CONSTRUCTING PROPENSITY SCORE WEIGHTED AND MATCHED SAMPLES Stacey
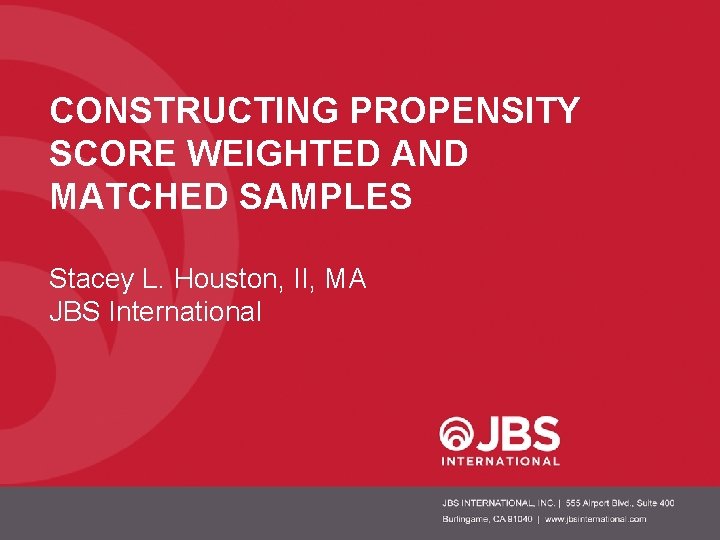
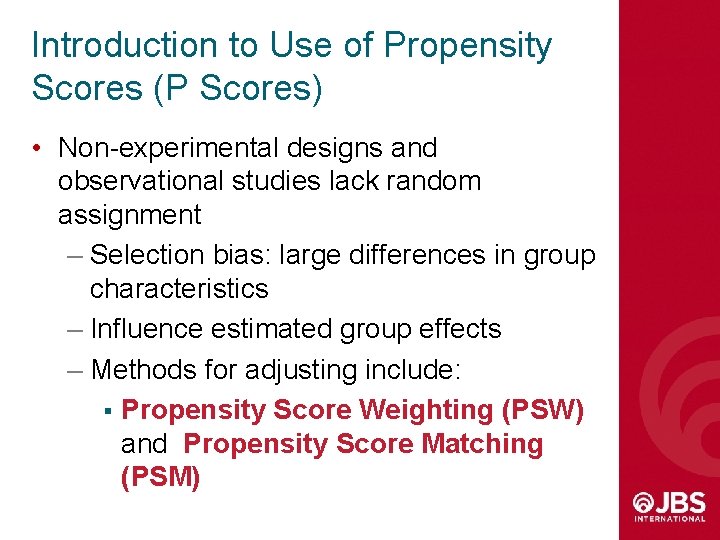
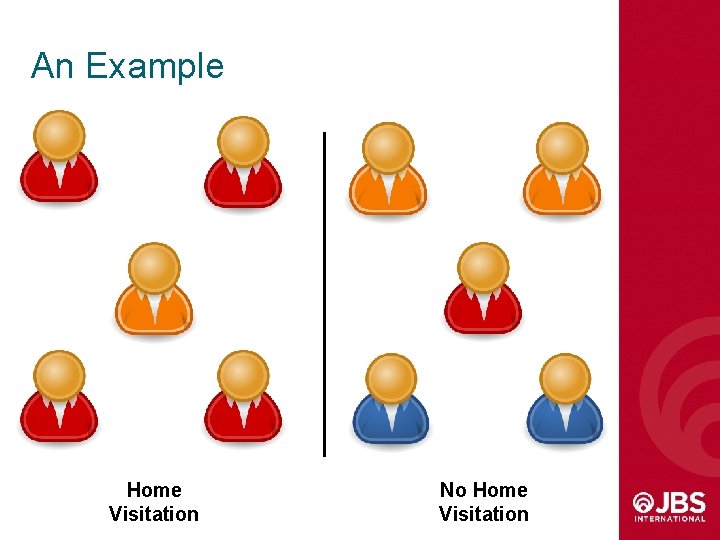
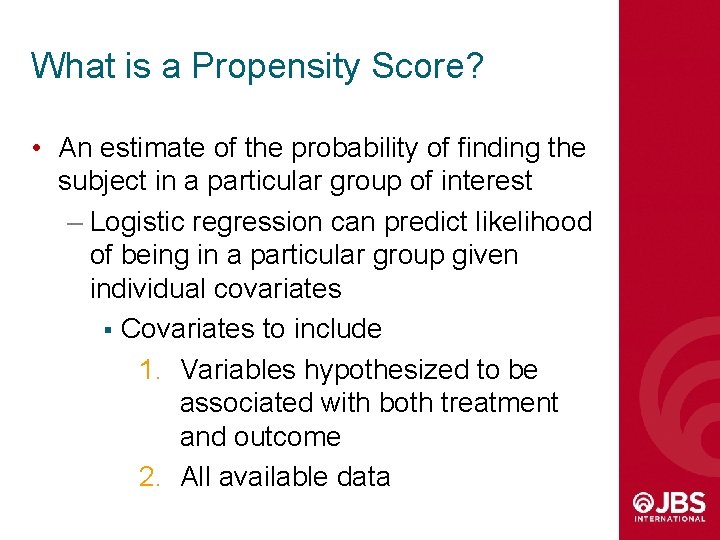
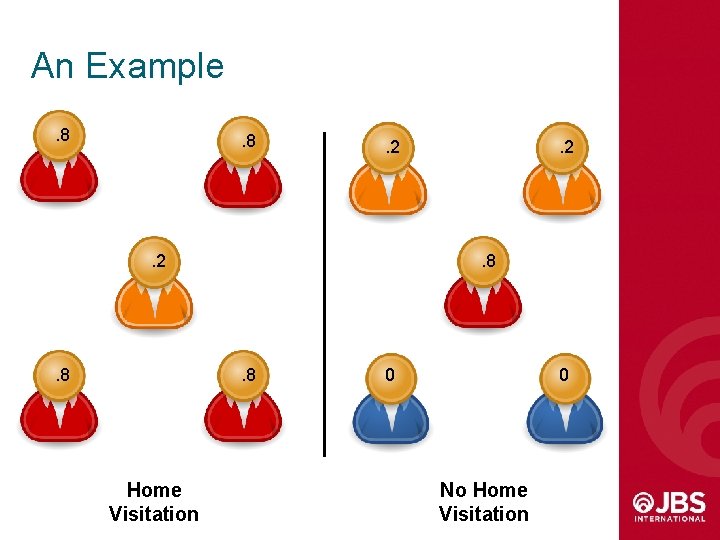
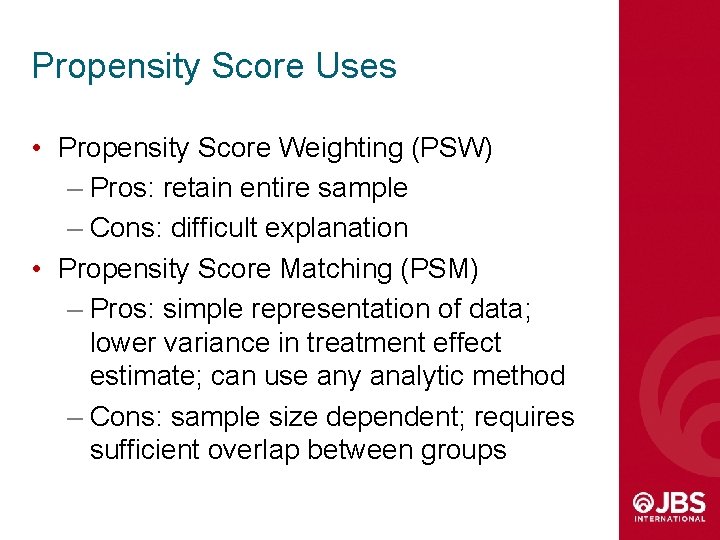
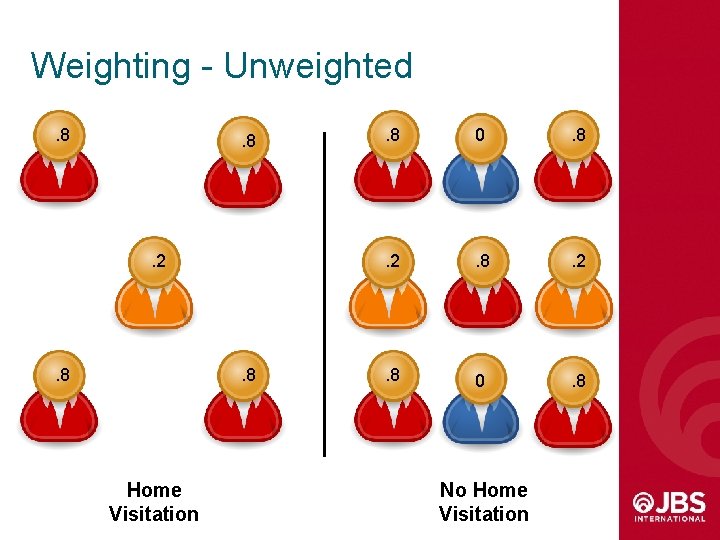
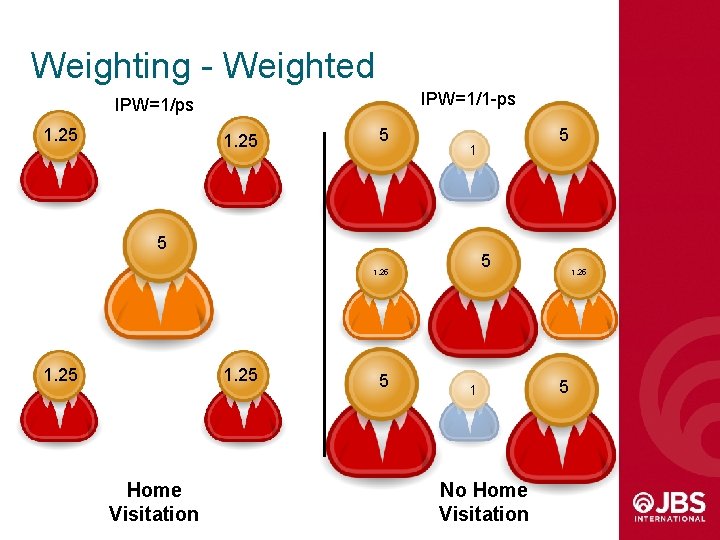
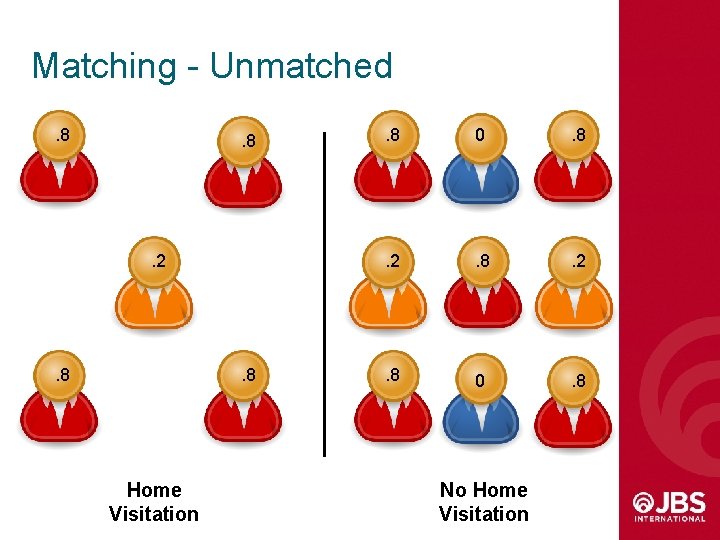
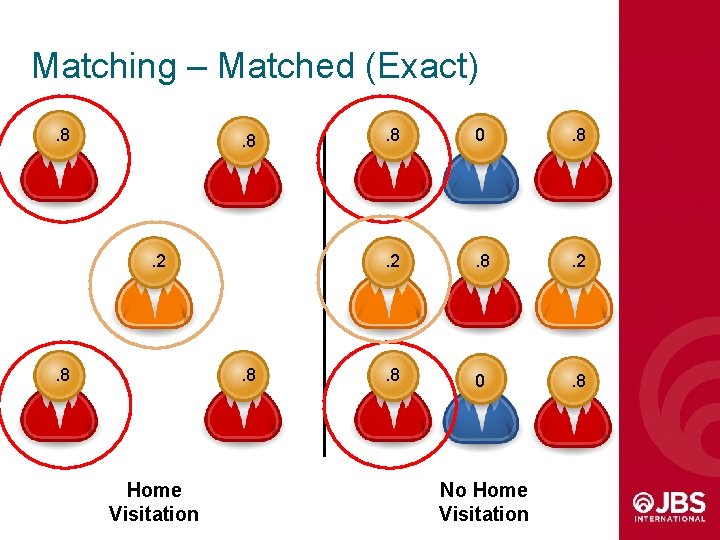
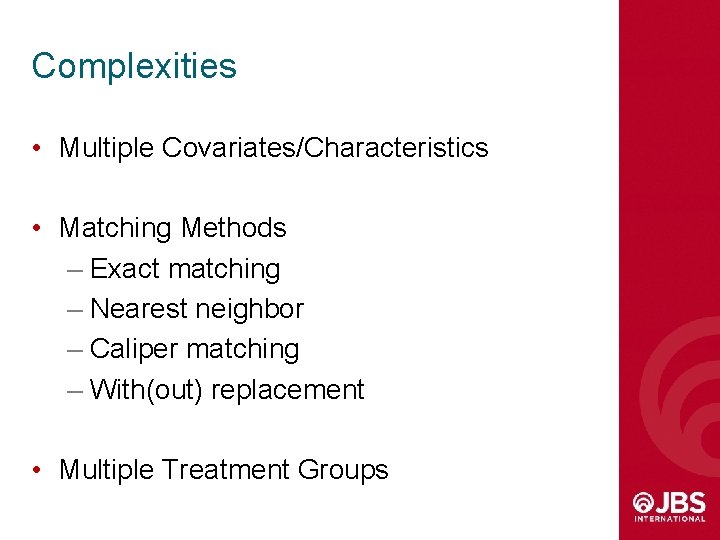
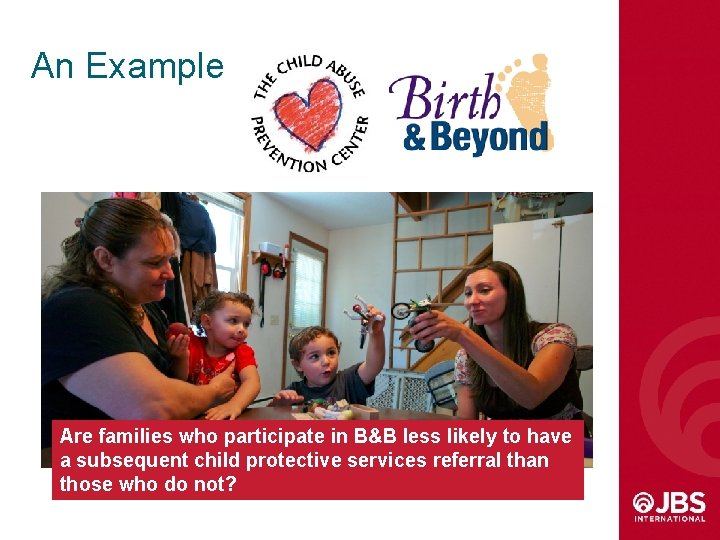
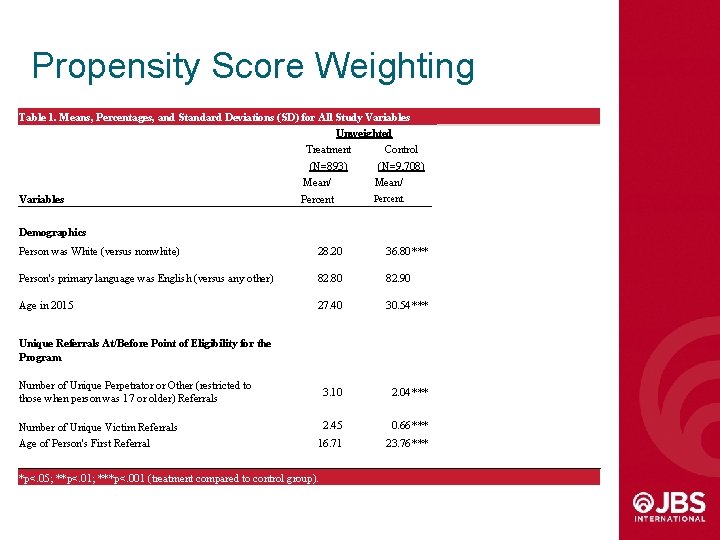
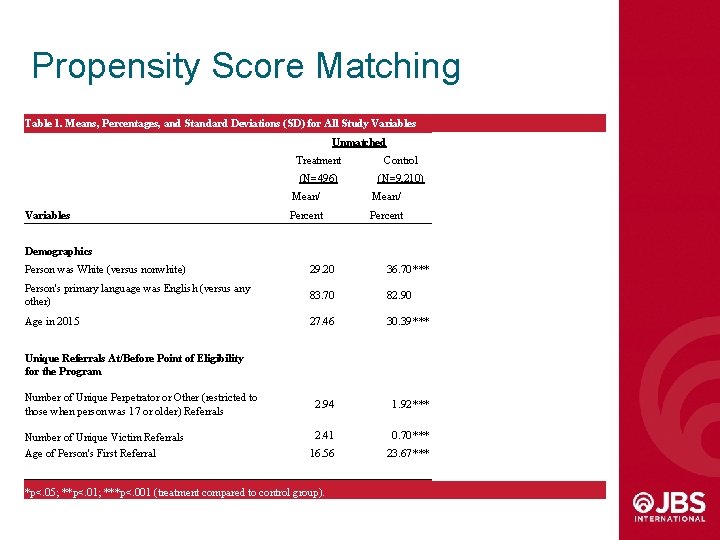
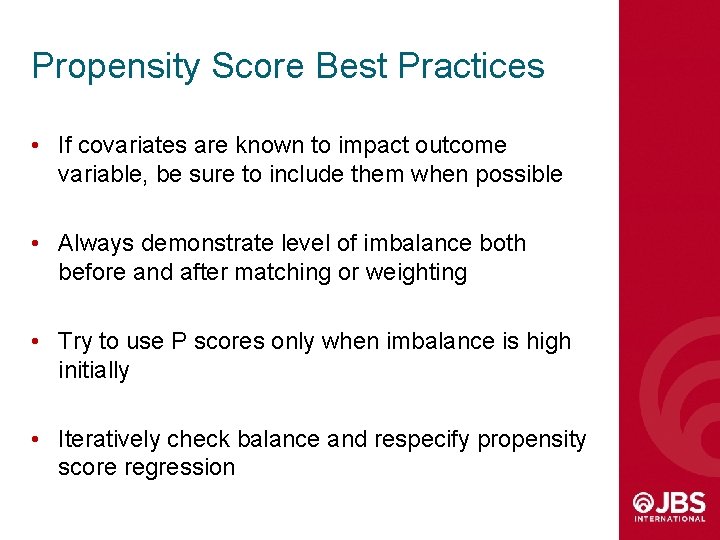
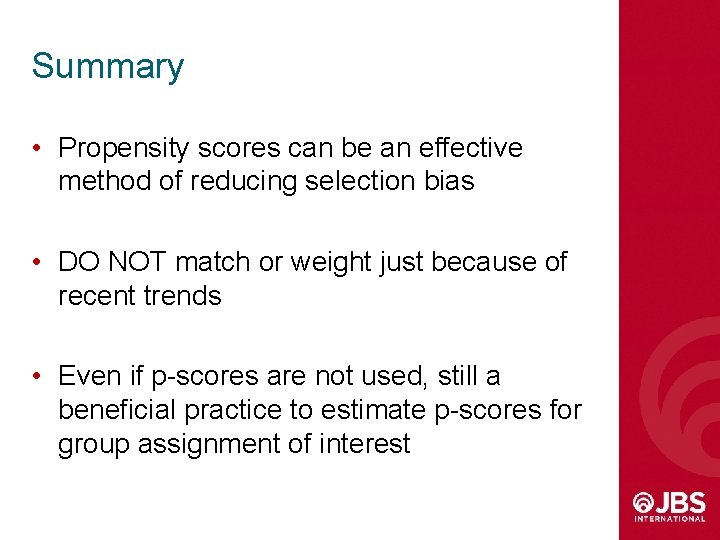
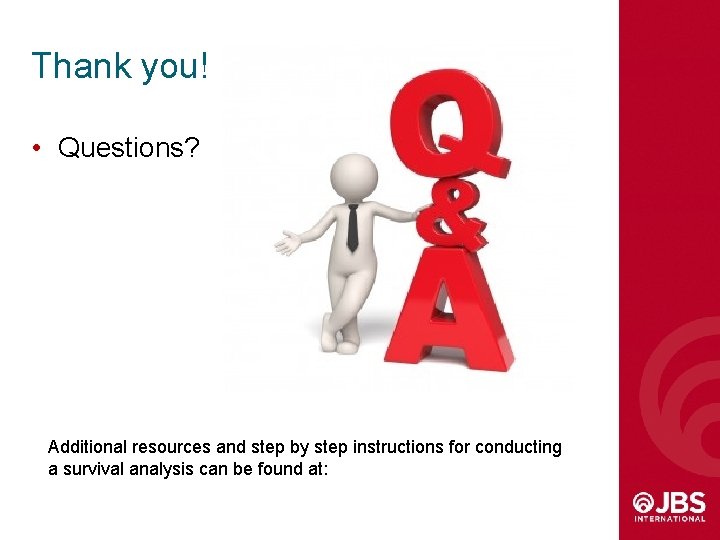
- Slides: 17
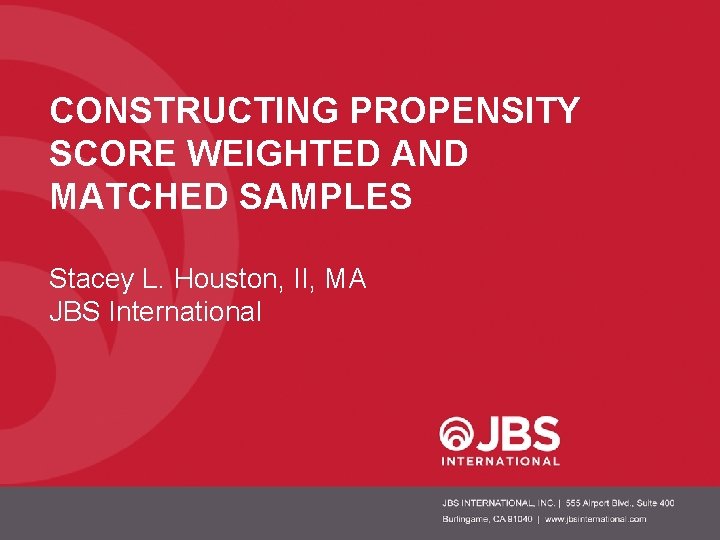
CONSTRUCTING PROPENSITY SCORE WEIGHTED AND MATCHED SAMPLES Stacey L. Houston, II, MA JBS International
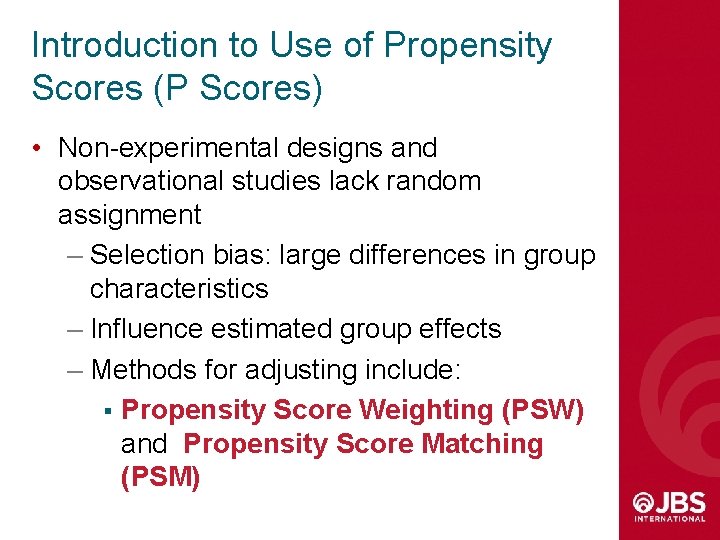
Introduction to Use of Propensity Scores (P Scores) • Non-experimental designs and observational studies lack random assignment – Selection bias: large differences in group characteristics – Influence estimated group effects – Methods for adjusting include: § Propensity Score Weighting (PSW) and Propensity Score Matching (PSM)
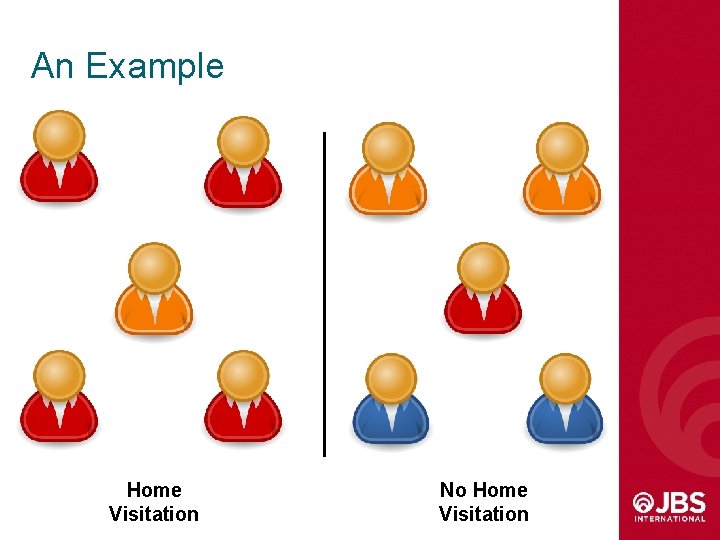
An Example Home Visitation No Home Visitation
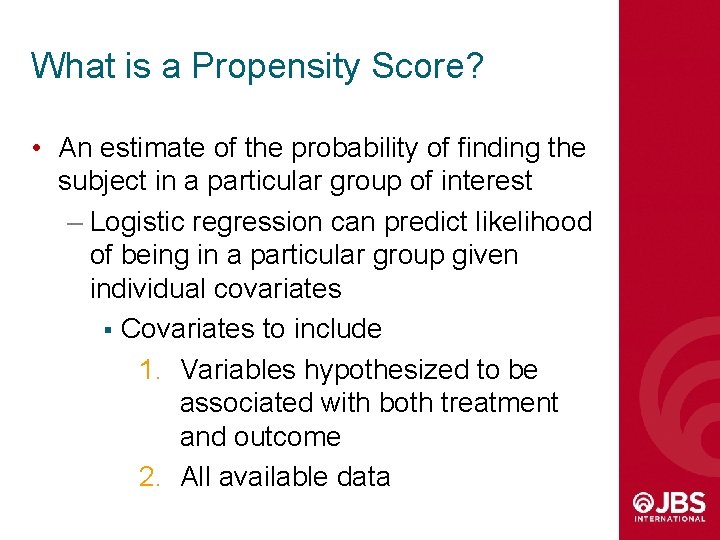
What is a Propensity Score? • An estimate of the probability of finding the subject in a particular group of interest – Logistic regression can predict likelihood of being in a particular group given individual covariates § Covariates to include 1. Variables hypothesized to be associated with both treatment and outcome 2. All available data
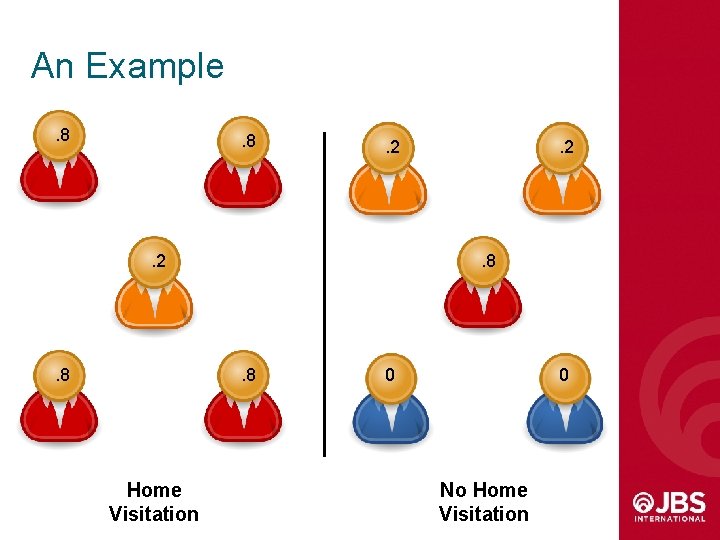
An Example. 8 . 2 . 8 . 8 Home Visitation . 2 0 0 No Home Visitation
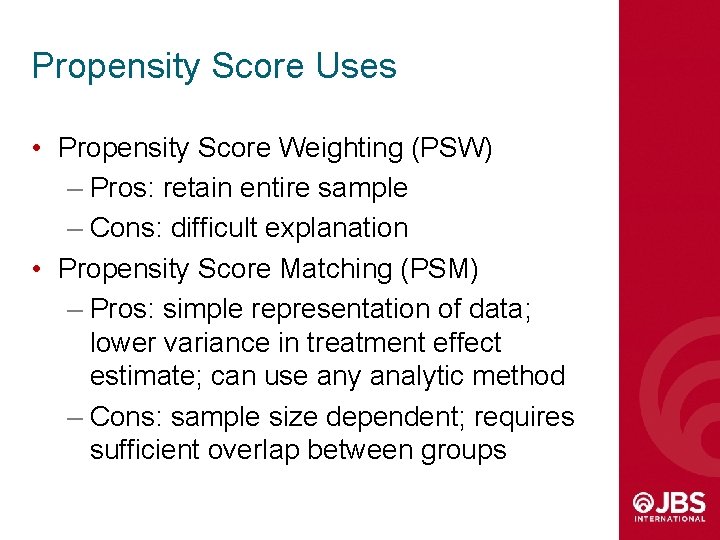
Propensity Score Uses • Propensity Score Weighting (PSW) – Pros: retain entire sample – Cons: difficult explanation • Propensity Score Matching (PSM) – Pros: simple representation of data; lower variance in treatment effect estimate; can use any analytic method – Cons: sample size dependent; requires sufficient overlap between groups
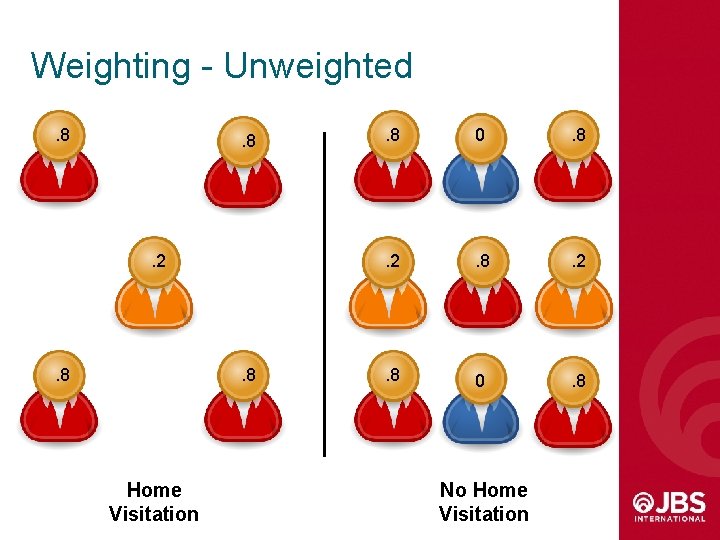
Weighting - Unweighted. 8 . 2 . 8 Home Visitation . 8 0 . 8 . 2 . 8 0 . 8 No Home Visitation
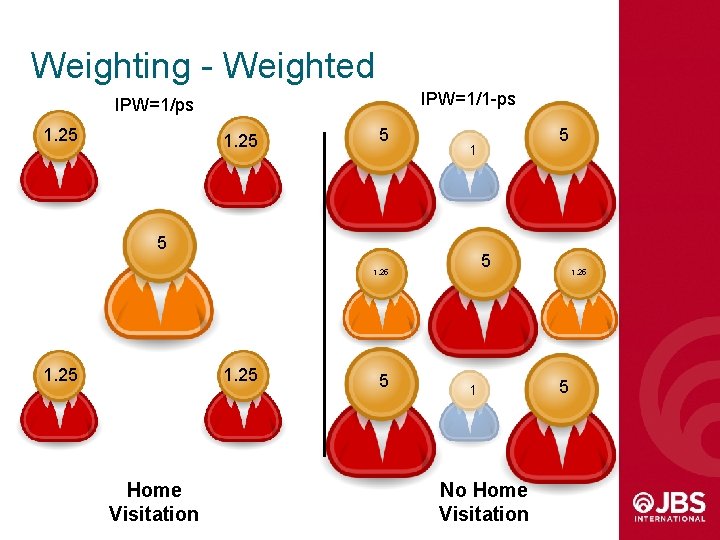
Weighting - Weighted IPW=1/1 -ps IPW=1/ps 1. 25 5 5 5 1. 25 Home Visitation 5 5 1 1 No Home Visitation 1. 25 5
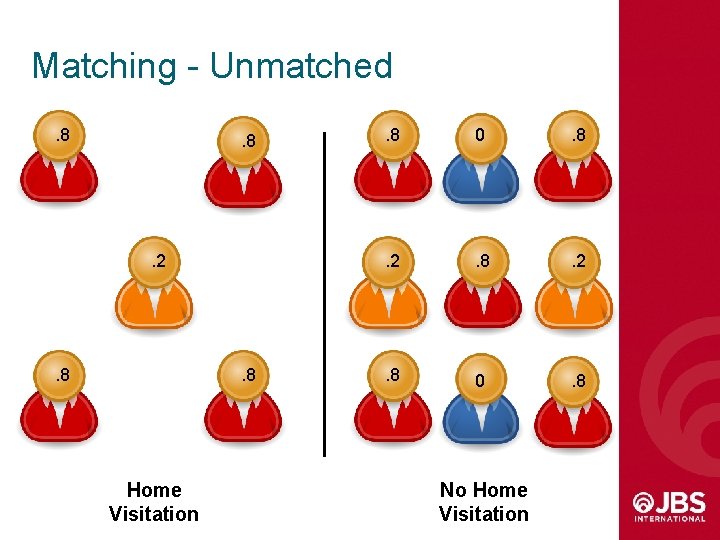
Matching - Unmatched. 8 . 2 . 8 Home Visitation . 8 0 . 8 . 2 . 8 0 . 8 No Home Visitation
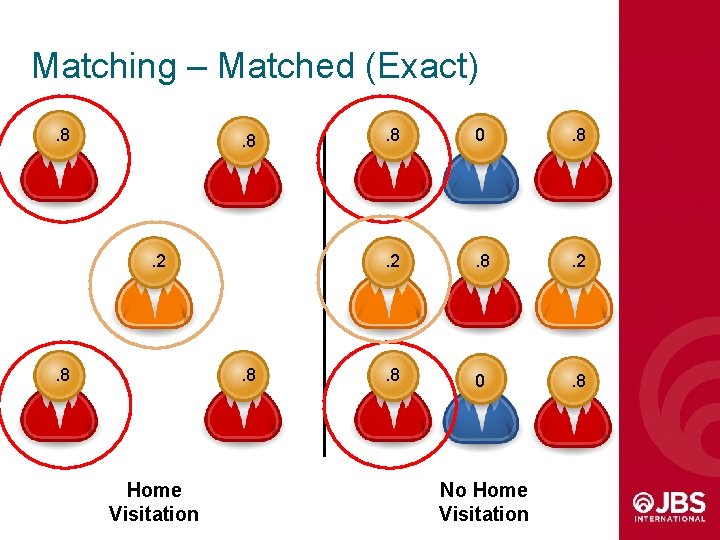
Matching – Matched (Exact). 8 . 2 . 8 Home Visitation . 8 0 . 8 . 2 . 8 0 . 8 No Home Visitation
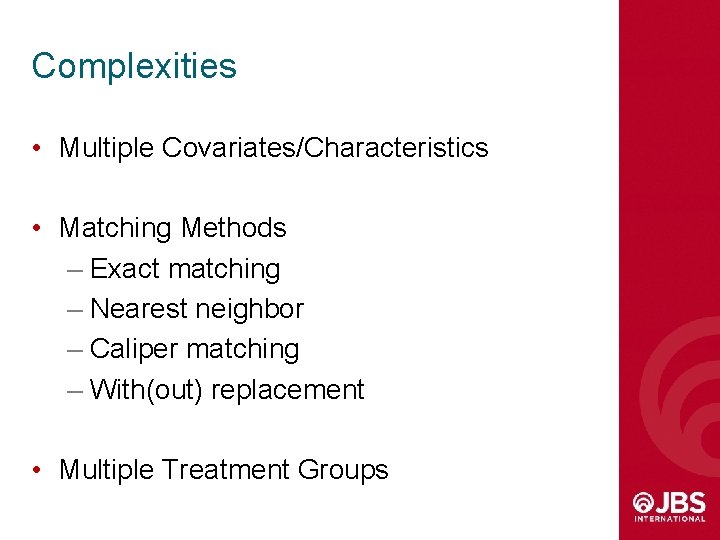
Complexities • Multiple Covariates/Characteristics • Matching Methods – Exact matching – Nearest neighbor – Caliper matching – With(out) replacement • Multiple Treatment Groups
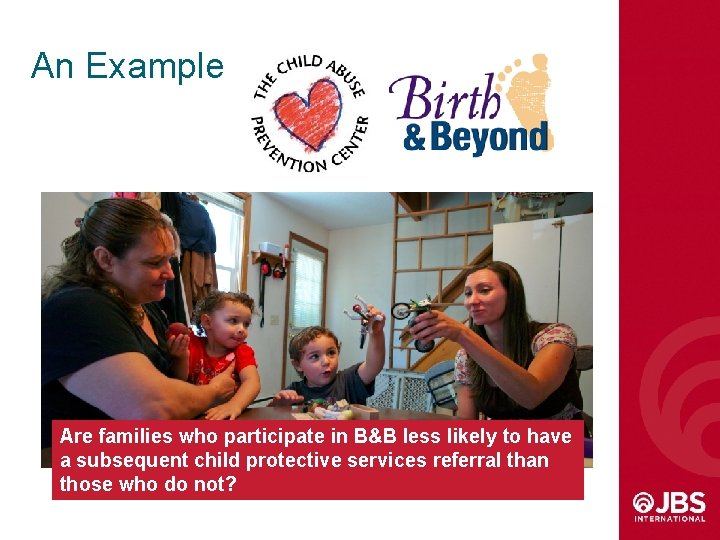
An Example Are families who participate in B&B less likely to have a subsequent child protective services referral than those who do not?
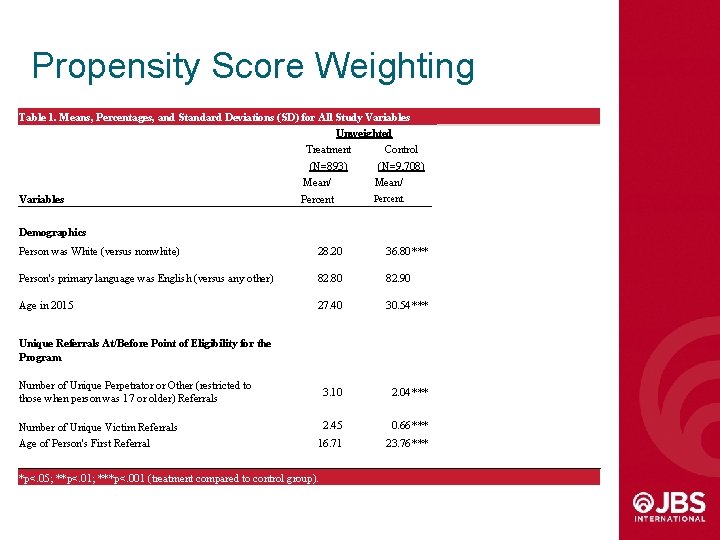
Propensity Score Weighting Table 1. Means, Percentages, and Standard Deviations (SD) for All Study Variables Unweighted Treatment Control (N=893) (N=9, 708) Variables Propensity Score Weighted Treatment Control (N=893) (N=9, 708) Mean/ Percent Demographics Person was White (versus nonwhite) 28. 20 36. 80 *** 37. 40 Person's primary language was English (versus any other) 82. 80 82. 90 81. 40 82. 90 Age in 2015 27. 40 30. 54 *** 30. 94 30. 29 ** 3. 10 2. 04 *** 2. 41 2. 14 ** 2. 45 0. 66 *** 0. 81 0. 82 16. 71 23. 76 *** 23. 61 23. 17 Unique Referrals At/Before Point of Eligibility for the Program Number of Unique Perpetrator or Other (restricted to those when person was 17 or older) Referrals Number of Unique Victim Referrals Age of Person's First Referral *p<. 05; **p<. 01; ***p<. 001 (treatment compared to control group). 36. 10
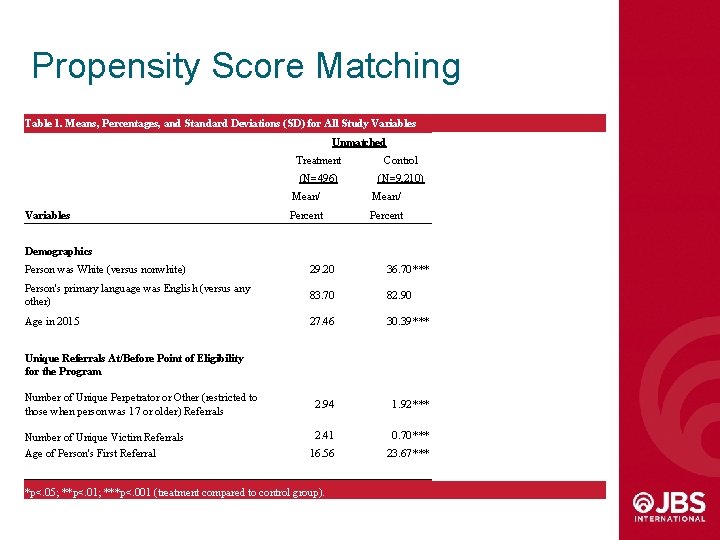
Propensity Score Matching Table 1. Means, Percentages, and Standard Deviations (SD) for All Study Variables Unmatched Treatment Control (N=496) (N=9, 210) (N=493) (N=985) Mean/ Variables Matched 2: 1 Percent Mean/ Percent Demographics Person was White (versus nonwhite) 29. 20 36. 70 *** 29. 40 Person's primary language was English (versus any other) 83. 70 82. 90 83. 60 87. 00 Age in 2015 27. 46 30. 39 *** 27. 48 27. 54 Unique Referrals At/Before Point of Eligibility for the Program Number of Unique Perpetrator or Other (restricted to those when person was 17 or older) Referrals Number of Unique Victim Referrals Age of Person's First Referral 30. 60 2. 94 1. 92 *** 2. 87 2. 61 2. 41 0. 70 *** 2. 35 16. 56 23. 67 *** 16. 63 16. 62 *p<. 05; **p<. 01; ***p<. 001 (treatment compared to control group).
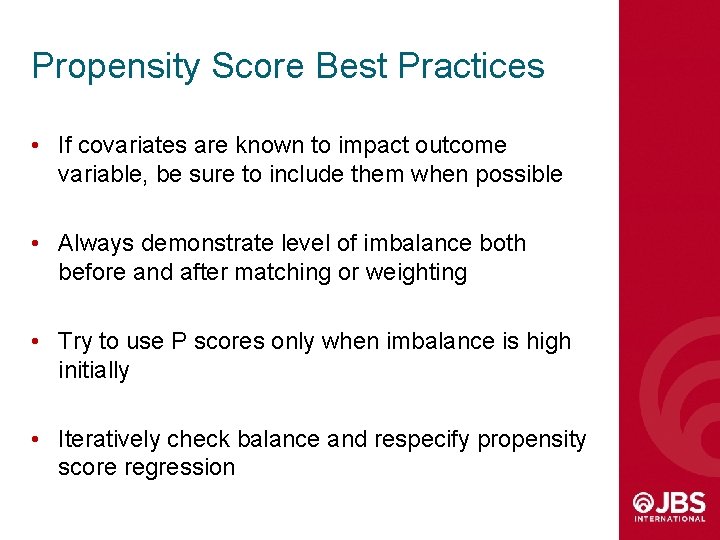
Propensity Score Best Practices • If covariates are known to impact outcome variable, be sure to include them when possible • Always demonstrate level of imbalance both before and after matching or weighting • Try to use P scores only when imbalance is high initially • Iteratively check balance and respecify propensity score regression
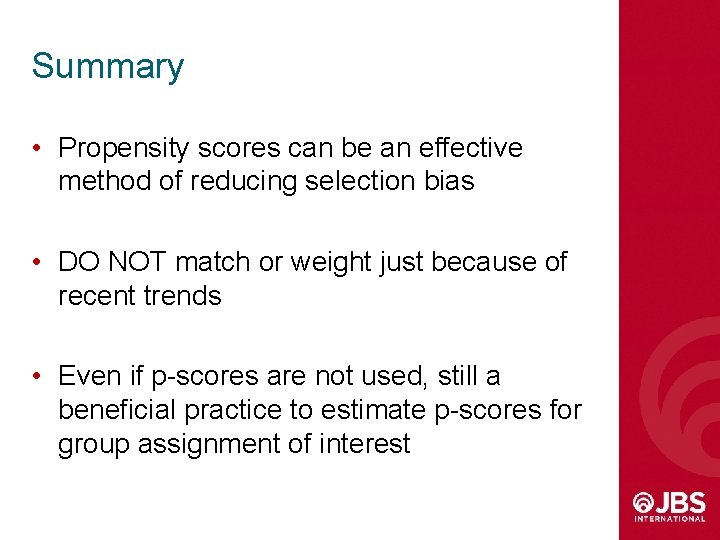
Summary • Propensity scores can be an effective method of reducing selection bias • DO NOT match or weight just because of recent trends • Even if p-scores are not used, still a beneficial practice to estimate p-scores for group assignment of interest
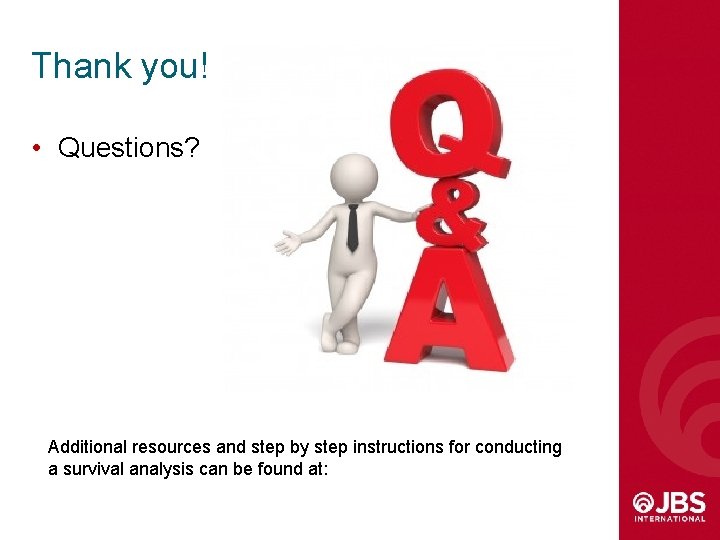
Thank you! • Questions? Additional resources and step by step instructions for conducting a survival analysis can be found at:
Propensity score theorem
Matched groups design
Weighted and non weighted binary codes
Propensity model meaning
Marginal propensity to consume graph
T-score statistics
The art and science of designing and constructing buildings
Repeated measures design psychology
Subjunctive with adverbial clauses
Stacey lambour
Stacey riddell
Matrice di stacey
Stacey barta
What bridge is the strongest
Stacey cozart
Alice ann stacey
Gas guzzler tax
Purdue math