Chapter 18 Multivariate Data Analysis Discriminant Analysis Factor
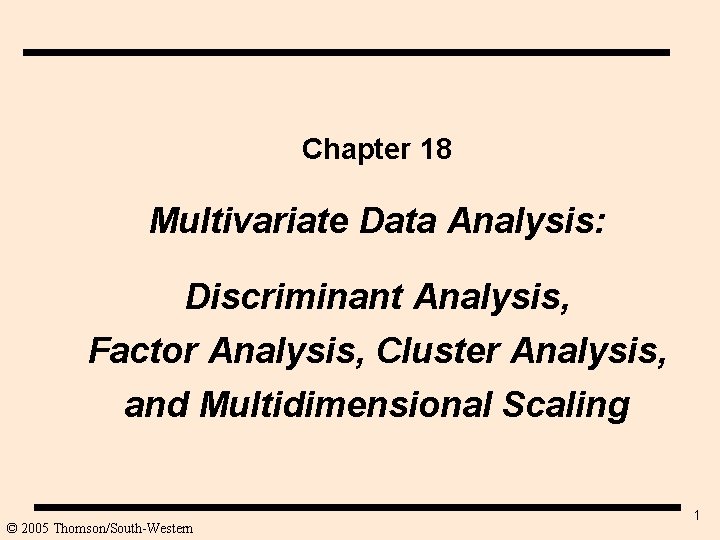
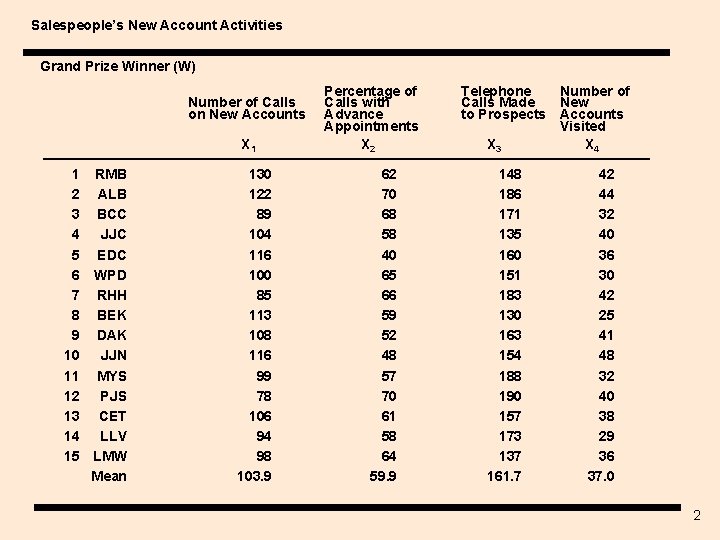
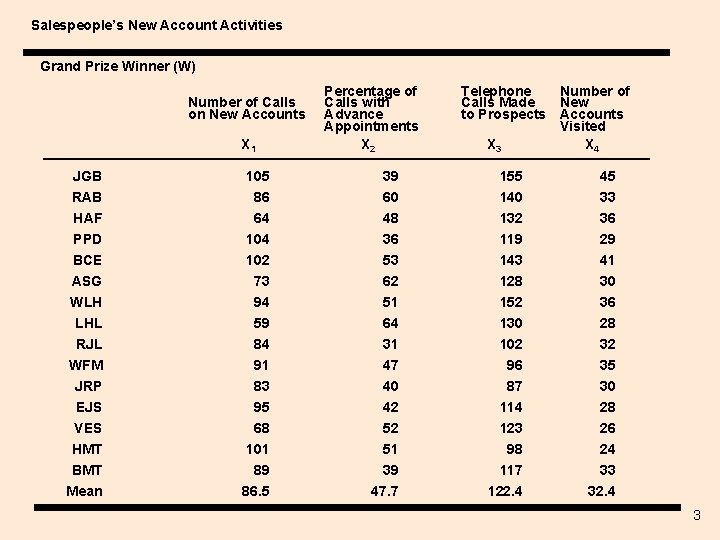
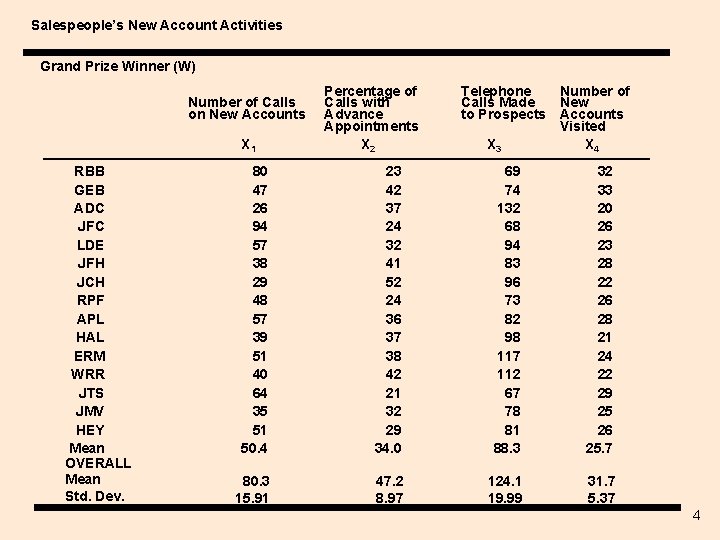
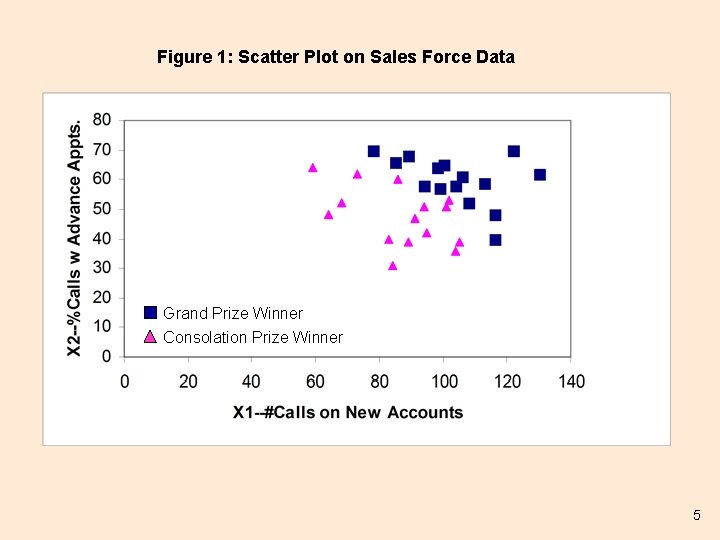
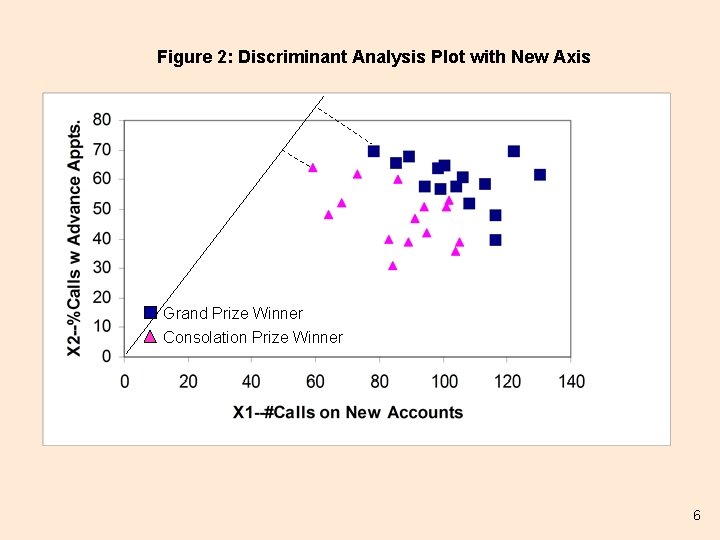
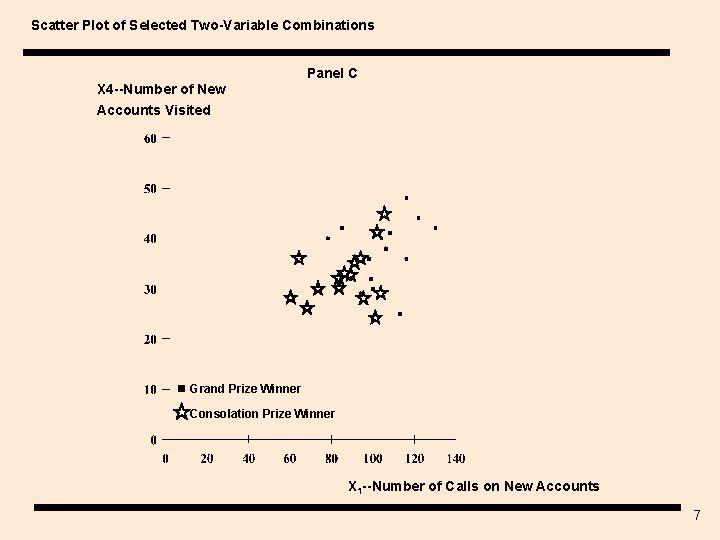
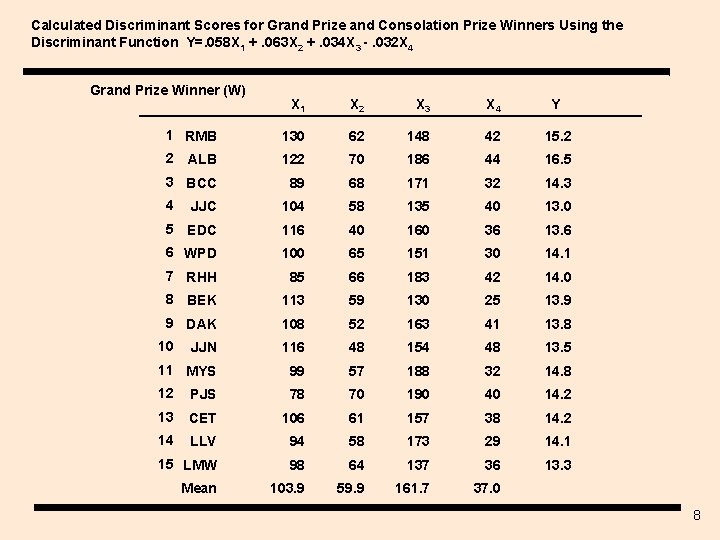
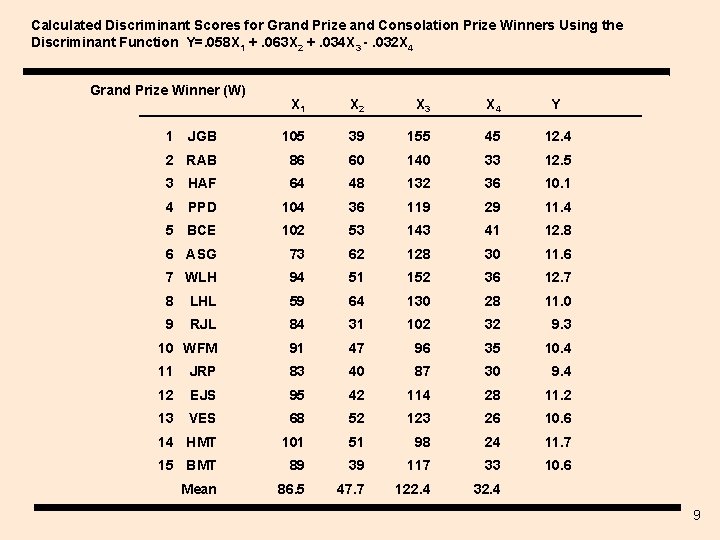
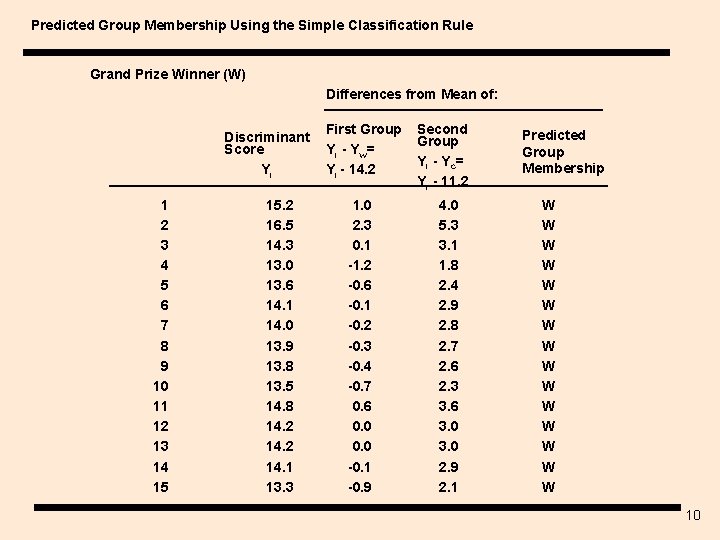
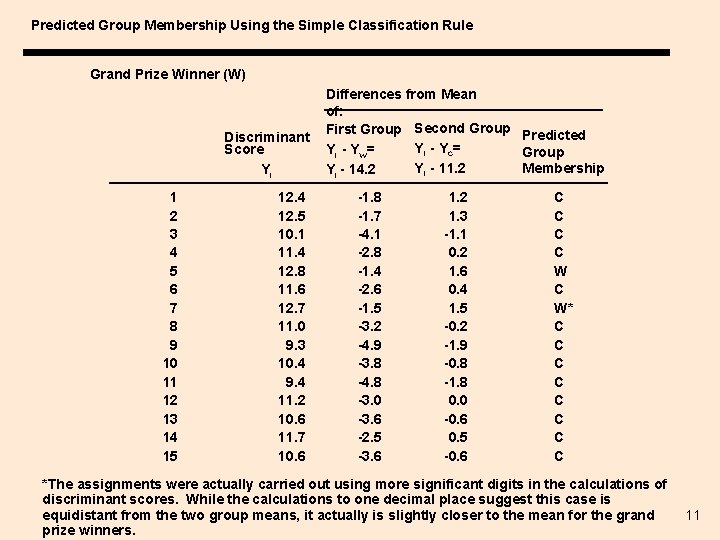
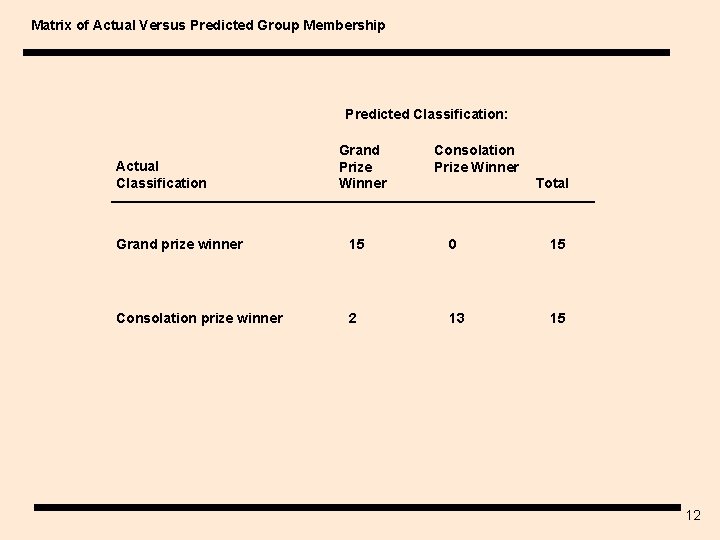
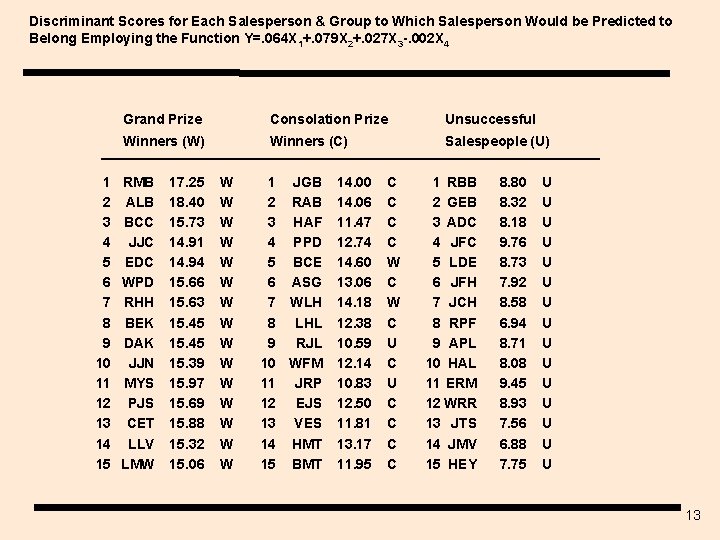
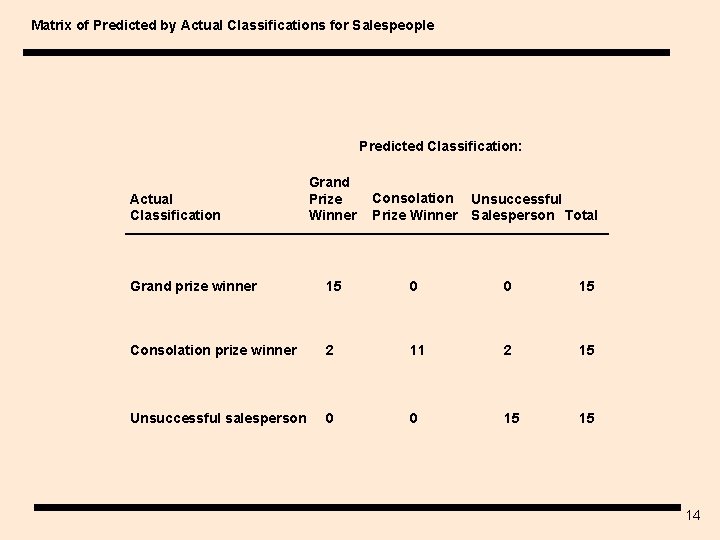
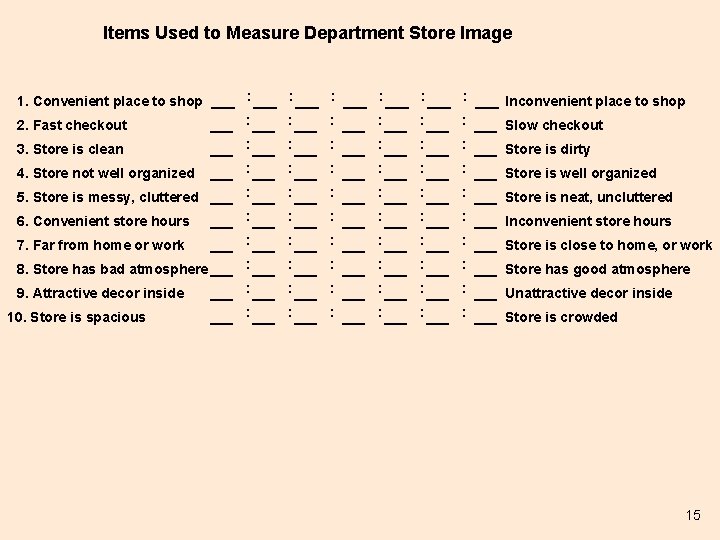
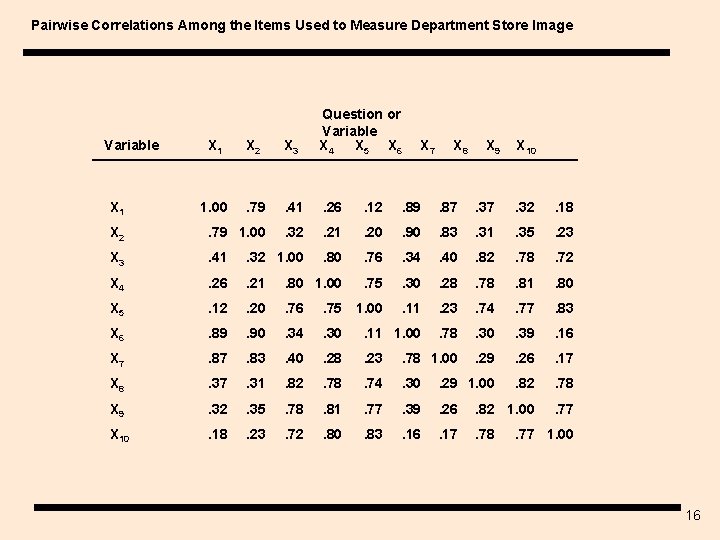
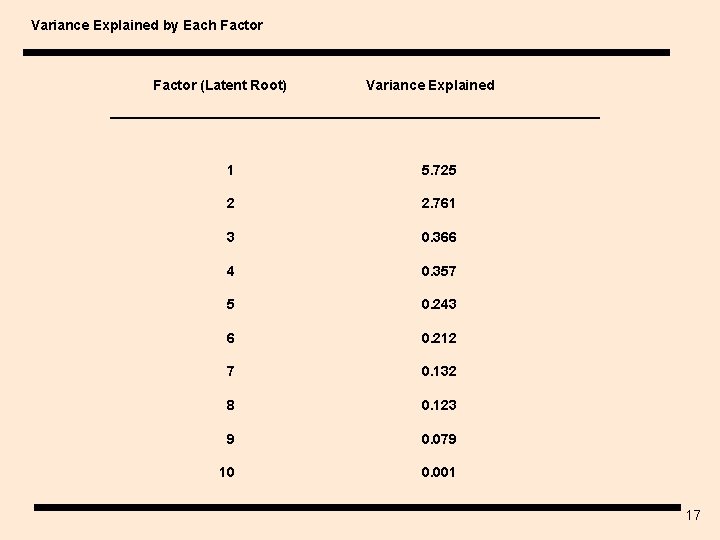
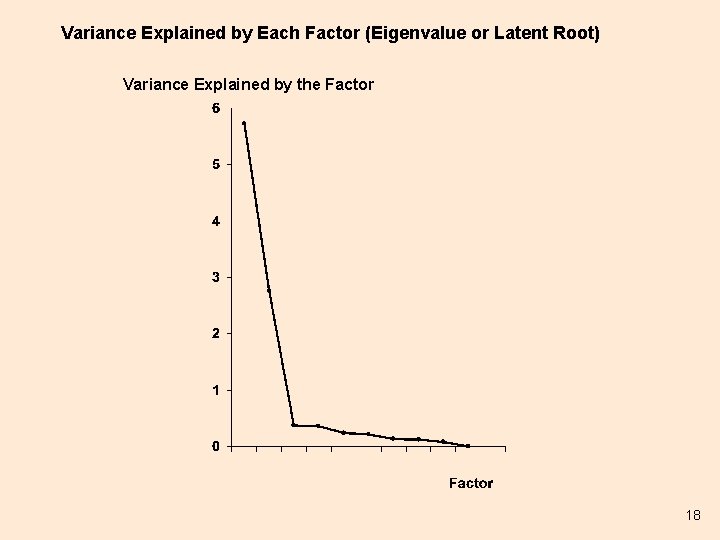
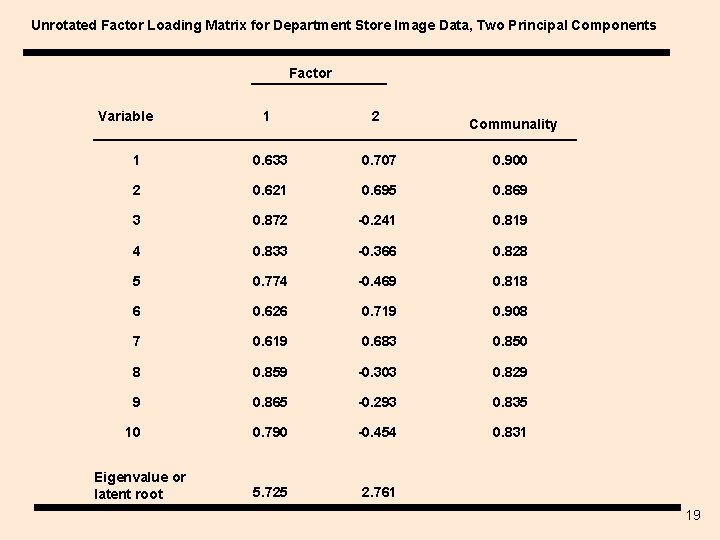
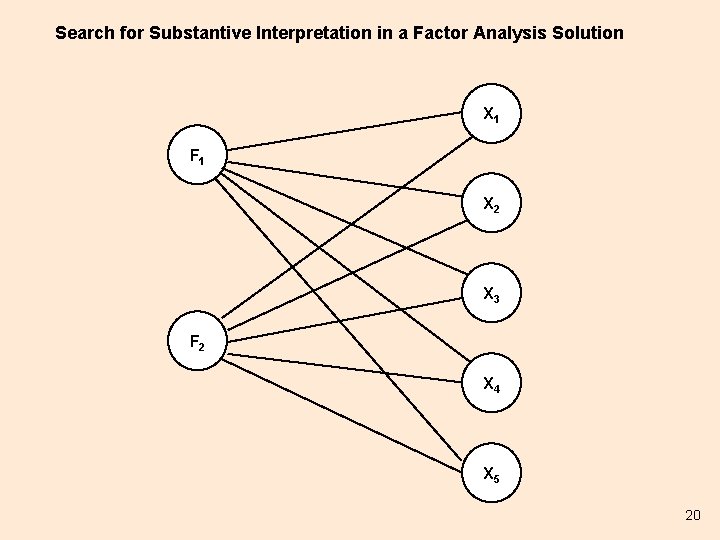
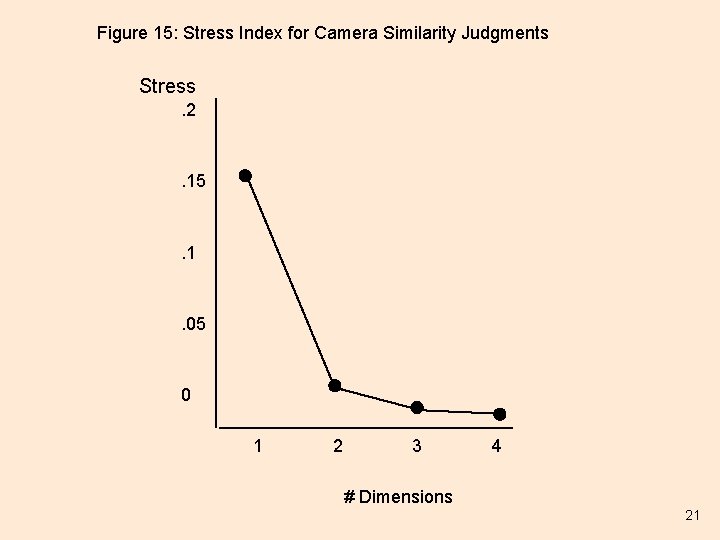
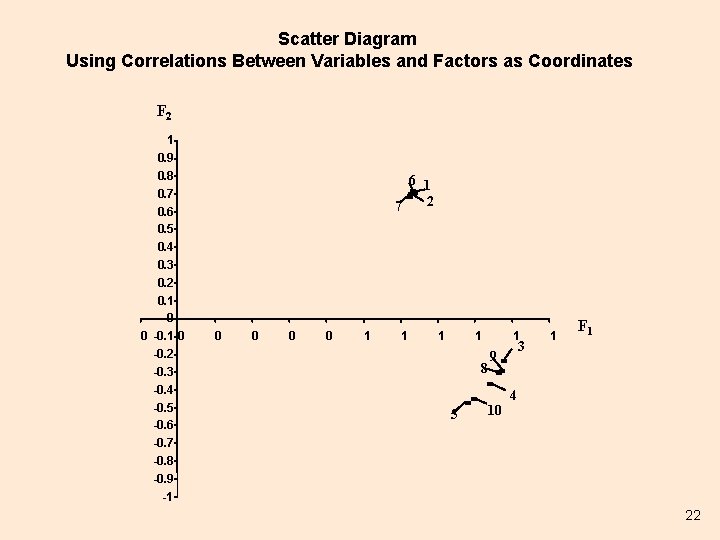
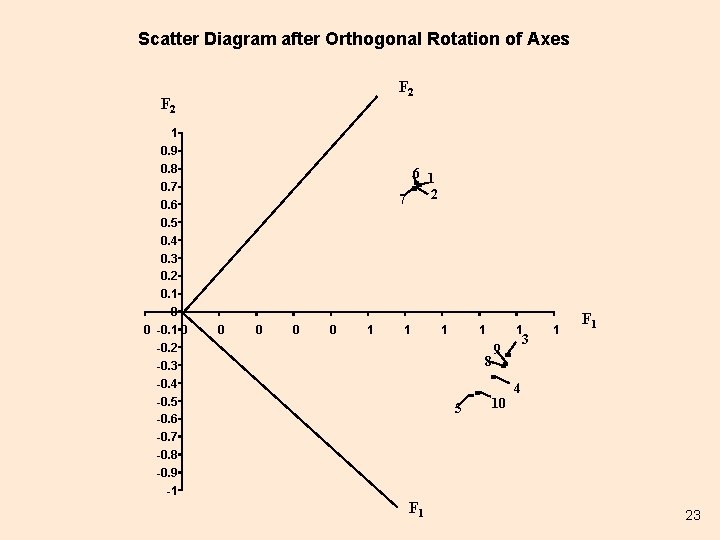
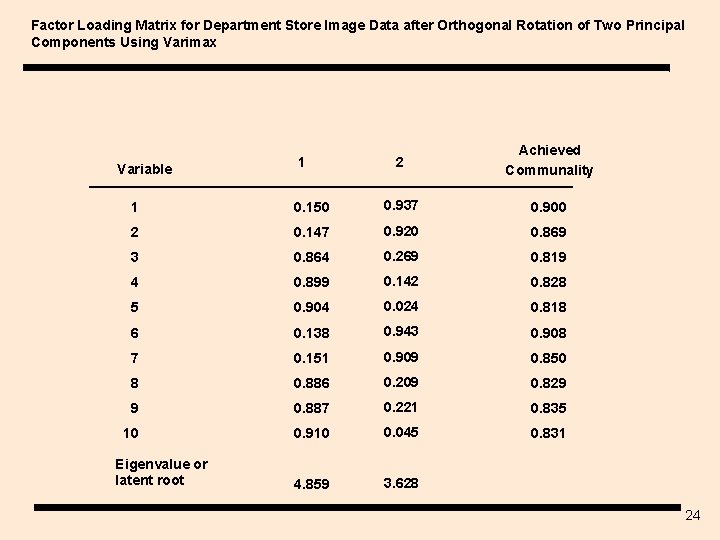
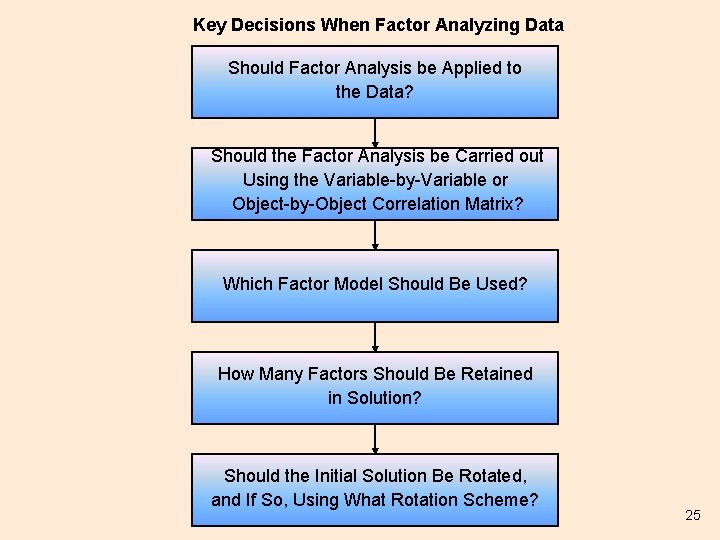
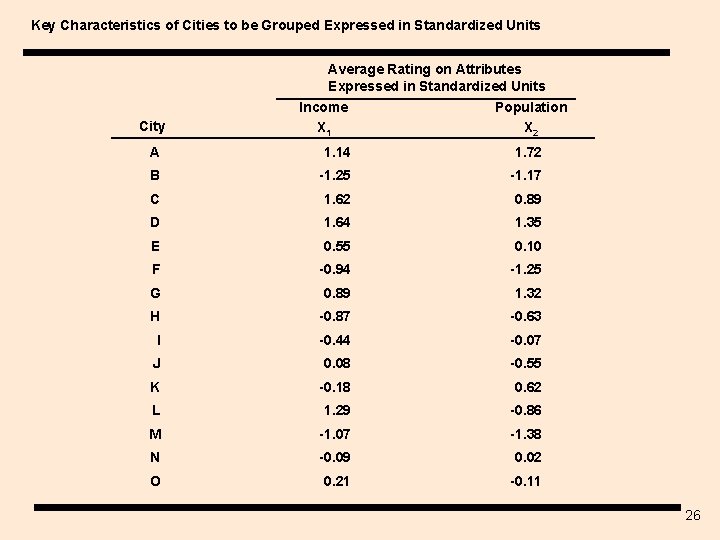
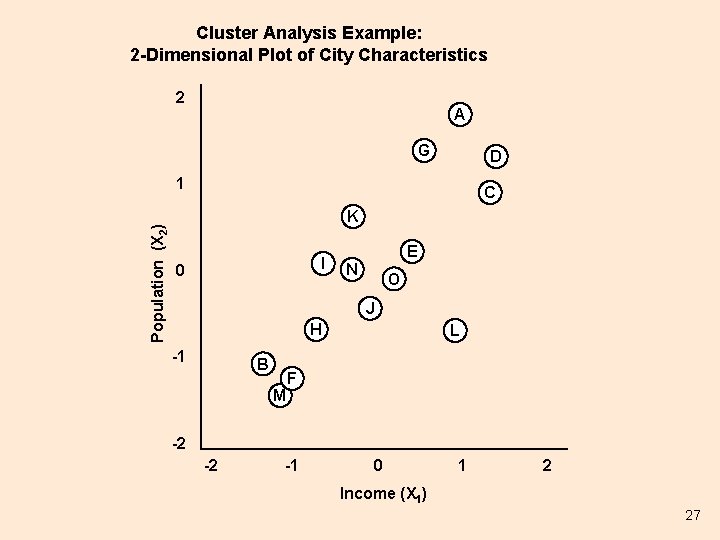
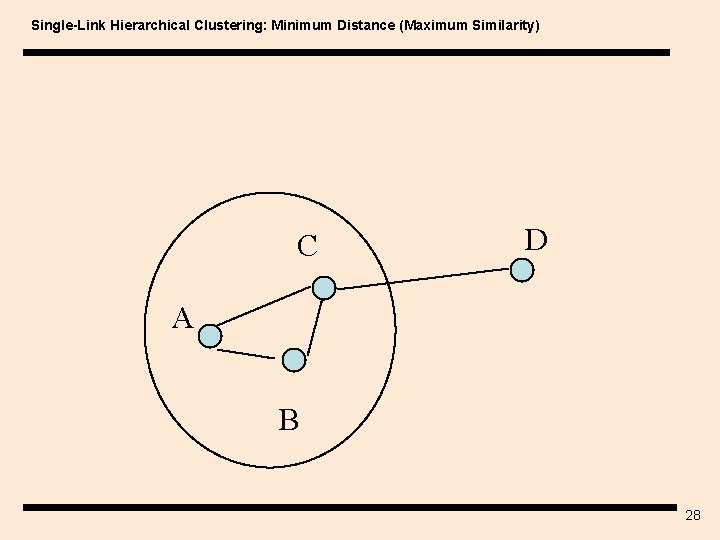
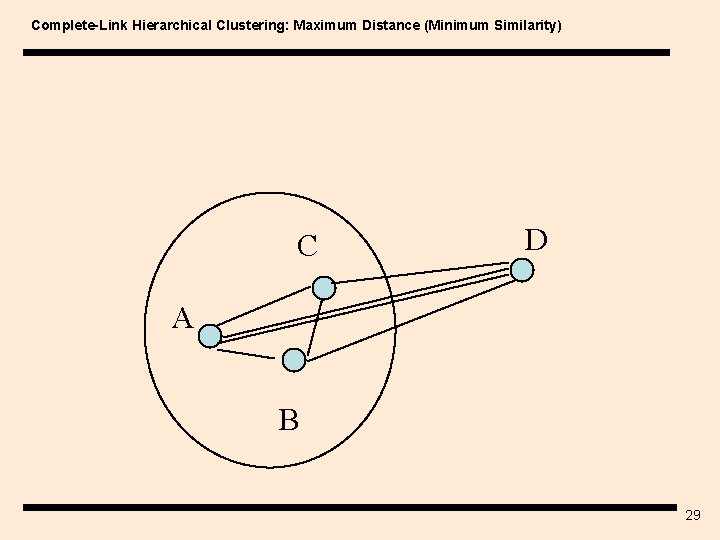
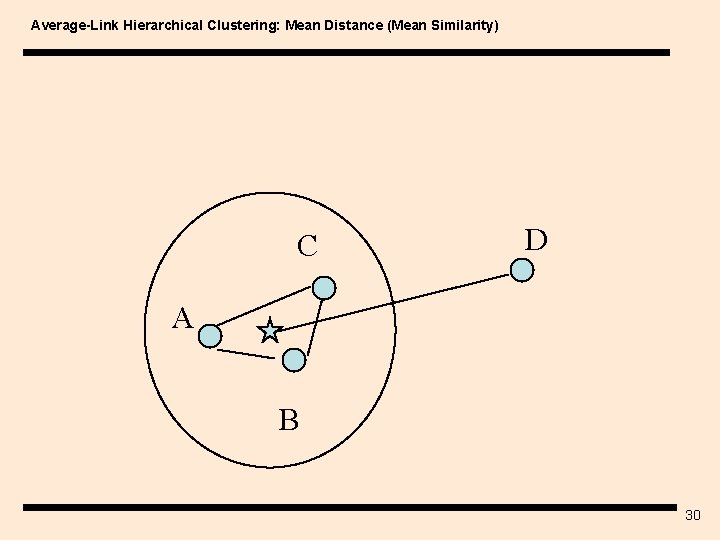
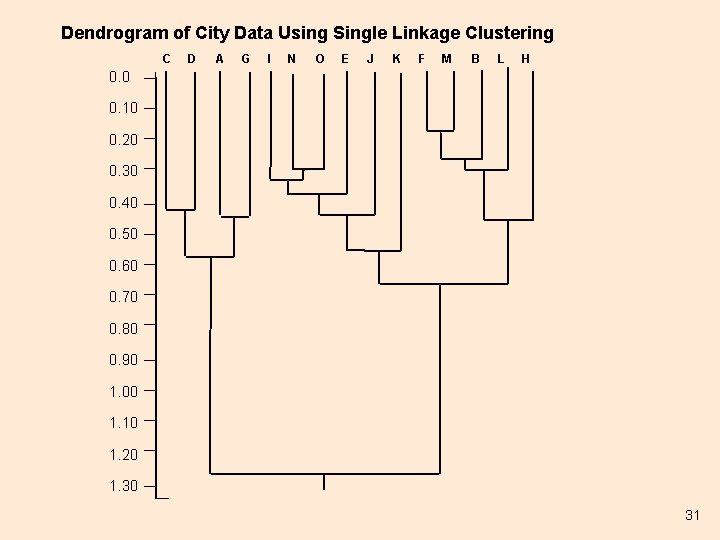
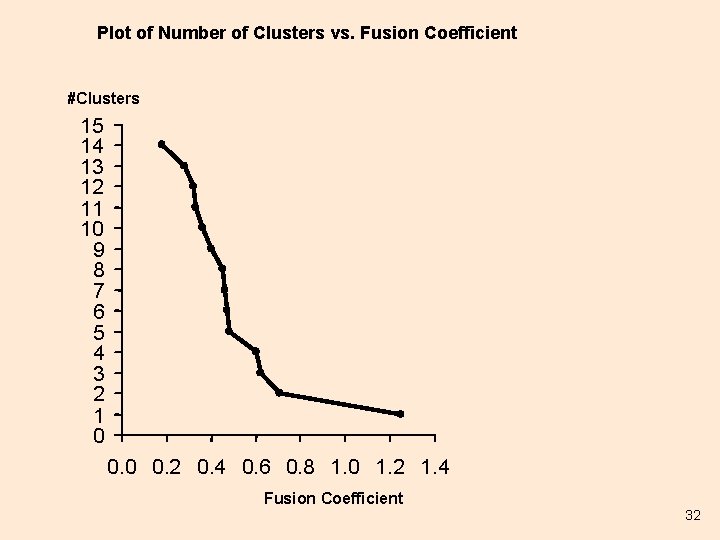
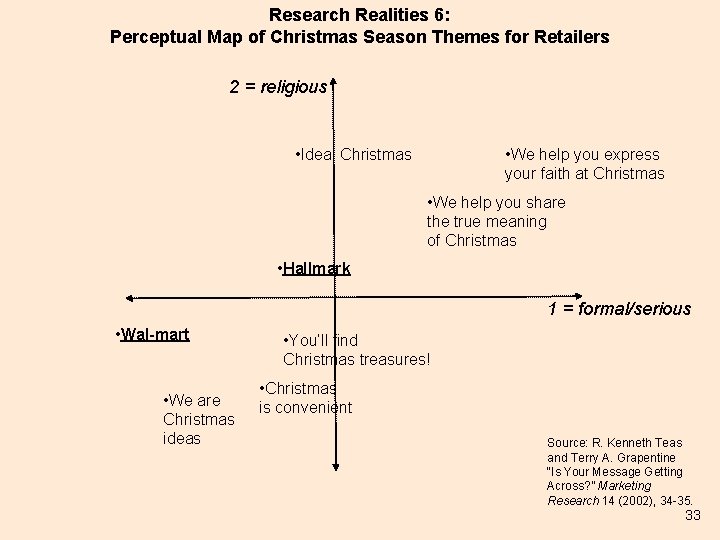
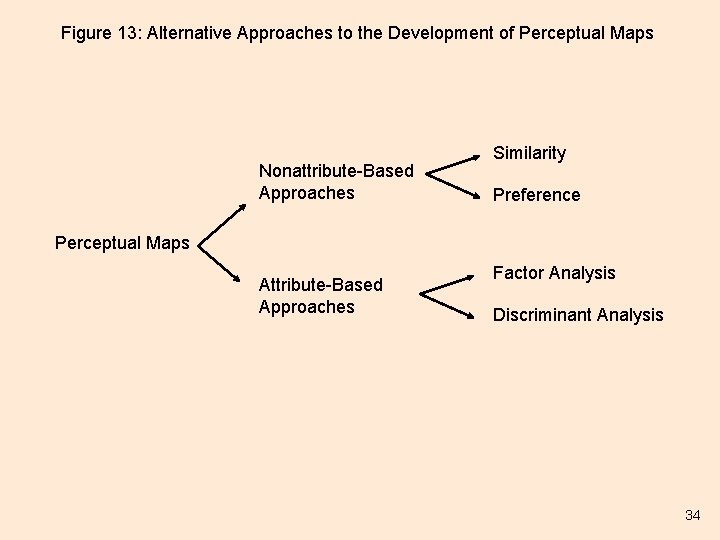
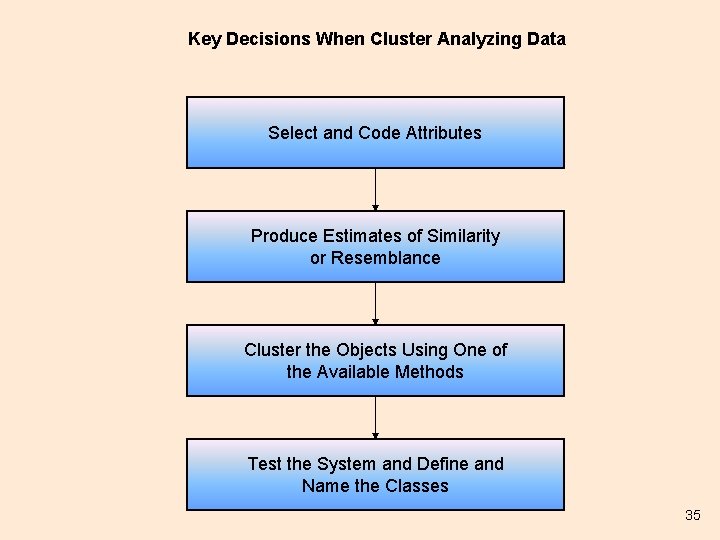
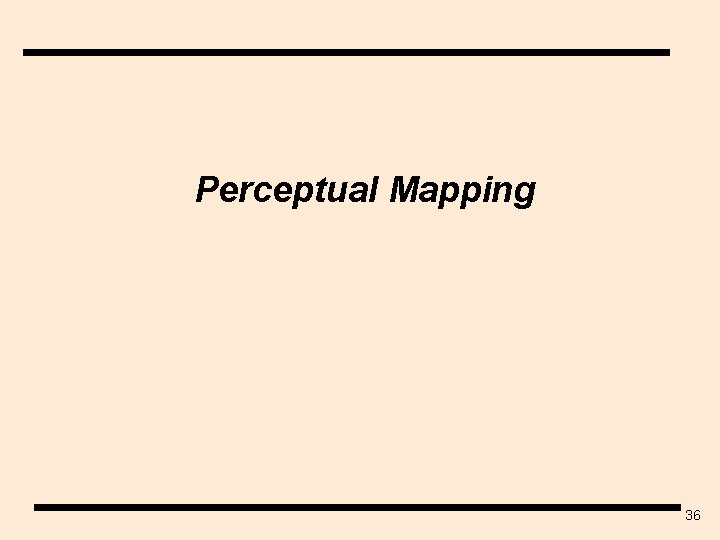
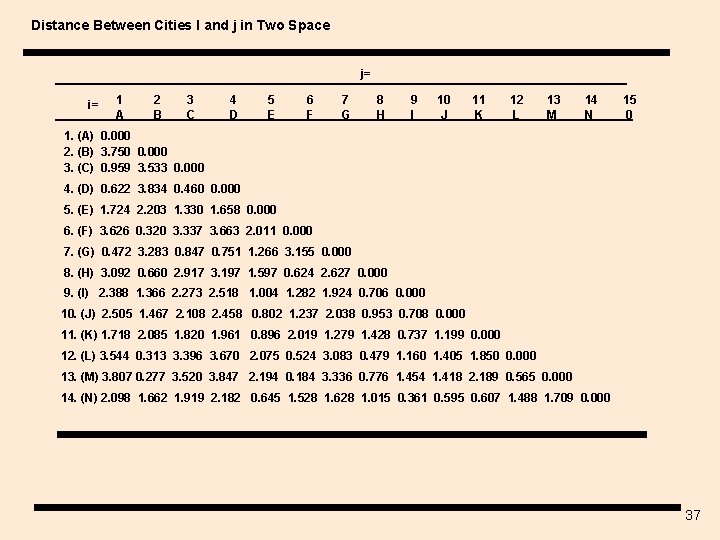
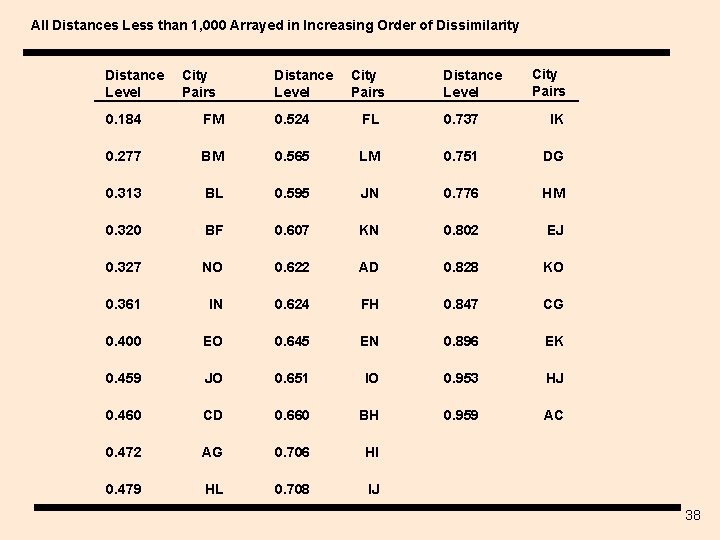
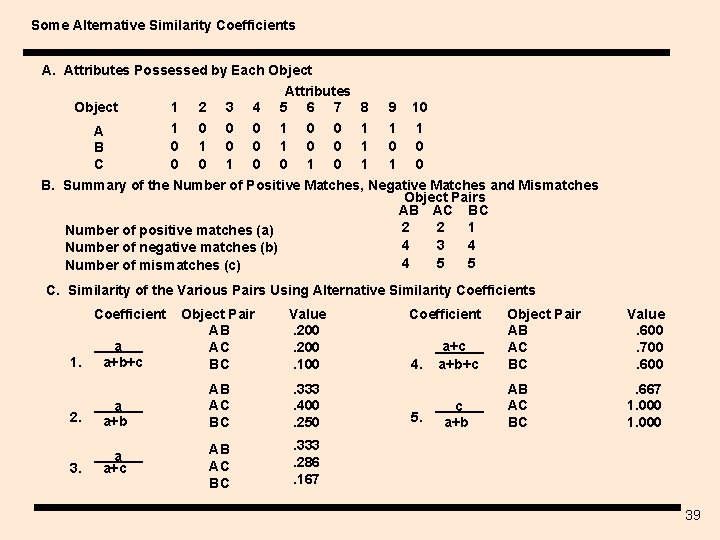
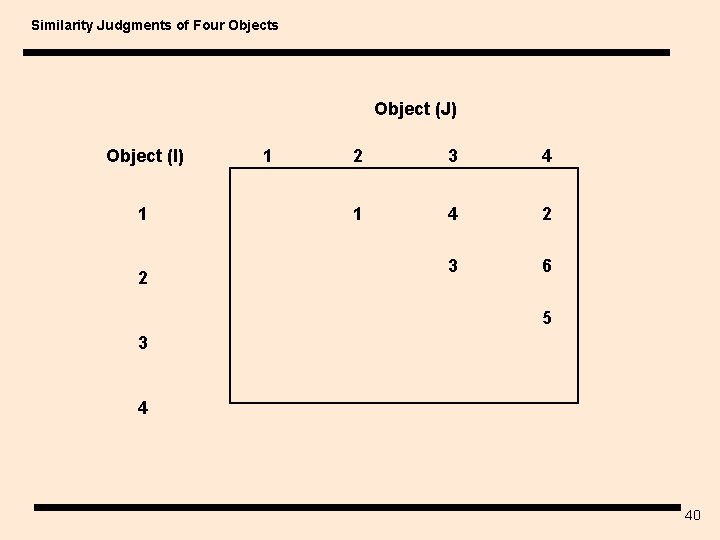
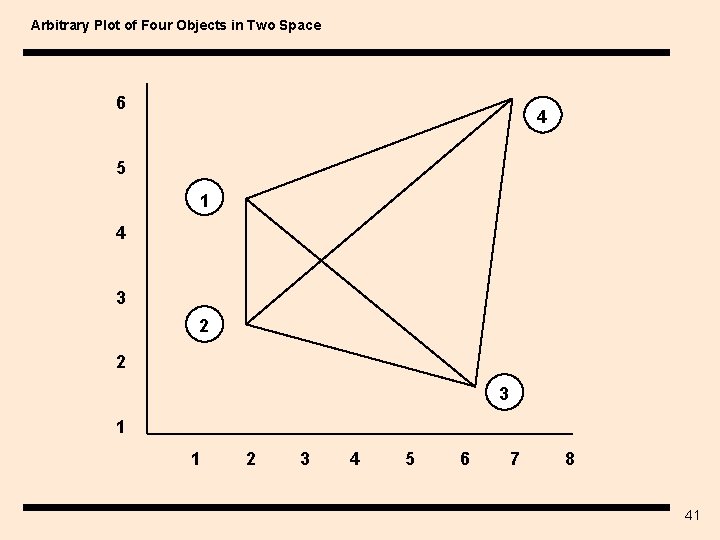
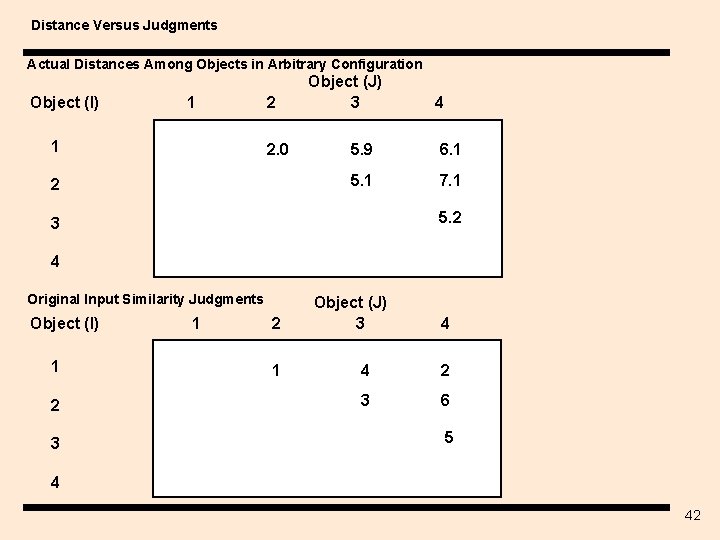
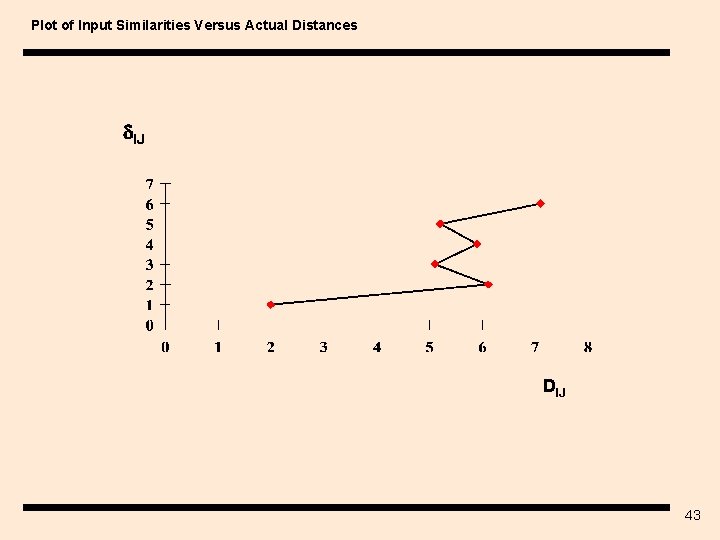
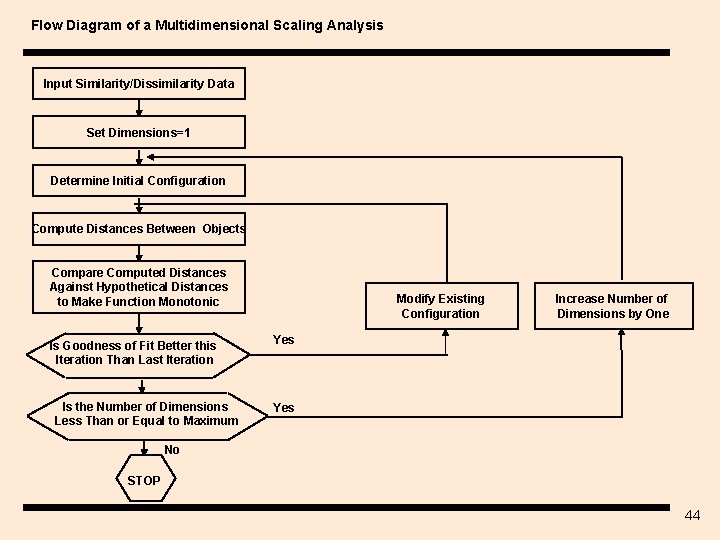
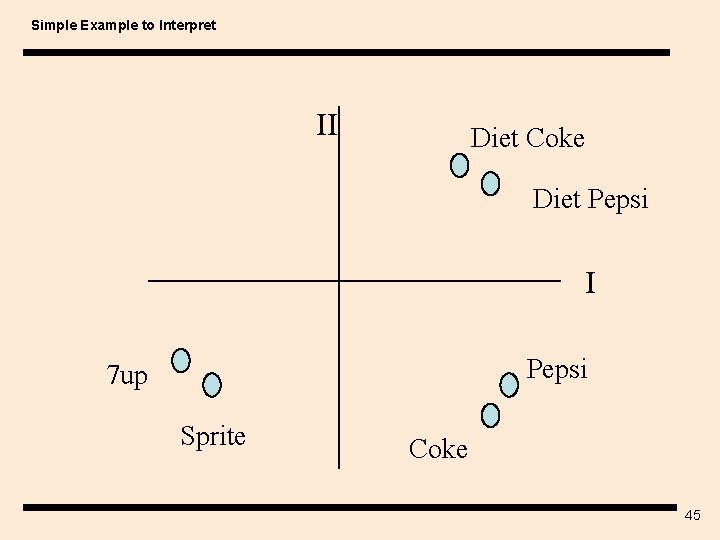
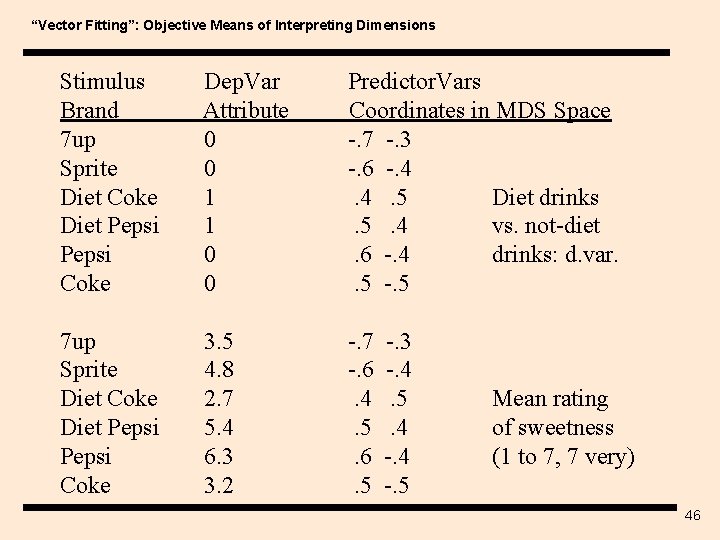
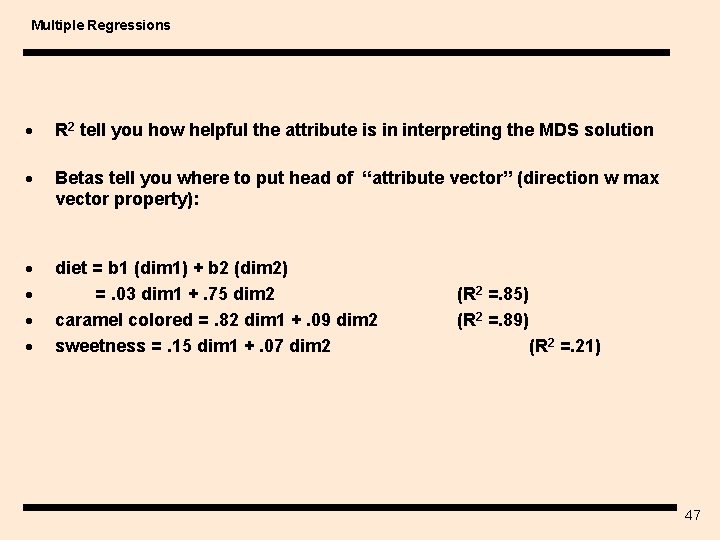
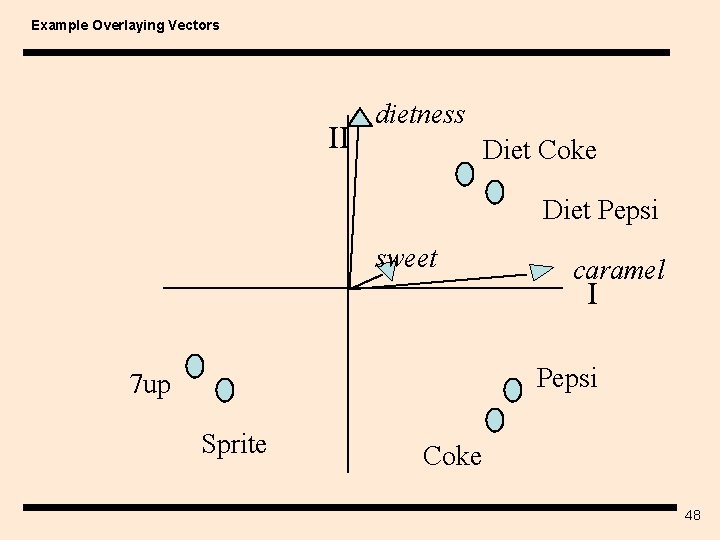
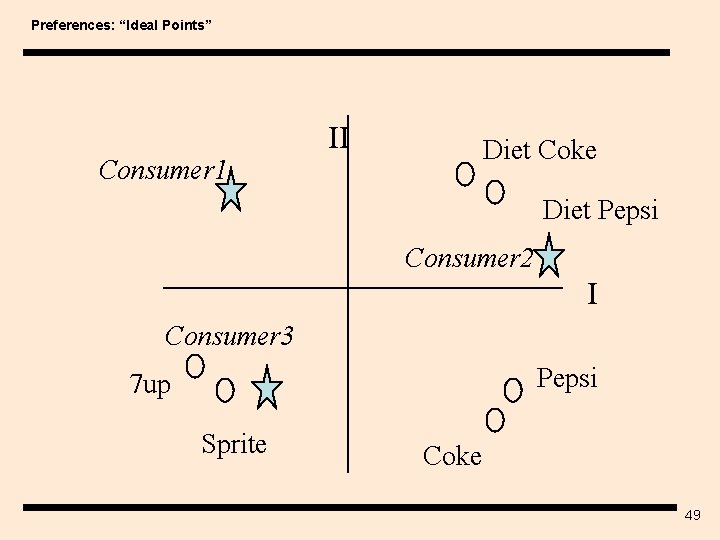
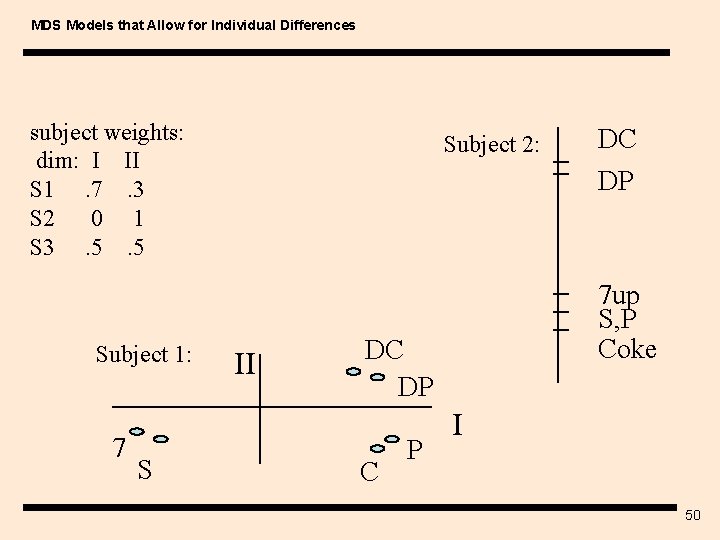
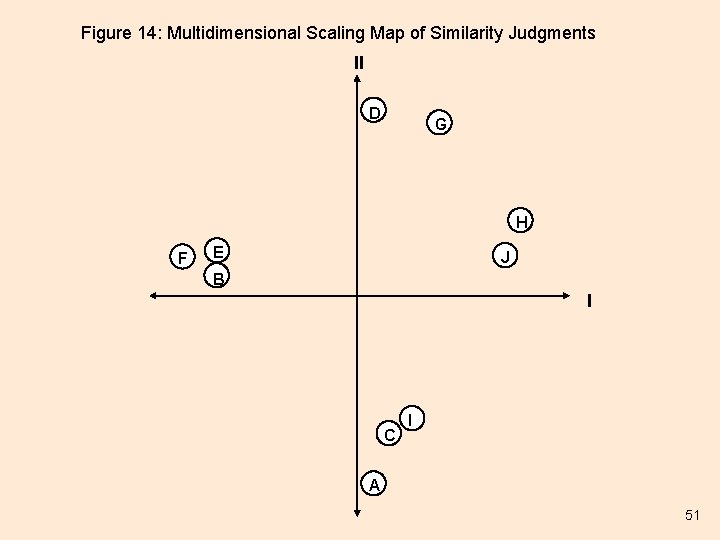
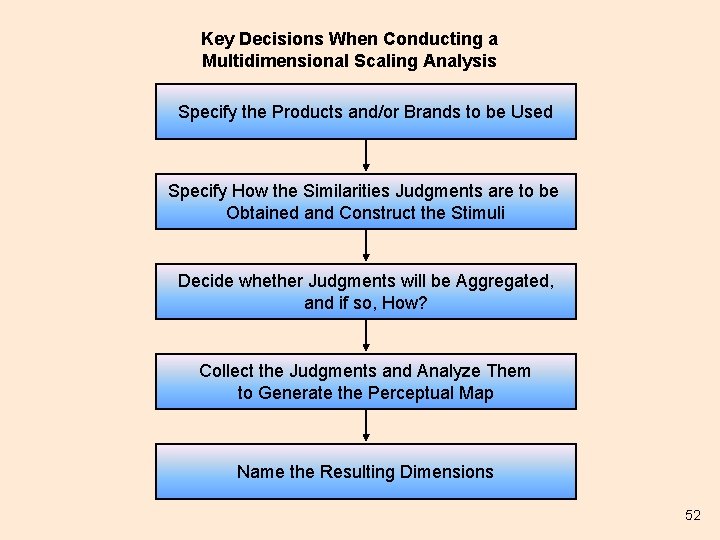
- Slides: 52
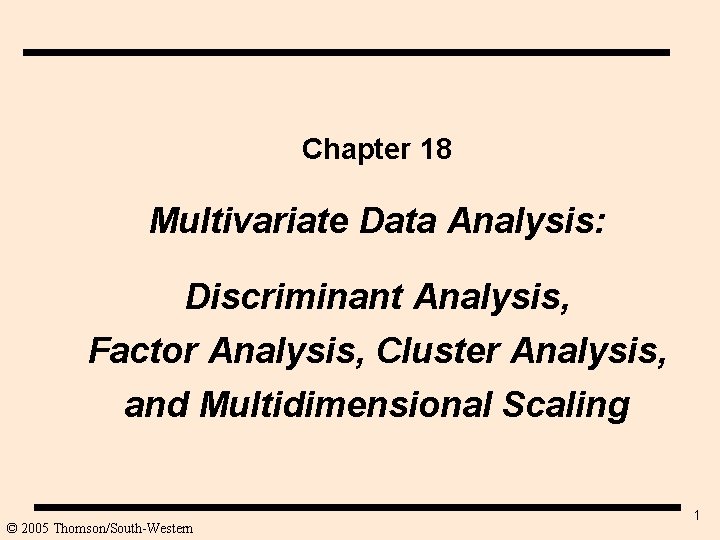
Chapter 18 Multivariate Data Analysis: Discriminant Analysis, Factor Analysis, Cluster Analysis, and Multidimensional Scaling © 2005 Thomson/South-Western 1
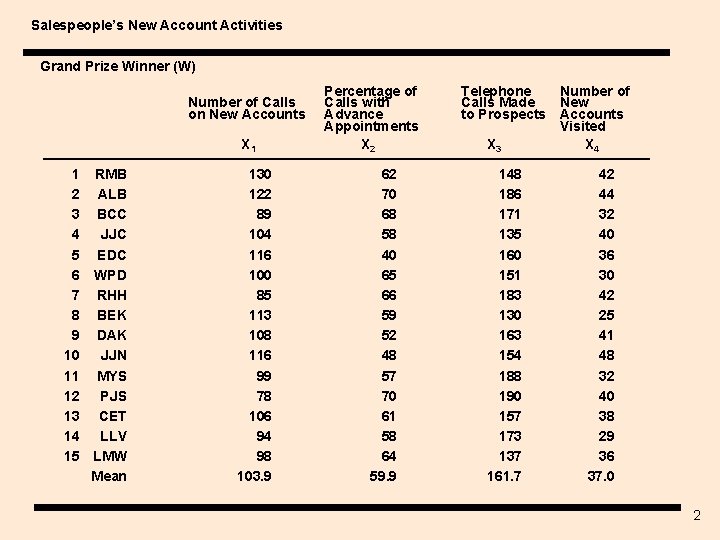
Salespeople’s New Account Activities Grand Prize Winner (W) Number of Calls on New Accounts X 1 1 RMB 2 ALB 3 BCC 4 JJC 5 EDC 6 WPD 7 RHH 8 BEK 9 DAK 10 JJN 11 MYS 12 PJS 13 CET 14 LLV 15 LMW Mean 130 122 89 104 116 100 85 113 108 116 99 78 106 94 98 103. 9 Percentage of Calls with Advance Appointments X 2 62 70 68 58 40 65 66 59 52 48 57 70 61 58 64 59. 9 Telephone Number of Calls Made New to Prospects Accounts Visited X 3 X 4 148 186 171 135 160 151 183 130 163 154 188 190 157 173 137 161. 7 42 44 32 40 36 30 42 25 41 48 32 40 38 29 36 37. 0 2
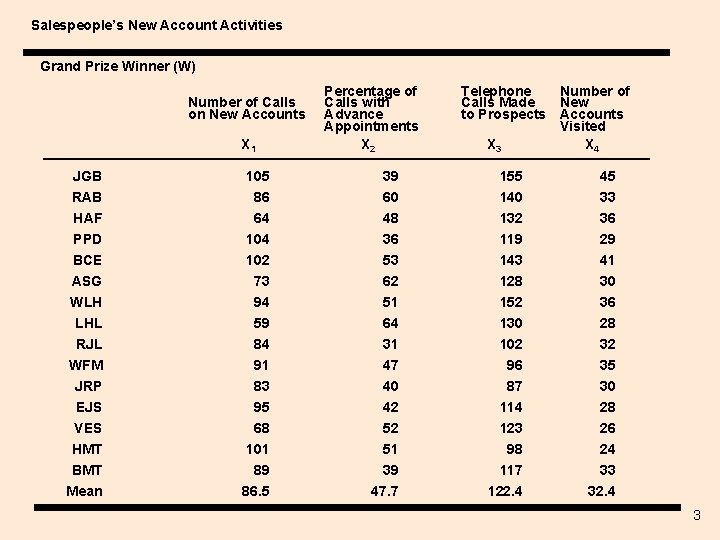
Salespeople’s New Account Activities Grand Prize Winner (W) Number of Calls on New Accounts X 1 Percentage of Calls with Advance Appointments X 2 Telephone Number of Calls Made New to Prospects Accounts Visited X 3 X 4 JGB 105 39 155 45 RAB HAF PPD 86 64 104 60 48 36 140 132 119 33 36 29 BCE 102 53 143 41 ASG 73 62 128 30 WLH LHL RJL WFM JRP EJS VES HMT BMT Mean 94 59 84 91 83 95 68 101 89 86. 5 51 64 31 47 40 42 52 51 39 47. 7 152 130 102 96 87 114 123 98 117 122. 4 36 28 32 35 30 28 26 24 33 32. 4 3
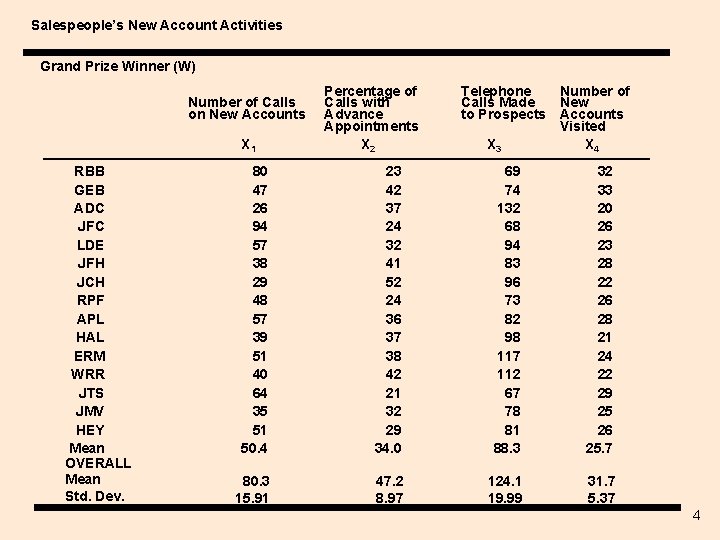
Salespeople’s New Account Activities Grand Prize Winner (W) Number of Calls on New Accounts X 1 RBB GEB ADC JFC LDE JFH JCH RPF APL HAL ERM WRR JTS JMV HEY Mean OVERALL Mean Std. Dev. Percentage of Calls with Advance Appointments X 2 Telephone Number of Calls Made New to Prospects Accounts Visited X 3 X 4 80 47 26 94 57 38 29 48 57 39 51 40 64 35 51 50. 4 23 42 37 24 32 41 52 24 36 37 38 42 21 32 29 34. 0 69 74 132 68 94 83 96 73 82 98 117 112 67 78 81 88. 3 32 33 20 26 23 28 22 26 28 21 24 22 29 25 26 25. 7 80. 3 15. 91 47. 2 8. 97 124. 1 19. 99 31. 7 5. 37 4
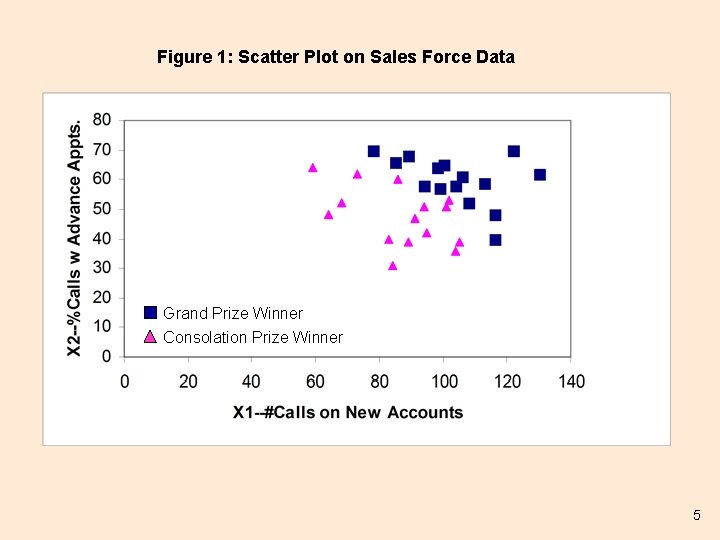
Figure 1: Scatter Plot on Sales Force Data Grand Prize Winner Consolation Prize Winner 5
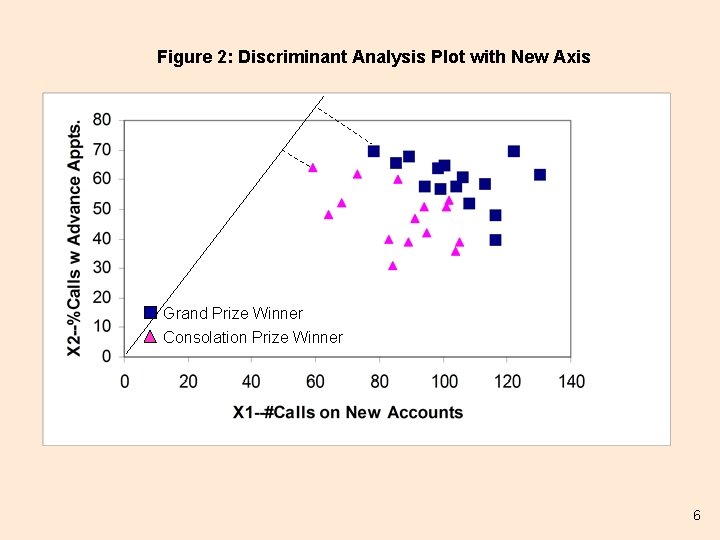
Figure 2: Discriminant Analysis Plot with New Axis Grand Prize Winner Consolation Prize Winner 6
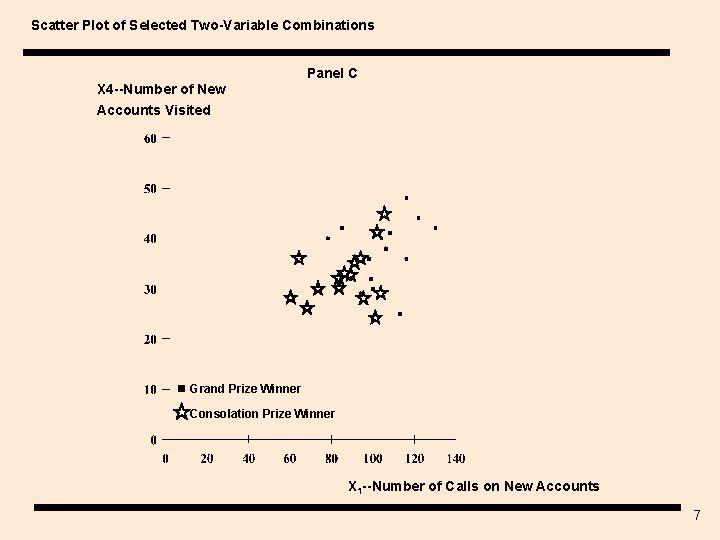
Scatter Plot of Selected Two-Variable Combinations Panel C X 4 --Number of New Accounts Visited Grand Prize Winner Consolation Prize Winner X 1 --Number of Calls on New Accounts 7
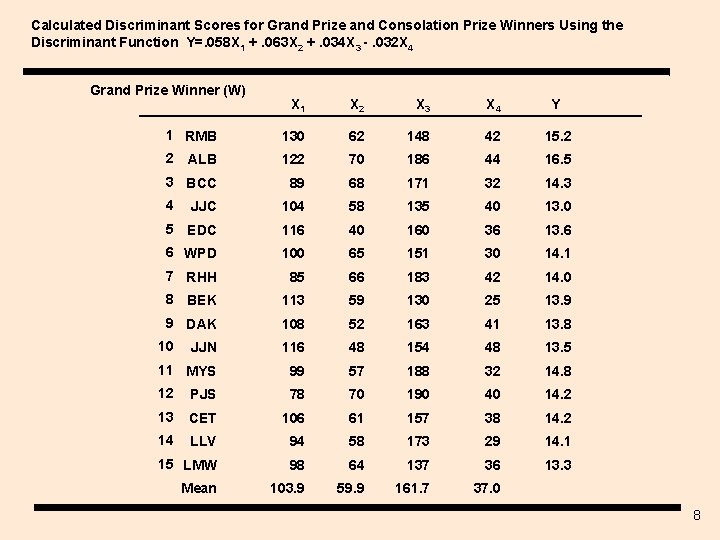
Calculated Discriminant Scores for Grand Prize and Consolation Prize Winners Using the Discriminant Function Y=. 058 X 1 +. 063 X 2 +. 034 X 3 -. 032 X 4 Grand Prize Winner (W) X 1 X 2 X 3 X 4 Y 1 RMB 130 62 148 42 15. 2 2 ALB 122 70 186 44 16. 5 3 BCC 89 68 171 32 14. 3 JJC 104 58 135 40 13. 0 5 EDC 116 40 160 36 13. 6 6 WPD 100 65 151 30 14. 1 7 RHH 85 66 183 42 14. 0 8 BEK 113 59 130 25 13. 9 9 DAK 108 52 163 41 13. 8 JJN 116 48 154 48 13. 5 11 MYS 99 57 188 32 14. 8 12 PJS 78 70 190 40 14. 2 13 CET 106 61 157 38 14. 2 14 LLV 94 58 173 29 14. 1 15 LMW 98 64 137 36 13. 3 Mean 103. 9 59. 9 161. 7 37. 0 4 10 8
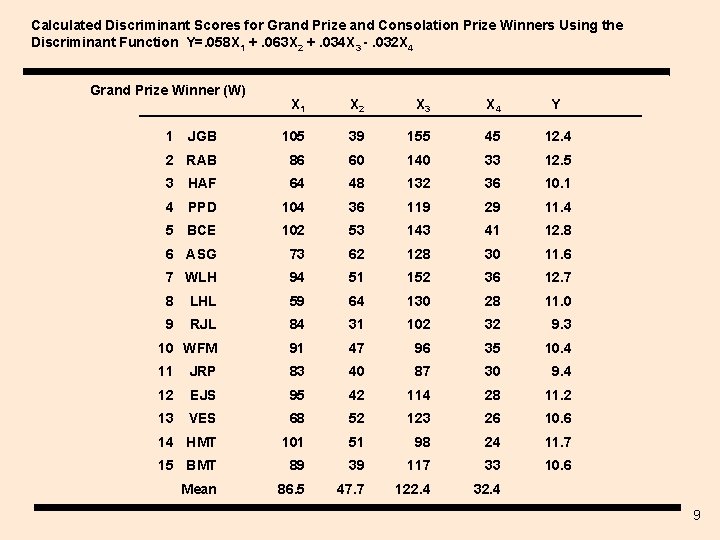
Calculated Discriminant Scores for Grand Prize and Consolation Prize Winners Using the Discriminant Function Y=. 058 X 1 +. 063 X 2 +. 034 X 3 -. 032 X 4 Grand Prize Winner (W) X 1 X 2 X 3 X 4 Y JGB 105 39 155 45 12. 4 2 RAB 86 60 140 33 12. 5 3 HAF 64 48 132 36 10. 1 4 PPD 104 36 119 29 11. 4 5 BCE 102 53 143 41 12. 8 6 ASG 73 62 128 30 11. 6 7 WLH 94 51 152 36 12. 7 8 LHL 59 64 130 28 11. 0 9 RJL 84 31 102 32 9. 3 10 WFM 91 47 96 35 10. 4 11 JRP 83 40 87 30 9. 4 12 EJS 95 42 114 28 11. 2 13 VES 68 52 123 26 10. 6 14 HMT 101 51 98 24 11. 7 15 BMT 89 39 117 33 10. 6 Mean 86. 5 47. 7 122. 4 32. 4 1 9
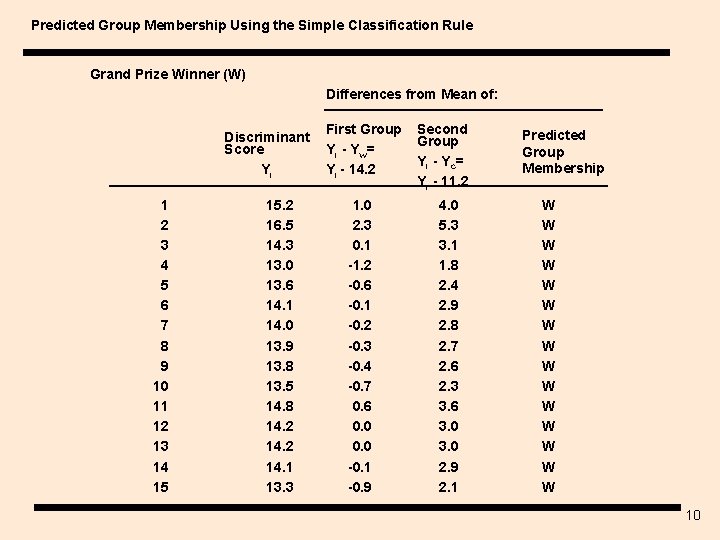
Predicted Group Membership Using the Simple Classification Rule Grand Prize Winner (W) Differences from Mean of: Discriminant Score Yi 1 2 3 4 5 6 7 8 9 10 11 12 13 14 15 15. 2 16. 5 14. 3 13. 0 13. 6 14. 1 14. 0 13. 9 13. 8 13. 5 14. 8 14. 2 14. 1 13. 3 First Group Yi - Yw= Yi - 14. 2 1. 0 2. 3 0. 1 -1. 2 -0. 6 -0. 1 -0. 2 -0. 3 -0. 4 -0. 7 0. 6 0. 0 -0. 1 -0. 9 Second Group Yi - Yc= Yi - 11. 2 4. 0 5. 3 3. 1 1. 8 2. 4 2. 9 2. 8 2. 7 2. 6 2. 3 3. 6 3. 0 2. 9 2. 1 Predicted Group Membership W W W W 10
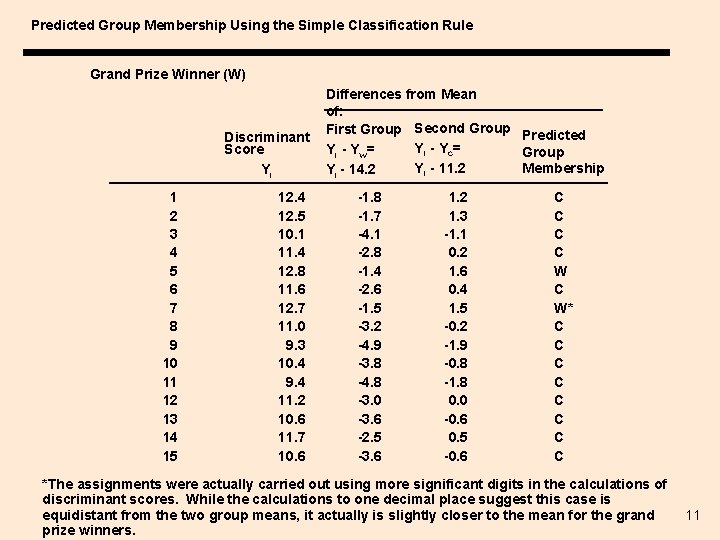
Predicted Group Membership Using the Simple Classification Rule Grand Prize Winner (W) Discriminant Score Yi 1 2 3 4 5 6 7 8 9 10 11 12 13 14 15 12. 4 12. 5 10. 1 11. 4 12. 8 11. 6 12. 7 11. 0 9. 3 10. 4 9. 4 11. 2 10. 6 11. 7 10. 6 Differences from Mean of: First Group Second Group Predicted Yi - Yc= Yi - Yw= Group Yi - 11. 2 Membership Yi - 14. 2 -1. 8 -1. 7 -4. 1 -2. 8 -1. 4 -2. 6 -1. 5 -3. 2 -4. 9 -3. 8 -4. 8 -3. 0 -3. 6 -2. 5 -3. 6 1. 2 1. 3 -1. 1 0. 2 1. 6 0. 4 1. 5 -0. 2 -1. 9 -0. 8 -1. 8 0. 0 -0. 6 0. 5 -0. 6 C C W C W* C C C C *The assignments were actually carried out using more significant digits in the calculations of discriminant scores. While the calculations to one decimal place suggest this case is equidistant from the two group means, it actually is slightly closer to the mean for the grand prize winners. 11
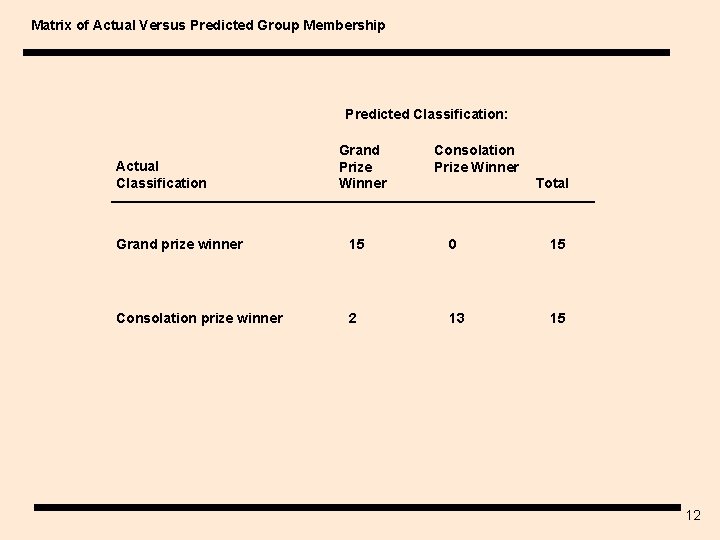
Matrix of Actual Versus Predicted Group Membership Predicted Classification: Actual Classification Grand Prize Winner Consolation Prize Winner Total Grand prize winner 15 0 15 Consolation prize winner 2 13 15 12
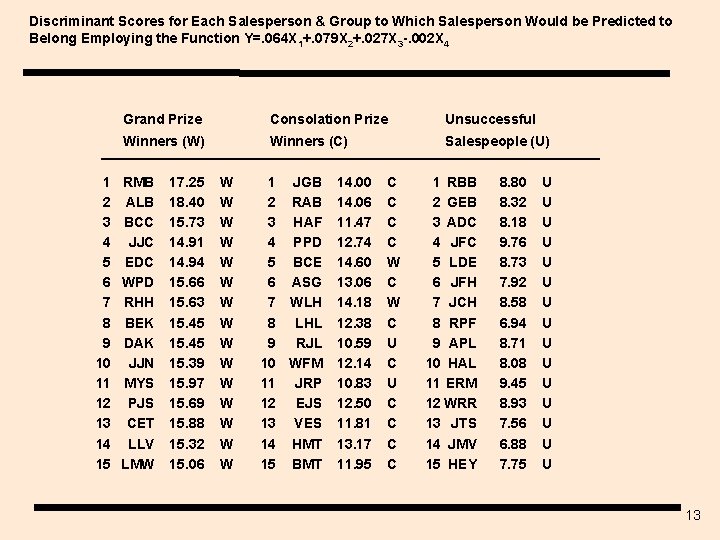
Discriminant Scores for Each Salesperson & Group to Which Salesperson Would be Predicted to Belong Employing the Function Y=. 064 X 1+. 079 X 2+. 027 X 3 -. 002 X 4 1 2 3 4 5 6 7 8 9 10 11 12 13 14 15 Grand Prize Consolation Prize Unsuccessful Winners (W) Winners (C) Salespeople (U) RMB ALB BCC JJC EDC WPD RHH BEK DAK JJN MYS PJS CET LLV LMW 17. 25 18. 40 15. 73 14. 91 14. 94 15. 66 15. 63 15. 45 15. 39 15. 97 15. 69 15. 88 15. 32 15. 06 W W W W 1 JGB 2 RAB 3 HAF 4 PPD 5 BCE 6 ASG 7 WLH 8 LHL 9 RJL 10 WFM 11 JRP 12 EJS 13 VES 14 HMT 15 BMT 14. 00 14. 06 11. 47 12. 74 14. 60 13. 06 14. 18 12. 38 10. 59 12. 14 10. 83 12. 50 11. 81 13. 17 11. 95 C C W C U C C C C 1 RBB 2 GEB 3 ADC 4 JFC 5 LDE 6 JFH 7 JCH 8 RPF 9 APL 10 HAL 11 ERM 12 WRR 13 JTS 14 JMV 15 HEY 8. 80 8. 32 8. 18 9. 76 8. 73 7. 92 8. 58 6. 94 8. 71 8. 08 9. 45 8. 93 7. 56 6. 88 7. 75 U U U U 13
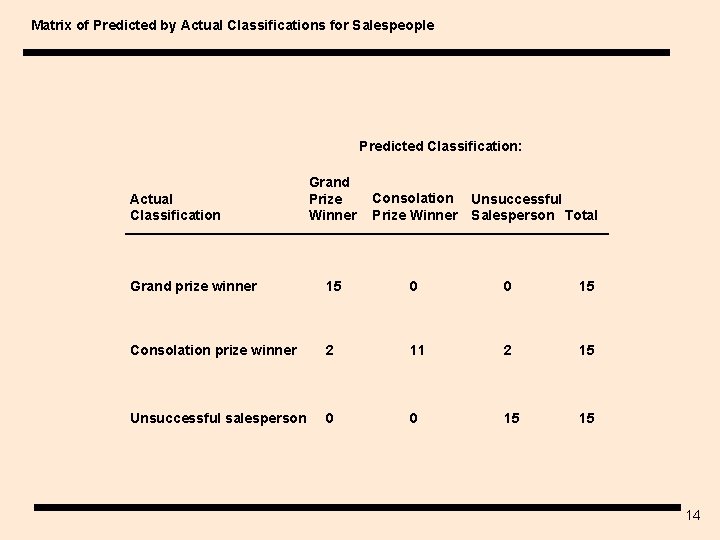
Matrix of Predicted by Actual Classifications for Salespeople Predicted Classification: Grand Prize Winner Consolation Prize Winner Grand prize winner 15 0 0 15 Consolation prize winner 2 11 2 15 Unsuccessful salesperson 0 0 15 15 Actual Classification Unsuccessful Salesperson Total 14
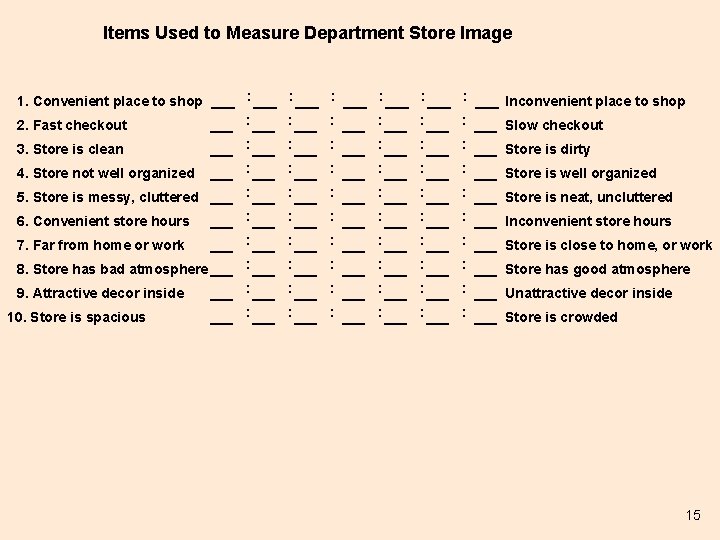
Items Used to Measure Department Store Image 1. Convenient place to shop : : : Inconvenient place to shop 2. Fast checkout : : : Slow checkout 3. Store is clean : : : Store is dirty 4. Store not well organized : : : Store is well organized 5. Store is messy, cluttered : : : Store is neat, uncluttered 6. Convenient store hours : : : Inconvenient store hours 7. Far from home or work : : : Store is close to home, or work 8. Store has bad atmosphere : : : Store has good atmosphere 9. Attractive decor inside : : : Unattractive decor inside : : : Store is crowded 10. Store is spacious 15
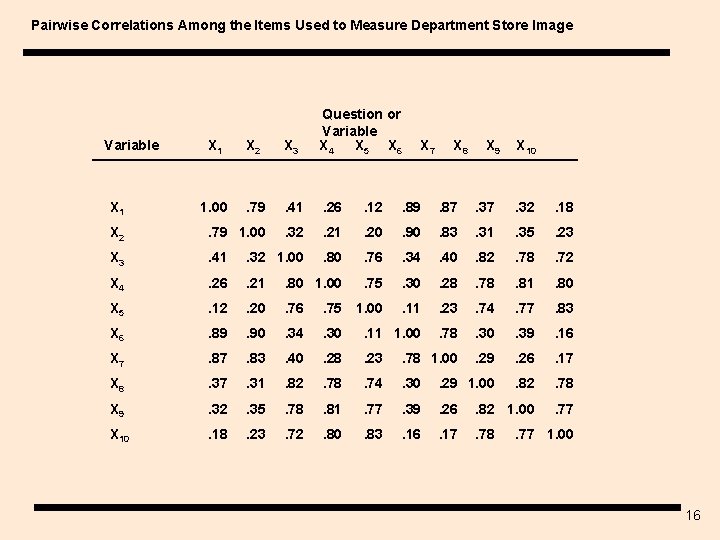
Pairwise Correlations Among the Items Used to Measure Department Store Image X 1 X 2 X 3 Question or Variable X 4 X 5 X 6 1. 00 . 79 . 41 . 26 . 12 . 89 . 87 . 32 . 18 X 2 . 79 1. 00 . 32 . 21 . 20 . 90 . 83 . 31 . 35 . 23 X 3 . 41 . 32 1. 00 . 80 . 76 . 34 . 40 . 82 . 78 . 72 X 4 . 26 . 21 . 80 1. 00 . 75 . 30 . 28 . 78 . 81 . 80 X 5 . 12 . 20 . 76 . 75 1. 00 . 11 . 23 . 74 . 77 . 83 X 6 . 89 . 90 . 34 . 30 . 11 1. 00 . 78 . 30 . 39 . 16 X 7 . 83 . 40 . 28 . 23 . 78 1. 00 . 29 . 26 . 17 X 8 . 37 . 31 . 82 . 78 . 74 . 30 . 29 1. 00 . 82 . 78 X 9 . 32 . 35 . 78 . 81 . 77 . 39 . 26 . 82 1. 00 . 77 X 10 . 18 . 23 . 72 . 80 . 83 . 16 . 17 . 78 Variable X 1 X 7 X 8 X 9 X 10 . 77 1. 00 16
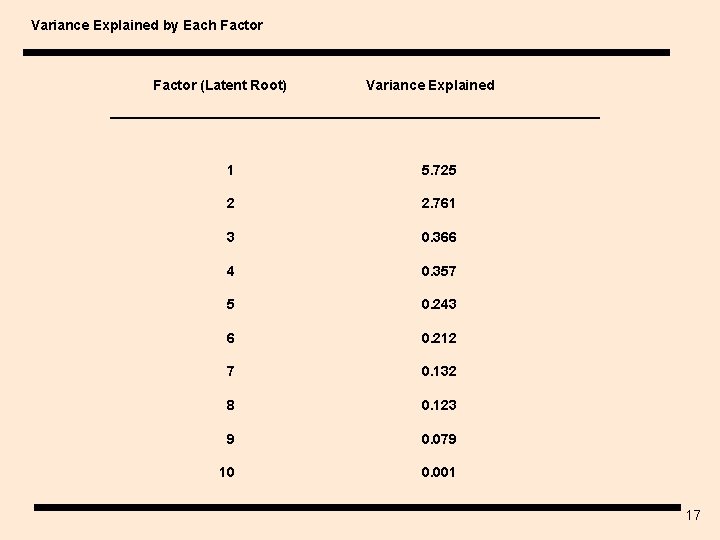
Variance Explained by Each Factor (Latent Root) Variance Explained 1 5. 725 2 2. 761 3 0. 366 4 0. 357 5 0. 243 6 0. 212 7 0. 132 8 0. 123 9 0. 079 10 0. 001 17
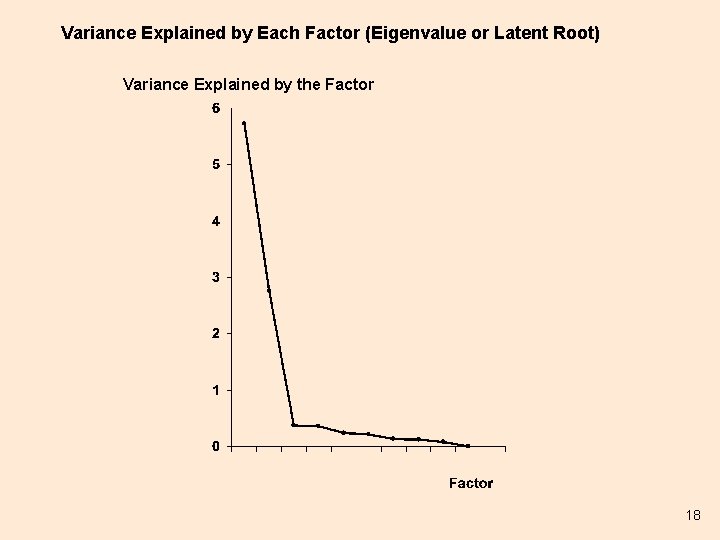
Variance Explained by Each Factor (Eigenvalue or Latent Root) Variance Explained by the Factor 18
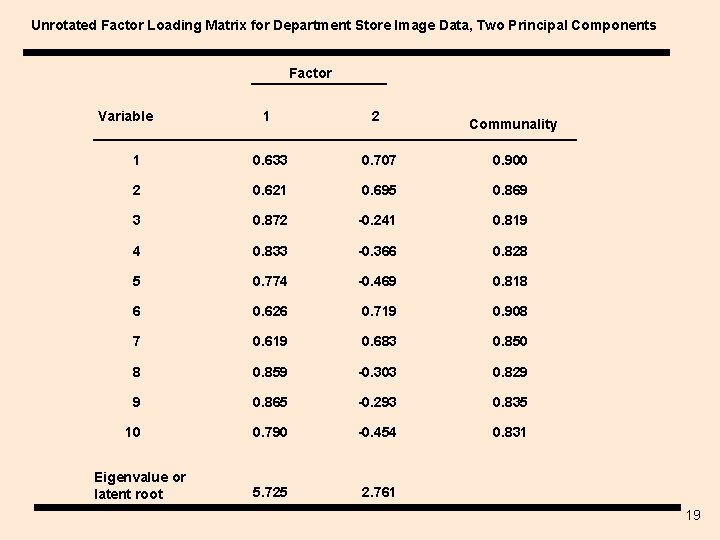
Unrotated Factor Loading Matrix for Department Store Image Data, Two Principal Components Factor Variable 1 2 1 0. 633 0. 707 0. 900 2 0. 621 0. 695 0. 869 3 0. 872 -0. 241 0. 819 4 0. 833 -0. 366 0. 828 5 0. 774 -0. 469 0. 818 6 0. 626 0. 719 0. 908 7 0. 619 0. 683 0. 850 8 0. 859 -0. 303 0. 829 9 0. 865 -0. 293 0. 835 10 0. 790 -0. 454 0. 831 5. 725 2. 761 Eigenvalue or latent root Communality 19
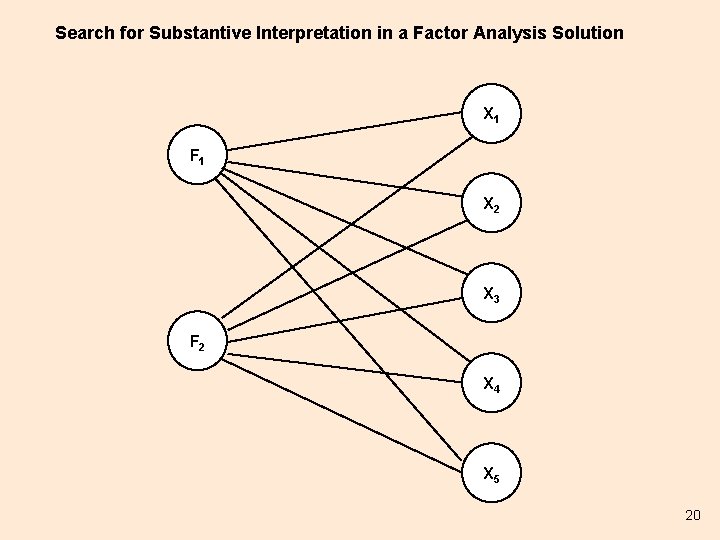
Search for Substantive Interpretation in a Factor Analysis Solution X 1 F 1 X 2 X 3 F 2 X 4 X 5 20
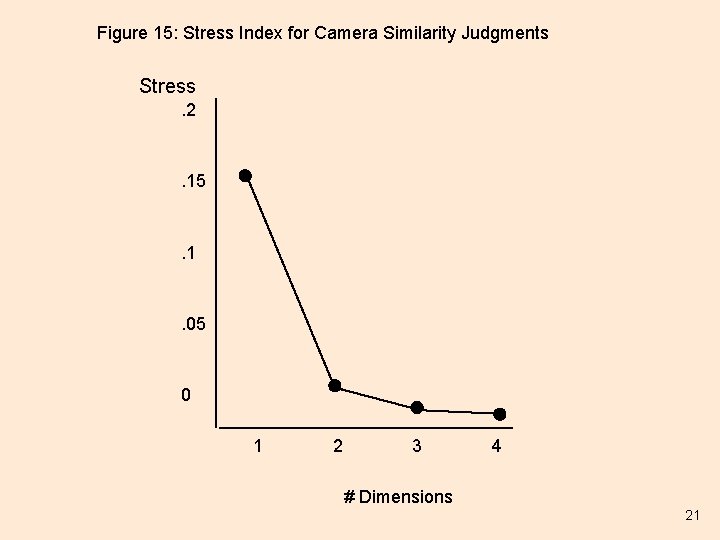
Figure 15: Stress Index for Camera Similarity Judgments Stress. 2 . 15 . 1 . 05 0 1 2 3 4 # Dimensions 21
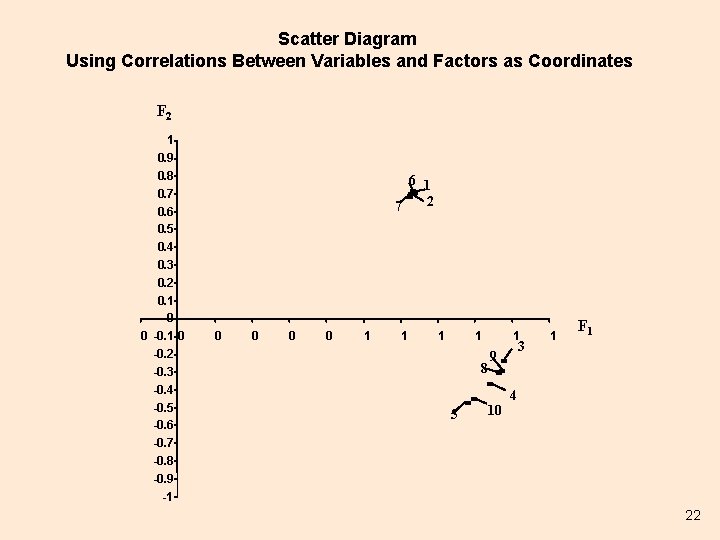
Scatter Diagram Using Correlations Between Variables and Factors as Coordinates F 2 1 0. 9 0. 8 0. 7 0. 6 0. 5 6 1 2 7 0. 4 0. 3 0. 2 0. 1 0 0 -0. 1 0 -0. 2 -0. 3 -0. 4 -0. 5 -0. 6 0 0 1 1 8 5 1 3 9 10 1 F 1 4 -0. 7 -0. 8 -0. 9 -1 22
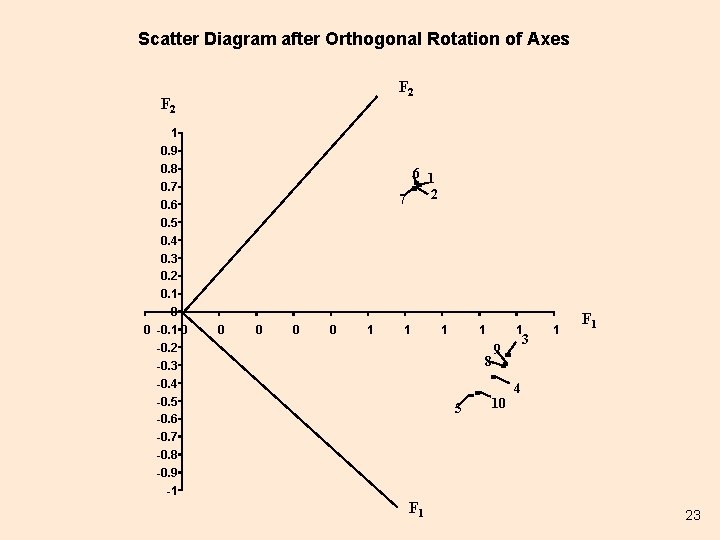
Scatter Diagram after Orthogonal Rotation of Axes F 2 1 0. 9 0. 8 0. 7 0. 6 0. 5 0. 4 0. 3 0. 2 0. 1 0 0 -0. 1 0 -0. 2 -0. 3 -0. 4 -0. 5 -0. 6 -0. 7 -0. 8 -0. 9 -1 6 1 2 7 0 0 1 1 8 5 F 1 1 3 9 10 1 F 1 4 23
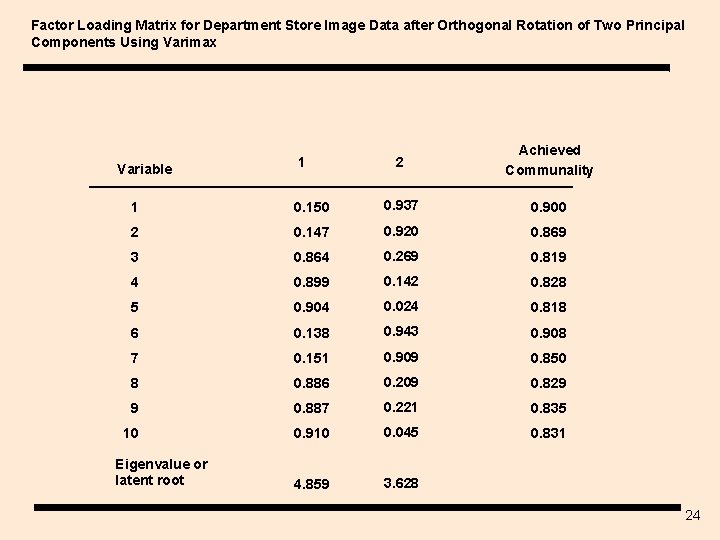
Factor Loading Matrix for Department Store Image Data after Orthogonal Rotation of Two Principal Components Using Varimax Variable 1 2 Achieved Communality 1 0. 150 0. 937 0. 900 2 0. 147 0. 920 0. 869 3 0. 864 0. 269 0. 819 4 0. 899 0. 142 0. 828 5 0. 904 0. 024 0. 818 6 0. 138 0. 943 0. 908 7 0. 151 0. 909 0. 850 8 0. 886 0. 209 0. 829 9 0. 887 0. 221 0. 835 10 0. 910 0. 045 0. 831 4. 859 3. 628 Eigenvalue or latent root 24
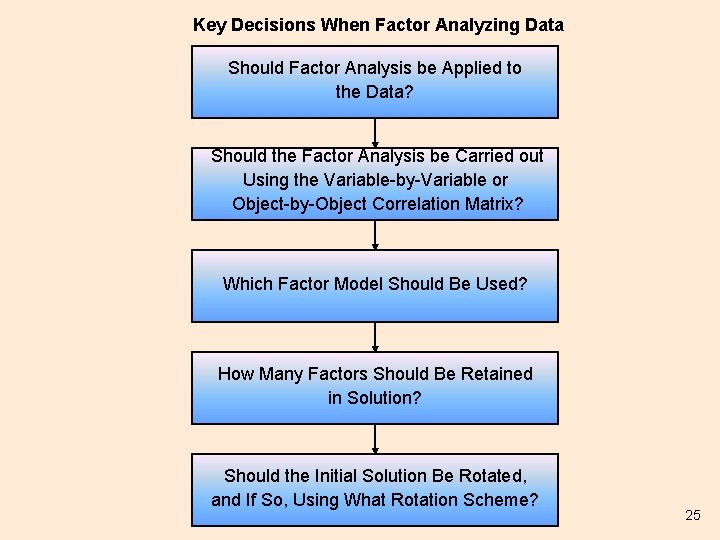
Key Decisions When Factor Analyzing Data Should Factor Analysis be Applied to the Data? Should the Factor Analysis be Carried out Using the Variable-by-Variable or Object-by-Object Correlation Matrix? Which Factor Model Should Be Used? How Many Factors Should Be Retained in Solution? Should the Initial Solution Be Rotated, and If So, Using What Rotation Scheme? 25
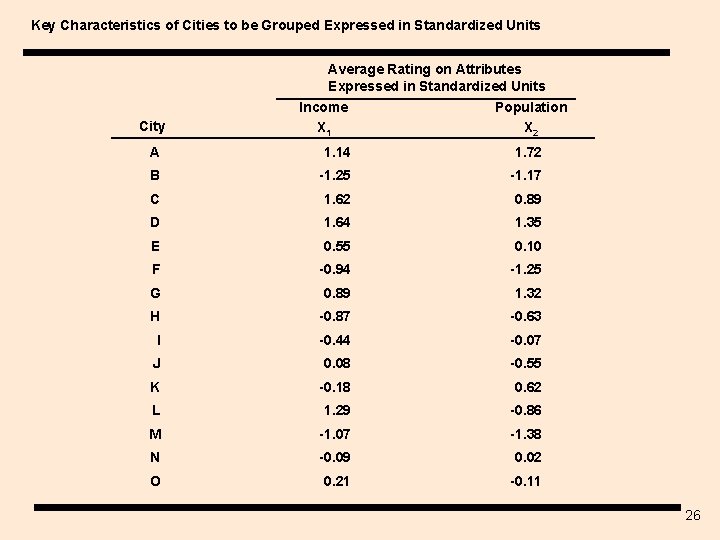
Key Characteristics of Cities to be Grouped Expressed in Standardized Units City Average Rating on Attributes Expressed in Standardized Units Income Population X 1 X 2 A 1. 14 1. 72 B -1. 25 -1. 17 C 1. 62 0. 89 D 1. 64 1. 35 E 0. 55 0. 10 F -0. 94 -1. 25 G 0. 89 1. 32 H -0. 87 -0. 63 I -0. 44 -0. 07 J 0. 08 -0. 55 K -0. 18 0. 62 L 1. 29 -0. 86 M -1. 07 -1. 38 N -0. 09 0. 02 O 0. 21 -0. 11 26
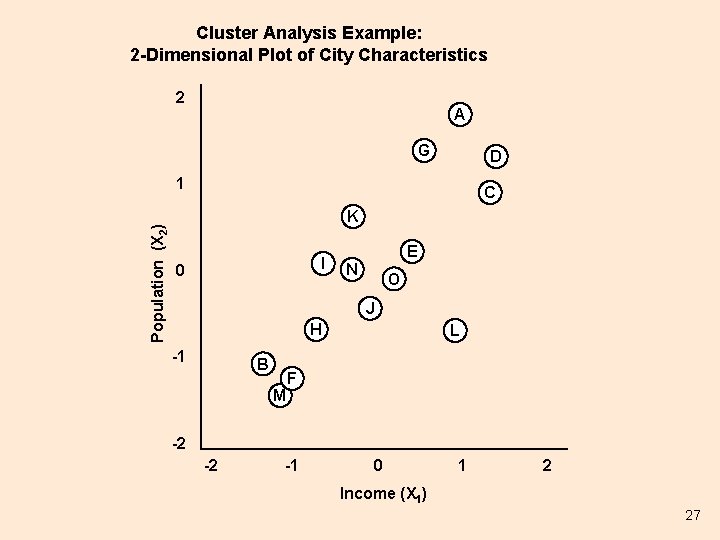
Cluster Analysis Example: 2 -Dimensional Plot of City Characteristics 2 A G D Population (X 2) 1 C K I 0 E N O J H -1 B M L F -2 -2 -1 0 1 2 Income (X 1) 27
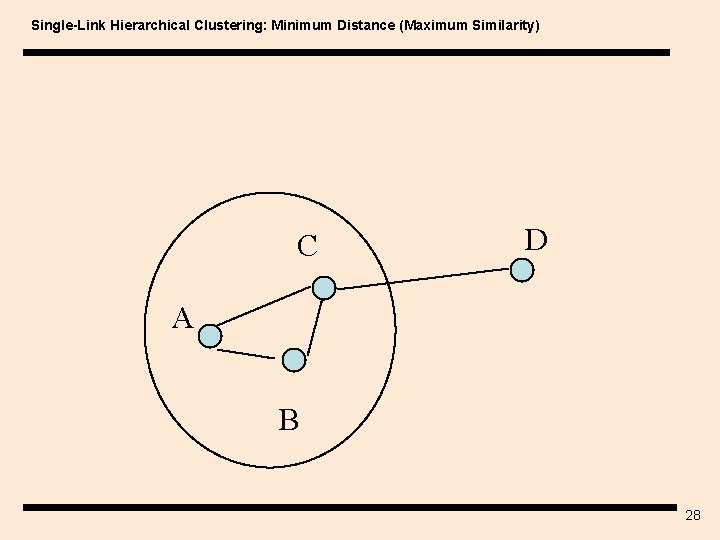
Single-Link Hierarchical Clustering: Minimum Distance (Maximum Similarity) C D A B 28
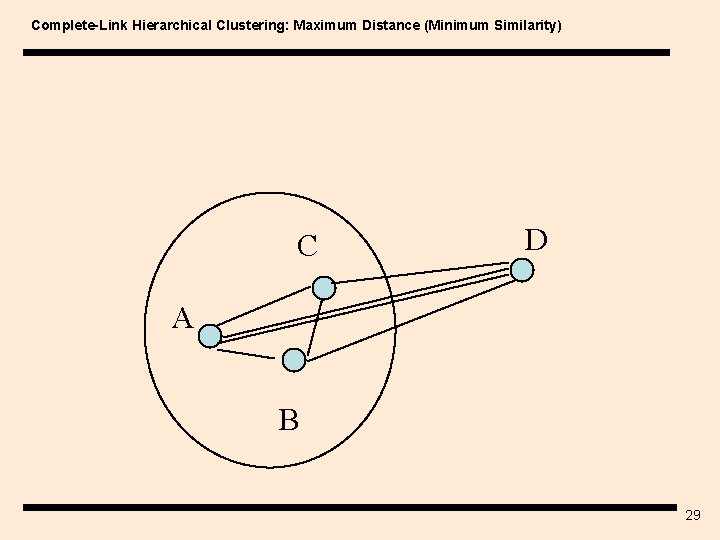
Complete-Link Hierarchical Clustering: Maximum Distance (Minimum Similarity) C D A B 29
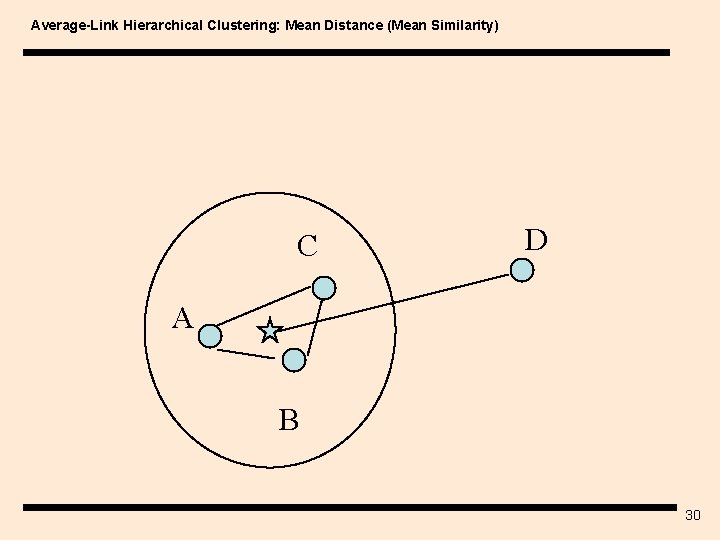
Average-Link Hierarchical Clustering: Mean Distance (Mean Similarity) C D A B 30
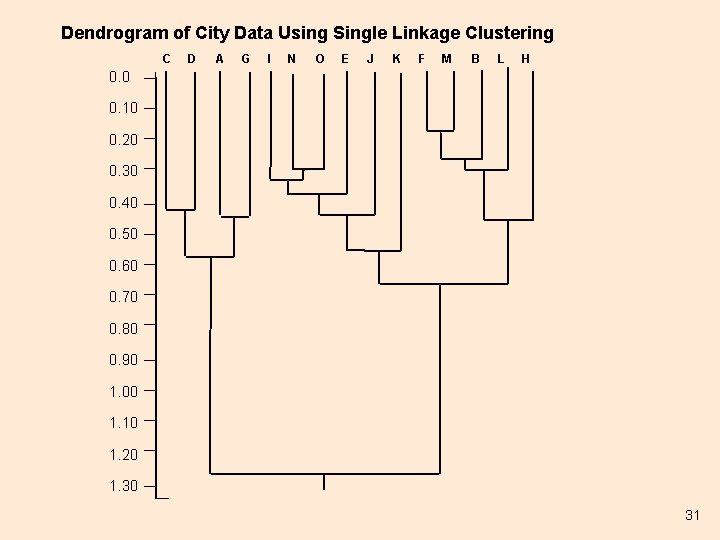
Dendrogram of City Data Using Single Linkage Clustering C D A G I N O E J K F M B L H 0. 0 0. 10 0. 20 0. 30 0. 40 0. 50 0. 60 0. 70 0. 80 0. 90 1. 00 1. 10 1. 20 1. 30 31
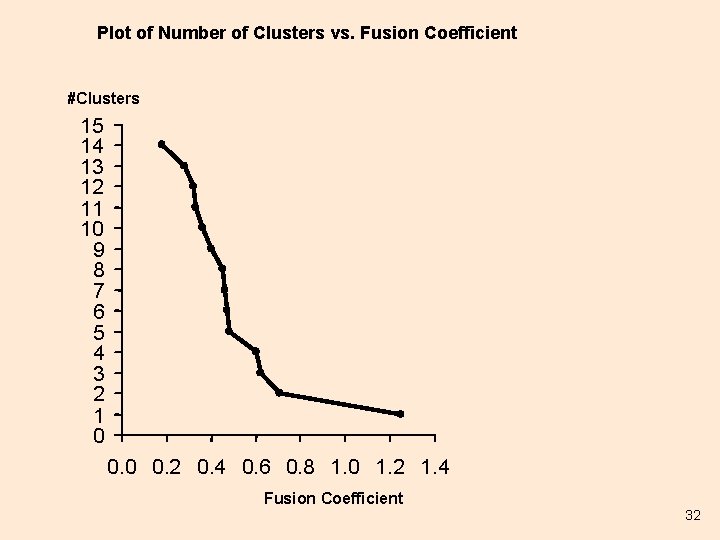
Plot of Number of Clusters vs. Fusion Coefficient #Clusters 15 14 13 12 11 10 9 8 7 6 5 4 3 2 1 0 0. 2 0. 4 0. 6 0. 8 1. 0 1. 2 1. 4 Fusion Coefficient 32
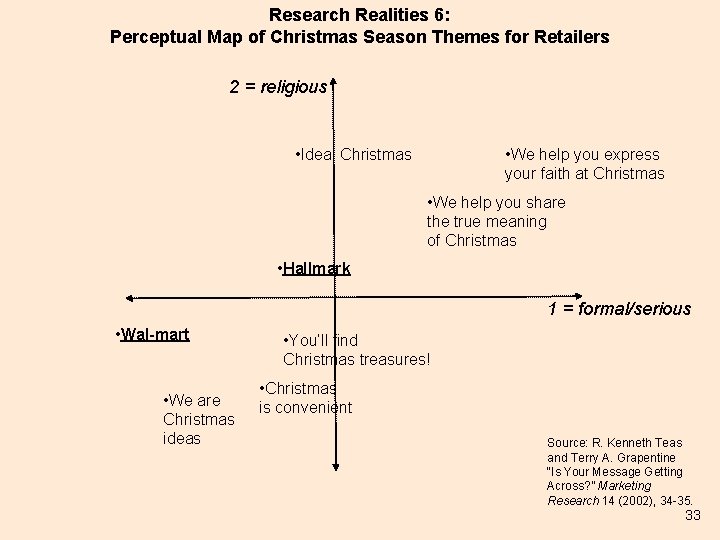
Research Realities 6: Perceptual Map of Christmas Season Themes for Retailers 2 = religious • Ideal Christmas • We help you express your faith at Christmas • We help you share the true meaning of Christmas • Hallmark 1 = formal/serious • Wal-mart • We are Christmas ideas • You’ll find Christmas treasures! • Christmas is convenient Source: R. Kenneth Teas and Terry A. Grapentine “Is Your Message Getting Across? ” Marketing Research 14 (2002), 34 -35. 33
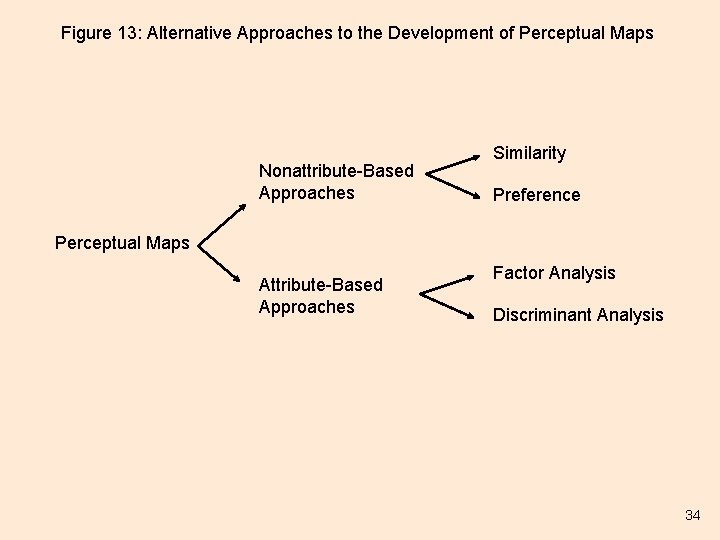
Figure 13: Alternative Approaches to the Development of Perceptual Maps Nonattribute-Based Approaches Similarity Preference Perceptual Maps Attribute-Based Approaches Factor Analysis Discriminant Analysis 34
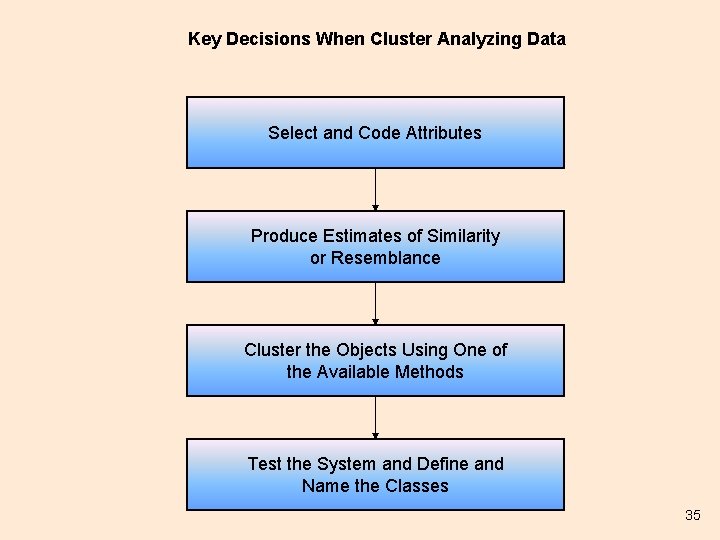
Key Decisions When Cluster Analyzing Data Select and Code Attributes Produce Estimates of Similarity or Resemblance Cluster the Objects Using One of the Available Methods Test the System and Define and Name the Classes 35
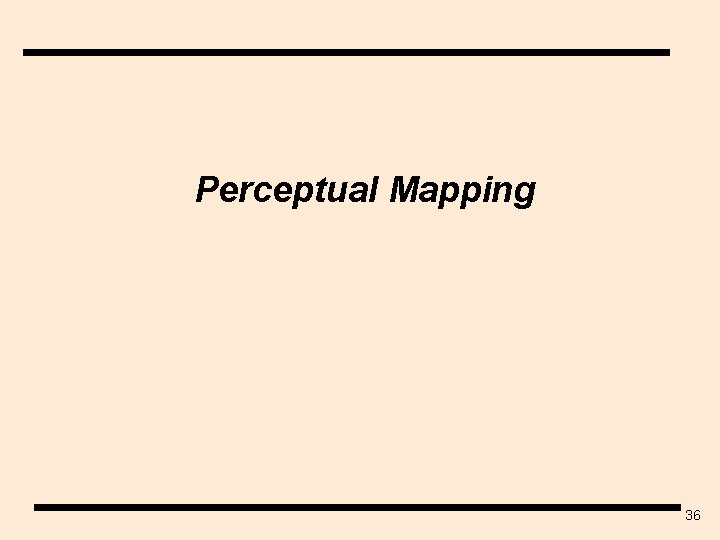
Perceptual Mapping 36
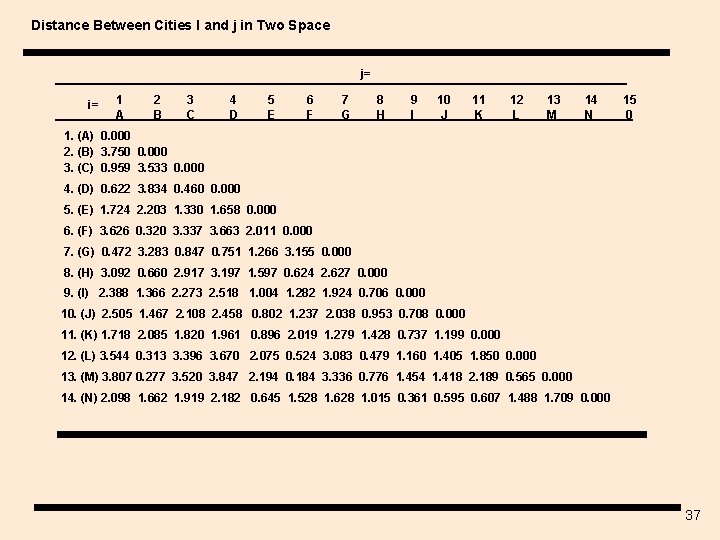
Distance Between Cities I and j in Two Space j= i= 1 A 2 B 3 C 4 D 5 E 6 F 7 G 8 H 9 I 10 J 11 K 12 L 13 M 14 N 15 0 1. (A) 0. 000 2. (B) 3. 750 0. 000 3. (C) 0. 959 3. 533 0. 000 4. (D) 0. 622 3. 834 0. 460 0. 000 5. (E) 1. 724 2. 203 1. 330 1. 658 0. 000 6. (F) 3. 626 0. 320 3. 337 3. 663 2. 011 0. 000 7. (G) 0. 472 3. 283 0. 847 0. 751 1. 266 3. 155 0. 000 8. (H) 3. 092 0. 660 2. 917 3. 197 1. 597 0. 624 2. 627 0. 000 9. (I) 2. 388 1. 366 2. 273 2. 518 1. 004 1. 282 1. 924 0. 706 0. 000 10. (J) 2. 505 1. 467 2. 108 2. 458 0. 802 1. 237 2. 038 0. 953 0. 708 0. 000 11. (K) 1. 718 2. 085 1. 820 1. 961 0. 896 2. 019 1. 279 1. 428 0. 737 1. 199 0. 000 12. (L) 3. 544 0. 313 3. 396 3. 670 2. 075 0. 524 3. 083 0. 479 1. 160 1. 405 1. 850 0. 000 13. (M) 3. 807 0. 277 3. 520 3. 847 2. 194 0. 184 3. 336 0. 776 1. 454 1. 418 2. 189 0. 565 0. 000 14. (N) 2. 098 1. 662 1. 919 2. 182 0. 645 1. 528 1. 628 1. 015 0. 361 0. 595 0. 607 1. 488 1. 709 0. 000 37
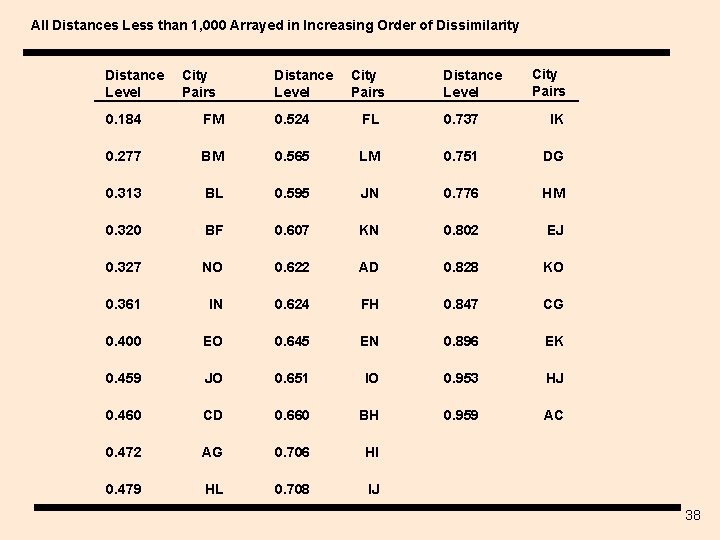
All Distances Less than 1, 000 Arrayed in Increasing Order of Dissimilarity Distance Level City Pairs 0. 184 FM 0. 524 FL 0. 737 IK 0. 277 BM 0. 565 LM 0. 751 DG 0. 313 BL 0. 595 JN 0. 776 HM 0. 320 BF 0. 607 KN 0. 802 EJ 0. 327 NO 0. 622 AD 0. 828 KO 0. 361 IN 0. 624 FH 0. 847 CG 0. 400 EO 0. 645 EN 0. 896 EK 0. 459 JO 0. 651 IO 0. 953 HJ 0. 460 CD 0. 660 BH 0. 959 AC 0. 472 AG 0. 706 HI 0. 479 HL 0. 708 IJ 38
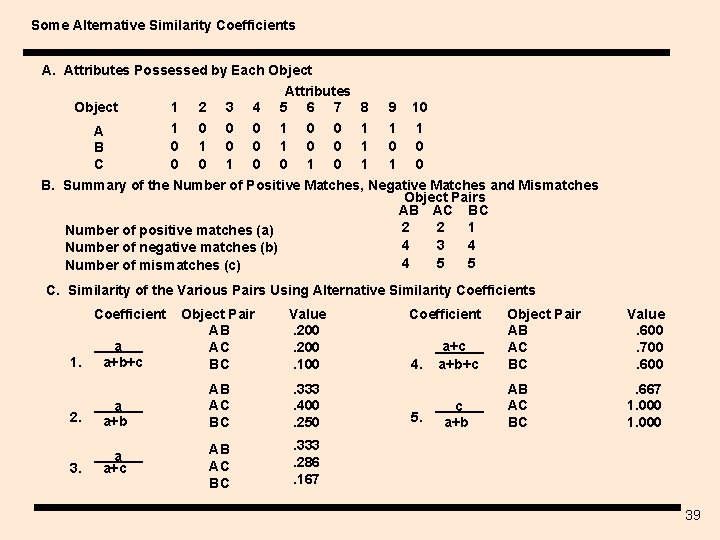
Some Alternative Similarity Coefficients A. Attributes Possessed by Each Object 1 2 3 4 Attributes 5 6 7 8 A B C 1 0 0 0 1 0 0 0 1 1 1 9 10 1 1 0 0 B. Summary of the Number of Positive Matches, Negative Matches and Mismatches Object Pairs AB AC BC 2 2 1 Number of positive matches (a) 4 3 4 Number of negative matches (b) 4 5 5 Number of mismatches (c) C. Similarity of the Various Pairs Using Alternative Similarity Coefficients Coefficient 1. a a+b+c 2. a a+b 3. a a+c Object Pair AB AC BC Value. 200. 100 AB AC BC . 333. 400. 250 AB AC BC . 333. 286. 167 Coefficient 4. 5. a+c a+b+c c a+b Object Pair AB AC BC Value. 600. 700. 600 AB AC BC . 667 1. 000 39
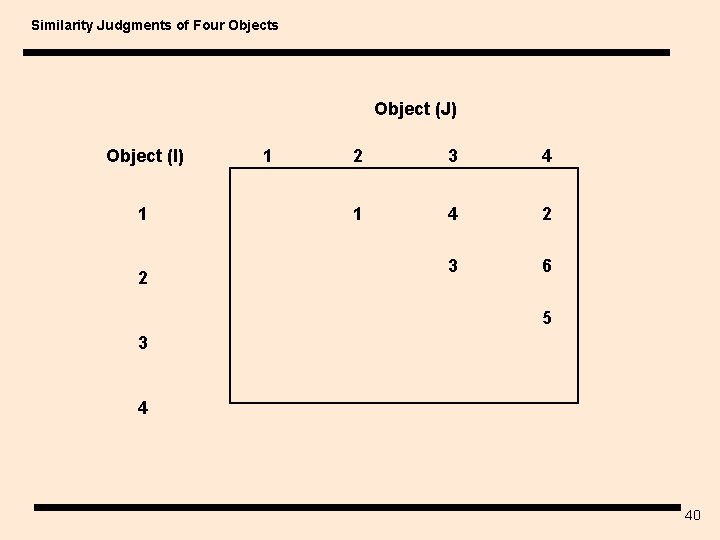
Similarity Judgments of Four Objects Object (J) Object (I) 1 2 3 4 1 4 2 3 6 5 3 4 40
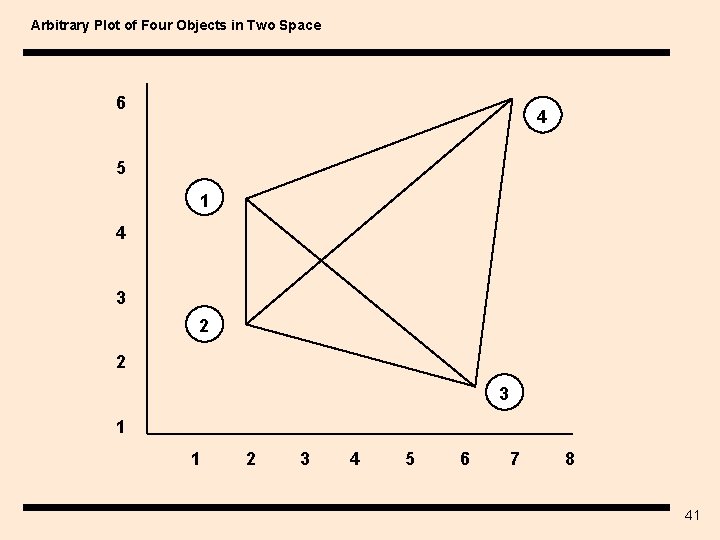
Arbitrary Plot of Four Objects in Two Space 6 4 5 1 4 3 2 2 3 1 1 2 3 4 5 6 7 8 41
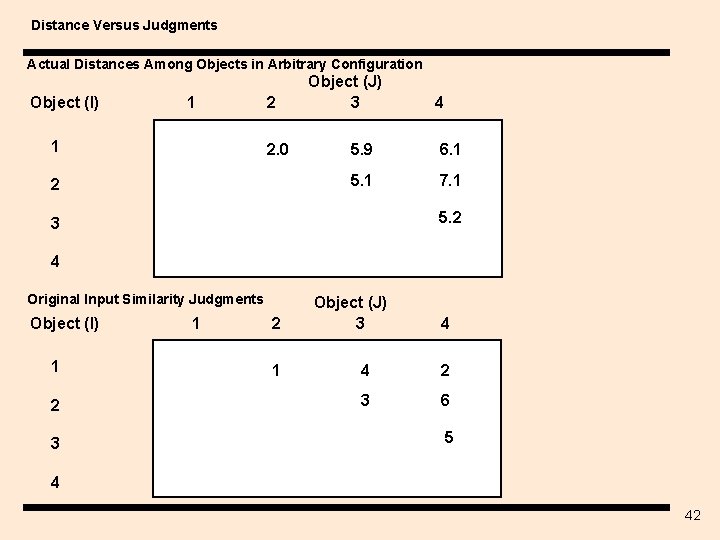
Distance Versus Judgments Actual Distances Among Objects in Arbitrary Configuration Object (I) 1 1 2 2. 0 2 Object (J) 3 4 5. 9 6. 1 5. 1 7. 1 5. 2 3 4 Original Input Similarity Judgments Object (I) 1 2 3 1 2 1 Object (J) 3 4 4 2 3 6 5 4 42
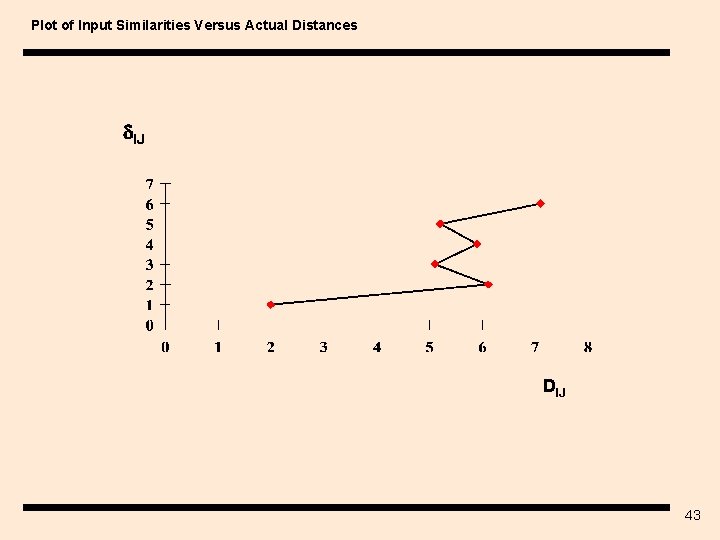
Plot of Input Similarities Versus Actual Distances IJ DIJ 43
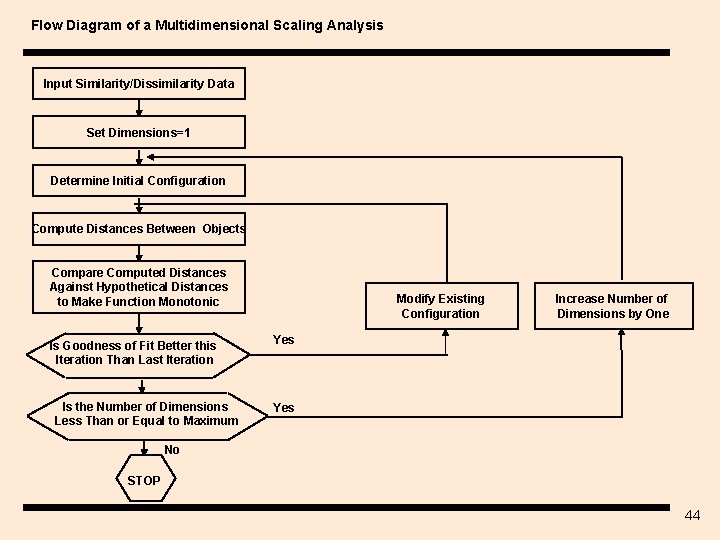
Flow Diagram of a Multidimensional Scaling Analysis Input Similarity/Dissimilarity Data Set Dimensions=1 Determine Initial Configuration Compute Distances Between Objects Compare Computed Distances Against Hypothetical Distances to Make Function Monotonic Is Goodness of Fit Better this Iteration Than Last Iteration Is the Number of Dimensions Less Than or Equal to Maximum Modify Existing Configuration Increase Number of Dimensions by One Yes No STOP 44
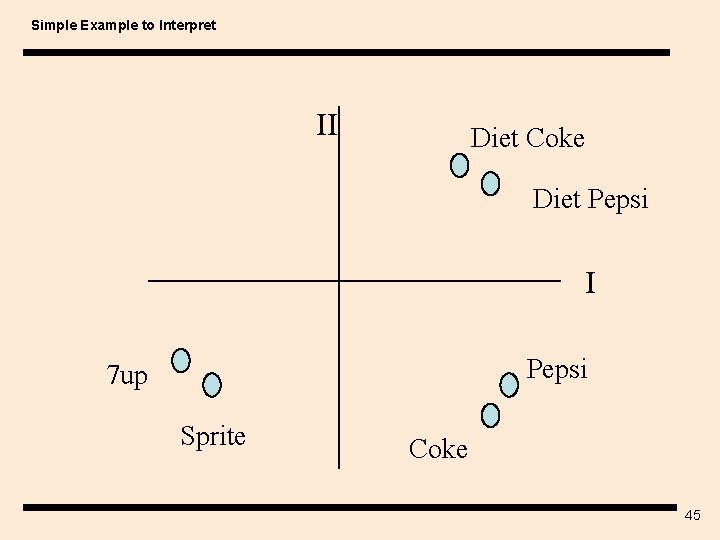
Simple Example to Interpret II Diet Coke Diet Pepsi I Pepsi 7 up Sprite Coke 45
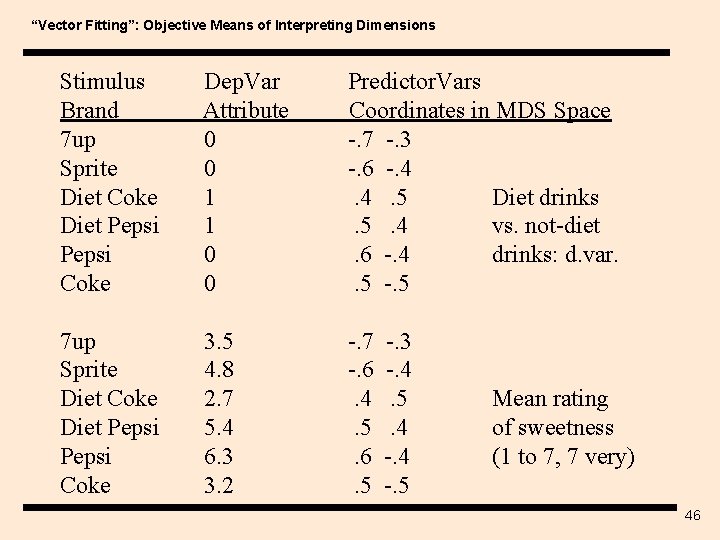
“Vector Fitting”: Objective Means of Interpreting Dimensions Stimulus Brand 7 up Sprite Diet Coke Diet Pepsi Coke Dep. Var Attribute 0 0 1 1 0 0 Predictor. Vars Coordinates in MDS Space -. 7 -. 3 -. 6 -. 4. 4. 5 Diet drinks. 5. 4 vs. not-diet. 6 -. 4 drinks: d. var. . 5 -. 5 7 up Sprite Diet Coke Diet Pepsi Coke 3. 5 4. 8 2. 7 5. 4 6. 3 3. 2 -. 7 -. 6. 4. 5. 6. 5 -. 3 -. 4. 5. 4 -. 5 Mean rating of sweetness (1 to 7, 7 very) 46
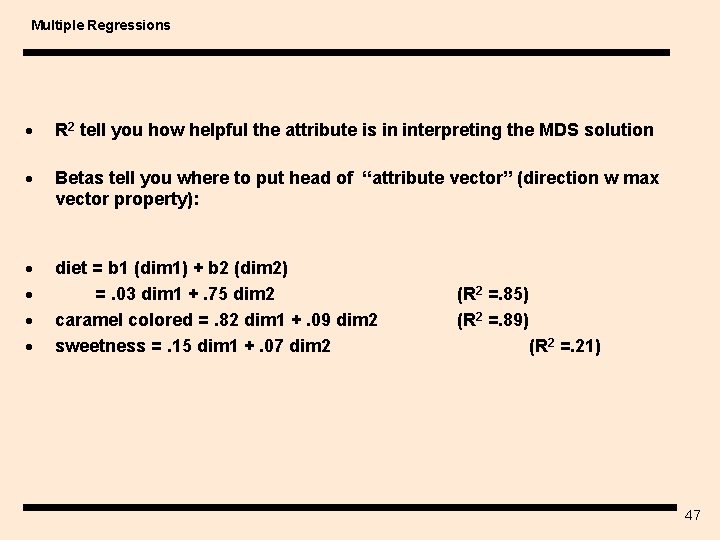
Multiple Regressions · R 2 tell you how helpful the attribute is in interpreting the MDS solution · Betas tell you where to put head of “attribute vector” (direction w max vector property): · · diet = b 1 (dim 1) + b 2 (dim 2) =. 03 dim 1 +. 75 dim 2 caramel colored =. 82 dim 1 +. 09 dim 2 sweetness =. 15 dim 1 +. 07 dim 2 (R 2 =. 85) (R 2 =. 89) (R 2 =. 21) 47
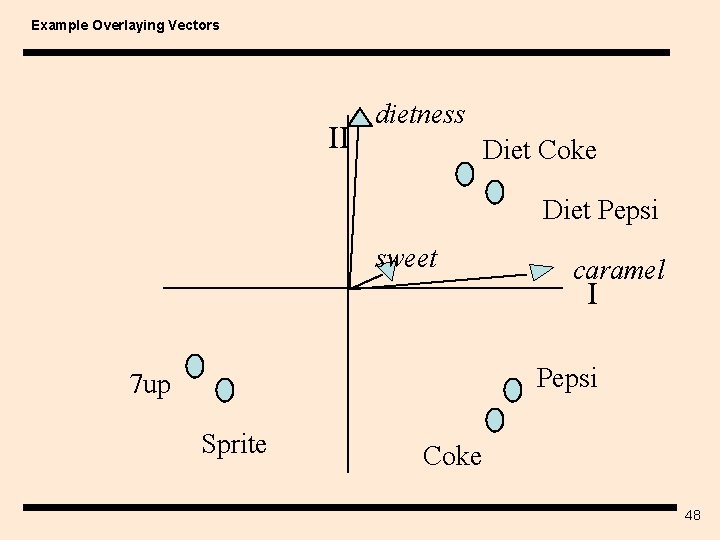
Example Overlaying Vectors II dietness Diet Coke Diet Pepsi sweet caramel I Pepsi 7 up Sprite Coke 48
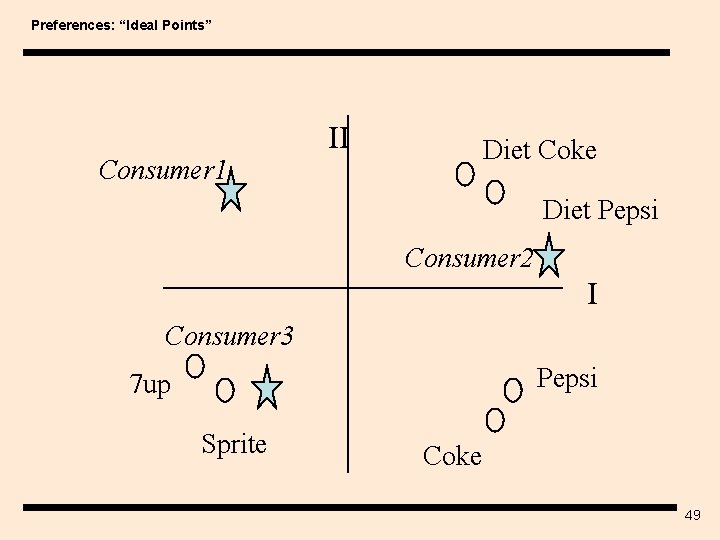
Preferences: “Ideal Points” Consumer 1 II Diet Coke Diet Pepsi Consumer 2 I Consumer 3 Pepsi 7 up Sprite Coke 49
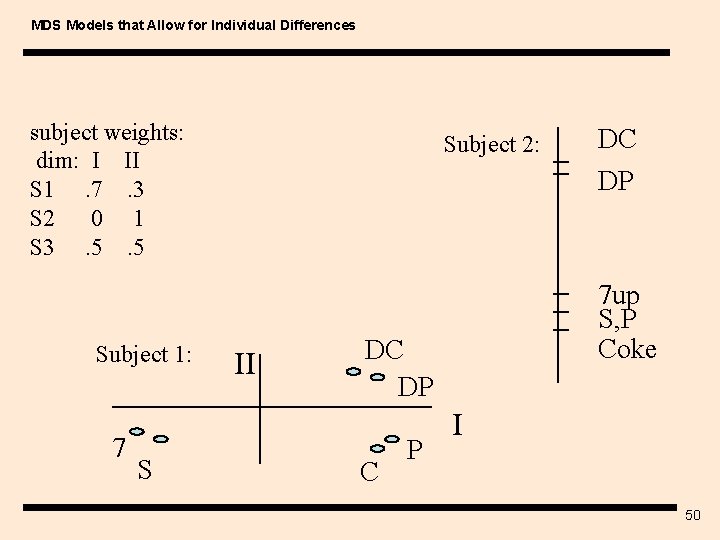
MDS Models that Allow for Individual Differences subject weights: dim: I II S 1. 7. 3 S 2 0 1 S 3. 5. 5 Subject 1: 7 S Subject 2: II 7 up S, P Coke DC DP C P DC DP I 50
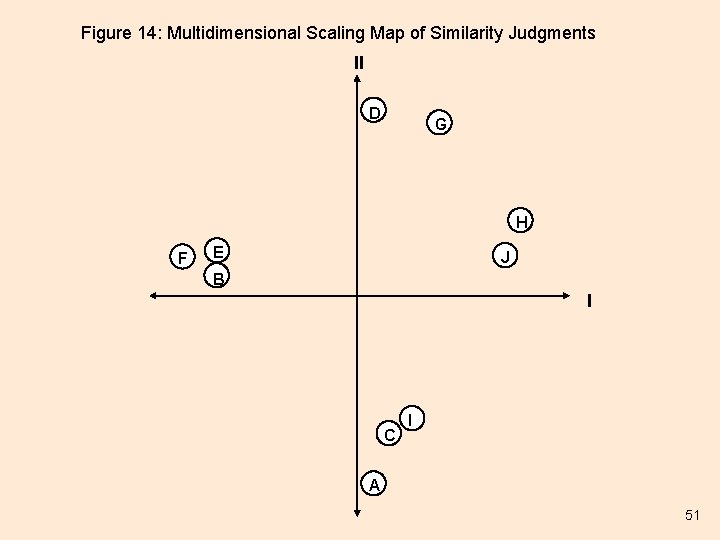
Figure 14: Multidimensional Scaling Map of Similarity Judgments II D G H F E J B I C I A 51
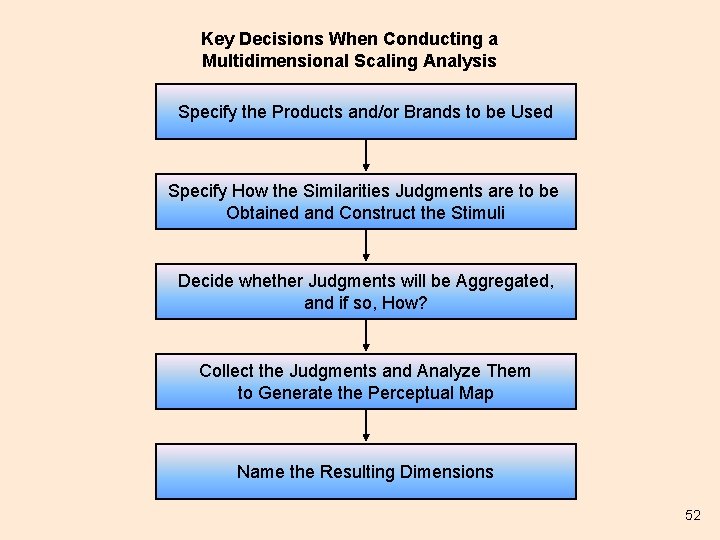
Key Decisions When Conducting a Multidimensional Scaling Analysis Specify the Products and/or Brands to be Used Specify How the Similarities Judgments are to be Obtained and Construct the Stimuli Decide whether Judgments will be Aggregated, and if so, How? Collect the Judgments and Analyze Them to Generate the Perceptual Map Name the Resulting Dimensions 52
Jaundice
What is multivariate analysis?
Analysis of variance
Nature of multivariate analysis
Multivariate analysis of variance and covariance
Multivariate analysis
Multivariate statistical analysis
Multivariate analysis
Multivariate analysis
Multivariate pattern analysis
Discriminant analysis assumptions
Canonical discriminant function
Logistic regression and discriminant analysis
Flexible discriminant analysis
Discriminant analysis marketing
Hair et al
Discriminant analysis marketing
Multivariate logistische regression
Multivariate binomial distribution
Multivariate pdf
Multiple regression assumptions spss
Log likelihood of multivariate normal distribution
Maximum a posteriori estimation for multivariate gaussian
Multivariate pdf
Bivariate vs multivariate
Mixed design anova spss
Univariate vs multivariate logistic regression
Multivariate methods in machine learning
Multivariate verfahren psychologie
Univariate vs multivariate
Multivariate scatter plot
Advanced and multivariate statistical methods
Multivariate descriptive statistics
Multivariate statistics for the environmental sciences
Multivariate histogram
Factor analysis ordinal data
Factoring by gcf
Factor out the greatest common factor
Average of sine wave
Situation-relating question
Factor gcf
Common factors of 18 and 30
Descriminant formula
Types of discriminant solutions
Criterion related validity definition
Minus b formula
Is the discriminant negative or positive
Discriminant equation
Understanding the discriminant
Quadratuc equation
My hrw
Site:slidetodoc.com
Tetrahedron axis of rotation