Quantum Computing and Dynamical Quantum Models quantph0205059 Scott
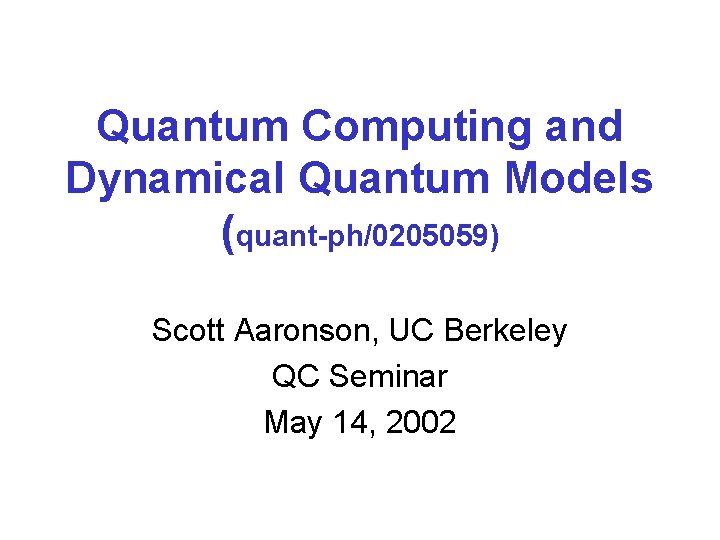
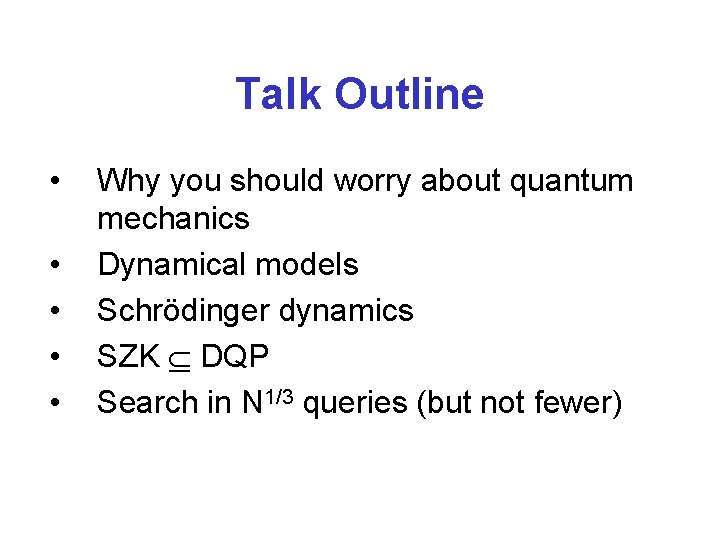
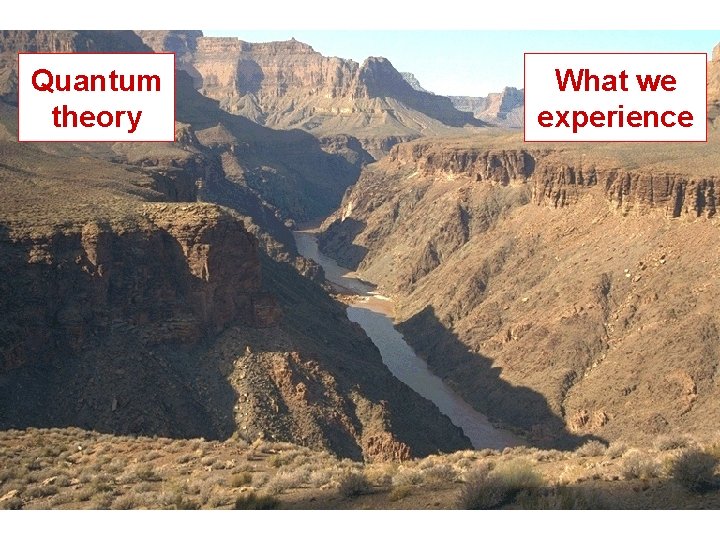
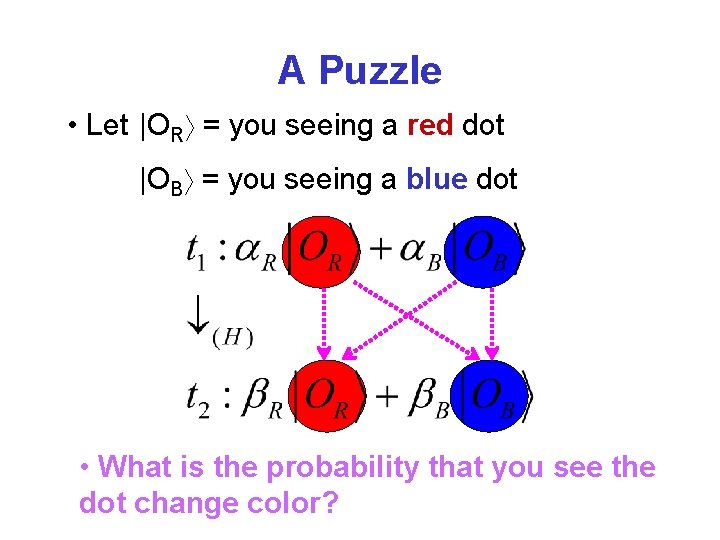
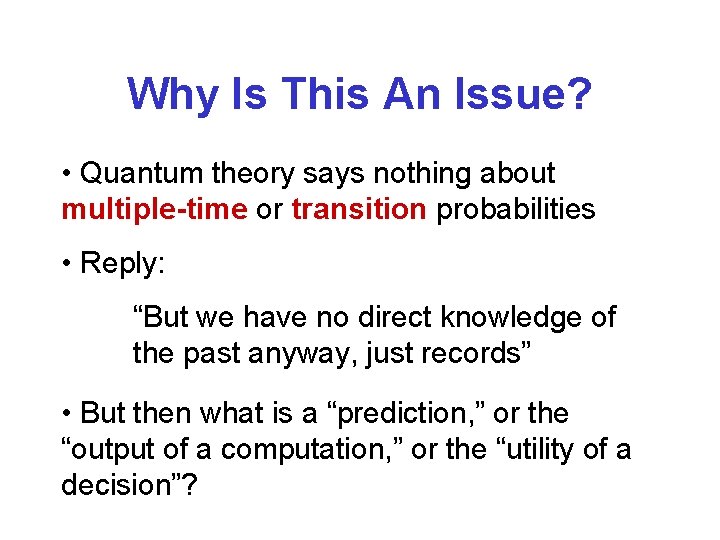
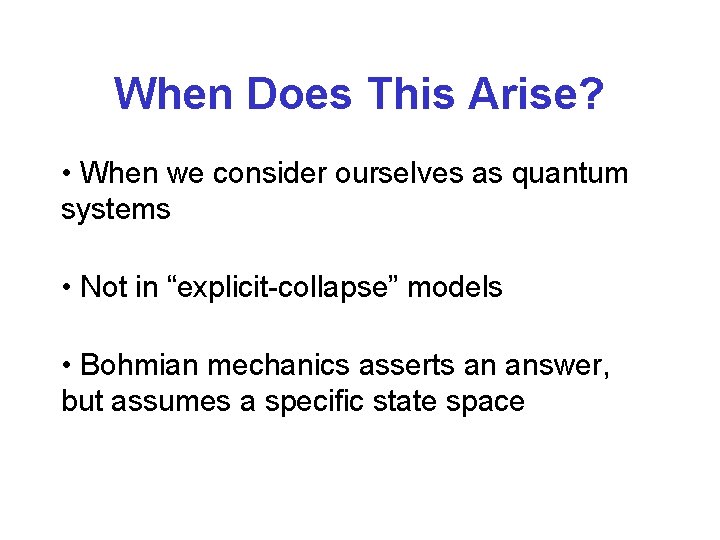
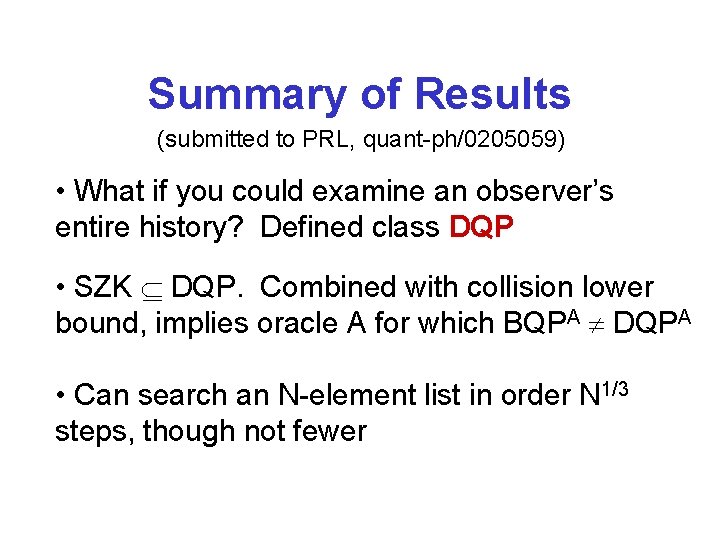
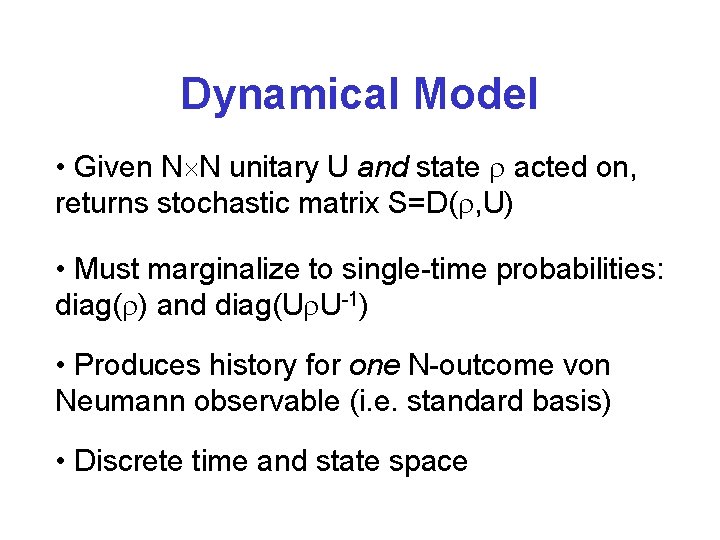
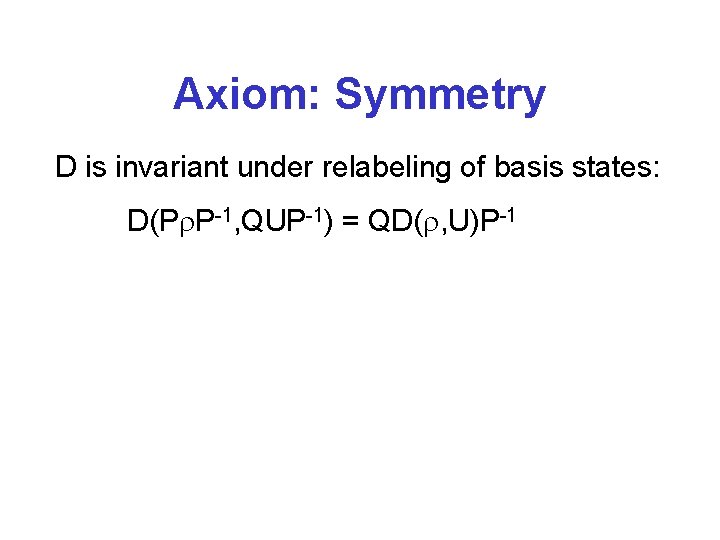
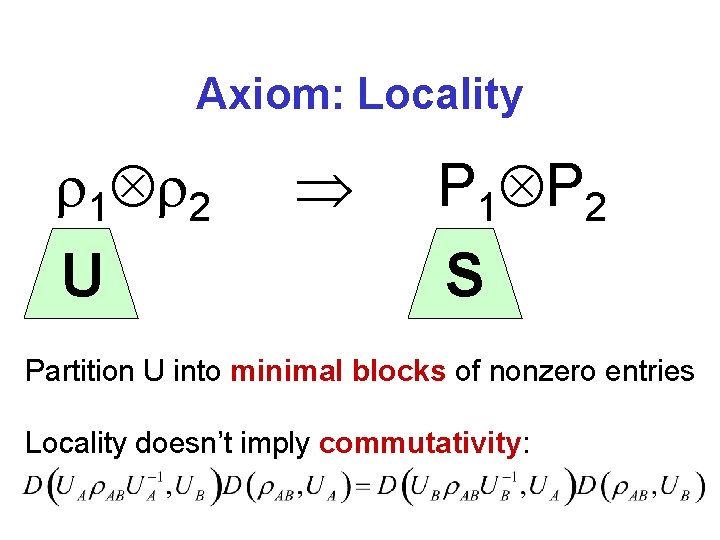
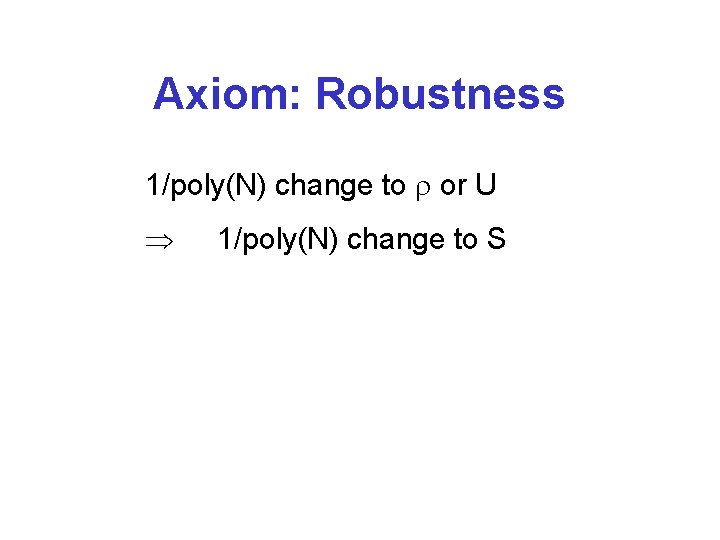
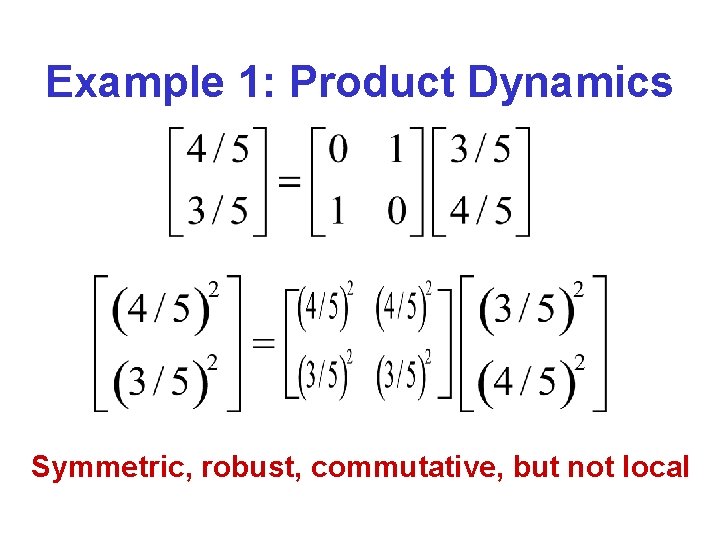
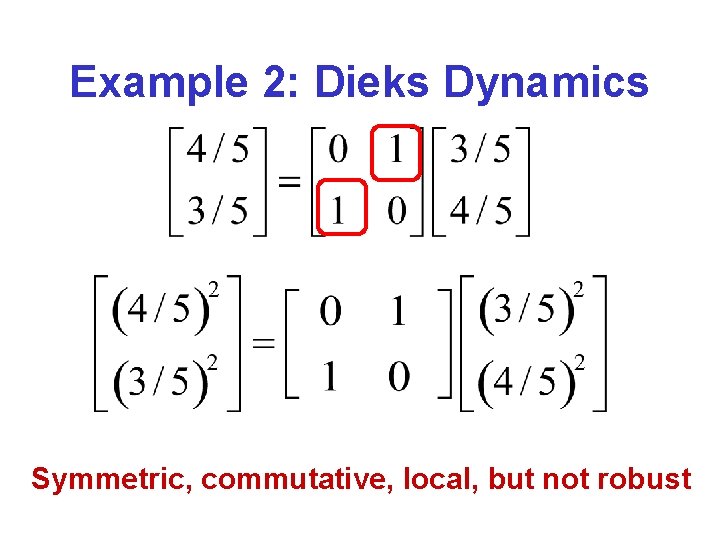
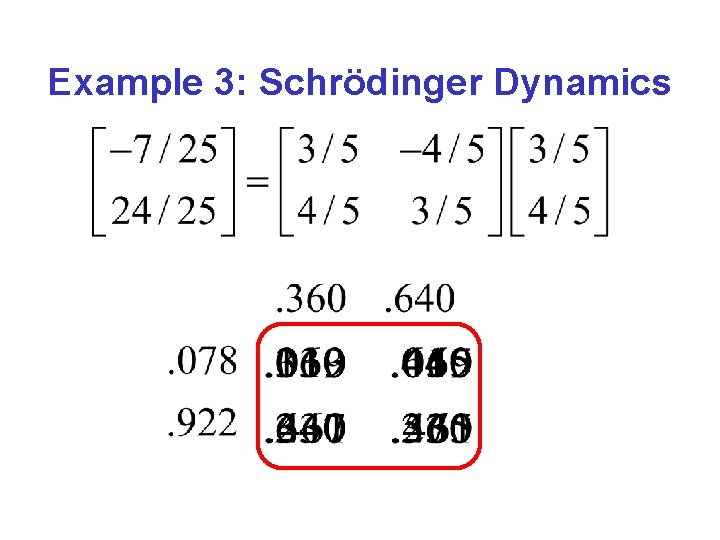
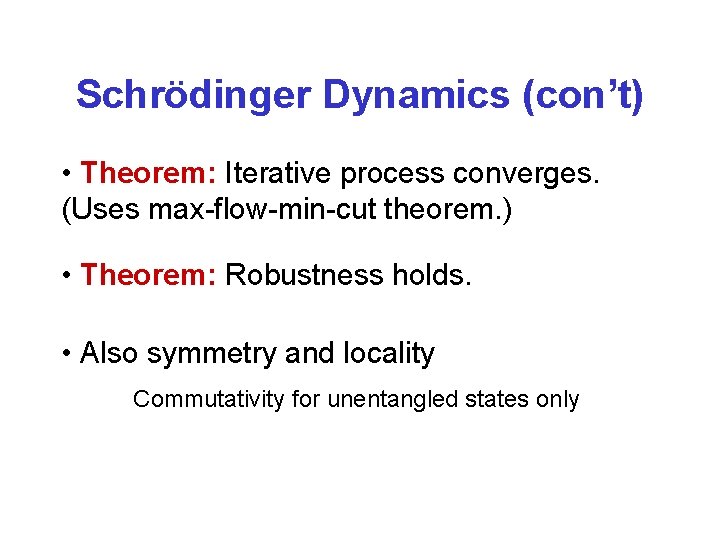
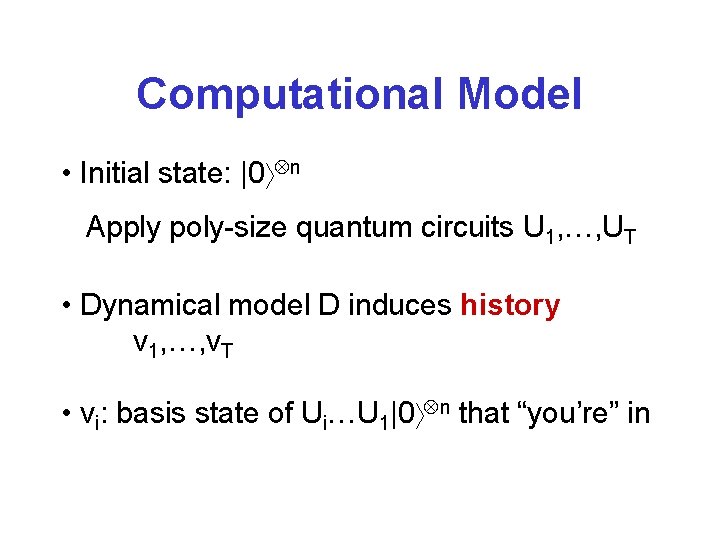
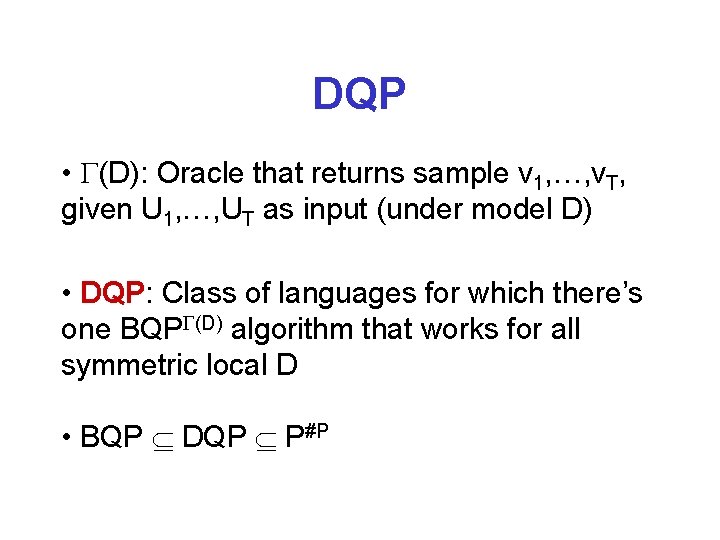
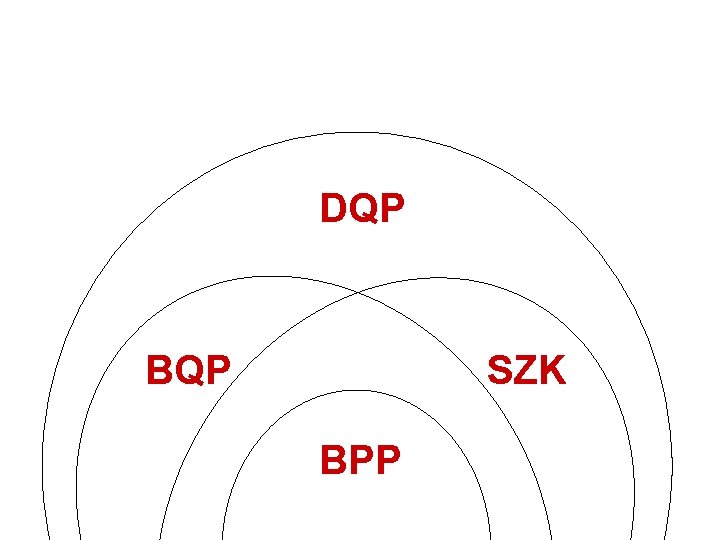
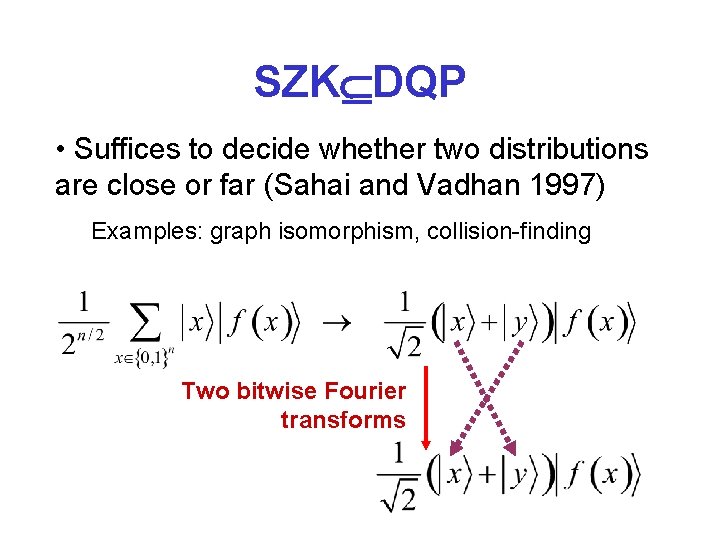
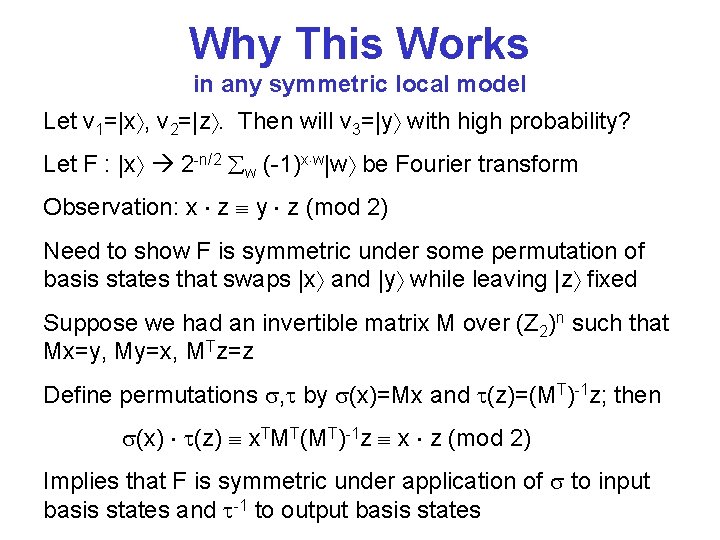
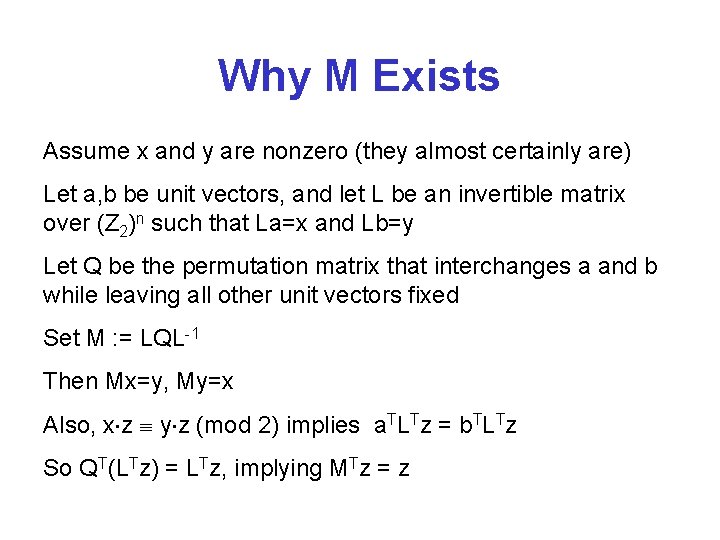
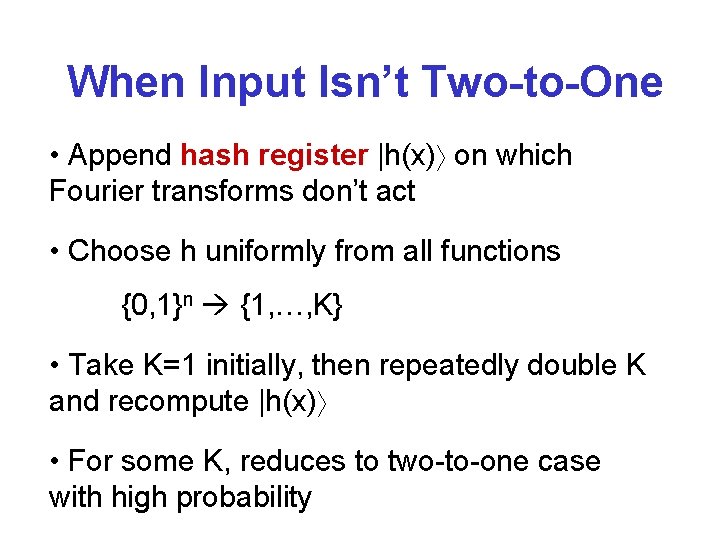
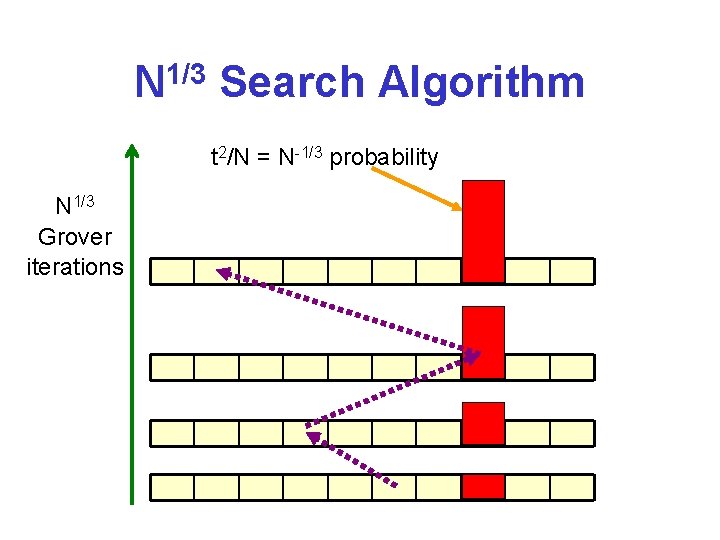
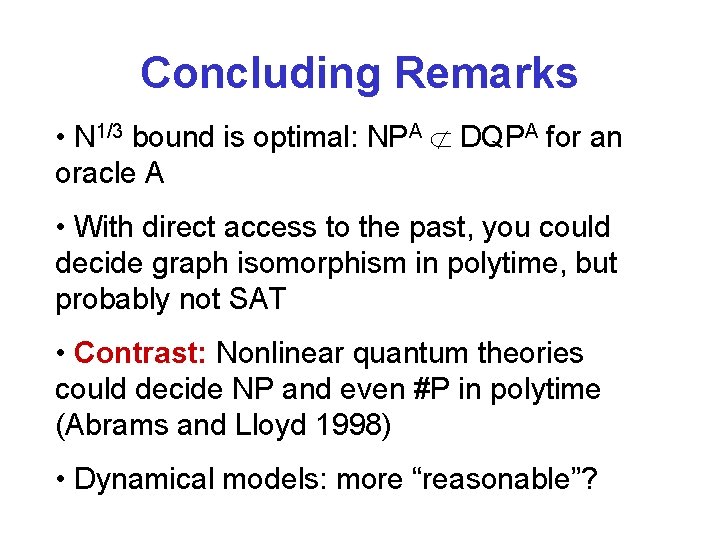
- Slides: 24
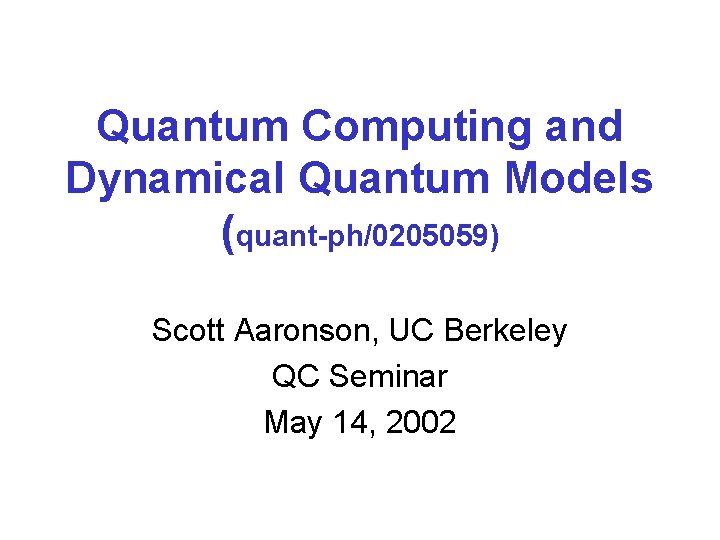
Quantum Computing and Dynamical Quantum Models (quant-ph/0205059) Scott Aaronson, UC Berkeley QC Seminar May 14, 2002
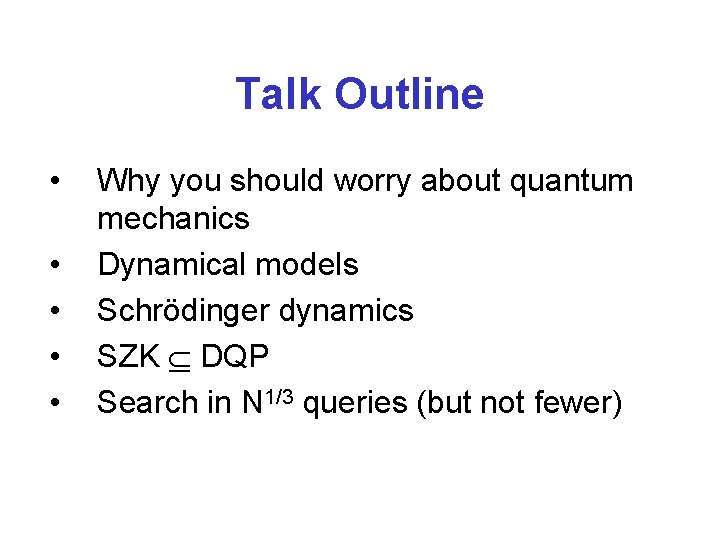
Talk Outline • • • Why you should worry about quantum mechanics Dynamical models Schrödinger dynamics SZK DQP Search in N 1/3 queries (but not fewer)
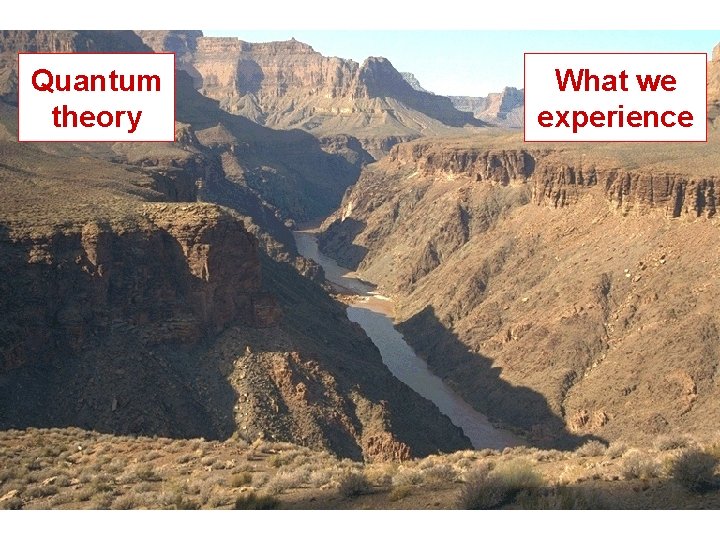
Quantum theory What we experience
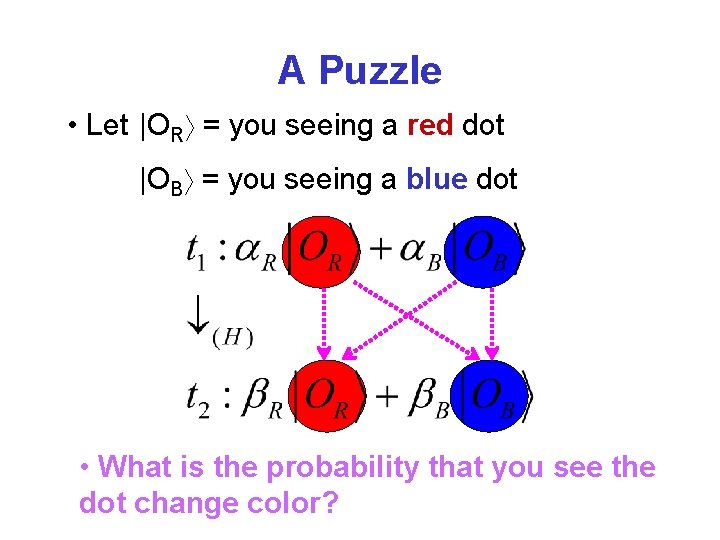
A Puzzle • Let |OR = you seeing a red dot |OB = you seeing a blue dot • What is the probability that you see the dot change color?
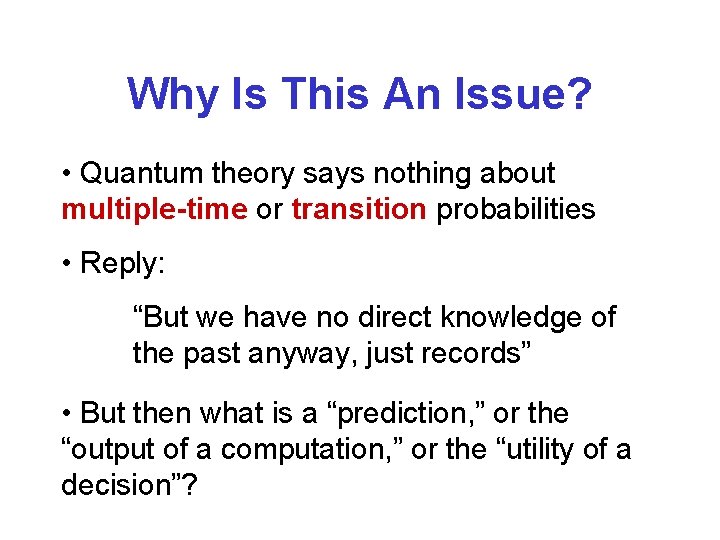
Why Is This An Issue? • Quantum theory says nothing about multiple-time or transition probabilities • Reply: “But we have no direct knowledge of the past anyway, just records” • But then what is a “prediction, ” or the “output of a computation, ” or the “utility of a decision”?
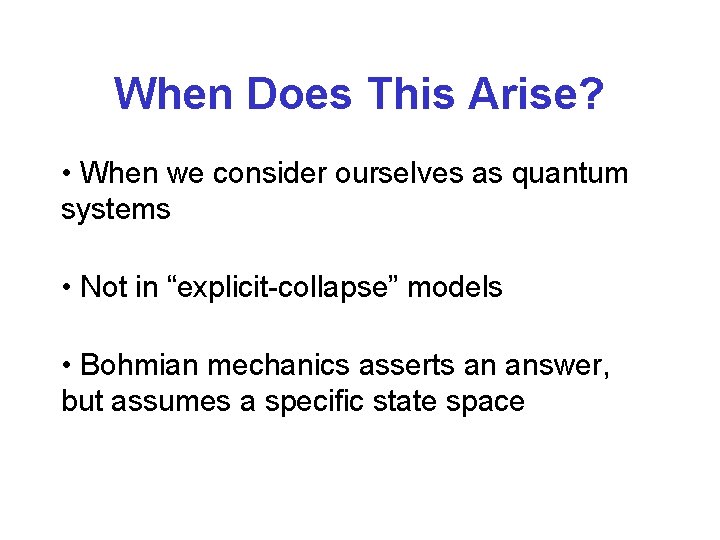
When Does This Arise? • When we consider ourselves as quantum systems • Not in “explicit-collapse” models • Bohmian mechanics asserts an answer, but assumes a specific state space
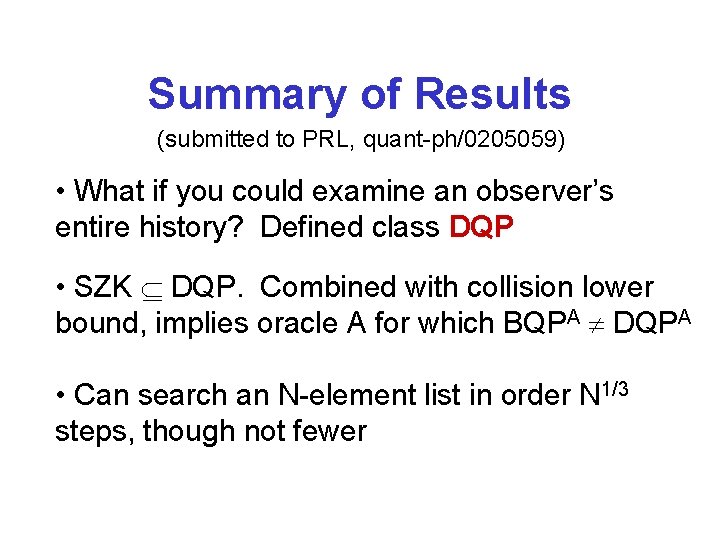
Summary of Results (submitted to PRL, quant-ph/0205059) • What if you could examine an observer’s entire history? Defined class DQP • SZK DQP. Combined with collision lower bound, implies oracle A for which BQPA DQPA • Can search an N-element list in order N 1/3 steps, though not fewer
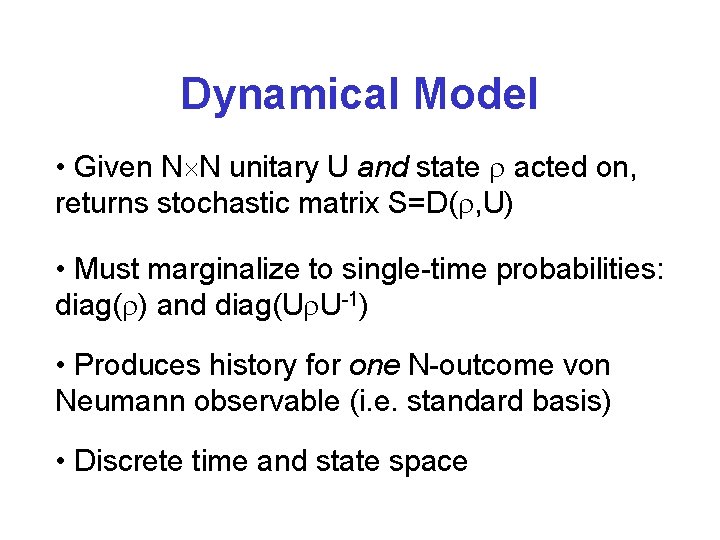
Dynamical Model • Given N N unitary U and state acted on, returns stochastic matrix S=D( , U) • Must marginalize to single-time probabilities: diag( ) and diag(U U-1) • Produces history for one N-outcome von Neumann observable (i. e. standard basis) • Discrete time and state space
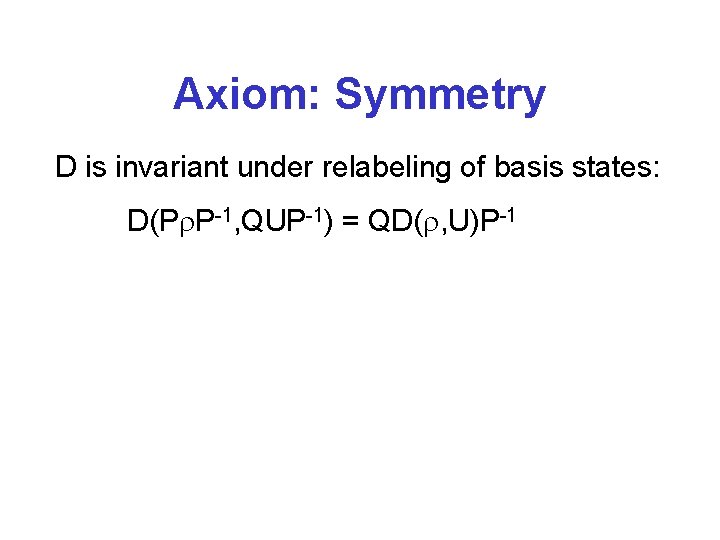
Axiom: Symmetry D is invariant under relabeling of basis states: D(P P-1, QUP-1) = QD( , U)P-1
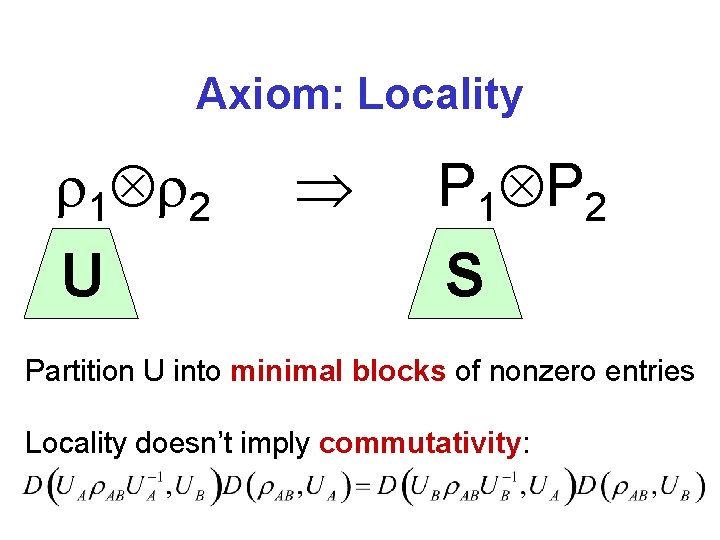
Axiom: Locality 1 2 U P 1 P 2 S Partition U into minimal blocks of nonzero entries Locality doesn’t imply commutativity:
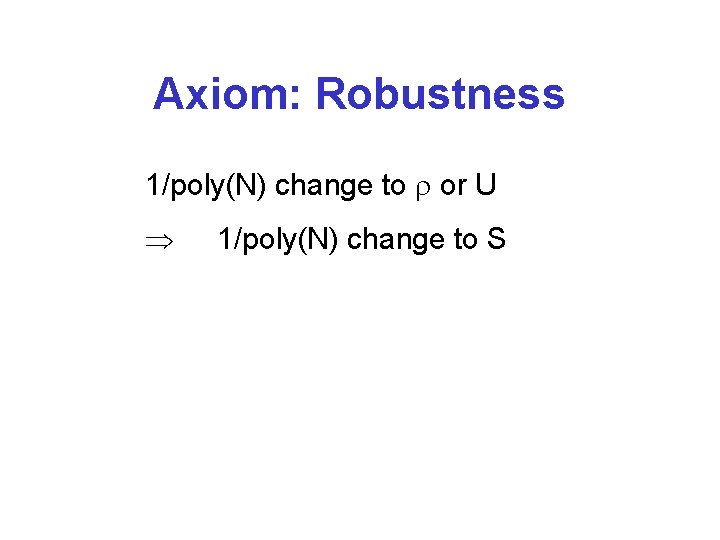
Axiom: Robustness 1/poly(N) change to or U 1/poly(N) change to S
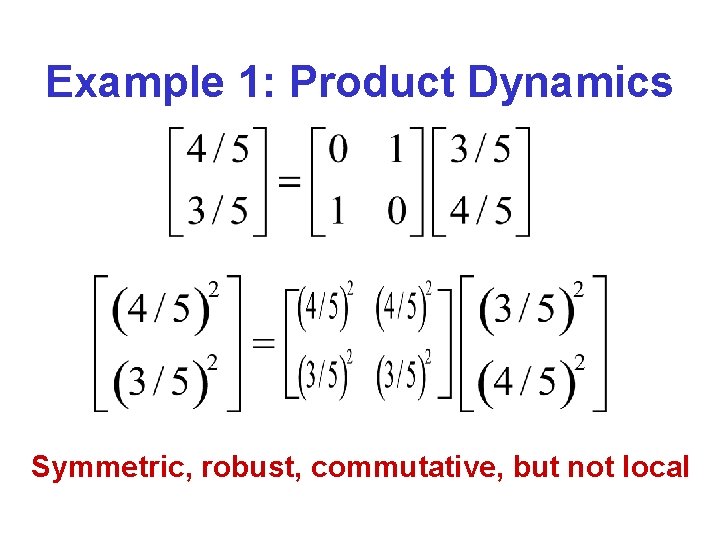
Example 1: Product Dynamics Symmetric, robust, commutative, but not local
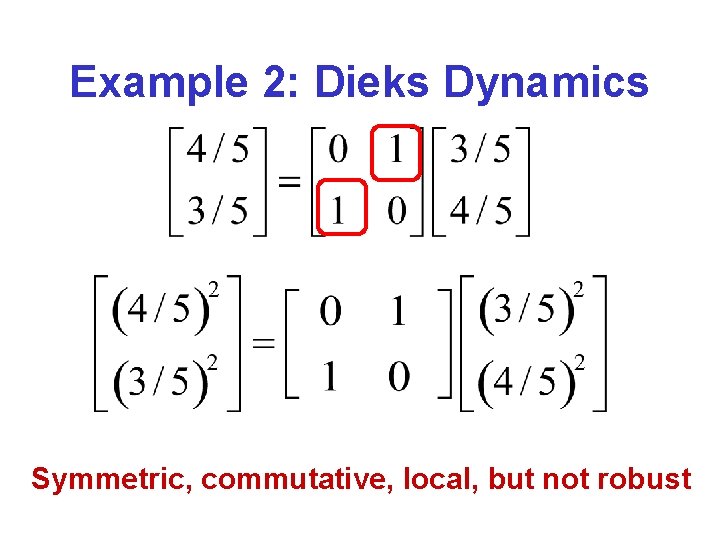
Example 2: Dieks Dynamics Symmetric, commutative, local, but not robust
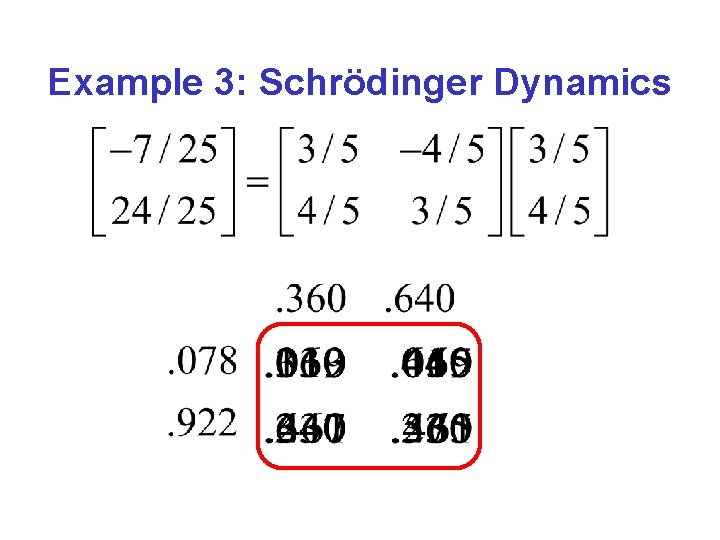
Example 3: Schrödinger Dynamics
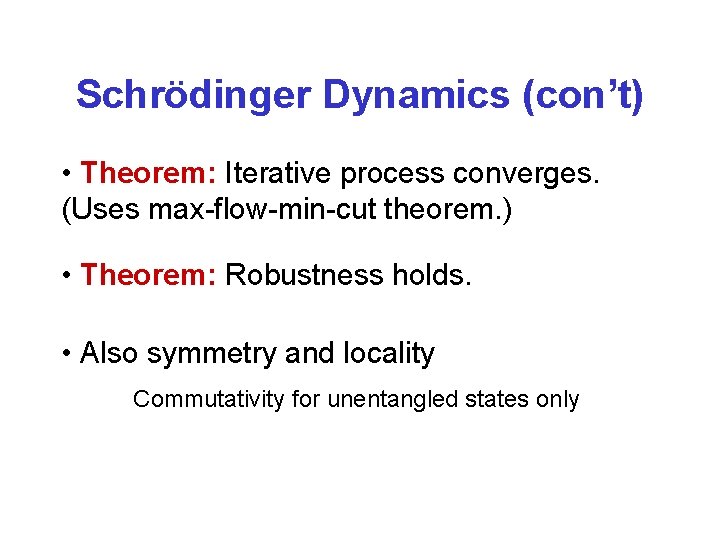
Schrödinger Dynamics (con’t) • Theorem: Iterative process converges. (Uses max-flow-min-cut theorem. ) • Theorem: Robustness holds. • Also symmetry and locality Commutativity for unentangled states only
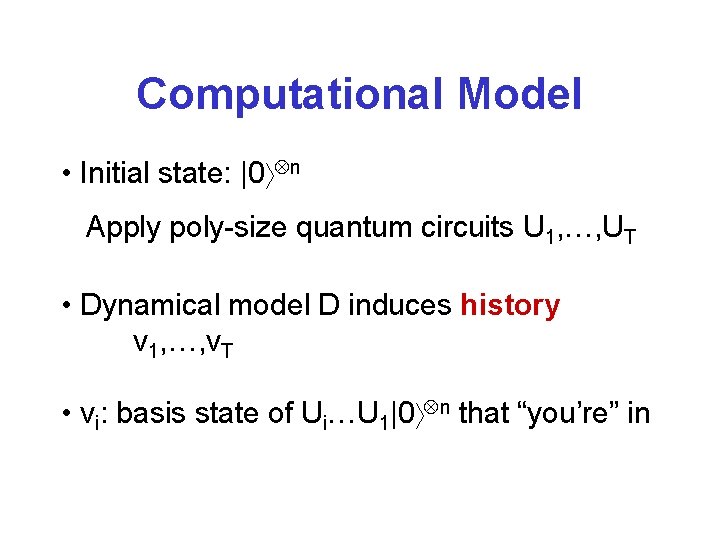
Computational Model • Initial state: |0 n Apply poly-size quantum circuits U 1, …, UT • Dynamical model D induces history v 1, …, v. T • vi: basis state of Ui U 1|0 n that “you’re” in
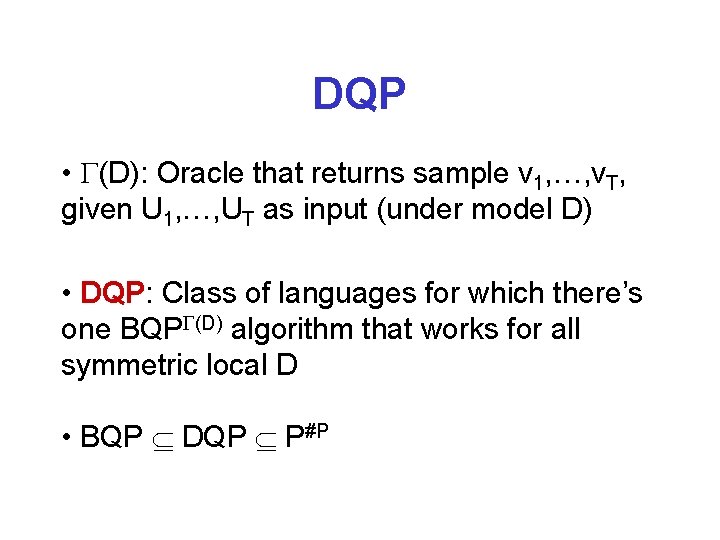
DQP • (D): Oracle that returns sample v 1, …, v. T, given U 1, …, UT as input (under model D) • DQP: Class of languages for which there’s one BQP (D) algorithm that works for all symmetric local D • BQP DQP P#P
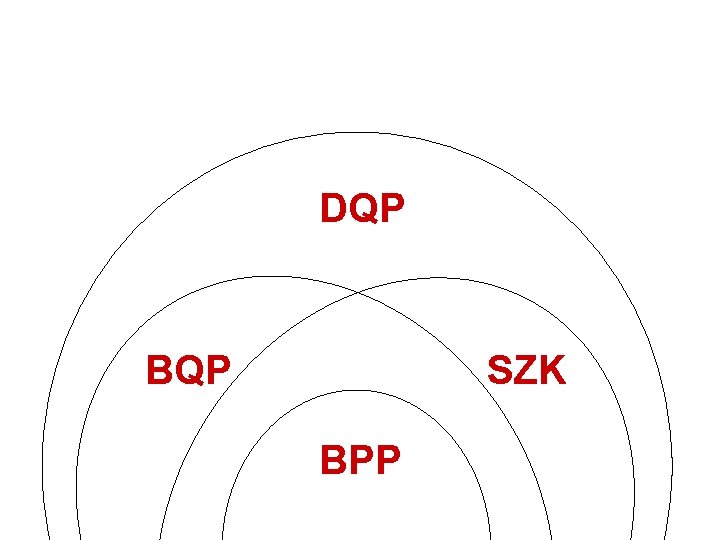
DQP BQP SZK BPP
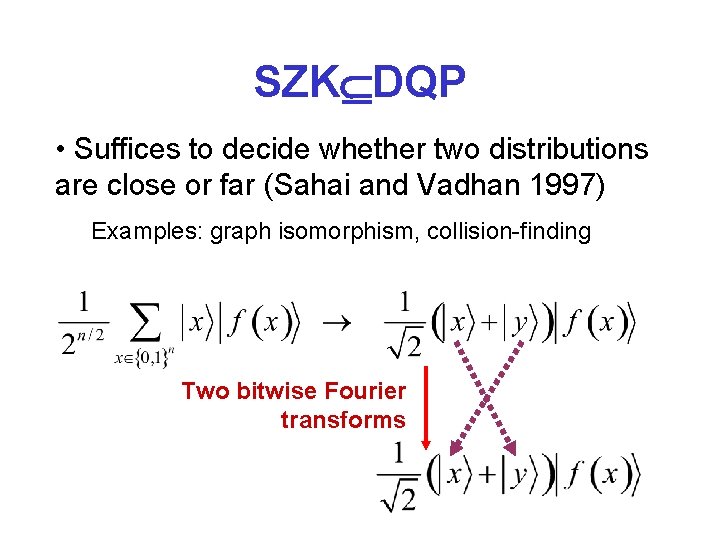
SZK DQP • Suffices to decide whether two distributions are close or far (Sahai and Vadhan 1997) Examples: graph isomorphism, collision-finding Two bitwise Fourier transforms
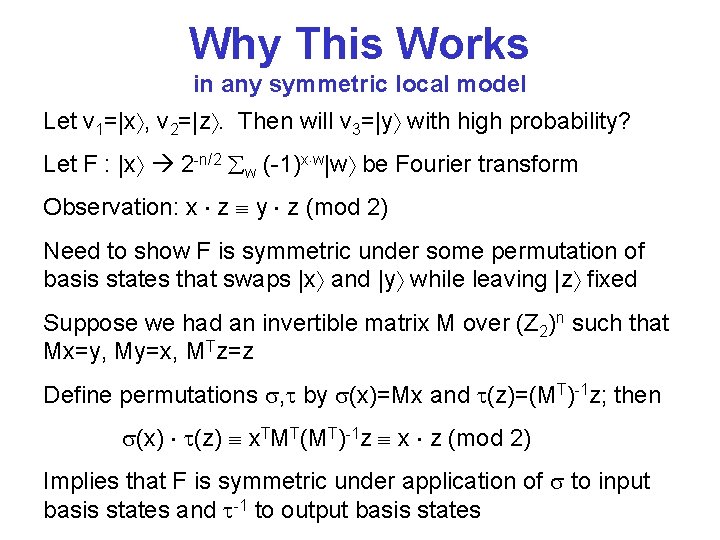
Why This Works in any symmetric local model Let v 1=|x , v 2=|z. Then will v 3=|y with high probability? Let F : |x 2 -n/2 w (-1)x w|w be Fourier transform Observation: x z y z (mod 2) Need to show F is symmetric under some permutation of basis states that swaps |x and |y while leaving |z fixed Suppose we had an invertible matrix M over (Z 2)n such that Mx=y, My=x, MTz=z Define permutations , by (x)=Mx and (z)=(MT)-1 z; then (x) (z) x. TMT(MT)-1 z x z (mod 2) Implies that F is symmetric under application of to input basis states and -1 to output basis states
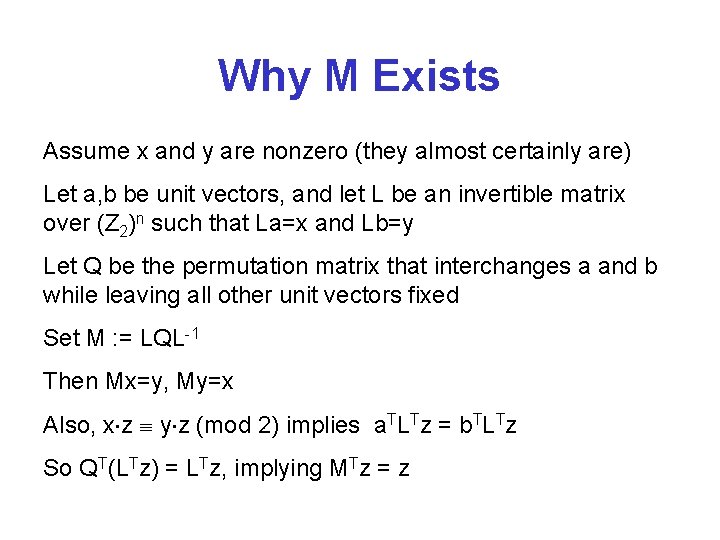
Why M Exists Assume x and y are nonzero (they almost certainly are) Let a, b be unit vectors, and let L be an invertible matrix over (Z 2)n such that La=x and Lb=y Let Q be the permutation matrix that interchanges a and b while leaving all other unit vectors fixed Set M : = LQL-1 Then Mx=y, My=x Also, x z y z (mod 2) implies a. TLTz = b. TLTz So QT(LTz) = LTz, implying MTz = z
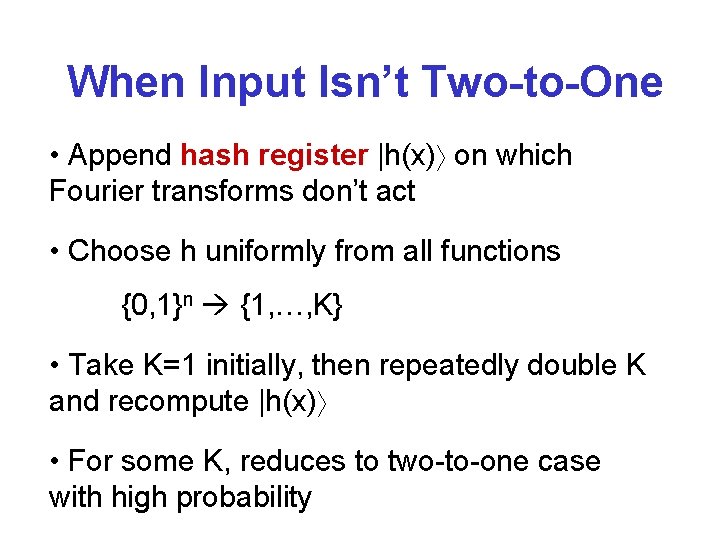
When Input Isn’t Two-to-One • Append hash register |h(x) on which Fourier transforms don’t act • Choose h uniformly from all functions {0, 1}n {1, …, K} • Take K=1 initially, then repeatedly double K and recompute |h(x) • For some K, reduces to two-to-one case with high probability
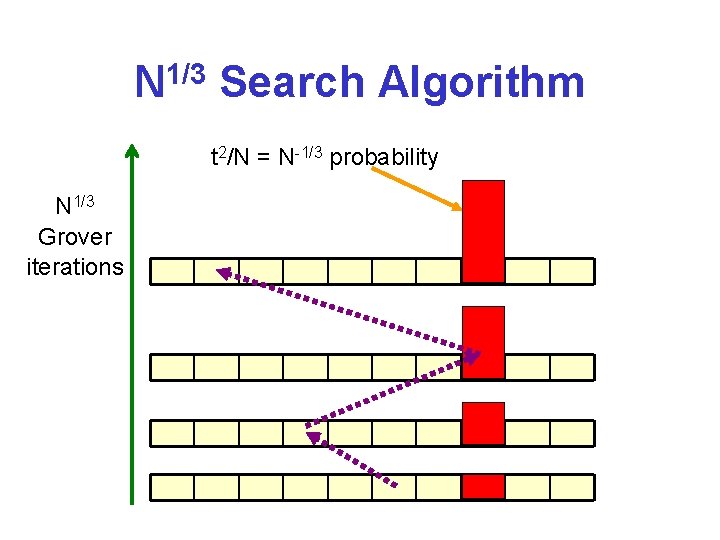
N 1/3 Search Algorithm t 2/N = N-1/3 probability N 1/3 Grover iterations
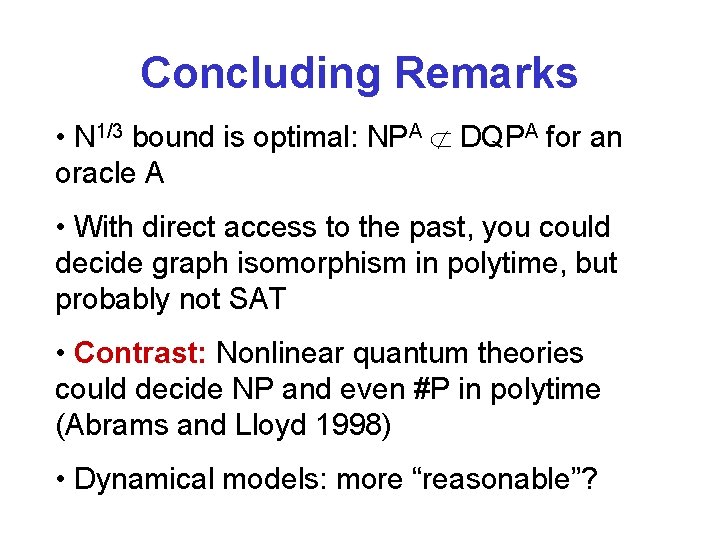
Concluding Remarks • N 1/3 bound is optimal: NPA DQPA for an oracle A • With direct access to the past, you could decide graph isomorphism in polytime, but probably not SAT • Contrast: Nonlinear quantum theories could decide NP and even #P in polytime (Abrams and Lloyd 1998) • Dynamical models: more “reasonable”?
Dynamical systems neuroscience
Motor learning theories
Barycentric dynamical time
Discrete dynamical systems examples
Dynamical mean-field theory
Solution in search of a problem
Automata
Siam conference on applications of dynamical systems
Quantum computing progress and prospects
Quantum computing
Semi modals examples
Explain system models for distributed and cloud computing
Conventional computing and intelligent computing
Quantum physics vs mechanics
Quantum physics vs quantum mechanics
Gil kalai quantum computing
Gil kalai quantum computing
Michael nielsen quantum
Tsp
Prerequisites for quantum computing
Open problems in quantum computing
Mit quantum computing
Mit quantum computing
Quantum computing meaning
Ut austin quantum computing