The central role of the propensity score in
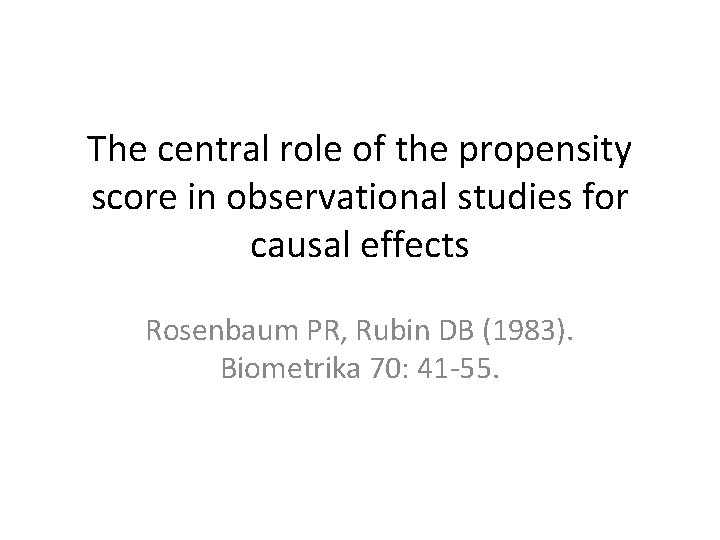
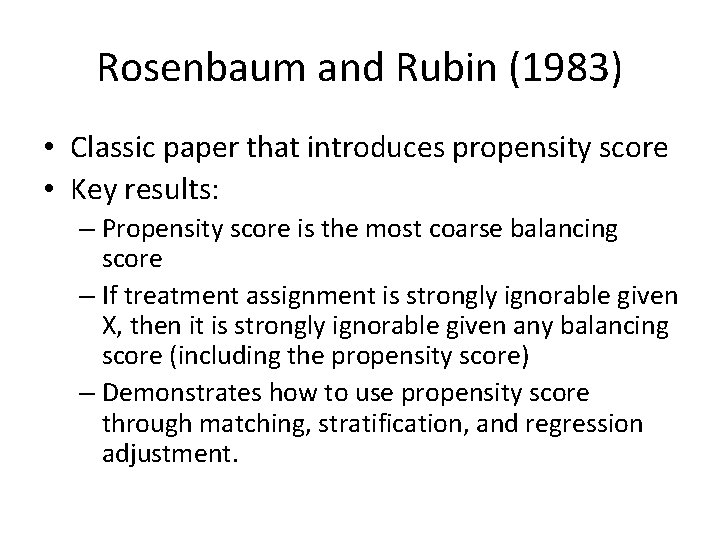
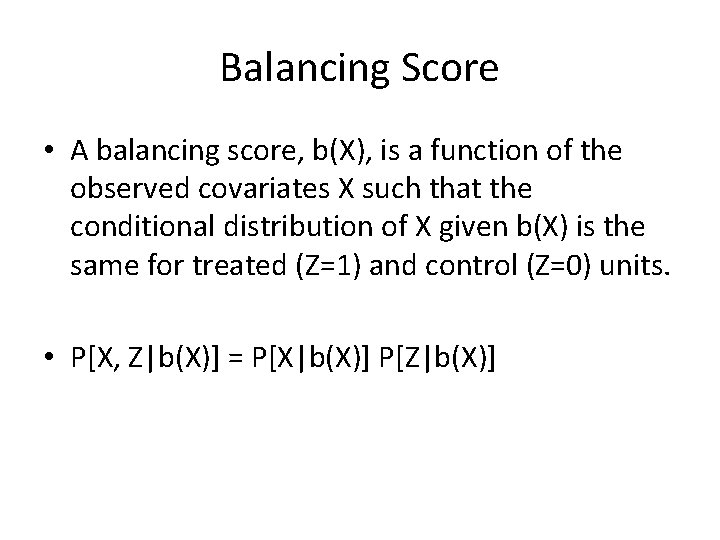
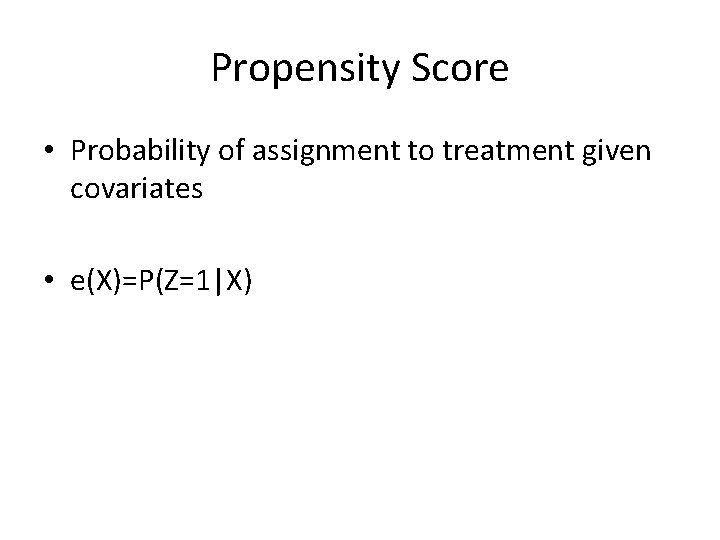
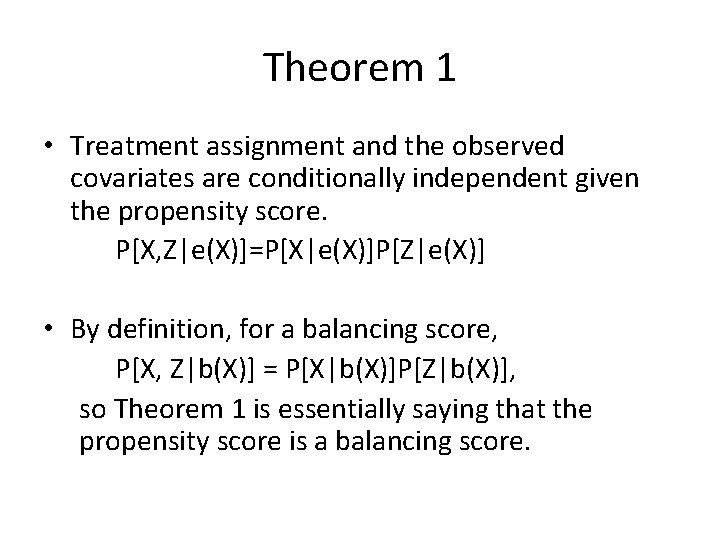
![Proof that the propensity score is a balancing score • Show that P[X, Z|e(X)]=P[X|e(X)]P[Z|e(X)] Proof that the propensity score is a balancing score • Show that P[X, Z|e(X)]=P[X|e(X)]P[Z|e(X)]](https://slidetodoc.com/presentation_image_h/93b73a7920f60b49bba5353472ad9b46/image-6.jpg)
![Theorem 2 • b(X) is a balancing score, that is, P[X, Z|b(X)]=P[X|b(X)]P[Z|b(X)], if and Theorem 2 • b(X) is a balancing score, that is, P[X, Z|b(X)]=P[X|b(X)]P[Z|b(X)], if and](https://slidetodoc.com/presentation_image_h/93b73a7920f60b49bba5353472ad9b46/image-7.jpg)
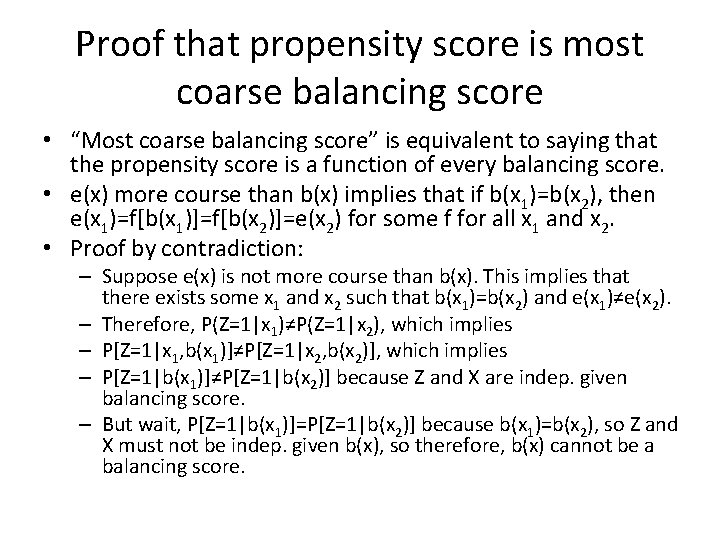
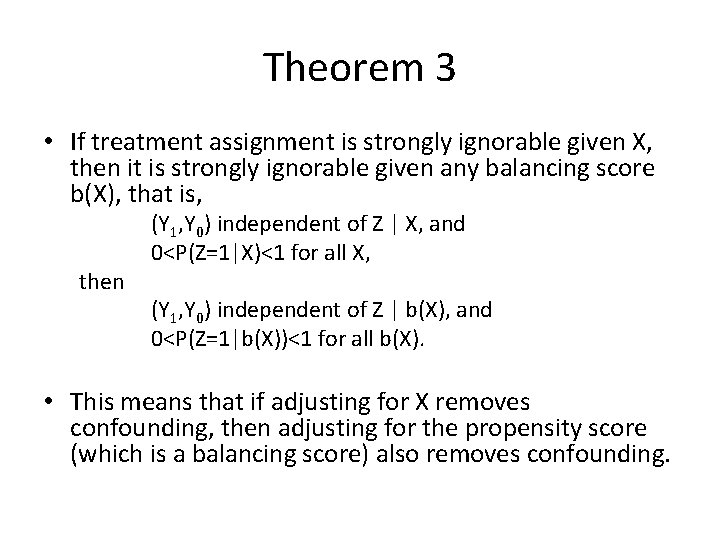
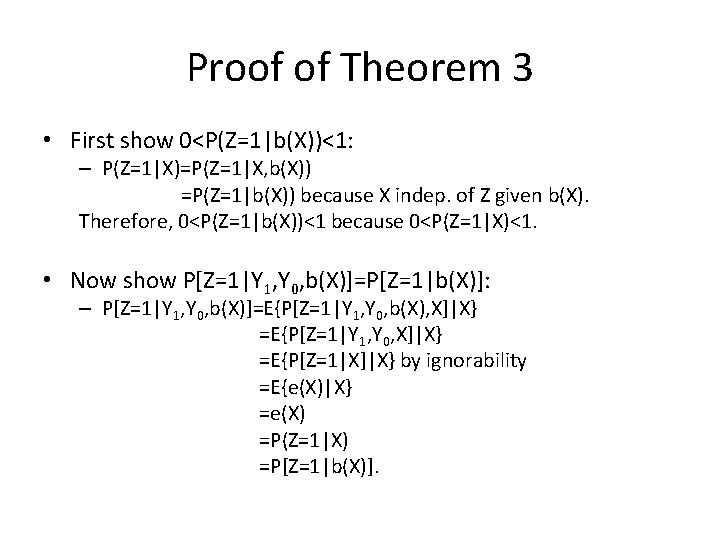
![Average Treatment Effect (Theorem 4) • E[Y 1 -Y 0] is what we want. Average Treatment Effect (Theorem 4) • E[Y 1 -Y 0] is what we want.](https://slidetodoc.com/presentation_image_h/93b73a7920f60b49bba5353472ad9b46/image-11.jpg)
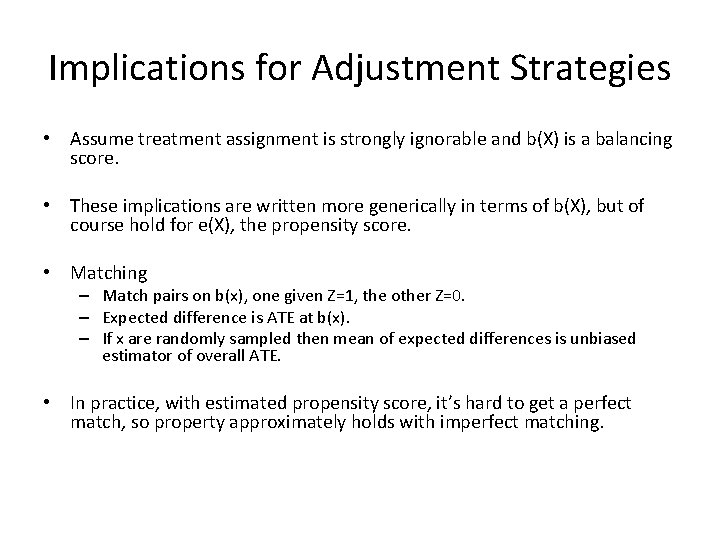
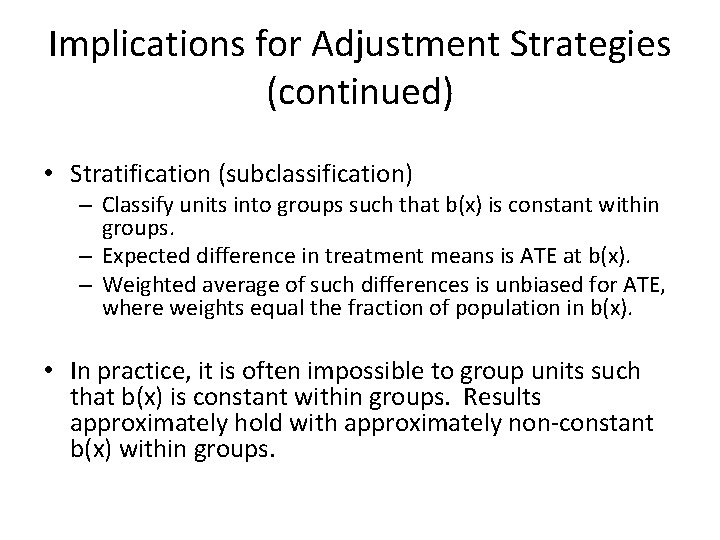
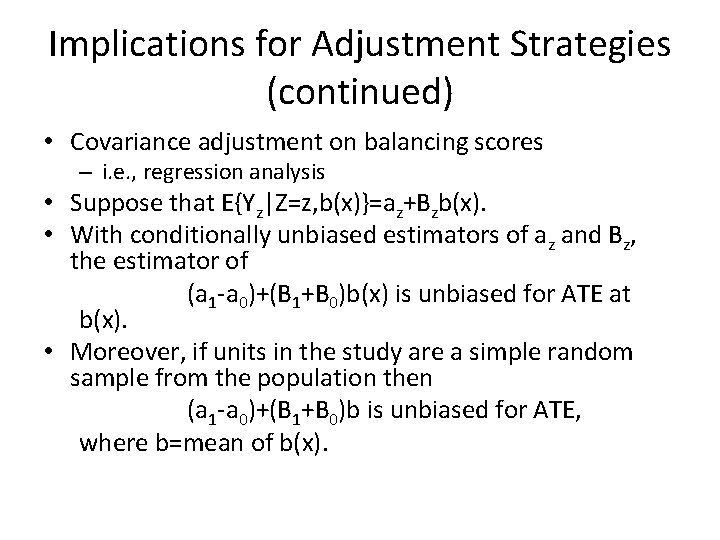
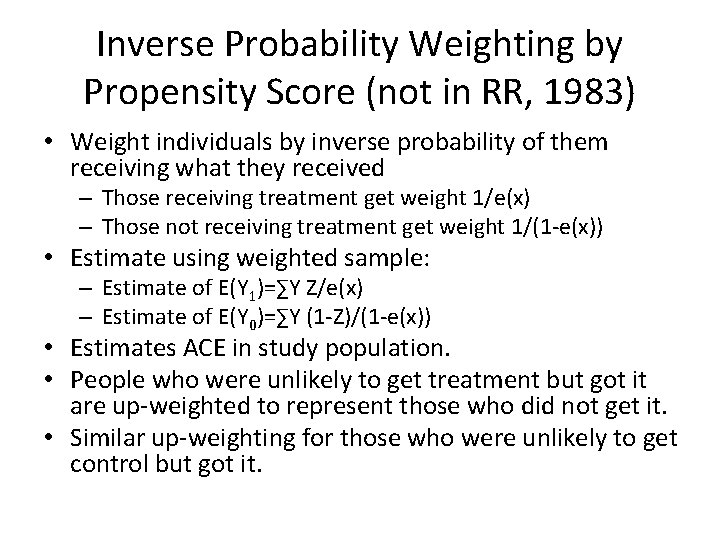
![Proof that IPW estimate is unbiased estimate of causal effect • E[Y Z /e(X)] Proof that IPW estimate is unbiased estimate of causal effect • E[Y Z /e(X)]](https://slidetodoc.com/presentation_image_h/93b73a7920f60b49bba5353472ad9b46/image-16.jpg)
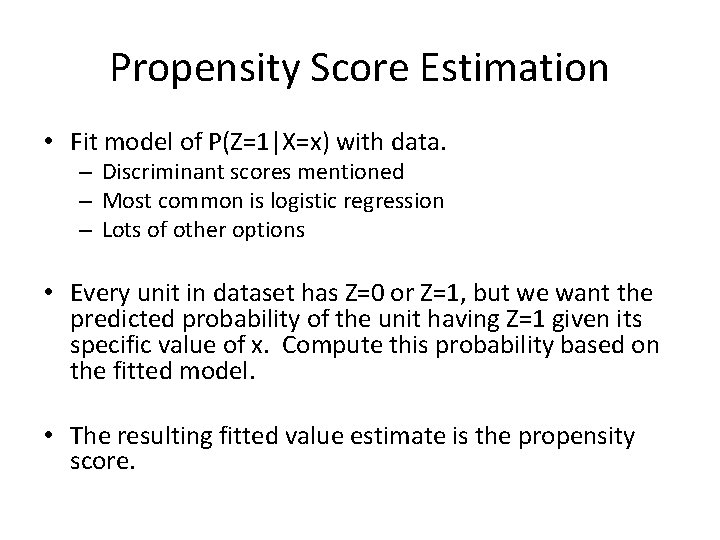
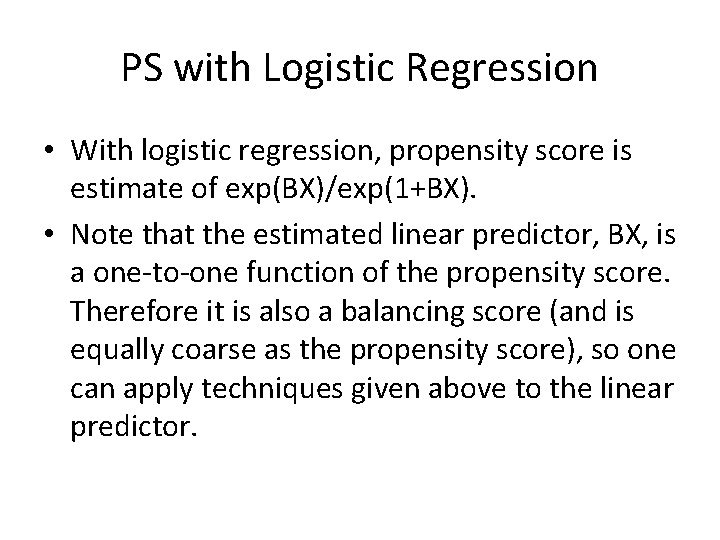
- Slides: 18
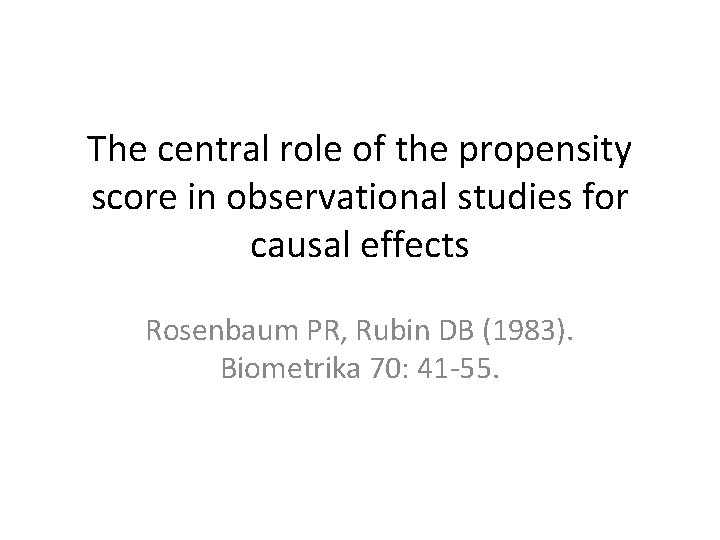
The central role of the propensity score in observational studies for causal effects Rosenbaum PR, Rubin DB (1983). Biometrika 70: 41 -55.
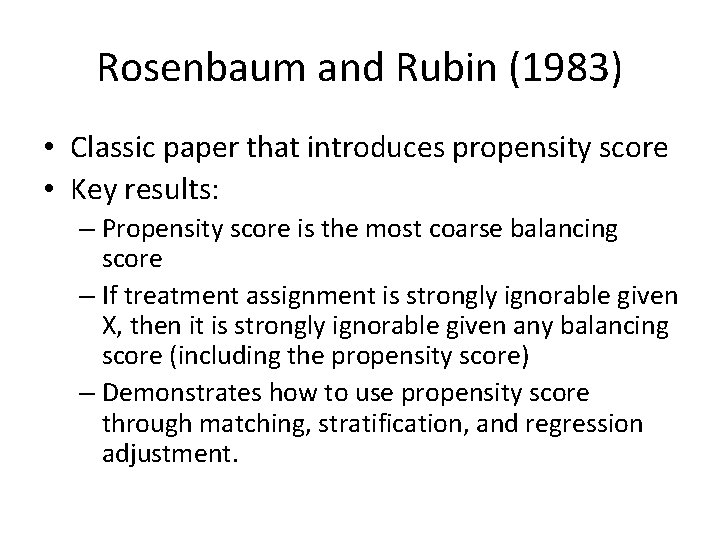
Rosenbaum and Rubin (1983) • Classic paper that introduces propensity score • Key results: – Propensity score is the most coarse balancing score – If treatment assignment is strongly ignorable given X, then it is strongly ignorable given any balancing score (including the propensity score) – Demonstrates how to use propensity score through matching, stratification, and regression adjustment.
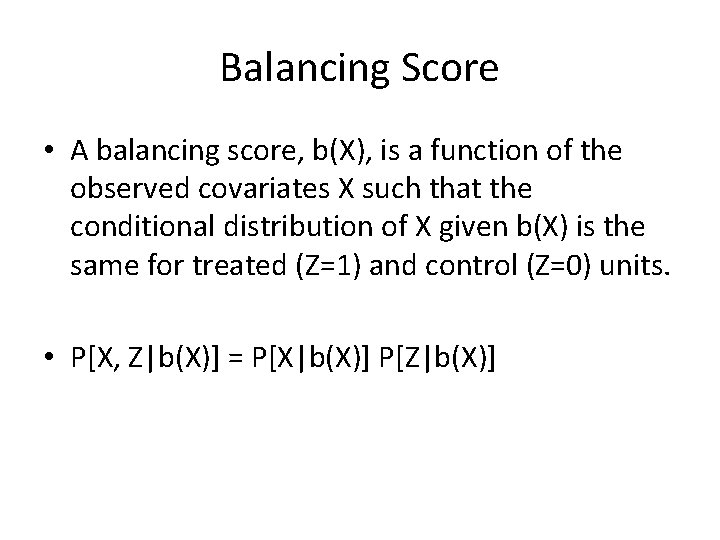
Balancing Score • A balancing score, b(X), is a function of the observed covariates X such that the conditional distribution of X given b(X) is the same for treated (Z=1) and control (Z=0) units. • P[X, Z|b(X)] = P[X|b(X)] P[Z|b(X)]
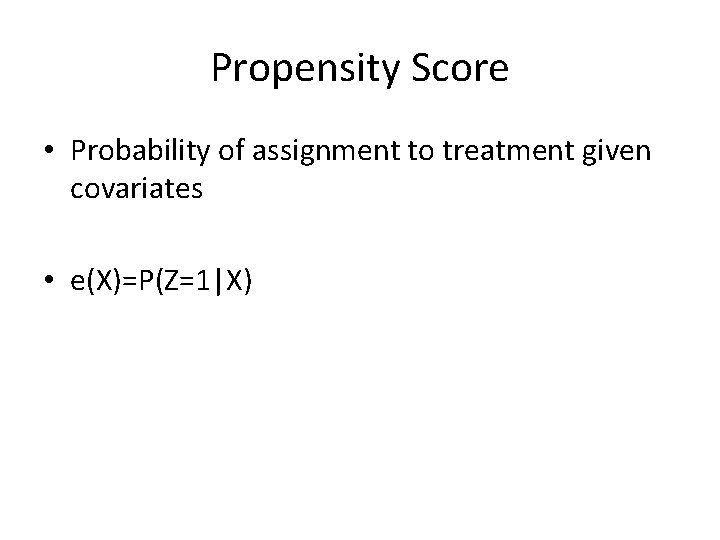
Propensity Score • Probability of assignment to treatment given covariates • e(X)=P(Z=1|X)
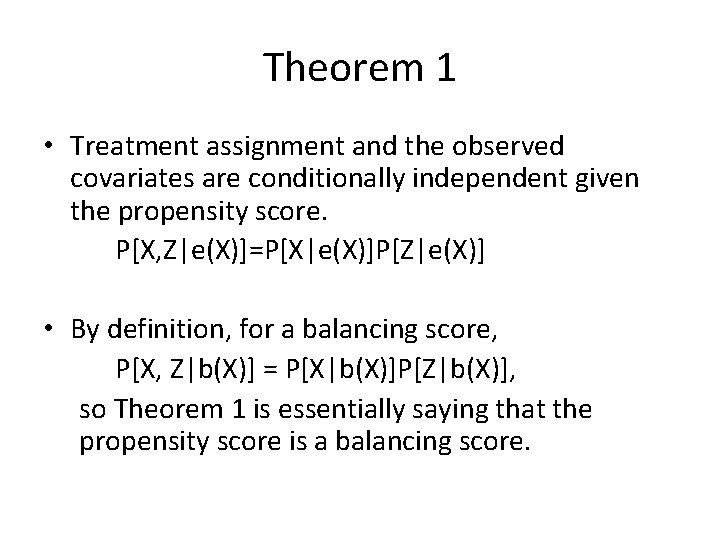
Theorem 1 • Treatment assignment and the observed covariates are conditionally independent given the propensity score. P[X, Z|e(X)]=P[X|e(X)]P[Z|e(X)] • By definition, for a balancing score, P[X, Z|b(X)] = P[X|b(X)]P[Z|b(X)], so Theorem 1 is essentially saying that the propensity score is a balancing score.
![Proof that the propensity score is a balancing score Show that PX ZeXPXeXPZeX Proof that the propensity score is a balancing score • Show that P[X, Z|e(X)]=P[X|e(X)]P[Z|e(X)]](https://slidetodoc.com/presentation_image_h/93b73a7920f60b49bba5353472ad9b46/image-6.jpg)
Proof that the propensity score is a balancing score • Show that P[X, Z|e(X)]=P[X|e(X)]P[Z|e(X)] or equivalently, P[Z=1|X, e(X)]=P[Z=1|e(X)]. • P[Z=1|X, e(X)]=P[Z=1|X]=e(X), and • P[Z=1|e(X)]=E[Z|e(X)] =E{E[Z|X, e(X)]|X} =E{E[Z|X]|X} =E{e(X)|X} =e(X). • Therefore, P[Z=1|X, e(X)]=P[Z=1|e(X)], and e(X) is a balancing score.
![Theorem 2 bX is a balancing score that is PX ZbXPXbXPZbX if and Theorem 2 • b(X) is a balancing score, that is, P[X, Z|b(X)]=P[X|b(X)]P[Z|b(X)], if and](https://slidetodoc.com/presentation_image_h/93b73a7920f60b49bba5353472ad9b46/image-7.jpg)
Theorem 2 • b(X) is a balancing score, that is, P[X, Z|b(X)]=P[X|b(X)]P[Z|b(X)], if and only if b(X) is finer than e(X) in the sense that e(X)=f[b(X)] for some function f. • This theorem states that the propensity score is the most coarse balancing score.
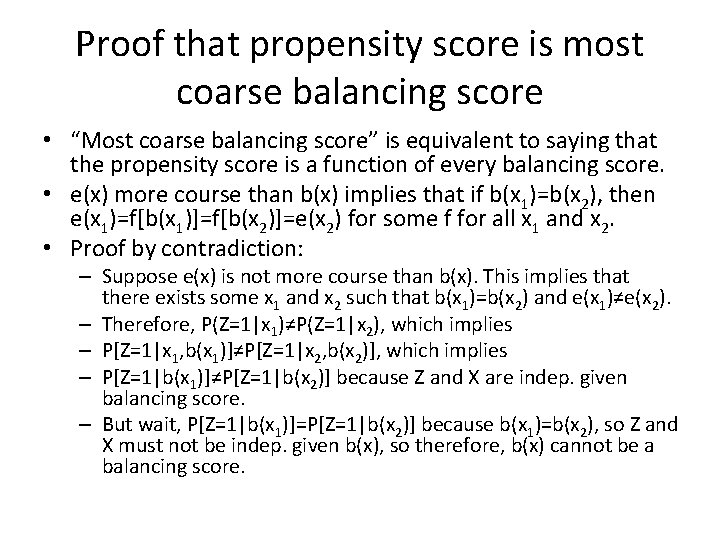
Proof that propensity score is most coarse balancing score • “Most coarse balancing score” is equivalent to saying that the propensity score is a function of every balancing score. • e(x) more course than b(x) implies that if b(x 1)=b(x 2), then e(x 1)=f[b(x 1)]=f[b(x 2)]=e(x 2) for some f for all x 1 and x 2. • Proof by contradiction: – Suppose e(x) is not more course than b(x). This implies that there exists some x 1 and x 2 such that b(x 1)=b(x 2) and e(x 1)≠e(x 2). – Therefore, P(Z=1|x 1)≠P(Z=1|x 2), which implies – P[Z=1|x 1, b(x 1)]≠P[Z=1|x 2, b(x 2)], which implies – P[Z=1|b(x 1)]≠P[Z=1|b(x 2)] because Z and X are indep. given balancing score. – But wait, P[Z=1|b(x 1)]=P[Z=1|b(x 2)] because b(x 1)=b(x 2), so Z and X must not be indep. given b(x), so therefore, b(x) cannot be a balancing score.
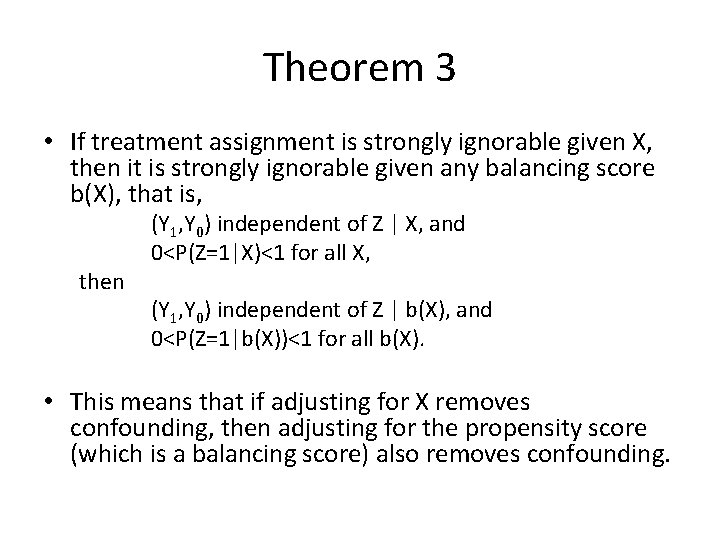
Theorem 3 • If treatment assignment is strongly ignorable given X, then it is strongly ignorable given any balancing score b(X), that is, then (Y 1, Y 0) independent of Z | X, and 0<P(Z=1|X)<1 for all X, (Y 1, Y 0) independent of Z | b(X), and 0<P(Z=1|b(X))<1 for all b(X). • This means that if adjusting for X removes confounding, then adjusting for the propensity score (which is a balancing score) also removes confounding.
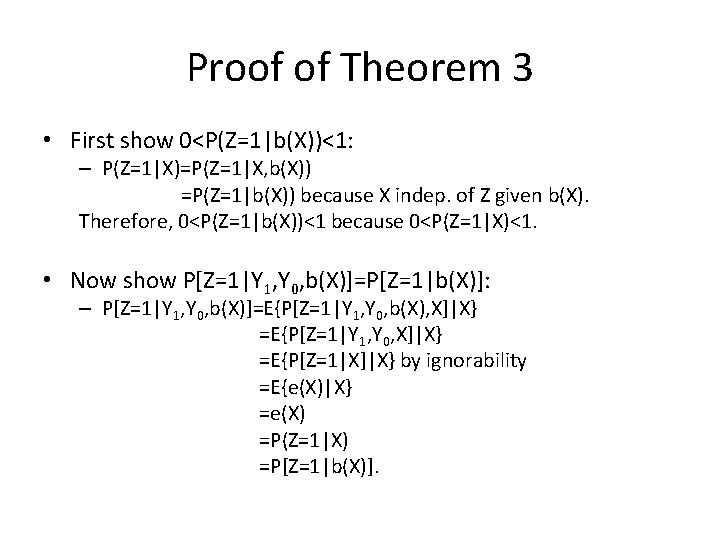
Proof of Theorem 3 • First show 0<P(Z=1|b(X))<1: – P(Z=1|X)=P(Z=1|X, b(X)) =P(Z=1|b(X)) because X indep. of Z given b(X). Therefore, 0<P(Z=1|b(X))<1 because 0<P(Z=1|X)<1. • Now show P[Z=1|Y 1, Y 0, b(X)]=P[Z=1|b(X)]: – P[Z=1|Y 1, Y 0, b(X)]=E{P[Z=1|Y 1, Y 0, b(X), X]|X} =E{P[Z=1|Y 1, Y 0, X]|X} =E{P[Z=1|X]|X} by ignorability =E{e(X)|X} =e(X) =P(Z=1|X) =P[Z=1|b(X)].
![Average Treatment Effect Theorem 4 EY 1 Y 0 is what we want Average Treatment Effect (Theorem 4) • E[Y 1 -Y 0] is what we want.](https://slidetodoc.com/presentation_image_h/93b73a7920f60b49bba5353472ad9b46/image-11.jpg)
Average Treatment Effect (Theorem 4) • E[Y 1 -Y 0] is what we want. • E[Y 1|Z=1]-E[Y 0|Z=0] is what we observe. • If treatment assignment is strongly ignorable given X, then E {E[Y 1|X, Z=1]-E[Y 0|X, Z=0]} =E{E[Y 1|X]-E[Y 0|X]} =E[Y 1 -Y 0]. • Similarly, E {E[Y 1|b(X), Z=1]-E[Y 0|b(X), Z=0]} =E{E[Y 1|b(X)]-E[Y 0|b(X)]} =E[Y 1 -Y 0].
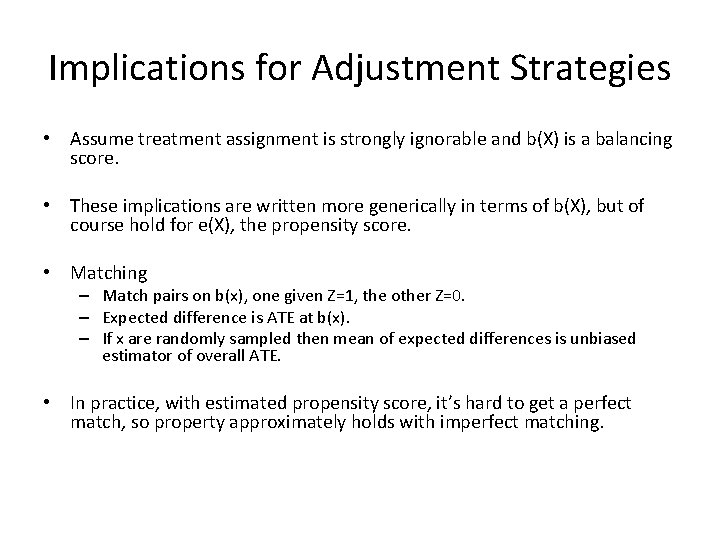
Implications for Adjustment Strategies • Assume treatment assignment is strongly ignorable and b(X) is a balancing score. • These implications are written more generically in terms of b(X), but of course hold for e(X), the propensity score. • Matching – Match pairs on b(x), one given Z=1, the other Z=0. – Expected difference is ATE at b(x). – If x are randomly sampled then mean of expected differences is unbiased estimator of overall ATE. • In practice, with estimated propensity score, it’s hard to get a perfect match, so property approximately holds with imperfect matching.
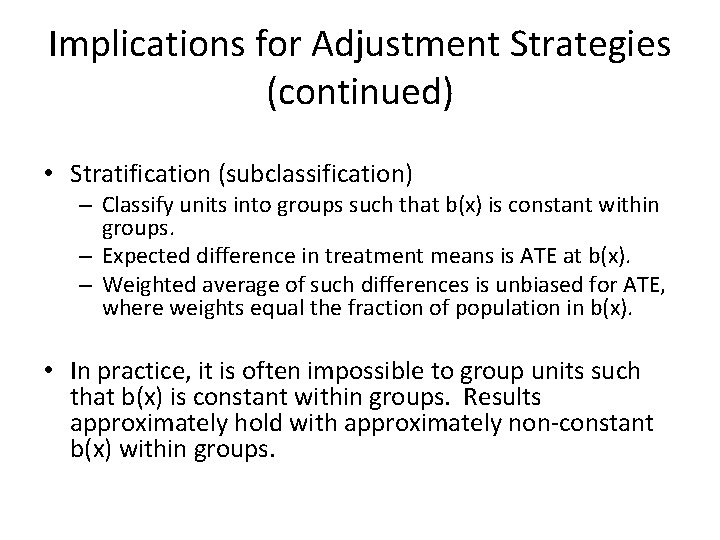
Implications for Adjustment Strategies (continued) • Stratification (subclassification) – Classify units into groups such that b(x) is constant within groups. – Expected difference in treatment means is ATE at b(x). – Weighted average of such differences is unbiased for ATE, where weights equal the fraction of population in b(x). • In practice, it is often impossible to group units such that b(x) is constant within groups. Results approximately hold with approximately non-constant b(x) within groups.
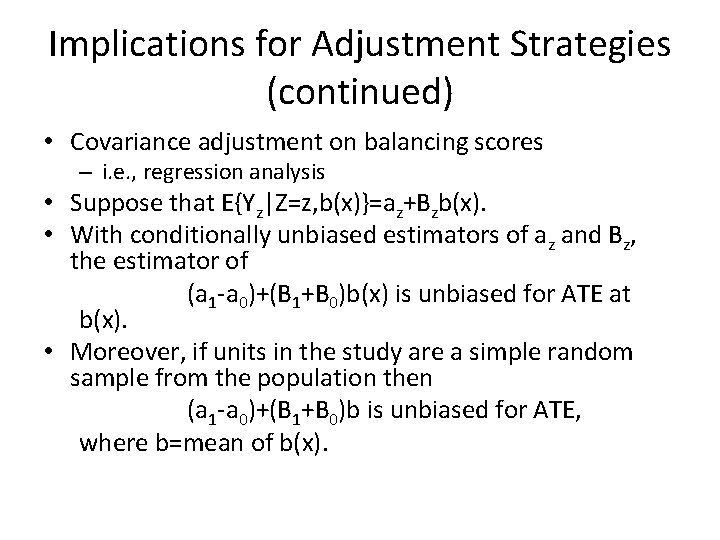
Implications for Adjustment Strategies (continued) • Covariance adjustment on balancing scores – i. e. , regression analysis • Suppose that E{Yz|Z=z, b(x)}=az+Bzb(x). • With conditionally unbiased estimators of az and Bz, the estimator of (a 1 -a 0)+(B 1+B 0)b(x) is unbiased for ATE at b(x). • Moreover, if units in the study are a simple random sample from the population then (a 1 -a 0)+(B 1+B 0)b is unbiased for ATE, where b=mean of b(x).
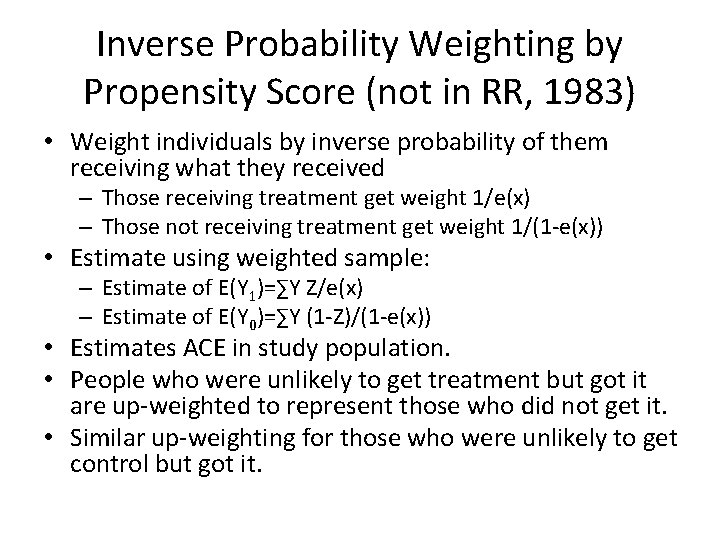
Inverse Probability Weighting by Propensity Score (not in RR, 1983) • Weight individuals by inverse probability of them receiving what they received – Those receiving treatment get weight 1/e(x) – Those not receiving treatment get weight 1/(1 -e(x)) • Estimate using weighted sample: – Estimate of E(Y 1)=∑Y Z/e(x) – Estimate of E(Y 0)=∑Y (1 -Z)/(1 -e(x)) • Estimates ACE in study population. • People who were unlikely to get treatment but got it are up-weighted to represent those who did not get it. • Similar up-weighting for those who were unlikely to get control but got it.
![Proof that IPW estimate is unbiased estimate of causal effect EY Z eX Proof that IPW estimate is unbiased estimate of causal effect • E[Y Z /e(X)]](https://slidetodoc.com/presentation_image_h/93b73a7920f60b49bba5353472ad9b46/image-16.jpg)
Proof that IPW estimate is unbiased estimate of causal effect • E[Y Z /e(X)] = E{E[Y Z /e(X) |X]} = E{E[Y 1|X] E[Z|X] /e(X)]} = E{E[Y 1|X]} = E[Y 1] Line 2 is by consistency, line 3 is by conditional ignorability, line 4 is by definition of propensity score; Proof similar for E{Y(1 -Z)/[1 -e(x)]}=E[Y 0].
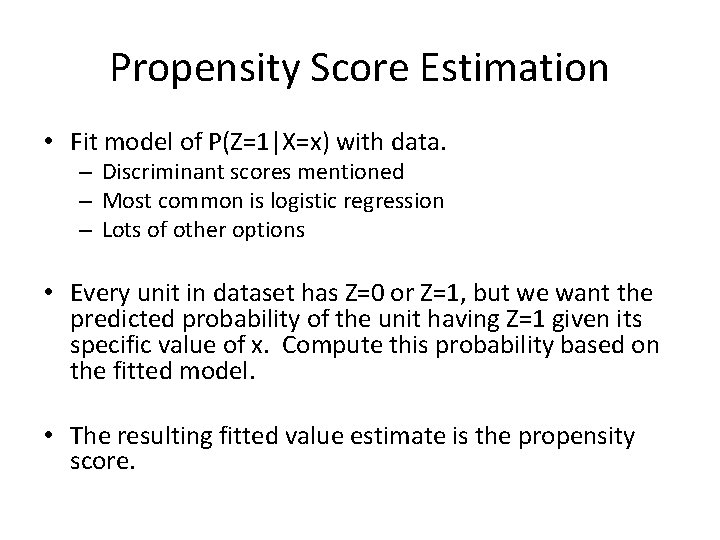
Propensity Score Estimation • Fit model of P(Z=1|X=x) with data. – Discriminant scores mentioned – Most common is logistic regression – Lots of other options • Every unit in dataset has Z=0 or Z=1, but we want the predicted probability of the unit having Z=1 given its specific value of x. Compute this probability based on the fitted model. • The resulting fitted value estimate is the propensity score.
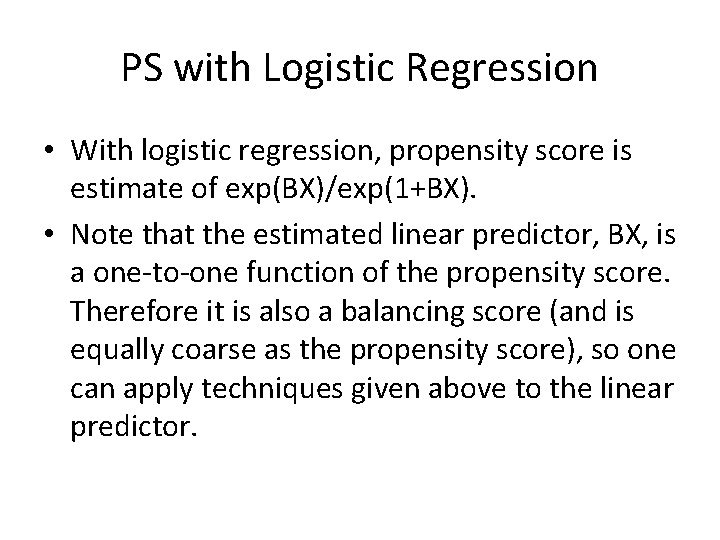
PS with Logistic Regression • With logistic regression, propensity score is estimate of exp(BX)/exp(1+BX). • Note that the estimated linear predictor, BX, is a one-to-one function of the propensity score. Therefore it is also a balancing score (and is equally coarse as the propensity score), so one can apply techniques given above to the linear predictor.
Propensity score theorem
Propensity model meaning
Government spending multiplier
Azure web role vs worker role
Krappmann schaubild
Role conflict occurs when fulfilling the role expectations
What is iscd
T score and z score difference
Hát kết hợp bộ gõ cơ thể
Slidetodoc
Bổ thể
Tỉ lệ cơ thể trẻ em
Gấu đi như thế nào
Thang điểm glasgow
Hát lên người ơi alleluia
Các môn thể thao bắt đầu bằng tiếng bóng
Thế nào là hệ số cao nhất
Các châu lục và đại dương trên thế giới
Cong thức tính động năng