Testing means part II The paired ttest Outline
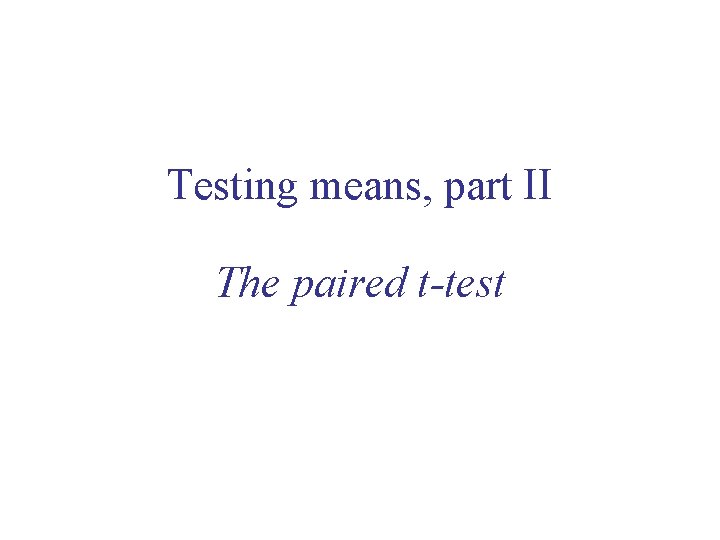
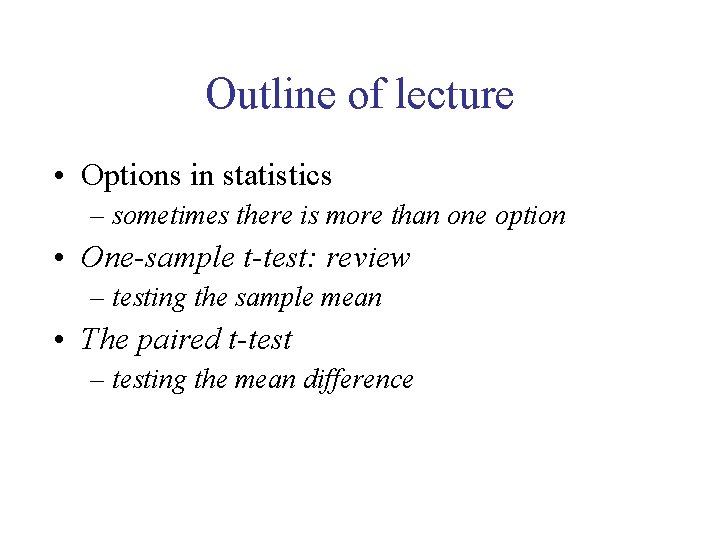
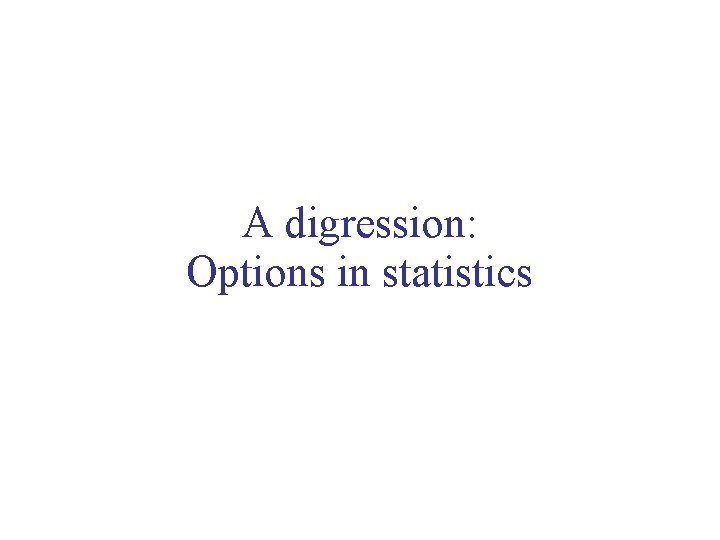
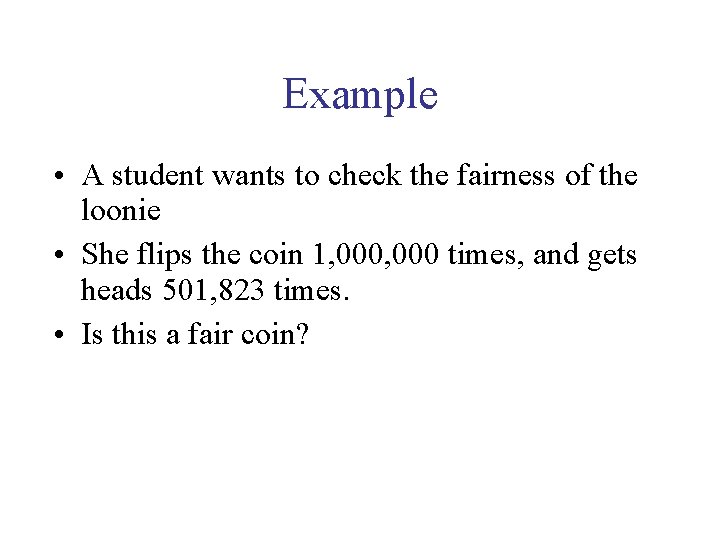
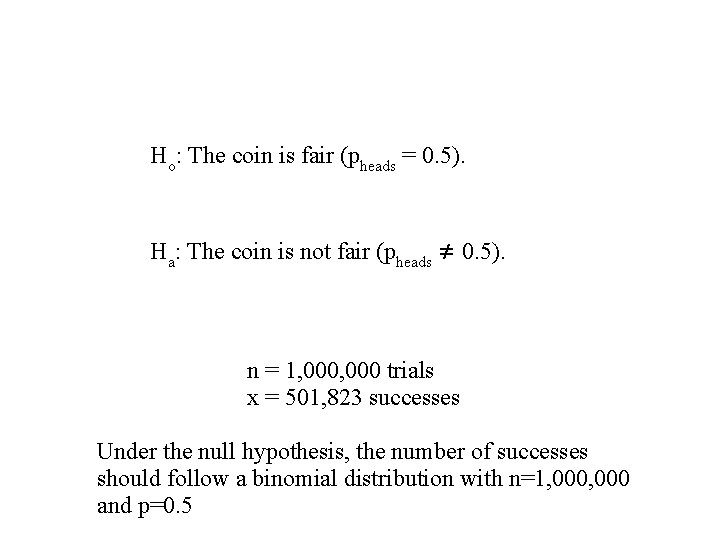
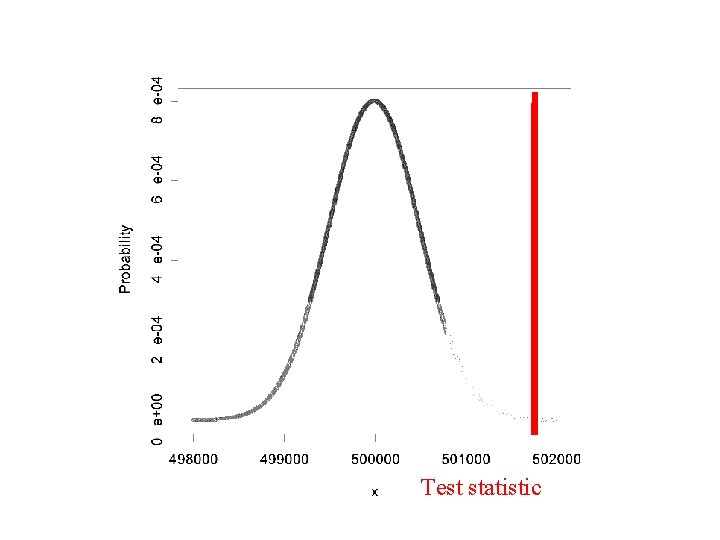
![Binomial test • P = 2*Pr[X≥ 501, 823] P = 2*(Pr[X = 501, 823] Binomial test • P = 2*Pr[X≥ 501, 823] P = 2*(Pr[X = 501, 823]](https://slidetodoc.com/presentation_image_h/141b123dadac6a2b615b159589fc3f1d/image-7.jpg)
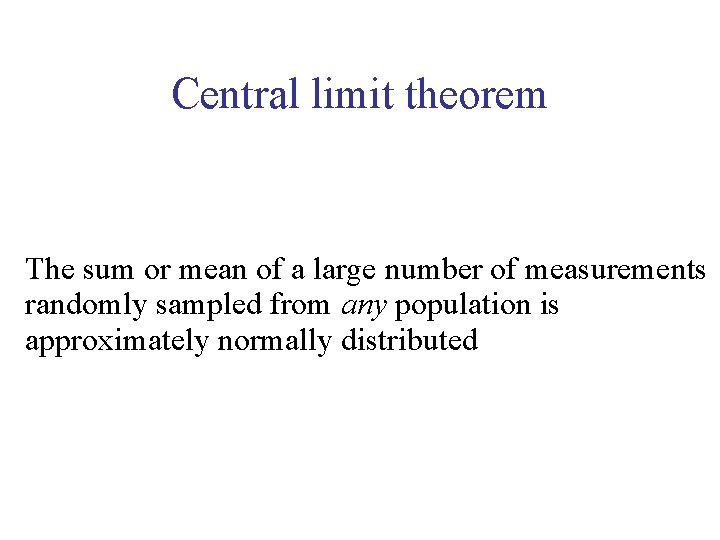
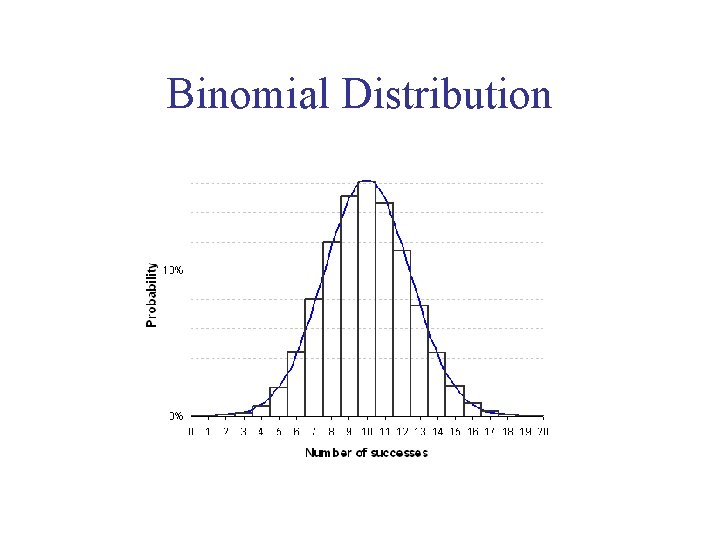
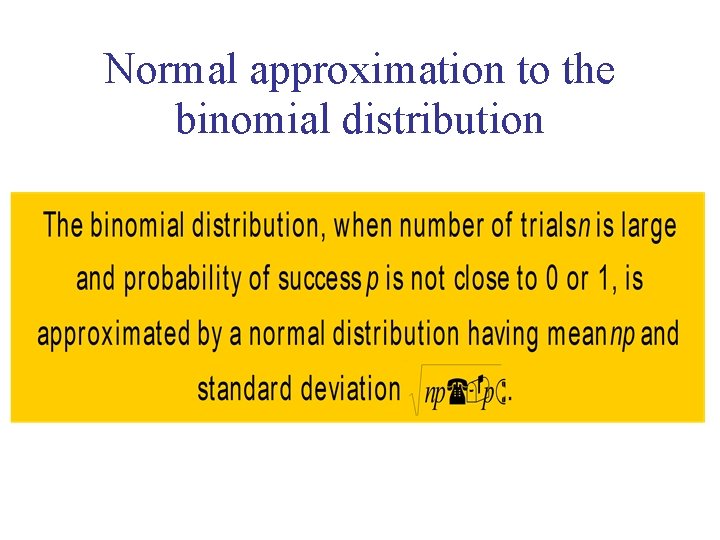
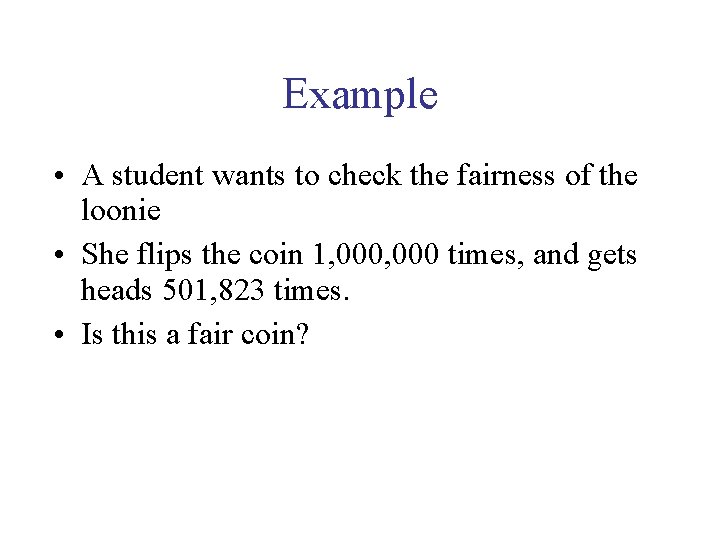
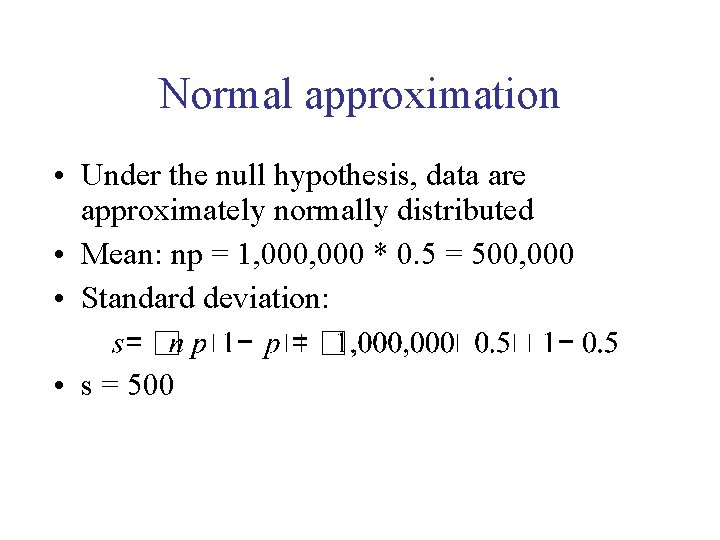
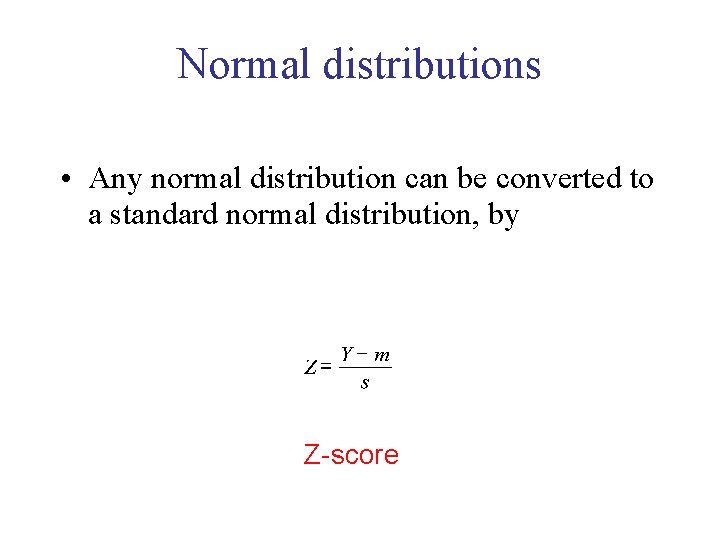
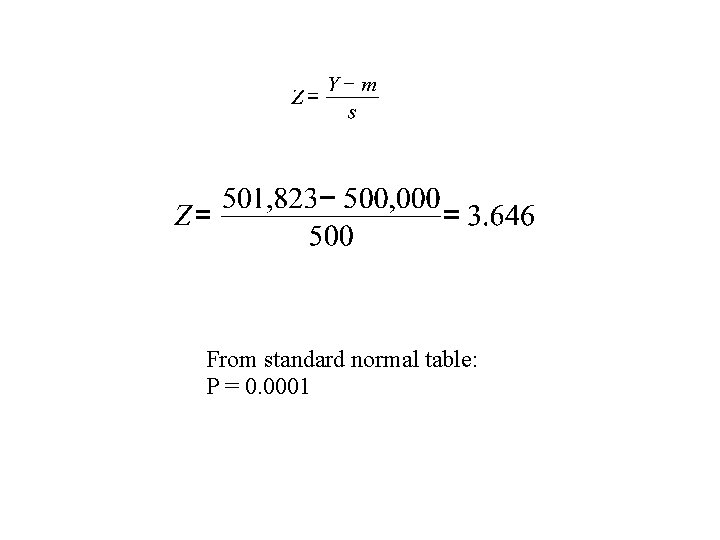
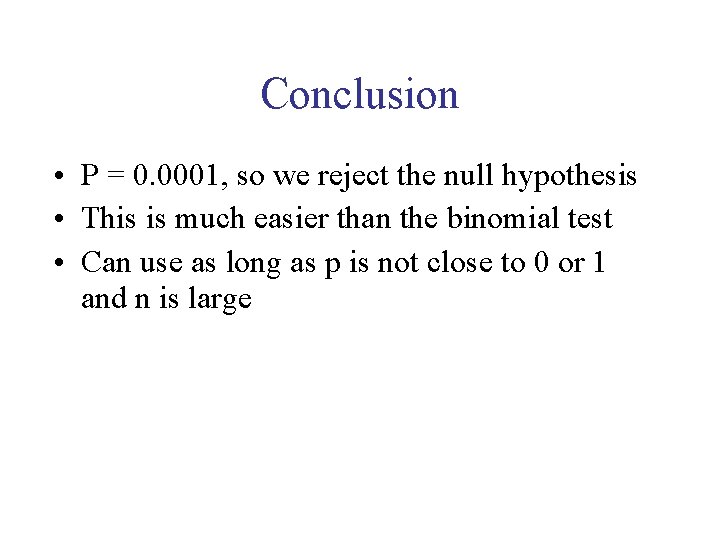
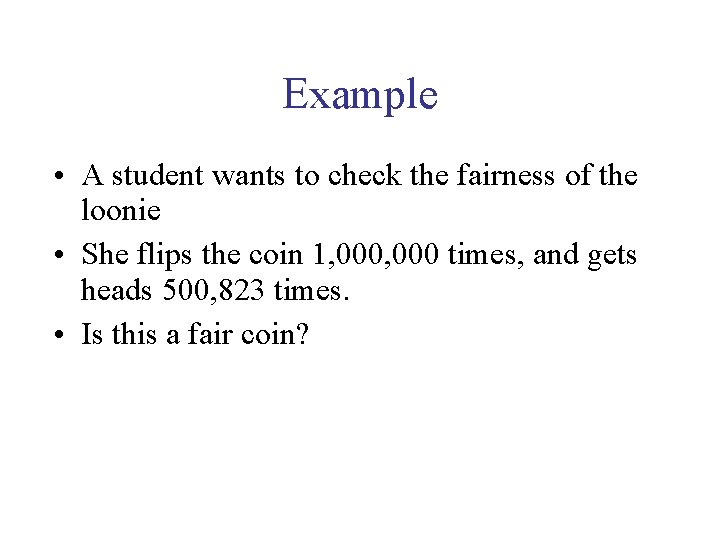
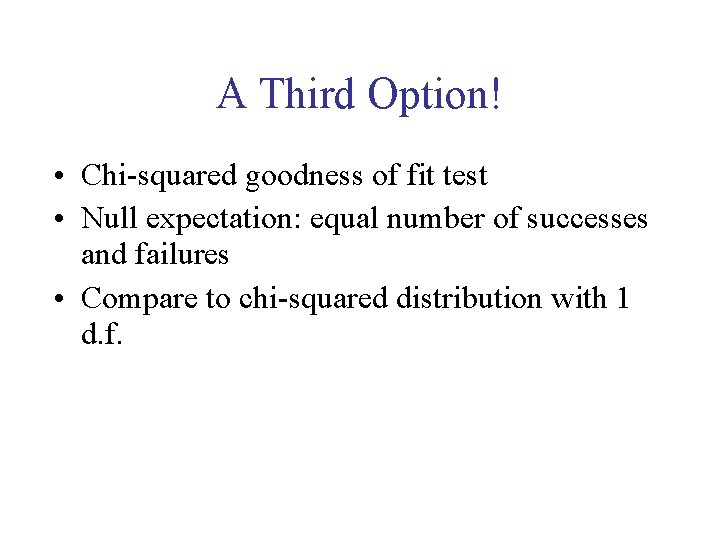
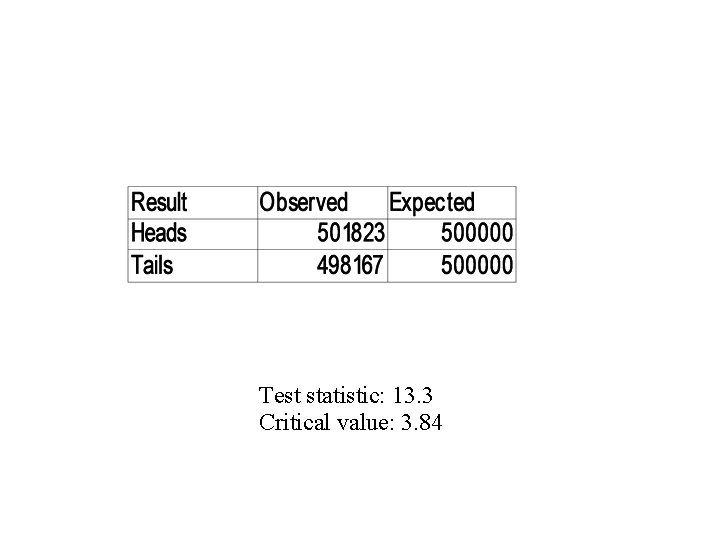
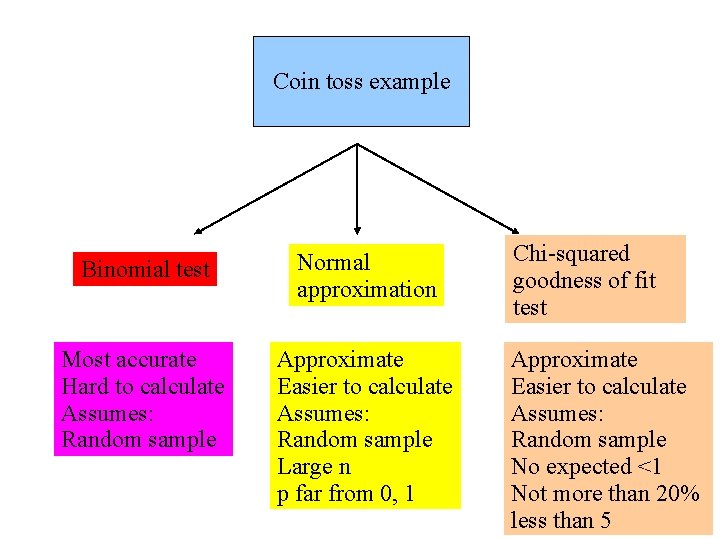
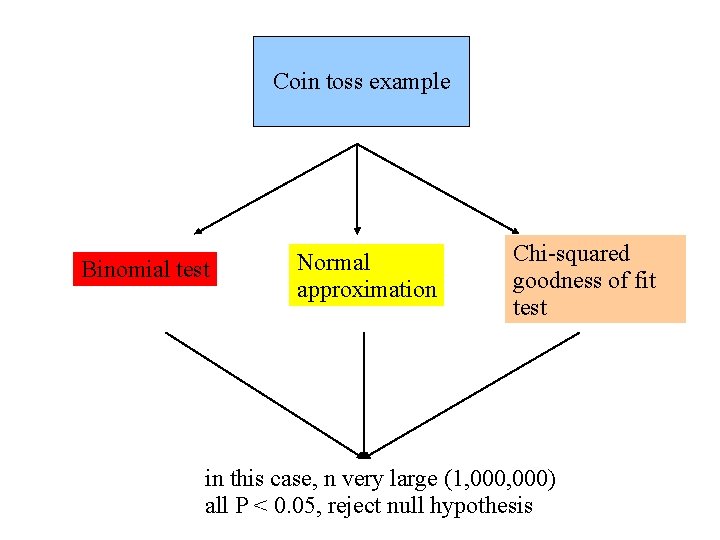
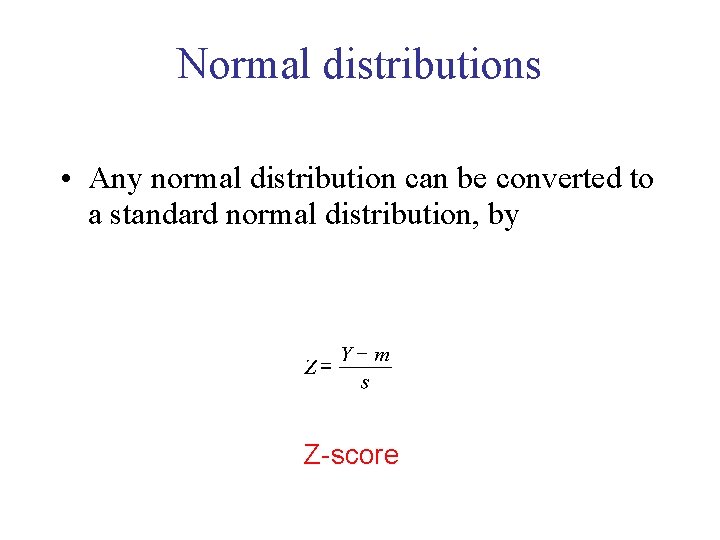
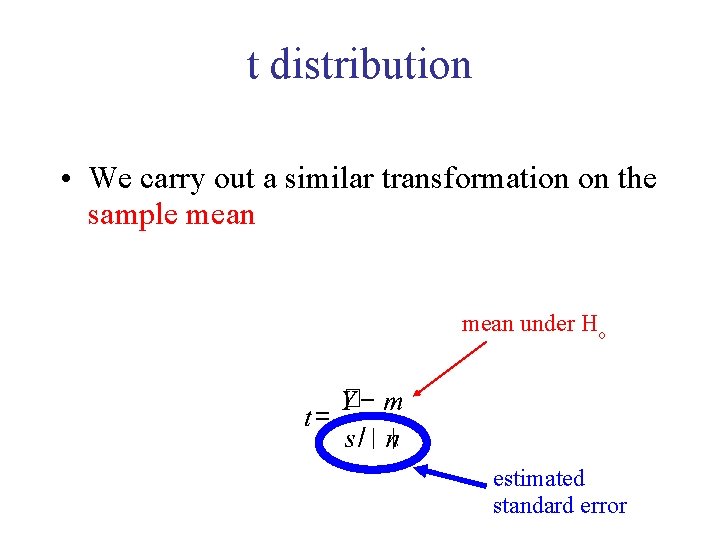
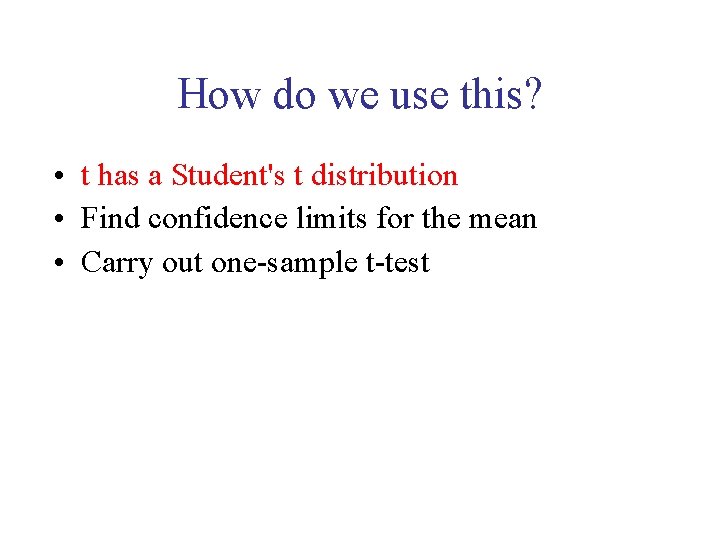
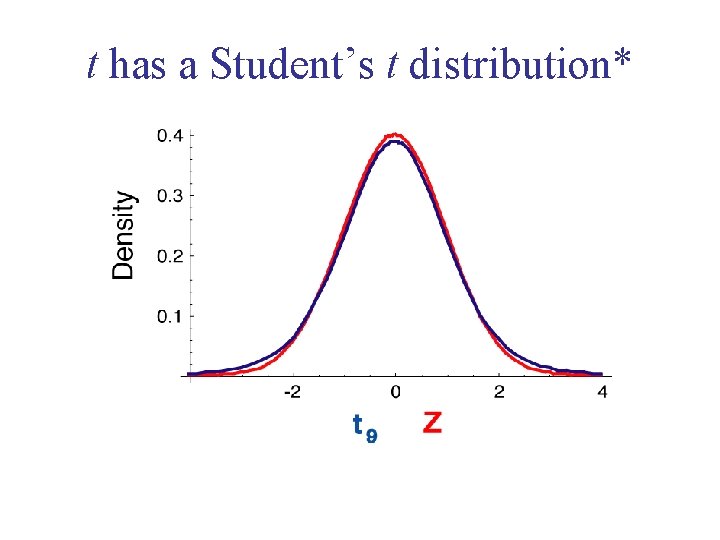
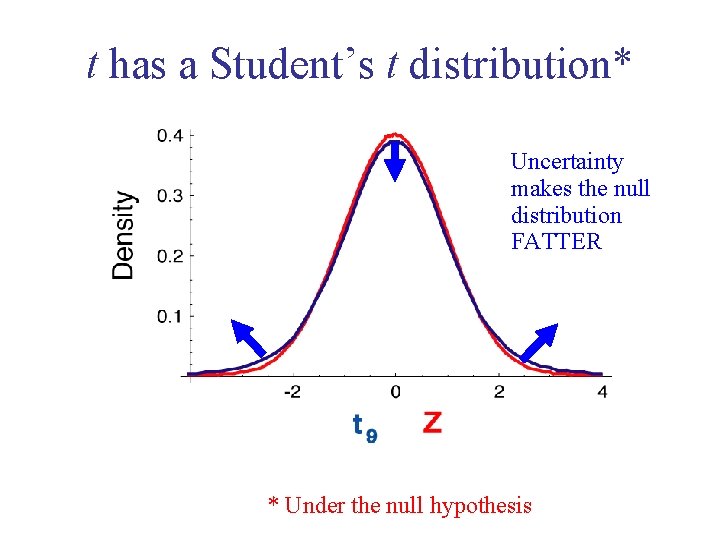
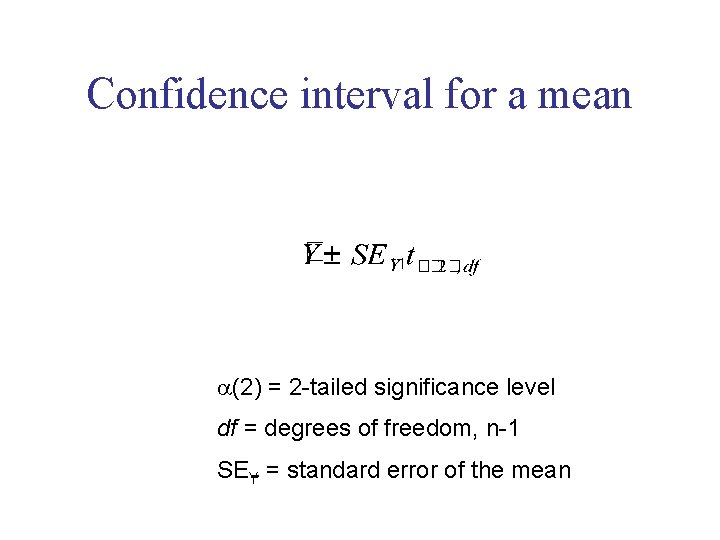
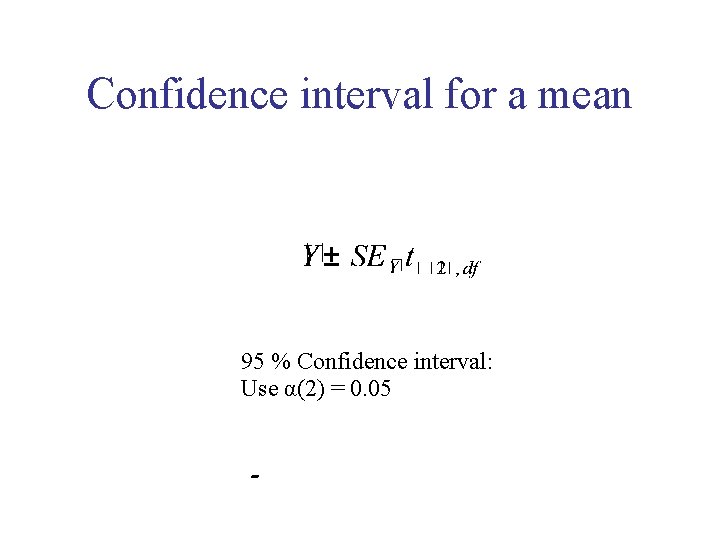
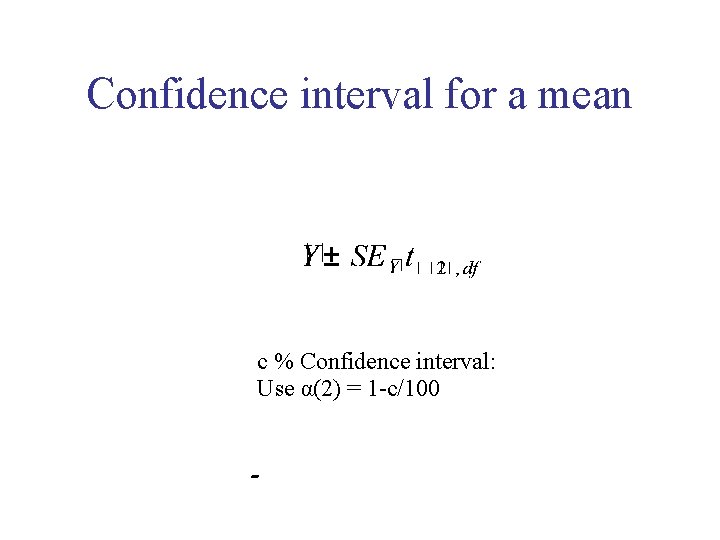
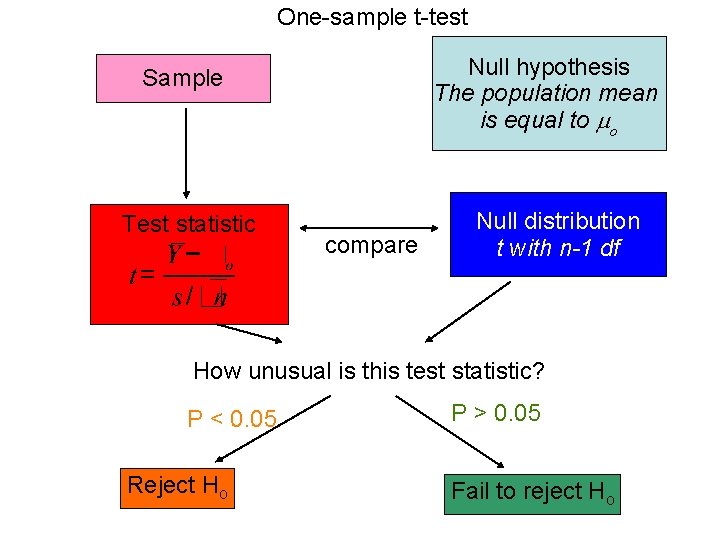
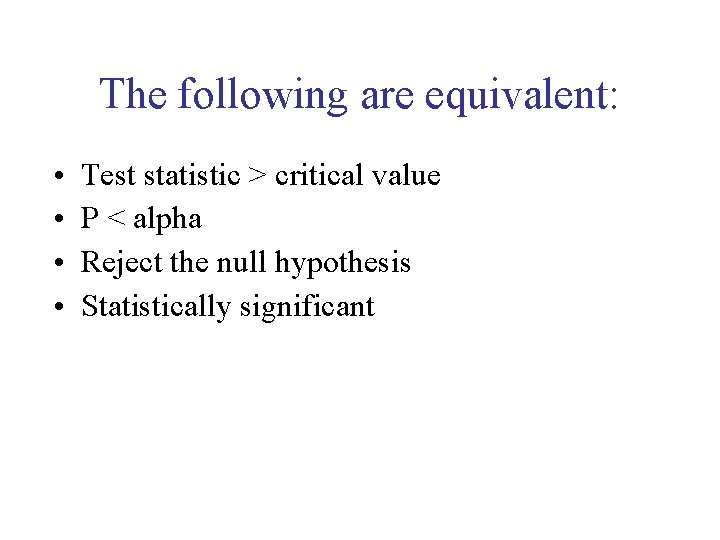
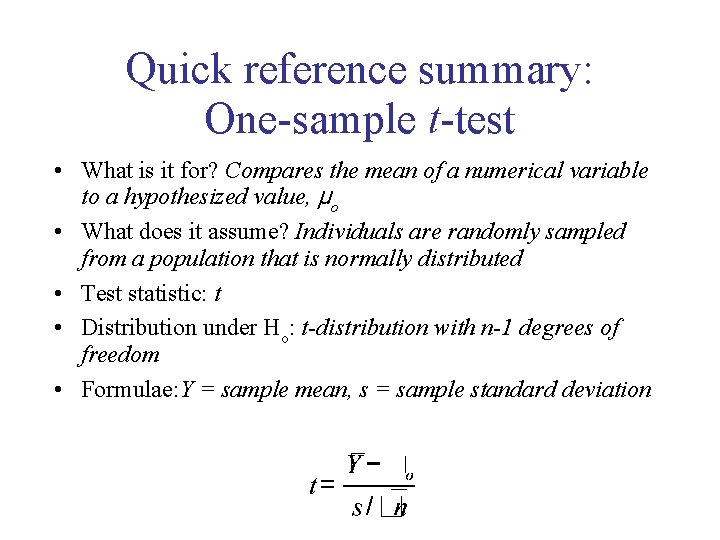
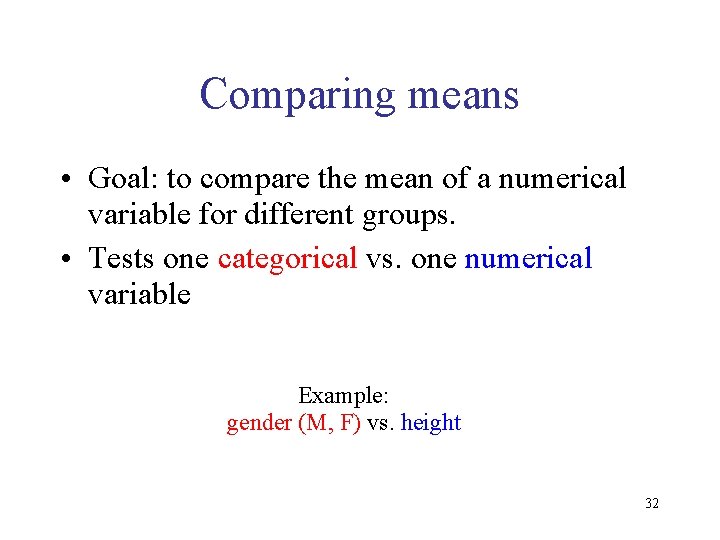
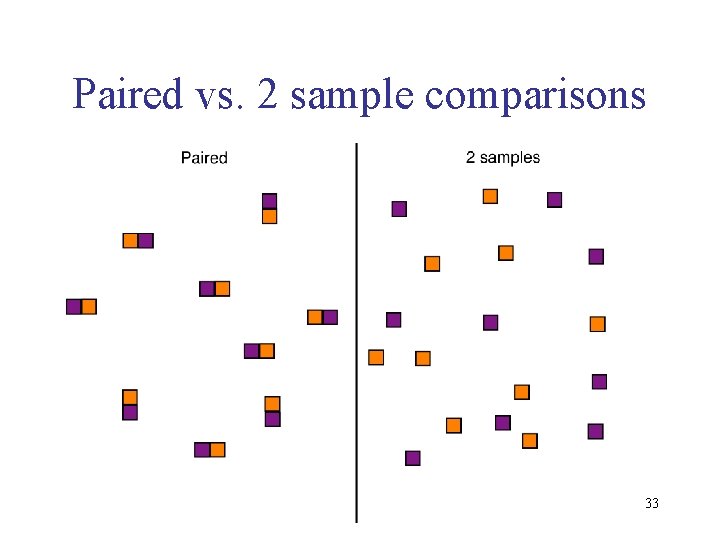
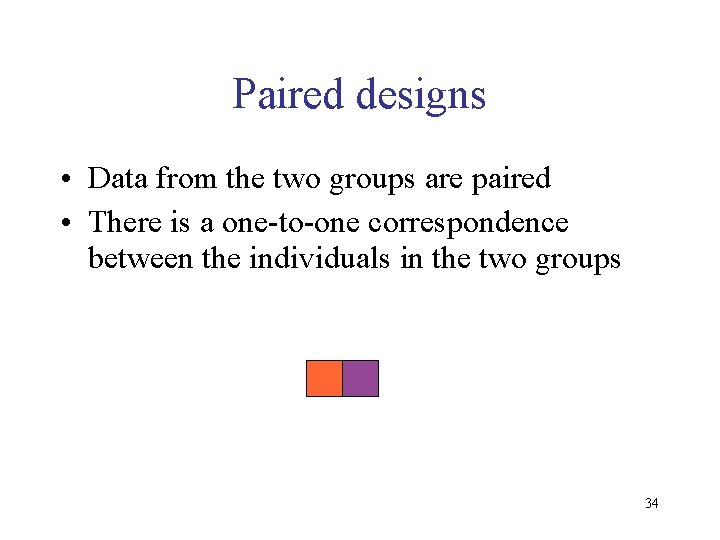
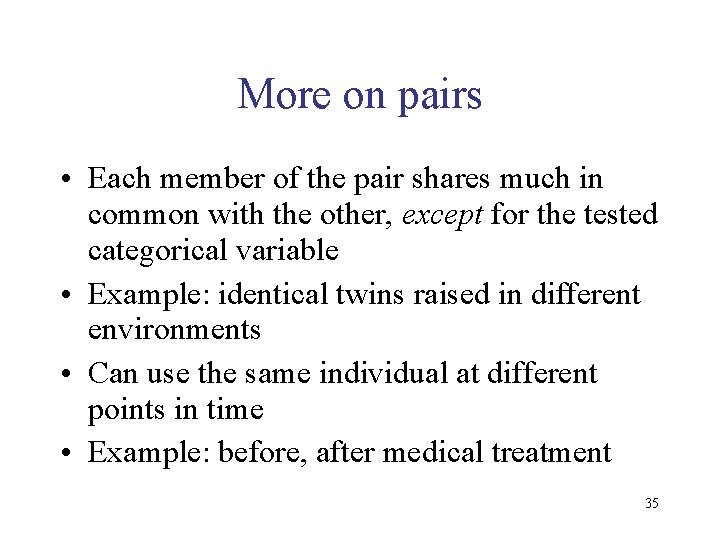
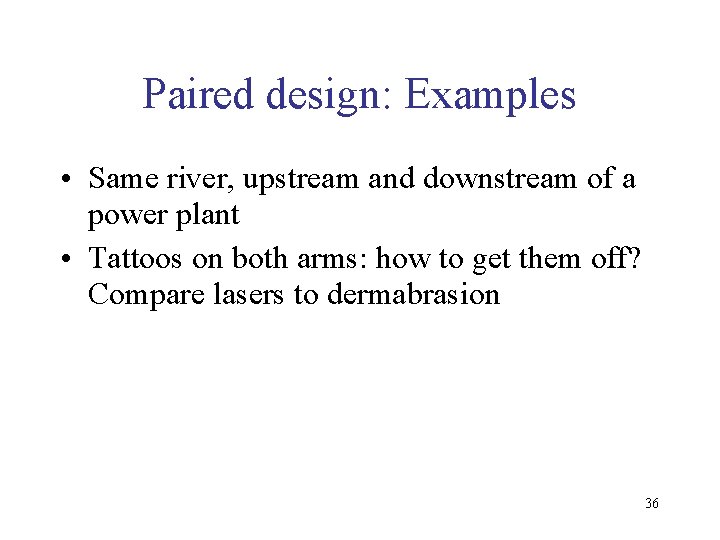
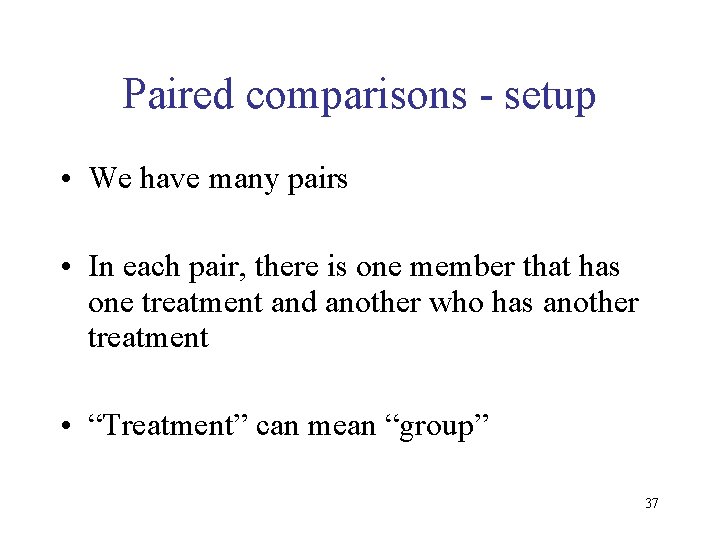
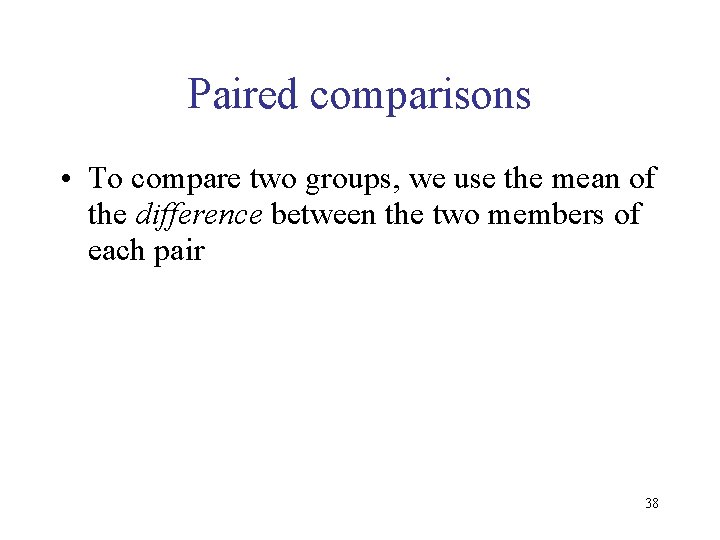
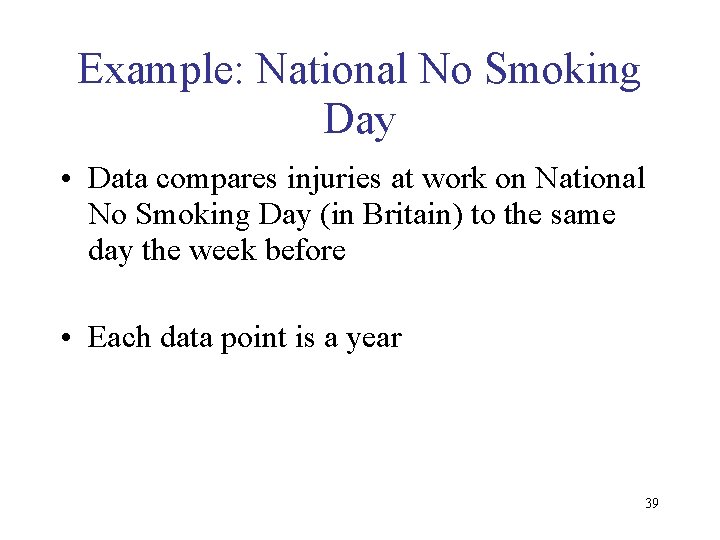
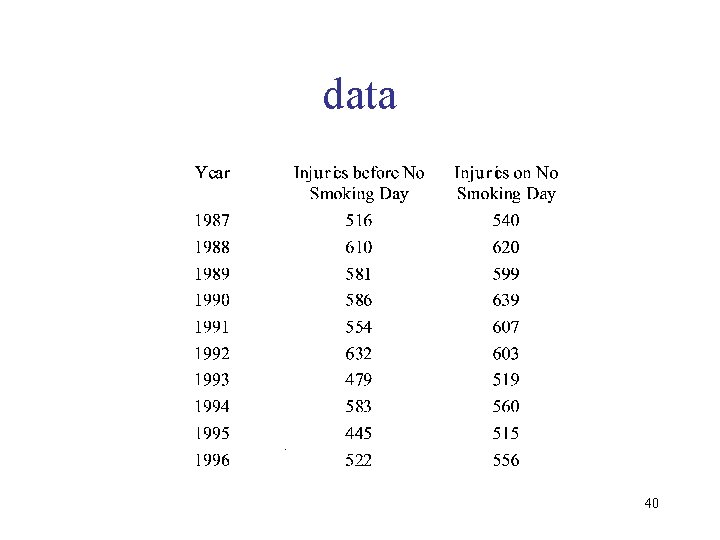
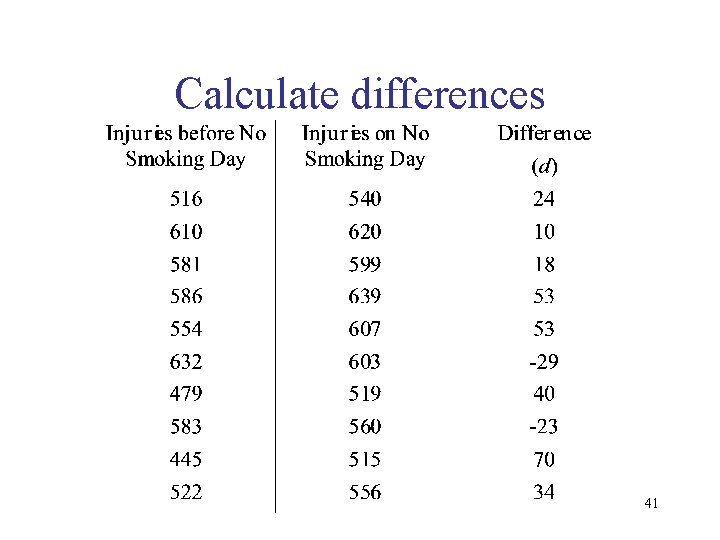
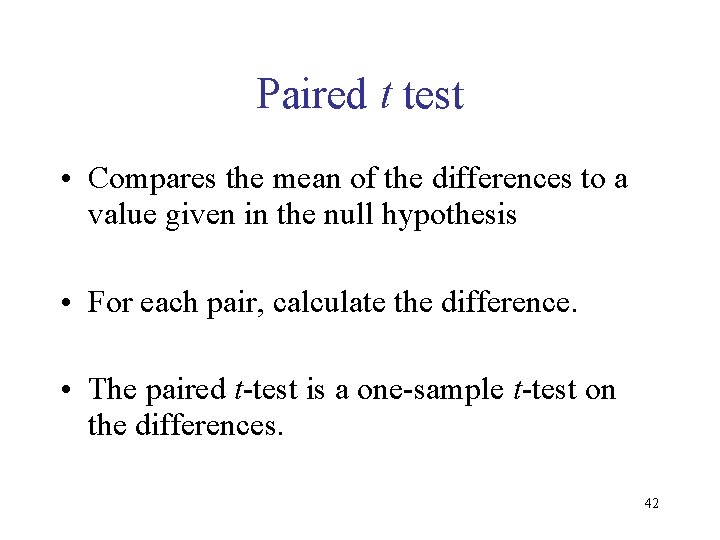
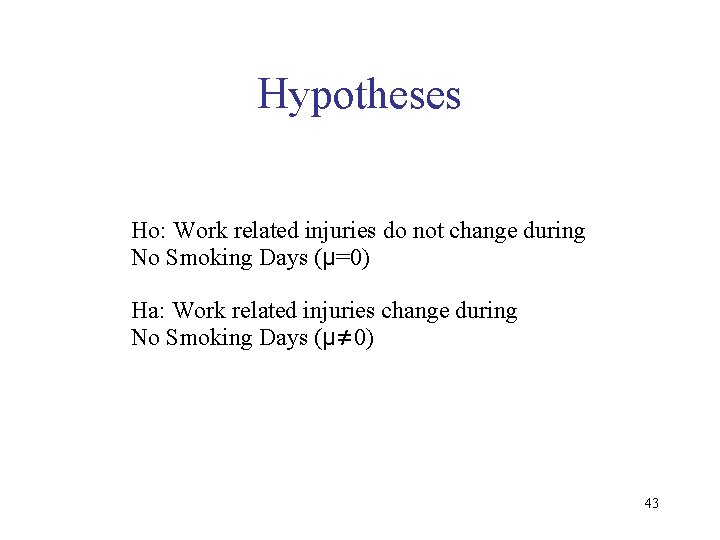
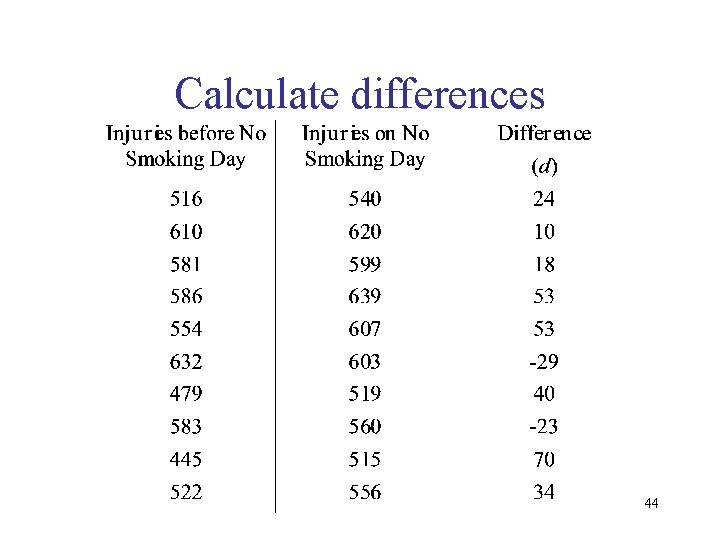
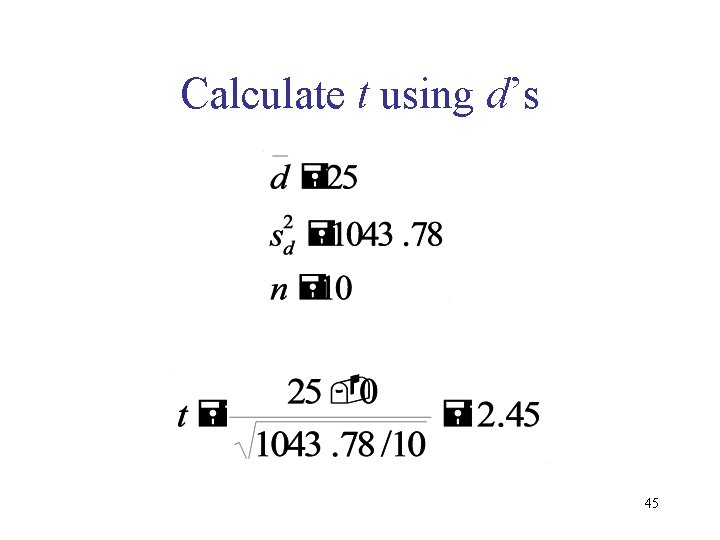
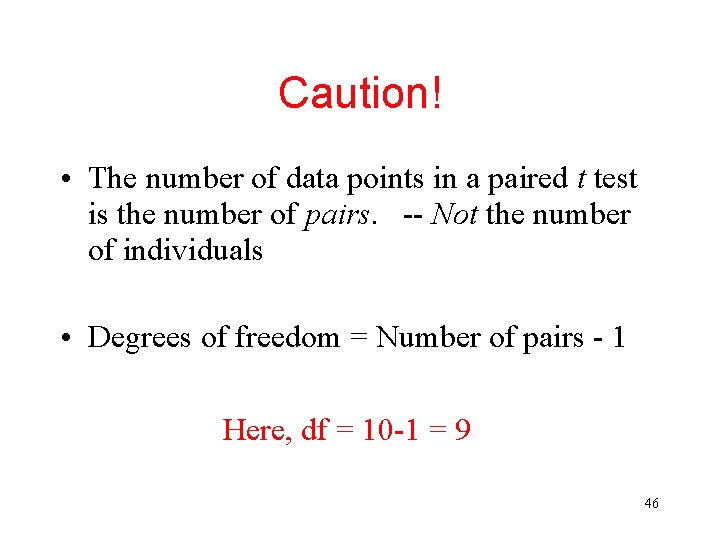
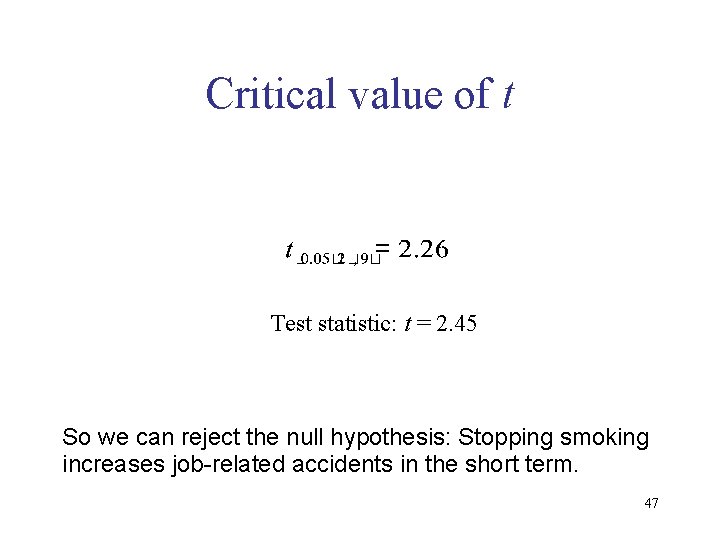
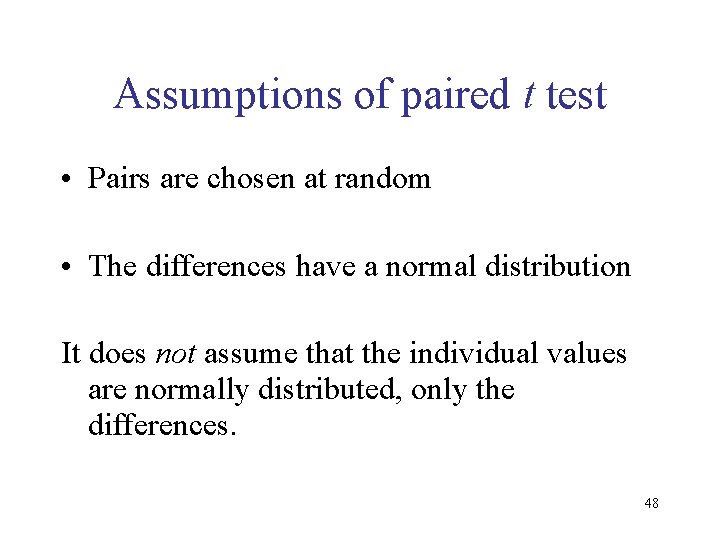
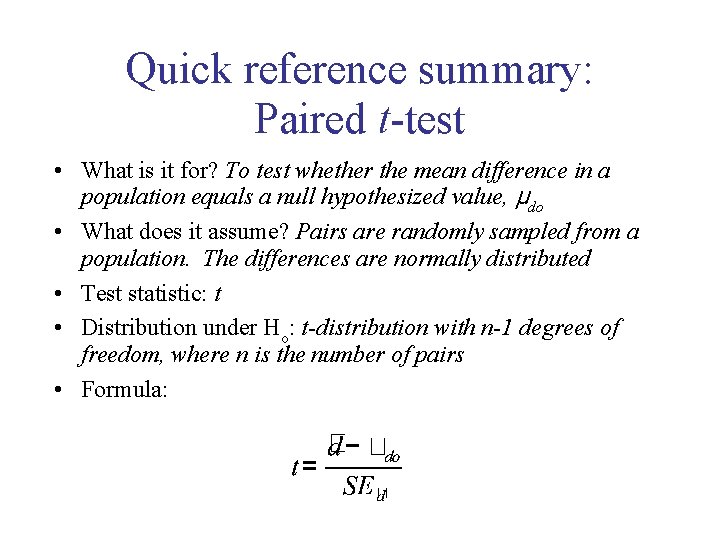
- Slides: 49
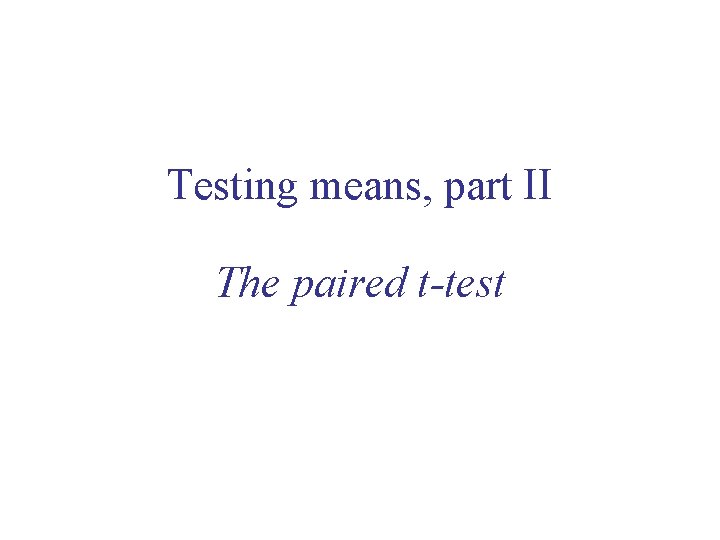
Testing means, part II The paired t-test
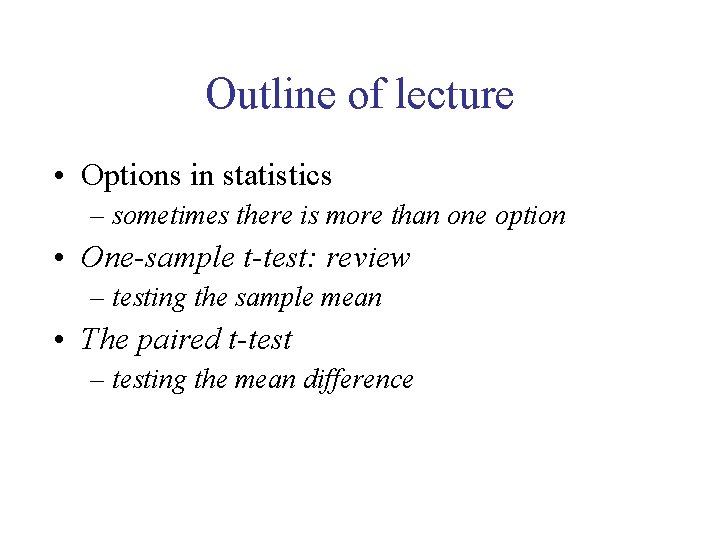
Outline of lecture • Options in statistics – sometimes there is more than one option • One-sample t-test: review – testing the sample mean • The paired t-test – testing the mean difference
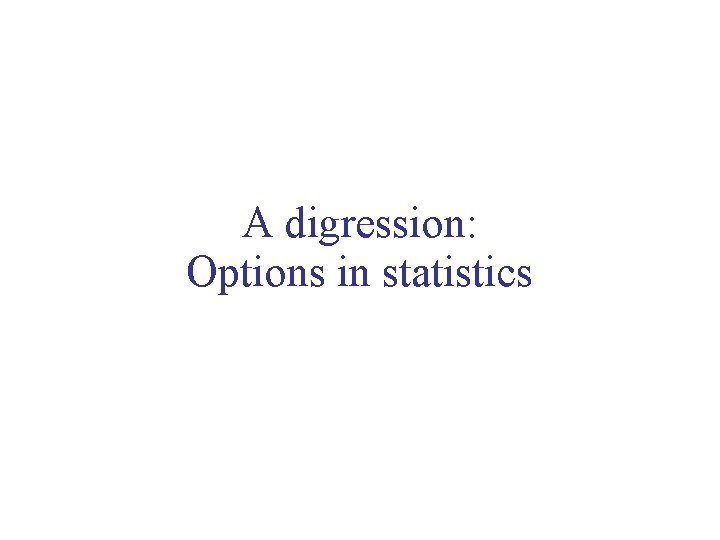
A digression: Options in statistics
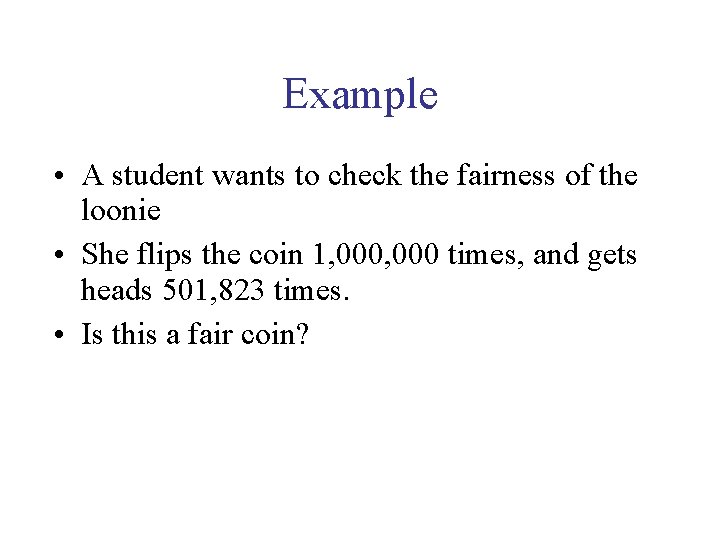
Example • A student wants to check the fairness of the loonie • She flips the coin 1, 000 times, and gets heads 501, 823 times. • Is this a fair coin?
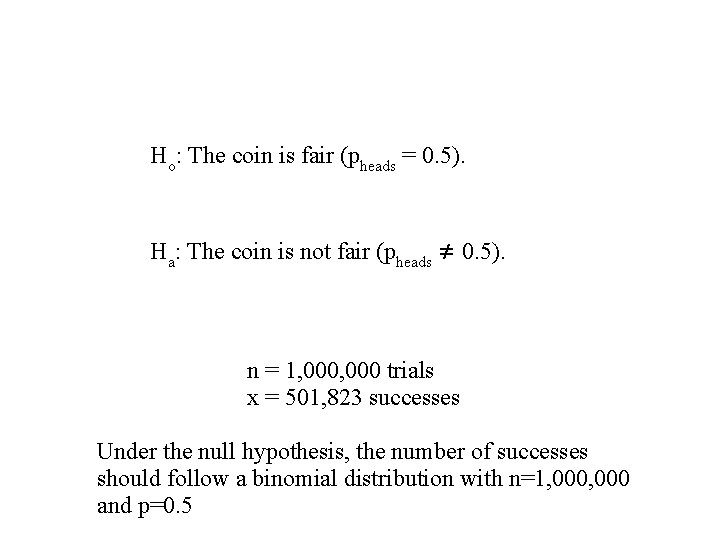
Ho: The coin is fair (pheads = 0. 5). Ha: The coin is not fair (pheads ≠ 0. 5). n = 1, 000 trials x = 501, 823 successes Under the null hypothesis, the number of successes should follow a binomial distribution with n=1, 000 and p=0. 5
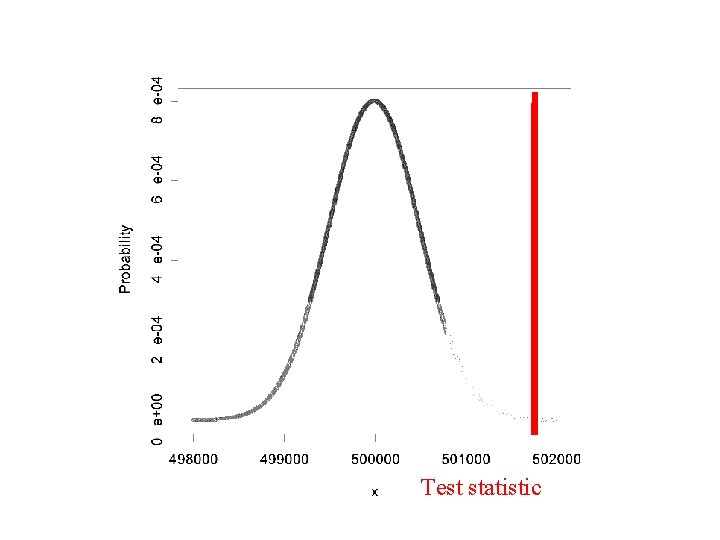
Test statistic
![Binomial test P 2PrX 501 823 P 2PrX 501 823 Binomial test • P = 2*Pr[X≥ 501, 823] P = 2*(Pr[X = 501, 823]](https://slidetodoc.com/presentation_image_h/141b123dadac6a2b615b159589fc3f1d/image-7.jpg)
Binomial test • P = 2*Pr[X≥ 501, 823] P = 2*(Pr[X = 501, 823] + Pr[X = 501, 824] + Pr[X = 501, 825] + Pr[X = 501, 826] +. . . + Pr[X = 999, 999] + Pr[X = 1, 000]
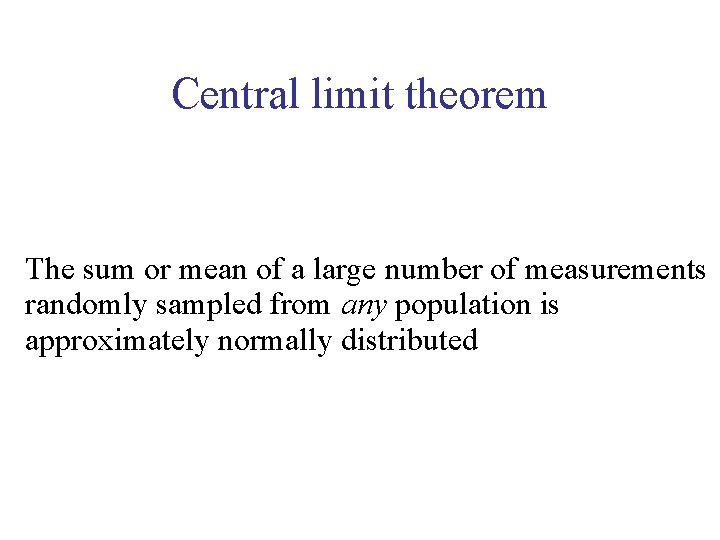
Central limit theorem The sum or mean of a large number of measurements randomly sampled from any population is approximately normally distributed
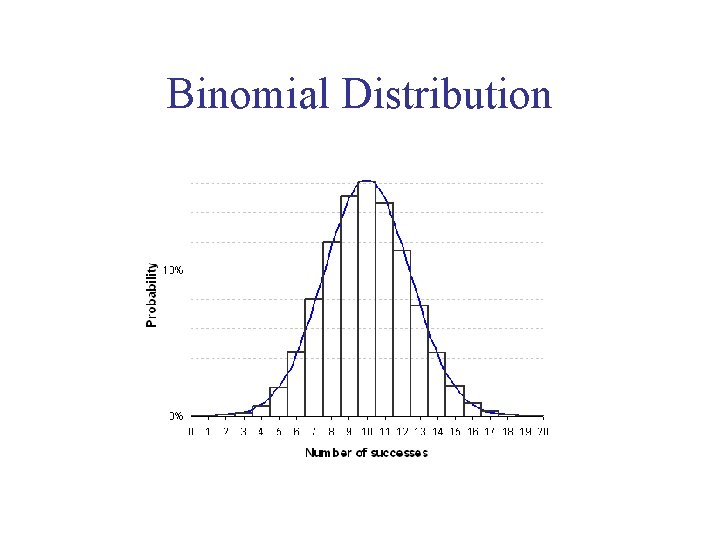
Binomial Distribution
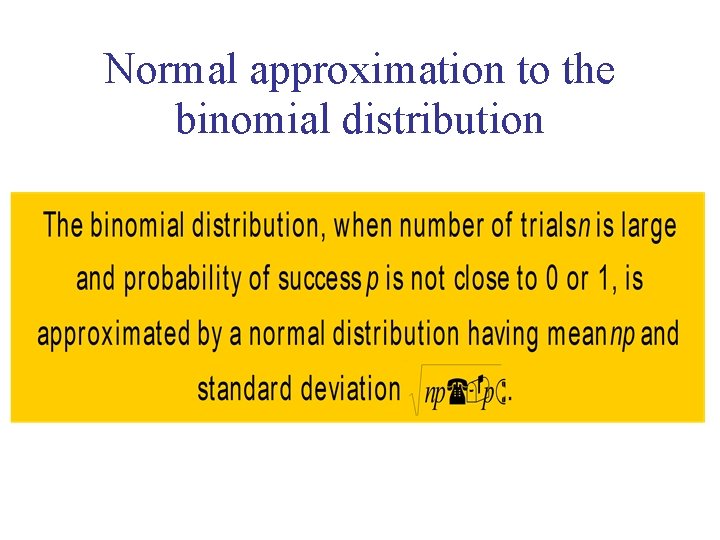
Normal approximation to the binomial distribution
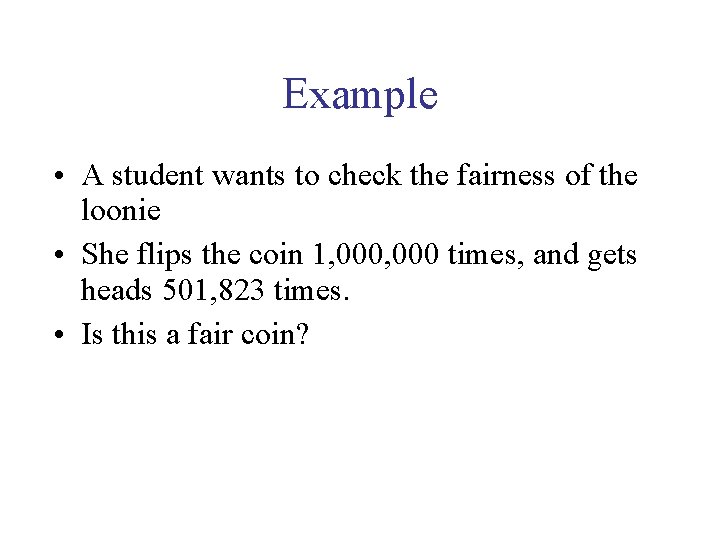
Example • A student wants to check the fairness of the loonie • She flips the coin 1, 000 times, and gets heads 501, 823 times. • Is this a fair coin?
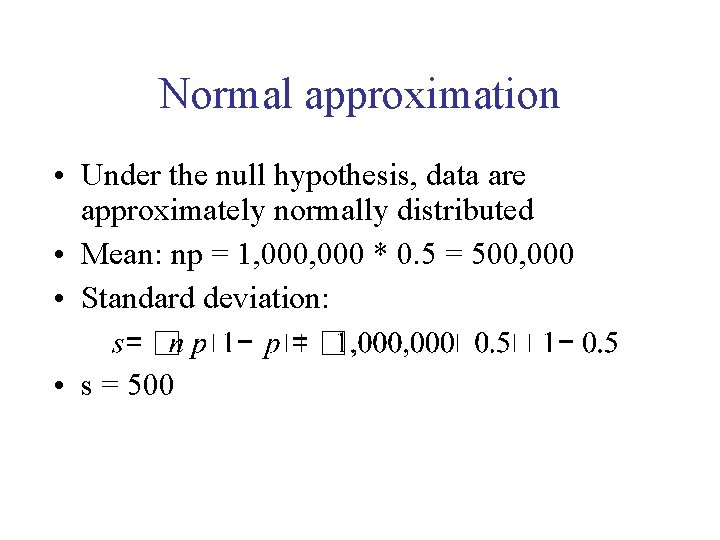
Normal approximation • Under the null hypothesis, data are approximately normally distributed • Mean: np = 1, 000 * 0. 5 = 500, 000 • Standard deviation: • s = 500
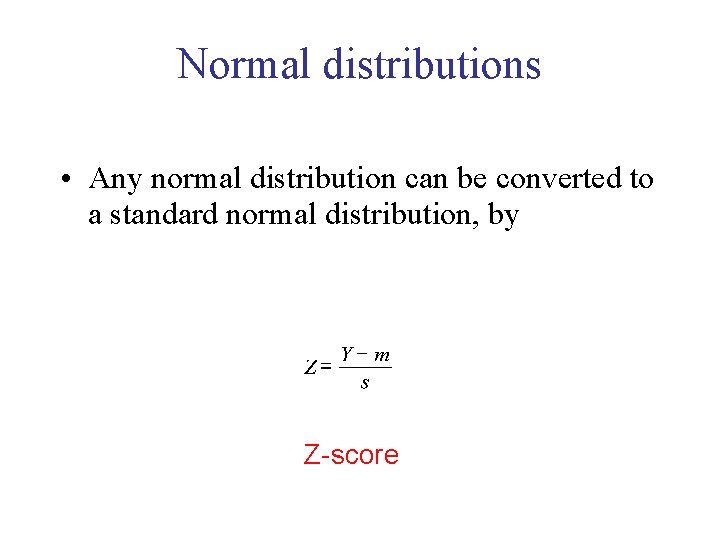
Normal distributions • Any normal distribution can be converted to a standard normal distribution, by Z-score
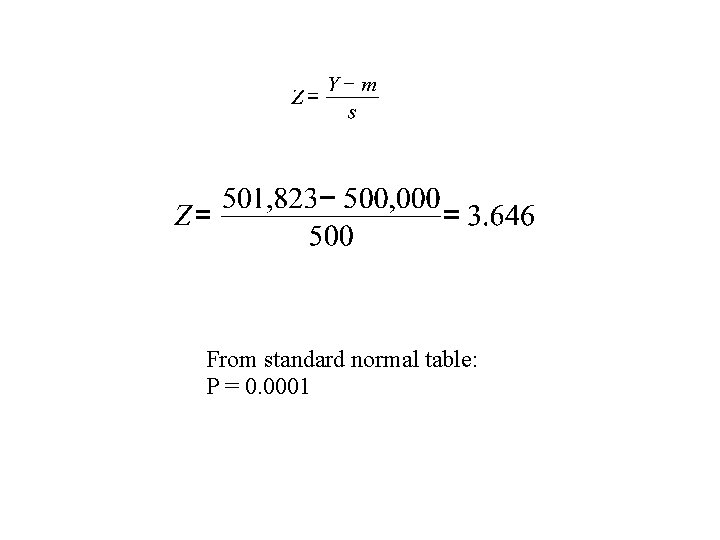
From standard normal table: P = 0. 0001
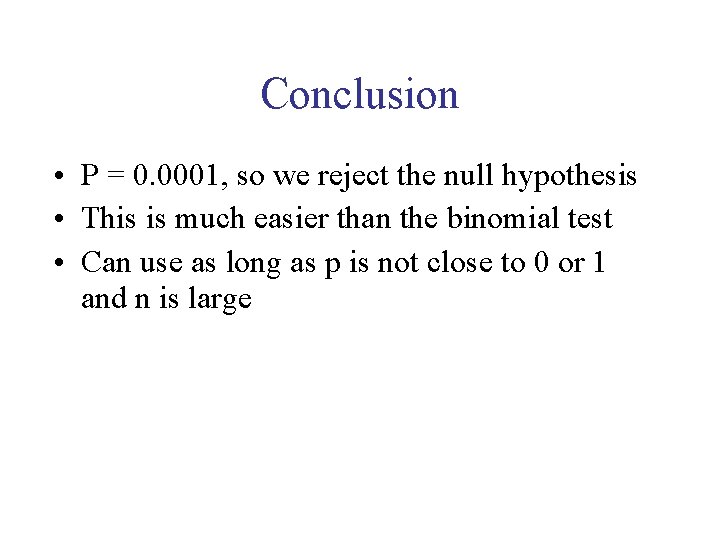
Conclusion • P = 0. 0001, so we reject the null hypothesis • This is much easier than the binomial test • Can use as long as p is not close to 0 or 1 and n is large
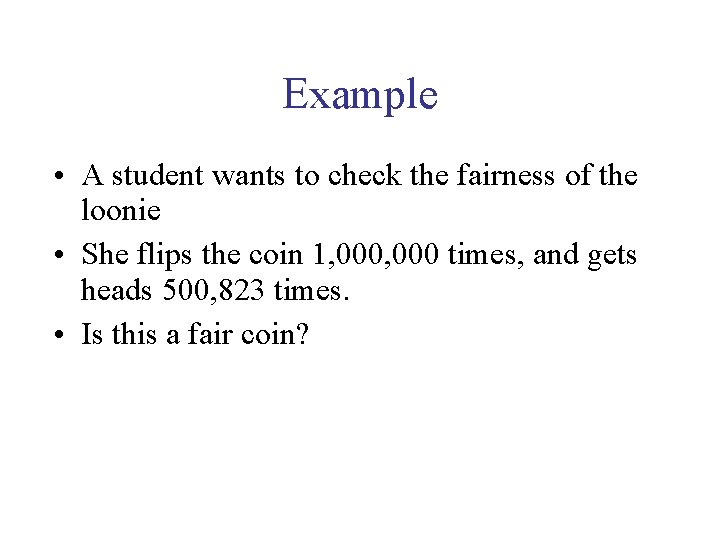
Example • A student wants to check the fairness of the loonie • She flips the coin 1, 000 times, and gets heads 500, 823 times. • Is this a fair coin?
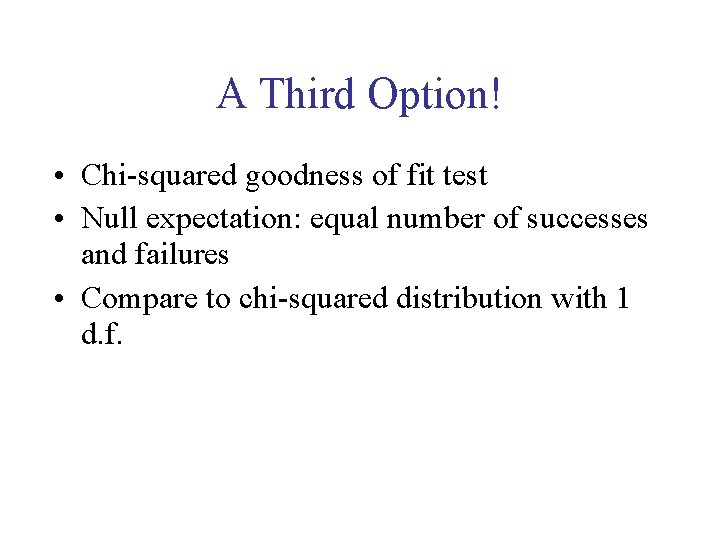
A Third Option! • Chi-squared goodness of fit test • Null expectation: equal number of successes and failures • Compare to chi-squared distribution with 1 d. f.
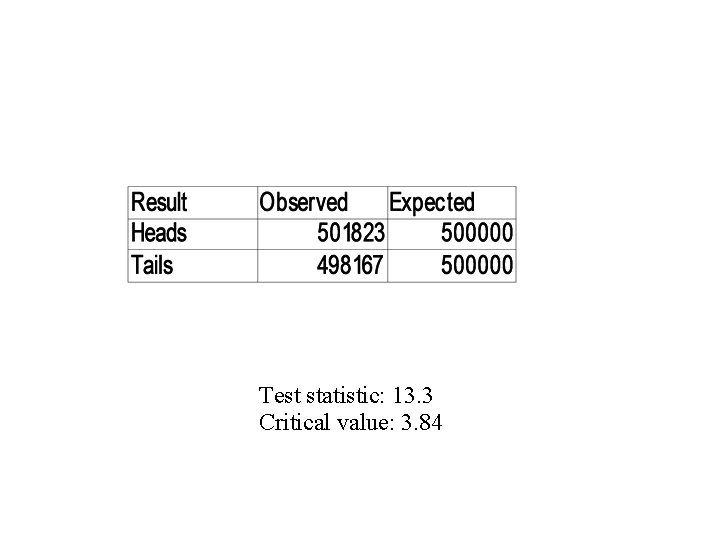
Test statistic: 13. 3 Critical value: 3. 84
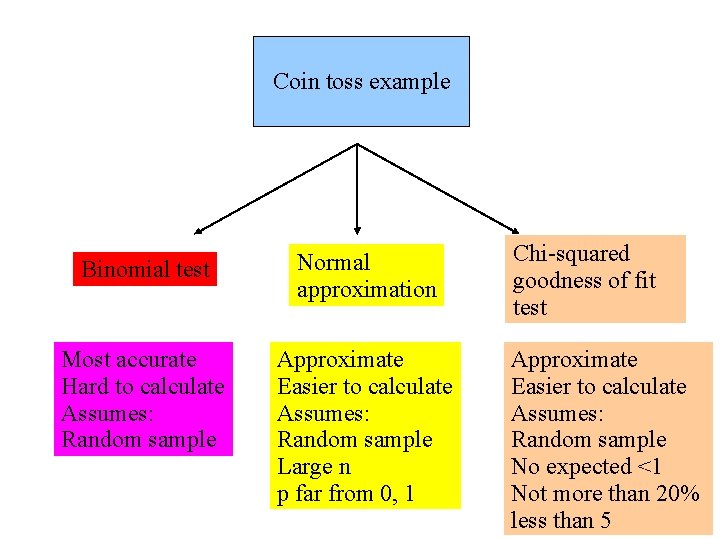
Coin toss example Binomial test Normal approximation Most accurate Hard to calculate Assumes: Random sample Approximate Easier to calculate Assumes: Random sample Large n p far from 0, 1 Chi-squared goodness of fit test Approximate Easier to calculate Assumes: Random sample No expected <1 Not more than 20% less than 5
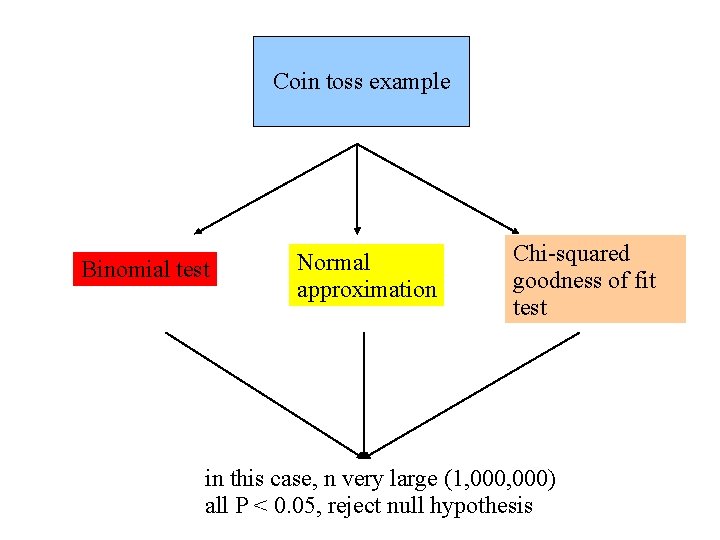
Coin toss example Binomial test Normal approximation Chi-squared goodness of fit test in this case, n very large (1, 000) all P < 0. 05, reject null hypothesis
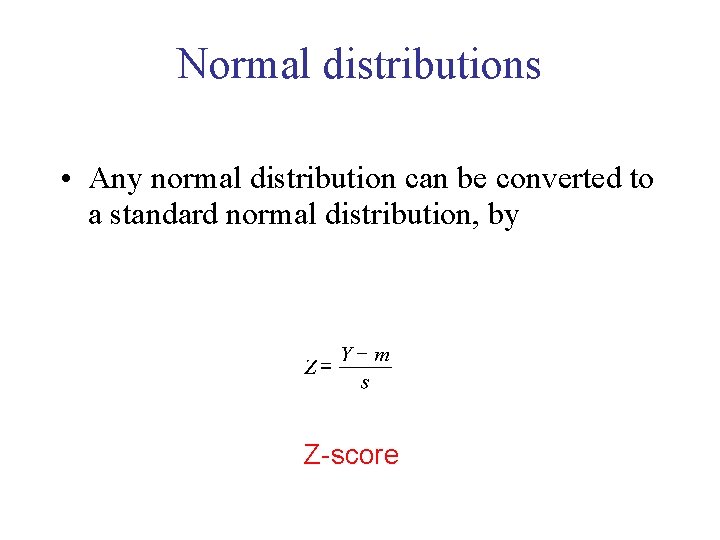
Normal distributions • Any normal distribution can be converted to a standard normal distribution, by Z-score
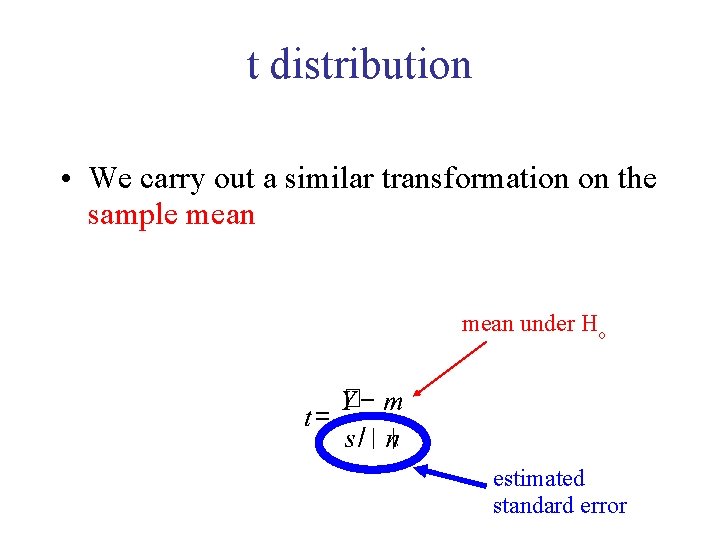
t distribution • We carry out a similar transformation on the sample mean under Ho estimated standard error
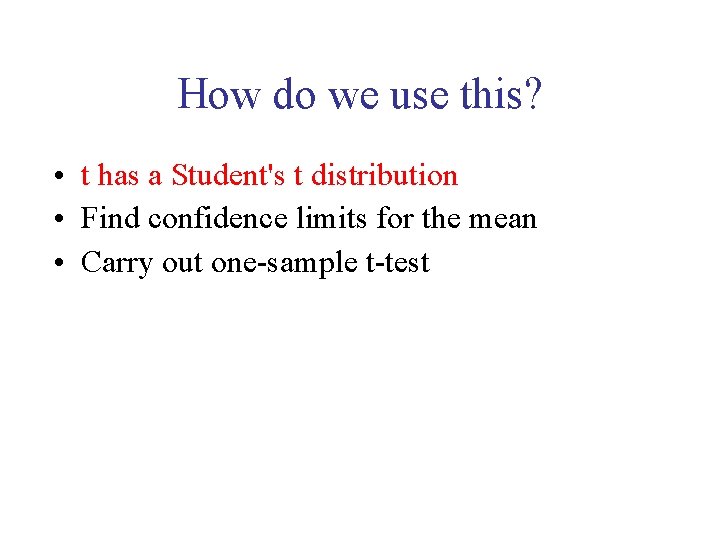
How do we use this? • t has a Student's t distribution • Find confidence limits for the mean • Carry out one-sample t-test
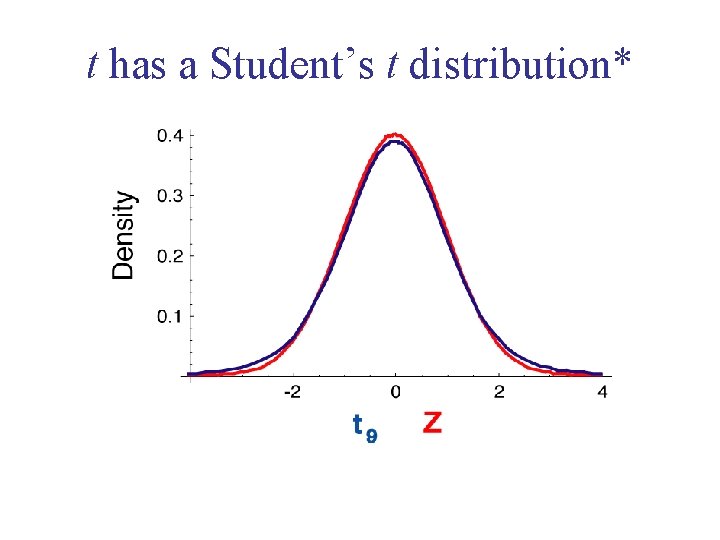
t has a Student’s t distribution*
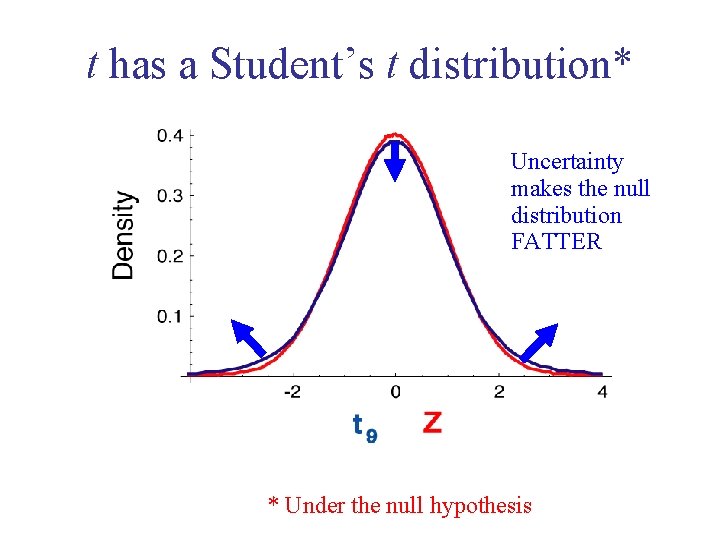
t has a Student’s t distribution* Uncertainty makes the null distribution FATTER * Under the null hypothesis
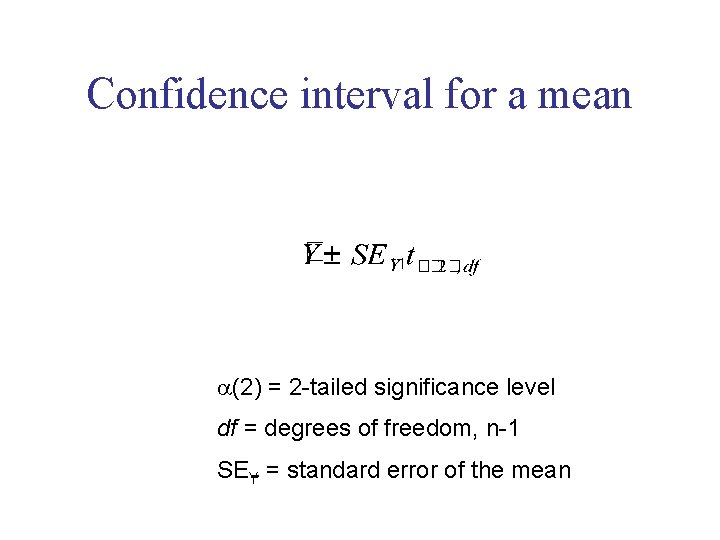
Confidence interval for a mean (2) = 2 -tailed significance level df = degrees of freedom, n-1 SEY = standard error of the mean
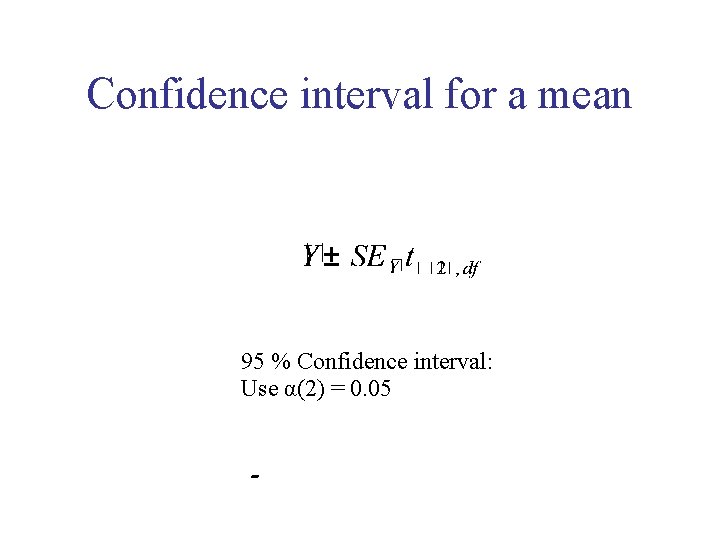
Confidence interval for a mean 95 % Confidence interval: Use α(2) = 0. 05
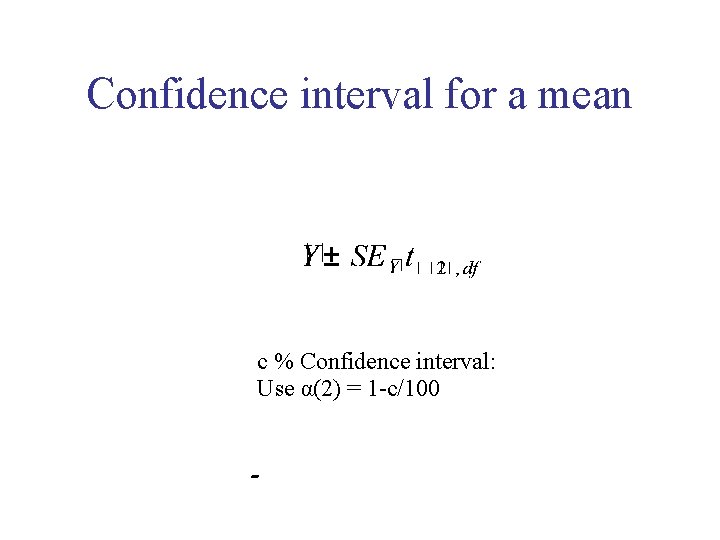
Confidence interval for a mean c % Confidence interval: Use α(2) = 1 -c/100
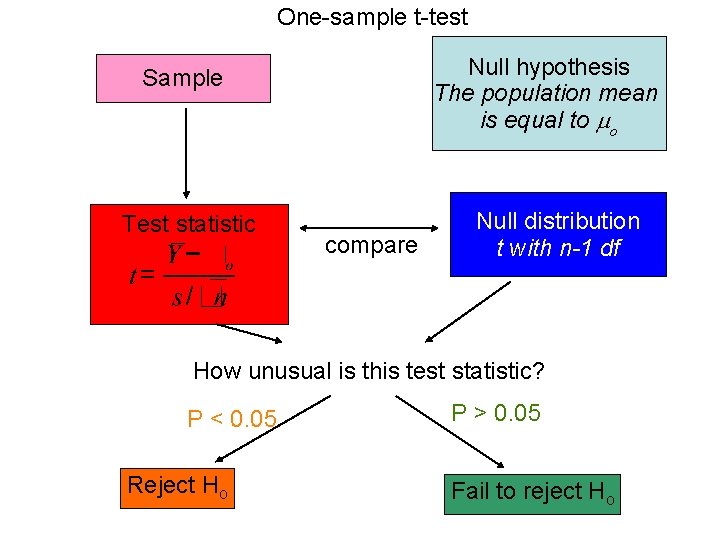
One-sample t-test Null hypothesis The population mean is equal to o Sample Test statistic compare Null distribution t with n-1 df How unusual is this test statistic? P < 0. 05 Reject Ho P > 0. 05 Fail to reject Ho
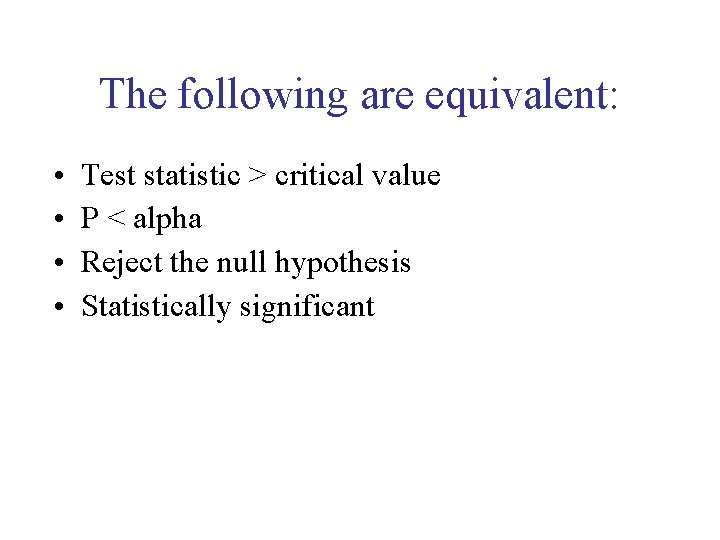
The following are equivalent: • • Test statistic > critical value P < alpha Reject the null hypothesis Statistically significant
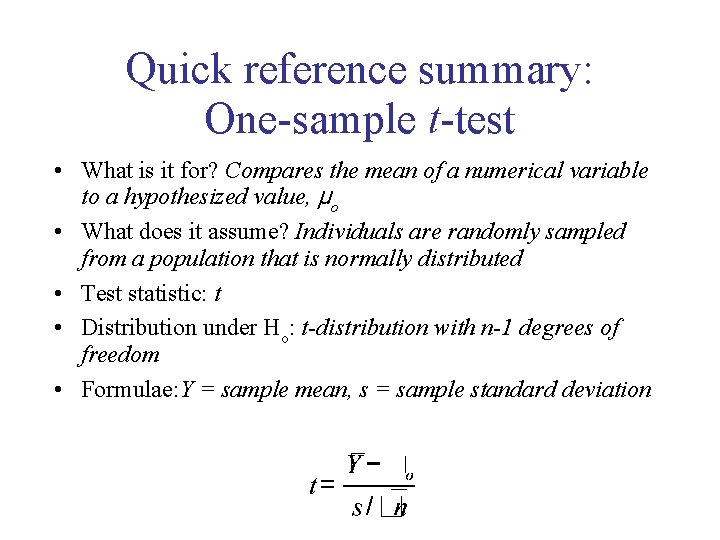
Quick reference summary: One-sample t-test • What is it for? Compares the mean of a numerical variable to a hypothesized value, μo • What does it assume? Individuals are randomly sampled from a population that is normally distributed • Test statistic: t • Distribution under Ho: t-distribution with n-1 degrees of freedom • Formulae: Y = sample mean, s = sample standard deviation
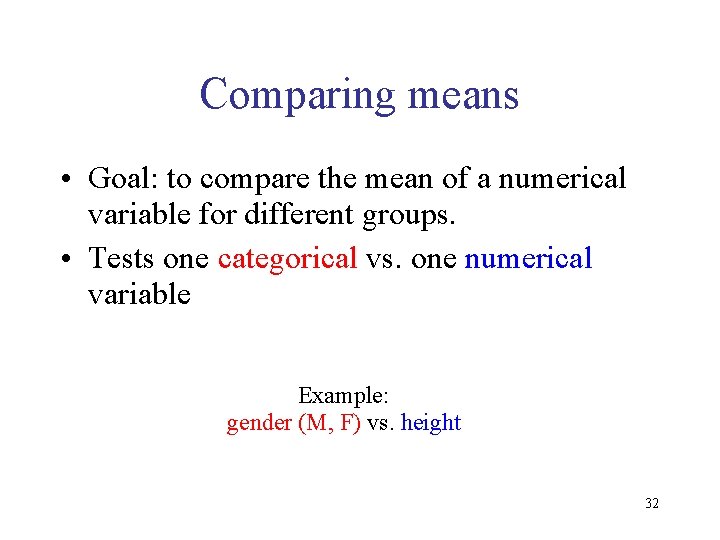
Comparing means • Goal: to compare the mean of a numerical variable for different groups. • Tests one categorical vs. one numerical variable Example: gender (M, F) vs. height 32
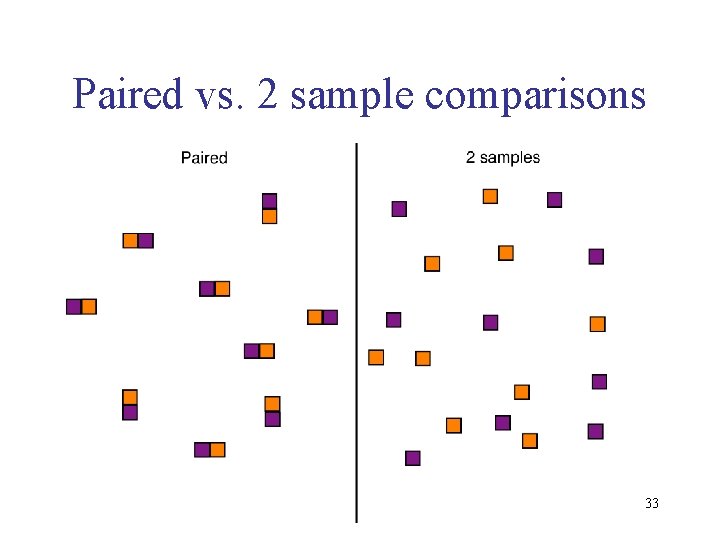
Paired vs. 2 sample comparisons 33
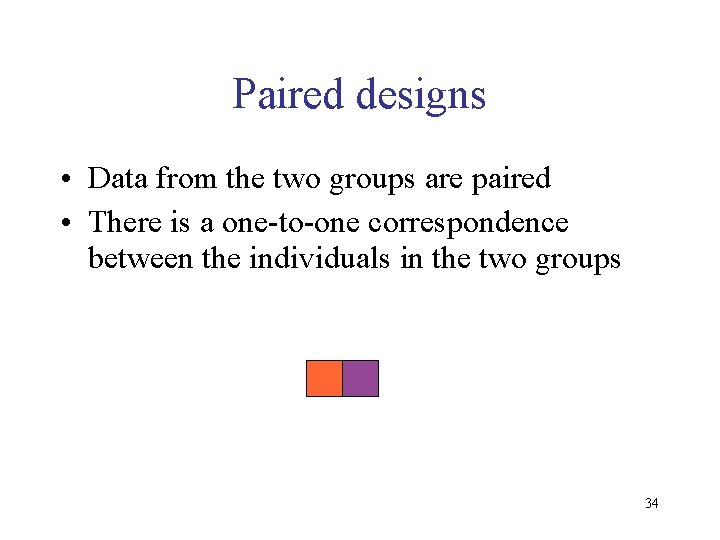
Paired designs • Data from the two groups are paired • There is a one-to-one correspondence between the individuals in the two groups 34
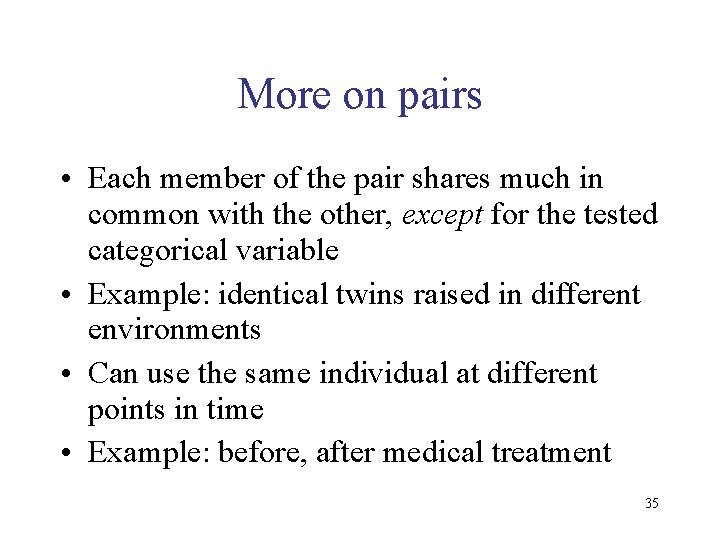
More on pairs • Each member of the pair shares much in common with the other, except for the tested categorical variable • Example: identical twins raised in different environments • Can use the same individual at different points in time • Example: before, after medical treatment 35
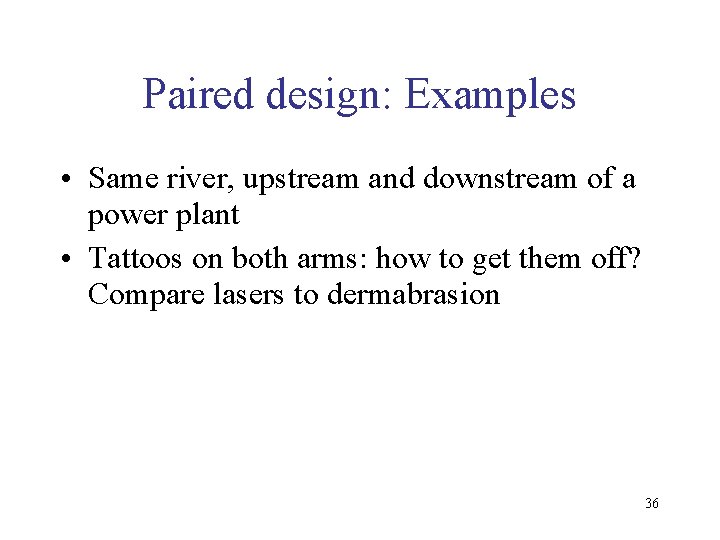
Paired design: Examples • Same river, upstream and downstream of a power plant • Tattoos on both arms: how to get them off? Compare lasers to dermabrasion 36
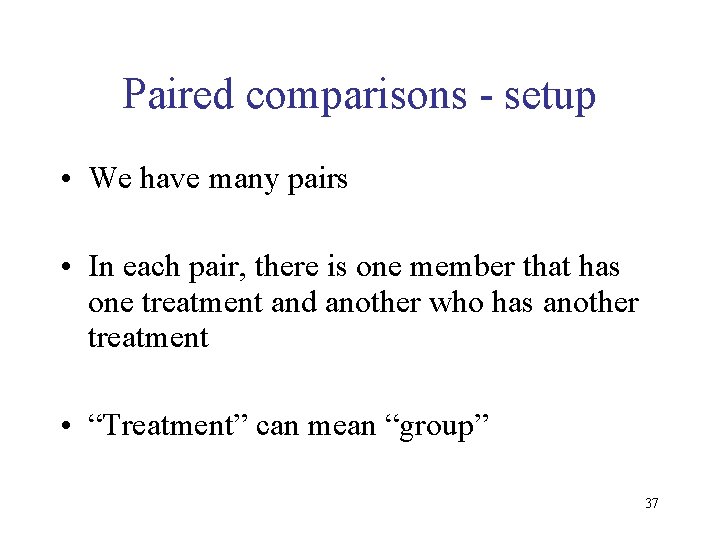
Paired comparisons - setup • We have many pairs • In each pair, there is one member that has one treatment and another who has another treatment • “Treatment” can mean “group” 37
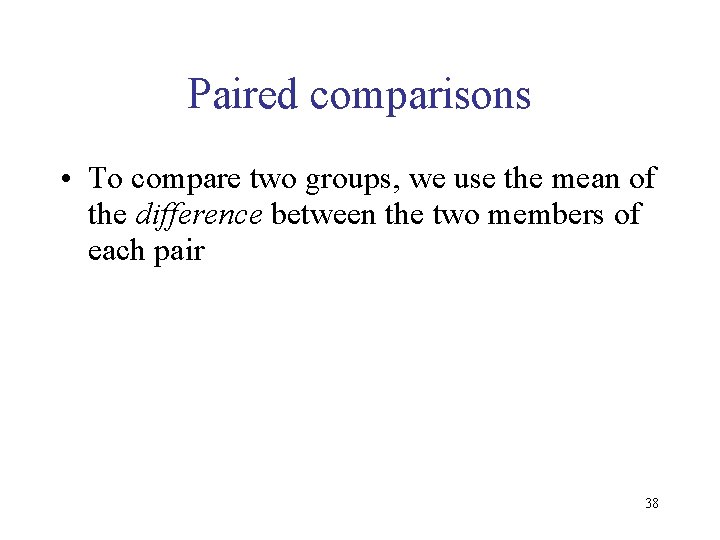
Paired comparisons • To compare two groups, we use the mean of the difference between the two members of each pair 38
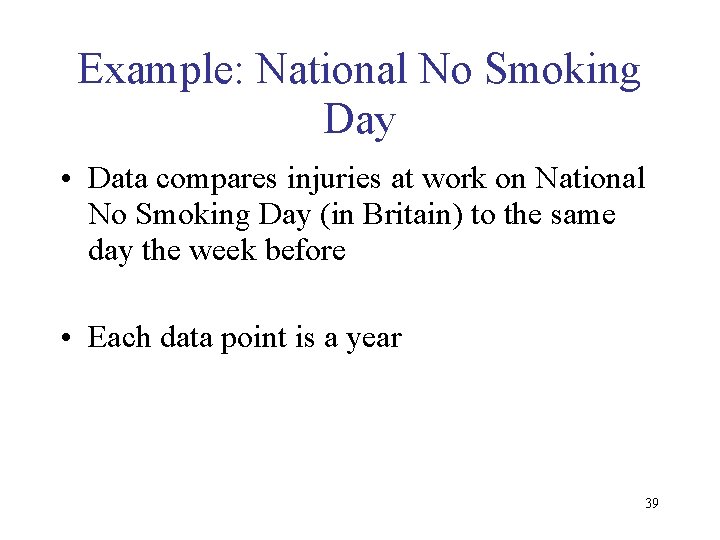
Example: National No Smoking Day • Data compares injuries at work on National No Smoking Day (in Britain) to the same day the week before • Each data point is a year 39
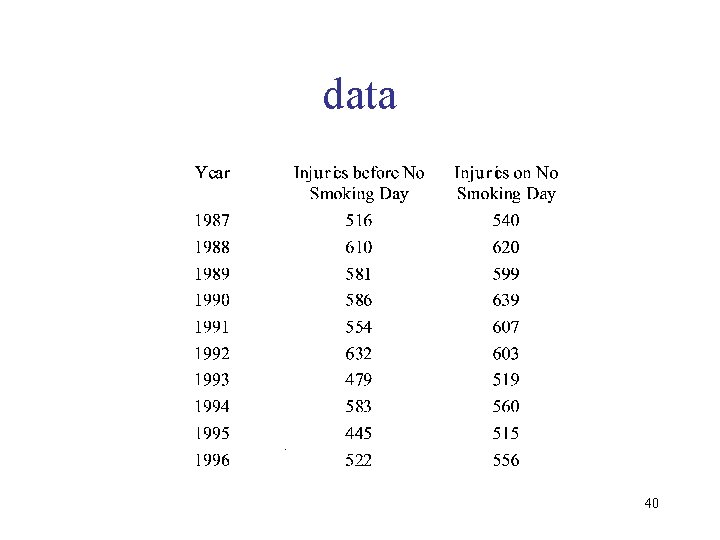
data 40
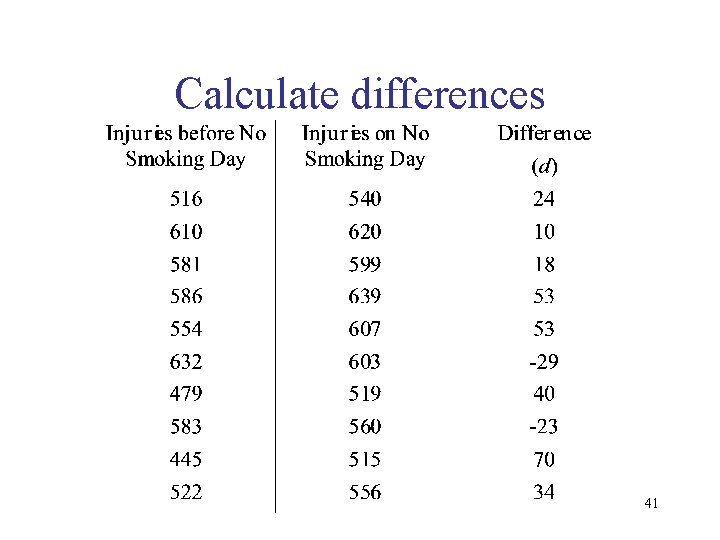
Calculate differences 41
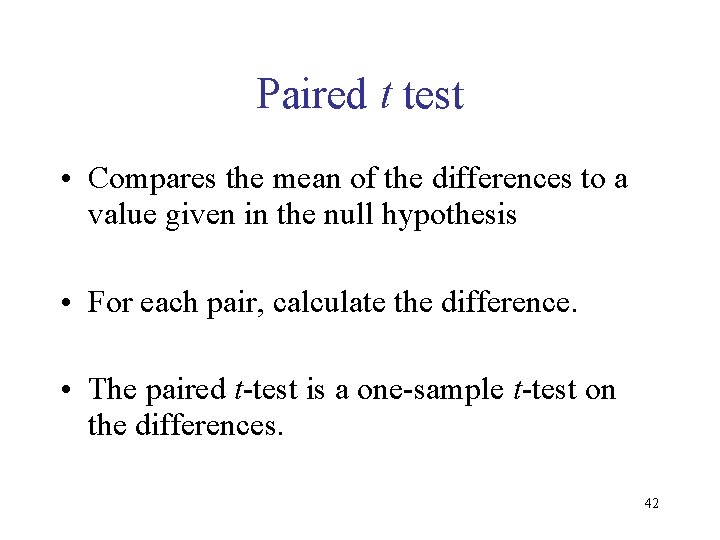
Paired t test • Compares the mean of the differences to a value given in the null hypothesis • For each pair, calculate the difference. • The paired t-test is a one-sample t-test on the differences. 42
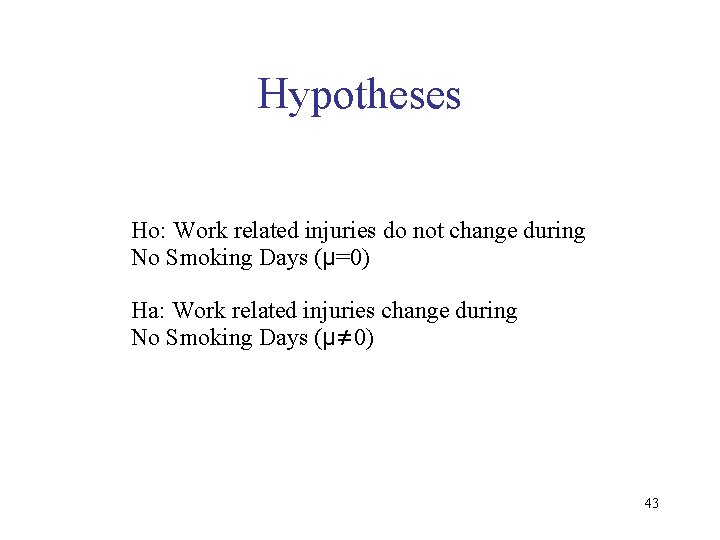
Hypotheses Ho: Work related injuries do not change during No Smoking Days (μ=0) Ha: Work related injuries change during No Smoking Days (μ≠ 0) 43
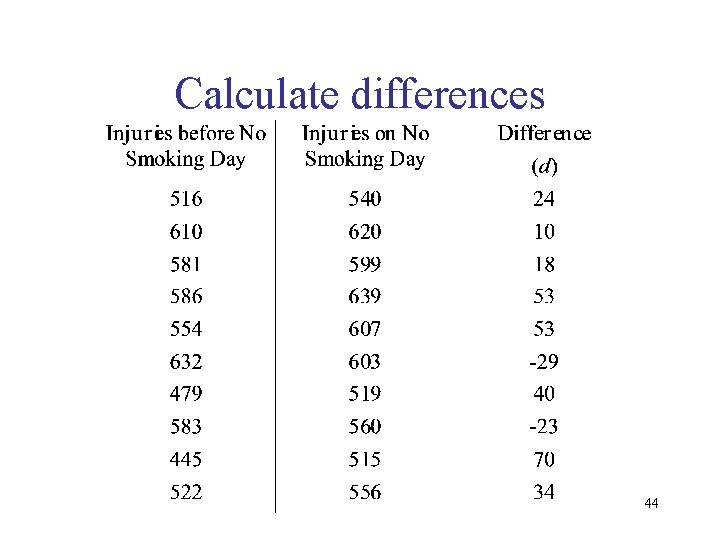
Calculate differences 44
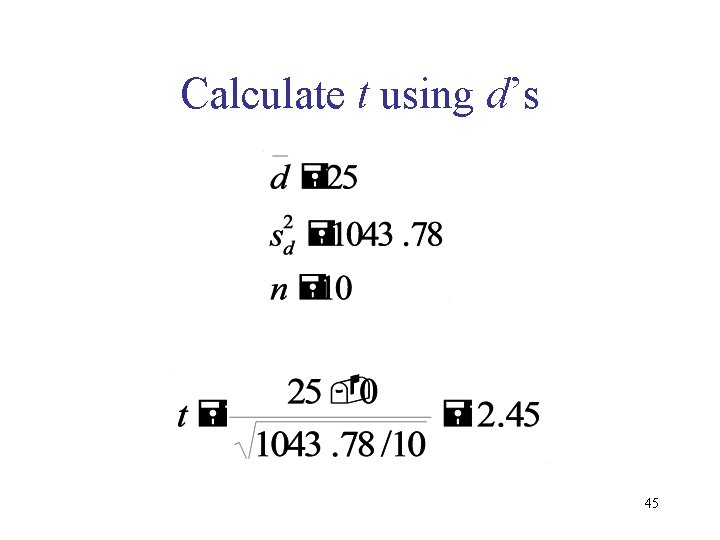
Calculate t using d’s 45
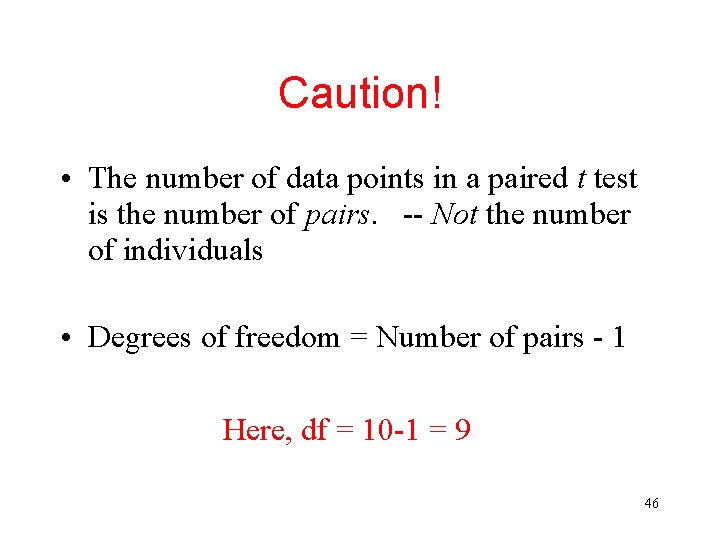
Caution! • The number of data points in a paired t test is the number of pairs. -- Not the number of individuals • Degrees of freedom = Number of pairs - 1 Here, df = 10 -1 = 9 46
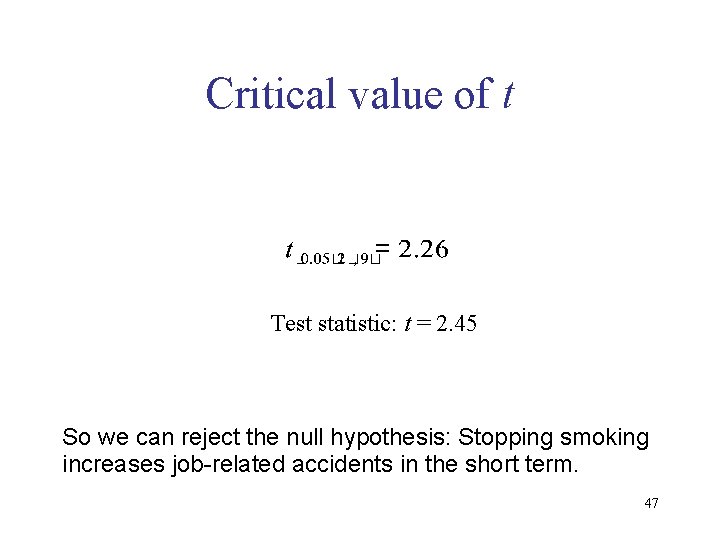
Critical value of t Test statistic: t = 2. 45 So we can reject the null hypothesis: Stopping smoking increases job-related accidents in the short term. 47
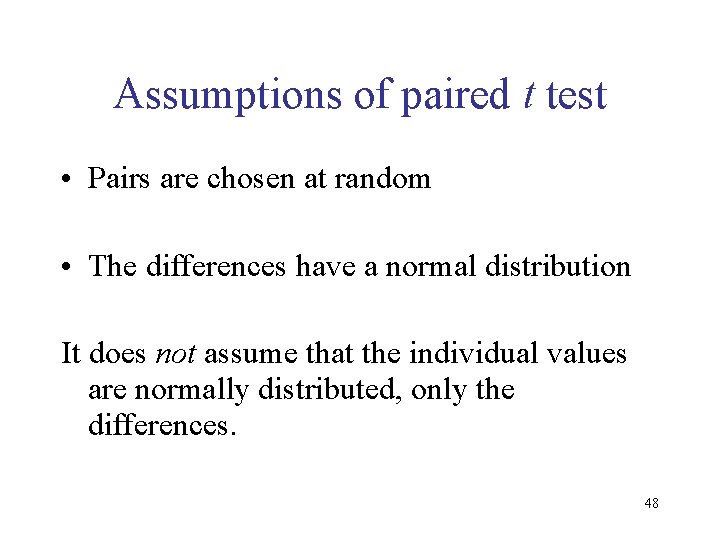
Assumptions of paired t test • Pairs are chosen at random • The differences have a normal distribution It does not assume that the individual values are normally distributed, only the differences. 48
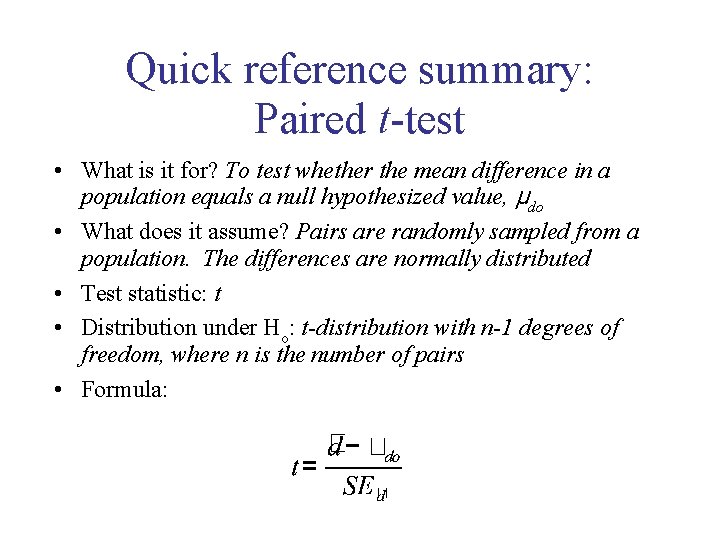
Quick reference summary: Paired t-test • What is it for? To test whether the mean difference in a population equals a null hypothesized value, μdo • What does it assume? Pairs are randomly sampled from a population. The differences are normally distributed • Test statistic: t • Distribution under Ho: t-distribution with n-1 degrees of freedom, where n is the number of pairs • Formula:
Dependent sampling
Dependent ttest
Between subjects vs within subjects
Vertical line test calculator
T-test beispielrechnung
Proc means options
Ttest ind
Quote sandwich example
Testing outline
Domain closure in software testing
Logic based testing
Data flow testing strategies in software testing
Positive negative testing
Cs 3250
Localization globalization testing
Functional testing vs unit testing
Cause effect graph for triangle problem
Control structure testing in software engineering
Decision table testing in software testing
Decision table based testing
Metode black box
Behavior testing adalah
Decision table based testing in software testing
Rigorous testing in software testing
Testing blindness in software testing
Component testing is a black box testing
Domain testing example
A dietitian wishes to see if a person's cholesterol
Is ap research worth it
What is the name of the first part of an outline?
Skill 12 agreement after expressions of quantity
Why was achilles almost-undefeatable?
Difference between a paired and unpaired t test
Quadrat sampling example
How to calculate sample size in research
Soft paired cone shaped organs
Graphic rating scale example
Paired comparison method of performance appraisal
Paired conjunctions
Paired metamorphic belts
2 sample t-test formula
Mesosalpinx
For identifying organisms through key usually
Types of appraisal methods in hrm
Unpaired vs paired t test
Unpaired vs paired t test
Parallel structure with paired conjunctions
Chapter 24 paired samples and blocks
Chapter 24 paired samples and blocks
Veins of the arm