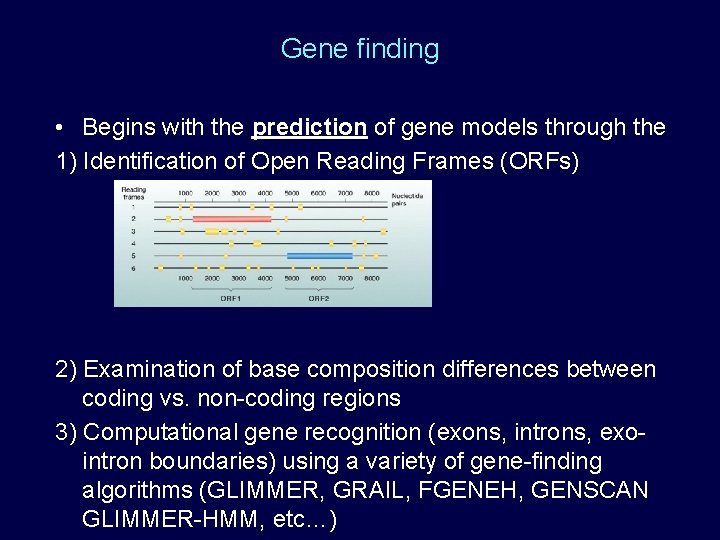
Gene finding • Begins with the prediction of gene models through the 1) Identification of Open Reading Frames (ORFs) 2) Examination of base composition differences between coding vs. non-coding regions 3) Computational gene recognition (exons, introns, exointron boundaries) using a variety of gene-finding algorithms (GLIMMER, GRAIL, FGENEH, GENSCAN GLIMMER-HMM, etc…)
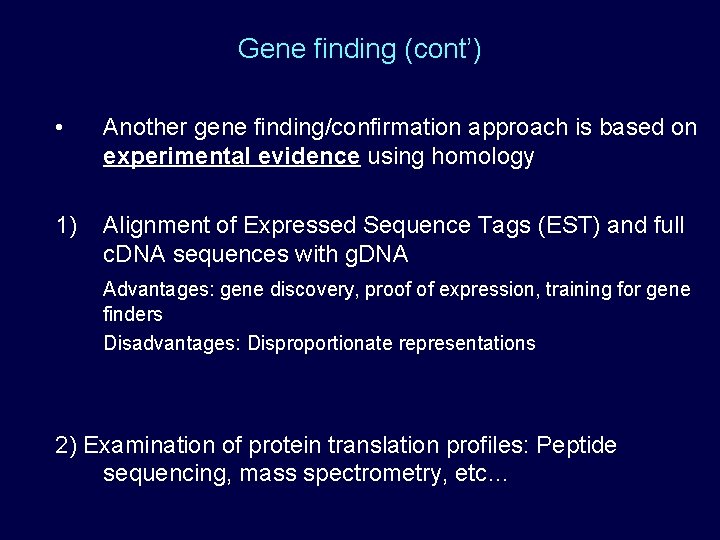
Gene finding (cont’) • Another gene finding/confirmation approach is based on experimental evidence using homology 1) Alignment of Expressed Sequence Tags (EST) and full c. DNA sequences with g. DNA Advantages: gene discovery, proof of expression, training for gene finders Disadvantages: Disproportionate representations 2) Examination of protein translation profiles: Peptide sequencing, mass spectrometry, etc…
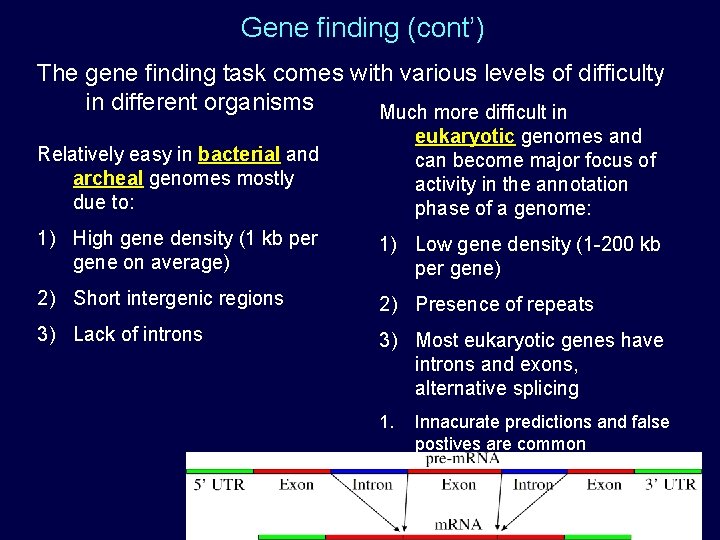
Gene finding (cont’) The gene finding task comes with various levels of difficulty in different organisms Much more difficult in Relatively easy in bacterial and archeal genomes mostly due to: eukaryotic genomes and can become major focus of activity in the annotation phase of a genome: 1) High gene density (1 kb per gene on average) 1) Low gene density (1 -200 kb per gene) 2) Short intergenic regions 2) Presence of repeats 3) Lack of introns 3) Most eukaryotic genes have introns and exons, alternative splicing 1. Innacurate predictions and false postives are common
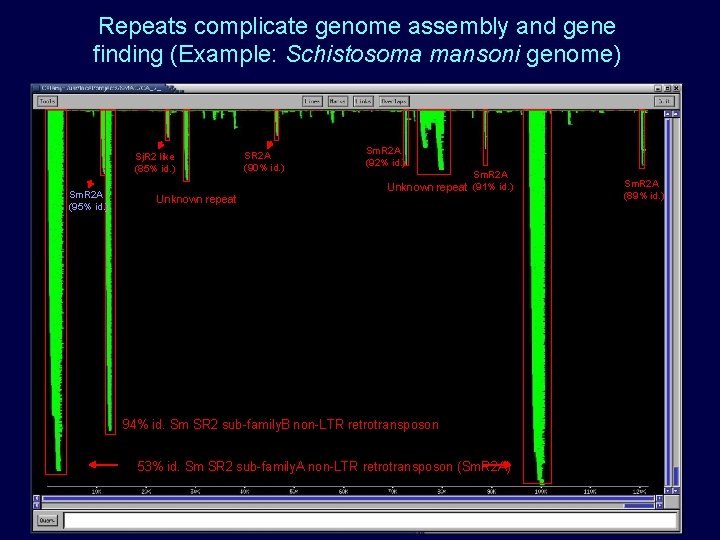
Repeats complicate genome assembly and gene finding (Example: Schistosoma mansoni genome) Sj. R 2 like (85% id. ) Sm. R 2 A (95% id. ) Unknown repeat SR 2 A (90% id. ) Sm. R 2 A (92% id. ) Sm. R 2 A Unknown repeat (91% id. ) 94% id. Sm SR 2 sub-family. B non-LTR retrotransposon 53% id. Sm SR 2 sub-family. A non-LTR retrotransposon (Sm. R 2 A) Sm. R 2 A (89% id. )
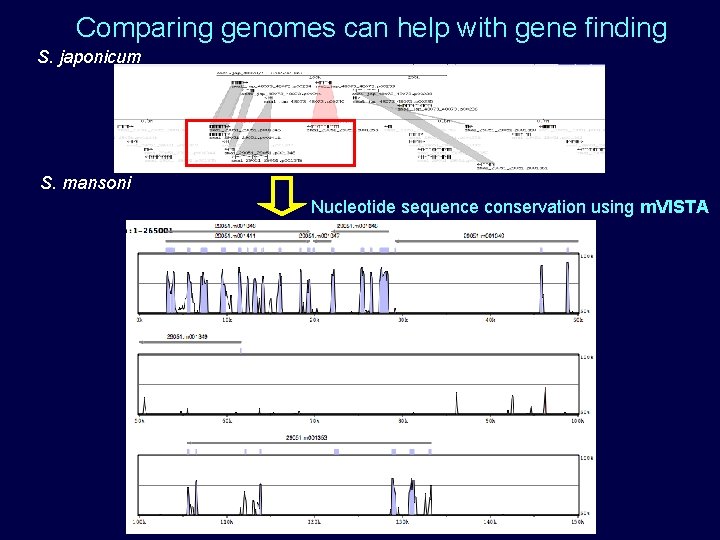
Comparing genomes can help with gene finding S. japonicum S. mansoni Nucleotide sequence conservation using m. VISTA
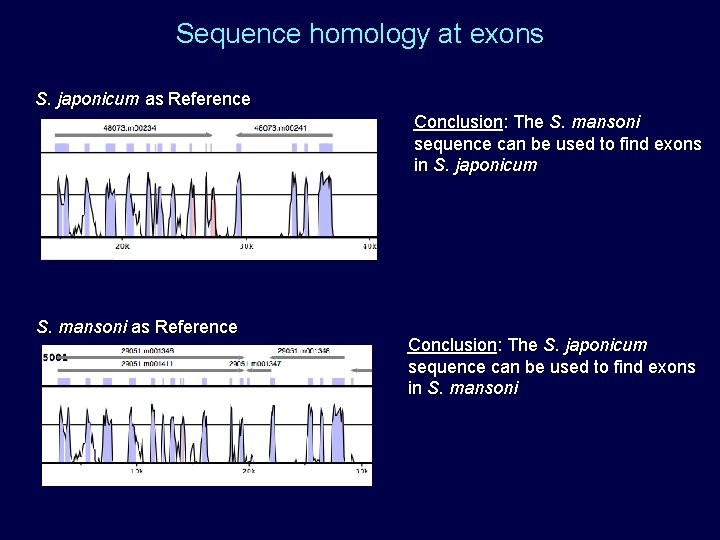
Sequence homology at exons S. japonicum as Reference Conclusion: The S. mansoni sequence can be used to find exons in S. japonicum S. mansoni as Reference Conclusion: The S. japonicum sequence can be used to find exons in S. mansoni
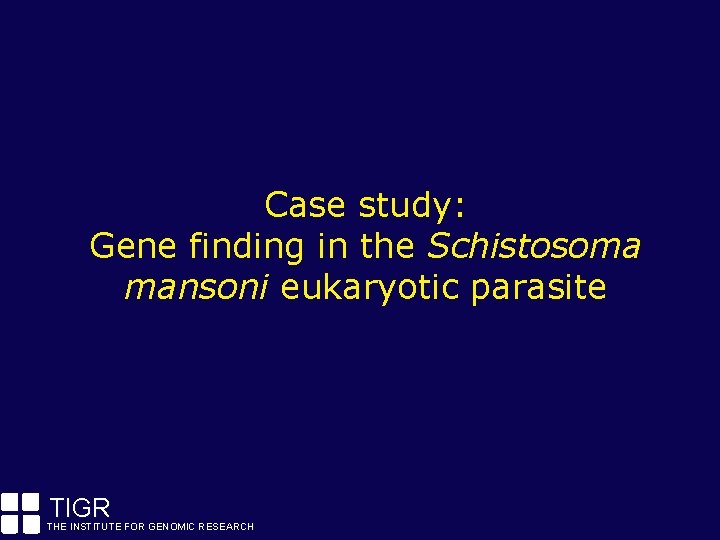
Case study: Gene finding in the Schistosoma mansoni eukaryotic parasite TIGR THE INSTITUTE FOR GENOMIC RESEARCH
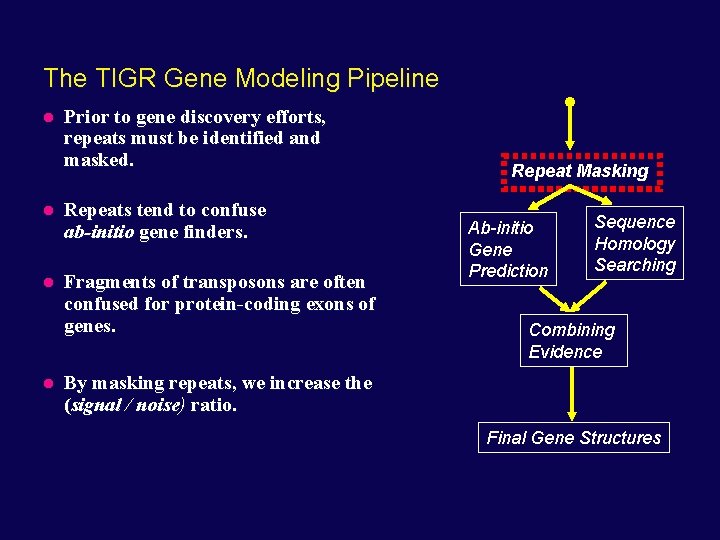
The TIGR Gene Modeling Pipeline Prior to gene discovery efforts, repeats must be identified and masked. Repeats tend to confuse ab-initio gene finders. Fragments of transposons are often confused for protein-coding exons of genes. Repeat Masking Ab-initio Gene Prediction Sequence Homology Searching Combining Evidence By masking repeats, we increase the (signal / noise) ratio. Final Gene Structures
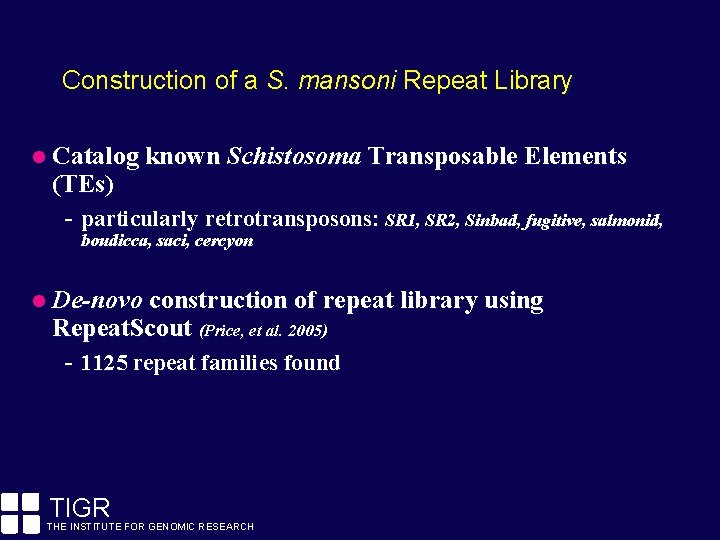
Construction of a S. mansoni Repeat Library Catalog known Schistosoma Transposable Elements (TEs) - particularly retrotransposons: SR 1, SR 2, Sinbad, fugitive, salmonid, boudicca, saci, cercyon De-novo construction of repeat library using Repeat. Scout (Price, et al. 2005) - 1125 repeat families found TIGR THE INSTITUTE FOR GENOMIC RESEARCH
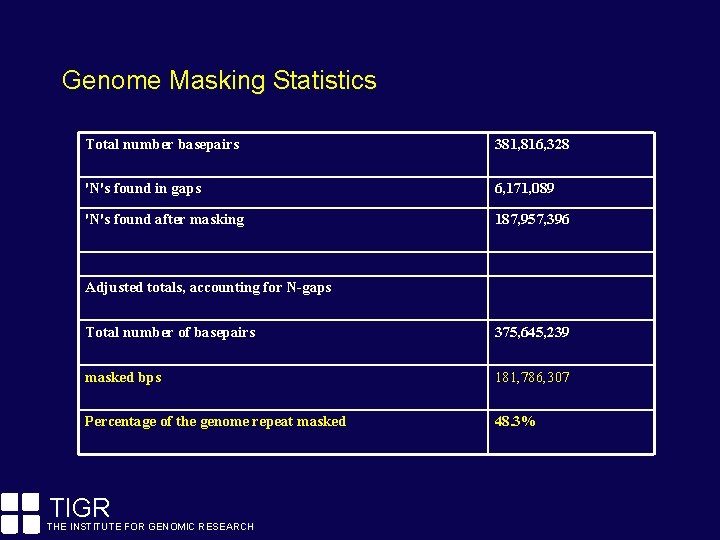
Genome Masking Statistics Total number basepairs 381, 816, 328 'N's found in gaps 6, 171, 089 'N's found after masking 187, 957, 396 Adjusted totals, accounting for N-gaps Total number of basepairs 375, 645, 239 masked bps 181, 786, 307 Percentage of the genome repeat masked 48. 3% TIGR THE INSTITUTE FOR GENOMIC RESEARCH
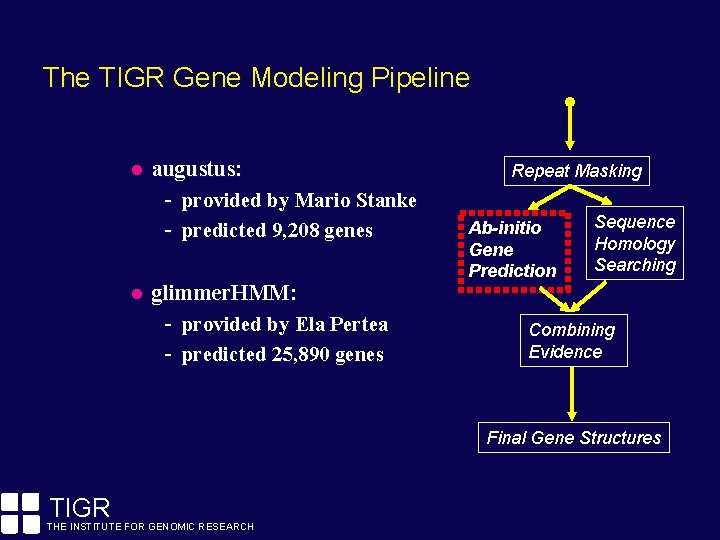
The TIGR Gene Modeling Pipeline augustus: - provided by Mario Stanke - predicted 9, 208 genes glimmer. HMM: - provided by Ela Pertea - predicted 25, 890 genes Repeat Masking Ab-initio Gene Prediction Sequence Homology Searching Combining Evidence Final Gene Structures TIGR THE INSTITUTE FOR GENOMIC RESEARCH
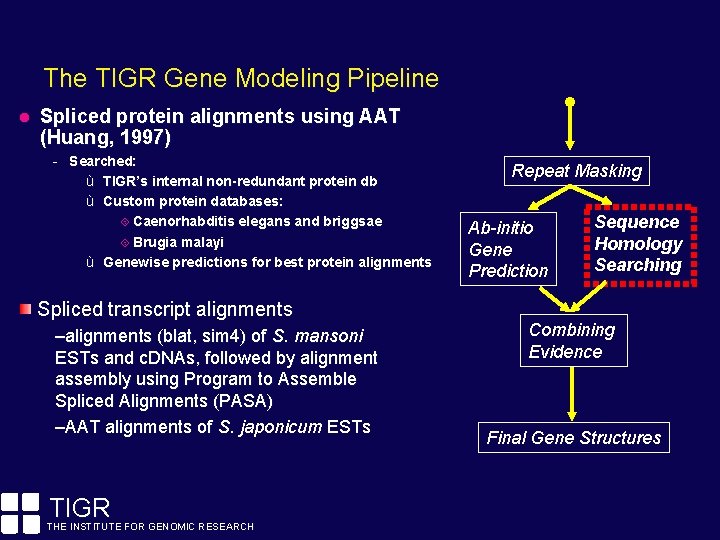
The TIGR Gene Modeling Pipeline Spliced protein alignments using AAT (Huang, 1997) - Searched: ù TIGR’s internal non-redundant protein db ù Custom protein databases: Caenorhabditis elegans and briggsae Brugia malayi ù Genewise predictions for best protein alignments Spliced transcript alignments –alignments (blat, sim 4) of S. mansoni ESTs and c. DNAs, followed by alignment assembly using Program to Assemble Spliced Alignments (PASA) –AAT alignments of S. japonicum ESTs TIGR THE INSTITUTE FOR GENOMIC RESEARCH Repeat Masking Ab-initio Gene Prediction Sequence Homology Searching Combining Evidence Final Gene Structures
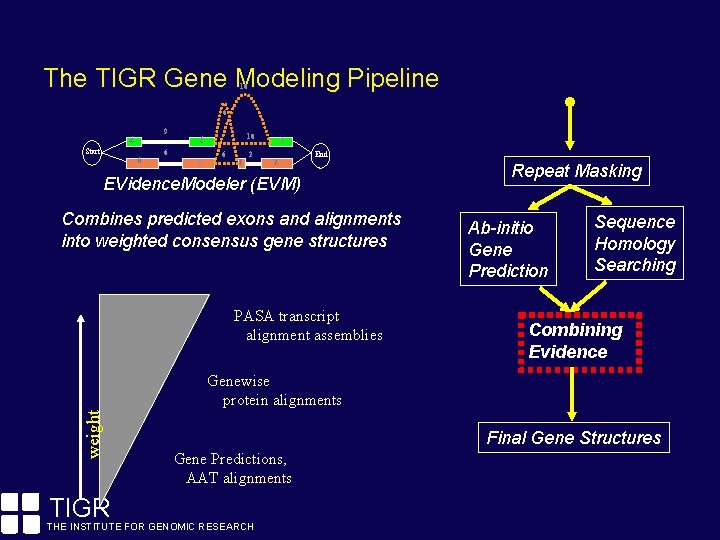
The TIGR Gene Modeling Pipeline 10 6 9 4 Start 6 10 6 6 7 6 1 2 6 7 End EVidence. Modeler (EVM) Combines predicted exons and alignments into weighted consensus gene structures PASA transcript alignment assemblies Repeat Masking Ab-initio Gene Prediction Sequence Homology Searching Combining Evidence weight Genewise protein alignments TIGR Final Gene Structures Gene Predictions, AAT alignments THE INSTITUTE FOR GENOMIC RESEARCH
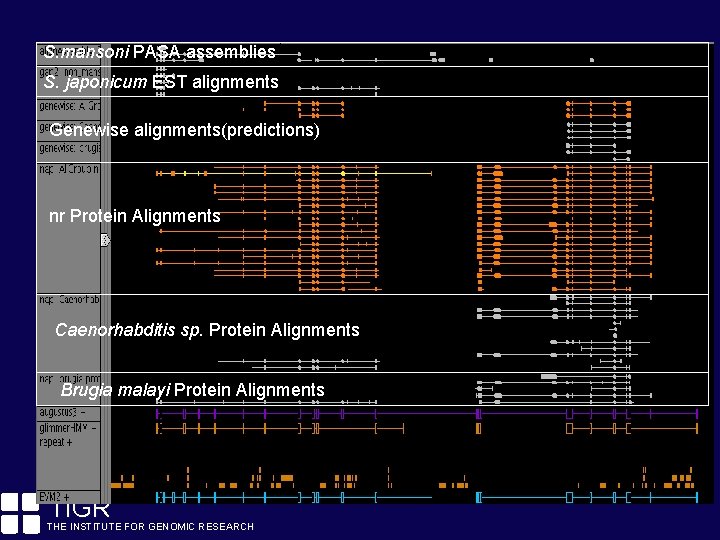
S. mansoni. View PASA assemblies Evidence S. japonicum EST alignments Genewise alignments(predictions) nr Protein Alignments Caenorhabditis sp. Protein Alignments Brugia malayi Protein Alignments TIGR THE INSTITUTE FOR GENOMIC RESEARCH
Genome assembly
Scale annotation pipeline
Tomato genome annotation
Semi-global alignment
Arithmetic and geometric sequences and series
10-2 practice arithmetic sequences and series
Unit 10 sequences and series homework 1 answers
Chapter 12 sequences and series answers
10-3 geometric sequences and series
Module 12 sequences and series
Sum of infinite geometric series
10-2 practice arithmetic sequences and series
10-1 sequences series and sigma notation
Aritmetic formula
Geometric and arithmetic sequences formulas
Explicit arithmetic formula
Geometric and arithmetic sequences formulas