The National Technical University of Athens QSAR Group
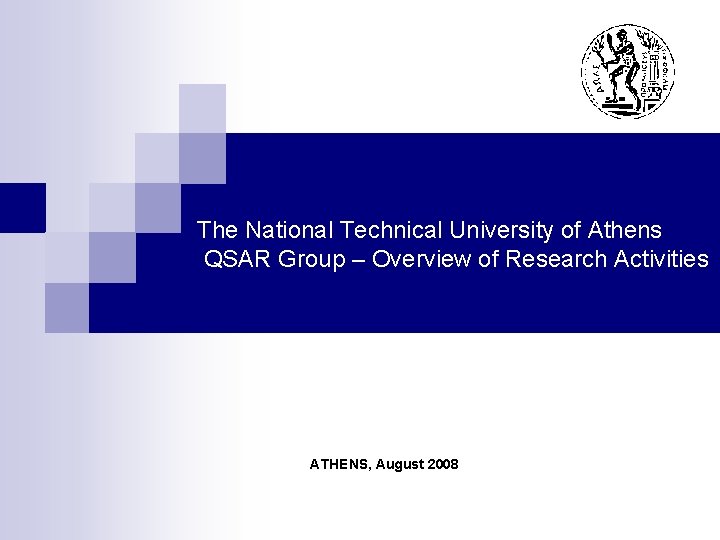
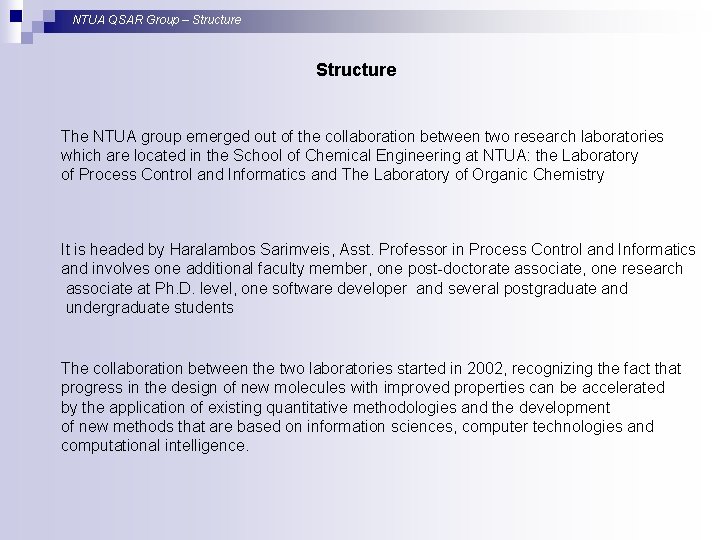
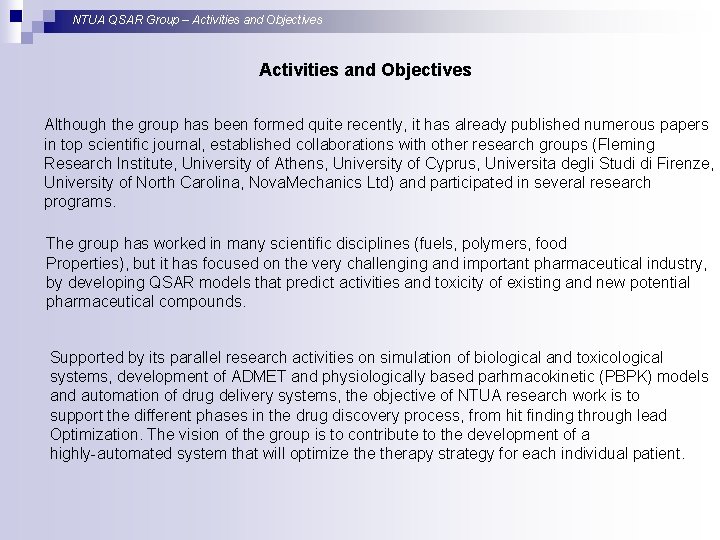
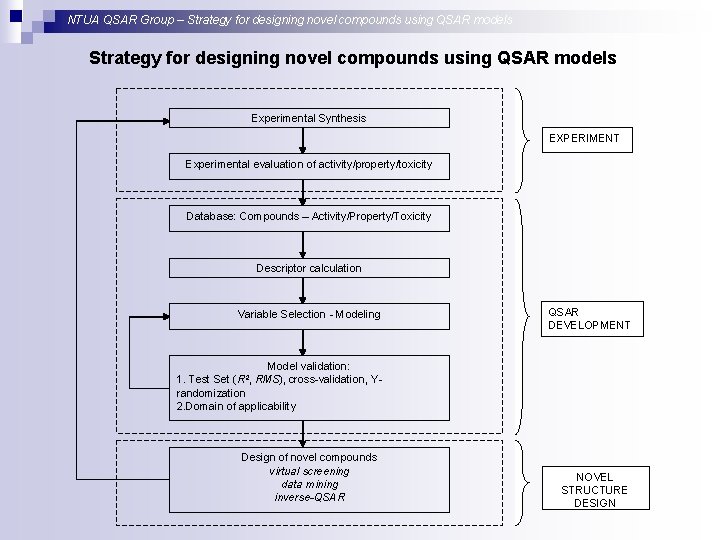
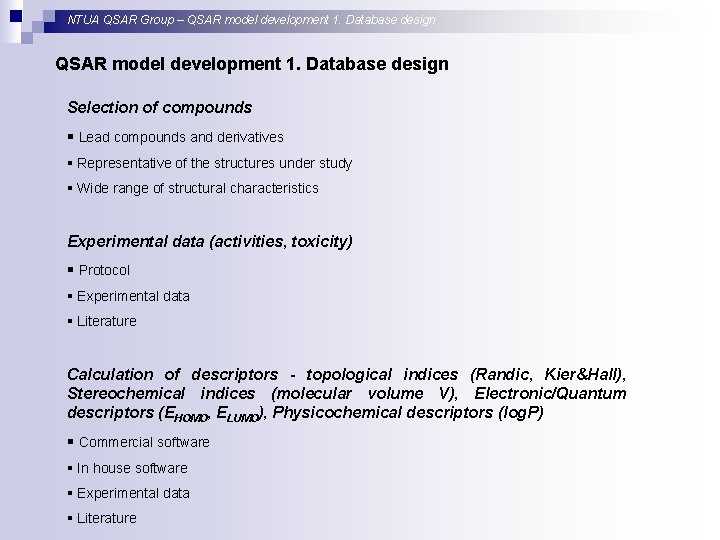
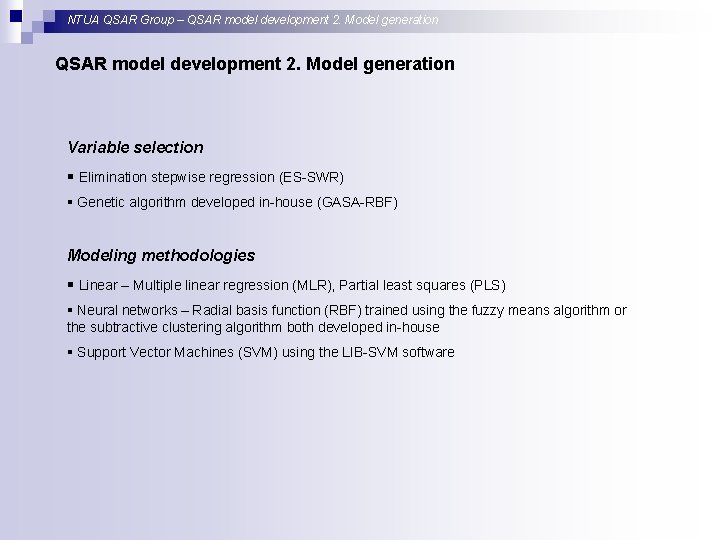
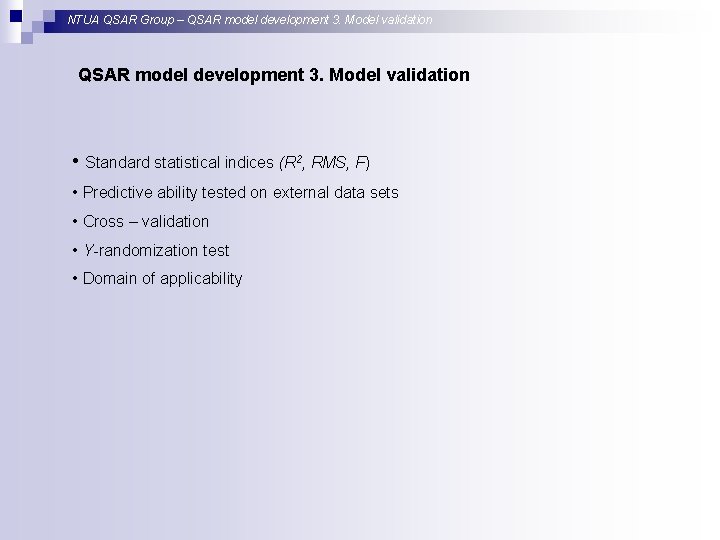
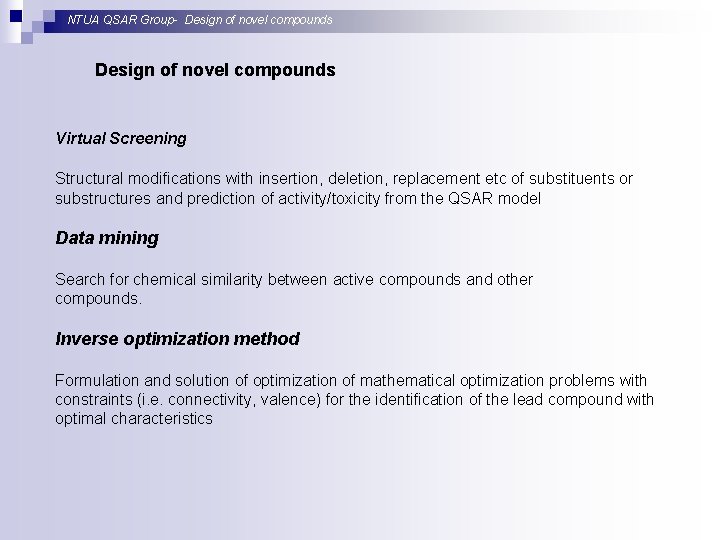
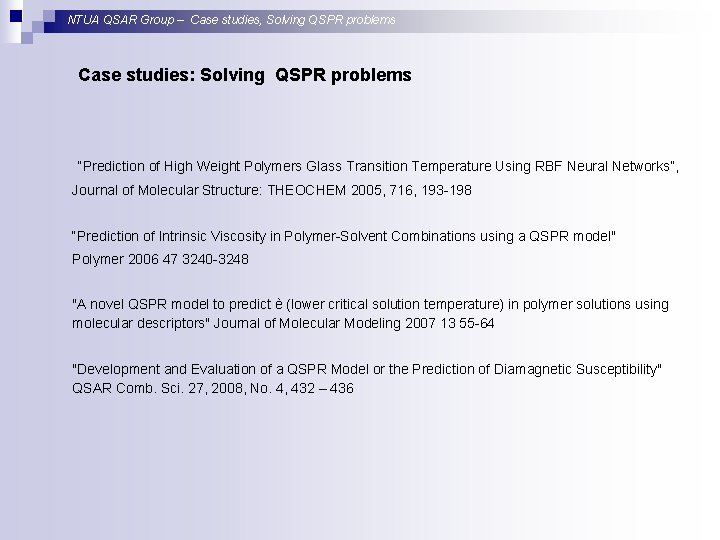
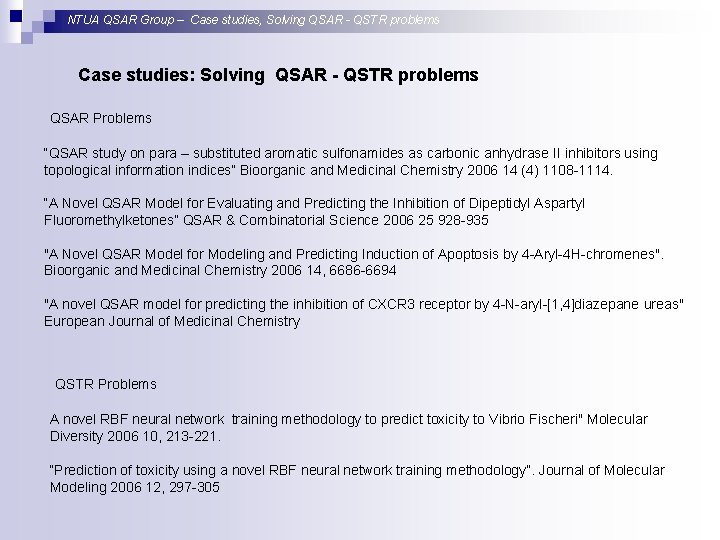
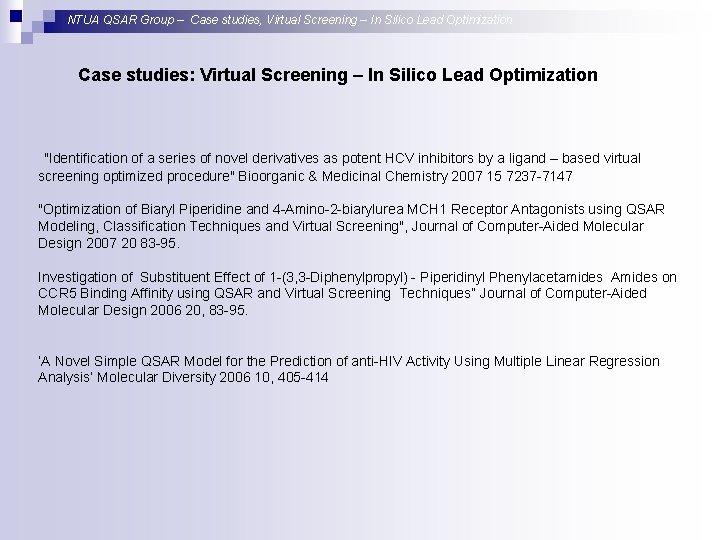
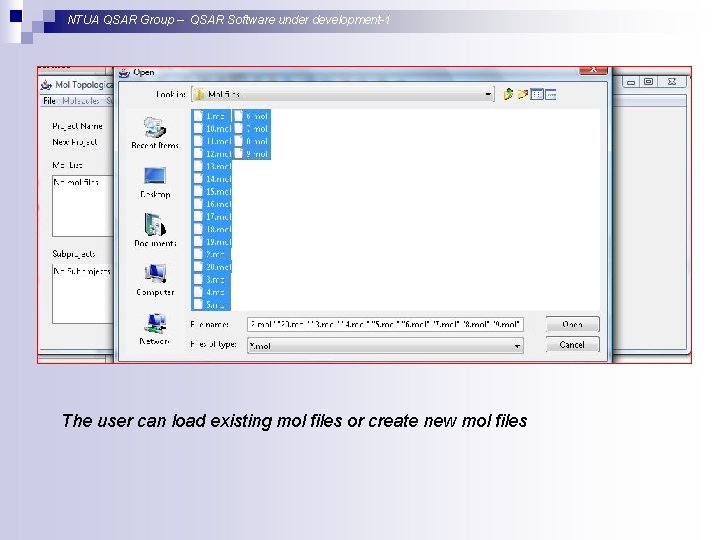
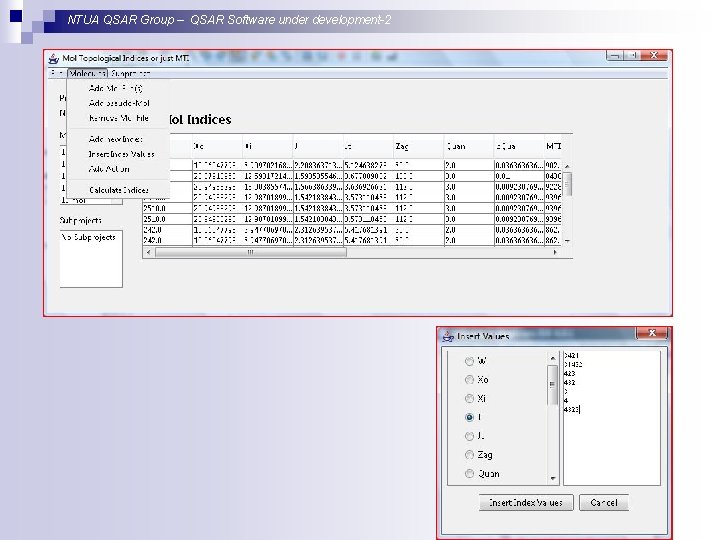
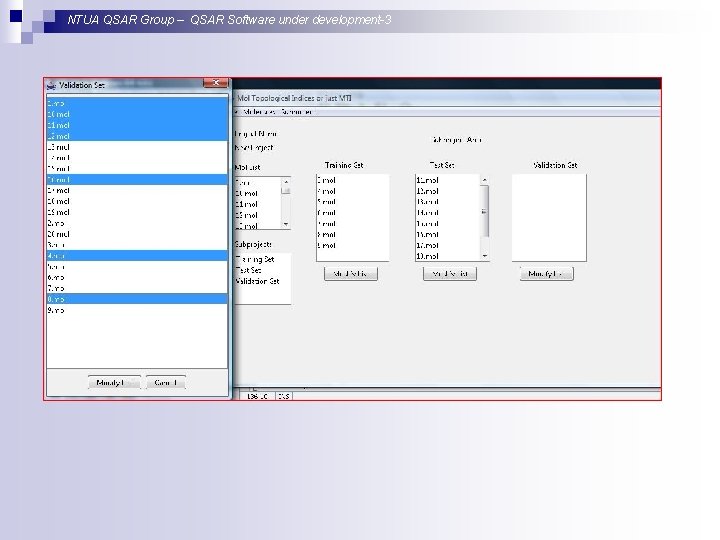
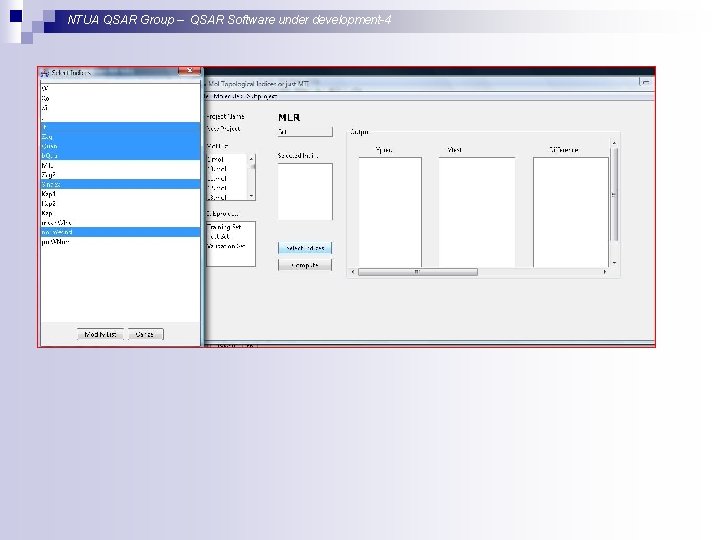
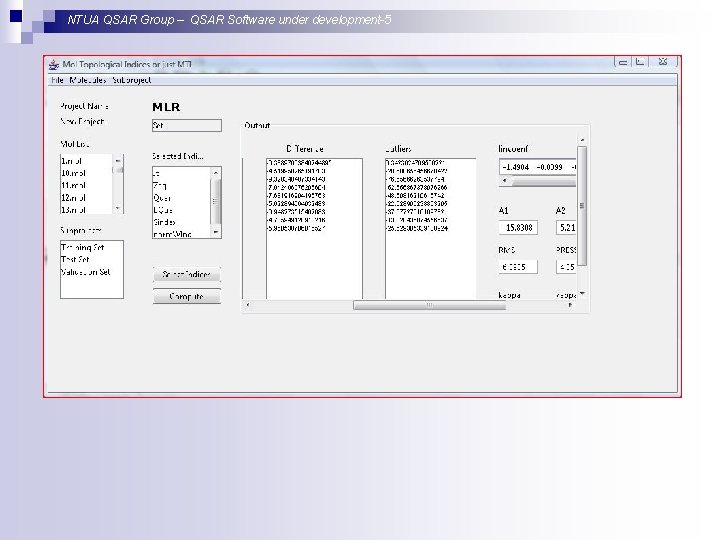
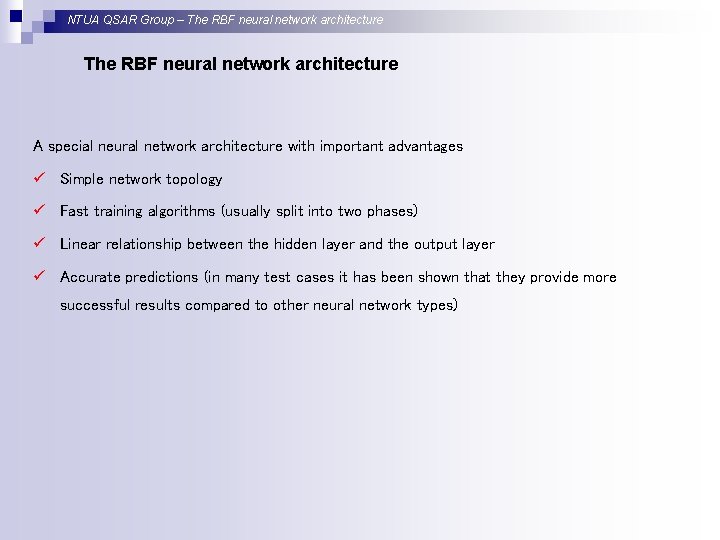
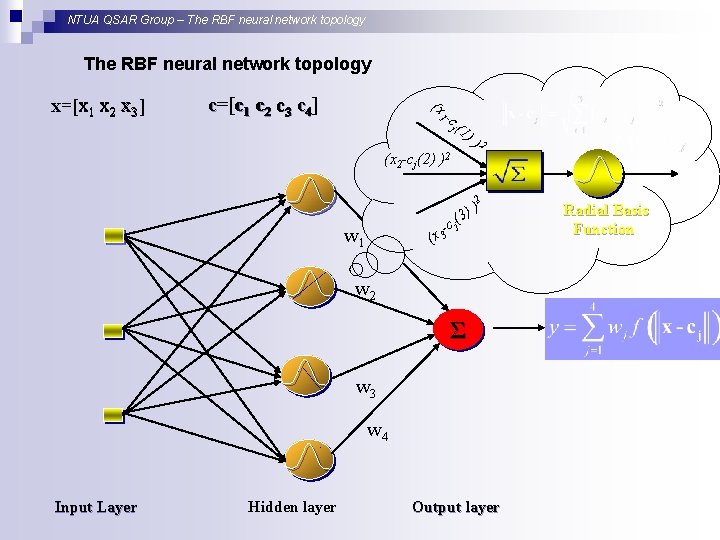
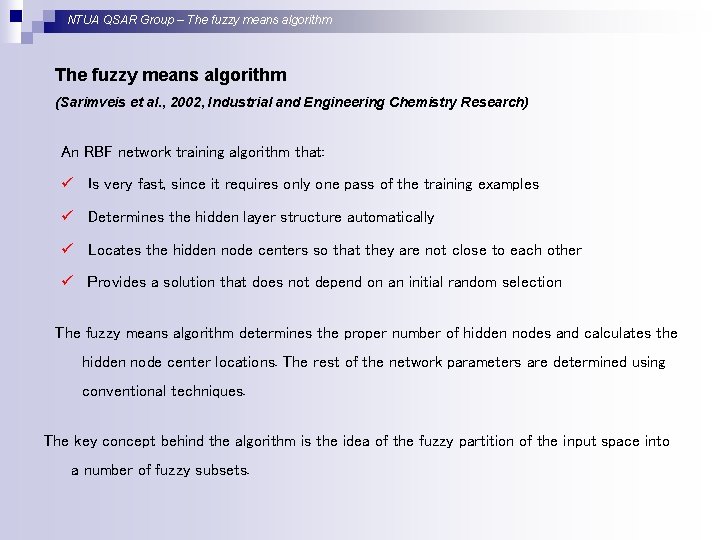
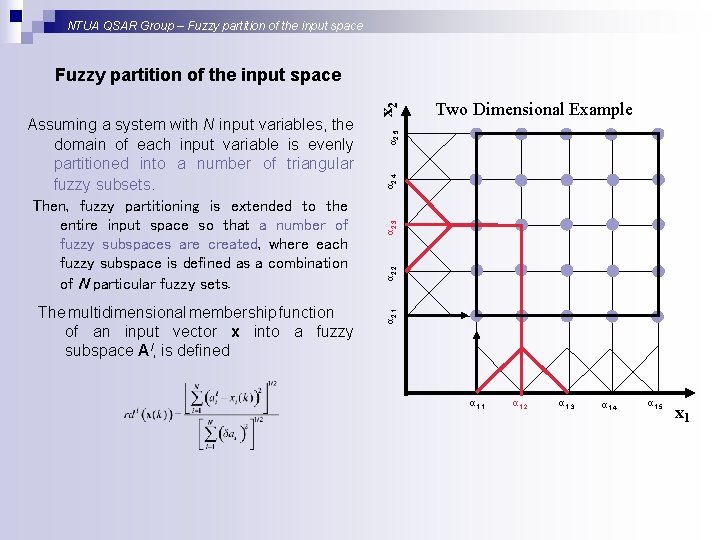
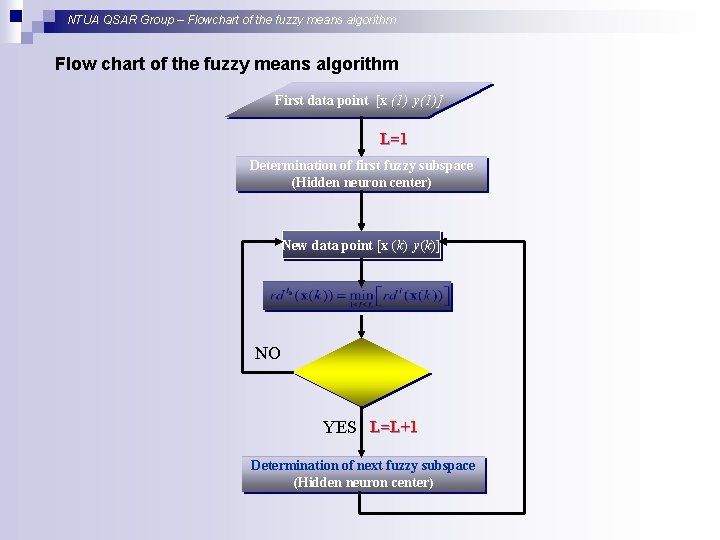
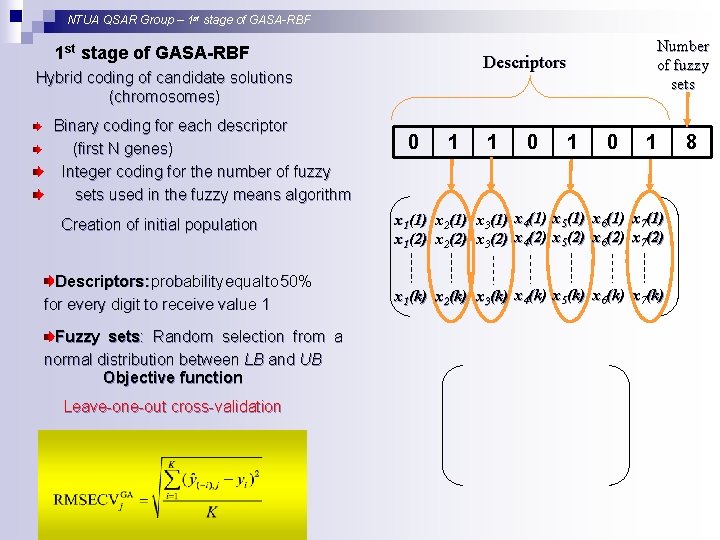
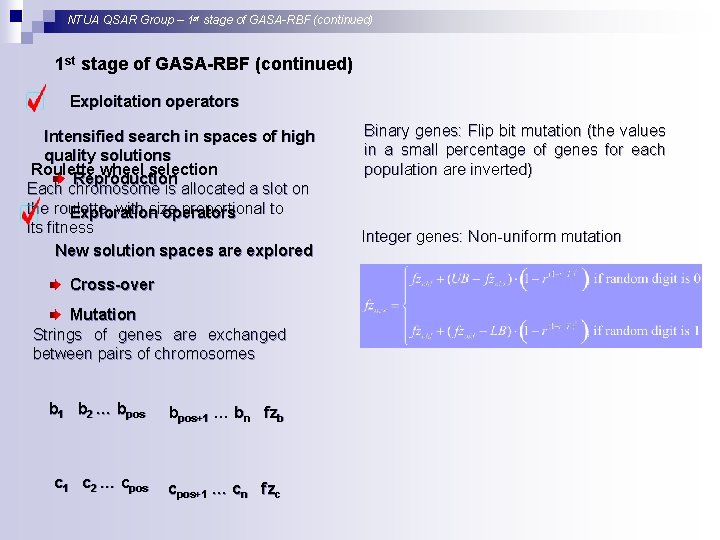
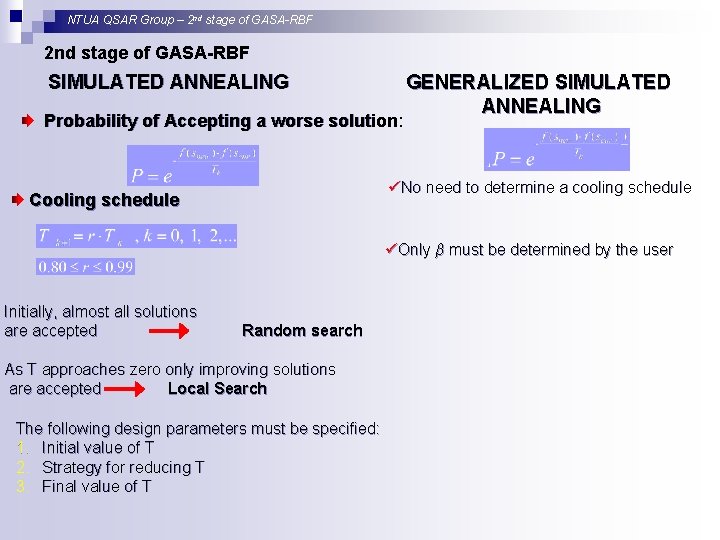
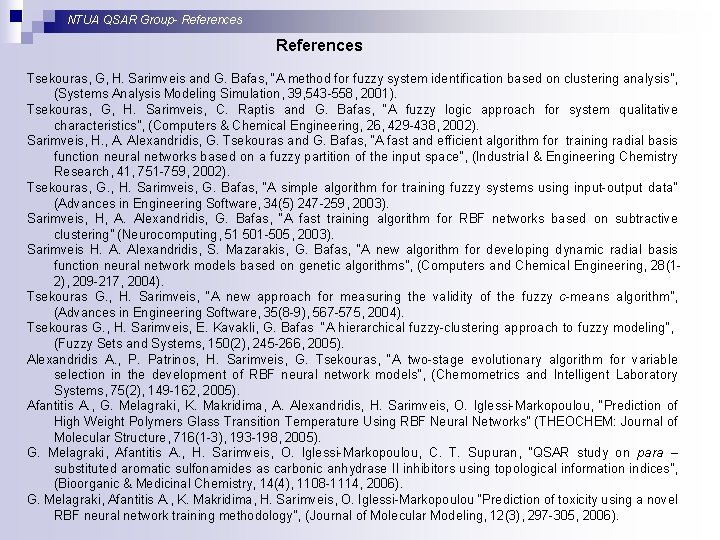
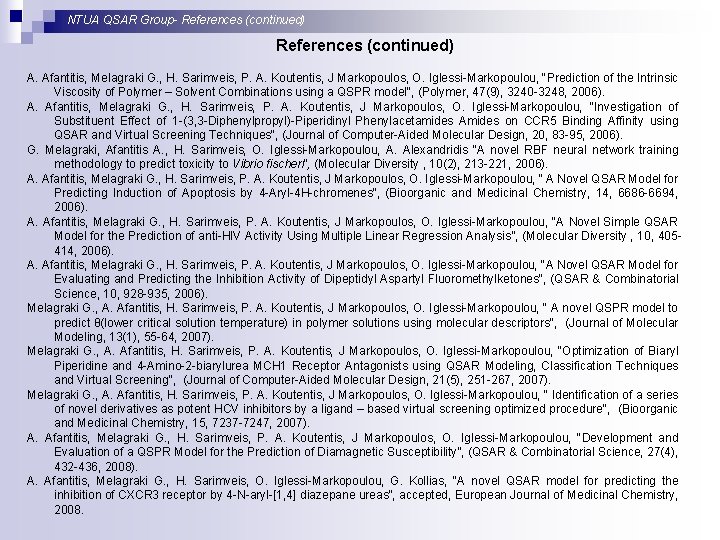
- Slides: 26
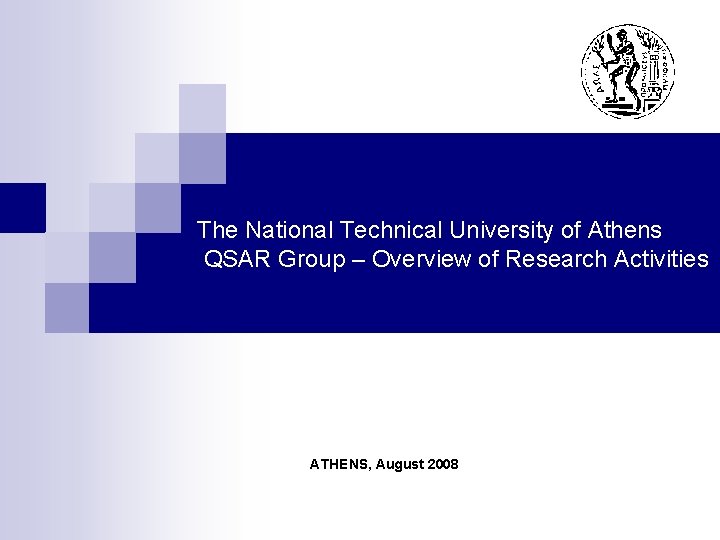
The National Technical University of Athens QSAR Group – Overview of Research Activities ATHENS, August 2008
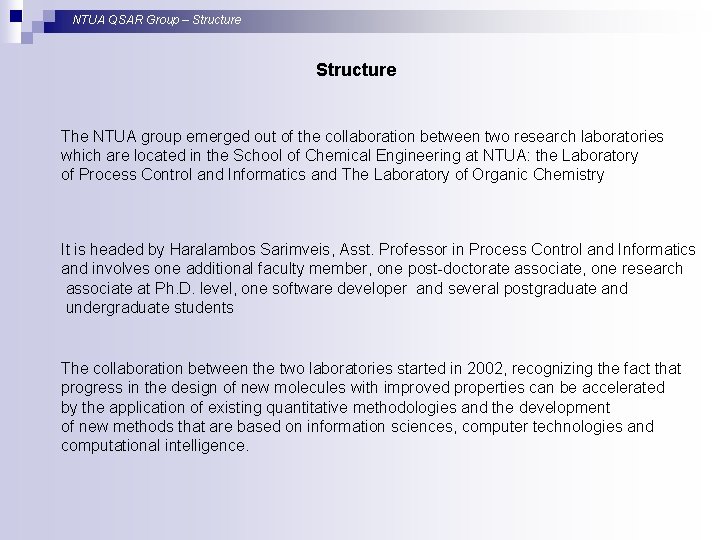
NTUA QSAR Group – Structure The NTUA group emerged out of the collaboration between two research laboratories which are located in the School of Chemical Engineering at NTUA: the Laboratory of Process Control and Informatics and The Laboratory of Organic Chemistry It is headed by Haralambos Sarimveis, Asst. Professor in Process Control and Informatics and involves one additional faculty member, one post-doctorate associate, one research associate at Ph. D. level, one software developer and several postgraduate and undergraduate students The collaboration between the two laboratories started in 2002, recognizing the fact that progress in the design of new molecules with improved properties can be accelerated by the application of existing quantitative methodologies and the development of new methods that are based on information sciences, computer technologies and computational intelligence.
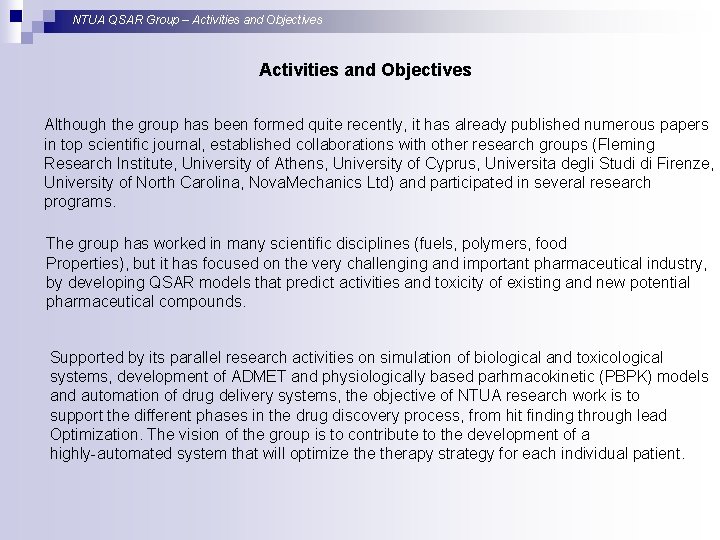
NTUA QSAR Group – Activities and Objectives Although the group has been formed quite recently, it has already published numerous papers in top scientific journal, established collaborations with other research groups (Fleming Research Institute, University of Athens, University of Cyprus, Universita degli Studi di Firenze, University of North Carolina, Nova. Mechanics Ltd) and participated in several research programs. The group has worked in many scientific disciplines (fuels, polymers, food Properties), but it has focused on the very challenging and important pharmaceutical industry, by developing QSAR models that predict activities and toxicity of existing and new potential pharmaceutical compounds. Supported by its parallel research activities on simulation of biological and toxicological systems, development of ADMET and physiologically based parhmacokinetic (PBPK) models and automation of drug delivery systems, the objective of NTUA research work is to support the different phases in the drug discovery process, from hit finding through lead Optimization. The vision of the group is to contribute to the development of a highly-automated system that will optimize therapy strategy for each individual patient.
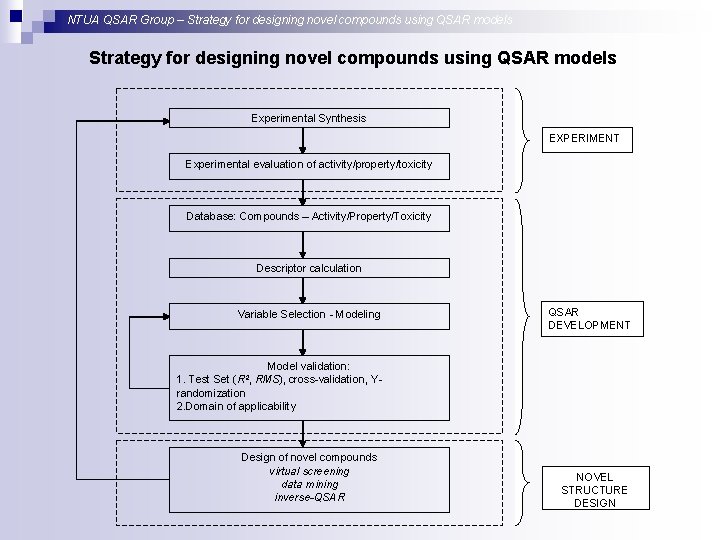
NTUA QSAR Group – Strategy for designing novel compounds using QSAR models Experimental Synthesis EXPERIMENT Experimental evaluation of activity/property/toxicity Database: Compounds – Activity/Property/Toxicity Descriptor calculation Variable Selection - Modeling QSAR DEVELOPMENT Model validation: 1. Test Set (R 2, RMS), cross-validation, Yrandomization 2. Domain of applicability Design of novel compounds virtual screening data mining inverse-QSAR NOVEL STRUCTURE DESIGN
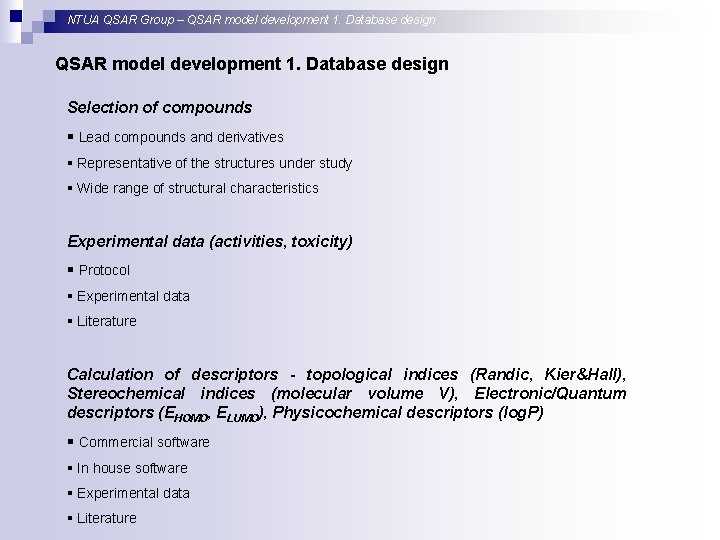
NTUA QSAR Group – QSAR model development 1. Database design Selection of compounds § Lead compounds and derivatives § Representative of the structures under study § Wide range of structural characteristics Experimental data (activities, toxicity) § Protocol § Experimental data § Literature Calculation of descriptors - topological indices (Randic, Kier&Hall), Stereochemical indices (molecular volume V), Electronic/Quantum descriptors (ΕHOMO, ELUMO), Physicochemical descriptors (log. P) § Commercial software § In house software § Experimental data § Literature
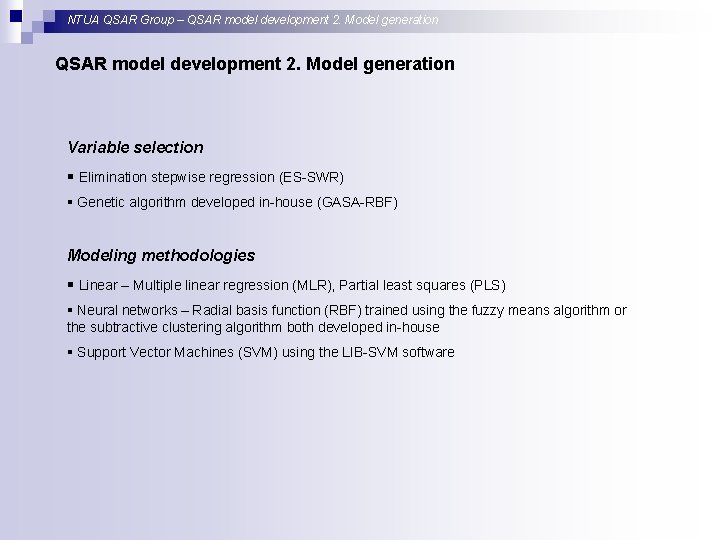
NTUA QSAR Group – QSAR model development 2. Model generation Variable selection § Elimination stepwise regression (ES-SWR) § Genetic algorithm developed in-house (GASA-RBF) Modeling methodologies § Linear – Multiple linear regression (MLR), Partial least squares (PLS) § Neural networks – Radial basis function (RBF) trained using the fuzzy means algorithm or the subtractive clustering algorithm both developed in-house § Support Vector Machines (SVM) using the LIB-SVM software
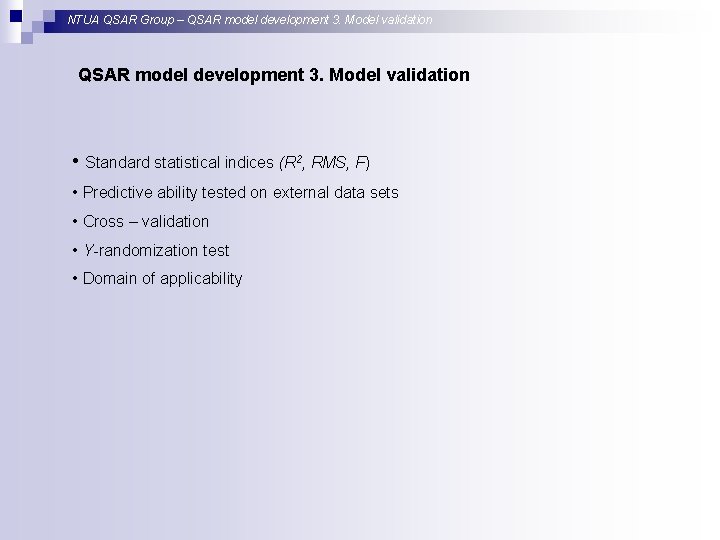
NTUA QSAR Group – QSAR model development 3. Model validation • Standard statistical indices (R 2, RMS, F) • Predictive ability tested on external data sets • Cross – validation • Y-randomization test • Domain of applicability
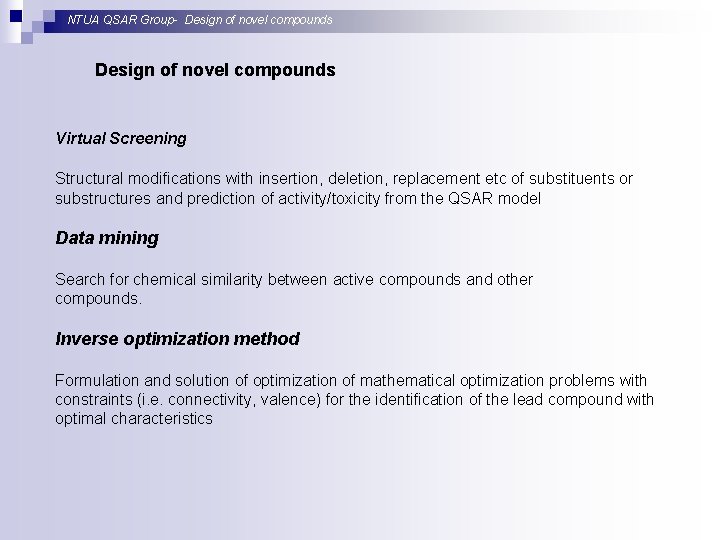
NTUA QSAR Group- Design of novel compounds Virtual Screening Structural modifications with insertion, deletion, replacement etc of substituents or substructures and prediction of activity/toxicity from the QSAR model Data mining Search for chemical similarity between active compounds and other compounds. Inverse optimization method Formulation and solution of optimization of mathematical optimization problems with constraints (i. e. connectivity, valence) for the identification of the lead compound with optimal characteristics
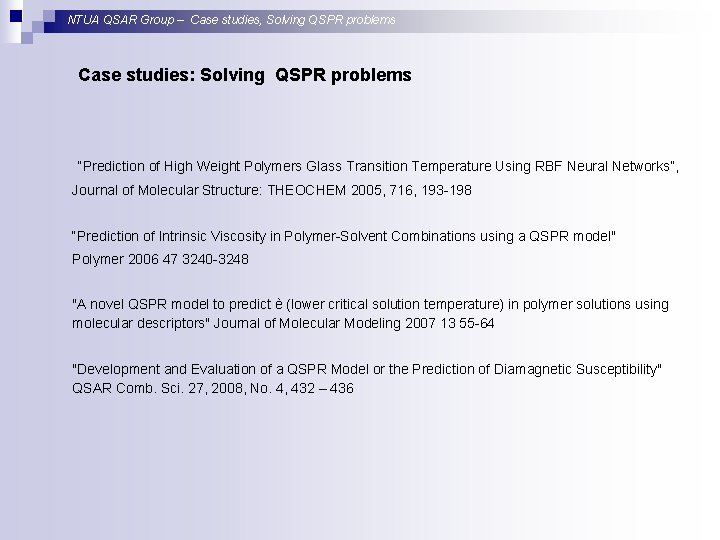
NTUA QSAR Group – Case studies, Solving QSPR problems Case studies: Solving QSPR problems “Prediction of High Weight Polymers Glass Transition Temperature Using RBF Neural Networks”, Journal of Molecular Structure: THEOCHEM 2005, 716, 193 -198 “Prediction of Intrinsic Viscosity in Polymer-Solvent Combinations using a QSPR model" Polymer 2006 47 3240 -3248 "A novel QSPR model to predict è (lower critical solution temperature) in polymer solutions using molecular descriptors" Journal of Molecular Modeling 2007 13 55 -64 "Development and Evaluation of a QSPR Model or the Prediction of Diamagnetic Susceptibility" QSAR Comb. Sci. 27, 2008, No. 4, 432 – 436
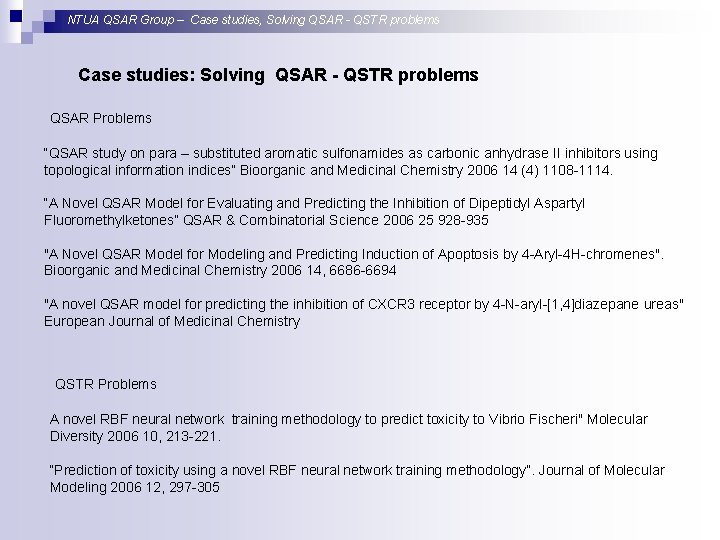
NTUA QSAR Group – Case studies, Solving QSAR - QSTR problems Case studies: Solving QSAR - QSTR problems QSAR Problems “QSAR study on para – substituted aromatic sulfonamides as carbonic anhydrase II inhibitors using topological information indices” Bioorganic and Medicinal Chemistry 2006 14 (4) 1108 -1114. “A Novel QSAR Model for Evaluating and Predicting the Inhibition of Dipeptidyl Aspartyl Fluoromethylketones” QSAR & Combinatorial Science 2006 25 928 -935 "A Novel QSAR Model for Modeling and Predicting Induction of Apoptosis by 4 -Aryl-4 H-chromenes". Bioorganic and Medicinal Chemistry 2006 14, 6686 -6694 "A novel QSAR model for predicting the inhibition of CXCR 3 receptor by 4 -N-aryl-[1, 4]diazepane ureas" European Journal of Medicinal Chemistry QSTR Problems A novel RBF neural network training methodology to predict toxicity to Vibrio Fischeri" Molecular Diversity 2006 10, 213 -221. “Prediction of toxicity using a novel RBF neural network training methodology”. Journal of Molecular Modeling 2006 12, 297 -305
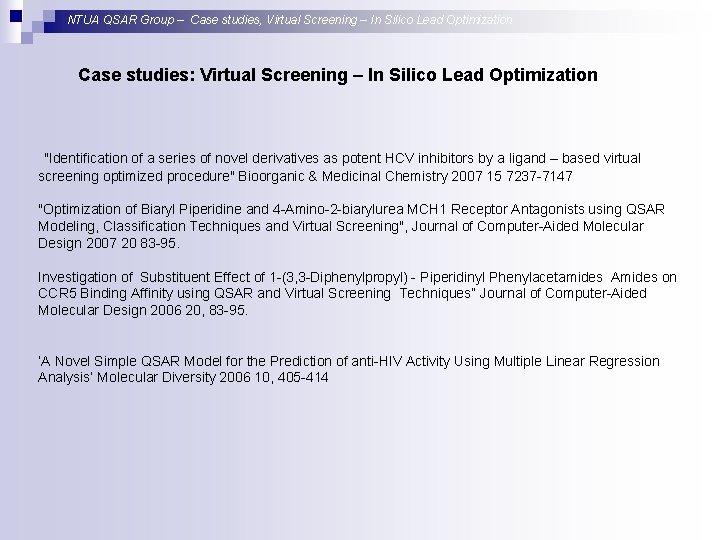
NTUA QSAR Group – Case studies, Virtual Screening – In Silico Lead Optimization Case studies: Virtual Screening – In Silico Lead Optimization "Identification of a series of novel derivatives as potent HCV inhibitors by a ligand – based virtual screening optimized procedure" Bioorganic & Medicinal Chemistry 2007 15 7237 -7147 "Optimization of Biaryl Piperidine and 4 -Amino-2 -biarylurea MCH 1 Receptor Antagonists using QSAR Modeling, Classification Techniques and Virtual Screening", Journal of Computer-Aided Molecular Design 2007 20 83 -95. Investigation of Substituent Effect of 1 -(3, 3 -Diphenylpropyl) - Piperidinyl Phenylacetamides Amides on CCR 5 Binding Affinity using QSAR and Virtual Screening Techniques” Journal of Computer-Aided Molecular Design 2006 20, 83 -95. ‘A Novel Simple QSAR Model for the Prediction of anti-HIV Activity Using Multiple Linear Regression Analysis’ Molecular Diversity 2006 10, 405 -414
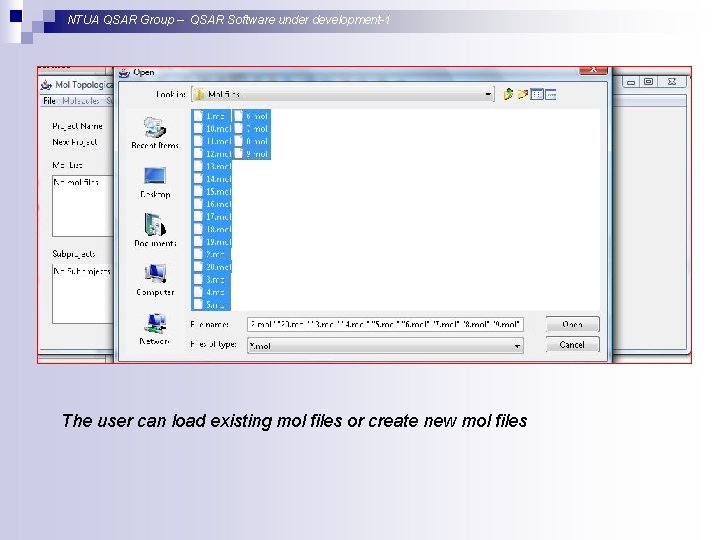
NTUA QSAR Group – QSAR Software under development-1 The user can load existing mol files or create new mol files
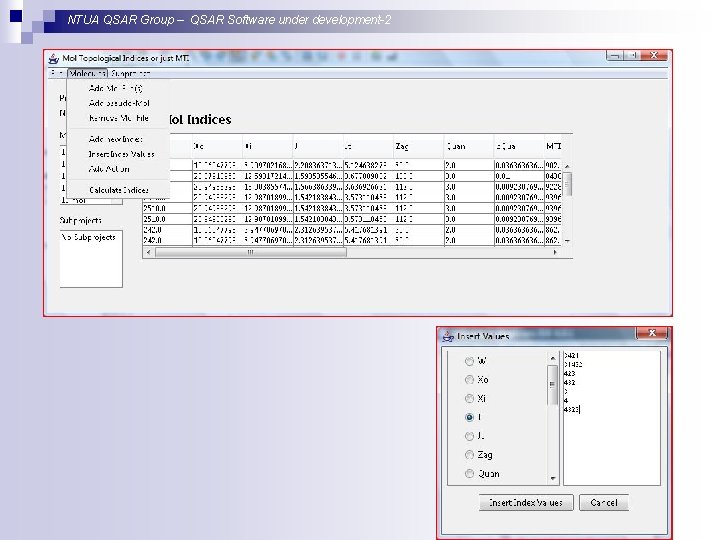
NTUA QSAR Group – QSAR Software under development-2
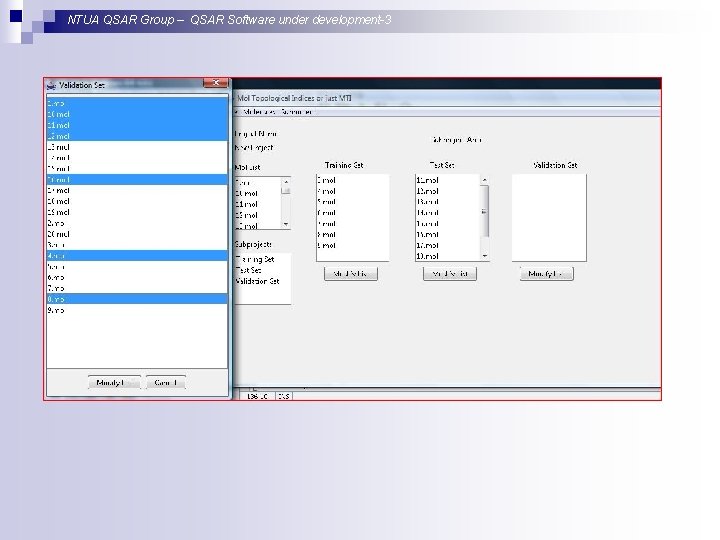
NTUA QSAR Group – QSAR Software under development-3
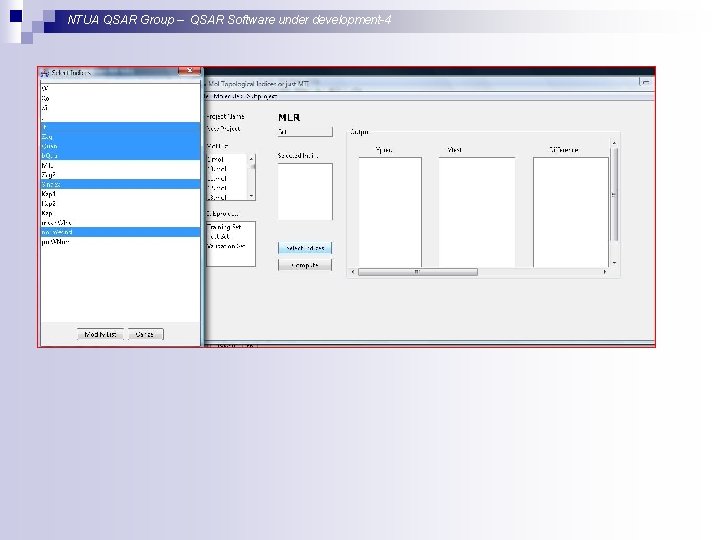
NTUA QSAR Group – QSAR Software under development-4
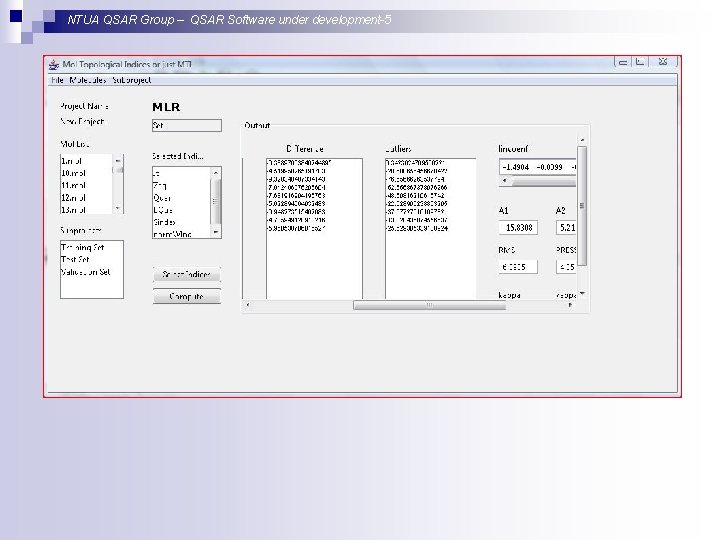
NTUA QSAR Group – QSAR Software under development-5
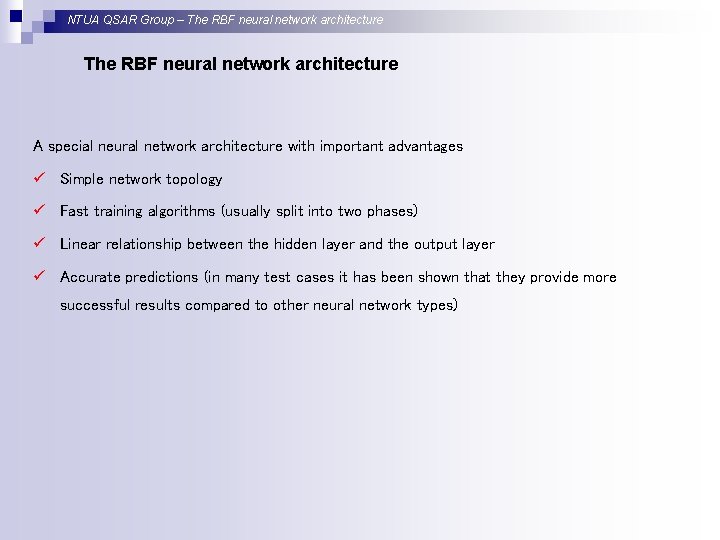
NTUA QSAR Group – The RBF neural network architecture A special neural network architecture with important advantages ü Simple network topology ü Fast training algorithms (usually split into two phases) ü Linear relationship between the hidden layer and the output layer ü Accurate predictions (in many test cases it has been shown that they provide more successful results compared to other neural network types)
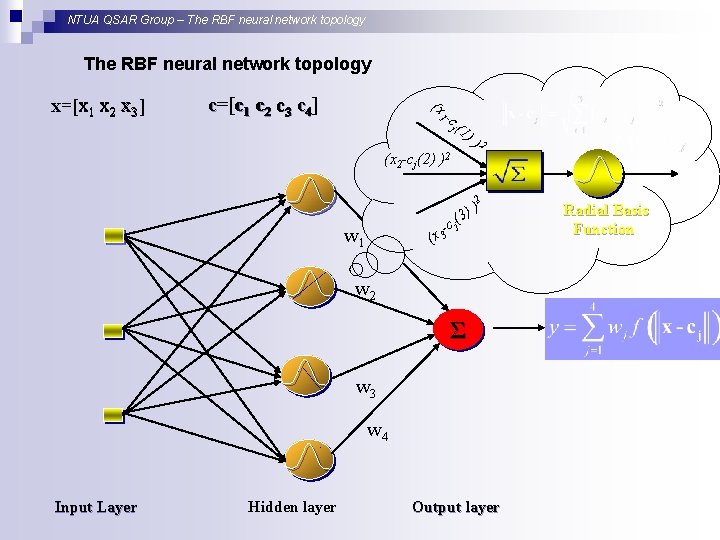
NTUA QSAR Group – The RBF neural network topology x=[xx 1 x 2 x 3 ] c=[c 11 c 2 c 3 c 4] (x 1 -c j (1 ))2 (x 2 -cj(2) )2 2 ) ) 3 c (j (x 3 w 1 w 2 Σ w 3 w 4 Input Layer Hidden layer Output layer Radial Basis Function
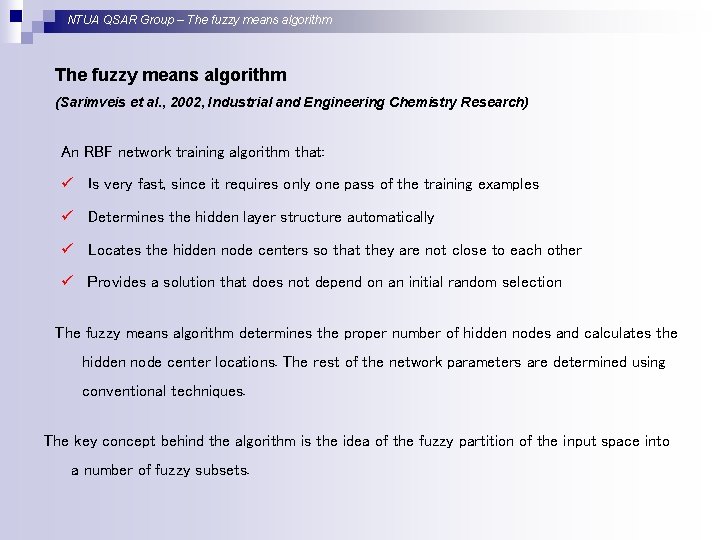
NTUA QSAR Group – The fuzzy means algorithm (Sarimveis et al. , 2002, Industrial and Engineering Chemistry Research) An RBF network training algorithm that: ü Is very fast, since it requires only one pass of the training examples ü Determines the hidden layer structure automatically ü Locates the hidden node centers so that they are not close to each other ü Provides a solution that does not depend on an initial random selection The fuzzy means algorithm determines the proper number of hidden nodes and calculates the hidden node center locations. The rest of the network parameters are determined using conventional techniques. The key concept behind the algorithm is the idea of the fuzzy partition of the input space into a number of fuzzy subsets.
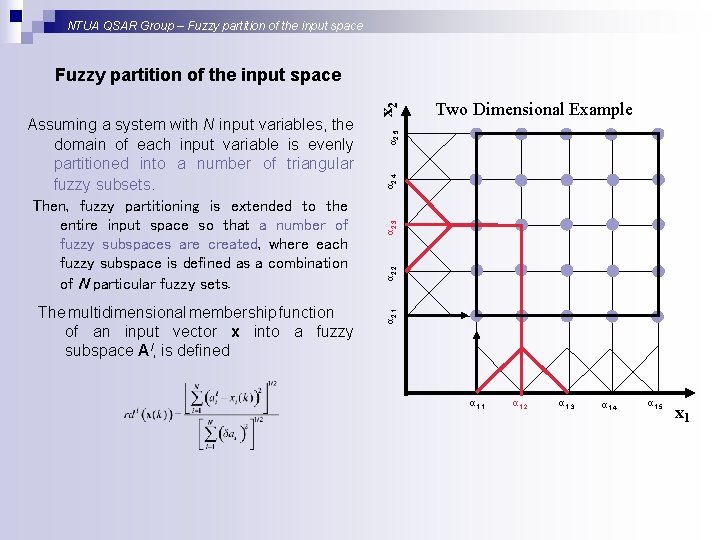
NTUA QSAR Group – Fuzzy partition of the input space The multidimensional membership function of an input vector x into a fuzzy subspace Al, is defined α 2, 2 α 2, 3 α 2, 4 α 2, 5 Two Dimensional Example α 2, 1 Assuming a system with N input variables, the domain of each input variable is evenly partitioned into a number of triangular fuzzy subsets. Then, fuzzy partitioning is extended to the entire input space so that a number of fuzzy subspaces are created, where each fuzzy subspace is defined as a combination of N particular fuzzy sets. x 2 Fuzzy partition of the input space α 1, 1 α 1, 2 α 1, 3 α 1, 4 α 1, 5 x 1
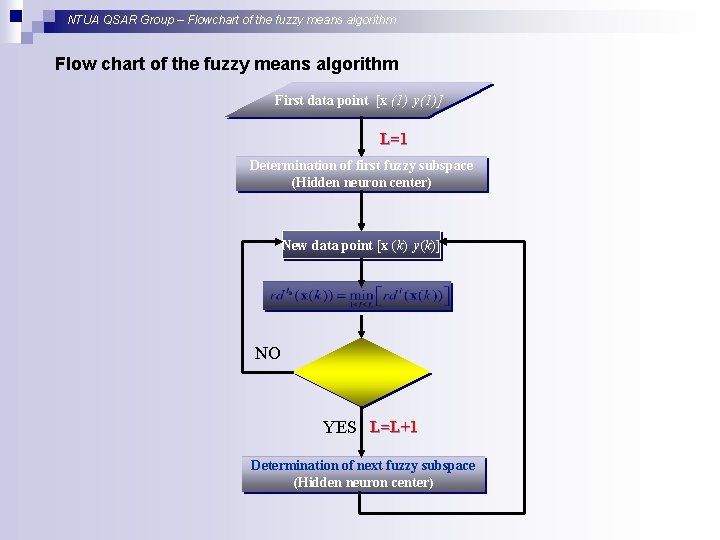
NTUA QSAR Group – Flowchart of the fuzzy means algorithm Flow chart of the fuzzy means algorithm First data point [x (1) y(1)] L=1 Determination of first fuzzy subspace (Hidden neuron center) New data point [x (k) y(k)] NO YES L=L+1 Determination of next fuzzy subspace (Hidden neuron center)
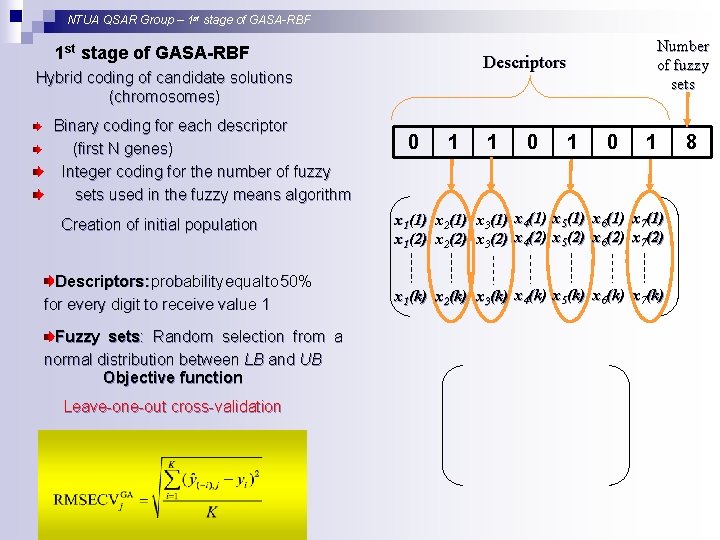
NTUA QSAR Group – 1 st stage of GASA-RBF Descriptors Hybrid coding of candidate solutions (chromosomes) Binary coding for each descriptor (first N genes) Integer coding for the number of fuzzy sets used in the fuzzy means algorithm Creation of initial population Descriptors: probability equal to 50% for every digit to receive value 1 Fuzzy sets: Random selection from a normal distribution between LB and UB Objective function Leave-one-out cross-validation Number of fuzzy sets 0 1 1 0 1 x 1(1) x 2(1) x 3(1) x 4(1) x 5(1) x 6(1) x 7(1) x 1(2) x 2(2) x 3(2) x 4(2) x 5(2) x 6(2) x 7(2) x 1(k) x 2(k) x 3(k) x 4(k) x 5(k) x 6(k) x 7(k) 8
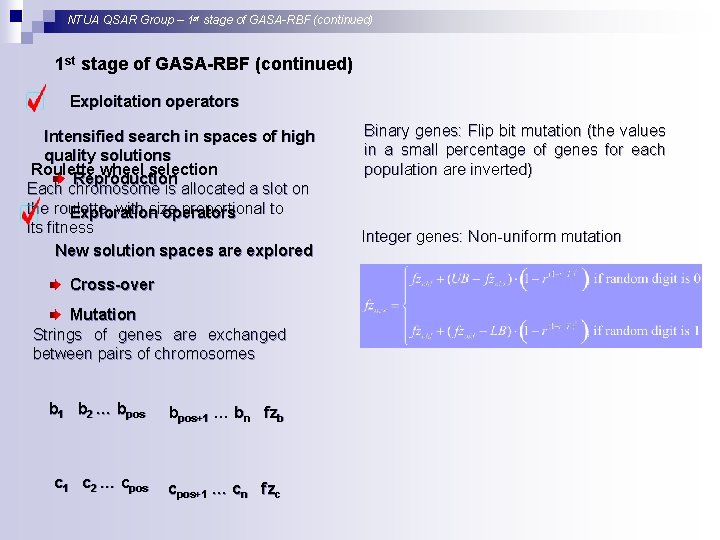
NTUA QSAR Group – 1 st stage of GASA-RBF (continued) Exploitation operators Intensified search in spaces of high quality solutions Roulette wheel selection Reproduction Each chromosome is allocated a slot on the roulette, with size proportional to Exploration operators its fitness New solution spaces are explored Cross-over Mutation Strings of genes are exchanged between pairs of chromosomes b 1 b 2 … bpos+1 … bn fzb c 1 c 2 … cpos+1 … cn fzc Binary genes: Flip bit mutation (the values in a small percentage of genes for each population are inverted) Integer genes: Non-uniform mutation
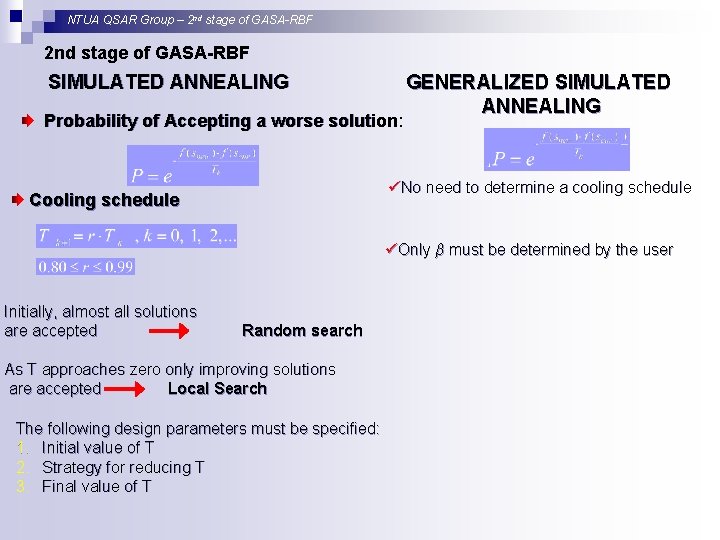
NTUA QSAR Group – 2 nd stage of GASA-RBF SIMULATED ANNEALING Probability of Accepting a worse solution: solution GENERALIZED SIMULATED ANNEALING üNo need to determine a cooling schedule Cooling schedule üOnly β must be determined by the user Initially, almost all solutions are accepted Random search As T approaches zero only improving solutions are accepted Local Search The following design parameters must be specified: 1. Initial value of T 2. Strategy for reducing Τ 3. Final value of Τ
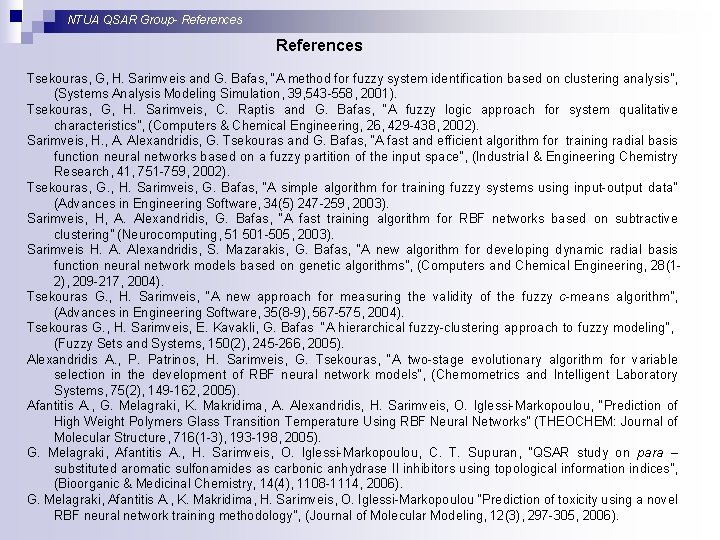
NTUA QSAR Group- References Tsekouras, G, H. Sarimveis and G. Bafas, “A method for fuzzy system identification based on clustering analysis”, (Systems Analysis Modeling Simulation, 39, 543 -558, 2001). Tsekouras, G, H. Sarimveis, C. Raptis and G. Bafas, “A fuzzy logic approach for system qualitative characteristics”, (Computers & Chemical Engineering, 26, 429 -438, 2002). Sarimveis, H. , A. Alexandridis, G. Tsekouras and G. Bafas, “A fast and efficient algorithm for training radial basis function neural networks based on a fuzzy partition of the input space”, (Industrial & Engineering Chemistry Research, 41, 751 -759, 2002). Tsekouras, G. , H. Sarimveis, G. Bafas, “A simple algorithm for training fuzzy systems using input-output data” (Advances in Engineering Software, 34(5) 247 -259, 2003). Sarimveis, H, A. Alexandridis, G. Bafas, “A fast training algorithm for RBF networks based on subtractive clustering” (Neurocomputing, 51 501 -505, 2003). Sarimveis H. Alexandridis, S. Mazarakis, G. Bafas, “A new algorithm for developing dynamic radial basis function neural network models based on genetic algorithms”, (Computers and Chemical Engineering, 28(12), 209 -217, 2004). Tsekouras G. , H. Sarimveis, “A new approach for measuring the validity of the fuzzy c-means algorithm”, (Advances in Engineering Software, 35(8 -9), 567 -575, 2004). Tsekouras G. , H. Sarimveis, E. Kavakli, G. Bafas “A hierarchical fuzzy-clustering approach to fuzzy modeling”, (Fuzzy Sets and Systems, 150(2), 245 -266, 2005). Alexandridis A. , P. Patrinos, H. Sarimveis, G. Tsekouras, “A two-stage evolutionary algorithm for variable selection in the development of RBF neural network models”, (Chemometrics and Intelligent Laboratory Systems, 75(2), 149 -162, 2005). Afantitis Α. , G. Melagraki, K. Makridima, A. Alexandridis, H. Sarimveis, O. Iglessi-Markopoulou, “Prediction of High Weight Polymers Glass Transition Temperature Using RBF Neural Networks” (ΤΗΕOCHEM: Journal of Molecular Structure, 716(1 -3), 193 -198, 2005). G. Melagraki, Afantitis Α. , H. Sarimveis, O. Iglessi-Markopoulou, C. T. Supuran, “QSAR study on para – substituted aromatic sulfonamides as carbonic anhydrase II inhibitors using topological information indices”, (Bioorganic & Medicinal Chemistry, 14(4), 1108 -1114, 2006). G. Melagraki, Afantitis Α. , K. Makridima, H. Sarimveis, O. Iglessi-Markopoulou “Prediction of toxicity using a novel RBF neural network training methodology”, (Journal of Molecular Modeling, 12(3), 297 -305, 2006).
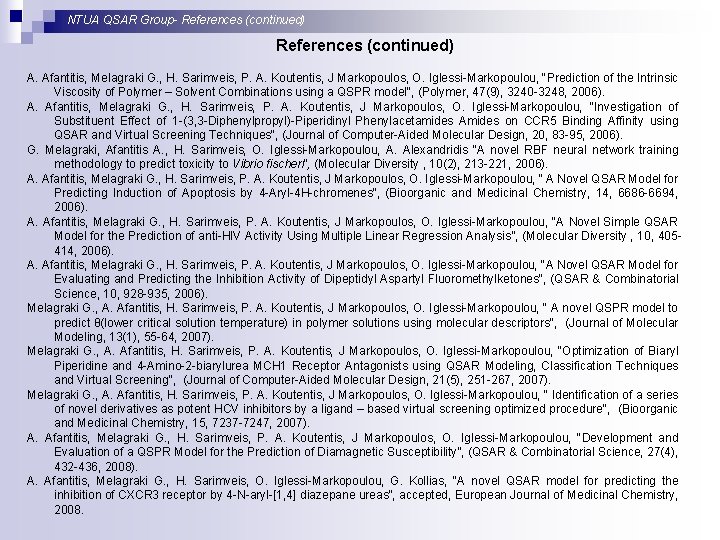
NTUA QSAR Group- References (continued) A. Afantitis, Melagraki G. , H. Sarimveis, P. A. Koutentis, J Markopoulos, O. Iglessi-Markopoulou, "Prediction of the Intrinsic Viscosity of Polymer – Solvent Combinations using a QSPR model", (Polymer, 47(9), 3240 -3248, 2006). A. Afantitis, Melagraki G. , H. Sarimveis, P. A. Koutentis, J Markopoulos, O. Iglessi-Markopoulou, "Investigation of Substituent Effect of 1 -(3, 3 -Diphenylpropyl)-Piperidinyl Phenylacetamides Amides on CCR 5 Binding Affinity using QSAR and Virtual Screening Techniques", (Journal of Computer-Aided Molecular Design, 20, 83 -95, 2006). G. Melagraki, Afantitis Α. , H. Sarimveis, O. Iglessi-Markopoulou, A. Alexandridis “A novel RBF neural network training methodology to predict toxicity to Vibrio fischeri”, (Molecular Diversity , 10(2), 213 -221, 2006). A. Afantitis, Melagraki G. , H. Sarimveis, P. A. Koutentis, J Markopoulos, O. Iglessi-Markopoulou, " A Novel QSAR Model for Predicting Induction of Apoptosis by 4 -Aryl-4 H-chromenes", (Bioorganic and Medicinal Chemistry, 14, 6686 -6694, 2006). A. Afantitis, Melagraki G. , H. Sarimveis, P. A. Koutentis, J Markopoulos, O. Iglessi-Markopoulou, “A Novel Simple QSAR Model for the Prediction of anti-HIV Activity Using Multiple Linear Regression Analysis”, (Molecular Diversity , 10, 405414, 2006). A. Afantitis, Melagraki G. , H. Sarimveis, P. A. Koutentis, J Markopoulos, O. Iglessi-Markopoulou, "A Novel QSAR Model for Evaluating and Predicting the Inhibition Activity of Dipeptidyl Aspartyl Fluoromethylketones", (QSAR & Combinatorial Science, 10, 928 -935, 2006). Melagraki G. , A. Afantitis, H. Sarimveis, P. A. Koutentis, J Markopoulos, O. Iglessi-Markopoulou, " A novel QSPR model to predict θ(lower critical solution temperature) in polymer solutions using molecular descriptors", (Journal of Molecular Modeling, 13(1), 55 -64, 2007). Melagraki G. , A. Afantitis, H. Sarimveis, P. A. Koutentis, J Markopoulos, O. Iglessi-Markopoulou, "Optimization of Biaryl Piperidine and 4 -Amino-2 -biarylurea MCH 1 Receptor Antagonists using QSAR Modeling, Classification Techniques and Virtual Screening", (Journal of Computer-Aided Molecular Design, 21(5), 251 -267, 2007). Melagraki G. , A. Afantitis, H. Sarimveis, P. A. Koutentis, J Markopoulos, O. Iglessi-Markopoulou, " Identification of a series of novel derivatives as potent HCV inhibitors by a ligand – based virtual screening optimized procedure", (Bioorganic and Medicinal Chemistry, 15, 7237 -7247, 2007). A. Afantitis, Melagraki G. , H. Sarimveis, P. A. Koutentis, J Markopoulos, O. Iglessi-Markopoulou, "Development and Evaluation of a QSPR Model for the Prediction of Diamagnetic Susceptibility”, (QSAR & Combinatorial Science, 27(4), 432 -436, 2008). A. Afantitis, Melagraki G. , H. Sarimveis, O. Iglessi-Markopoulou, G. Kollias, "A novel QSAR model for predicting the inhibition of CXCR 3 receptor by 4 -N-aryl-[1, 4] diazepane ureas”, accepted, European Journal of Medicinal Chemistry, 2008.
National and kapodistrian university of athens events
Donetsk national technical university
National technical university kharkiv polytechnic institute
Sar versus qsar
Qsar
Log 1/c qsar
Drug discovery process
In hansch equation π stands for
Oecd qsar toolbox
Qsar
Qsar toolbox 매뉴얼
Nths cord
Nts fullerton
National research center for career and technical education
Ytuerasmus
Ibm power systems technical university
Technical university sofia
"bauman moscow state technical university" "fraud"
Czech technical university mechanical engineering
Yaroslavl state technical university
University of kragujevac faculty of technical sciences
Technical university of sofia
Technical university of cluj-napoca academic staff
Odtü open course
Gh. asachi
Czech technical university erasmus
Czech technical university in prague civil engineering