Practical Machine Learning Unsupervised Learning Sven Mayer Unsupervised
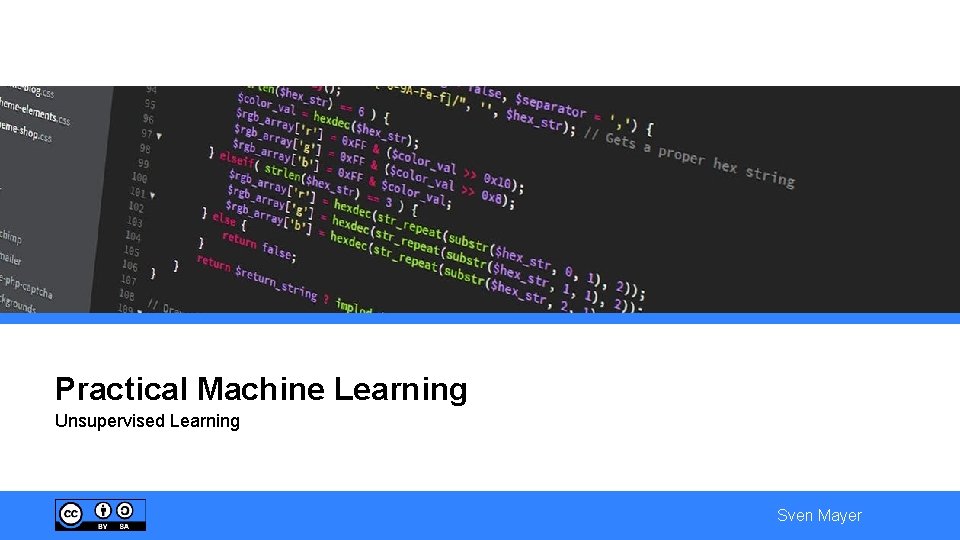
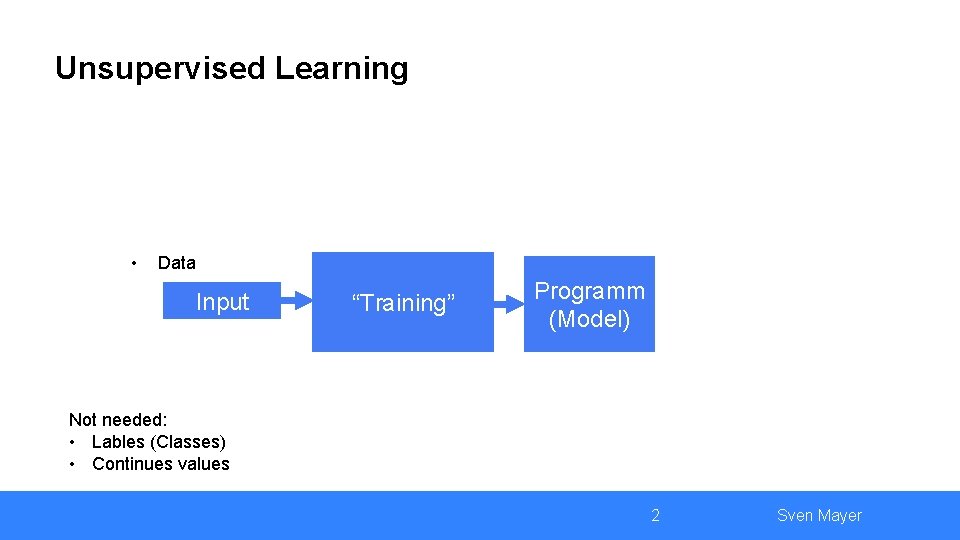
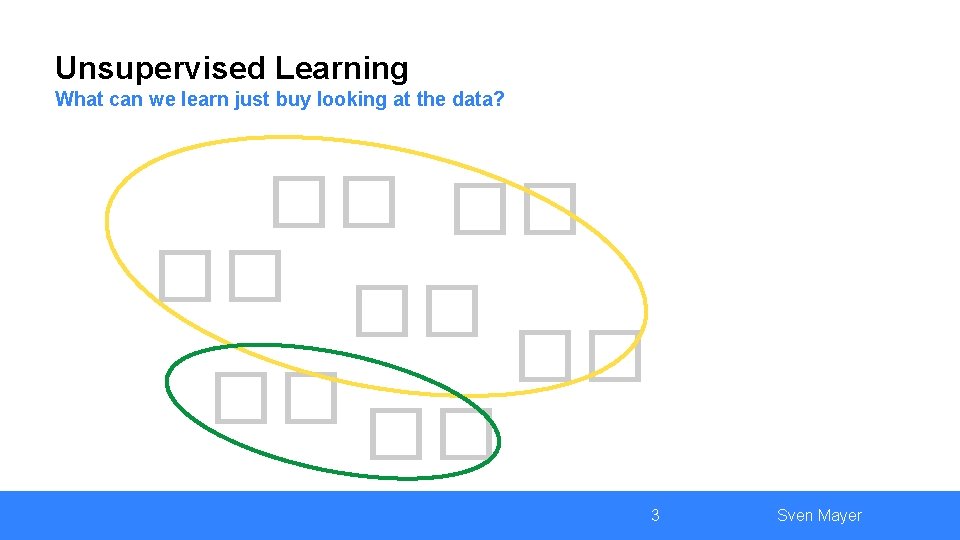
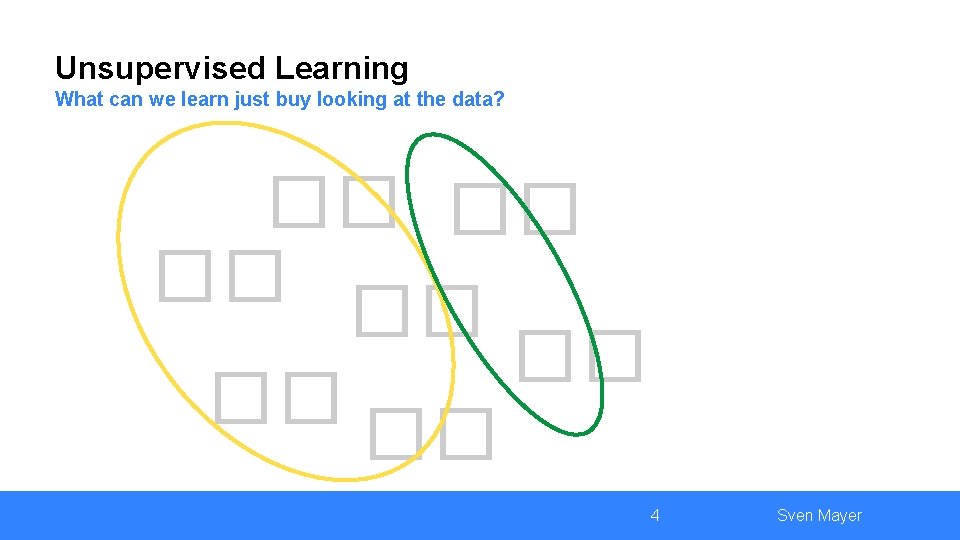
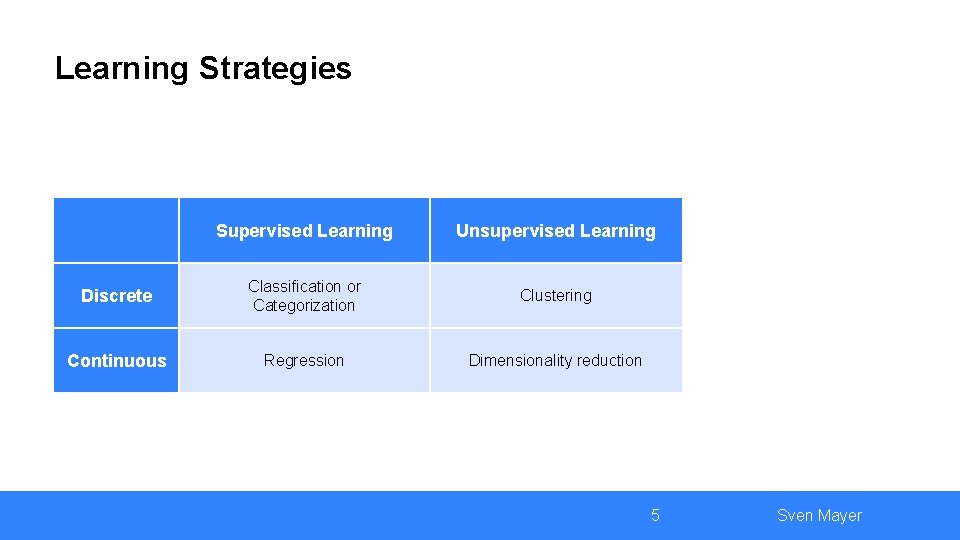
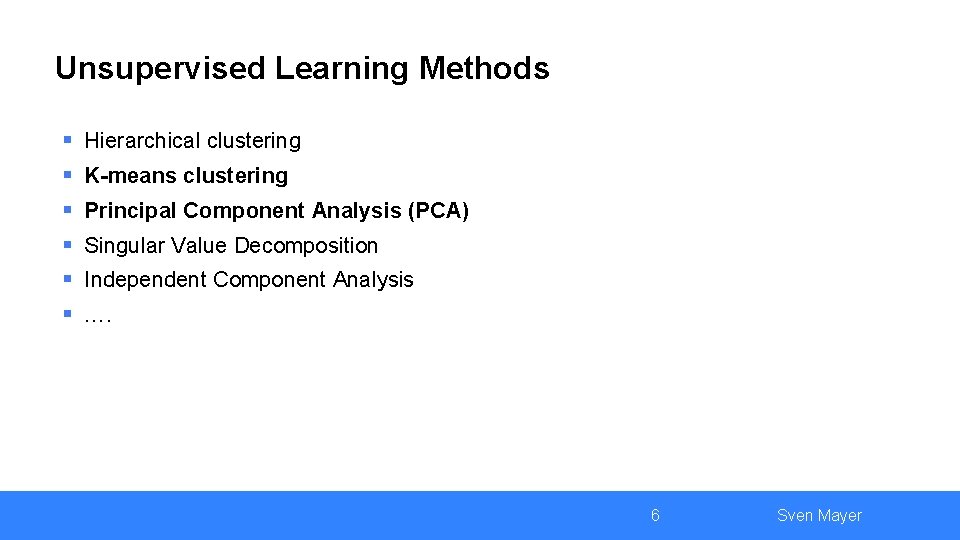
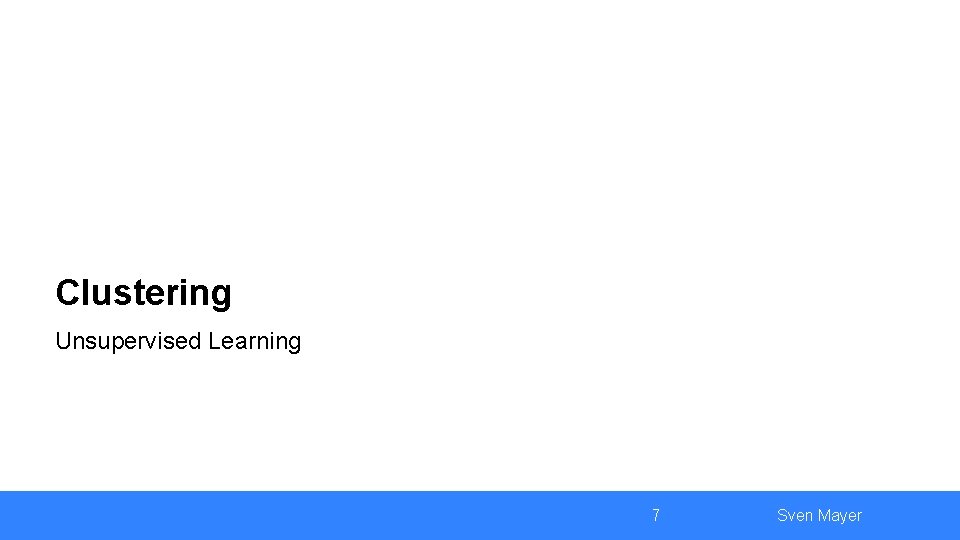
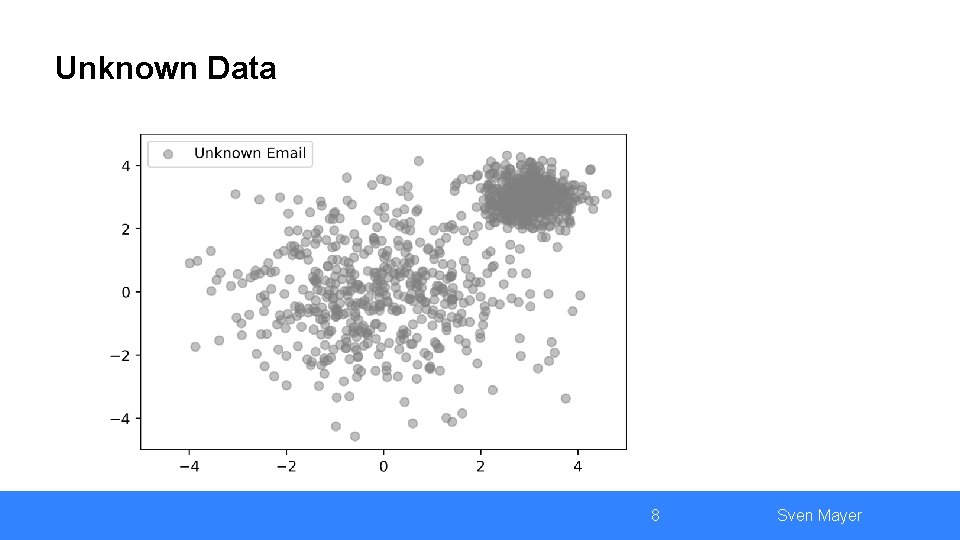
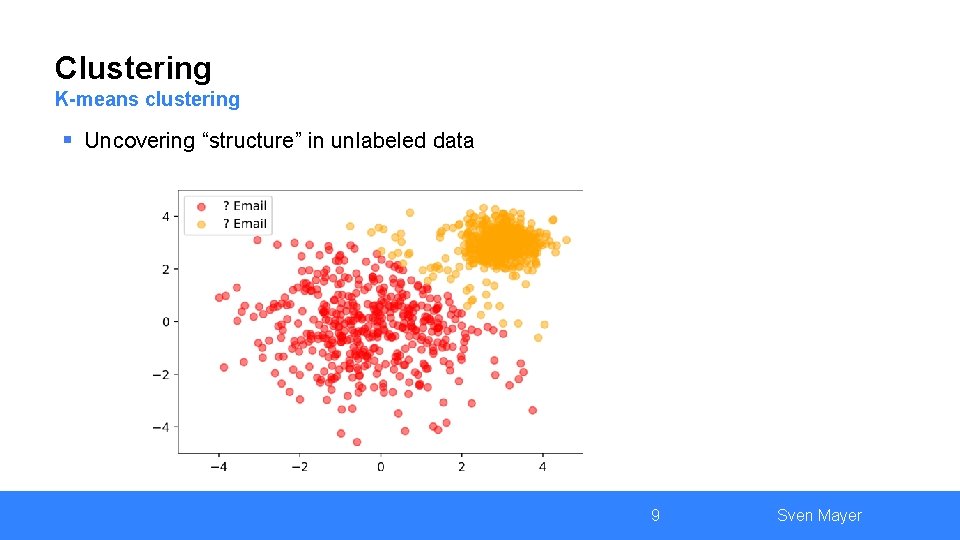
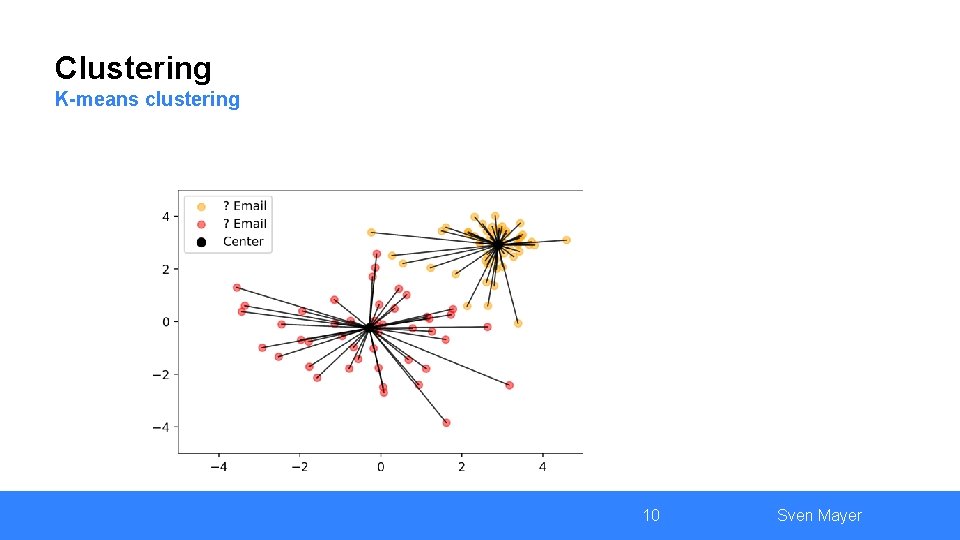
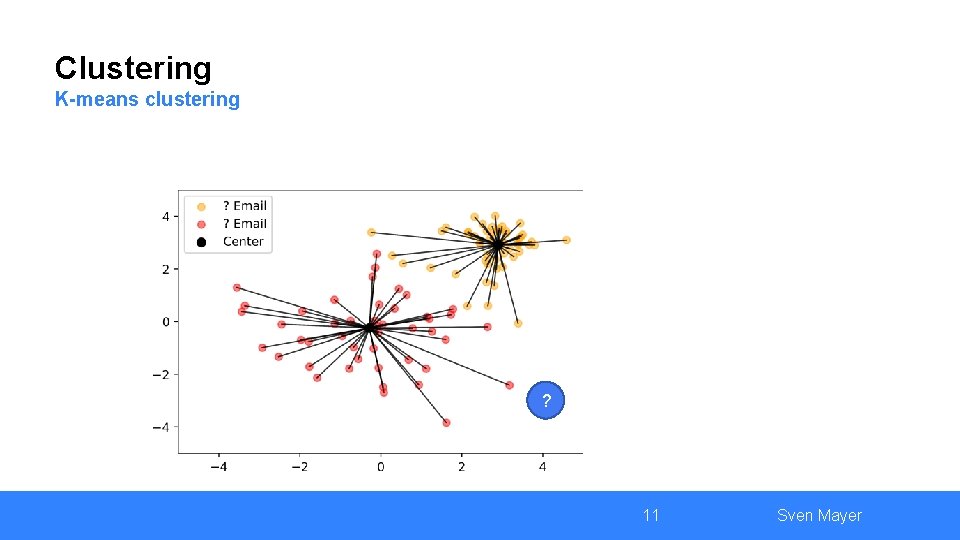
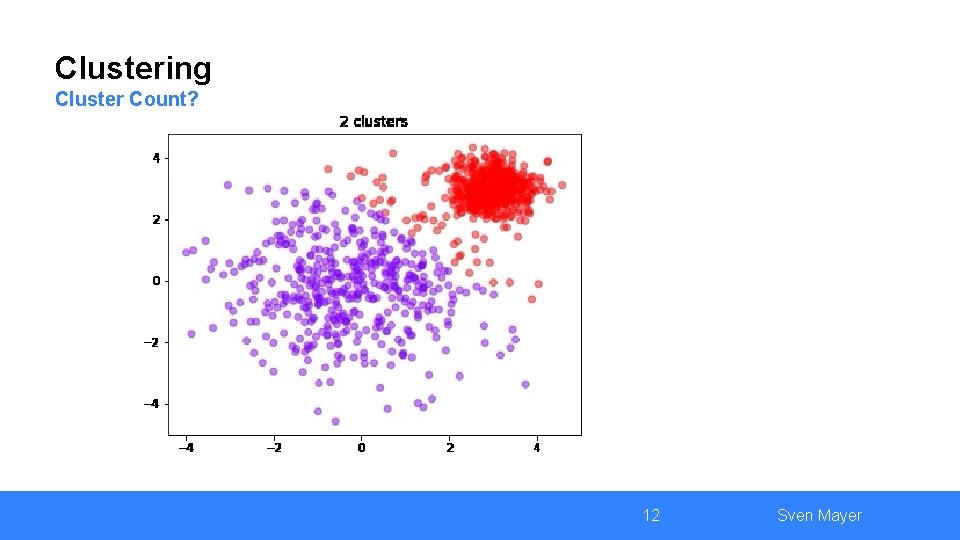
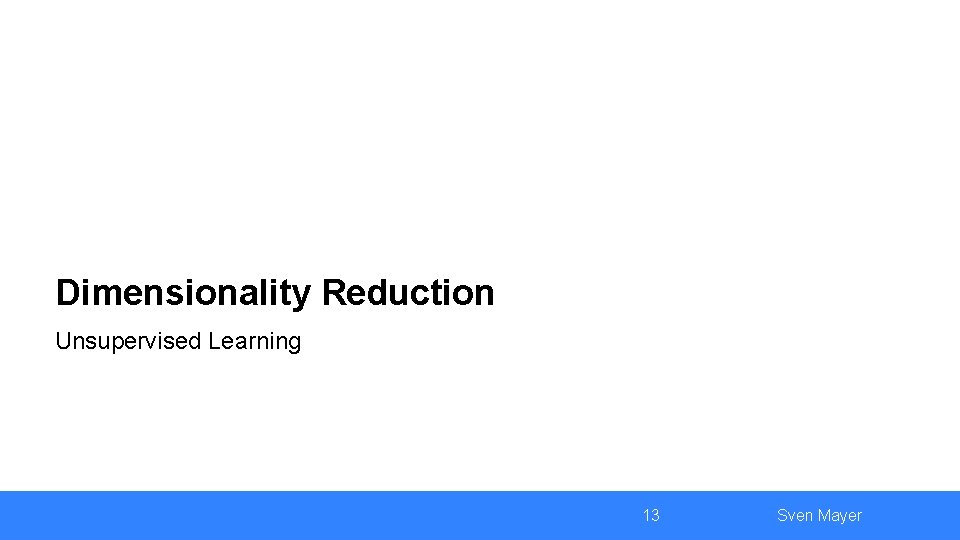
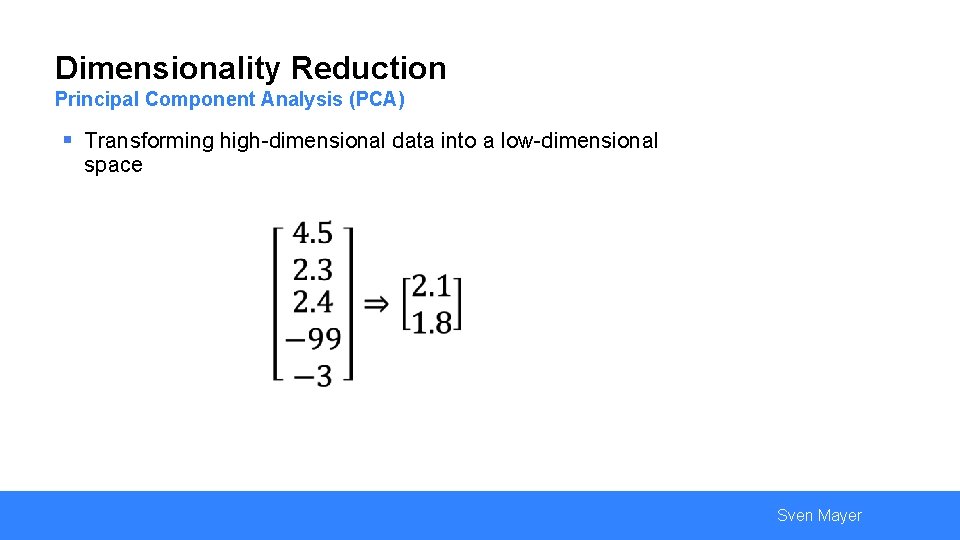
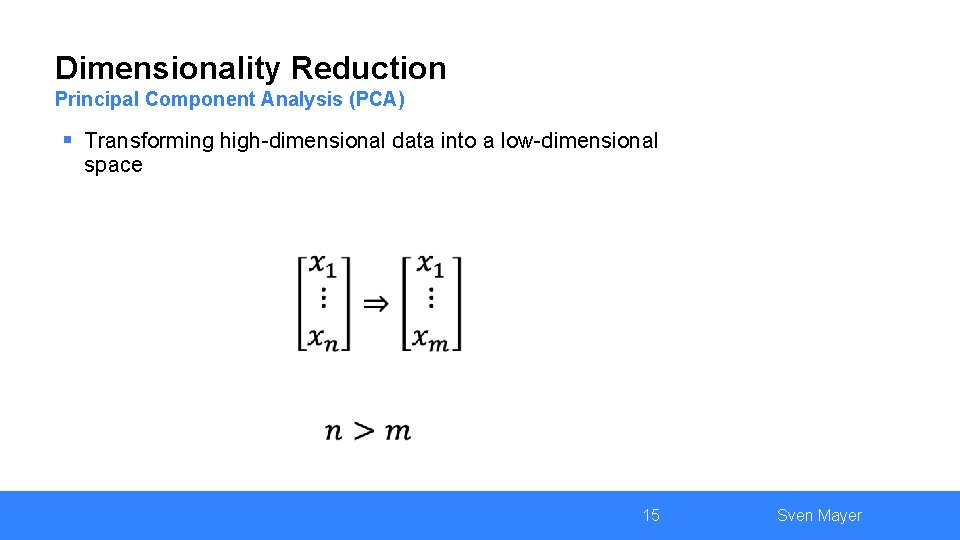
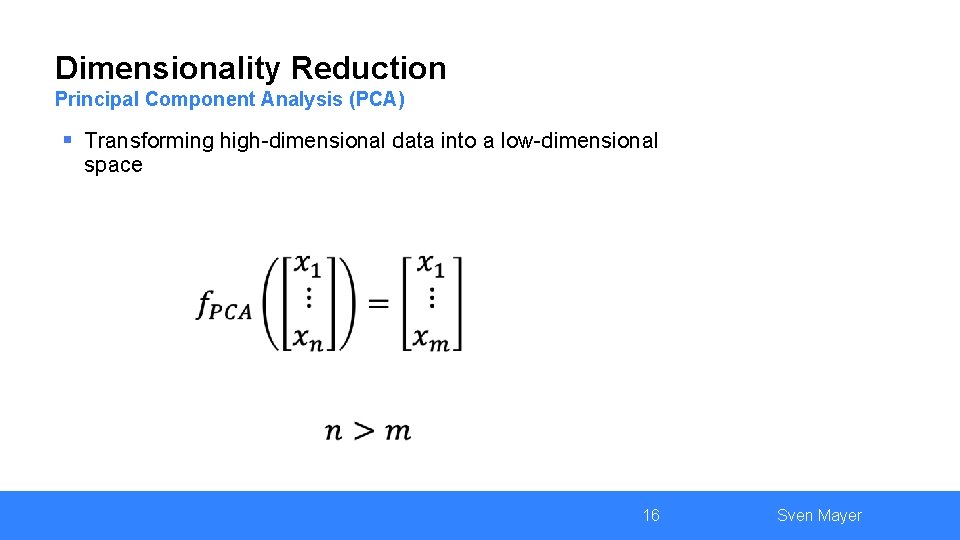
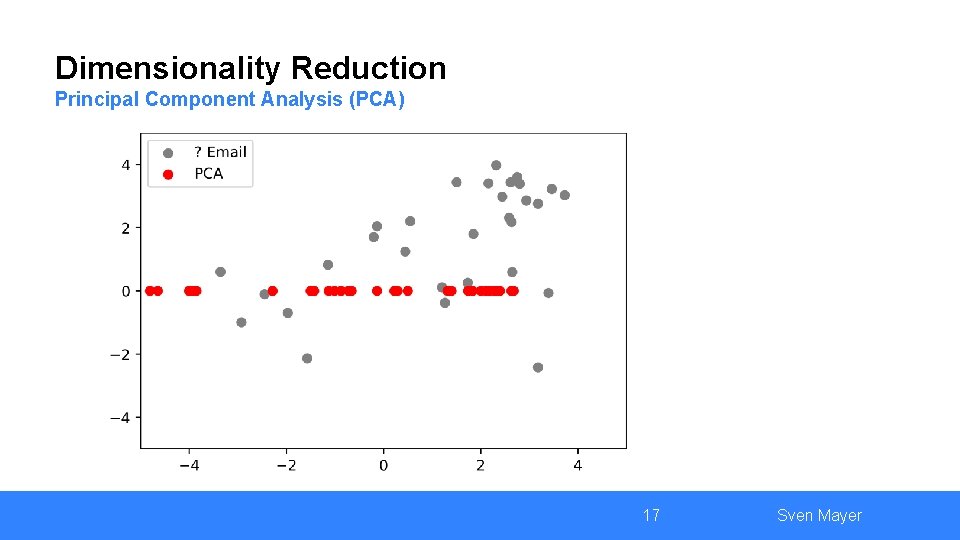
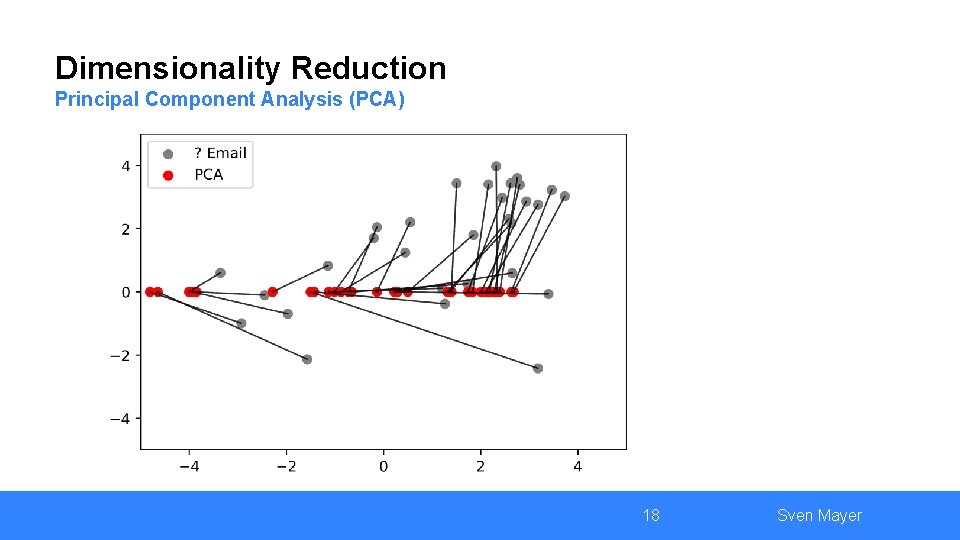
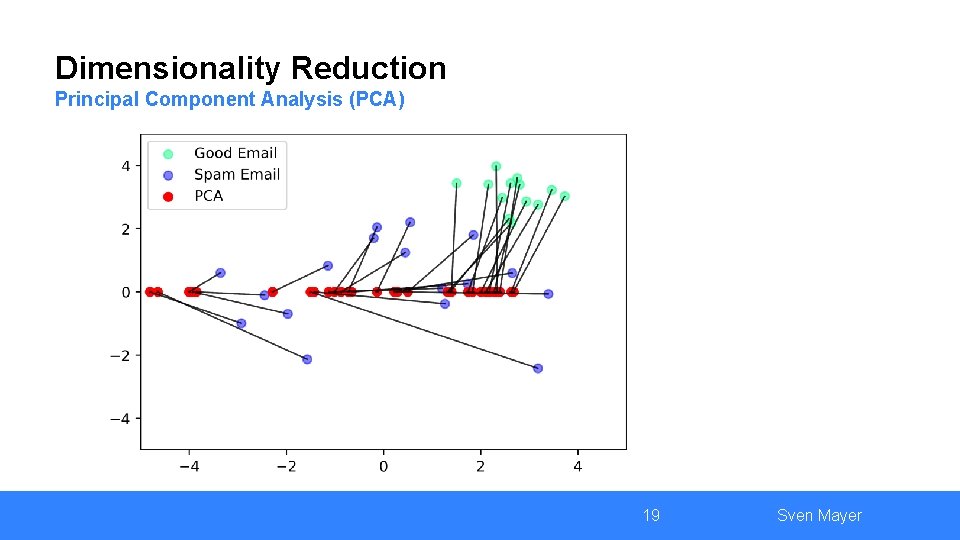
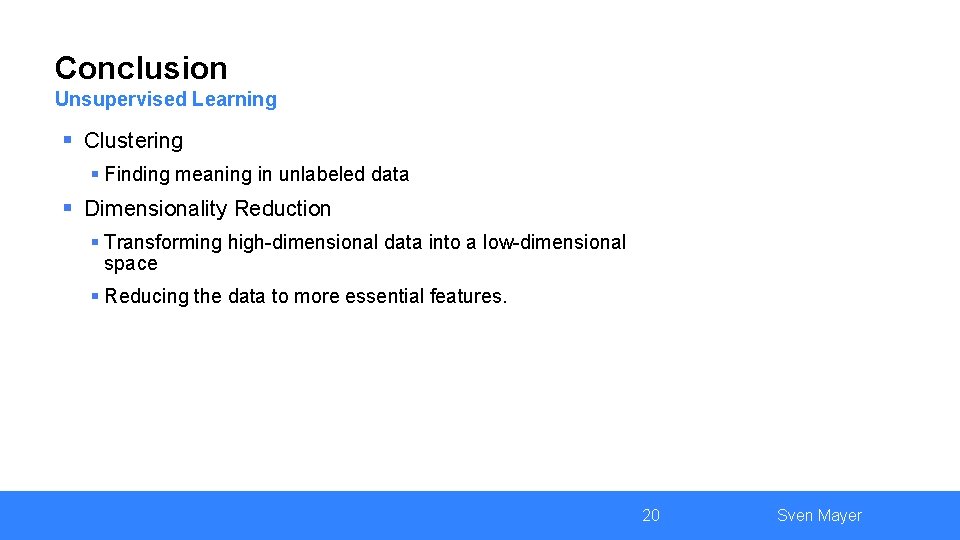
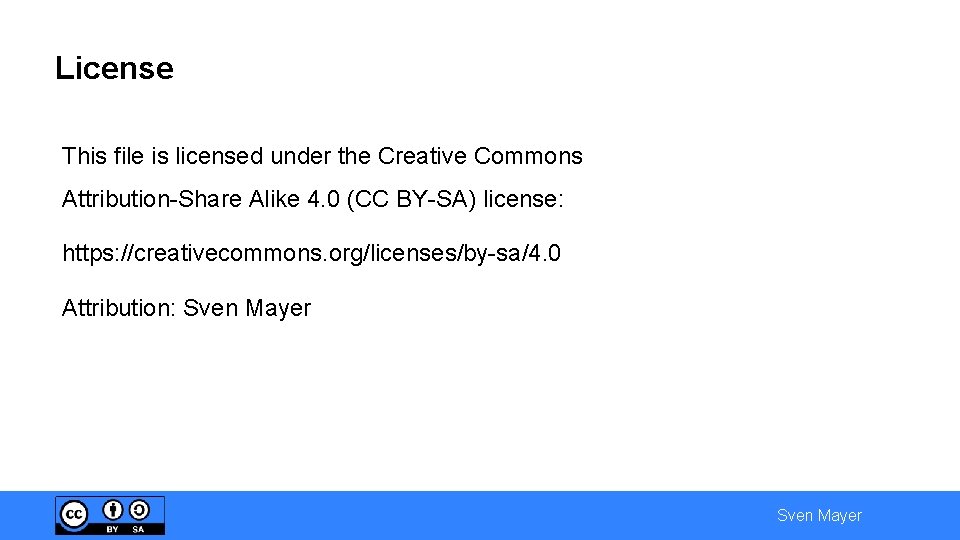
- Slides: 21
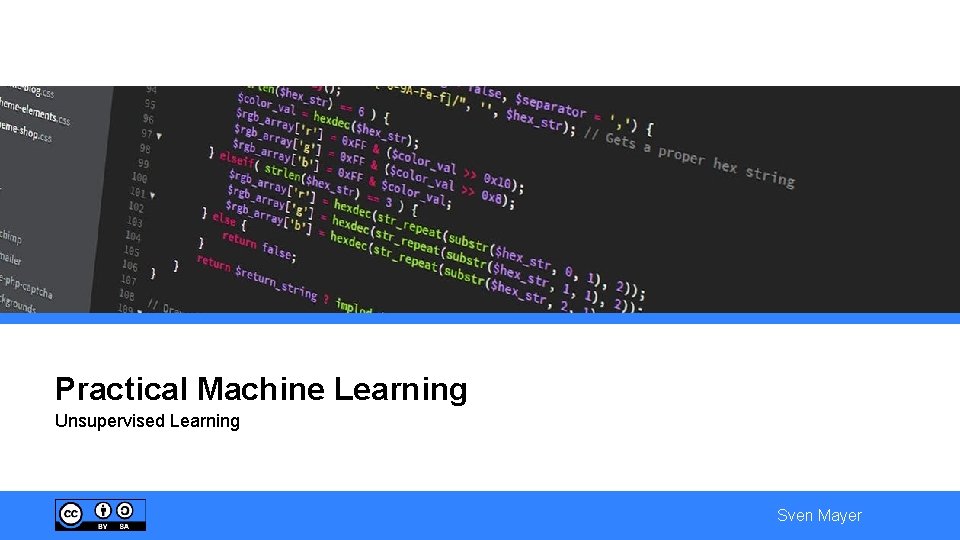
Practical Machine Learning Unsupervised Learning Sven Mayer
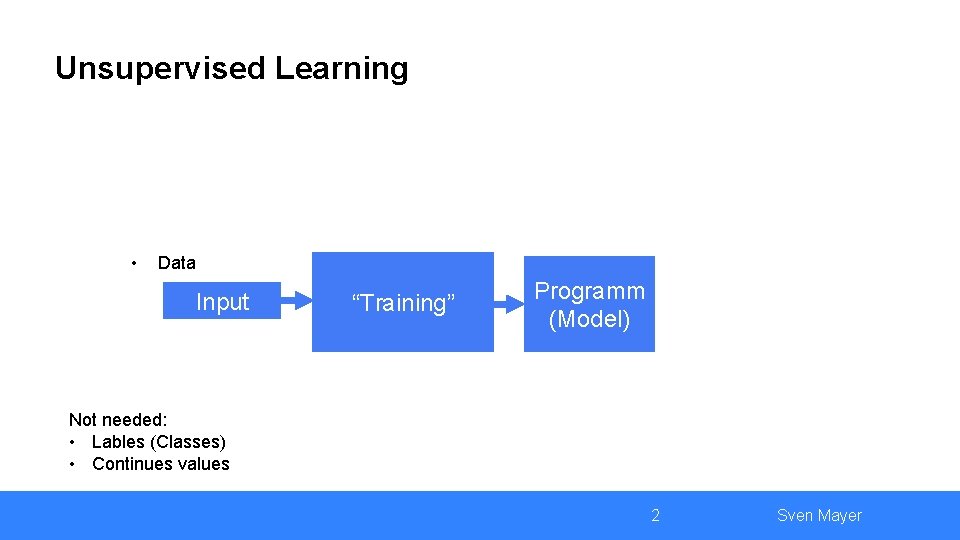
Unsupervised Learning • Data Input “Training” Programm (Model) Not needed: • Lables (Classes) • Continues values 2 Sven Mayer
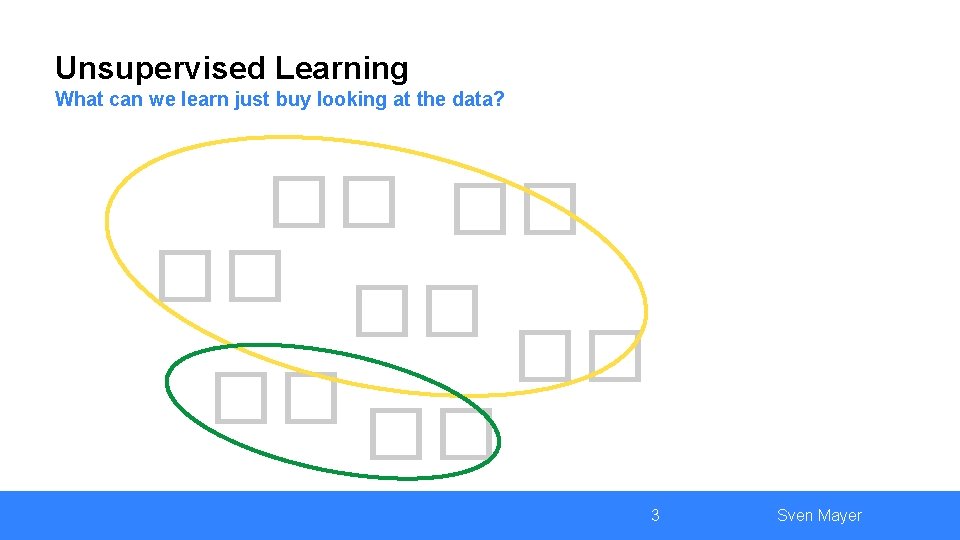
Unsupervised Learning What can we learn just buy looking at the data? �� �� 3 Sven Mayer
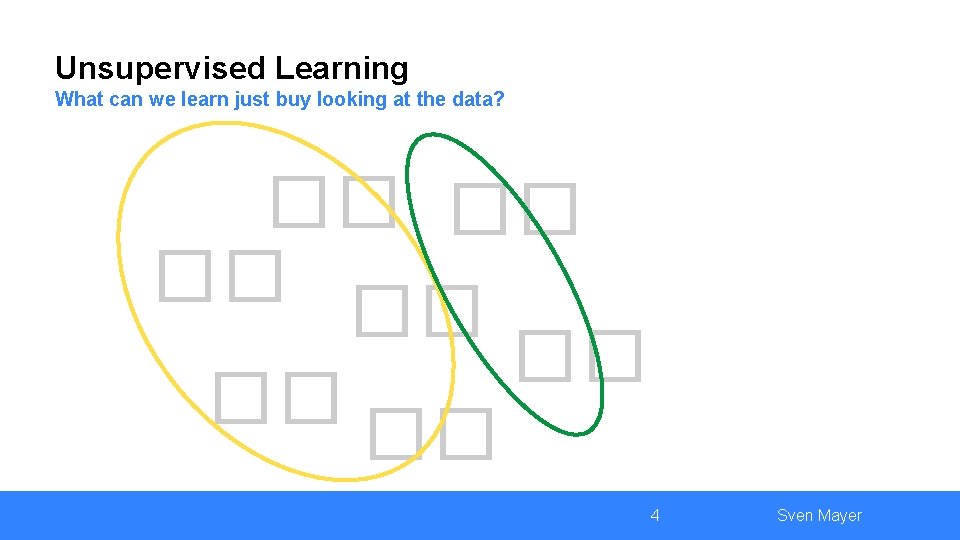
Unsupervised Learning What can we learn just buy looking at the data? �� �� 4 Sven Mayer
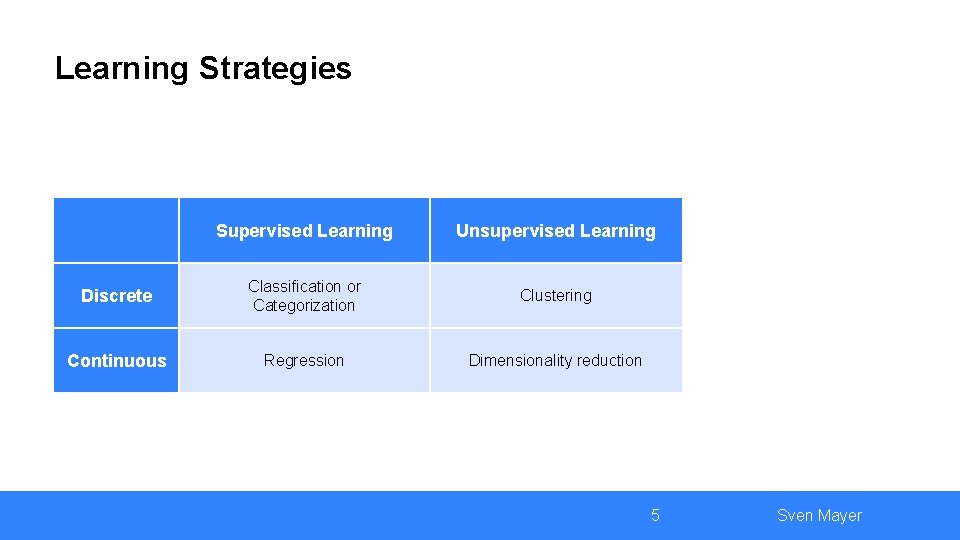
Learning Strategies Supervised Learning Unsupervised Learning Discrete Classification or Categorization Clustering Continuous Regression Dimensionality reduction 5 Sven Mayer
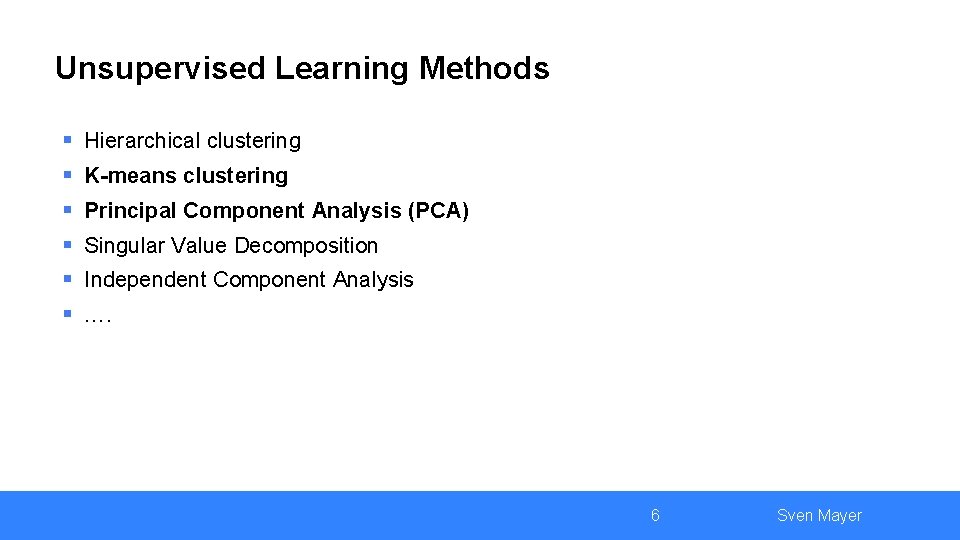
Unsupervised Learning Methods § § § Hierarchical clustering K-means clustering Principal Component Analysis (PCA) Singular Value Decomposition Independent Component Analysis …. 6 Sven Mayer
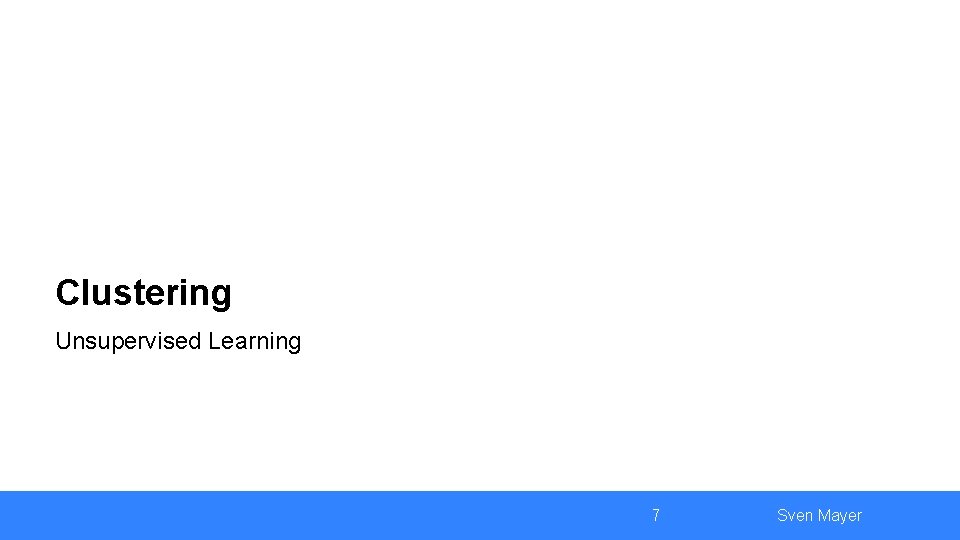
Clustering Unsupervised Learning 7 Sven Mayer
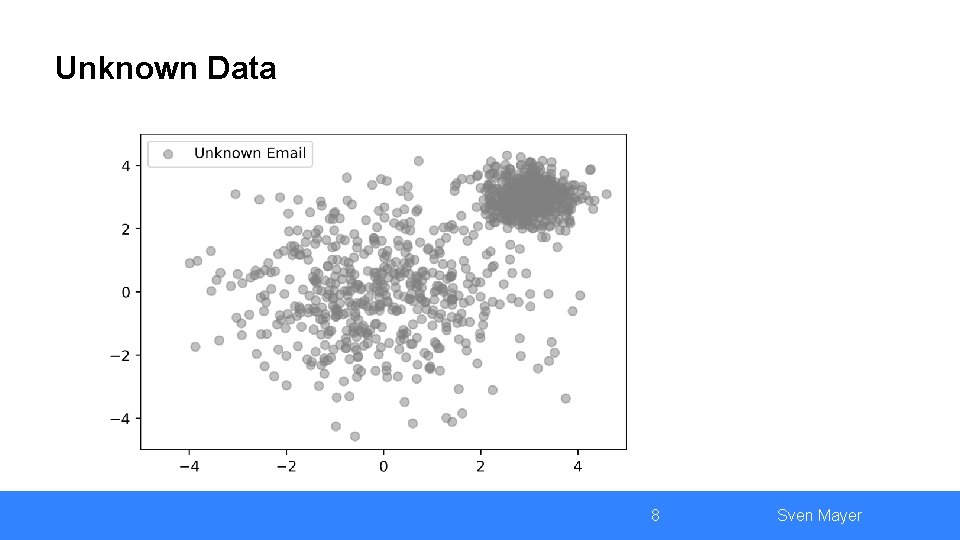
Unknown Data 8 Sven Mayer
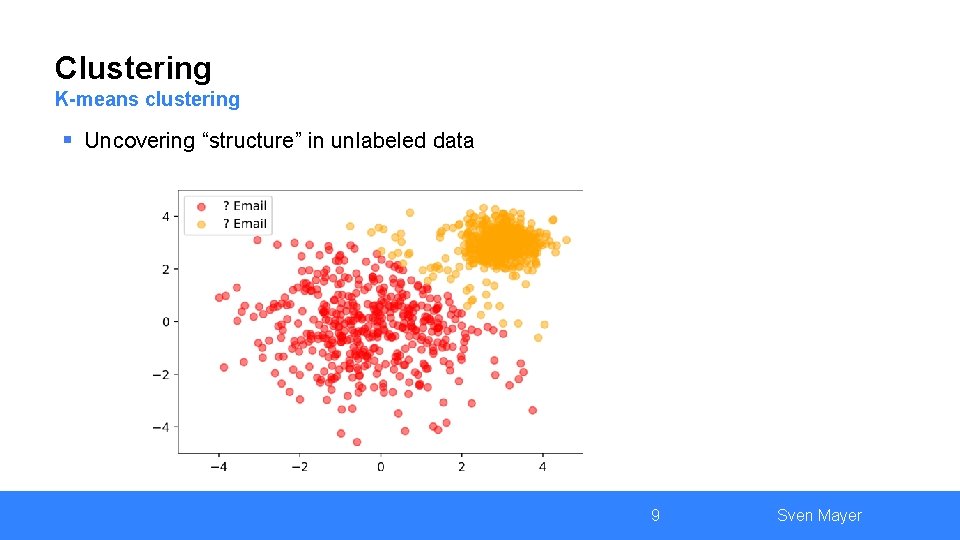
Clustering K-means clustering § Uncovering “structure” in unlabeled data 9 Sven Mayer
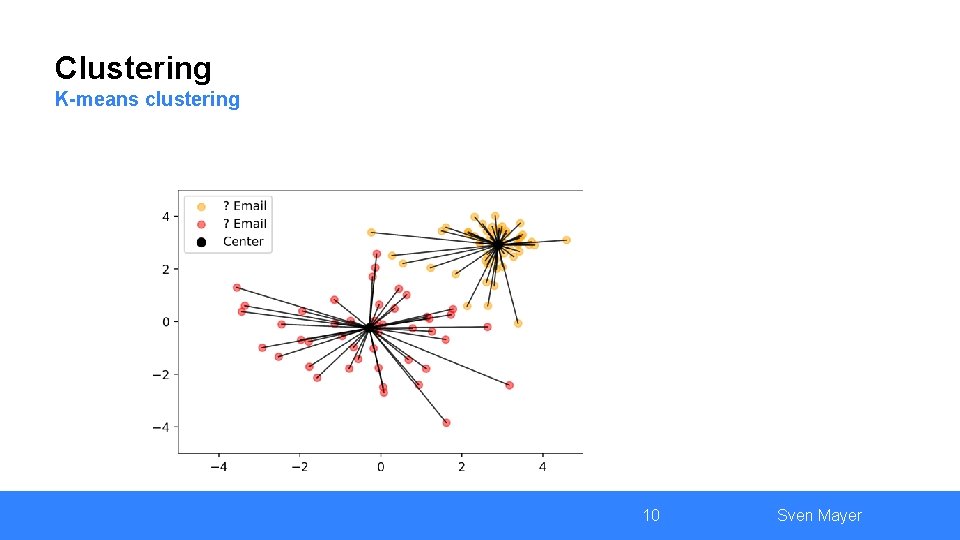
Clustering K-means clustering 10 Sven Mayer
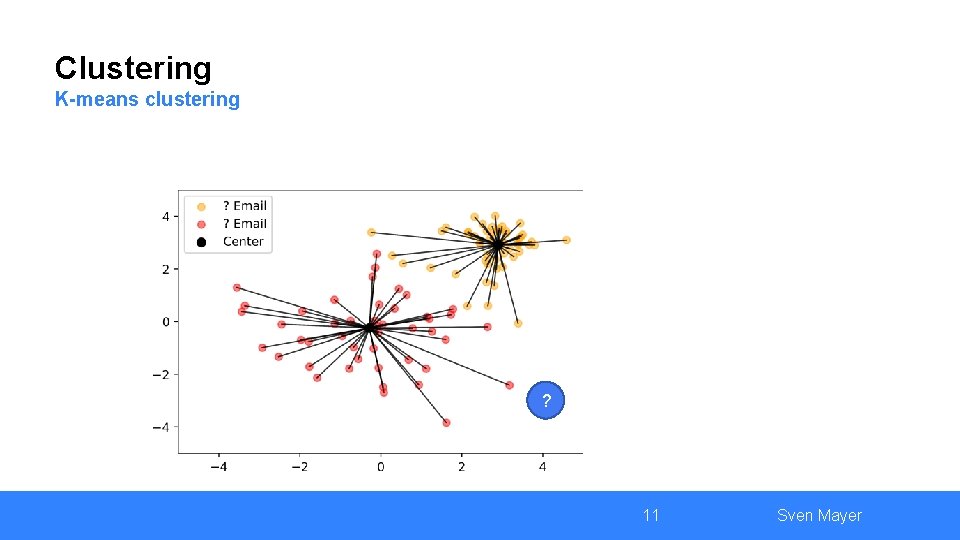
Clustering K-means clustering ? 11 Sven Mayer
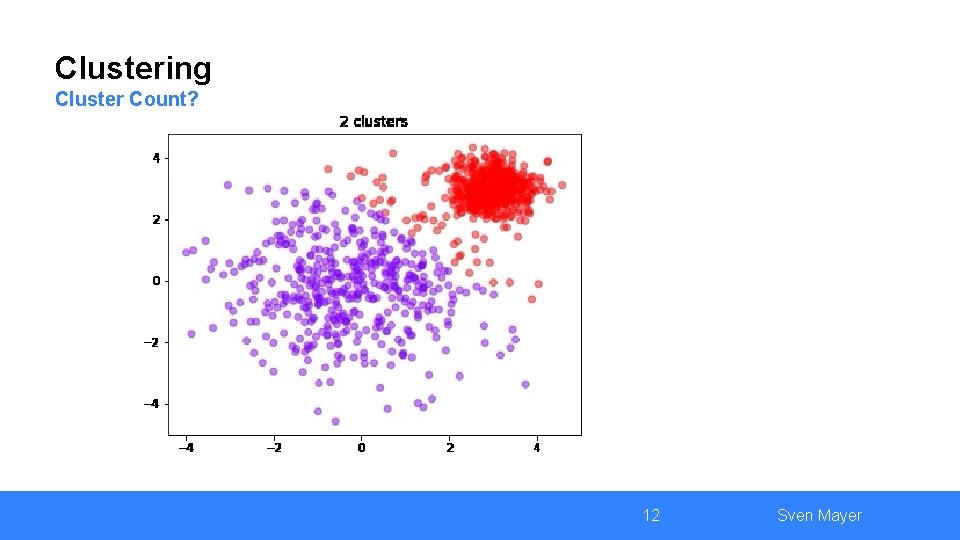
Clustering Cluster Count? 12 Sven Mayer
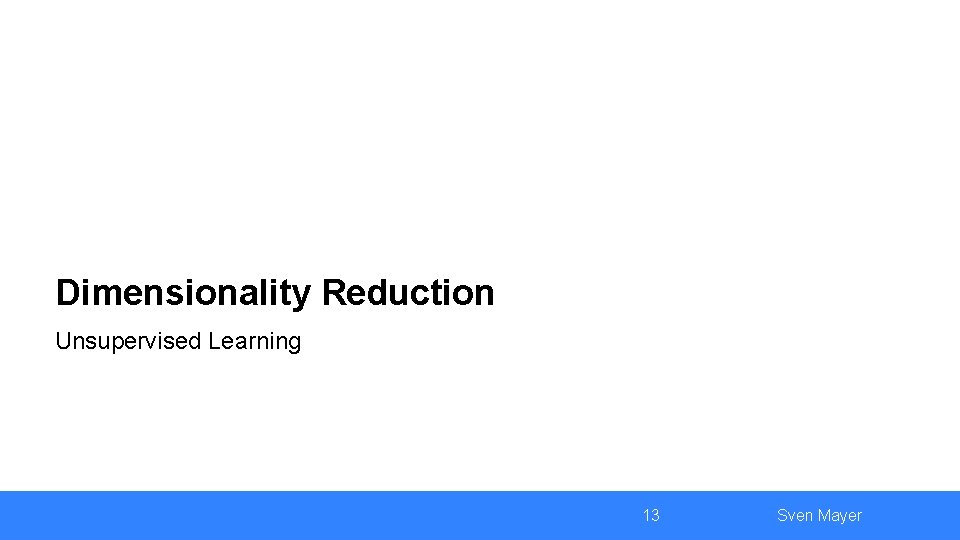
Dimensionality Reduction Unsupervised Learning 13 Sven Mayer
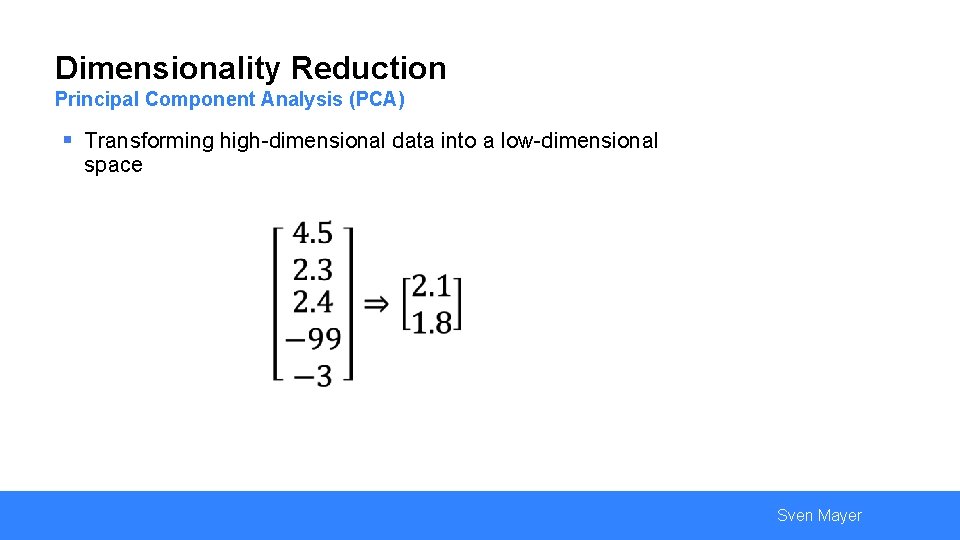
Dimensionality Reduction Principal Component Analysis (PCA) § Transforming high-dimensional data into a low-dimensional space Sven Mayer
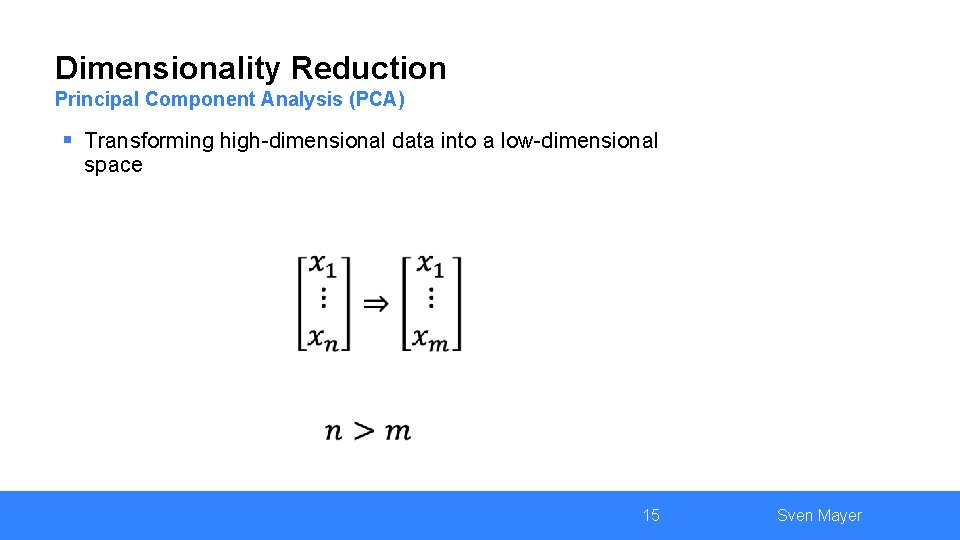
Dimensionality Reduction Principal Component Analysis (PCA) § Transforming high-dimensional data into a low-dimensional space 15 Sven Mayer
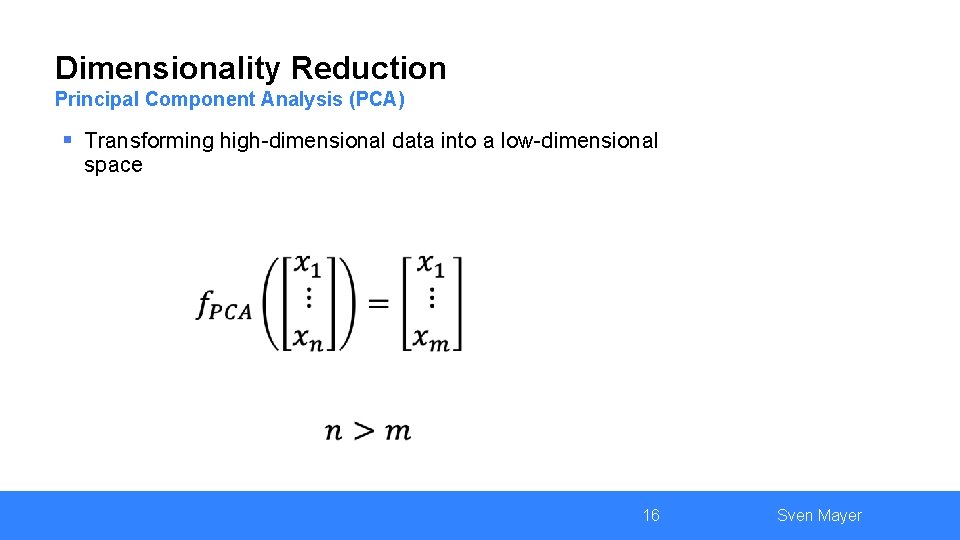
Dimensionality Reduction Principal Component Analysis (PCA) § Transforming high-dimensional data into a low-dimensional space 16 Sven Mayer
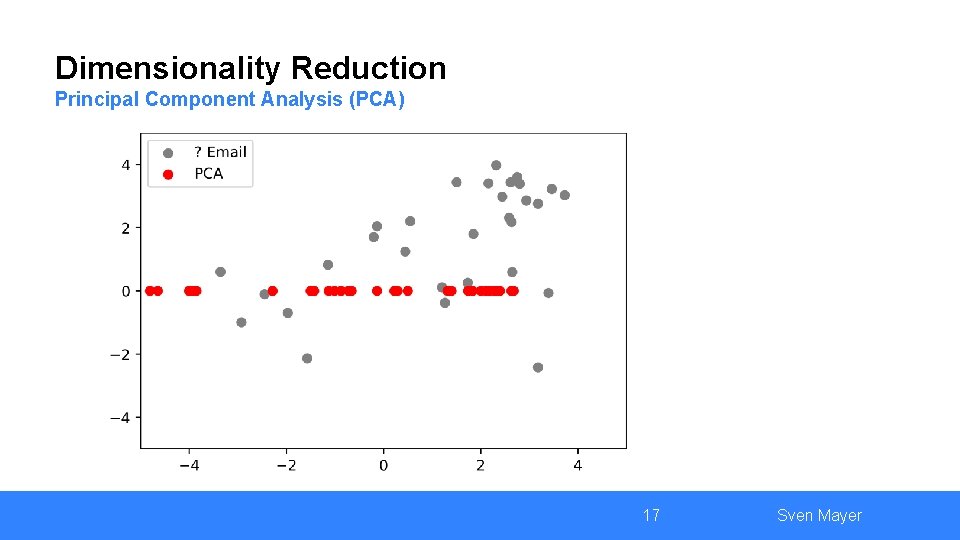
Dimensionality Reduction Principal Component Analysis (PCA) 17 Sven Mayer
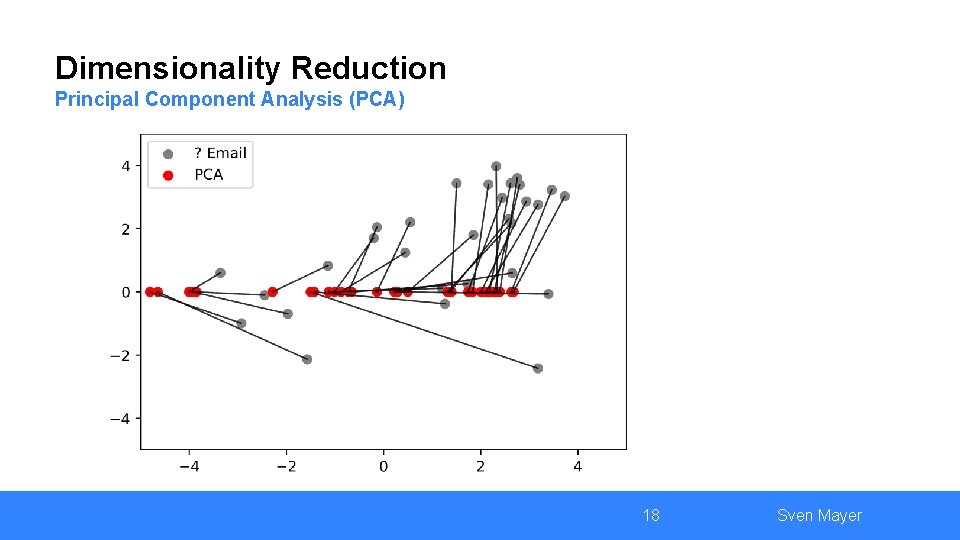
Dimensionality Reduction Principal Component Analysis (PCA) 18 Sven Mayer
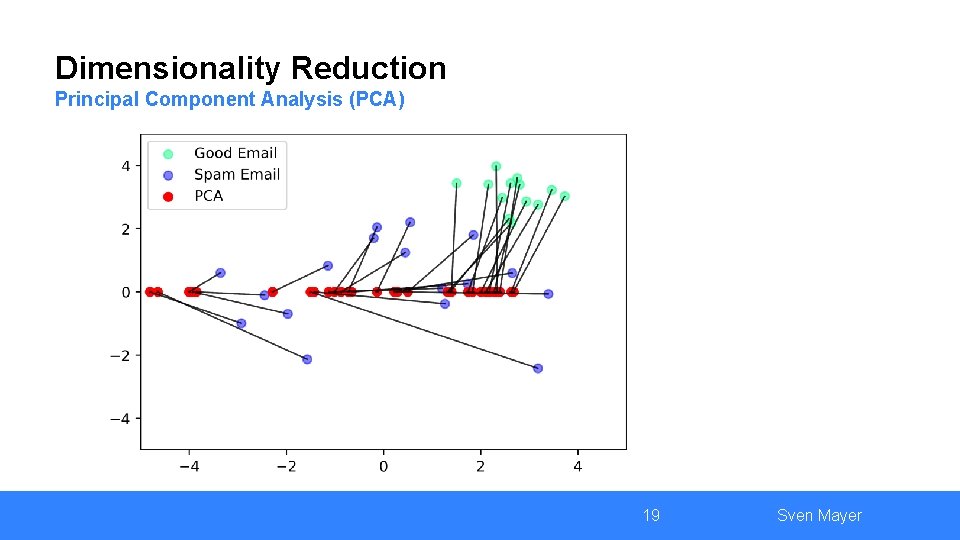
Dimensionality Reduction Principal Component Analysis (PCA) 19 Sven Mayer
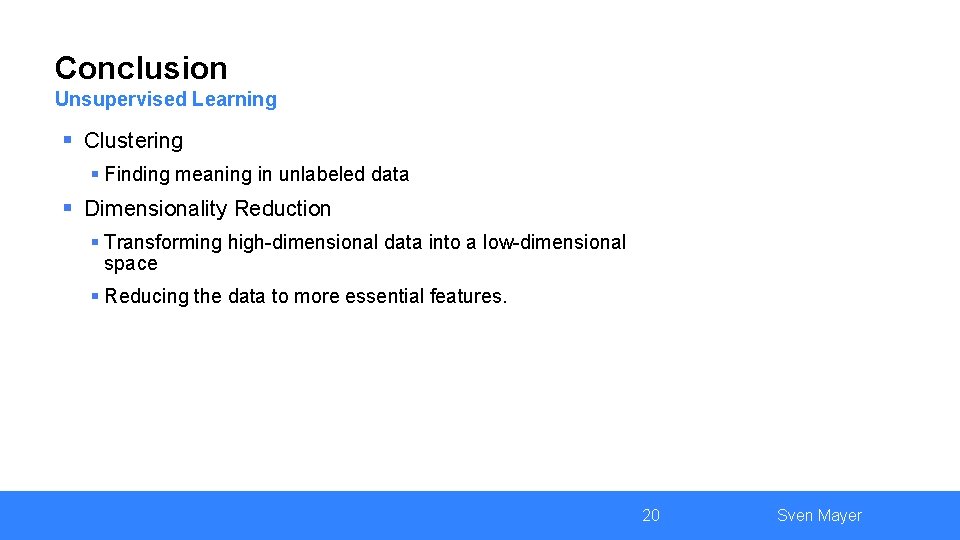
Conclusion Unsupervised Learning § Clustering § Finding meaning in unlabeled data § Dimensionality Reduction § Transforming high-dimensional data into a low-dimensional space § Reducing the data to more essential features. 20 Sven Mayer
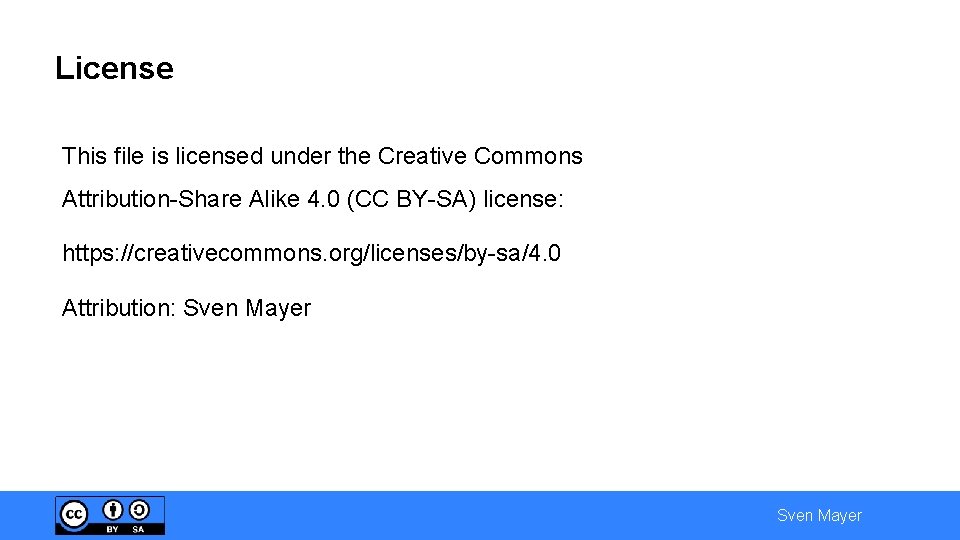
License This file is licensed under the Creative Commons Attribution-Share Alike 4. 0 (CC BY-SA) license: https: //creativecommons. org/licenses/by-sa/4. 0 Attribution: Sven Mayer
"deep reinforcement learning"
Pendekatan unsupervised learning adalah
Practical machine learning quiz 4
Practical machine learning quiz 2
Cognitive theory of multimedia learning (mayer)
Unsupervised learning in data mining
Transductive learning for unsupervised text style transfer
Autoencoders
Ann unsupervised learning
Is pca unsupervised learning
Unsupervised learning
What is unsupervised learning algorithm
Supervised vs unsupervised learning
Contractive autoencoder
Supervised and unsupervised learning
Tincion carmin
John mayer ciara
Relazione di meyer
Mayer 2001
Columbia pictures and paramount pictures
Ilse mayer
Gertrud meyer-denkmann