Arguments From Experience The PADUA Protocol Maya Wardeh
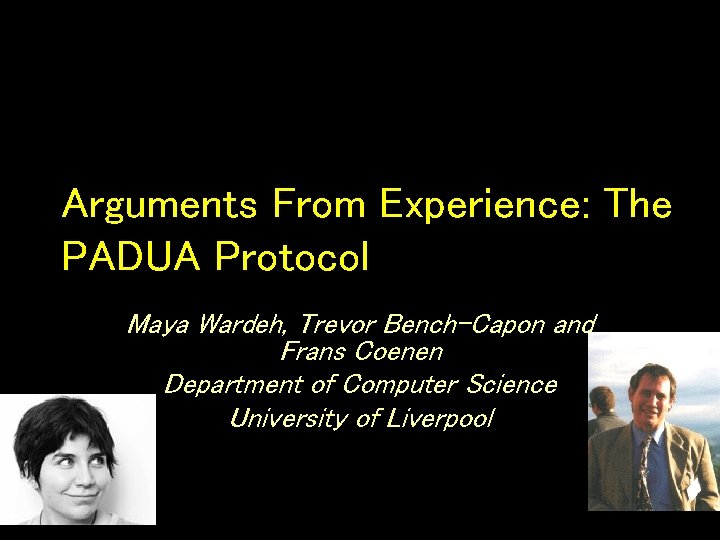
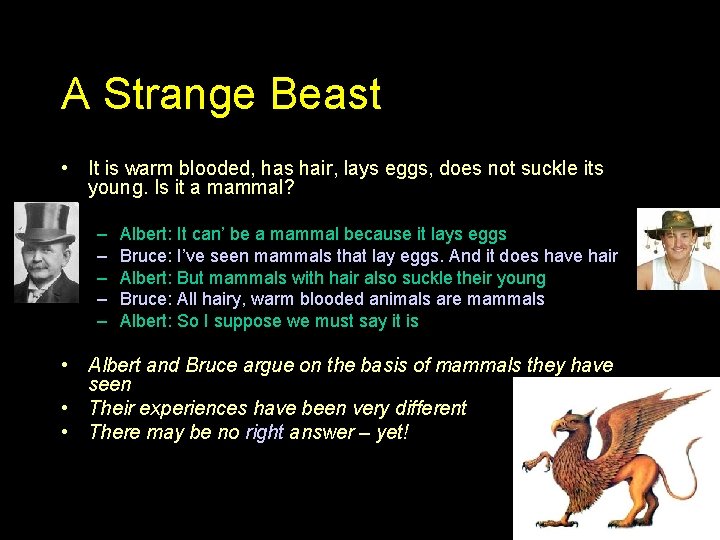
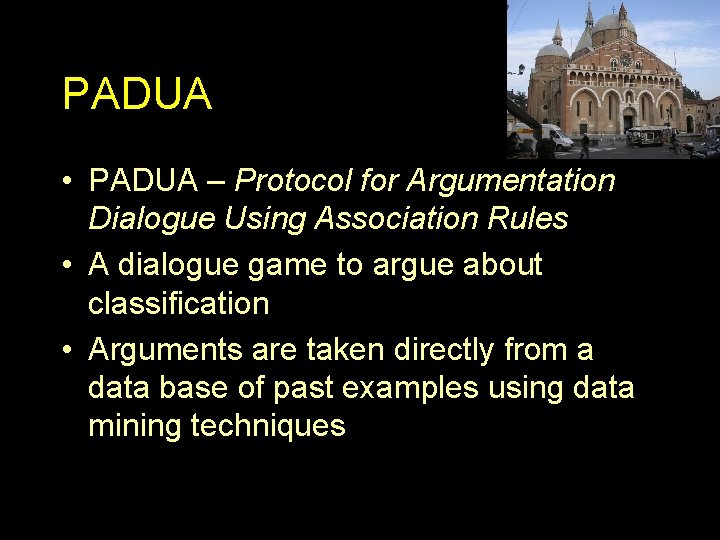
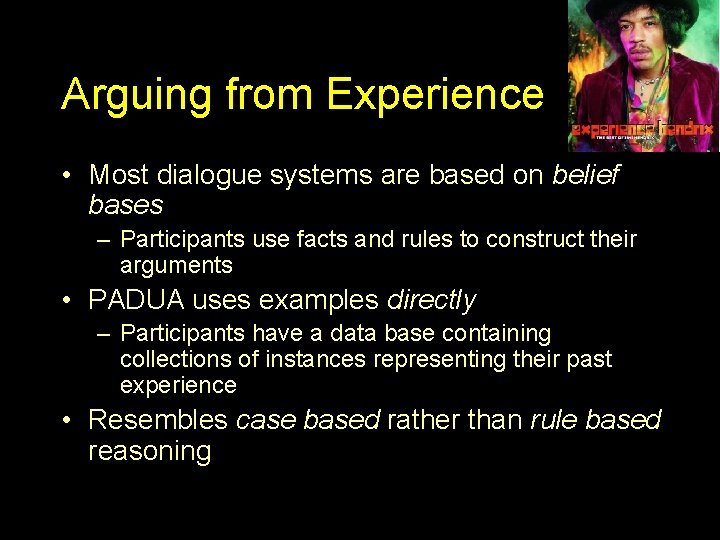
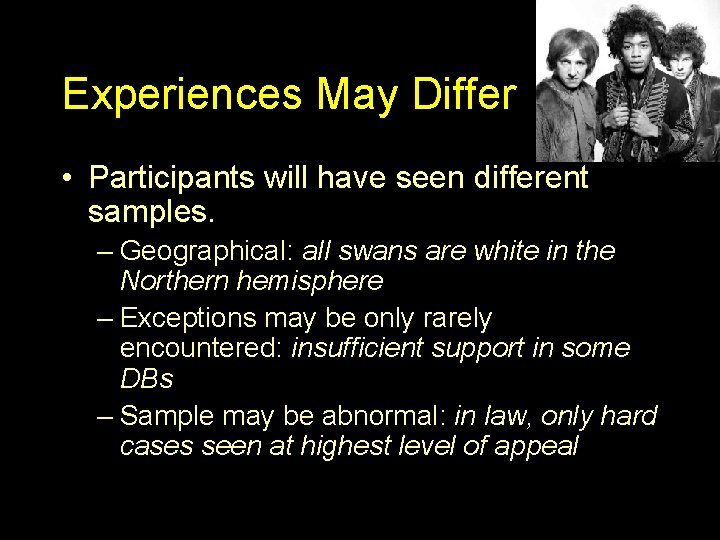
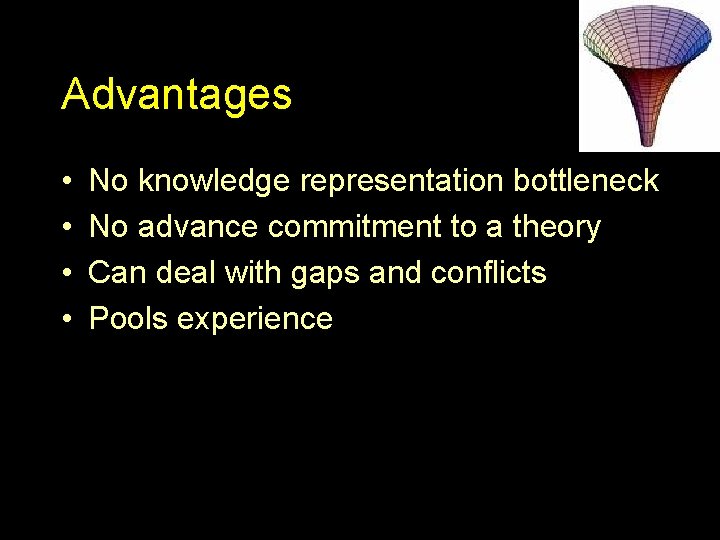
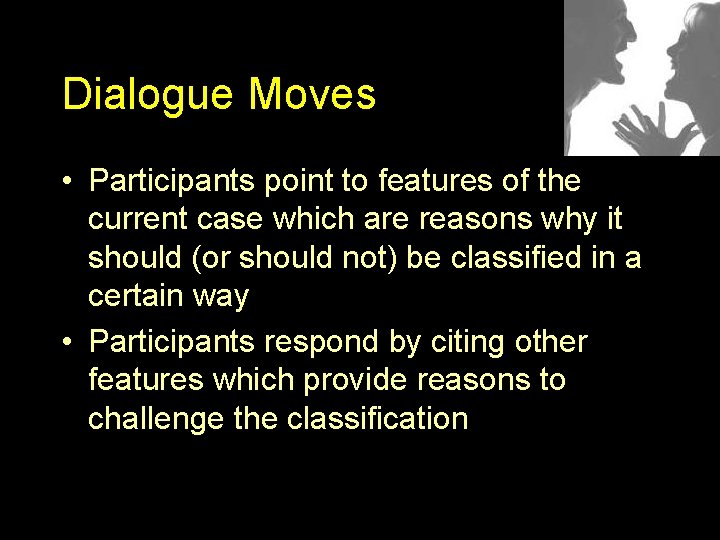
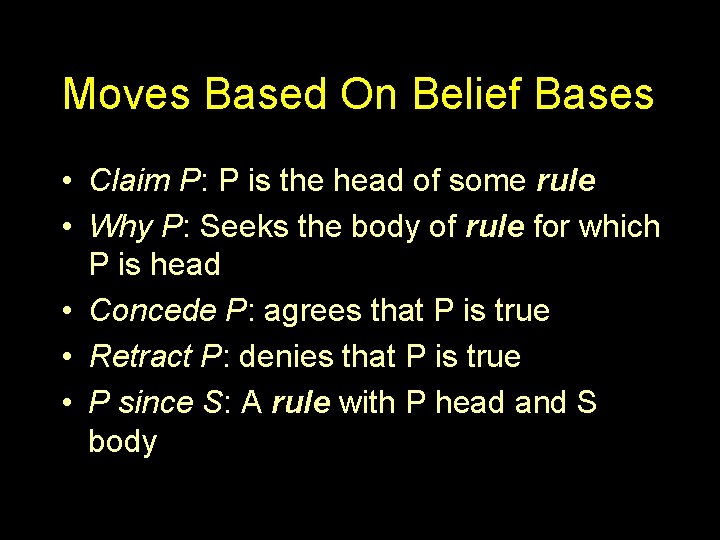
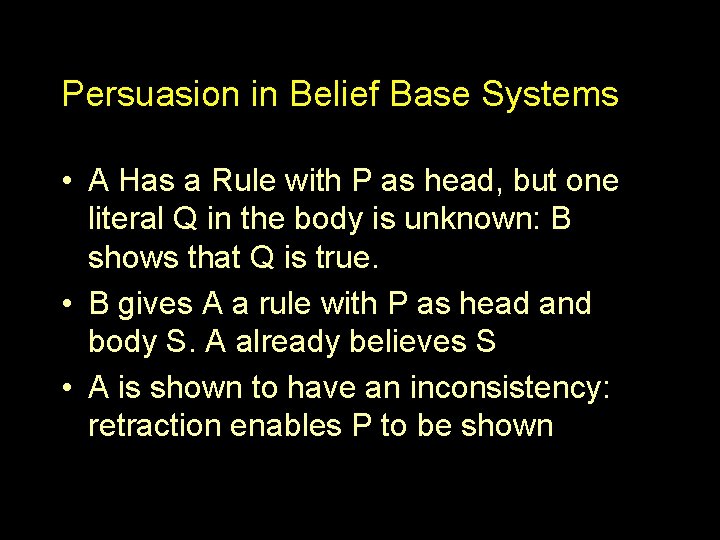
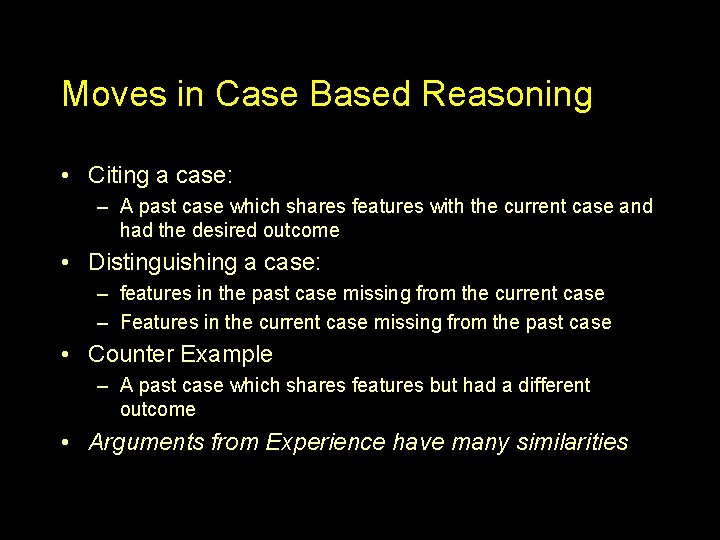
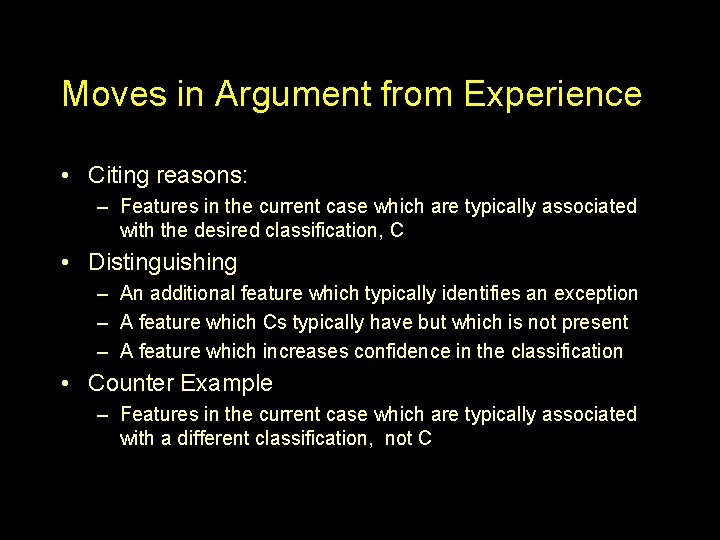
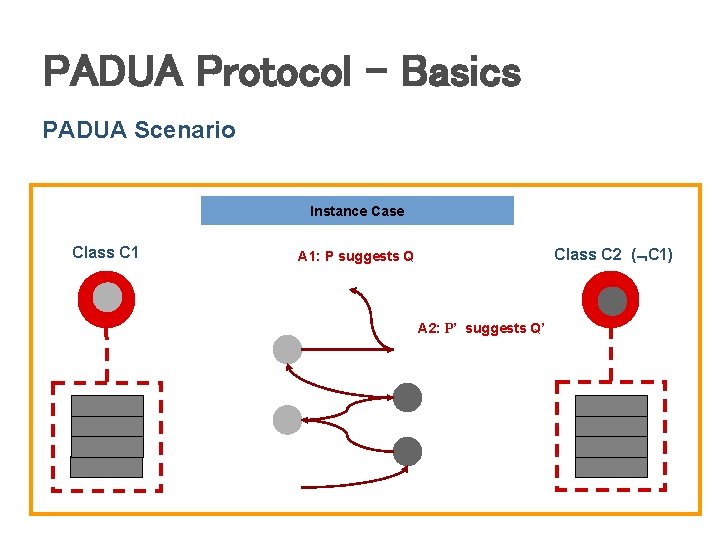
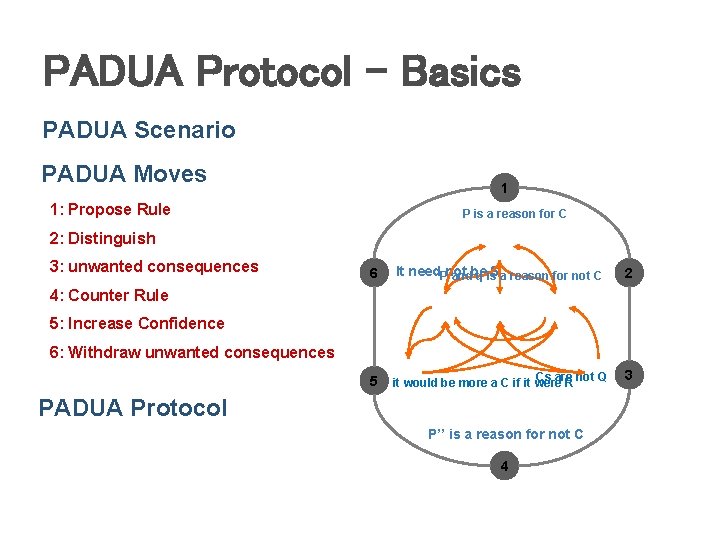
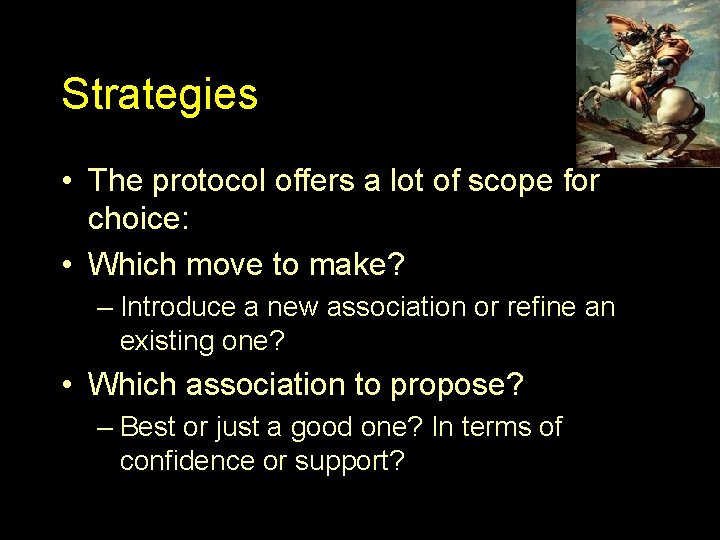
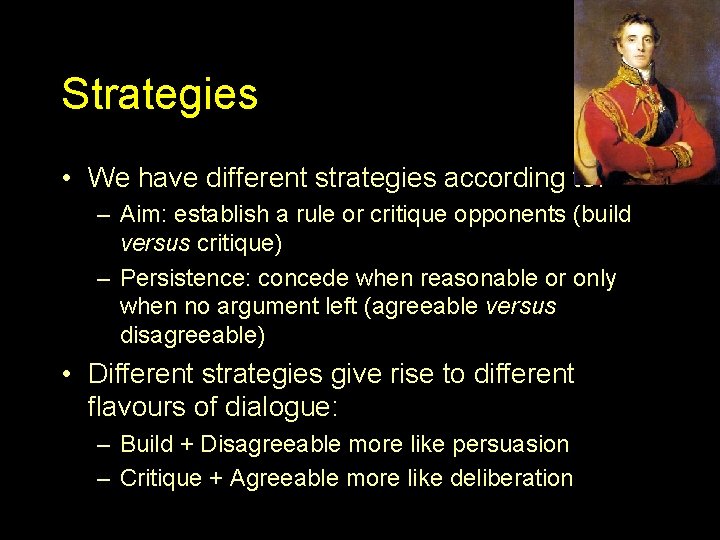
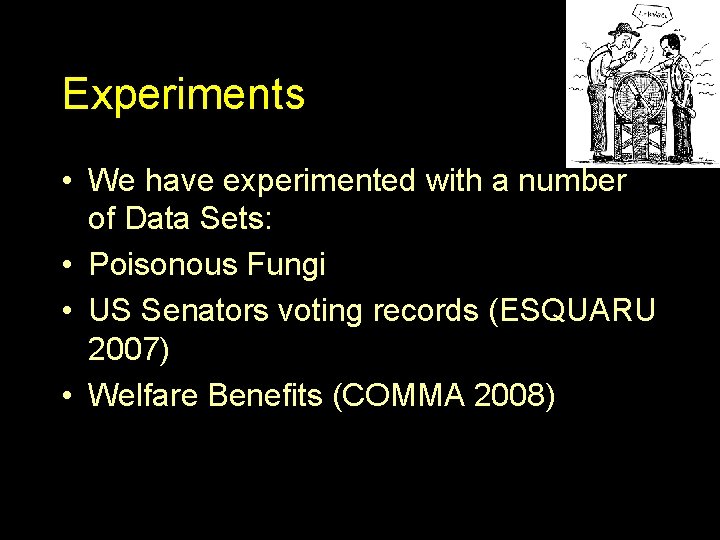
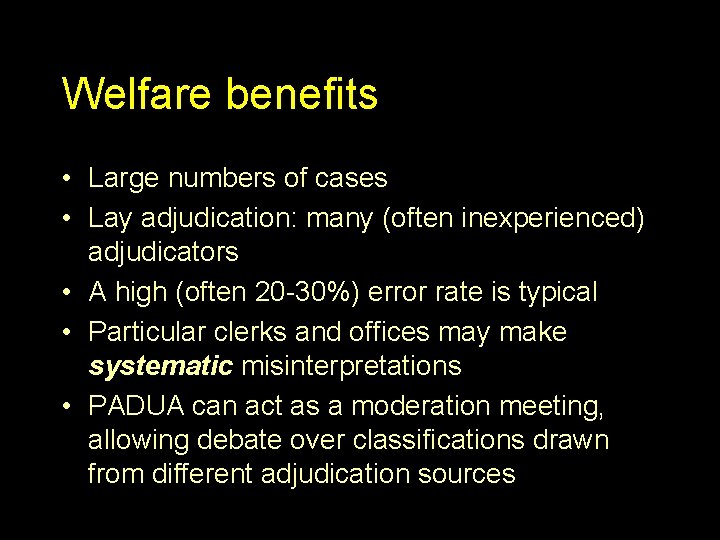
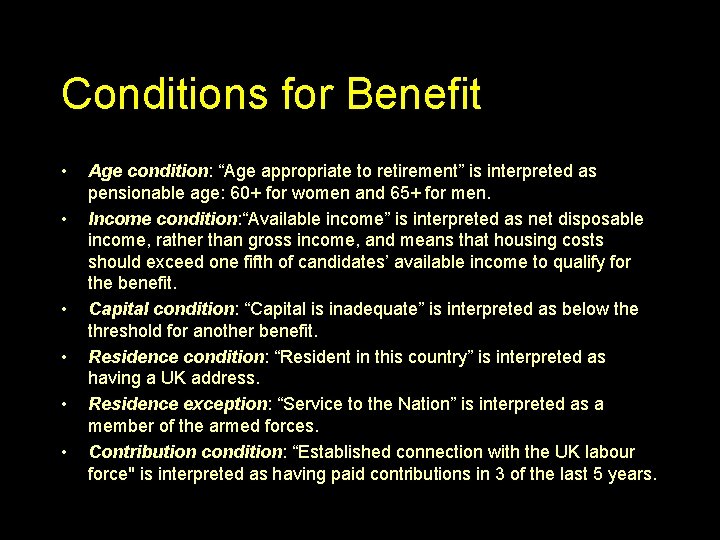
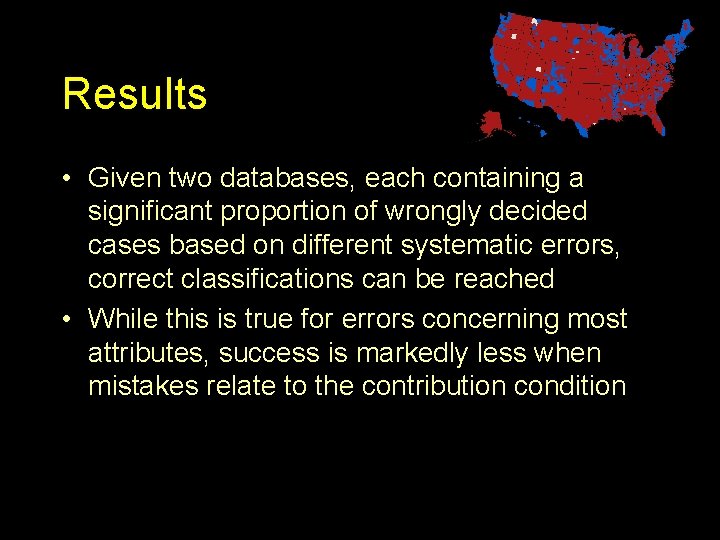
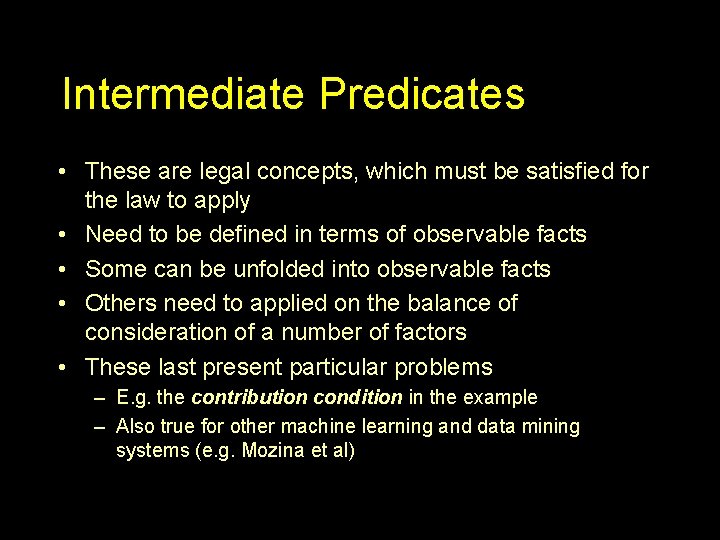
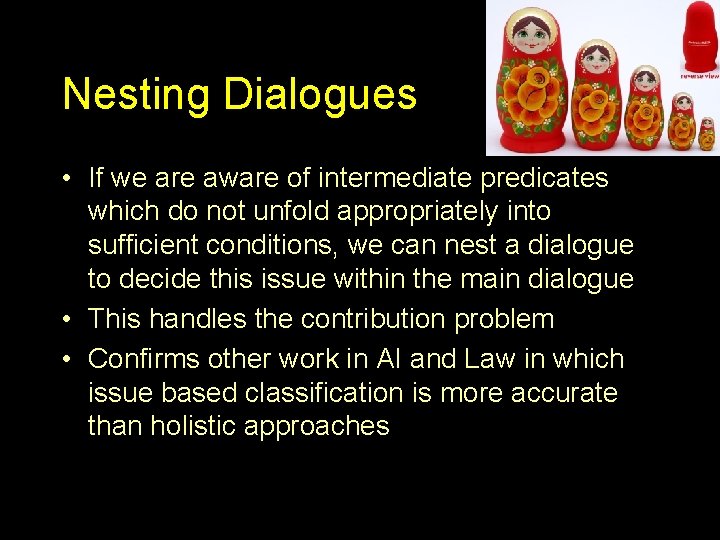
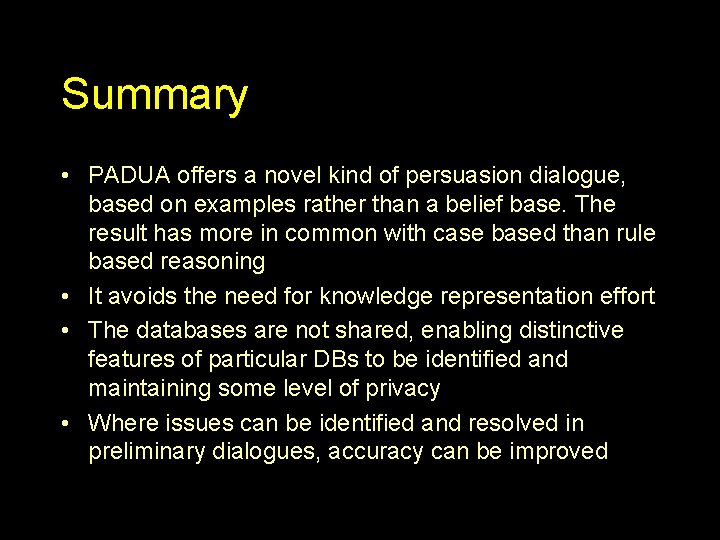
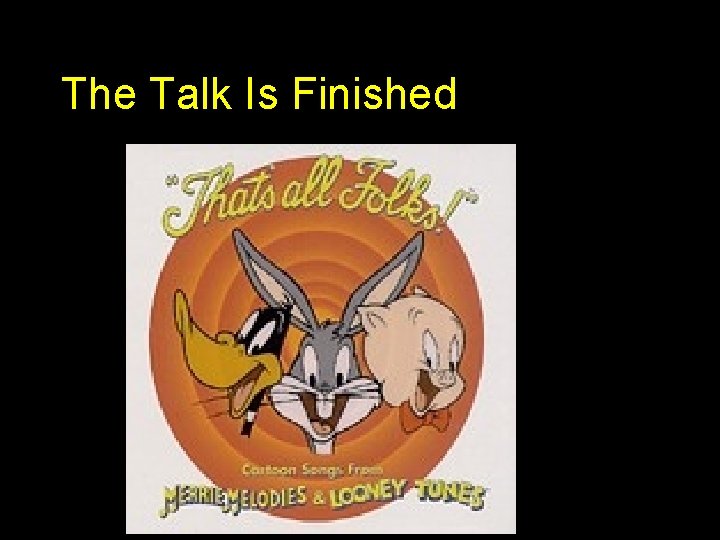
- Slides: 23
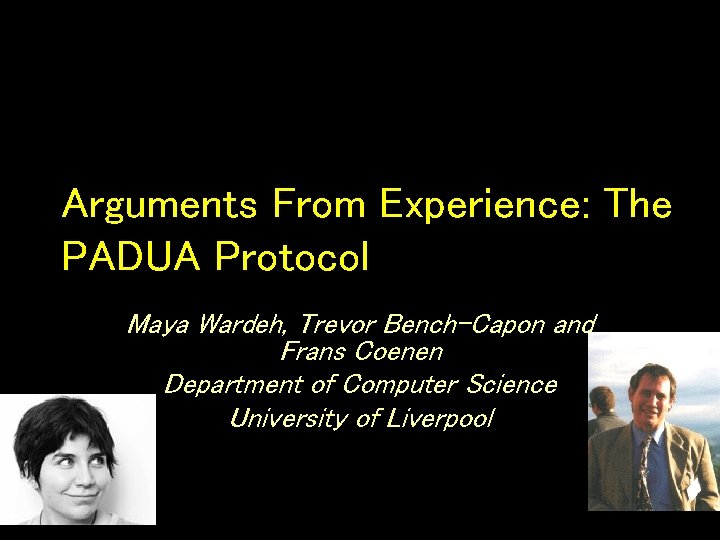
Arguments From Experience: The PADUA Protocol Maya Wardeh, Trevor Bench-Capon and Frans Coenen Department of Computer Science University of Liverpool
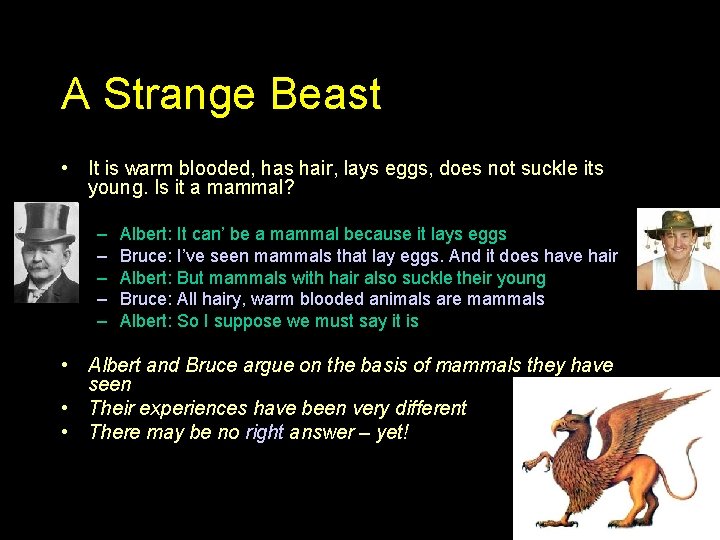
A Strange Beast • It is warm blooded, has hair, lays eggs, does not suckle its young. Is it a mammal? – – – Albert: It can’ be a mammal because it lays eggs Bruce: I’ve seen mammals that lay eggs. And it does have hair Albert: But mammals with hair also suckle their young Bruce: All hairy, warm blooded animals are mammals Albert: So I suppose we must say it is • Albert and Bruce argue on the basis of mammals they have seen • Their experiences have been very different • There may be no right answer – yet!
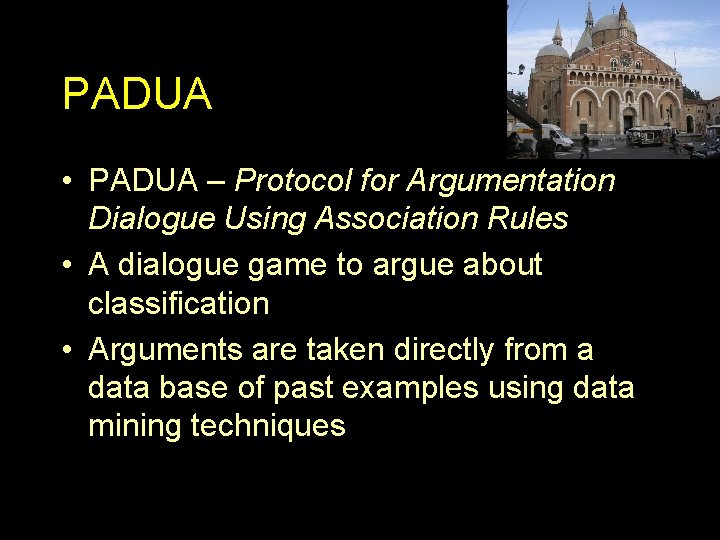
PADUA • PADUA – Protocol for Argumentation Dialogue Using Association Rules • A dialogue game to argue about classification • Arguments are taken directly from a data base of past examples using data mining techniques
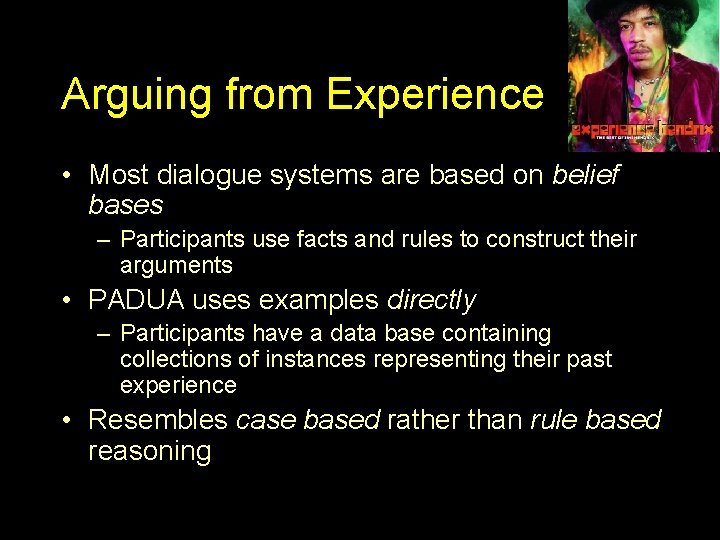
Arguing from Experience • Most dialogue systems are based on belief bases – Participants use facts and rules to construct their arguments • PADUA uses examples directly – Participants have a data base containing collections of instances representing their past experience • Resembles case based rather than rule based reasoning
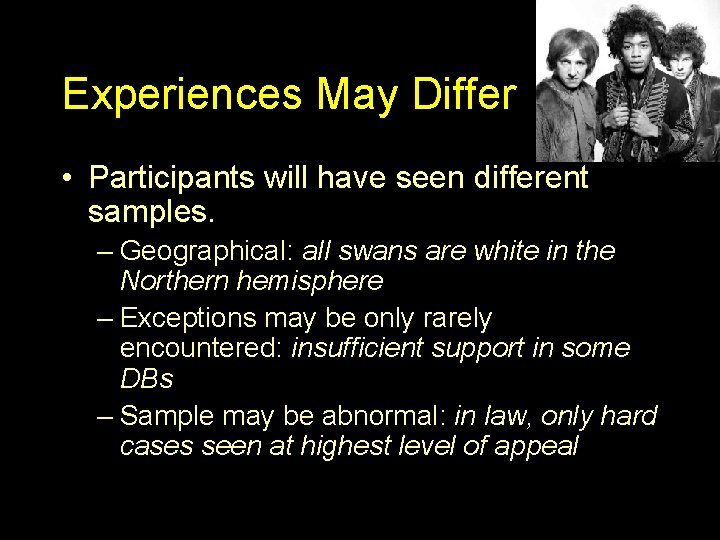
Experiences May Differ • Participants will have seen different samples. – Geographical: all swans are white in the Northern hemisphere – Exceptions may be only rarely encountered: insufficient support in some DBs – Sample may be abnormal: in law, only hard cases seen at highest level of appeal
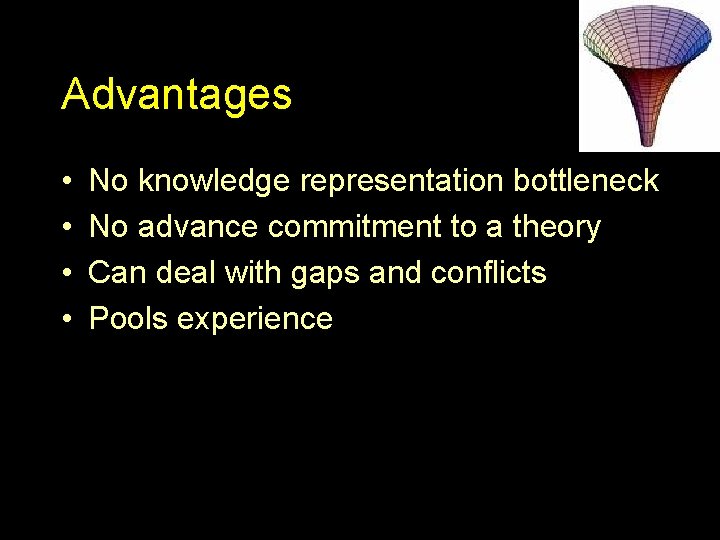
Advantages • • No knowledge representation bottleneck No advance commitment to a theory Can deal with gaps and conflicts Pools experience
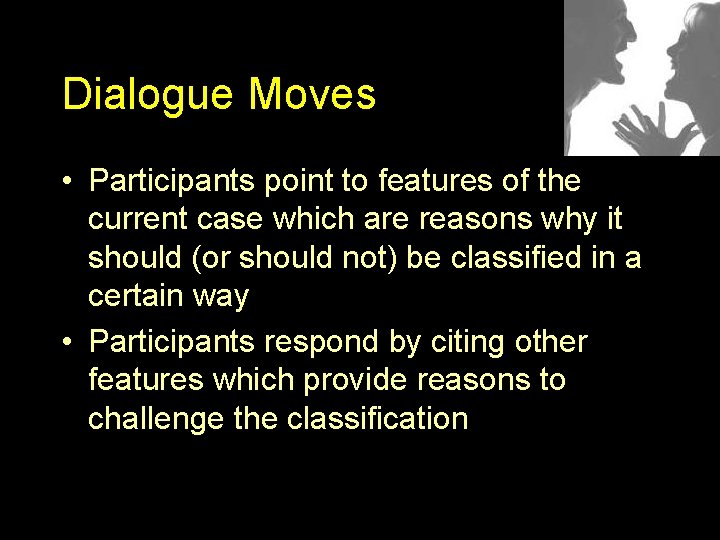
Dialogue Moves • Participants point to features of the current case which are reasons why it should (or should not) be classified in a certain way • Participants respond by citing other features which provide reasons to challenge the classification
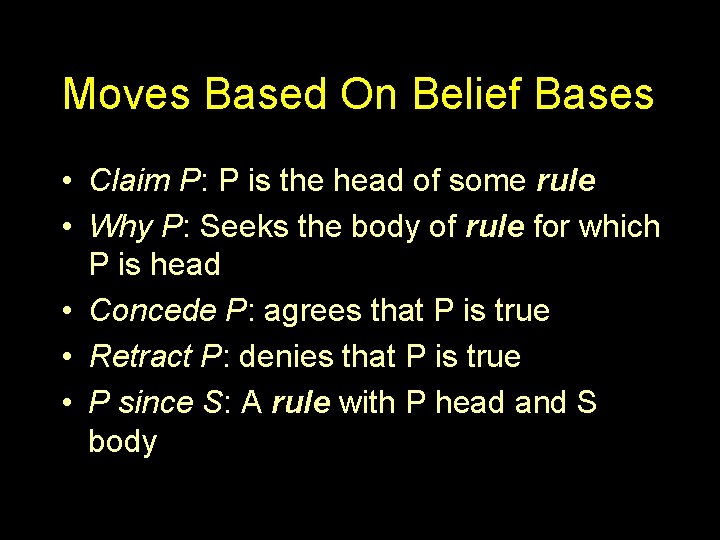
Moves Based On Belief Bases • Claim P: P is the head of some rule • Why P: Seeks the body of rule for which P is head • Concede P: agrees that P is true • Retract P: denies that P is true • P since S: A rule with P head and S body
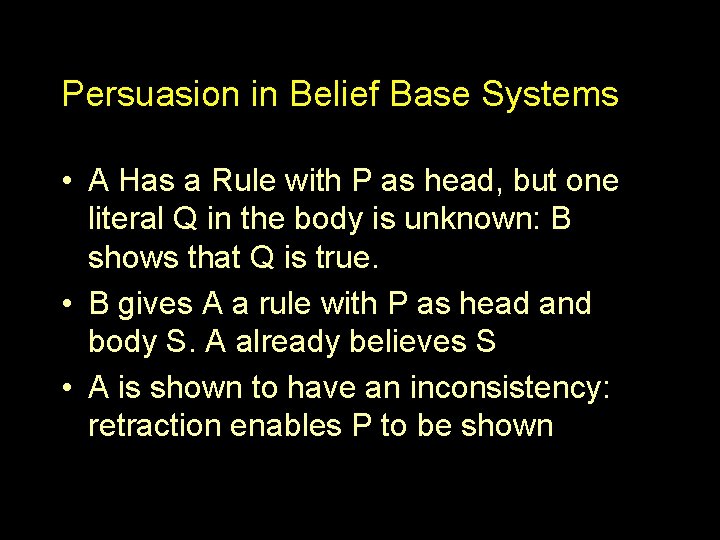
Persuasion in Belief Base Systems • A Has a Rule with P as head, but one literal Q in the body is unknown: B shows that Q is true. • B gives A a rule with P as head and body S. A already believes S • A is shown to have an inconsistency: retraction enables P to be shown
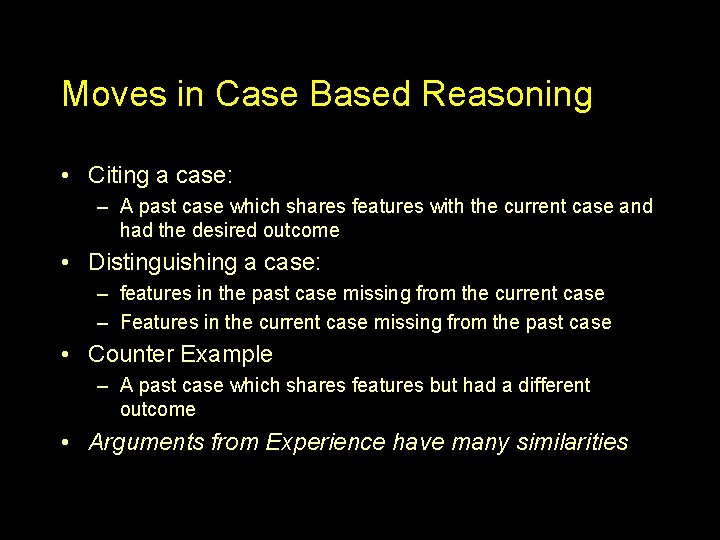
Moves in Case Based Reasoning • Citing a case: – A past case which shares features with the current case and had the desired outcome • Distinguishing a case: – features in the past case missing from the current case – Features in the current case missing from the past case • Counter Example – A past case which shares features but had a different outcome • Arguments from Experience have many similarities
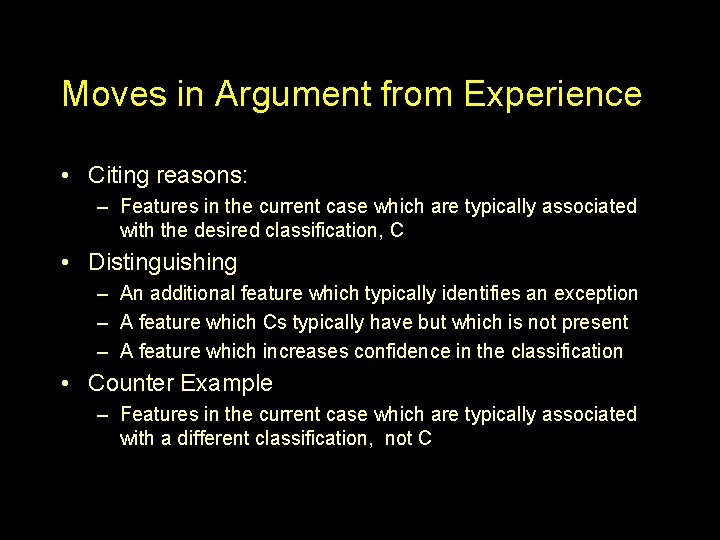
Moves in Argument from Experience • Citing reasons: – Features in the current case which are typically associated with the desired classification, C • Distinguishing – An additional feature which typically identifies an exception – A feature which Cs typically have but which is not present – A feature which increases confidence in the classification • Counter Example – Features in the current case which are typically associated with a different classification, not C
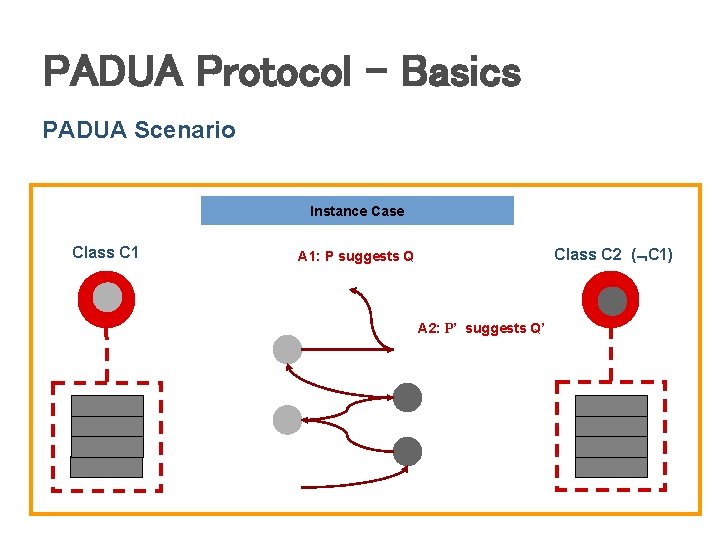
PADUA Protocol - Basics PADUA Scenario Instance Case Class C 1 Class C 2 ( C 1) A 1: P suggests Q A 1 A 2: P’ suggests Q’
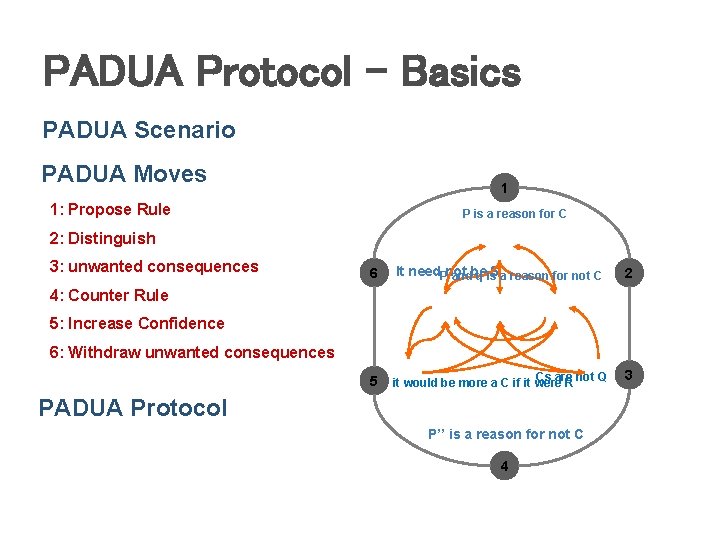
PADUA Protocol - Basics PADUA Scenario PADUA Moves 1 1: Propose Rule P is a reason for C 2: Distinguish 3: unwanted consequences 6 It need. Pnot S a reason for not C andbe q is 2 5 Cs are it would be more a C if it were R not Q 3 4: Counter Rule 5: Increase Confidence 6: Withdraw unwanted consequences PADUA Protocol P’’ is a reason for not C 4
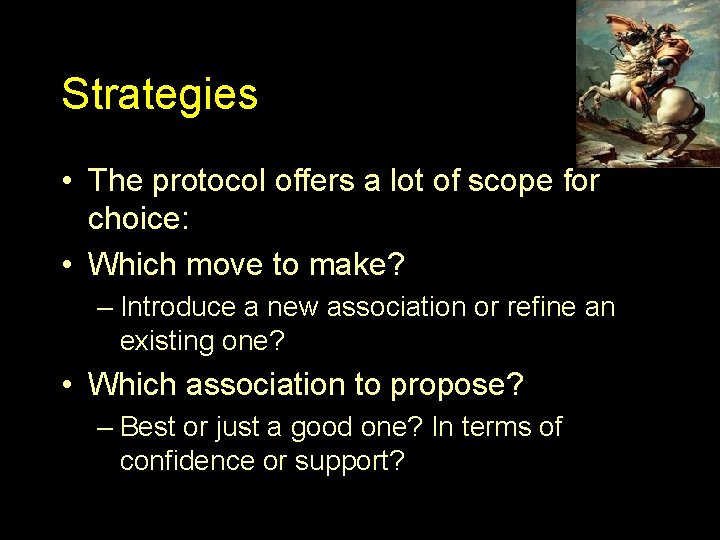
Strategies • The protocol offers a lot of scope for choice: • Which move to make? – Introduce a new association or refine an existing one? • Which association to propose? – Best or just a good one? In terms of confidence or support?
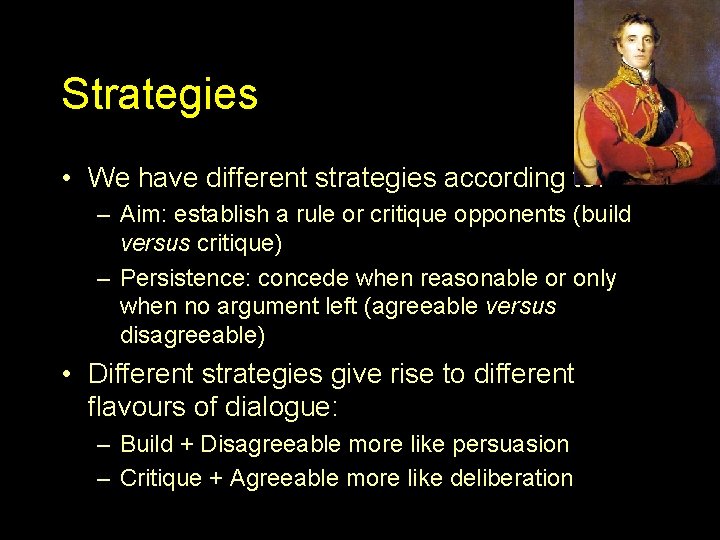
Strategies • We have different strategies according to: – Aim: establish a rule or critique opponents (build versus critique) – Persistence: concede when reasonable or only when no argument left (agreeable versus disagreeable) • Different strategies give rise to different flavours of dialogue: – Build + Disagreeable more like persuasion – Critique + Agreeable more like deliberation
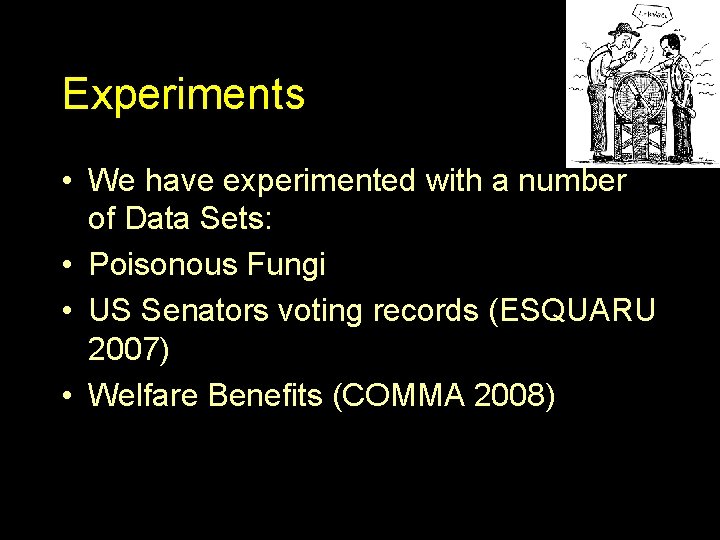
Experiments • We have experimented with a number of Data Sets: • Poisonous Fungi • US Senators voting records (ESQUARU 2007) • Welfare Benefits (COMMA 2008)
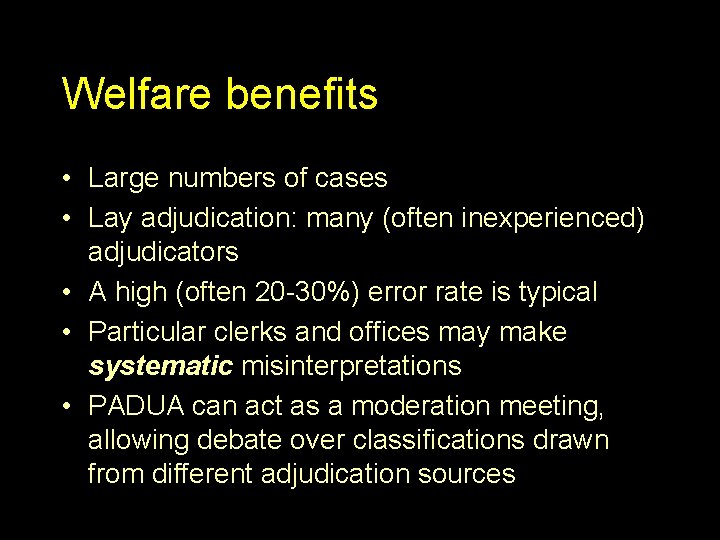
Welfare benefits • Large numbers of cases • Lay adjudication: many (often inexperienced) adjudicators • A high (often 20 -30%) error rate is typical • Particular clerks and offices may make systematic misinterpretations • PADUA can act as a moderation meeting, allowing debate over classifications drawn from different adjudication sources
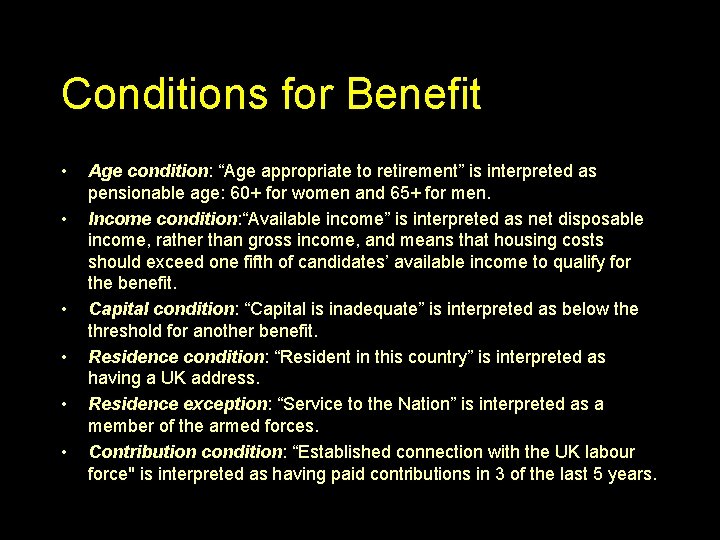
Conditions for Benefit • • • Age condition: “Age appropriate to retirement” is interpreted as pensionable age: 60+ for women and 65+ for men. Income condition: “Available income” is interpreted as net disposable income, rather than gross income, and means that housing costs should exceed one fifth of candidates’ available income to qualify for the benefit. Capital condition: “Capital is inadequate” is interpreted as below the threshold for another benefit. Residence condition: “Resident in this country” is interpreted as having a UK address. Residence exception: “Service to the Nation” is interpreted as a member of the armed forces. Contribution condition: “Established connection with the UK labour force" is interpreted as having paid contributions in 3 of the last 5 years.
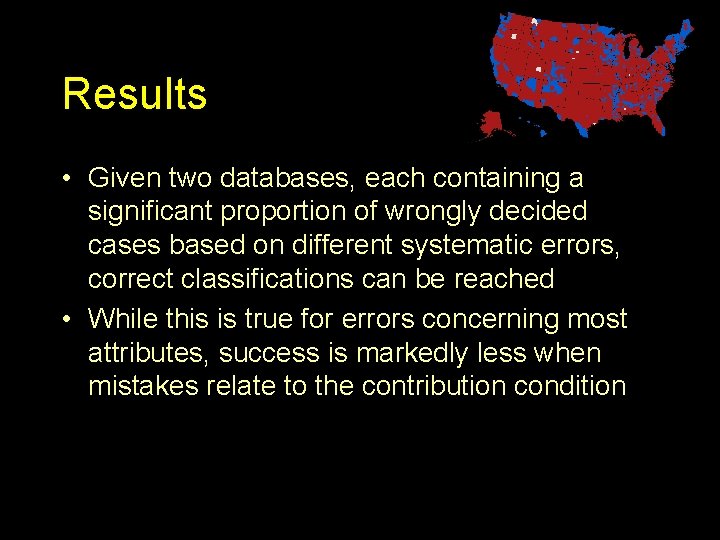
Results • Given two databases, each containing a significant proportion of wrongly decided cases based on different systematic errors, correct classifications can be reached • While this is true for errors concerning most attributes, success is markedly less when mistakes relate to the contribution condition
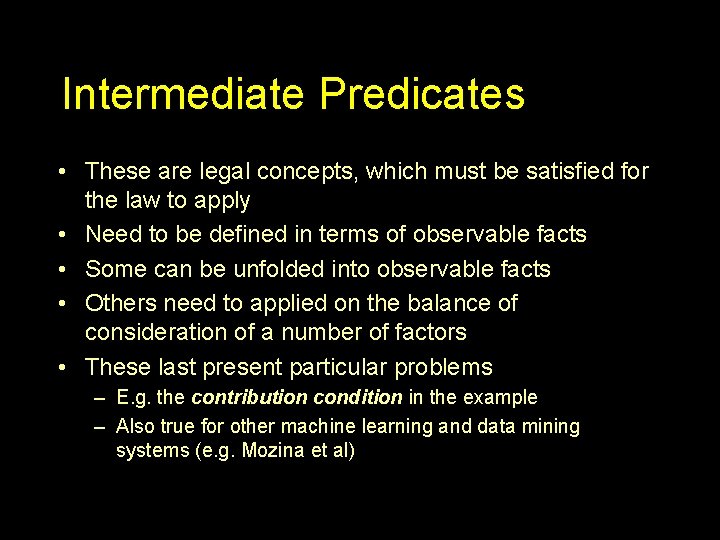
Intermediate Predicates • These are legal concepts, which must be satisfied for the law to apply • Need to be defined in terms of observable facts • Some can be unfolded into observable facts • Others need to applied on the balance of consideration of a number of factors • These last present particular problems – E. g. the contribution condition in the example – Also true for other machine learning and data mining systems (e. g. Mozina et al)
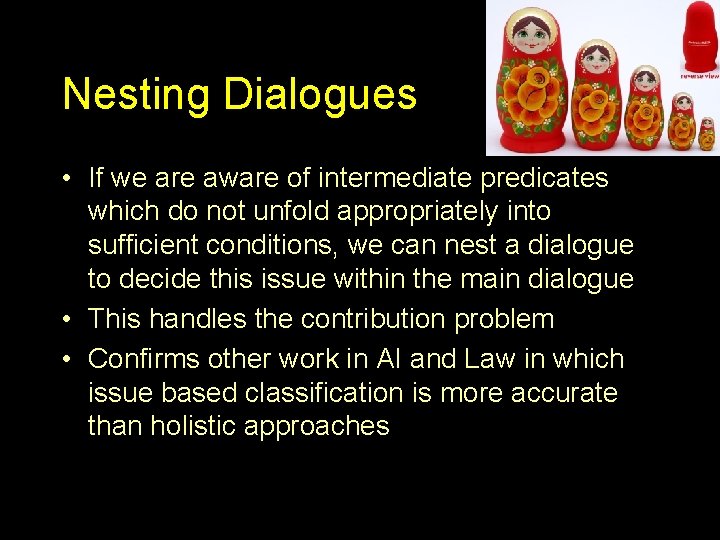
Nesting Dialogues • If we are aware of intermediate predicates which do not unfold appropriately into sufficient conditions, we can nest a dialogue to decide this issue within the main dialogue • This handles the contribution problem • Confirms other work in AI and Law in which issue based classification is more accurate than holistic approaches
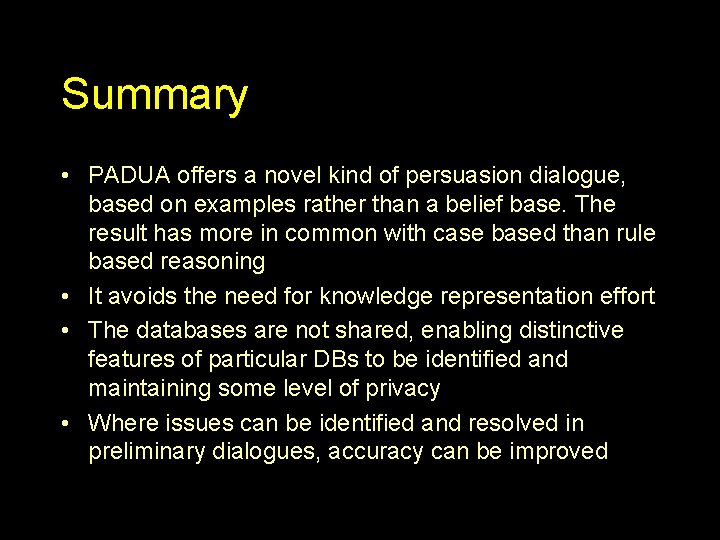
Summary • PADUA offers a novel kind of persuasion dialogue, based on examples rather than a belief base. The result has more in common with case based than rule based reasoning • It avoids the need for knowledge representation effort • The databases are not shared, enabling distinctive features of particular DBs to be identified and maintaining some level of privacy • Where issues can be identified and resolved in preliminary dialogues, accuracy can be improved
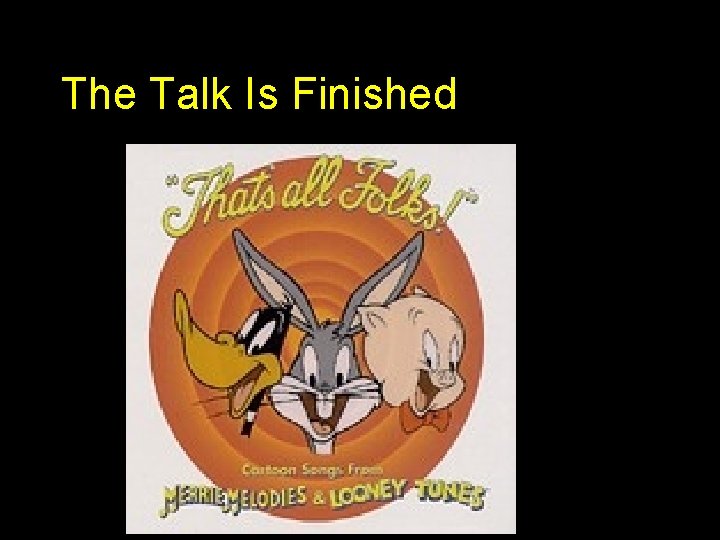
The Talk Is Finished
Maya wardeh
Nightingale health
Padua university medical school
Altar de san antonio de padua donatello
Netclassroom padua franciscan
Cassio de padua mestieri
Escore padua
Who is petruchio in romeo and juliet
What is indirect experience
Experience expectant vs experience dependent
Continuity vs discontinuity
Lời thề hippocrates
Các loại đột biến cấu trúc nhiễm sắc thể
Bổ thể
Vẽ hình chiếu đứng bằng cạnh của vật thể
độ dài liên kết
Môn thể thao bắt đầu bằng chữ đua
Sự nuôi và dạy con của hổ
điện thế nghỉ
Một số thể thơ truyền thống
Thế nào là sự mỏi cơ
Trời xanh đây là của chúng ta thể thơ
Gấu đi như thế nào
Số nguyên tố là