CHI SQUARE 2 Dangerous Curves Ahead Why Chi
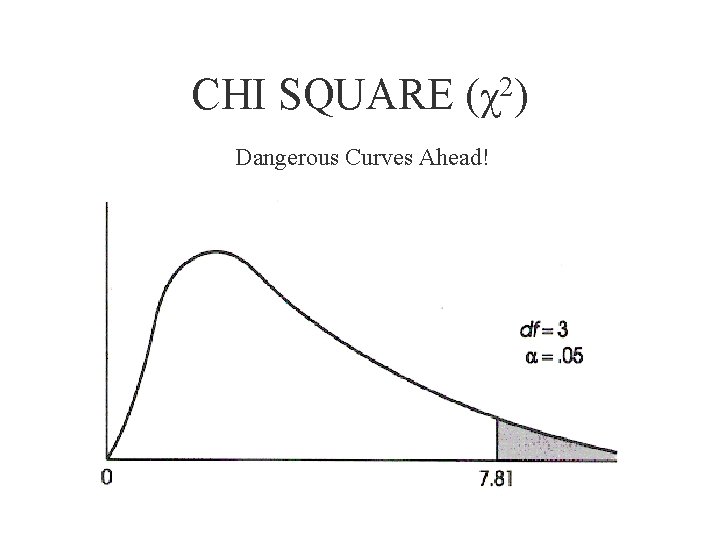
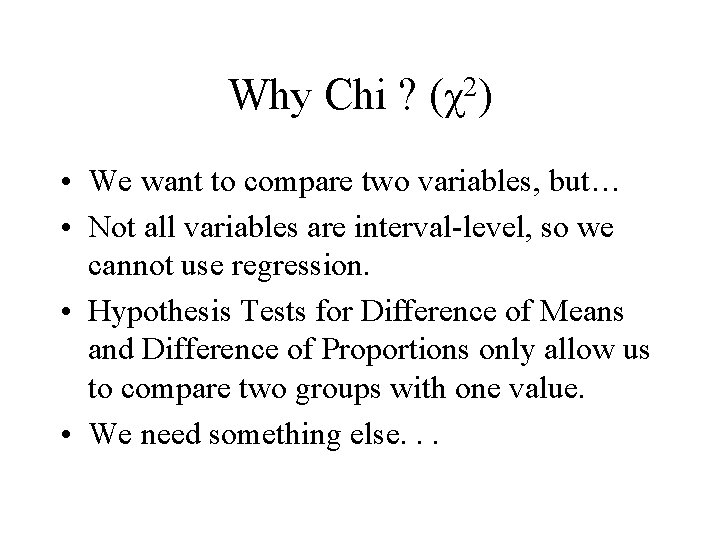
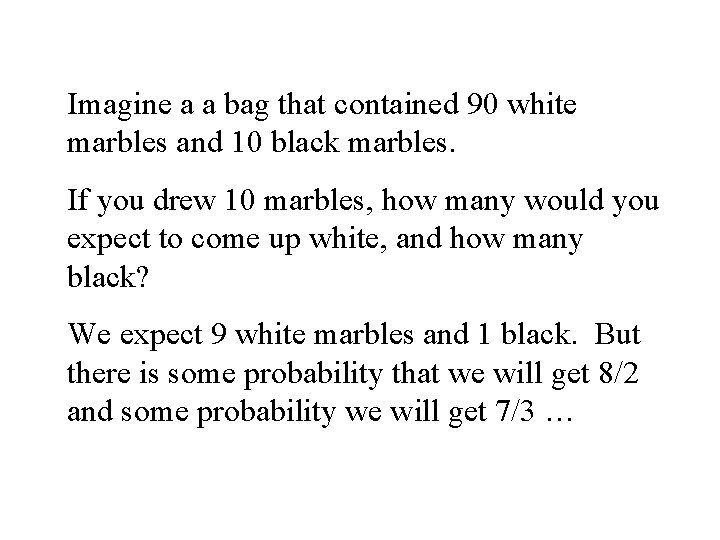
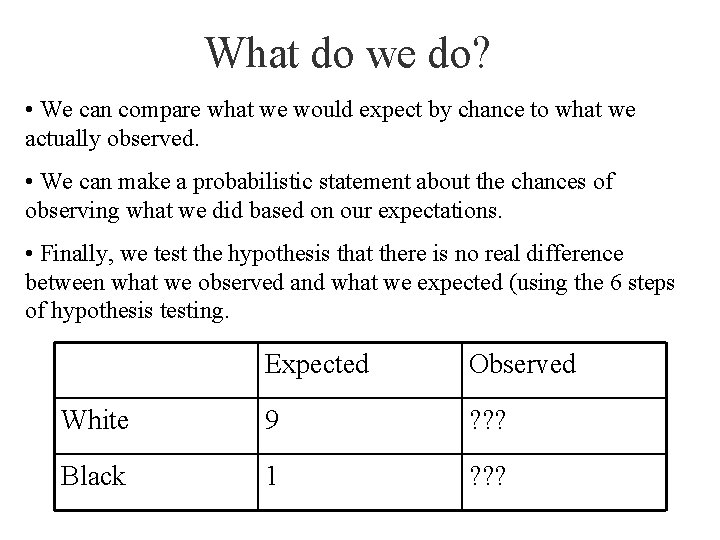
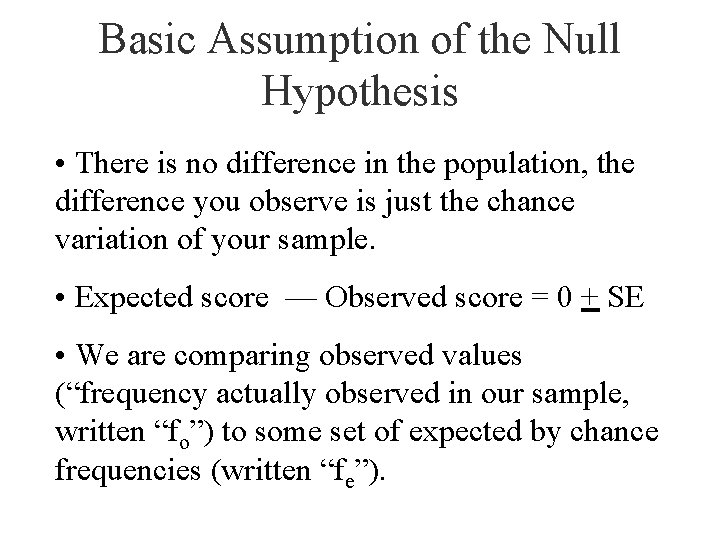
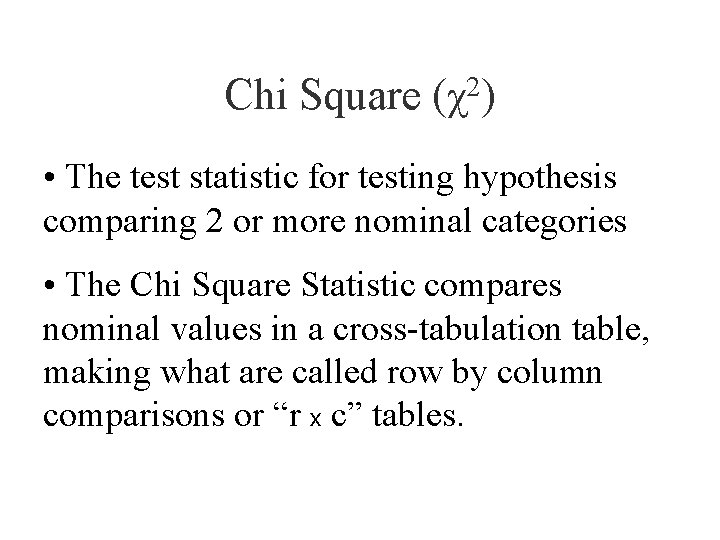
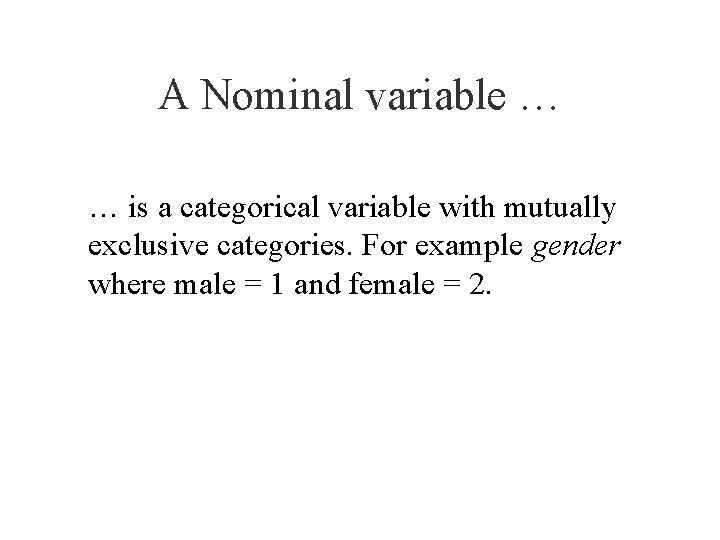
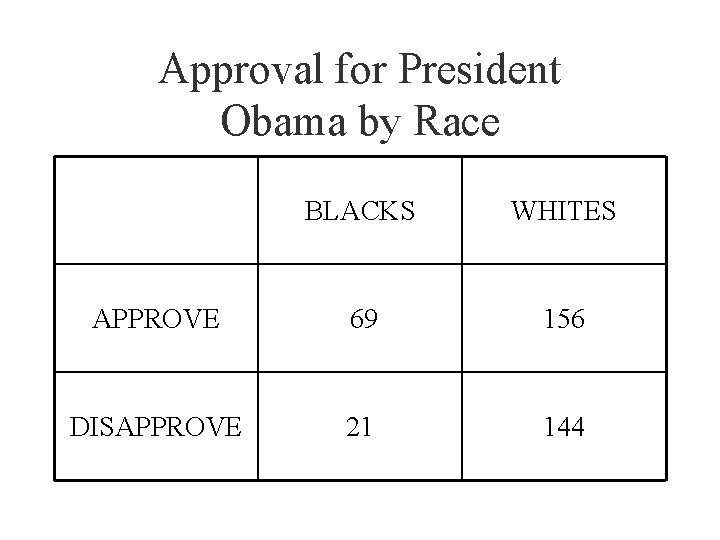
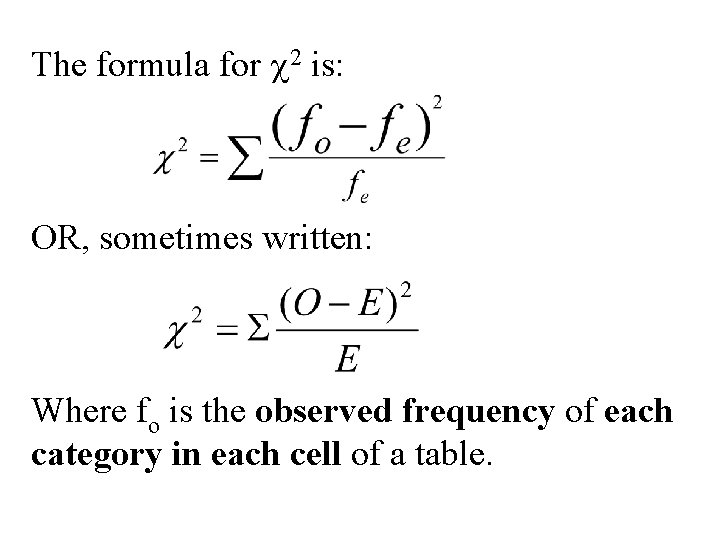
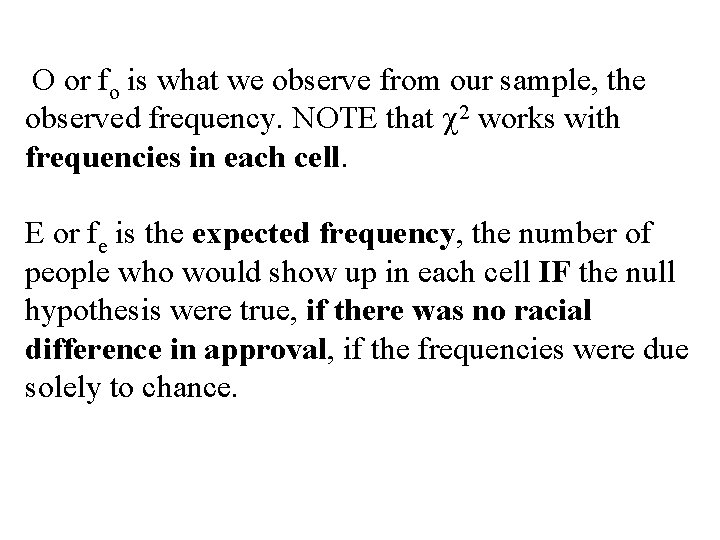
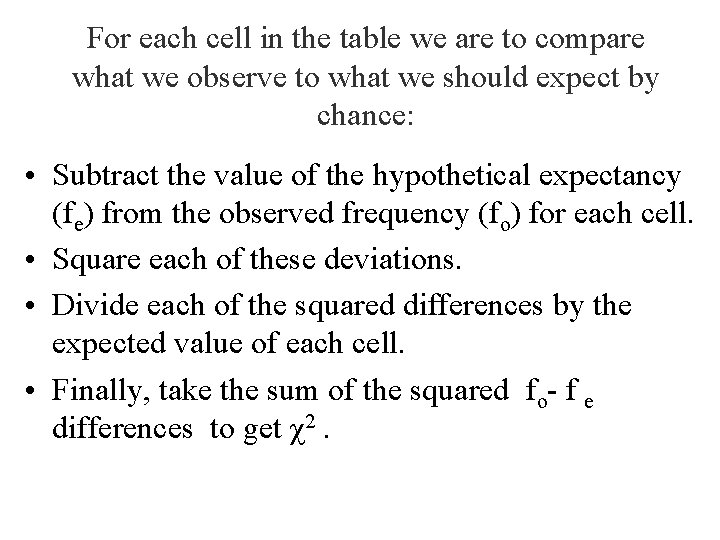
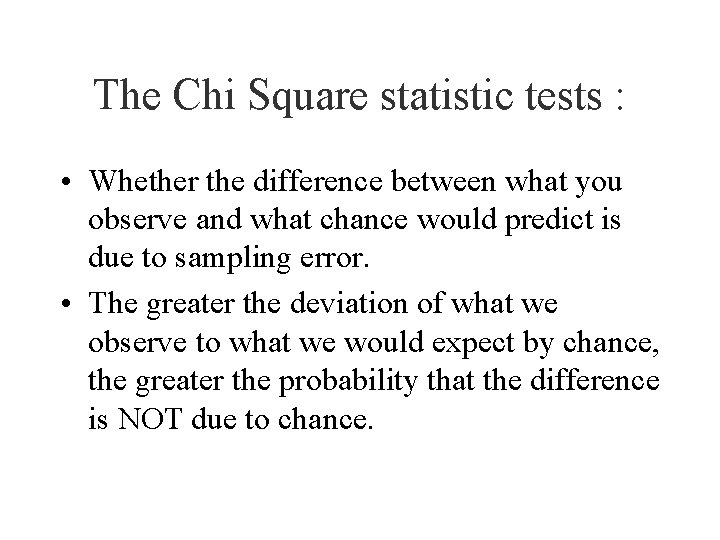
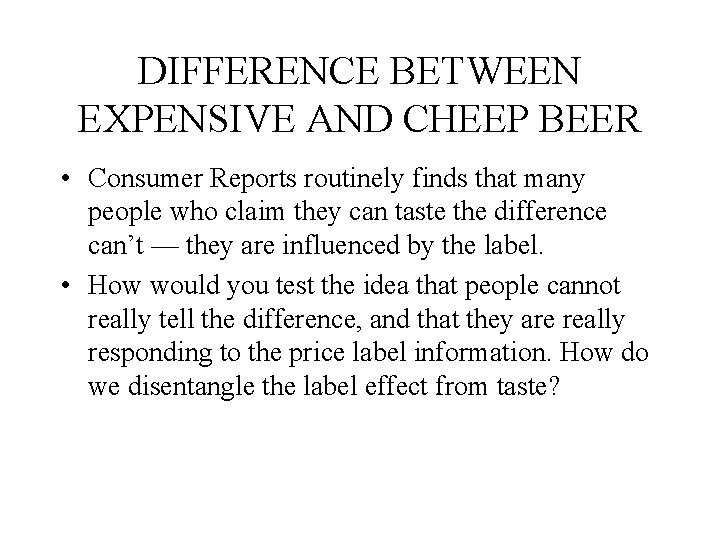
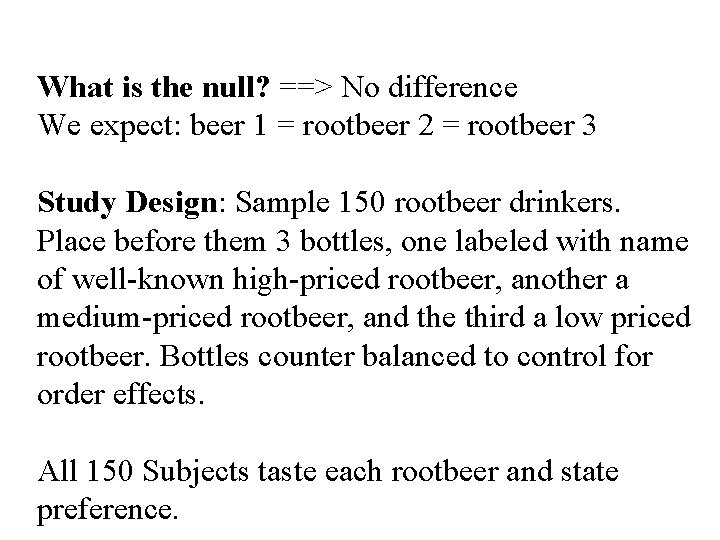
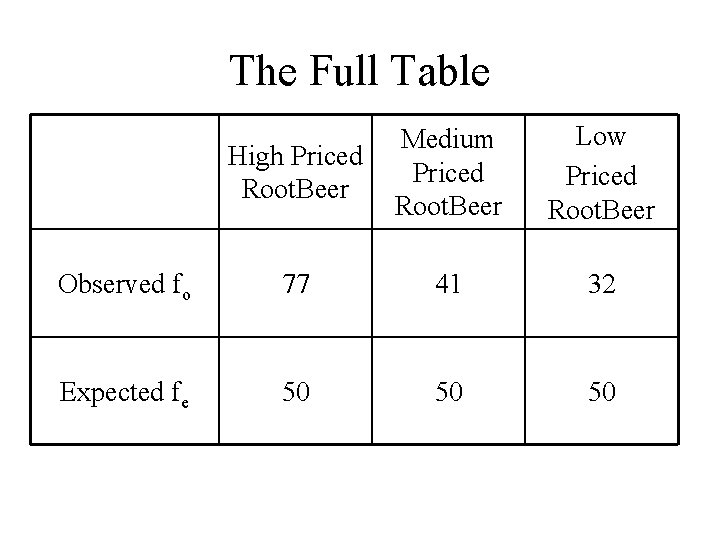
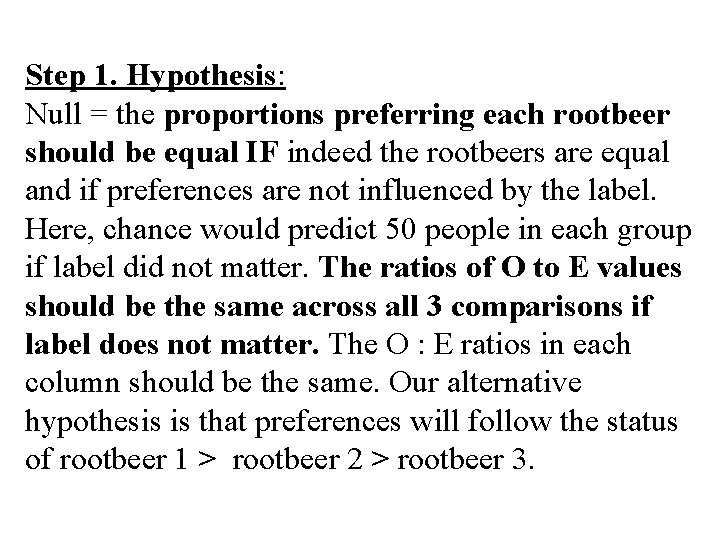
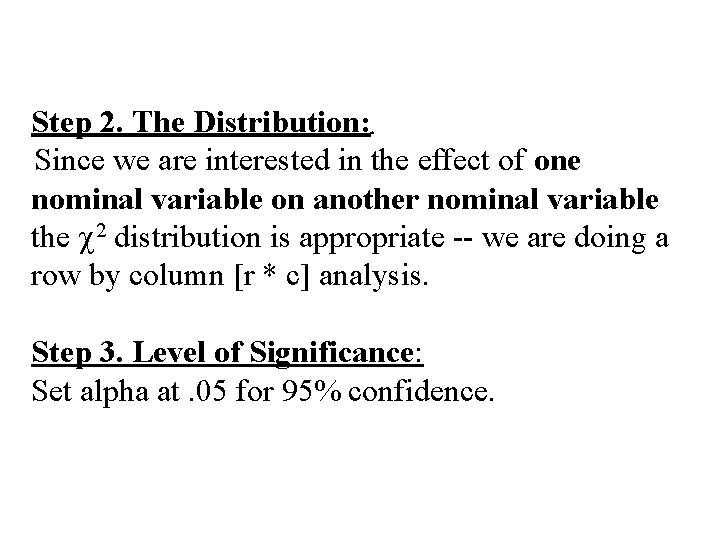
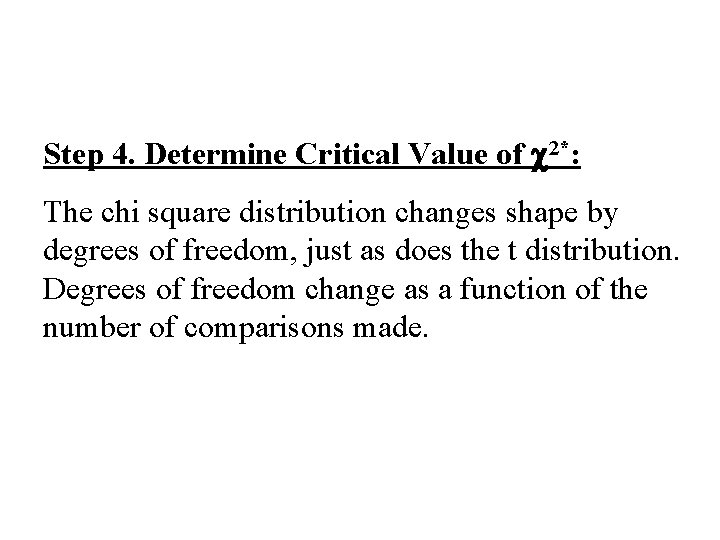
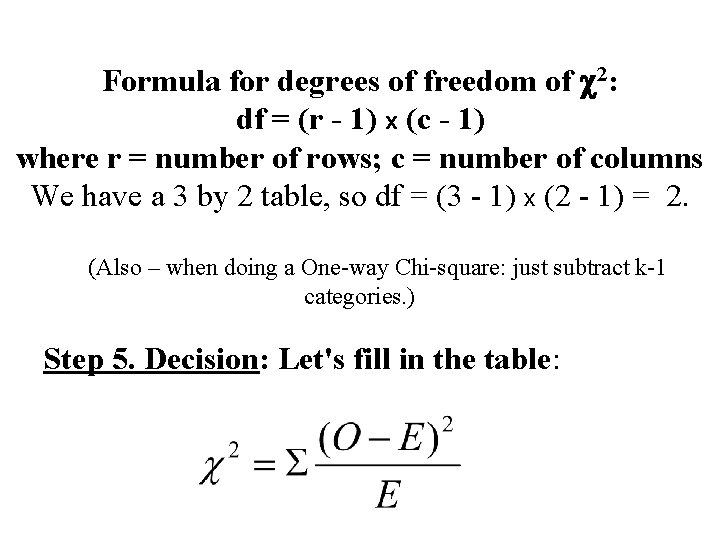
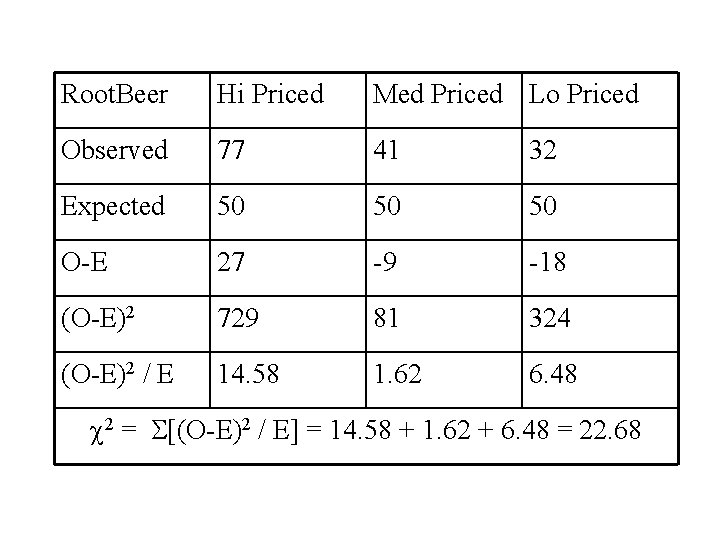
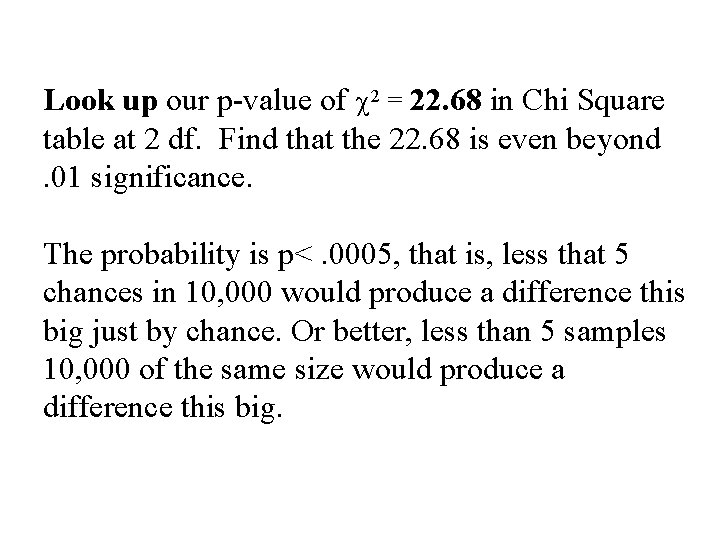
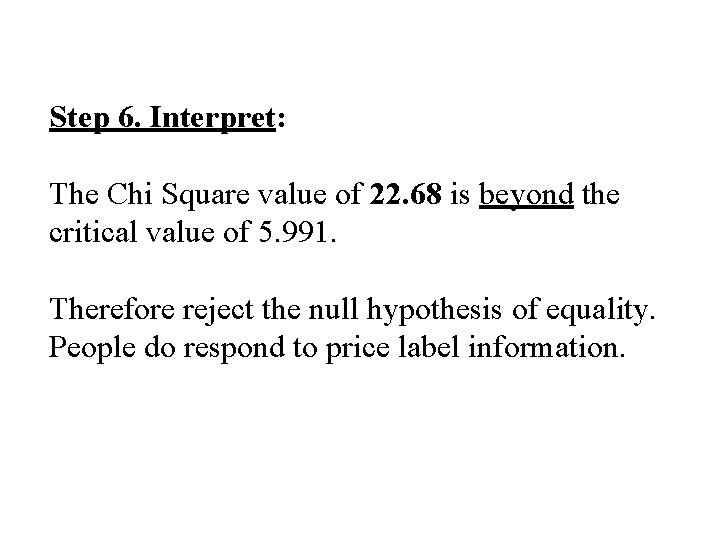
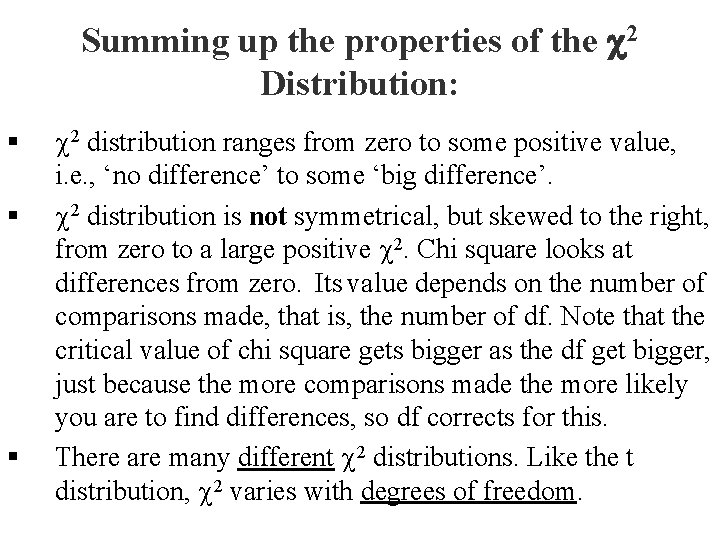
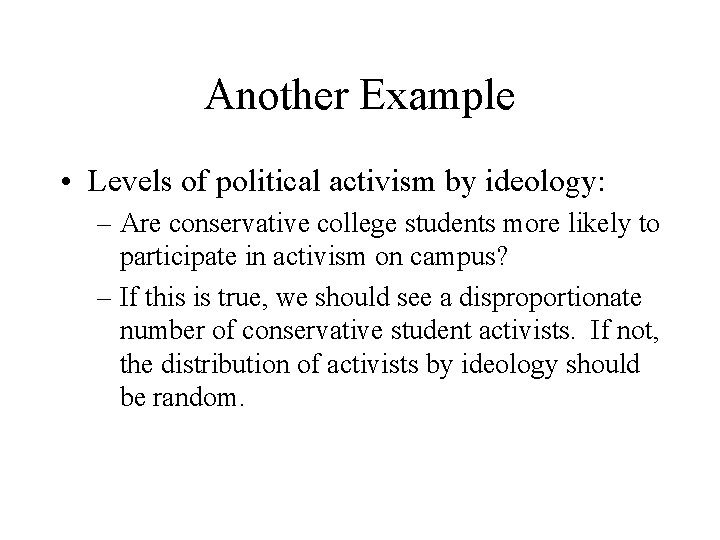
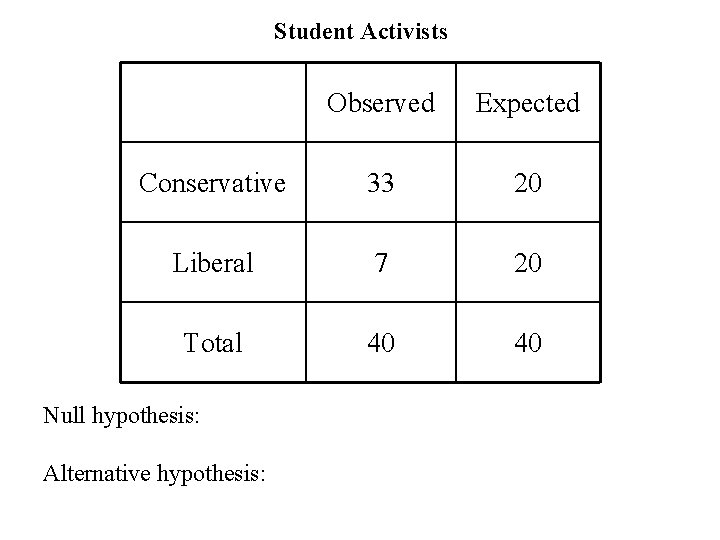
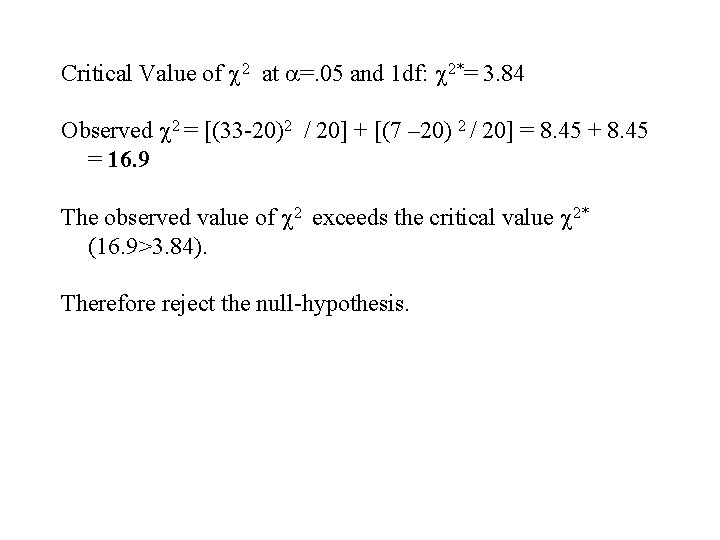
- Slides: 26
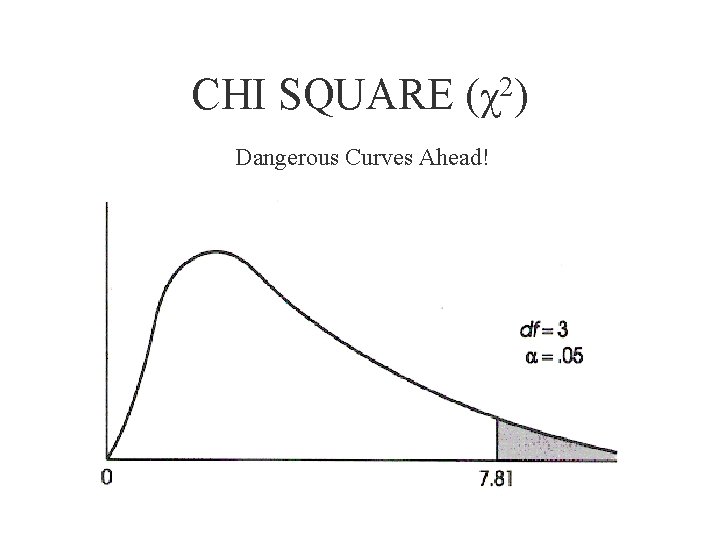
CHI SQUARE 2 (χ ) Dangerous Curves Ahead!
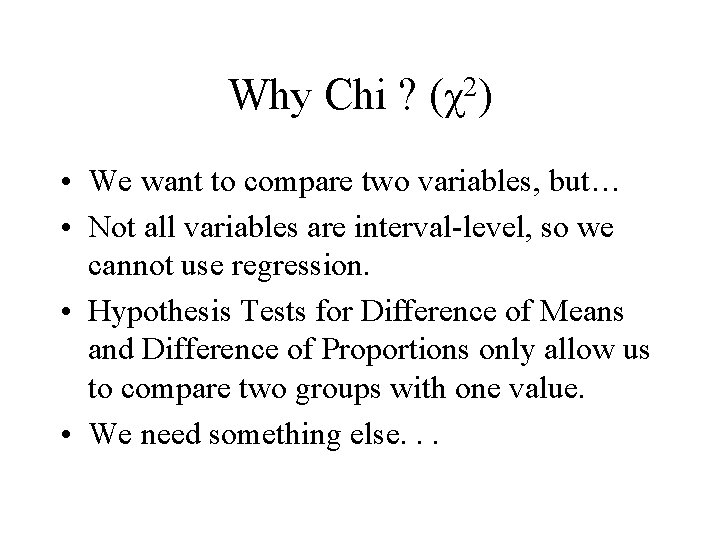
Why Chi ? 2 (χ ) • We want to compare two variables, but… • Not all variables are interval-level, so we cannot use regression. • Hypothesis Tests for Difference of Means and Difference of Proportions only allow us to compare two groups with one value. • We need something else. . .
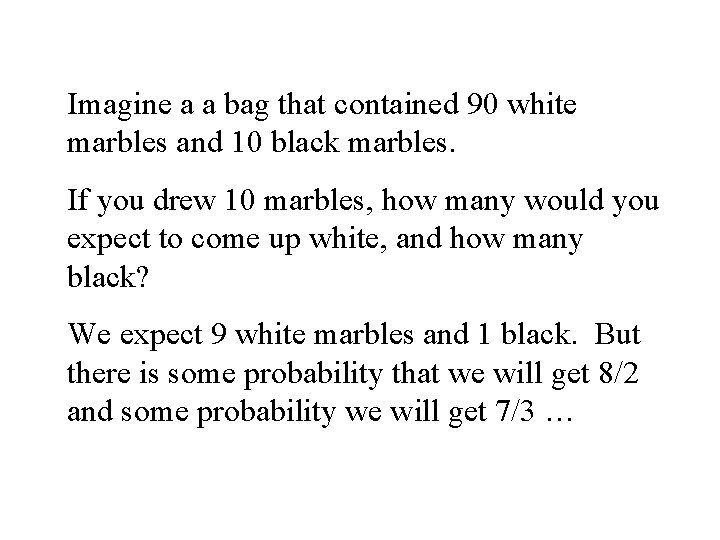
Imagine a a bag that contained 90 white marbles and 10 black marbles. If you drew 10 marbles, how many would you expect to come up white, and how many black? We expect 9 white marbles and 1 black. But there is some probability that we will get 8/2 and some probability we will get 7/3 …
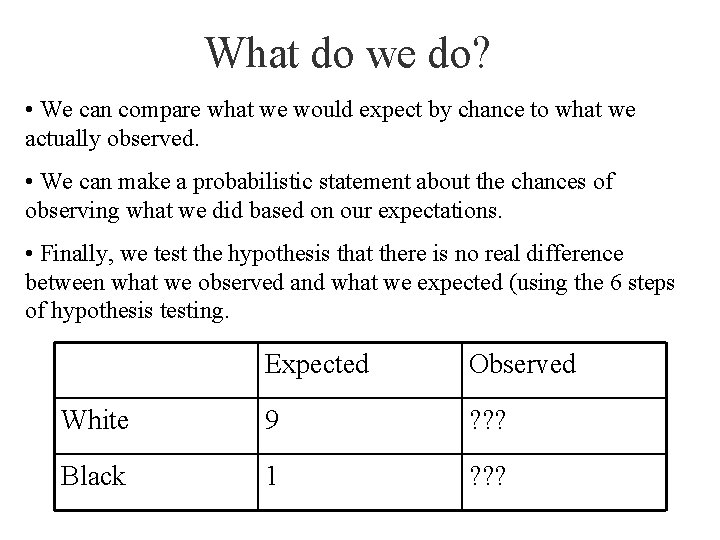
What do we do? • We can compare what we would expect by chance to what we actually observed. • We can make a probabilistic statement about the chances of observing what we did based on our expectations. • Finally, we test the hypothesis that there is no real difference between what we observed and what we expected (using the 6 steps of hypothesis testing. Expected Observed White 9 ? ? ? Black 1 ? ? ?
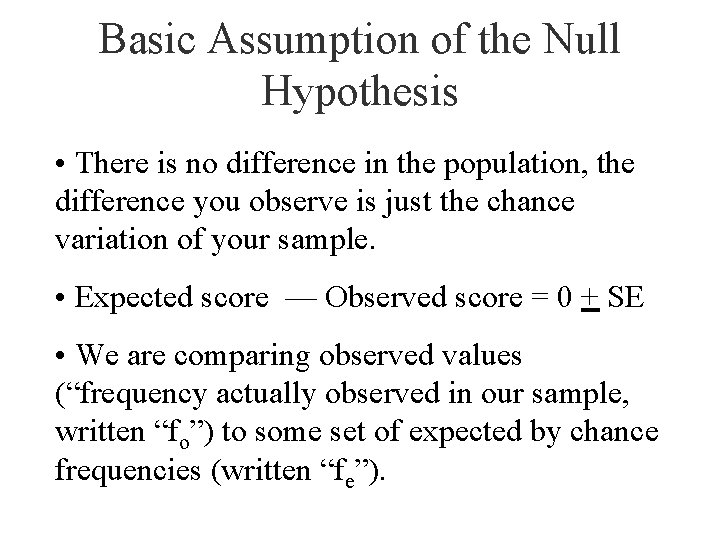
Basic Assumption of the Null Hypothesis • There is no difference in the population, the difference you observe is just the chance variation of your sample. • Expected score — Observed score = 0 + SE • We are comparing observed values (“frequency actually observed in our sample, written “fo”) to some set of expected by chance frequencies (written “fe”).
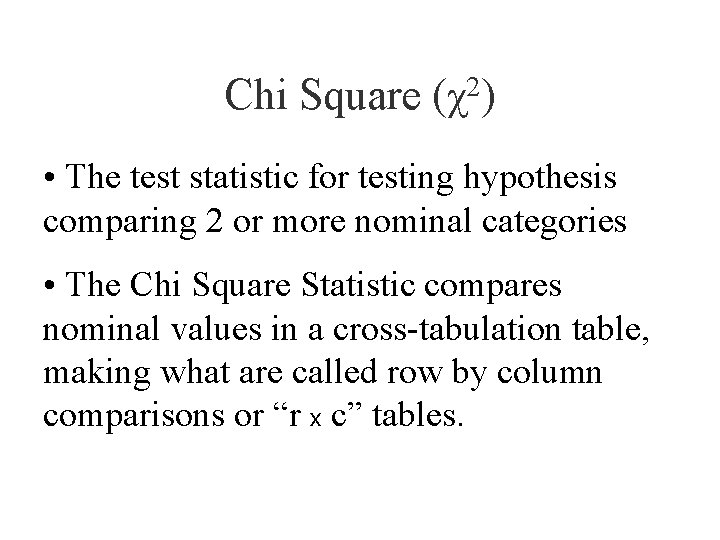
Chi Square 2 (χ ) • The test statistic for testing hypothesis comparing 2 or more nominal categories • The Chi Square Statistic compares nominal values in a cross-tabulation table, making what are called row by column comparisons or “r x c” tables.
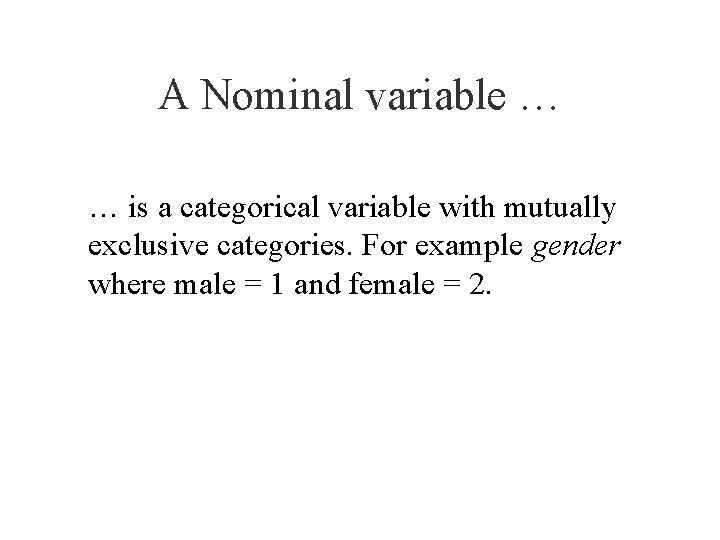
A Nominal variable … … is a categorical variable with mutually exclusive categories. For example gender where male = 1 and female = 2.
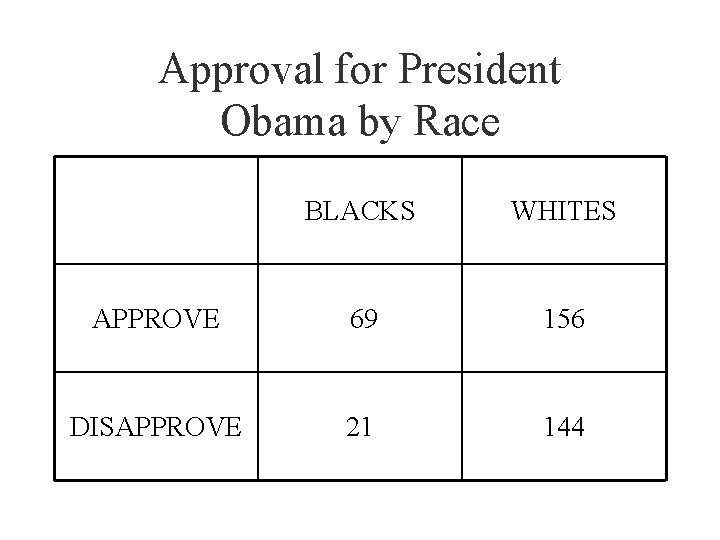
Approval for President Obama by Race BLACKS WHITES APPROVE 69 156 DISAPPROVE 21 144
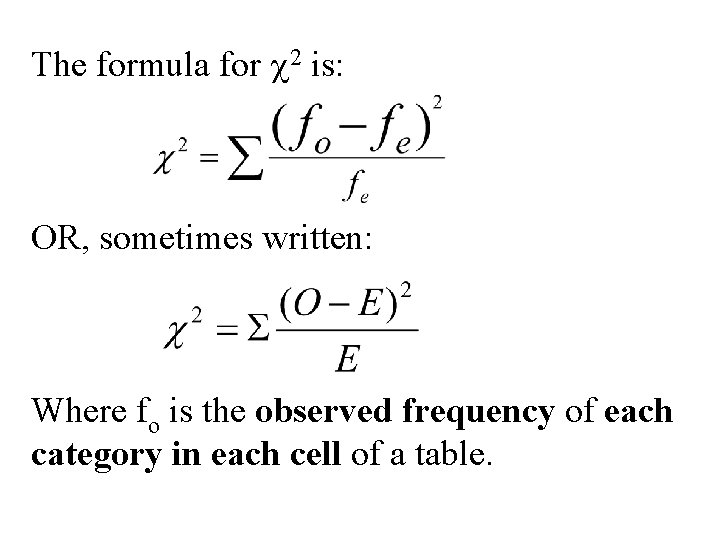
The formula for c 2 is: OR, sometimes written: Where fo is the observed frequency of each category in each cell of a table.
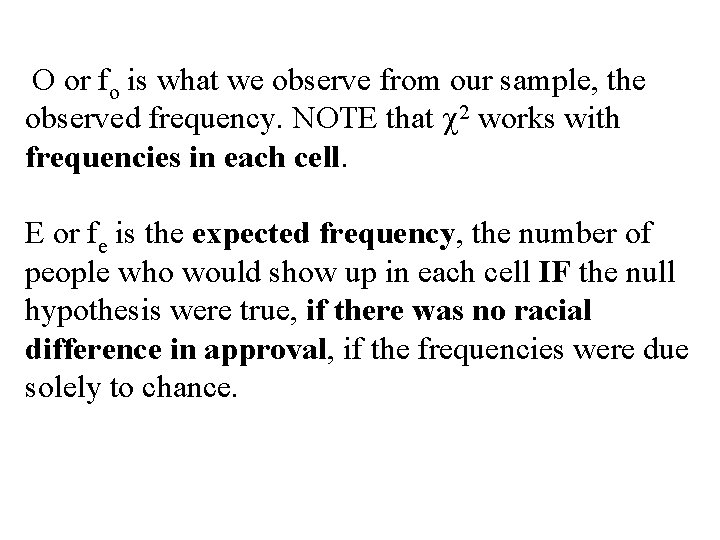
O or fo is what we observe from our sample, the observed frequency. NOTE that c 2 works with frequencies in each cell. E or fe is the expected frequency, the number of people who would show up in each cell IF the null hypothesis were true, if there was no racial difference in approval, if the frequencies were due solely to chance.
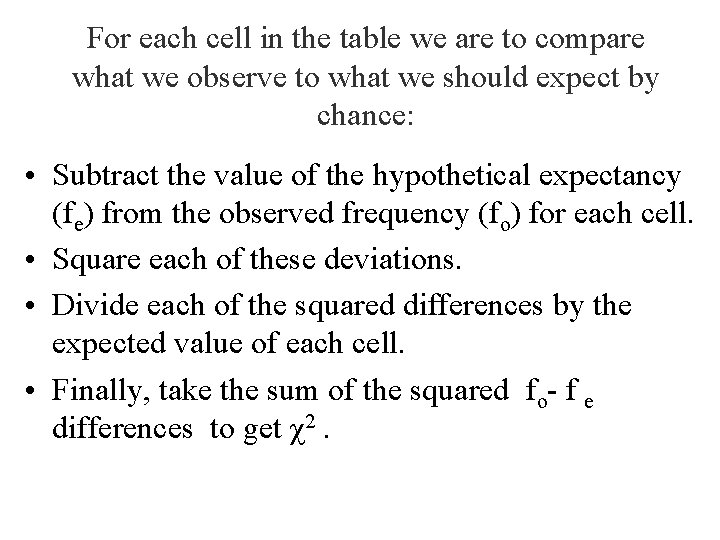
For each cell in the table we are to compare what we observe to what we should expect by chance: • Subtract the value of the hypothetical expectancy (fe) from the observed frequency (fo) for each cell. • Square each of these deviations. • Divide each of the squared differences by the expected value of each cell. • Finally, take the sum of the squared fo- f e differences to get χ2.
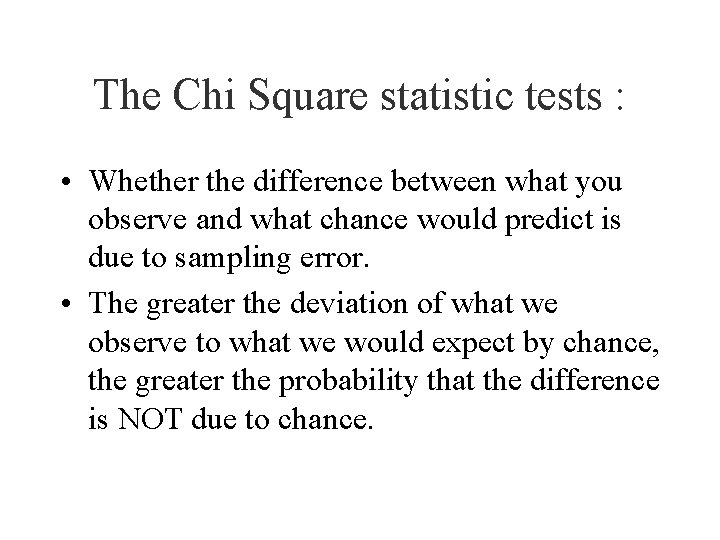
The Chi Square statistic tests : • Whether the difference between what you observe and what chance would predict is due to sampling error. • The greater the deviation of what we observe to what we would expect by chance, the greater the probability that the difference is NOT due to chance.
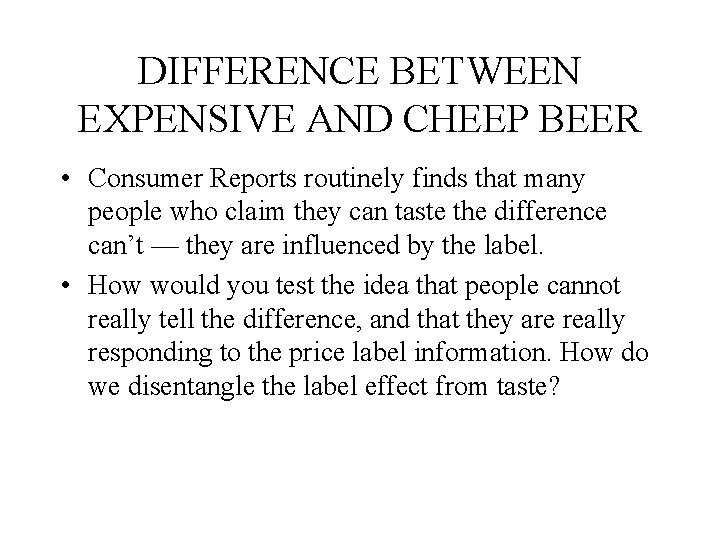
DIFFERENCE BETWEEN EXPENSIVE AND CHEEP BEER • Consumer Reports routinely finds that many people who claim they can taste the difference can’t — they are influenced by the label. • How would you test the idea that people cannot really tell the difference, and that they are really responding to the price label information. How do we disentangle the label effect from taste?
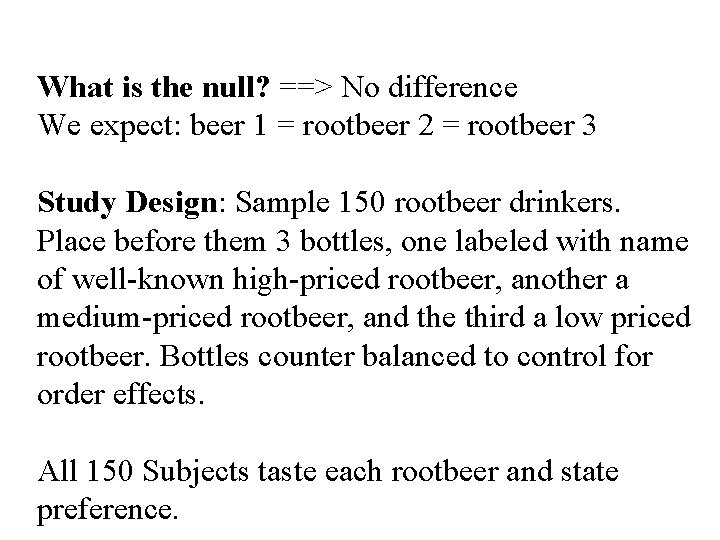
What is the null? ==> No difference We expect: beer 1 = rootbeer 2 = rootbeer 3 Study Design: Sample 150 rootbeer drinkers. Place before them 3 bottles, one labeled with name of well-known high-priced rootbeer, another a medium-priced rootbeer, and the third a low priced rootbeer. Bottles counter balanced to control for order effects. All 150 Subjects taste each rootbeer and state preference.
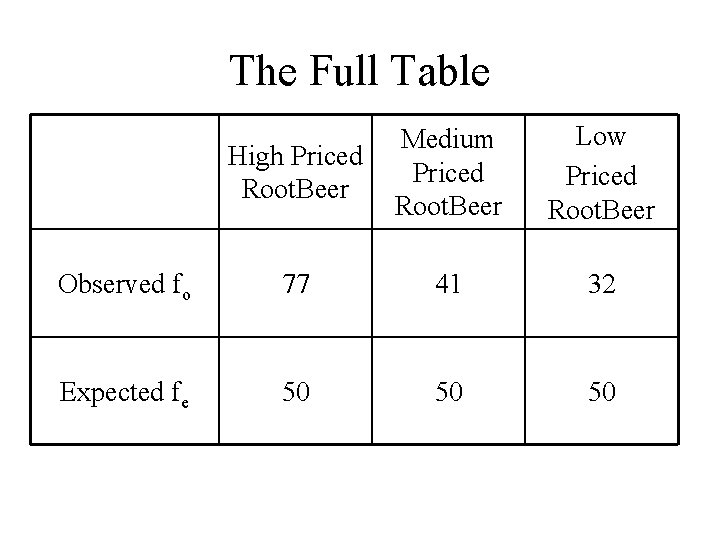
The Full Table High Priced Root. Beer Medium Priced Root. Beer Low Priced Root. Beer Observed fo 77 41 32 Expected fe 50 50 50
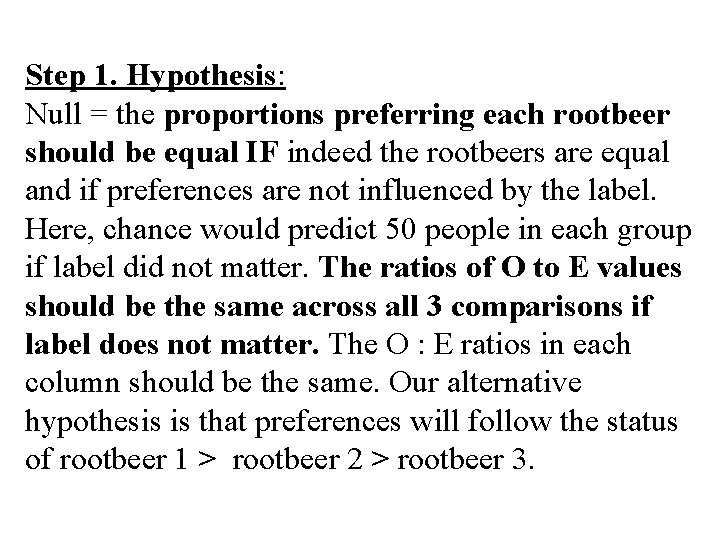
Step 1. Hypothesis: Null = the proportions preferring each rootbeer should be equal IF indeed the rootbeers are equal and if preferences are not influenced by the label. Here, chance would predict 50 people in each group if label did not matter. The ratios of O to E values should be the same across all 3 comparisons if label does not matter. The O : E ratios in each column should be the same. Our alternative hypothesis is that preferences will follow the status of rootbeer 1 > rootbeer 2 > rootbeer 3.
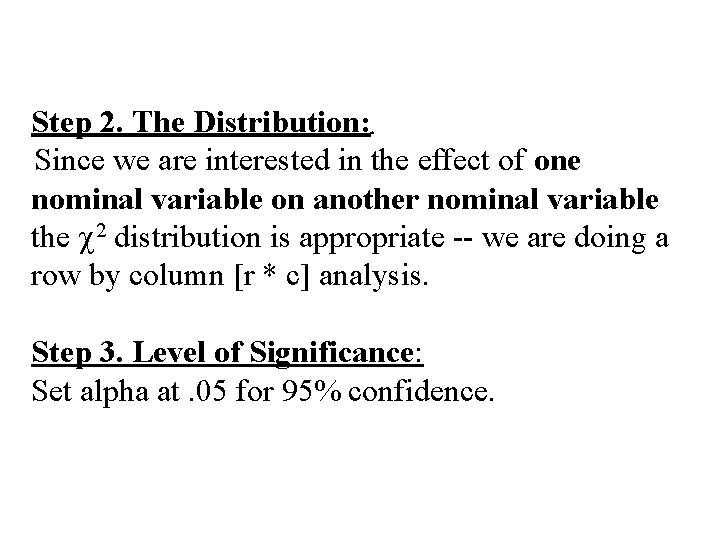
Step 2. The Distribution: Since we are interested in the effect of one nominal variable on another nominal variable the c 2 distribution is appropriate -- we are doing a row by column [r * c] analysis. . Step 3. Level of Significance: Set alpha at. 05 for 95% confidence.
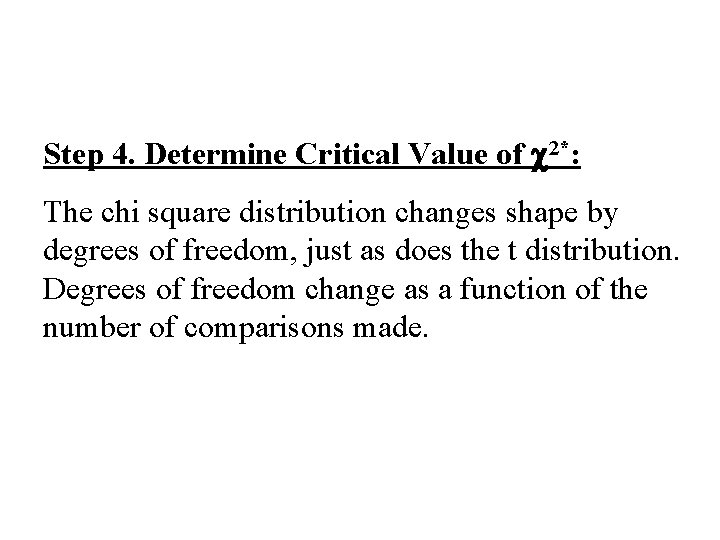
Step 4. Determine Critical Value of c 2*: The chi square distribution changes shape by degrees of freedom, just as does the t distribution. Degrees of freedom change as a function of the number of comparisons made.
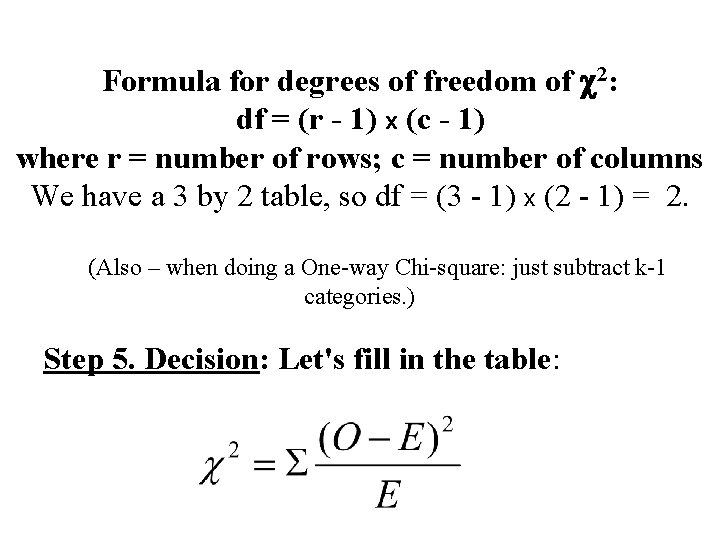
Formula for degrees of freedom of c 2: df = (r - 1) x (c - 1) where r = number of rows; c = number of columns We have a 3 by 2 table, so df = (3 - 1) x (2 - 1) = 2. (Also – when doing a One-way Chi-square: just subtract k-1 categories. ) Step 5. Decision: Let's fill in the table:
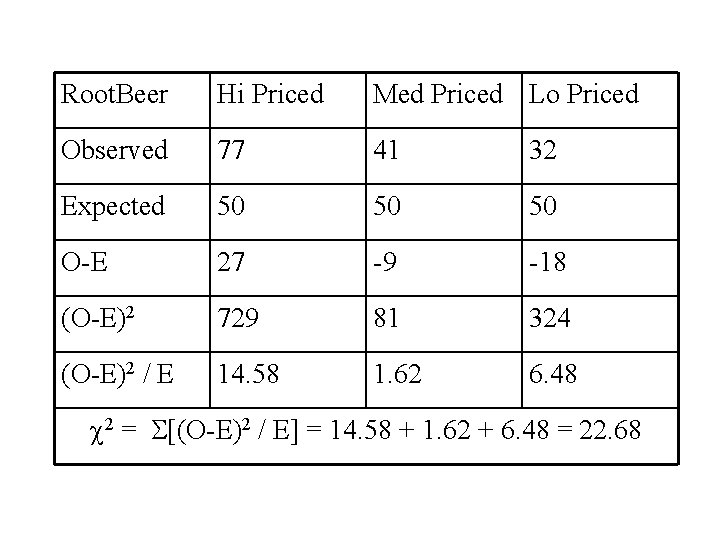
Root. Beer Hi Priced Med Priced Lo Priced Observed 77 41 32 Expected 50 50 50 O-E 27 -9 -18 (O-E)2 729 81 324 (O-E)2 / E 14. 58 1. 62 6. 48 c 2 = S[(O-E)2 / E] = 14. 58 + 1. 62 + 6. 48 = 22. 68
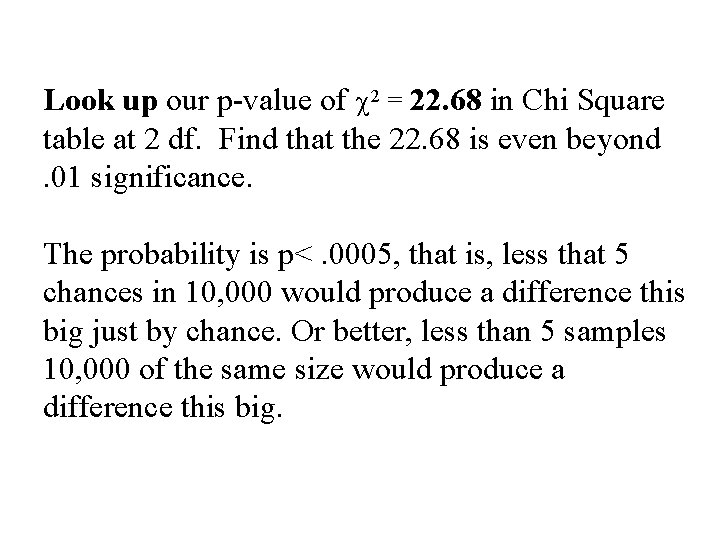
Look up our p-value of c 2 = 22. 68 in Chi Square table at 2 df. Find that the 22. 68 is even beyond. 01 significance. The probability is p<. 0005, that is, less that 5 chances in 10, 000 would produce a difference this big just by chance. Or better, less than 5 samples 10, 000 of the same size would produce a difference this big.
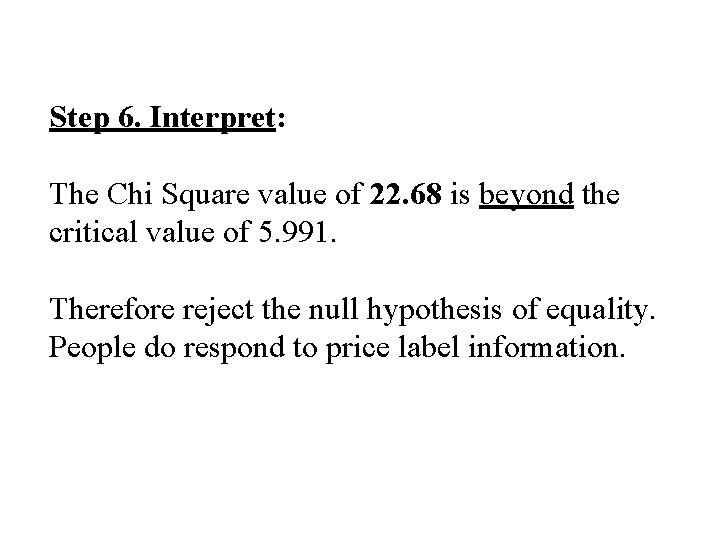
Step 6. Interpret: The Chi Square value of 22. 68 is beyond the critical value of 5. 991. Therefore reject the null hypothesis of equality. People do respond to price label information.
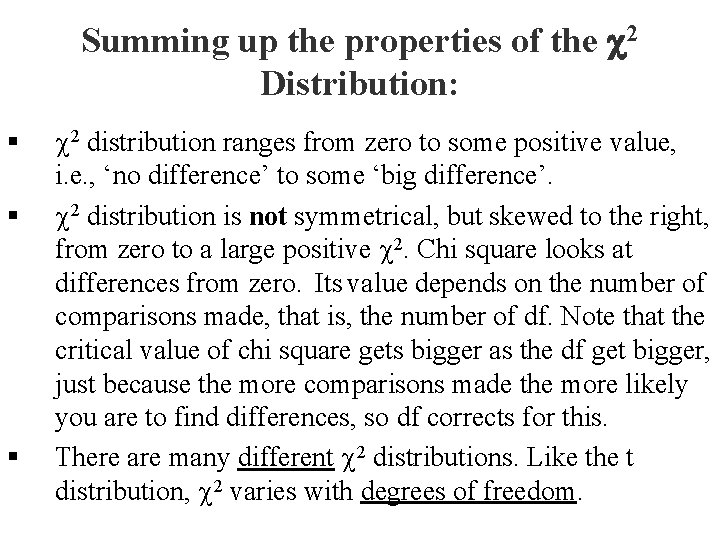
Summing up the properties of the c 2 Distribution: § § § c 2 distribution ranges from zero to some positive value, i. e. , ‘no difference’ to some ‘big difference’. c 2 distribution is not symmetrical, but skewed to the right, from zero to a large positive c 2. Chi square looks at differences from zero. Its value depends on the number of comparisons made, that is, the number of df. Note that the critical value of chi square gets bigger as the df get bigger, just because the more comparisons made the more likely you are to find differences, so df corrects for this. There are many different c 2 distributions. Like the t distribution, c 2 varies with degrees of freedom.
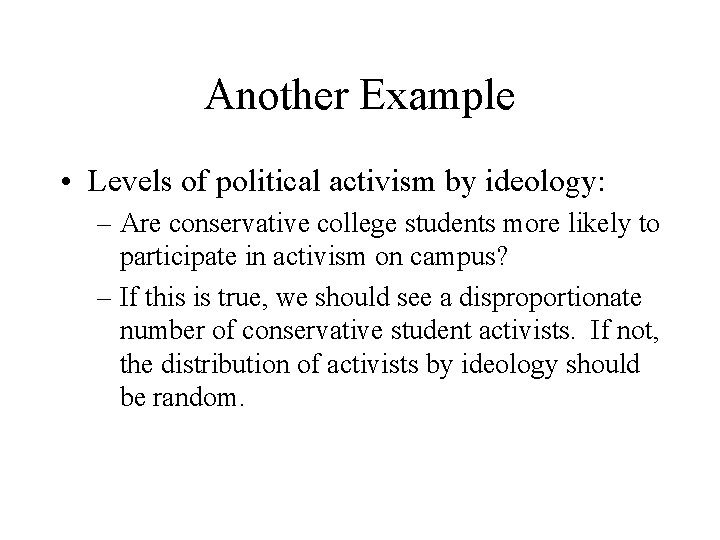
Another Example • Levels of political activism by ideology: – Are conservative college students more likely to participate in activism on campus? – If this is true, we should see a disproportionate number of conservative student activists. If not, the distribution of activists by ideology should be random.
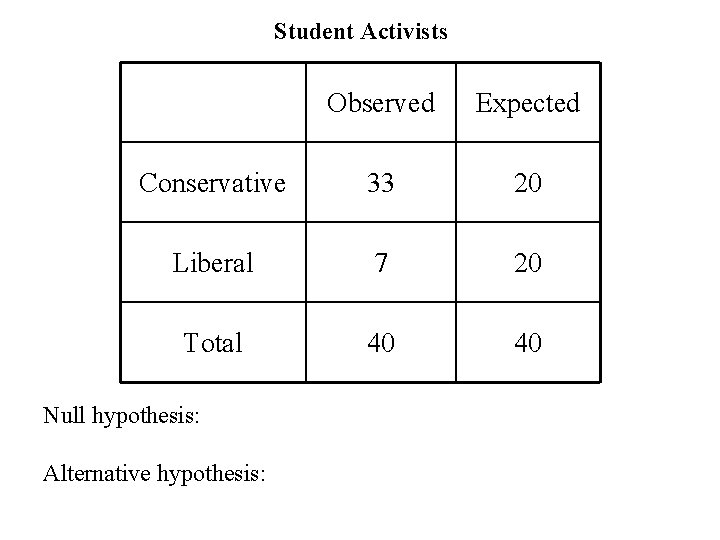
Student Activists Observed Expected Conservative 33 20 Liberal 7 20 Total 40 40 Null hypothesis: Alternative hypothesis:
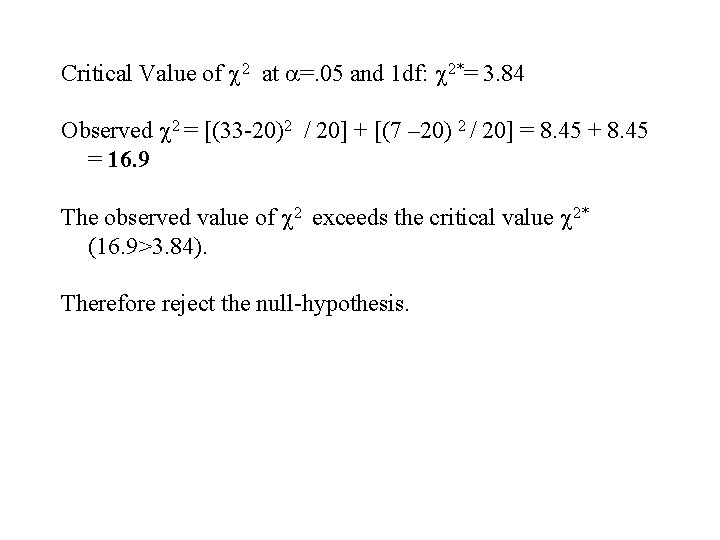
Critical Value of c 2 at a=. 05 and 1 df: c 2*= 3. 84 Observed c 2 = [(33 -20)2 / 20] + [(7 – 20) 2 / 20] = 8. 45 + 8. 45 = 16. 9 The observed value of c 2 exceeds the critical value c 2* (16. 9>3. 84). Therefore reject the null-hypothesis.
The zoo dangerous curves
Andreas carlsson bye bye bye
Why do titration curves flatten out
Why do titration curves flatten out
đổi địa chỉ logic sang địa chỉ vật lý
Chi chi does nnn
Chi chi tg tf
Chi offre e chi domanda lavoro
Dont ask
Conditions for chi-square test
Analisis chi square
Uji chi square
What is chi square used for
What is a chi square test used for
Chi square test goodness of fit
Chi square test for randomness
Tabel chi square
Casinos are required to verify that their games
Uji chi square
Chi square test for linkage
Chi square goodness of fit p value
Chi square conclusion
Chi squared ap bio
Types of chi-square test
Chi square test excel
Chi square symbol
Chi square ap biology