Regression Analysis Chapter 29 29 1 Regression Modeling
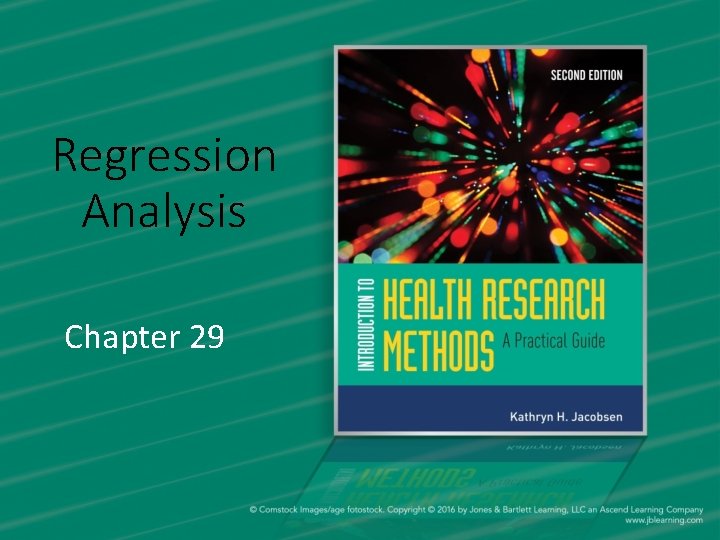
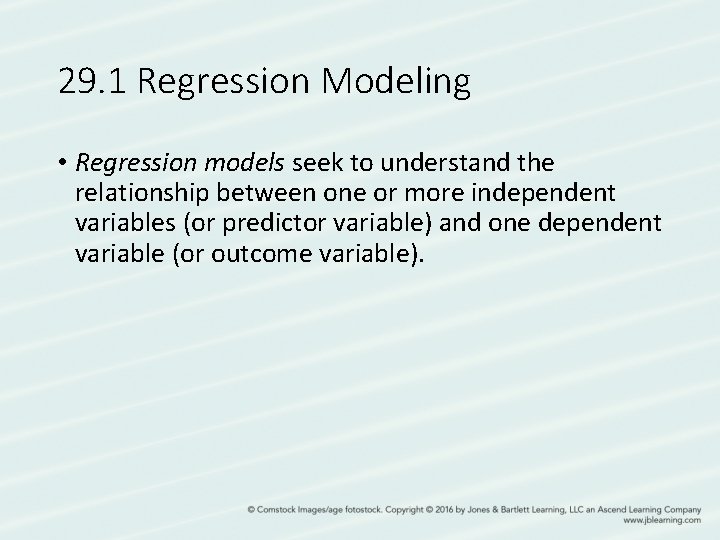
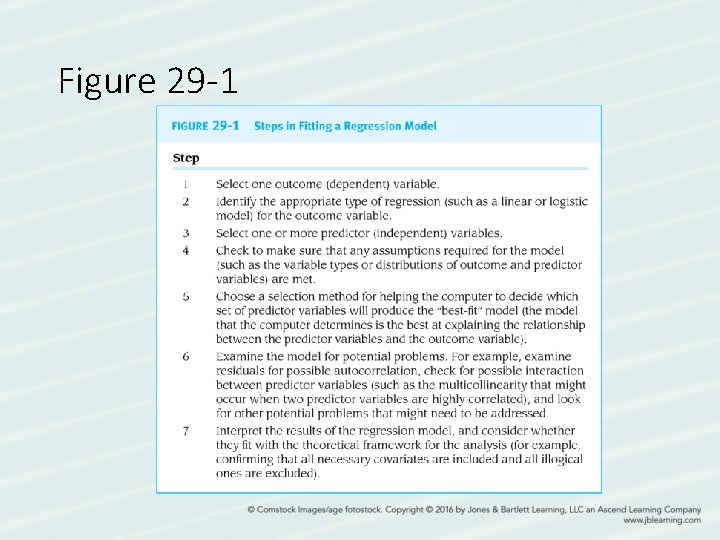
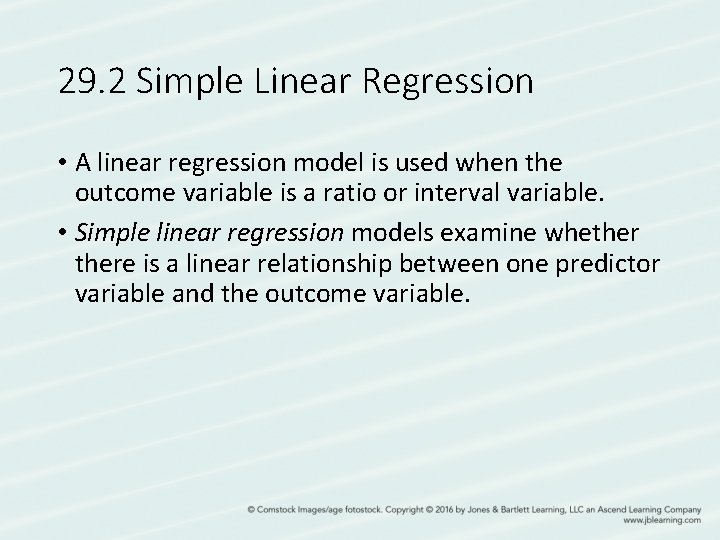
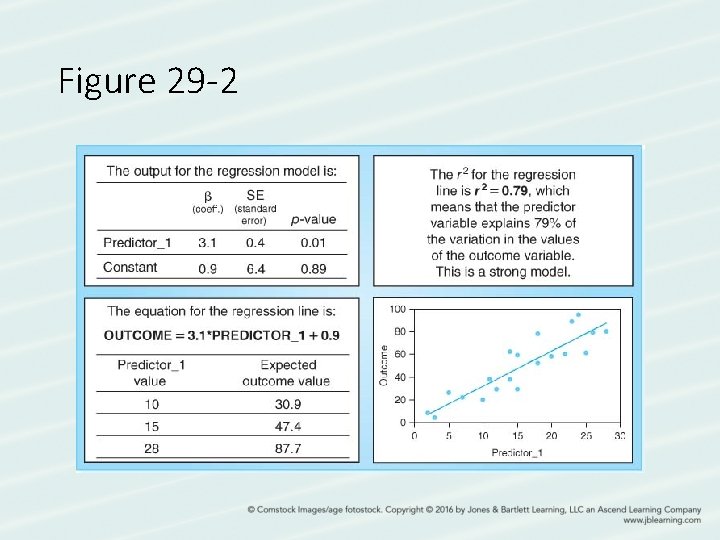
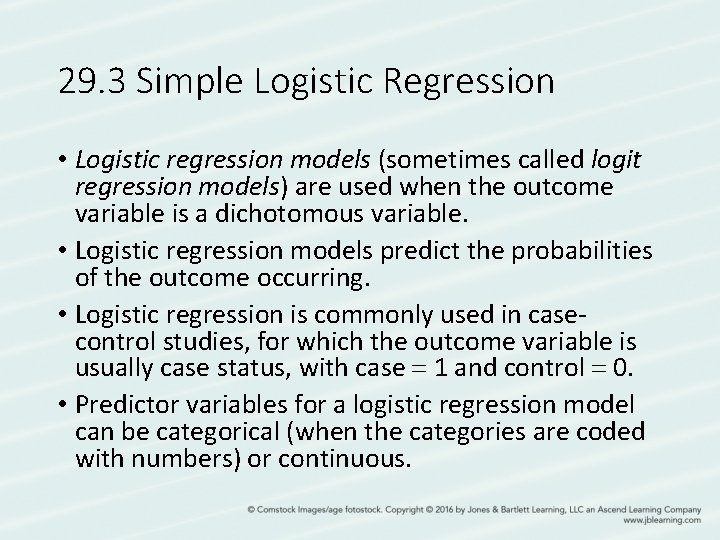
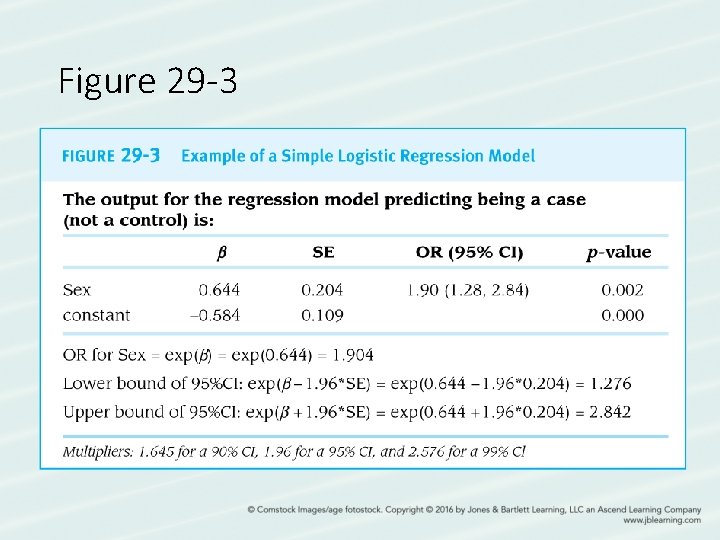
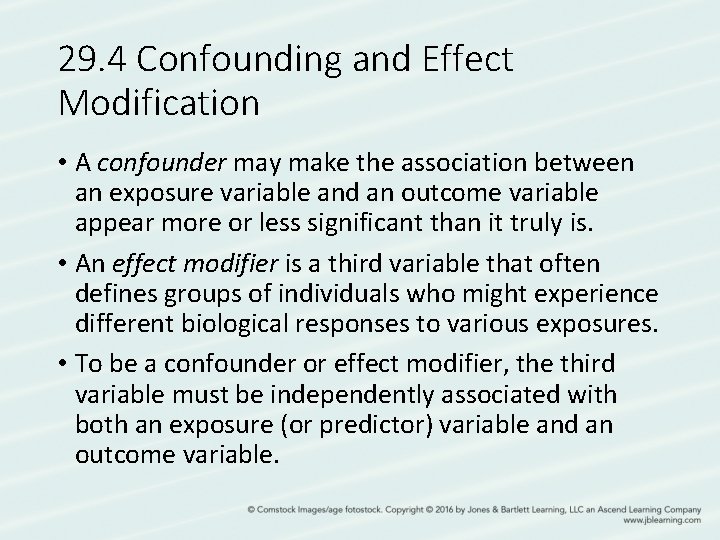
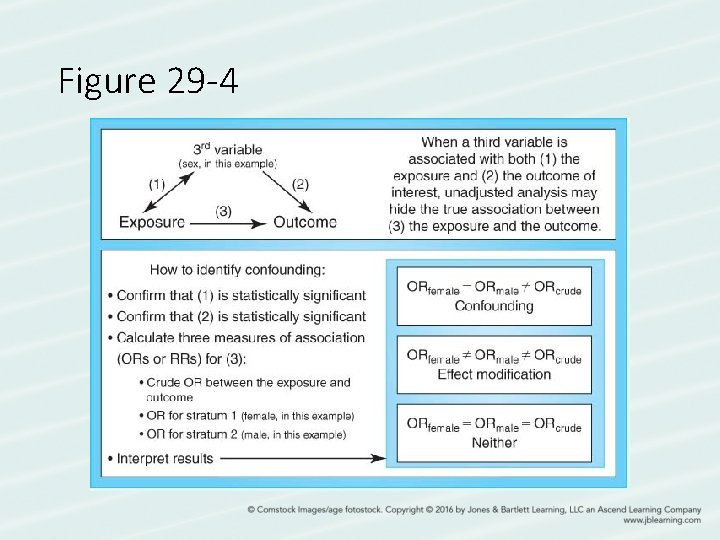
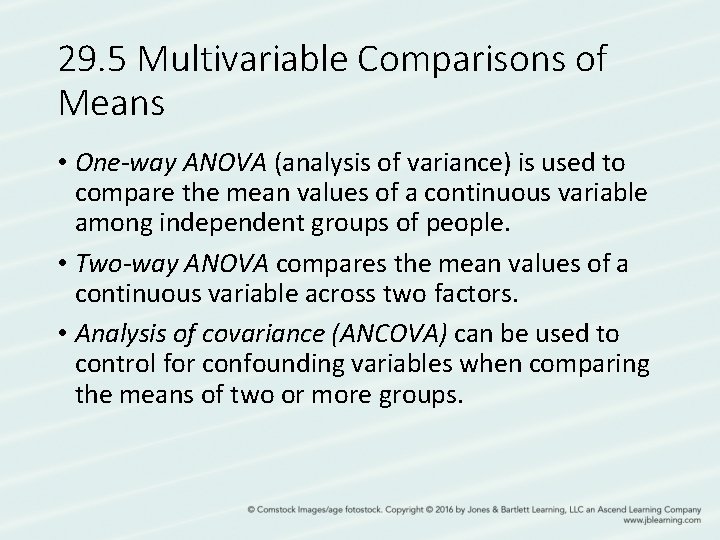
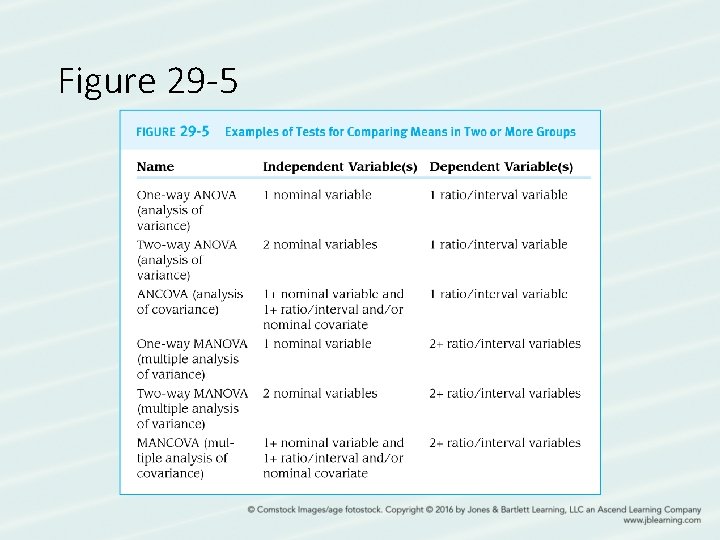
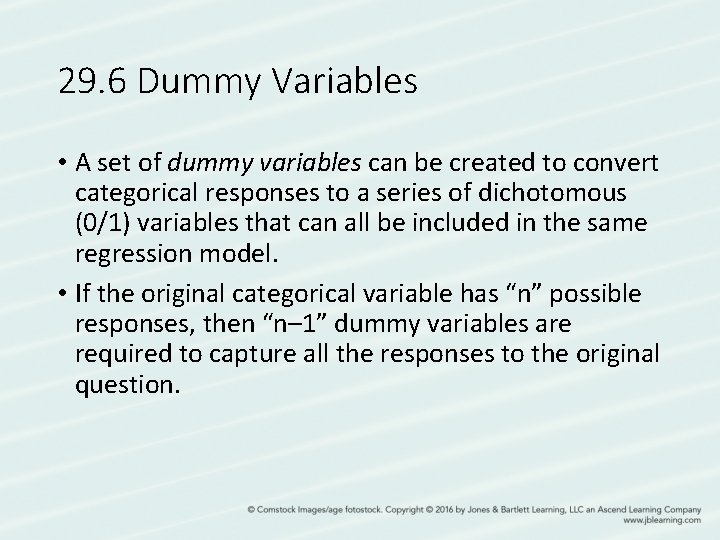
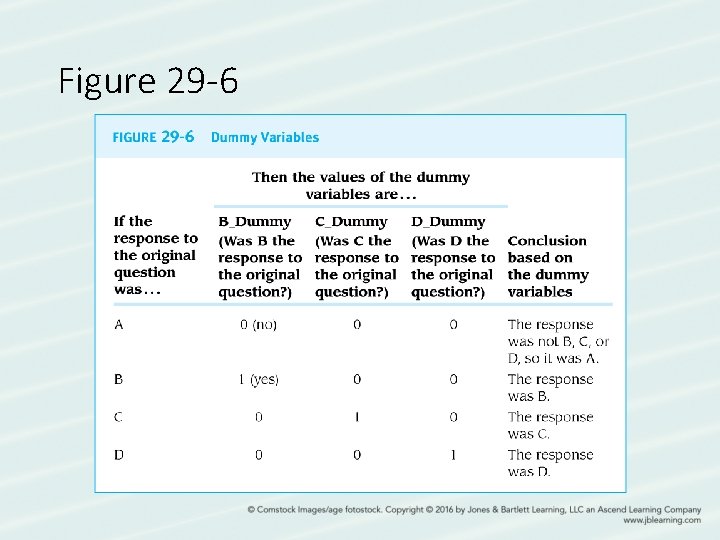
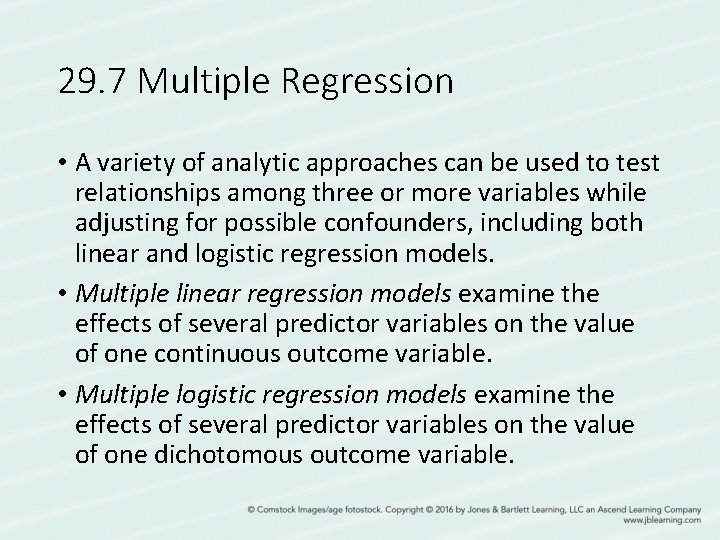
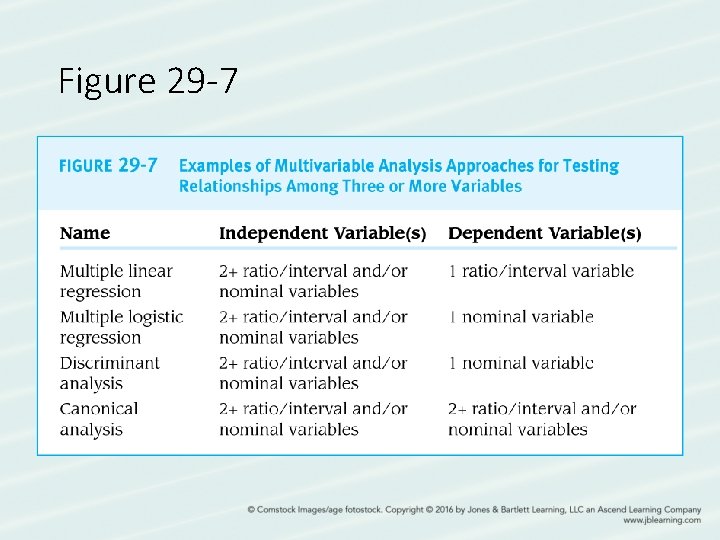
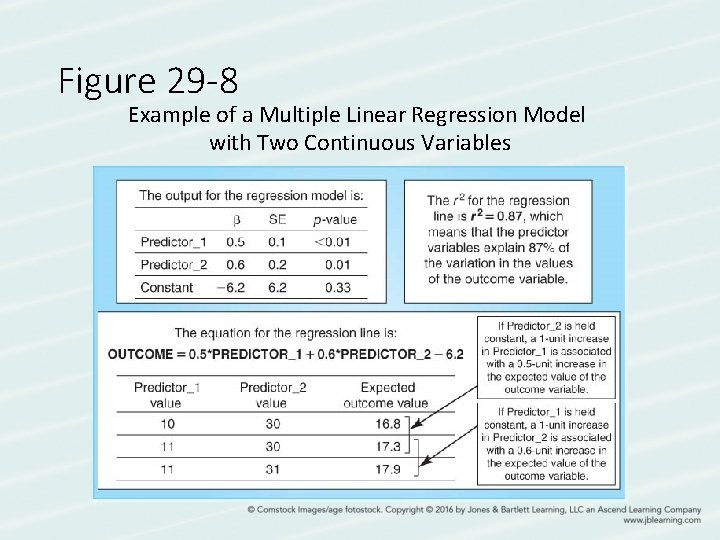
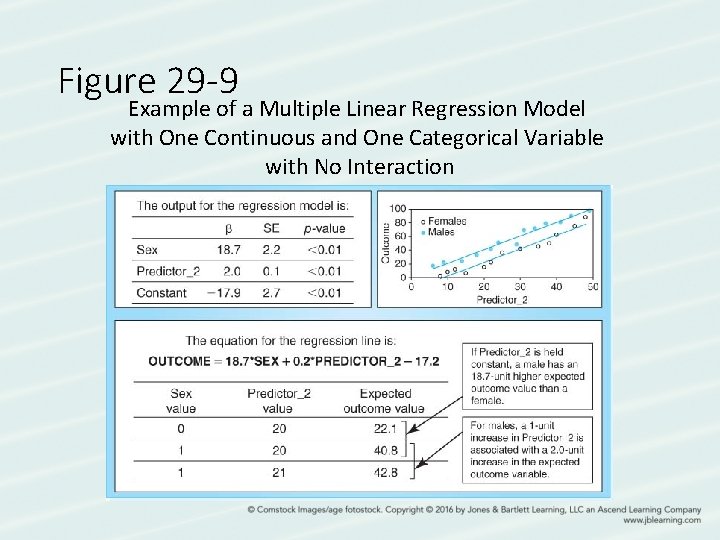
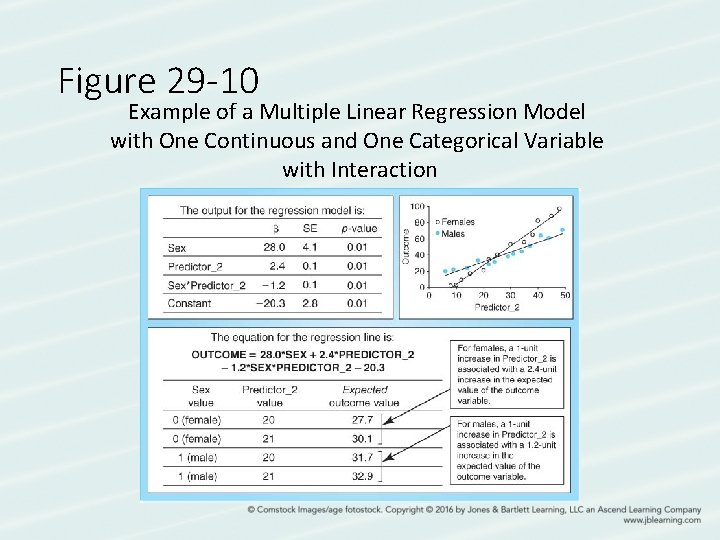
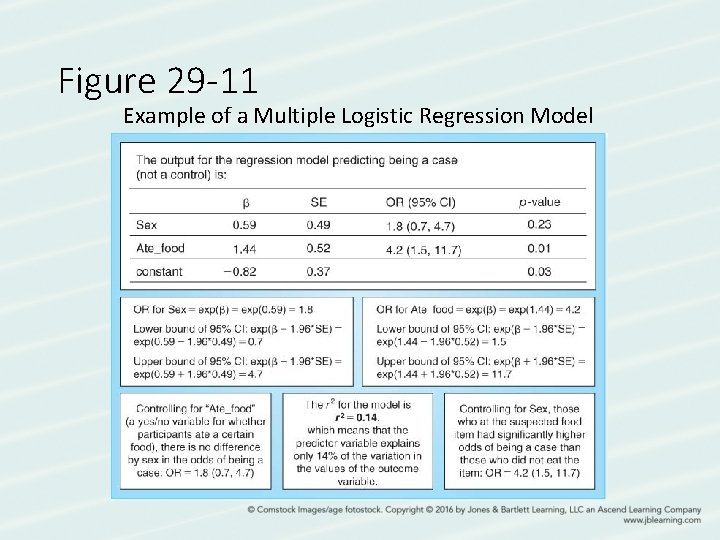
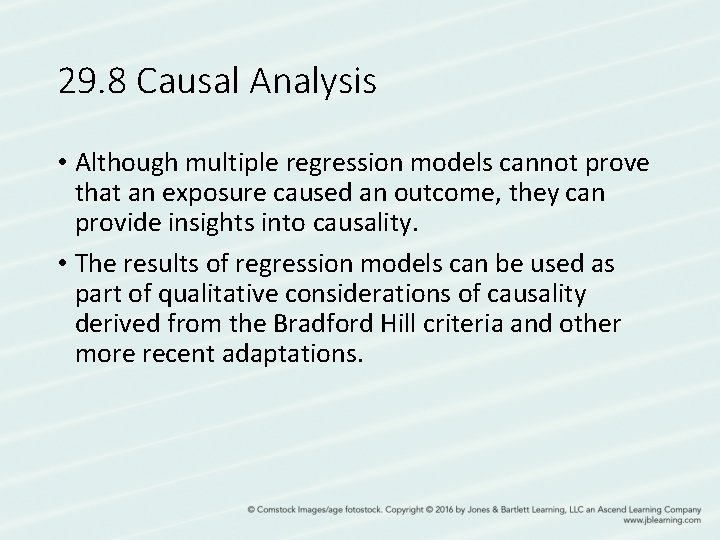
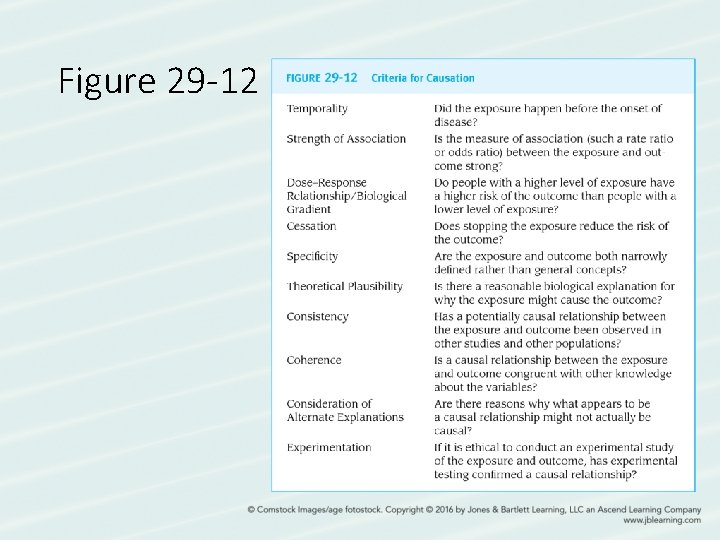
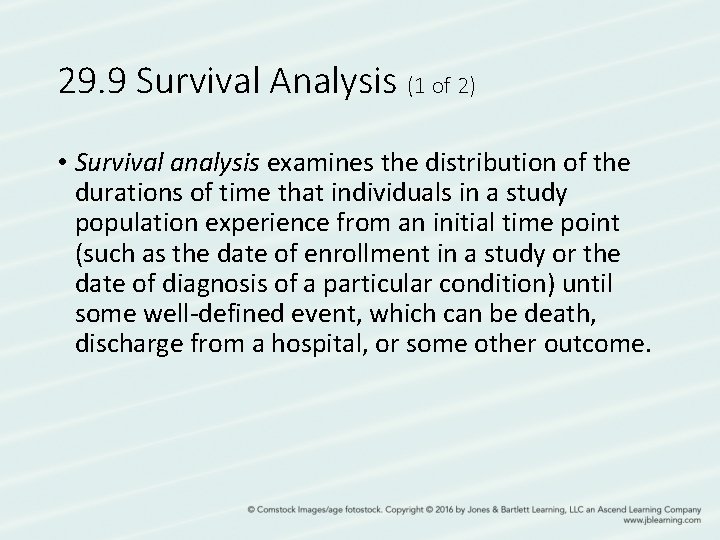
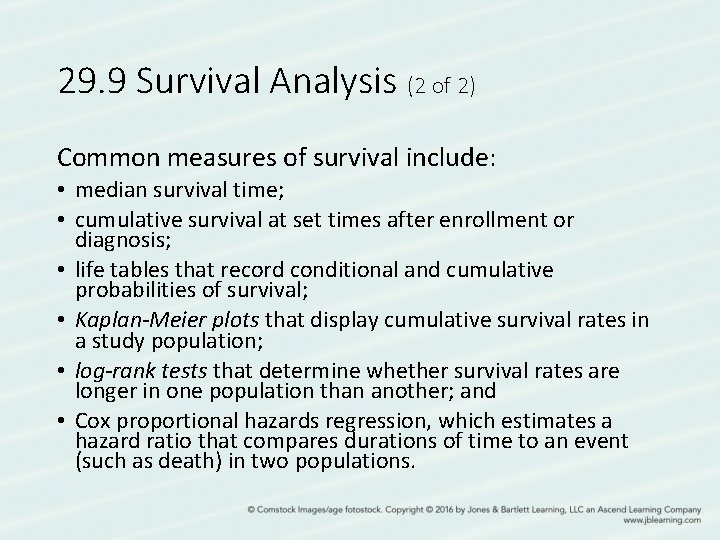
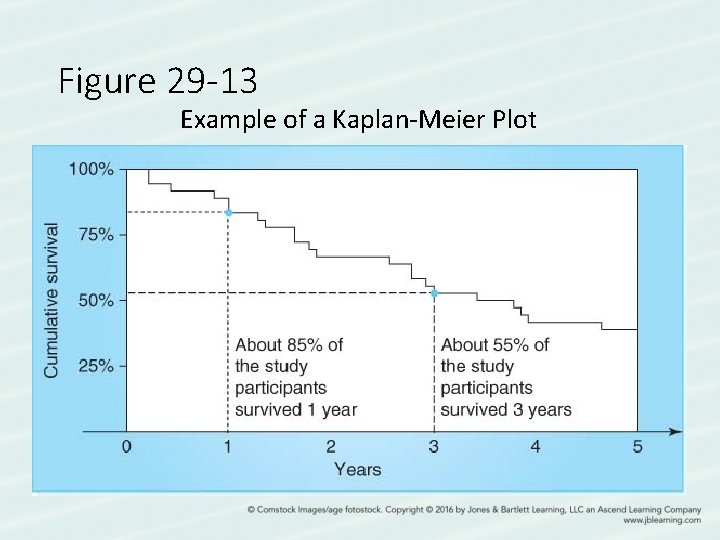
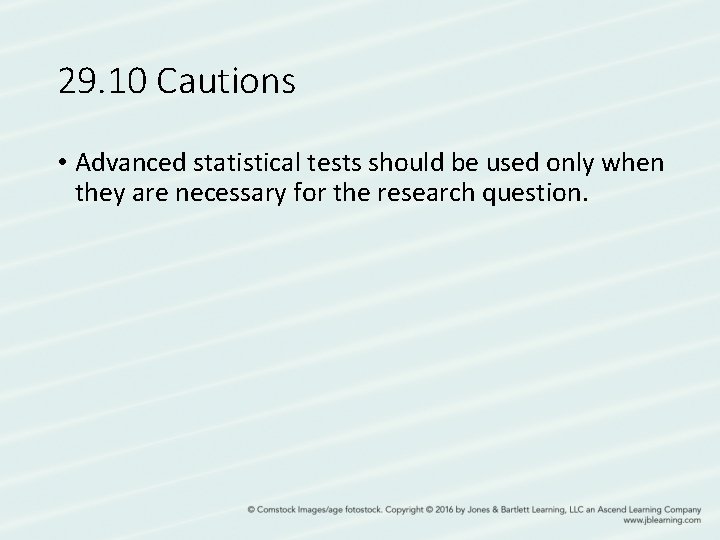
- Slides: 25
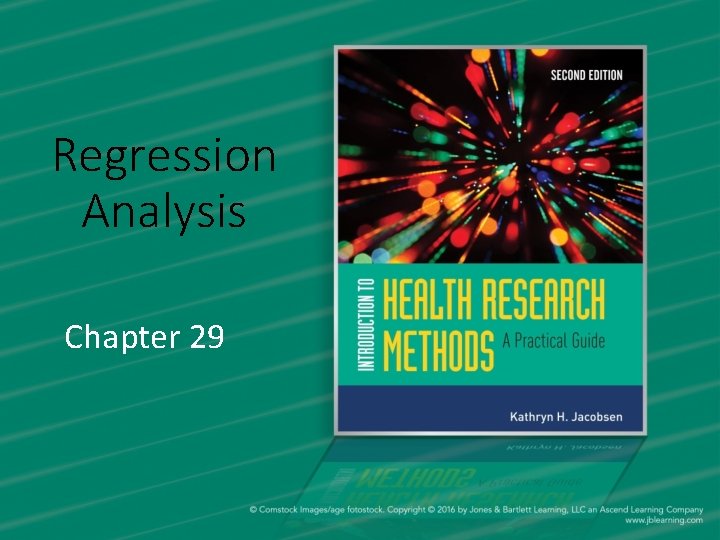
Regression Analysis Chapter 29
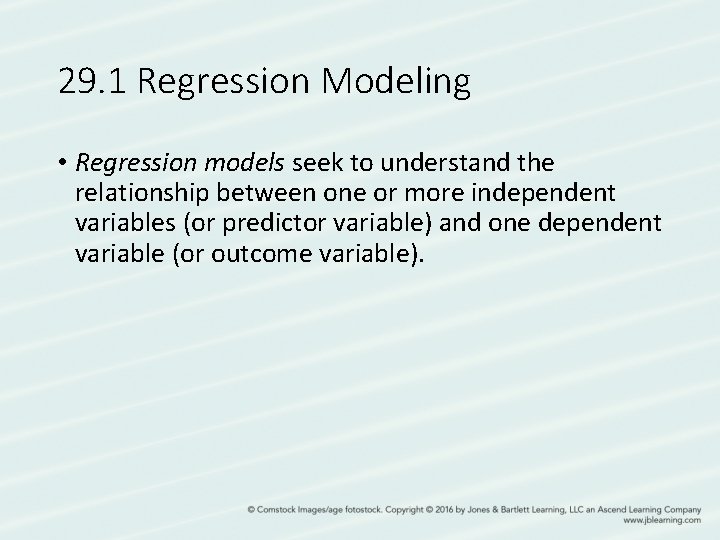
29. 1 Regression Modeling • Regression models seek to understand the relationship between one or more independent variables (or predictor variable) and one dependent variable (or outcome variable).
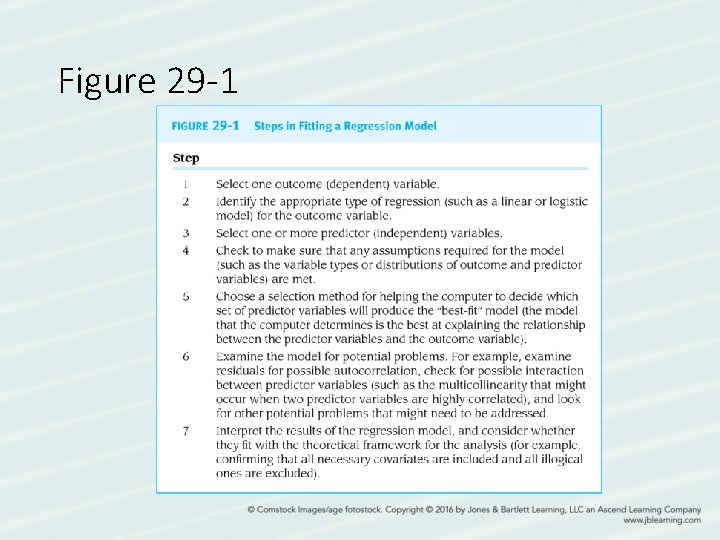
Figure 29 -1
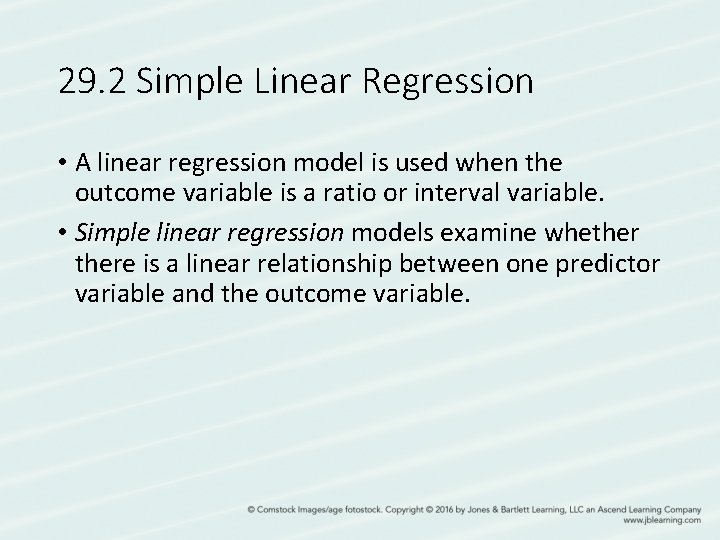
29. 2 Simple Linear Regression • A linear regression model is used when the outcome variable is a ratio or interval variable. • Simple linear regression models examine whethere is a linear relationship between one predictor variable and the outcome variable.
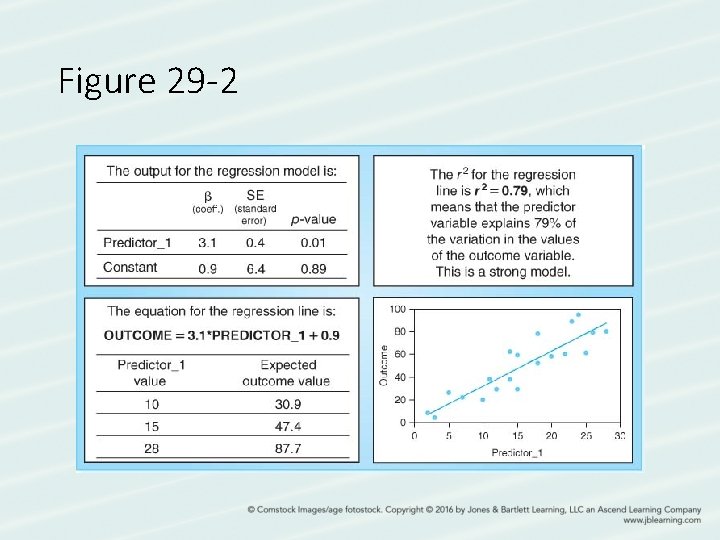
Figure 29 -2
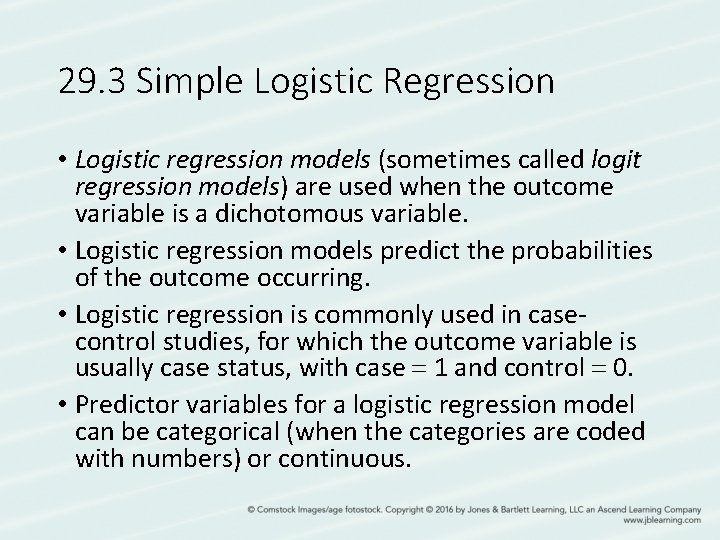
29. 3 Simple Logistic Regression • Logistic regression models (sometimes called logit regression models) are used when the outcome variable is a dichotomous variable. • Logistic regression models predict the probabilities of the outcome occurring. • Logistic regression is commonly used in casecontrol studies, for which the outcome variable is usually case status, with case 1 and control 0. • Predictor variables for a logistic regression model can be categorical (when the categories are coded with numbers) or continuous.
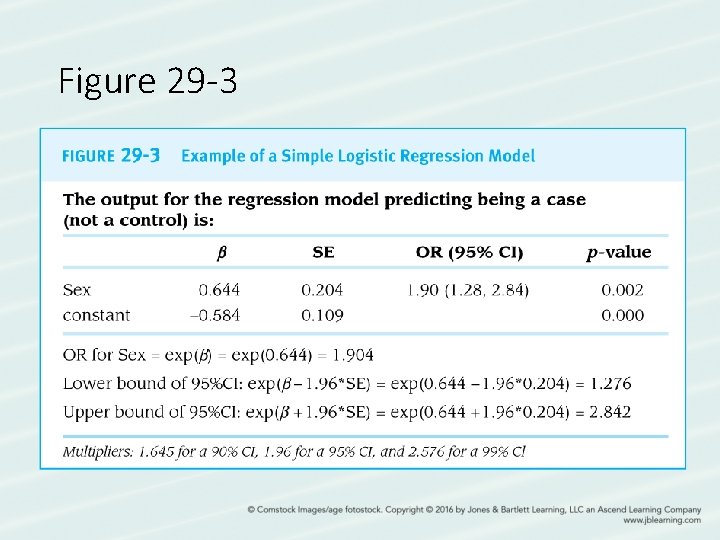
Figure 29 -3
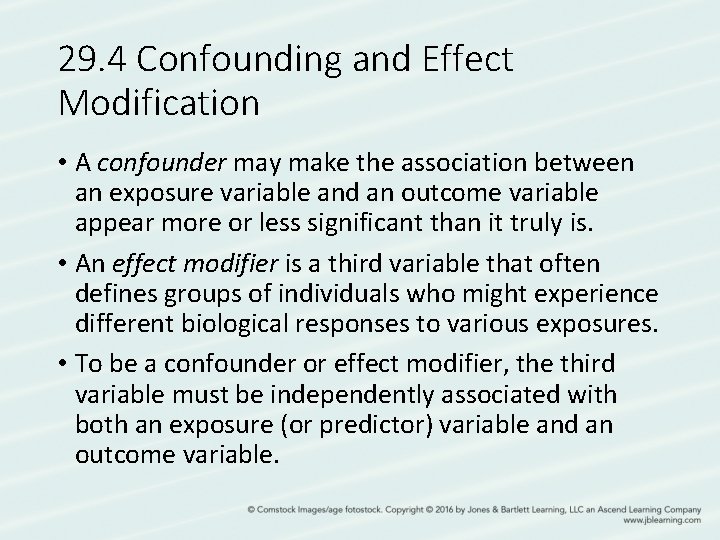
29. 4 Confounding and Effect Modification • A confounder may make the association between an exposure variable and an outcome variable appear more or less significant than it truly is. • An effect modifier is a third variable that often defines groups of individuals who might experience different biological responses to various exposures. • To be a confounder or effect modifier, the third variable must be independently associated with both an exposure (or predictor) variable and an outcome variable.
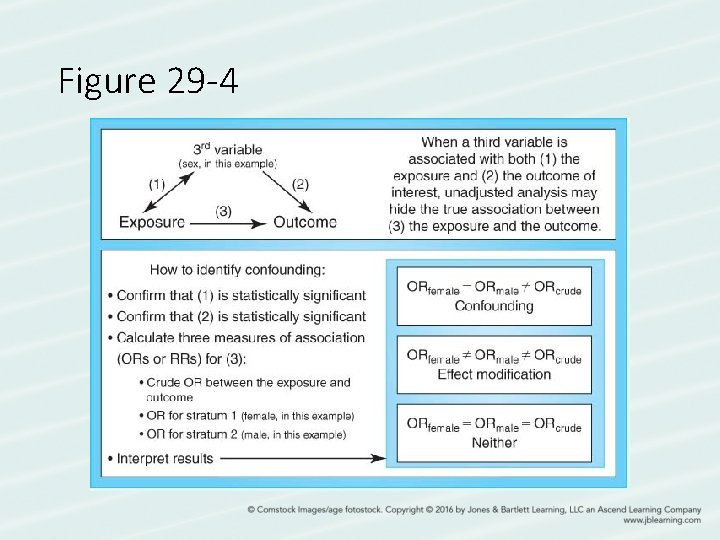
Figure 29 -4
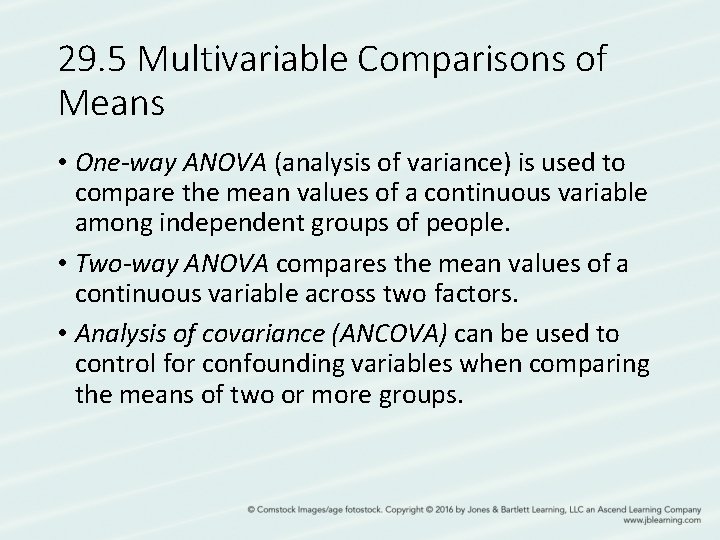
29. 5 Multivariable Comparisons of Means • One-way ANOVA (analysis of variance) is used to compare the mean values of a continuous variable among independent groups of people. • Two-way ANOVA compares the mean values of a continuous variable across two factors. • Analysis of covariance (ANCOVA) can be used to control for confounding variables when comparing the means of two or more groups.
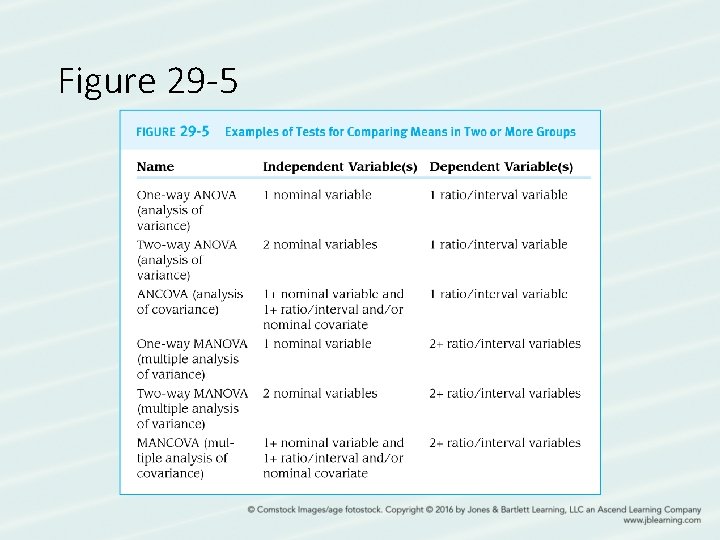
Figure 29 -5
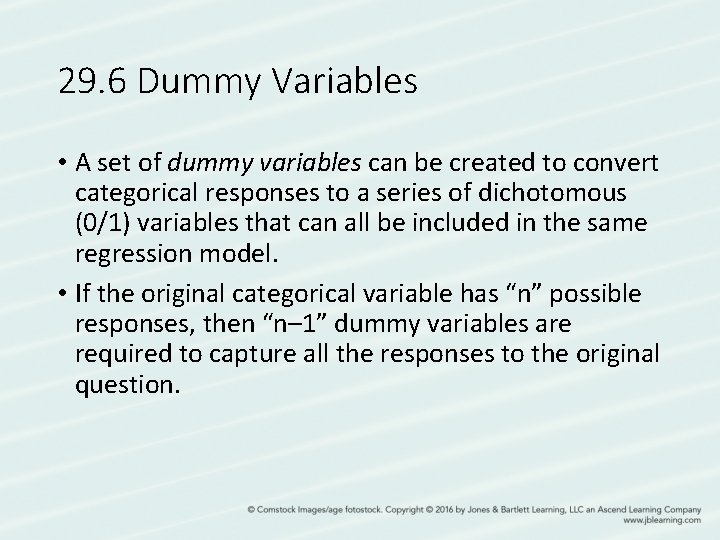
29. 6 Dummy Variables • A set of dummy variables can be created to convert categorical responses to a series of dichotomous (0/1) variables that can all be included in the same regression model. • If the original categorical variable has “n” possible responses, then “n– 1” dummy variables are required to capture all the responses to the original question.
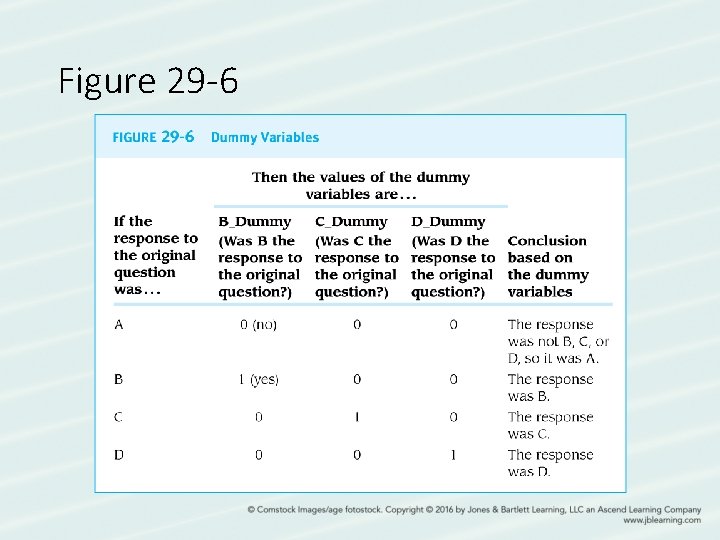
Figure 29 -6
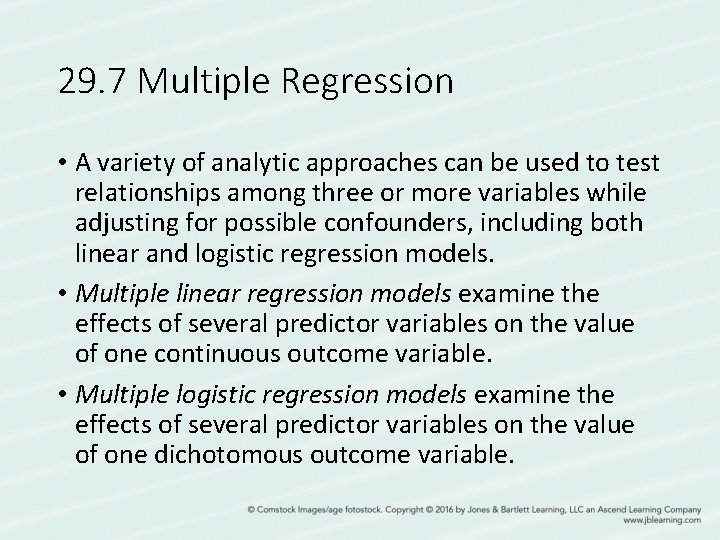
29. 7 Multiple Regression • A variety of analytic approaches can be used to test relationships among three or more variables while adjusting for possible confounders, including both linear and logistic regression models. • Multiple linear regression models examine the effects of several predictor variables on the value of one continuous outcome variable. • Multiple logistic regression models examine the effects of several predictor variables on the value of one dichotomous outcome variable.
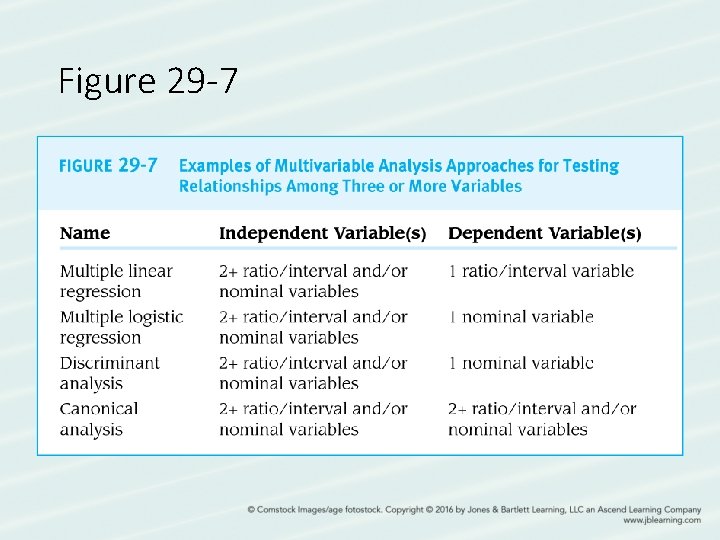
Figure 29 -7
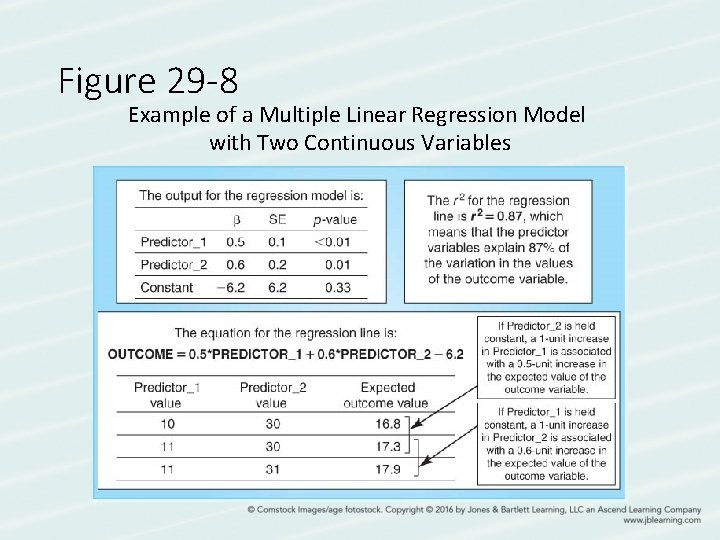
Figure 29 -8 Example of a Multiple Linear Regression Model with Two Continuous Variables
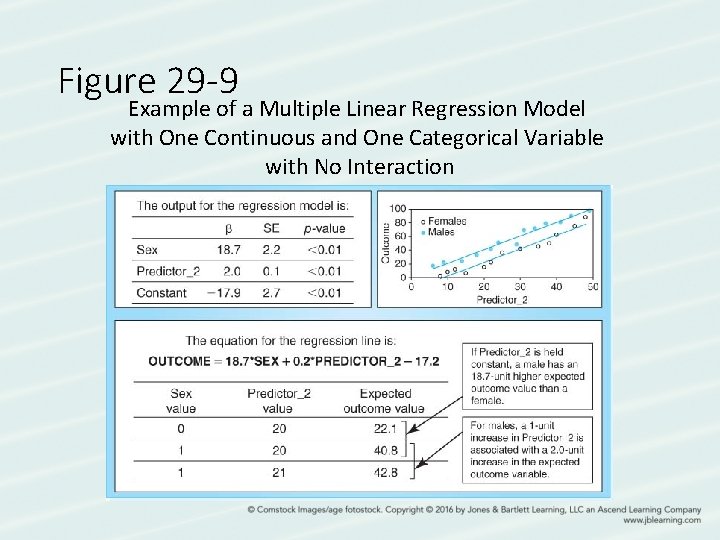
Figure 29 -9 Example of a Multiple Linear Regression Model with One Continuous and One Categorical Variable with No Interaction
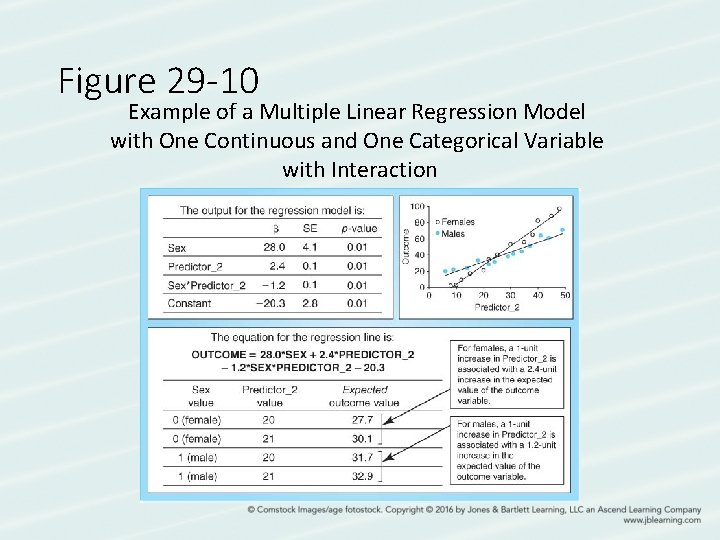
Figure 29 -10 Example of a Multiple Linear Regression Model with One Continuous and One Categorical Variable with Interaction
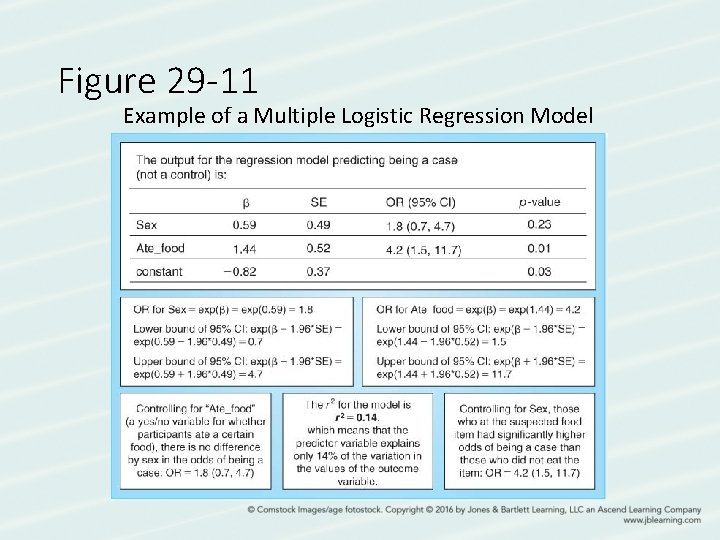
Figure 29 -11 Example of a Multiple Logistic Regression Model
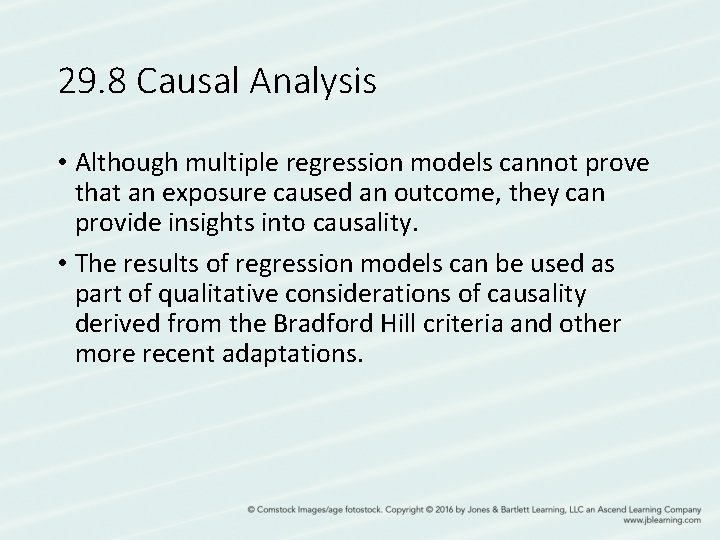
29. 8 Causal Analysis • Although multiple regression models cannot prove that an exposure caused an outcome, they can provide insights into causality. • The results of regression models can be used as part of qualitative considerations of causality derived from the Bradford Hill criteria and other more recent adaptations.
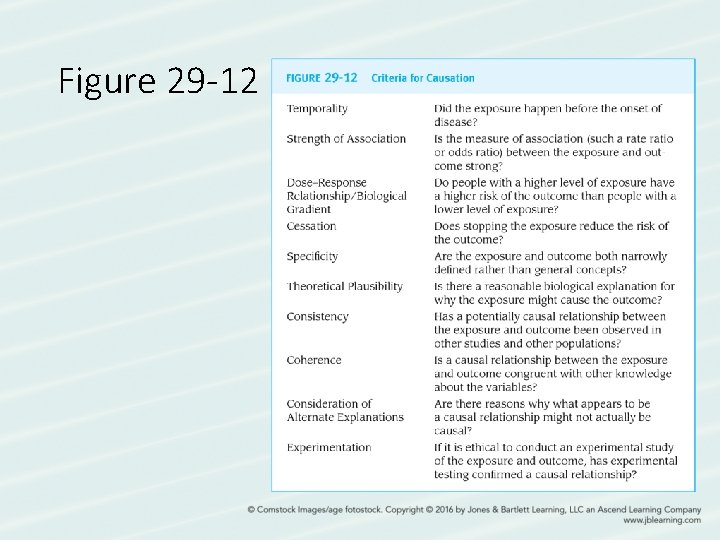
Figure 29 -12
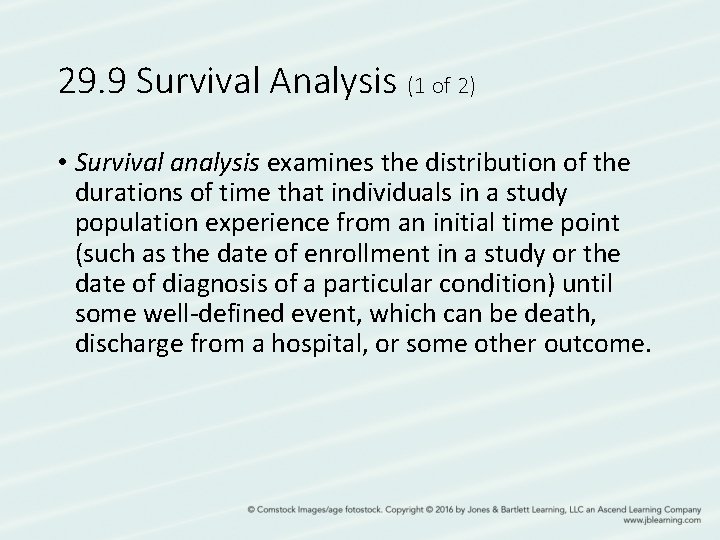
29. 9 Survival Analysis (1 of 2) • Survival analysis examines the distribution of the durations of time that individuals in a study population experience from an initial time point (such as the date of enrollment in a study or the date of diagnosis of a particular condition) until some well-defined event, which can be death, discharge from a hospital, or some other outcome.
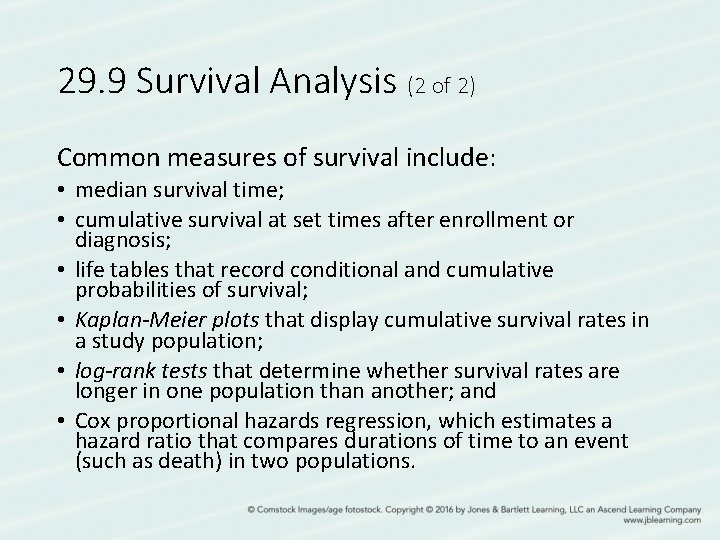
29. 9 Survival Analysis (2 of 2) Common measures of survival include: • median survival time; • cumulative survival at set times after enrollment or diagnosis; • life tables that record conditional and cumulative probabilities of survival; • Kaplan-Meier plots that display cumulative survival rates in a study population; • log-rank tests that determine whether survival rates are longer in one population than another; and • Cox proportional hazards regression, which estimates a hazard ratio that compares durations of time to an event (such as death) in two populations.
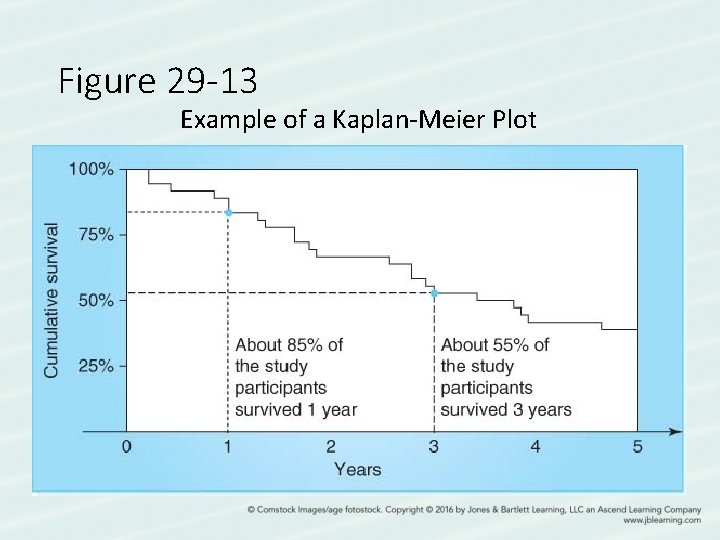
Figure 29 -13 Example of a Kaplan-Meier Plot
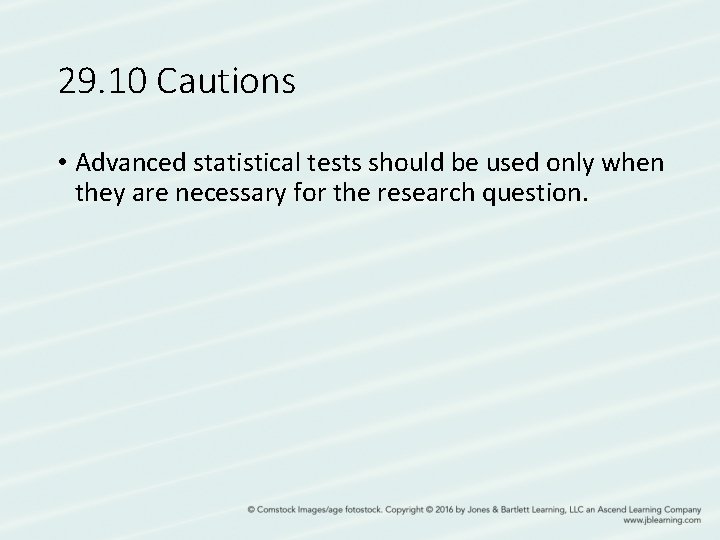
29. 10 Cautions • Advanced statistical tests should be used only when they are necessary for the research question.
Modeling role modeling theory
Dimensional modeling vs relational modeling
3-5 practice modeling with nonlinear regression
Simple linear regression and multiple linear regression
Multiple regression vs simple regression
Survival analysis vs logistic regression
Logistic regression vs linear regression
Simulation modeling and analysis law kelton
Answers key
System requirements checklist output example
Competency modeling vs job analysis
Manufacturing systems modeling and analysis
A job analysis that produces a valid selection
Chapter 2 modeling distributions of data
Chapter 2 modeling distributions of data
Bridge entity erd
Excel
Disadvantages of regression analysis
Minitab regression analysis
Vif in regression spss
Multiple regression analysis with qualitative information
Definition of multiple regression
Multiple regression analysis adalah
Calculation of coefficient of determination
Modelling relationships and trends in data
Regression equation in excel