The network approach to psychopathology opportunities for an
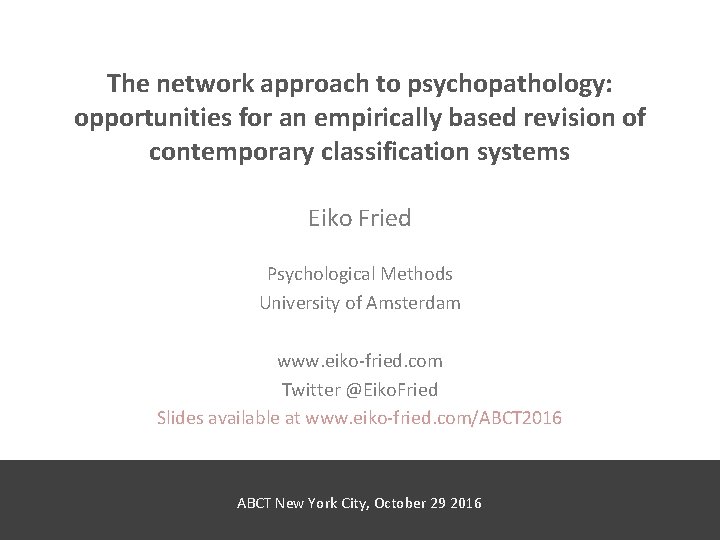
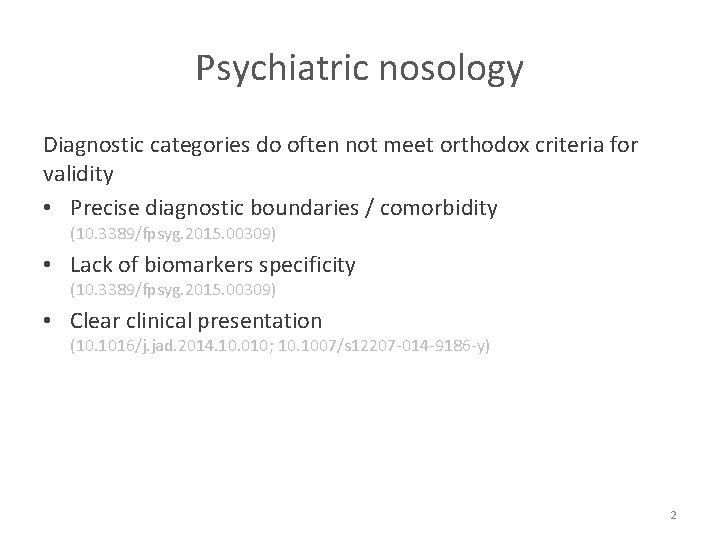
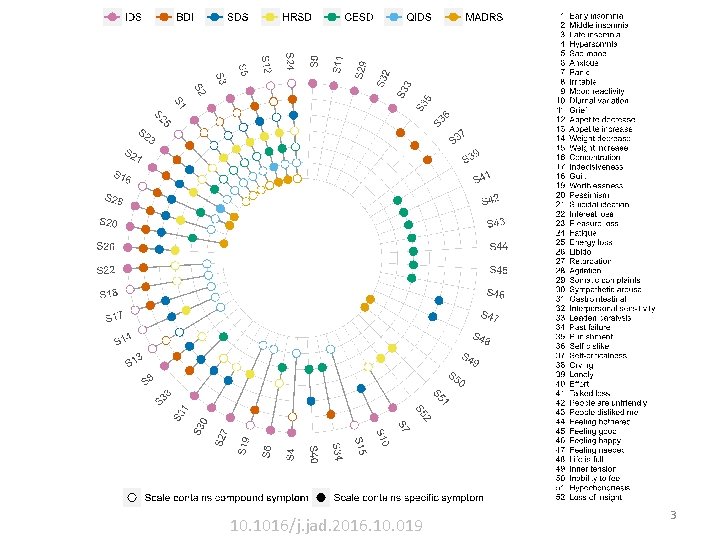
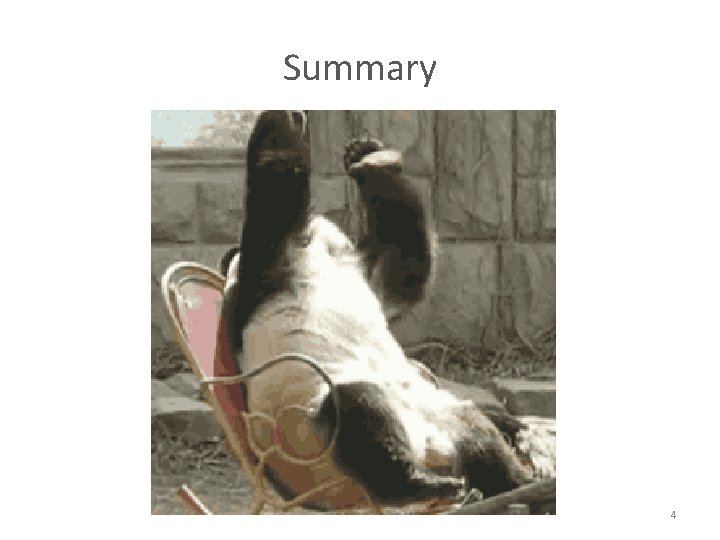
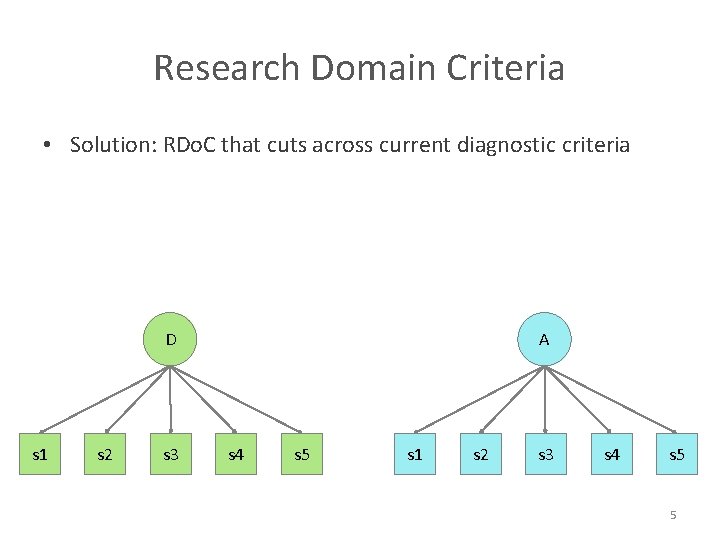
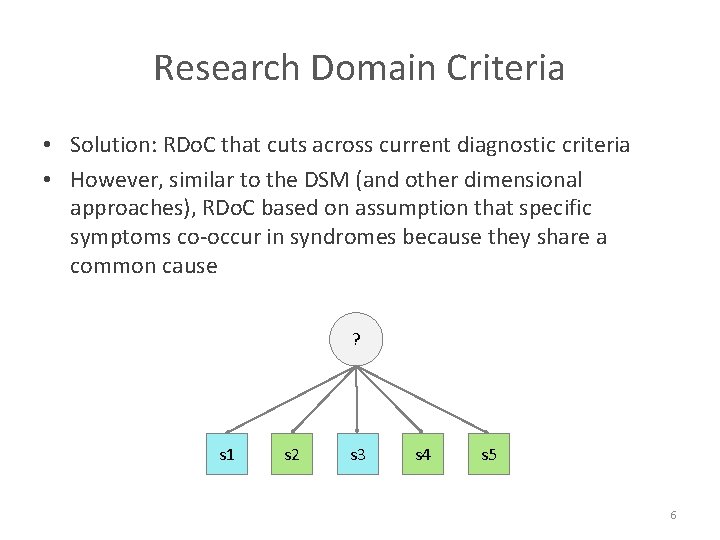
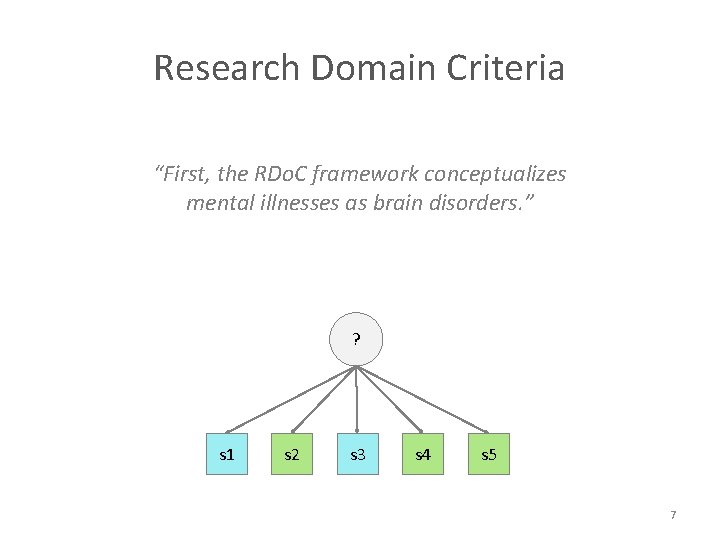
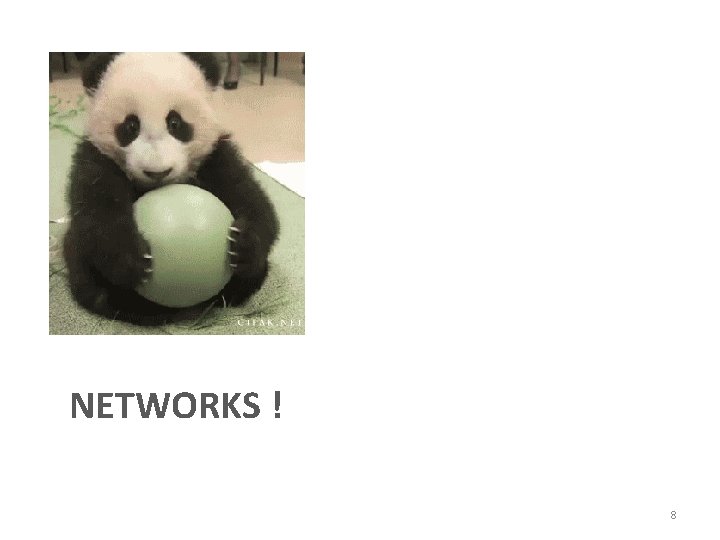
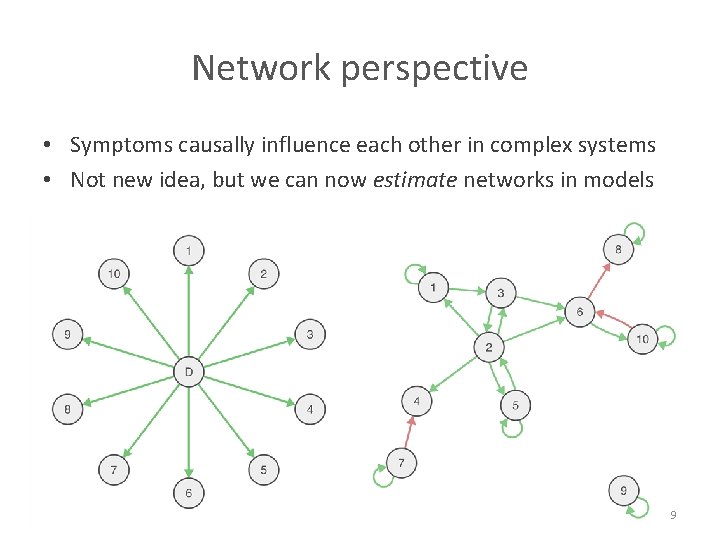
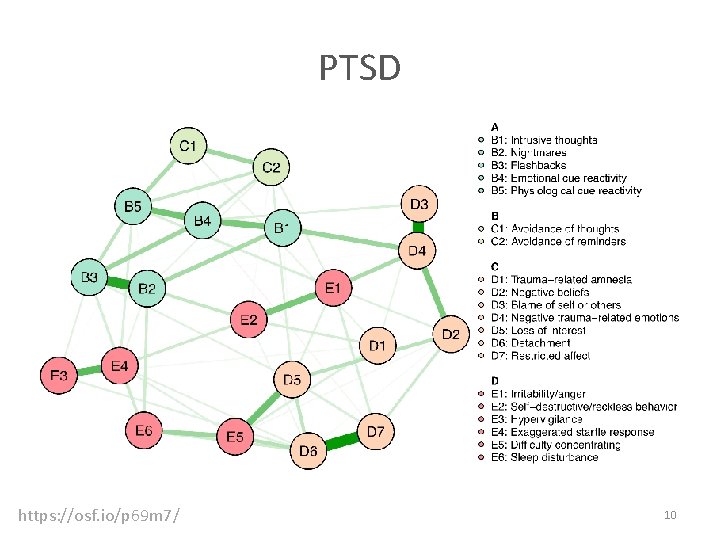
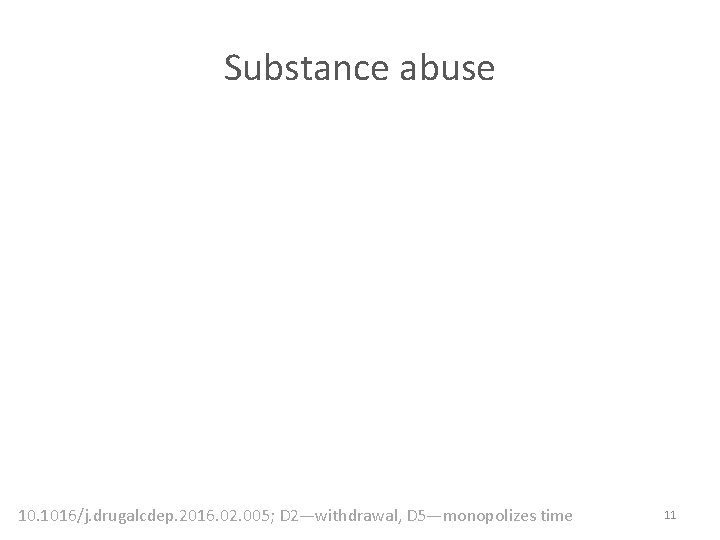
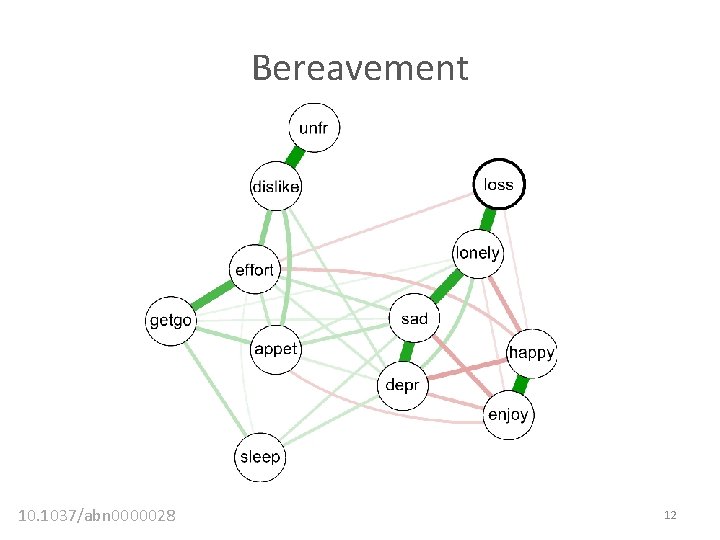
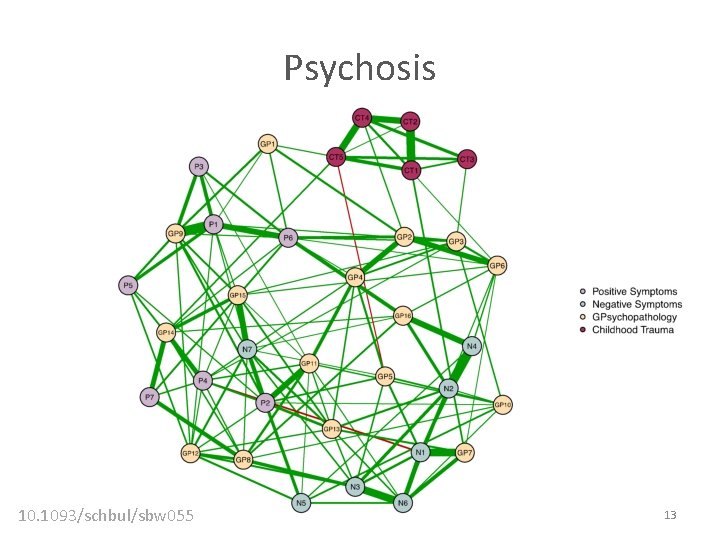
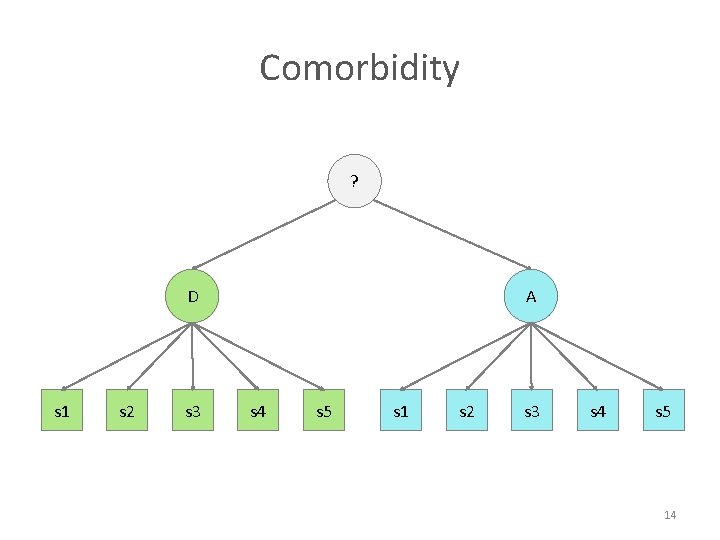
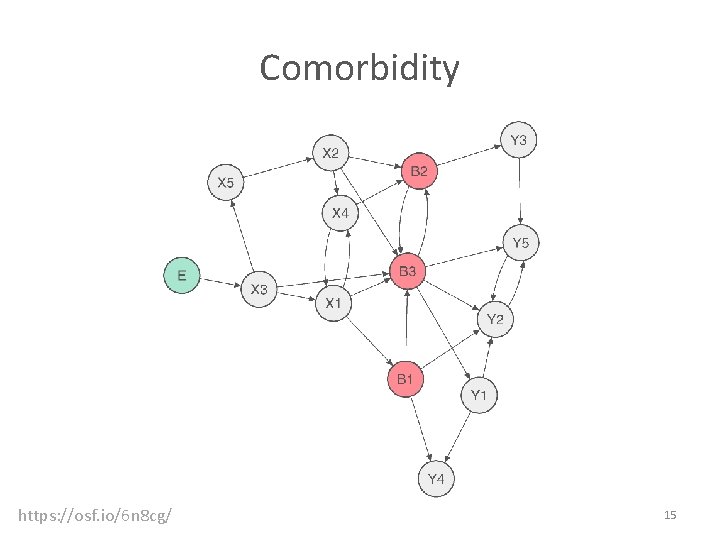
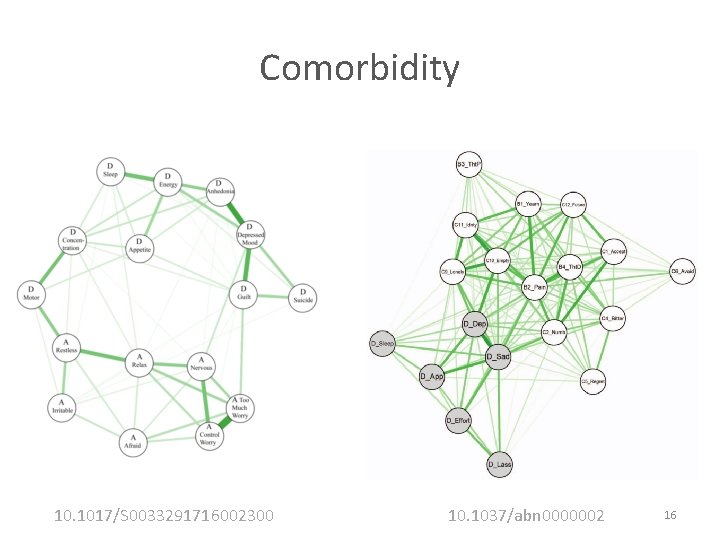
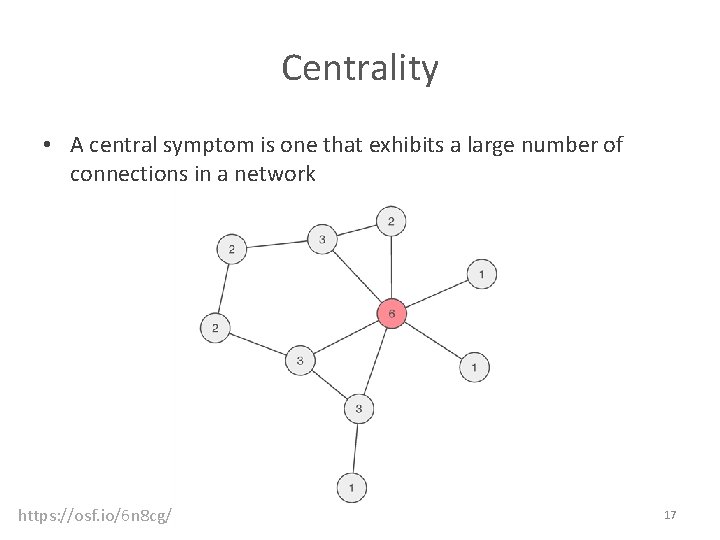
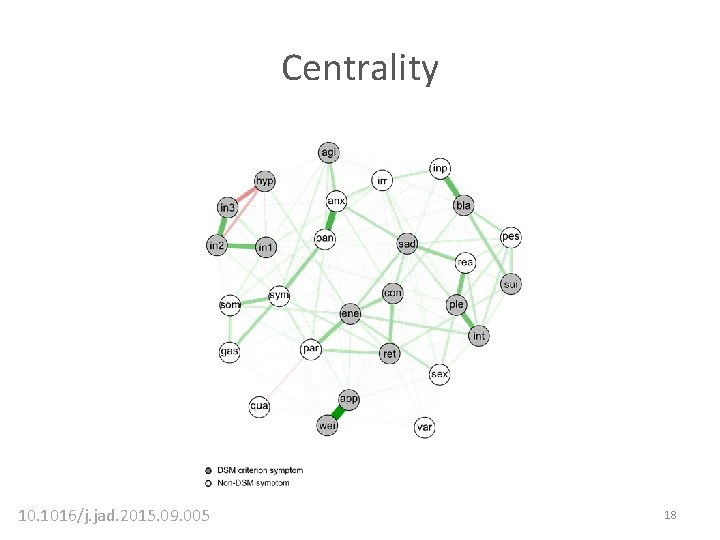
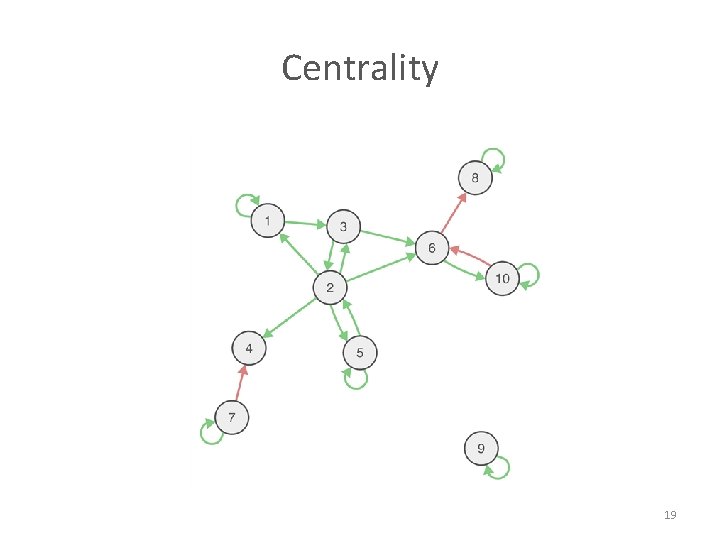
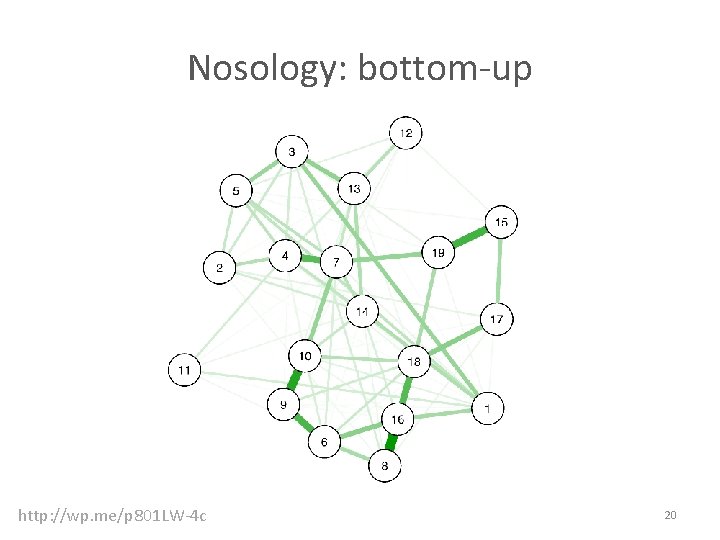
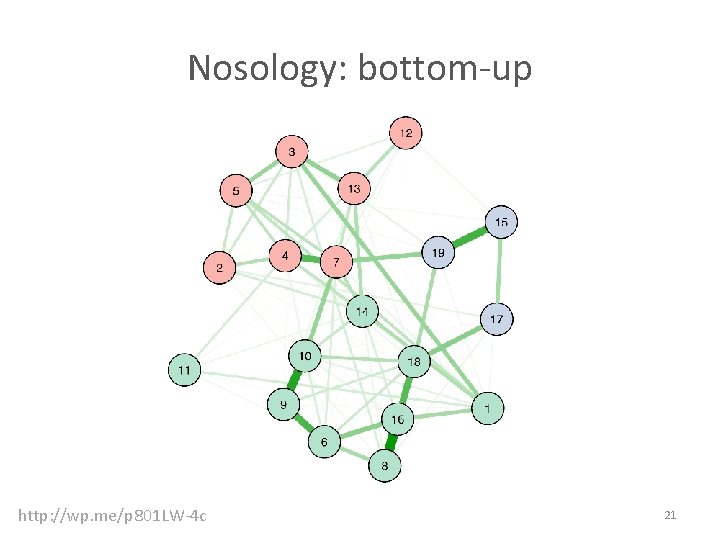
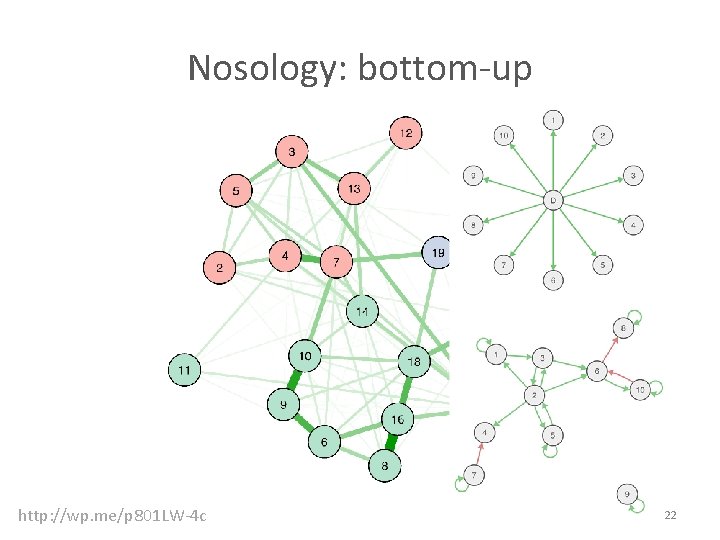
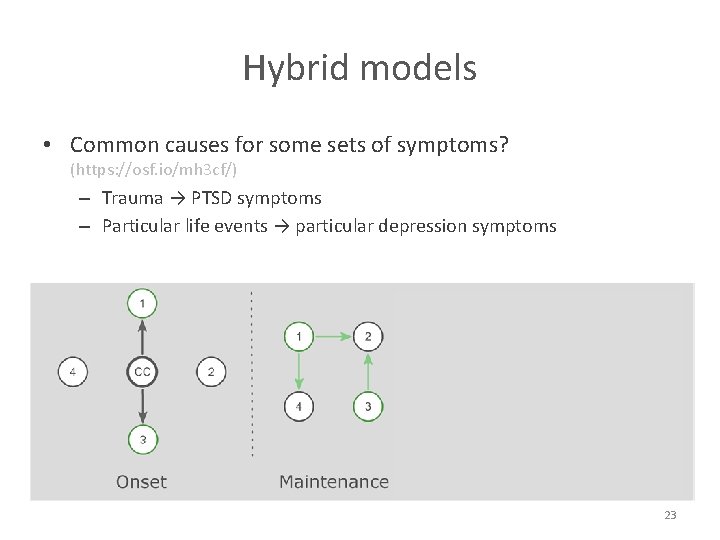
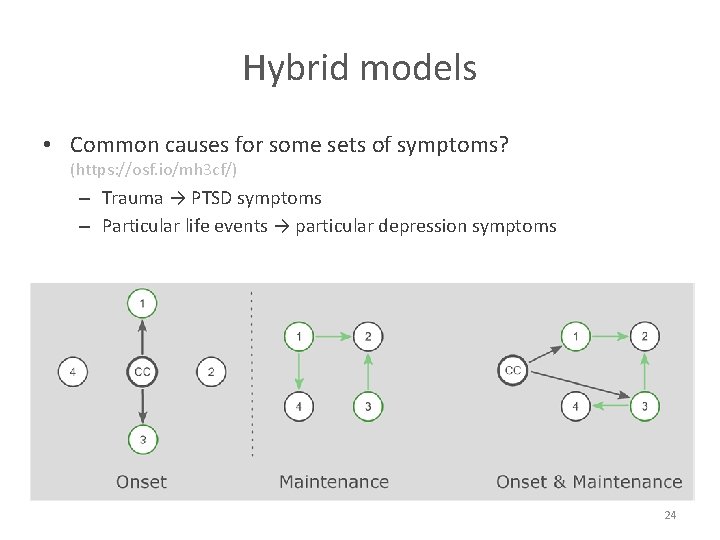
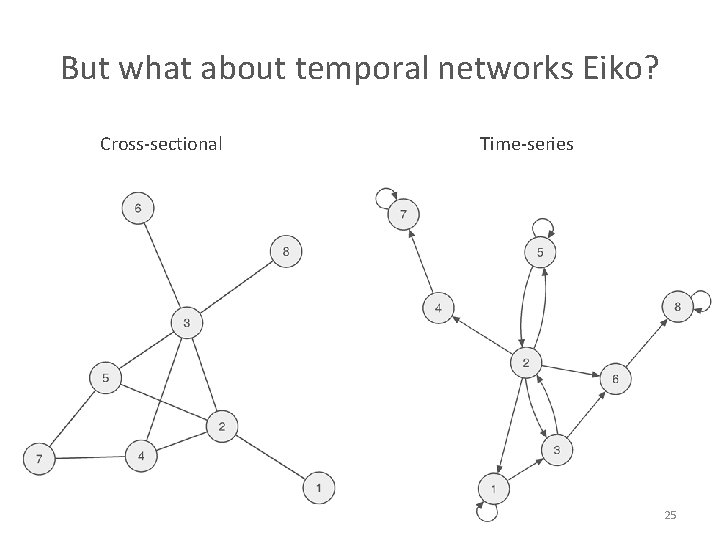
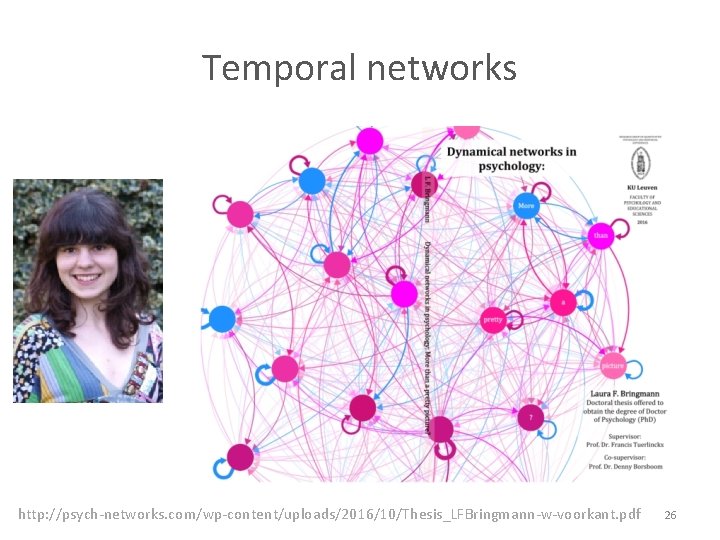
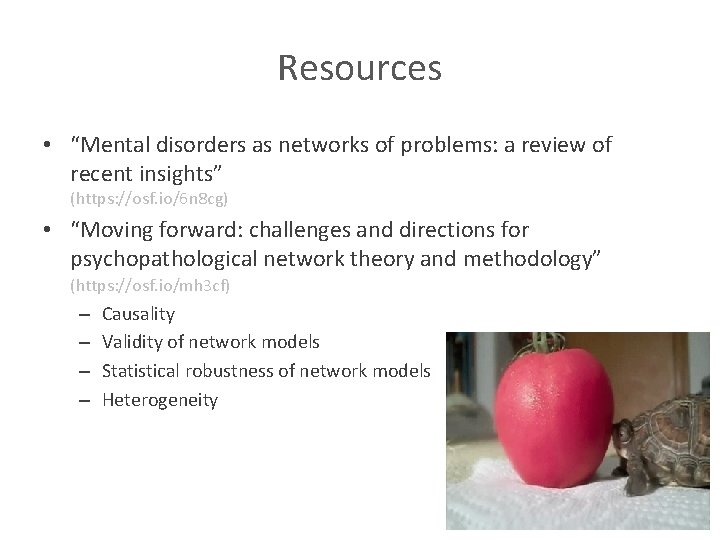
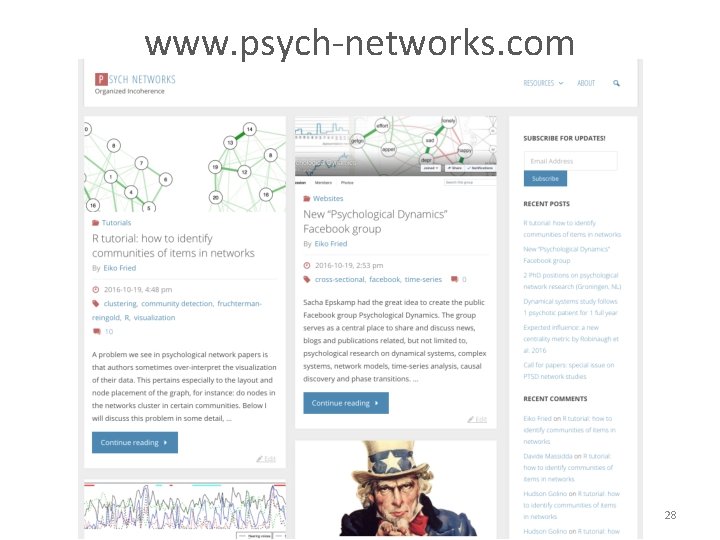
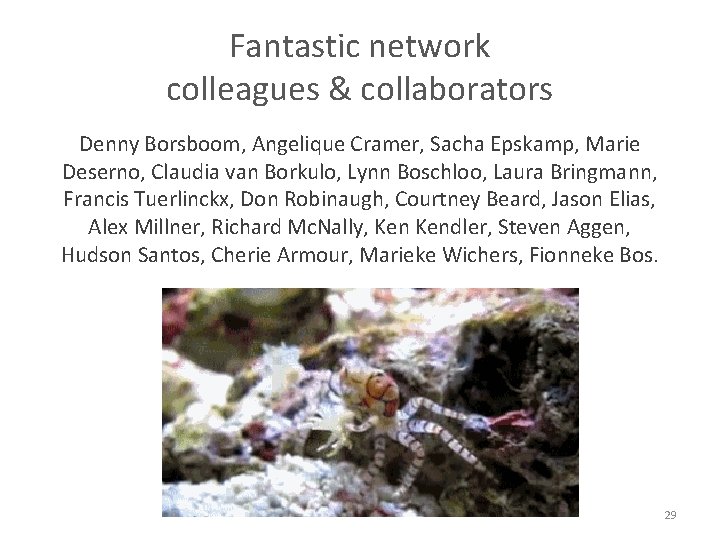
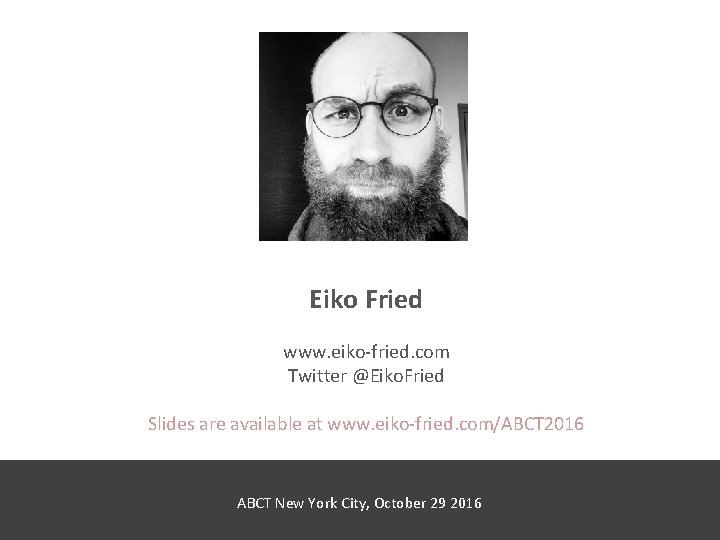
- Slides: 30
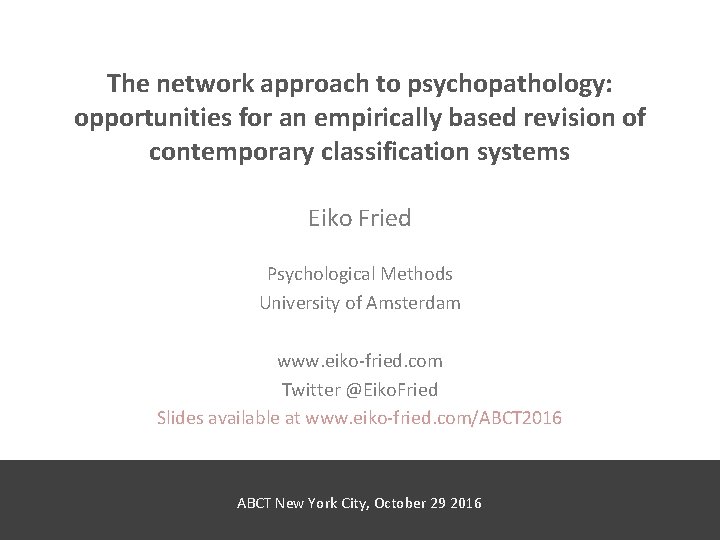
The network approach to psychopathology: opportunities for an empirically based revision of contemporary classification systems Eiko Fried Psychological Methods University of Amsterdam www. eiko-fried. com Twitter @Eiko. Fried Slides available at www. eiko-fried. com/ABCT 2016 ABCT New York City, October 29 2016
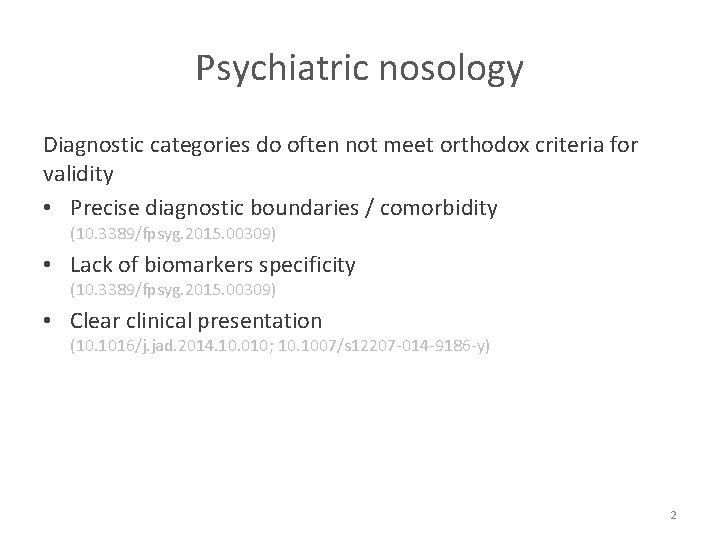
Psychiatric nosology Diagnostic categories do often not meet orthodox criteria for validity • Precise diagnostic boundaries / comorbidity (10. 3389/fpsyg. 2015. 00309) • Lack of biomarkers specificity (10. 3389/fpsyg. 2015. 00309) • Clear clinical presentation (10. 1016/j. jad. 2014. 10. 010; 10. 1007/s 12207 -014 -9186 -y) 2
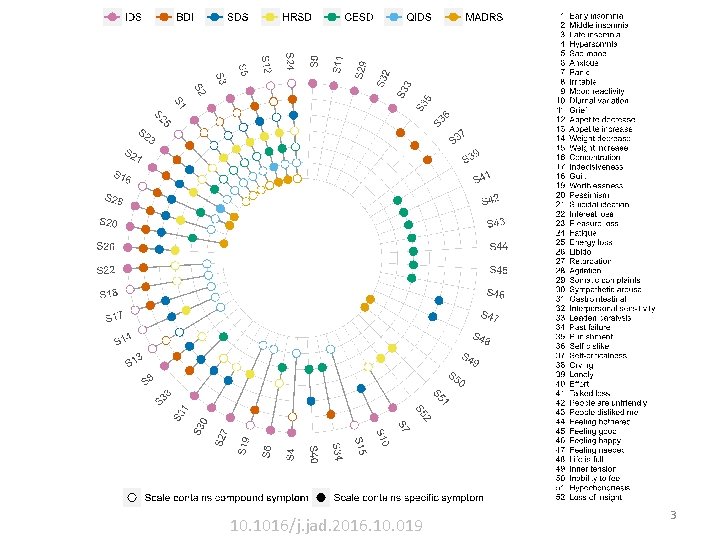
10. 1016/j. jad. 2016. 10. 019 3
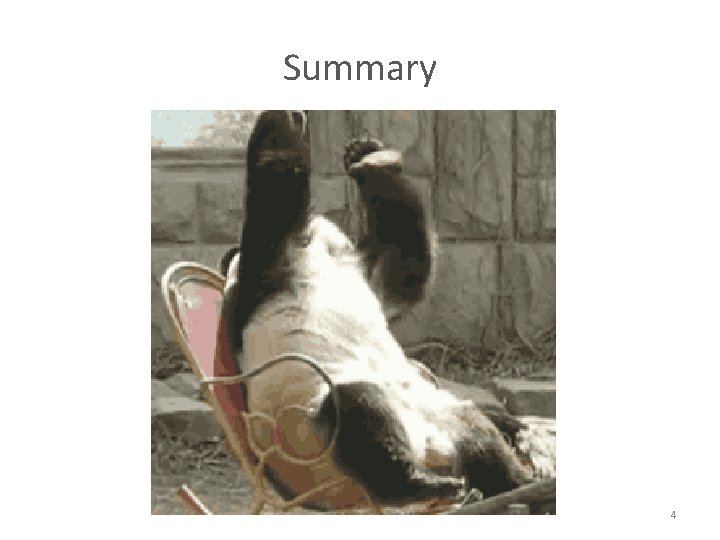
Summary 4
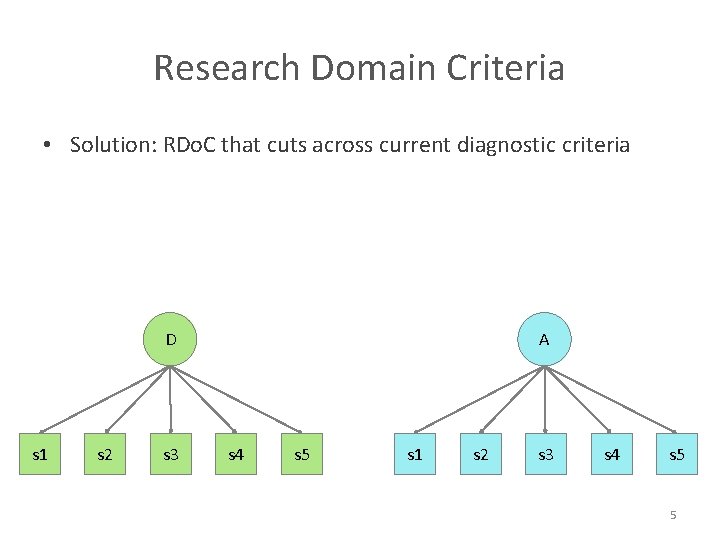
Research Domain Criteria • Solution: RDo. C that cuts across current diagnostic criteria D s 1 s 2 s 3 A s 4 s 5 s 1 s 2 s 3 s 4 s 5 5
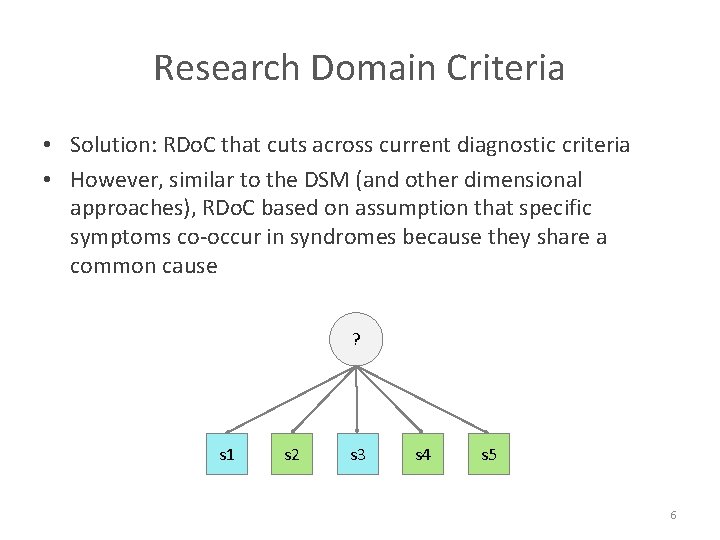
Research Domain Criteria • Solution: RDo. C that cuts across current diagnostic criteria • However, similar to the DSM (and other dimensional approaches), RDo. C based on assumption that specific symptoms co-occur in syndromes because they share a common cause ? s 1 s 2 s 3 s 4 s 5 6
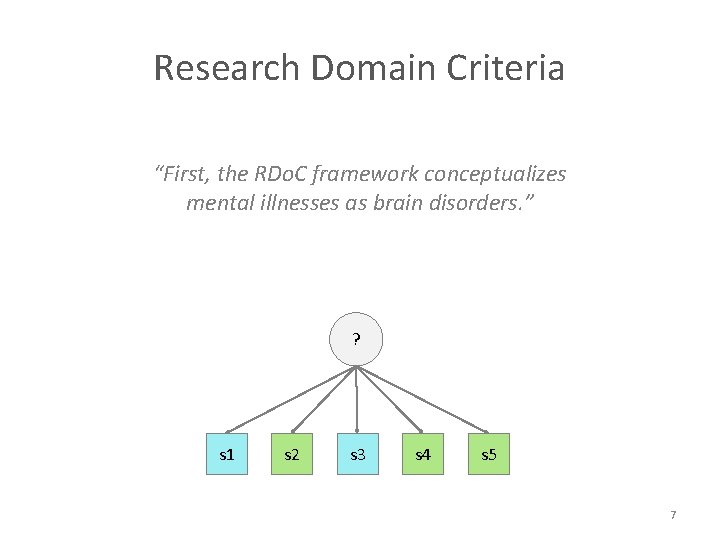
Research Domain Criteria “First, the RDo. C framework conceptualizes mental illnesses as brain disorders. ” ? s 1 s 2 s 3 s 4 s 5 7
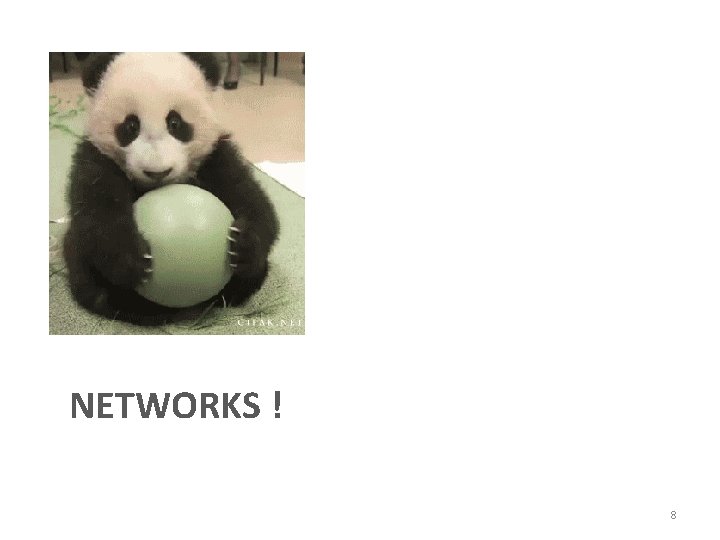
NETWORKS ! 8
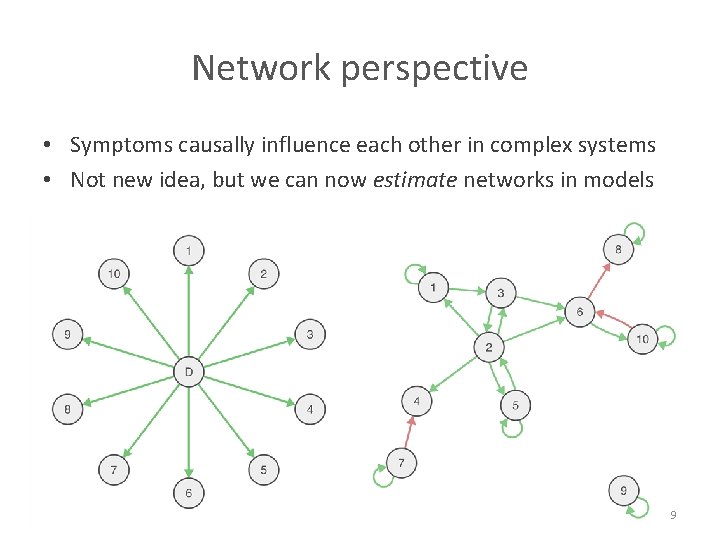
Network perspective • Symptoms causally influence each other in complex systems • Not new idea, but we can now estimate networks in models 9
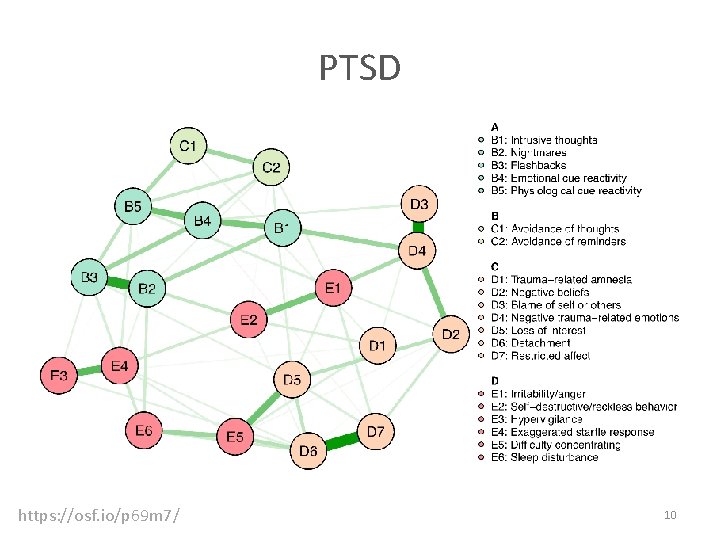
PTSD https: //osf. io/p 69 m 7/ 10
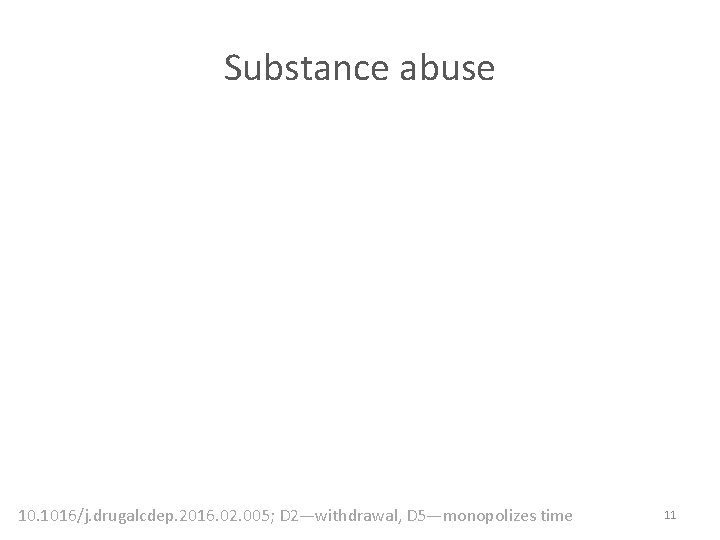
Substance abuse 10. 1016/j. drugalcdep. 2016. 02. 005; D 2—withdrawal, D 5—monopolizes time 11
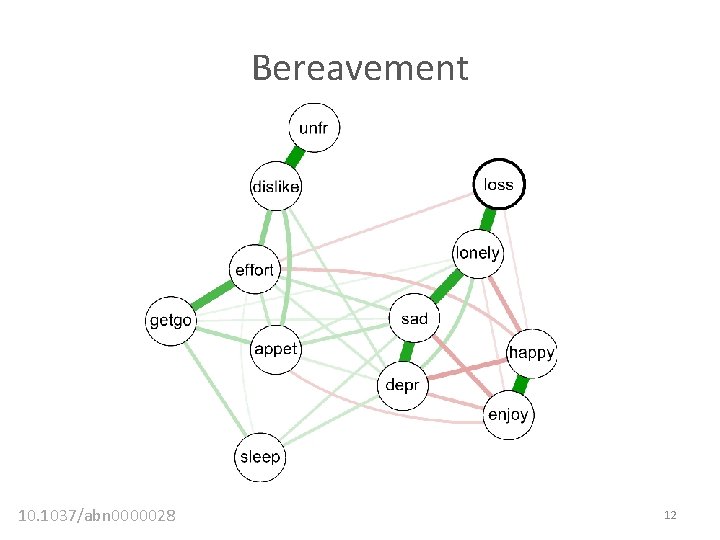
Bereavement 10. 1037/abn 0000028 12
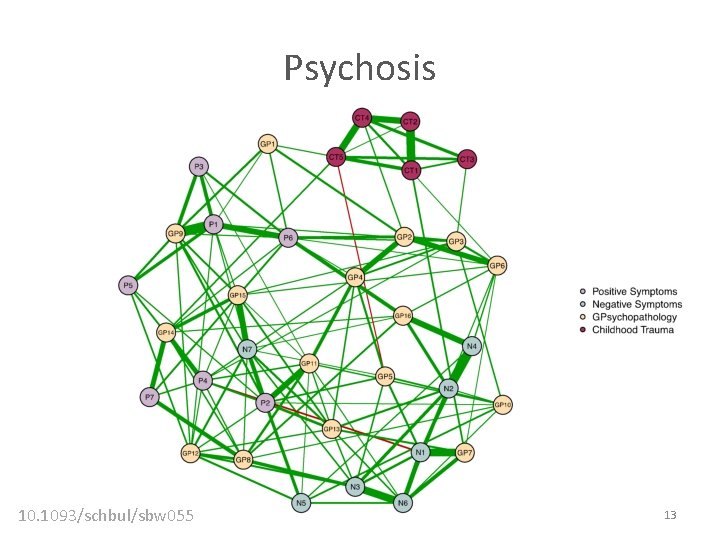
Psychosis 10. 1093/schbul/sbw 055 13
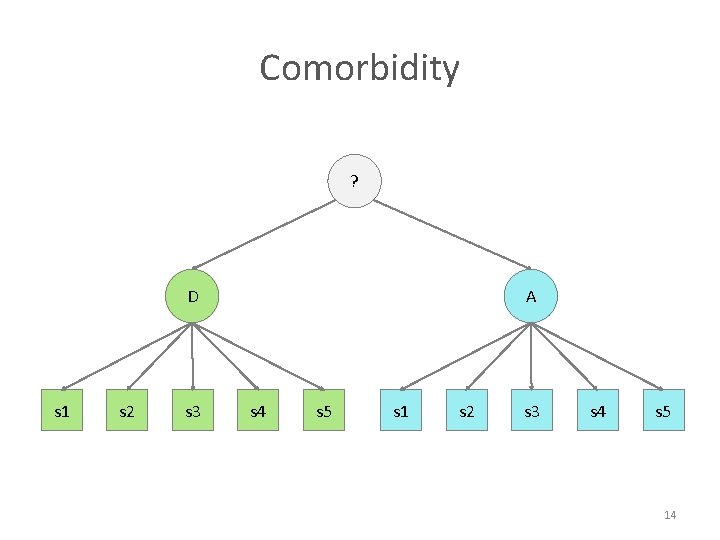
Comorbidity ? D s 1 s 2 s 3 A s 4 s 5 s 1 s 2 s 3 s 4 s 5 14
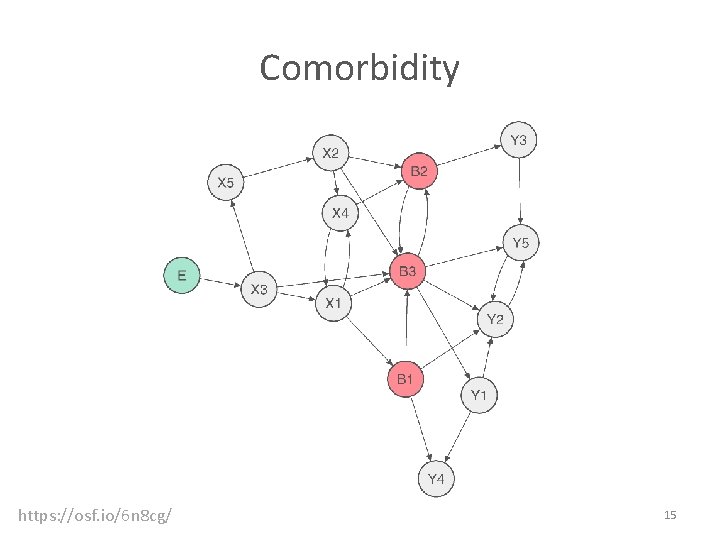
Comorbidity https: //osf. io/6 n 8 cg/ 15
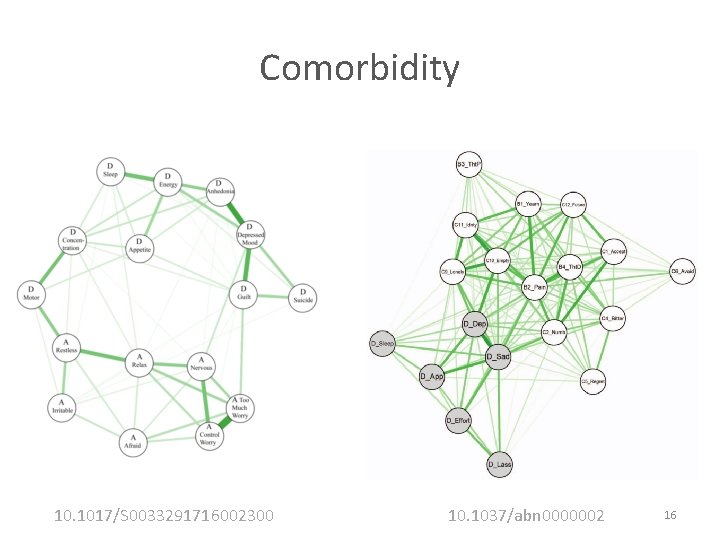
Comorbidity 10. 1017/S 0033291716002300 10. 1037/abn 0000002 16
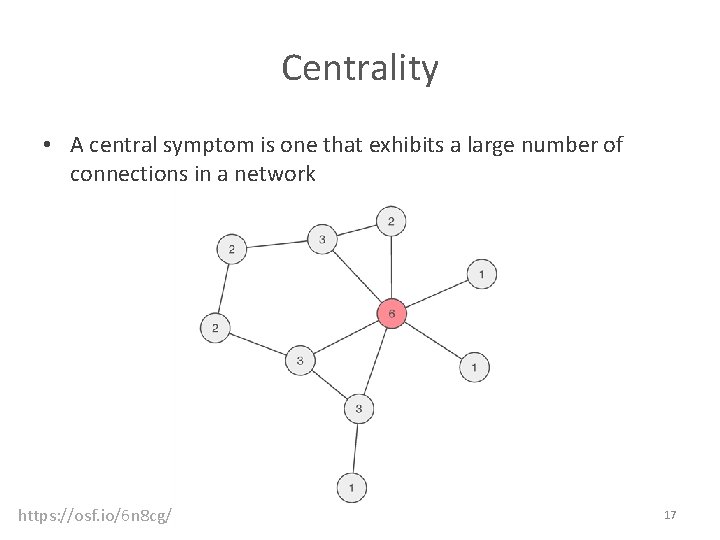
Centrality • A central symptom is one that exhibits a large number of connections in a network https: //osf. io/6 n 8 cg/ 17
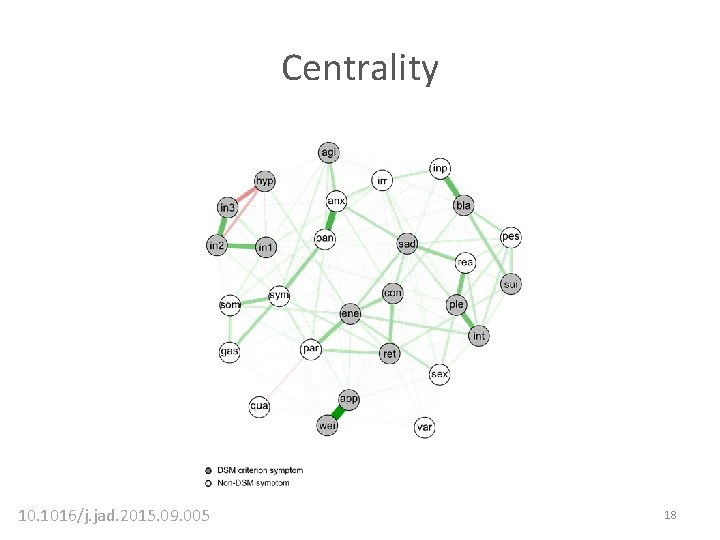
Centrality 10. 1016/j. jad. 2015. 09. 005 18
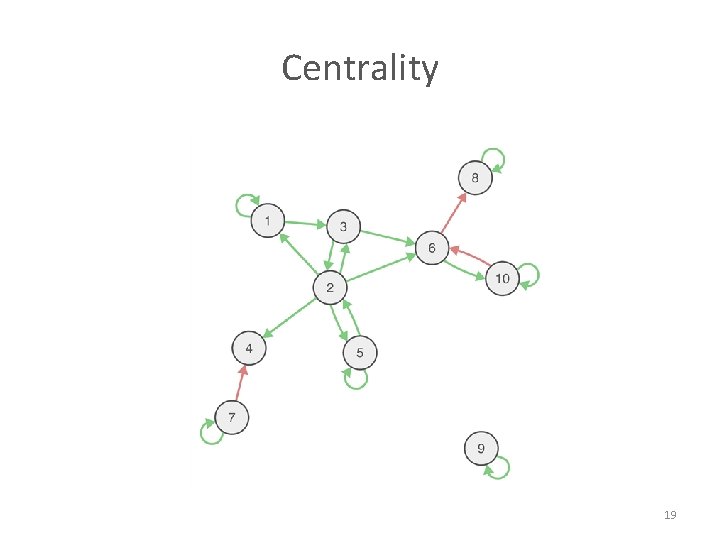
Centrality 19
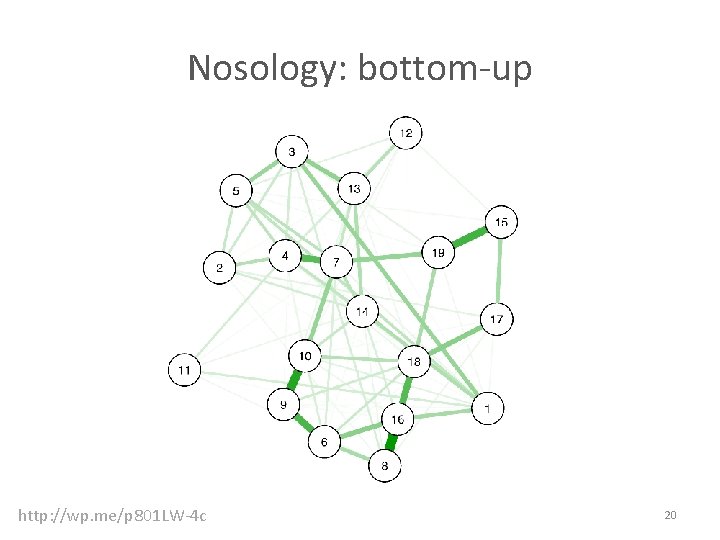
Nosology: bottom-up http: //wp. me/p 801 LW-4 c 20
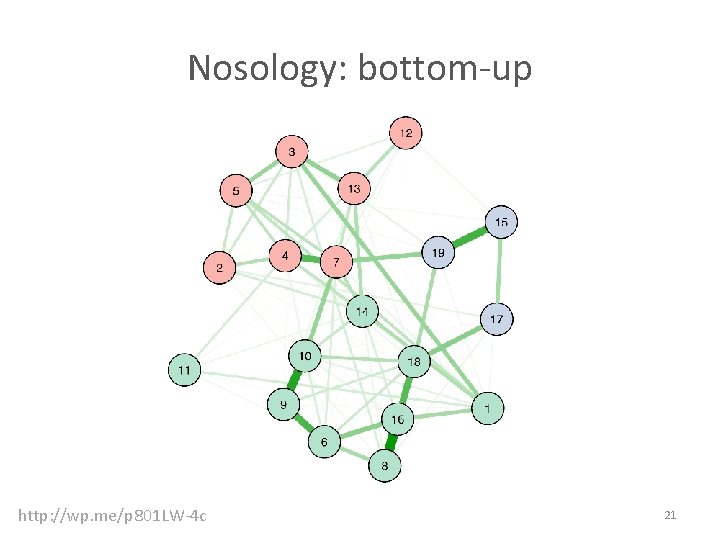
Nosology: bottom-up http: //wp. me/p 801 LW-4 c 21
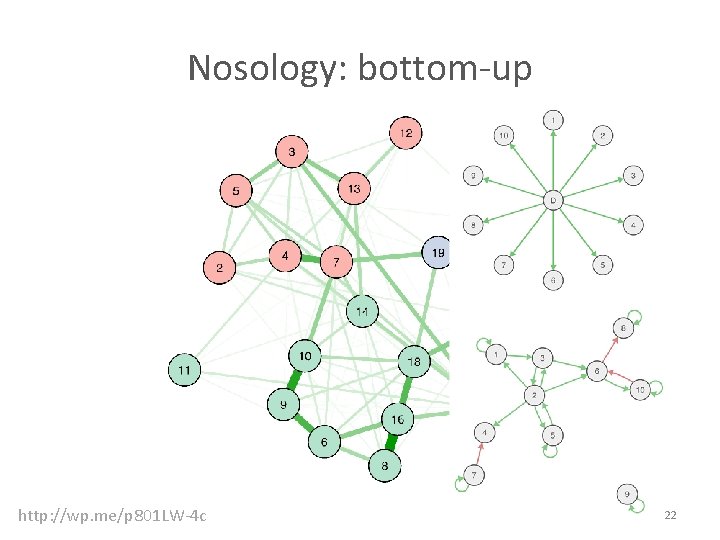
Nosology: bottom-up http: //wp. me/p 801 LW-4 c 22
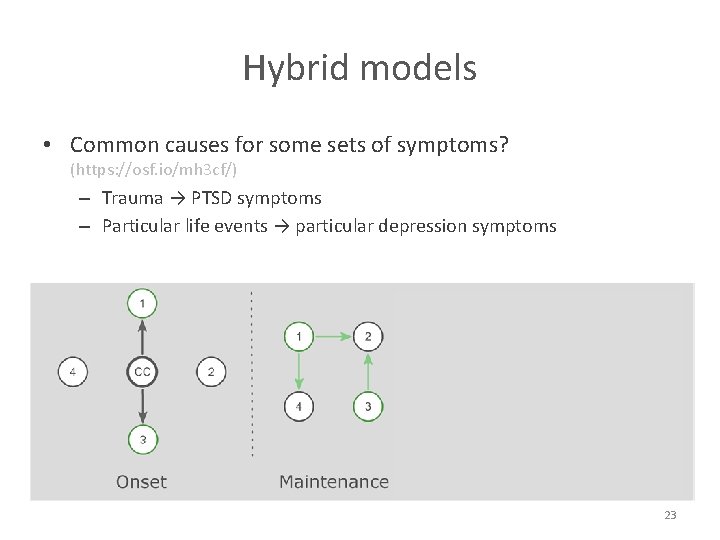
Hybrid models • Common causes for some sets of symptoms? (https: //osf. io/mh 3 cf/) – Trauma → PTSD symptoms – Particular life events → particular depression symptoms 23
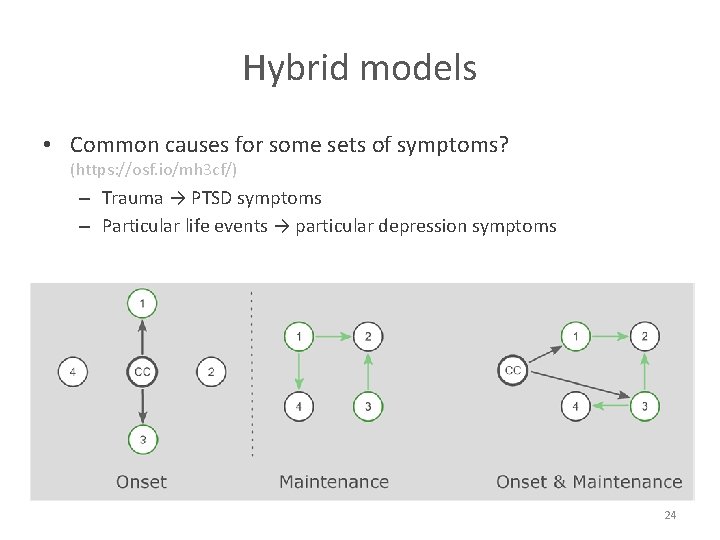
Hybrid models • Common causes for some sets of symptoms? (https: //osf. io/mh 3 cf/) – Trauma → PTSD symptoms – Particular life events → particular depression symptoms 24
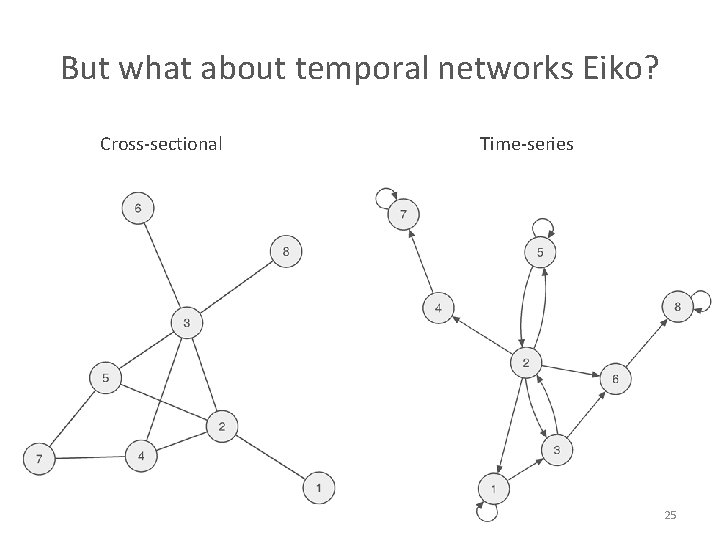
But what about temporal networks Eiko? Cross-sectional Time-series 25
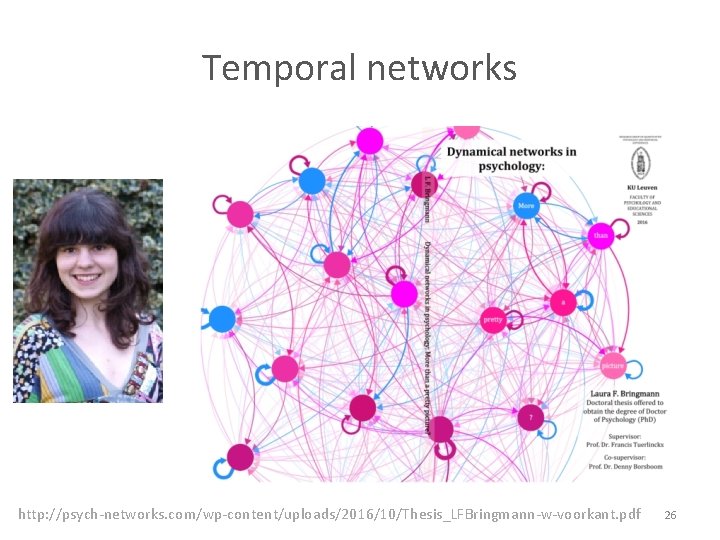
Temporal networks http: //psych-networks. com/wp-content/uploads/2016/10/Thesis_LFBringmann-w-voorkant. pdf 26
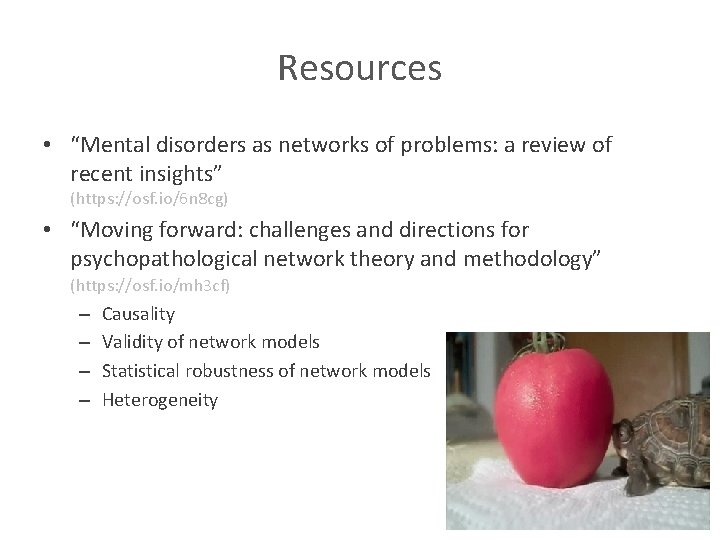
Resources • “Mental disorders as networks of problems: a review of recent insights” (https: //osf. io/6 n 8 cg) • “Moving forward: challenges and directions for psychopathological network theory and methodology” (https: //osf. io/mh 3 cf) – – Causality Validity of network models Statistical robustness of network models Heterogeneity 27
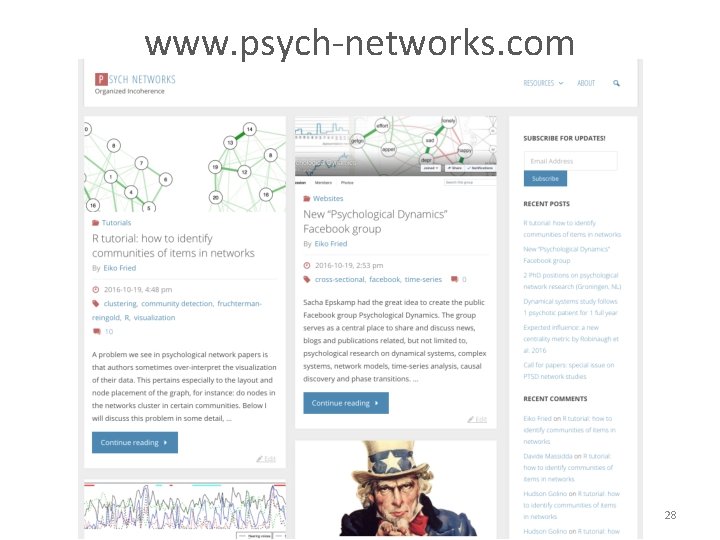
www. psych-networks. com 28
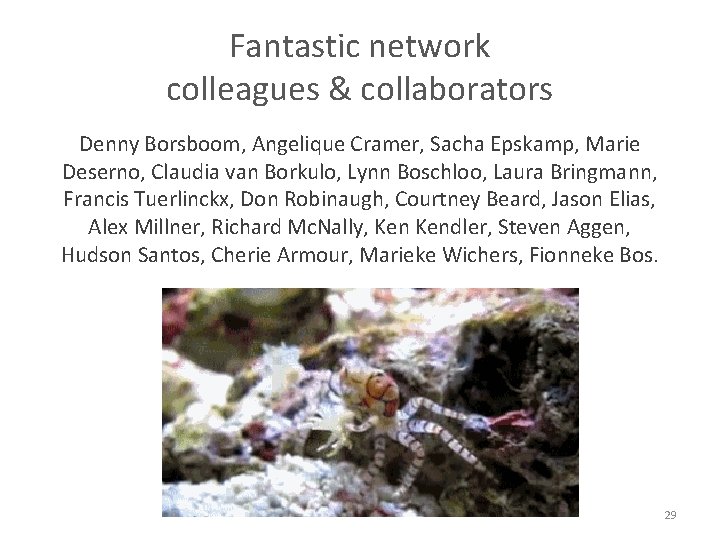
Fantastic network colleagues & collaborators Denny Borsboom, Angelique Cramer, Sacha Epskamp, Marie Deserno, Claudia van Borkulo, Lynn Boschloo, Laura Bringmann, Francis Tuerlinckx, Don Robinaugh, Courtney Beard, Jason Elias, Alex Millner, Richard Mc. Nally, Kendler, Steven Aggen, Hudson Santos, Cherie Armour, Marieke Wichers, Fionneke Bos. 29
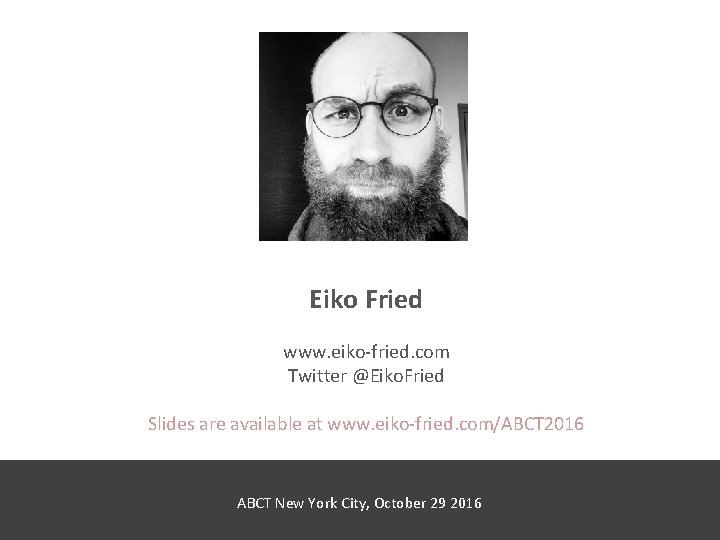
Eiko Fried www. eiko-fried. com Twitter @Eiko. Fried Slides are available at www. eiko-fried. com/ABCT 2016 ABCT New York City, October 29 2016
Multidimensional integrative approach
Developmental psychopathology approach
Integrative approach to psychopathology
Biological paradigm of psychopathology
Multipath model of psychopathology
What is psychopathology
Bacteria causing syphilis
Speech errors examples
Psychopathology
Kontinuitetshantering i praktiken
Typiska drag för en novell
Tack för att ni lyssnade bild
Ekologiskt fotavtryck
Varför kallas perioden 1918-1939 för mellankrigstiden
En lathund för arbete med kontinuitetshantering
Särskild löneskatt för pensionskostnader
Vilotidsbok
Sura för anatom
Vad är densitet
Datorkunskap för nybörjare
Tack för att ni lyssnade bild
Mall för debattartikel
Magnetsjukhus
Nyckelkompetenser för livslångt lärande
Påbyggnader för flakfordon
Arkimedes princip formel
Svenskt ramverk för digital samverkan
Lyckans minut erik lindorm analys
Presentera för publik crossboss
Argument för teckenspråk som minoritetsspråk
Kanaans land