On Infinity Continuity and TimeVarying Graphs Nicola Santoro
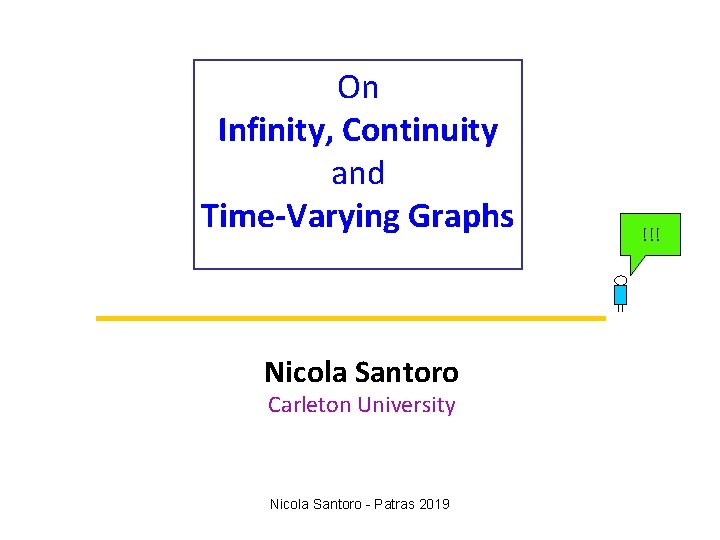
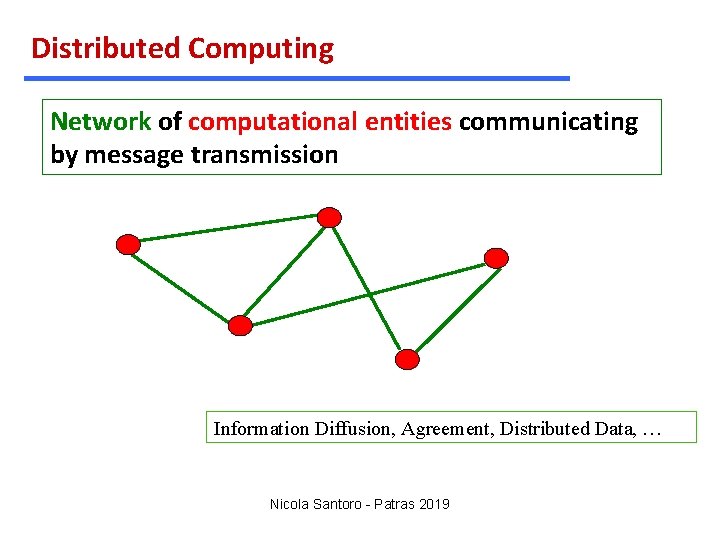
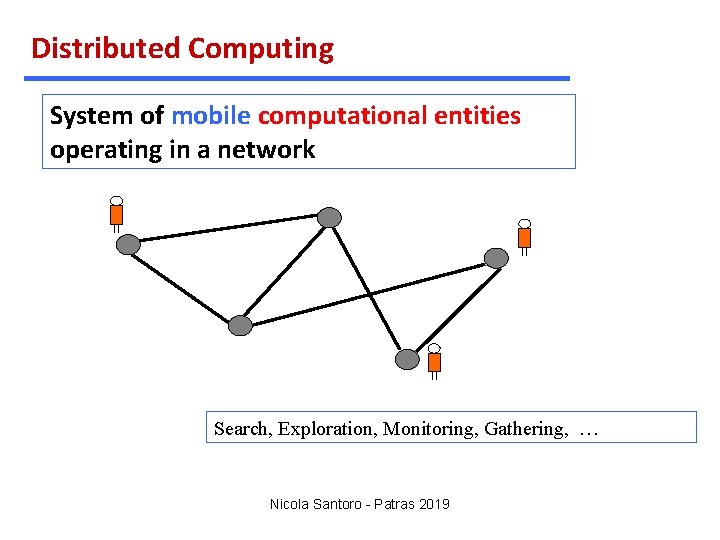
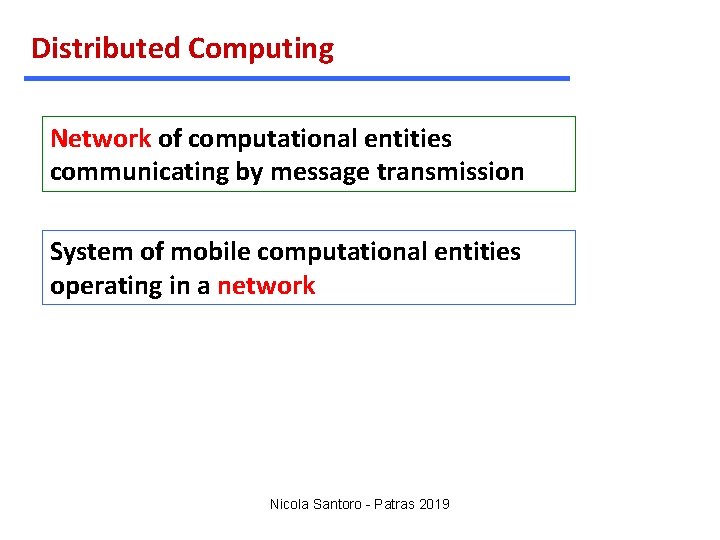
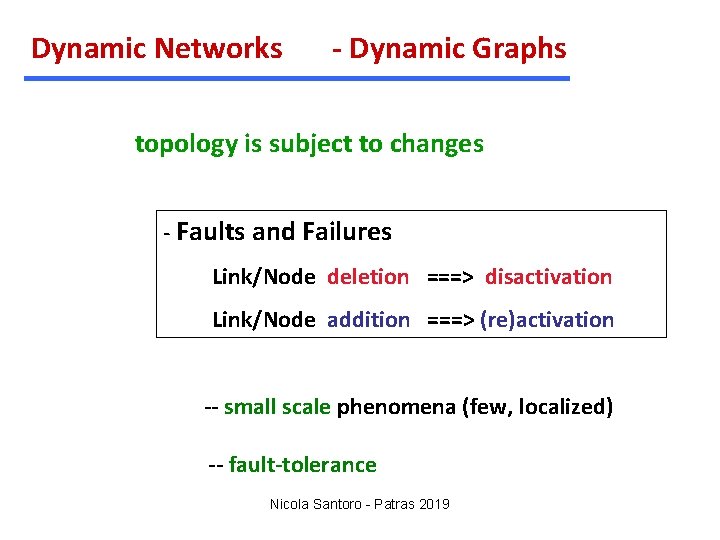
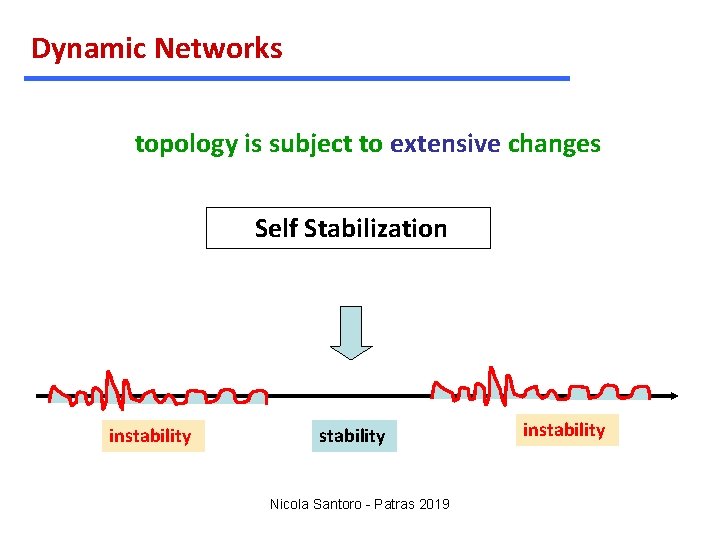
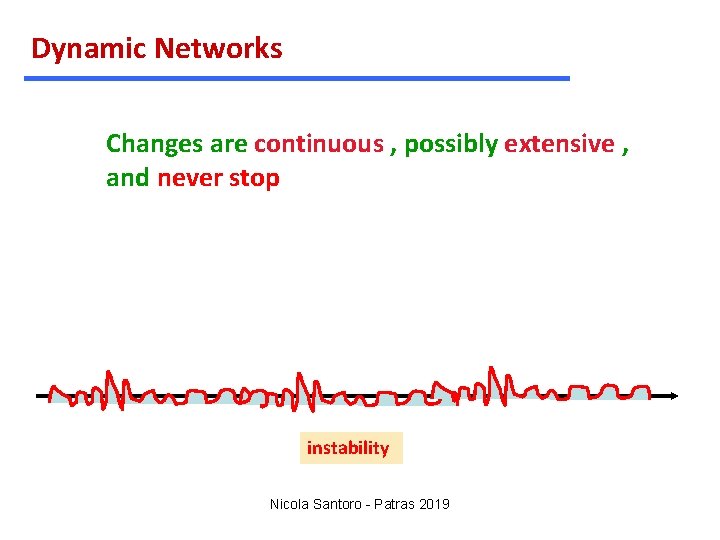
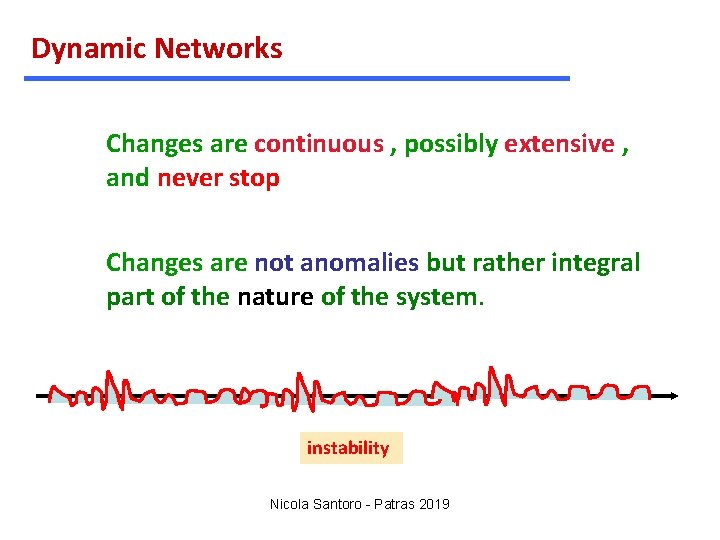
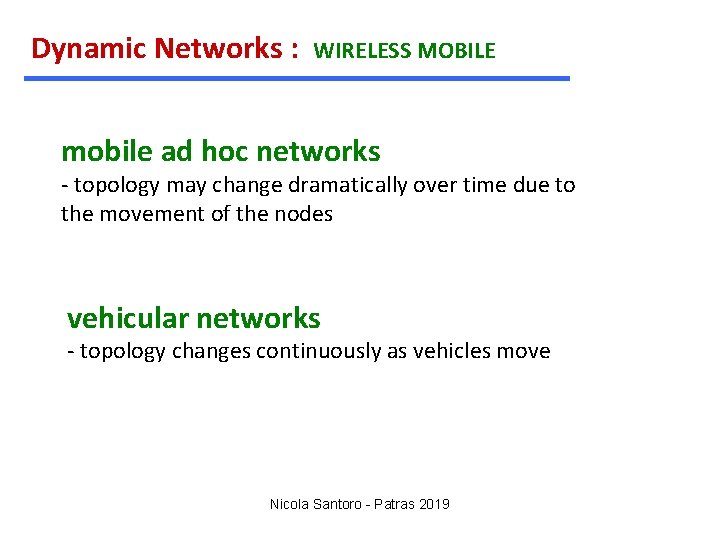
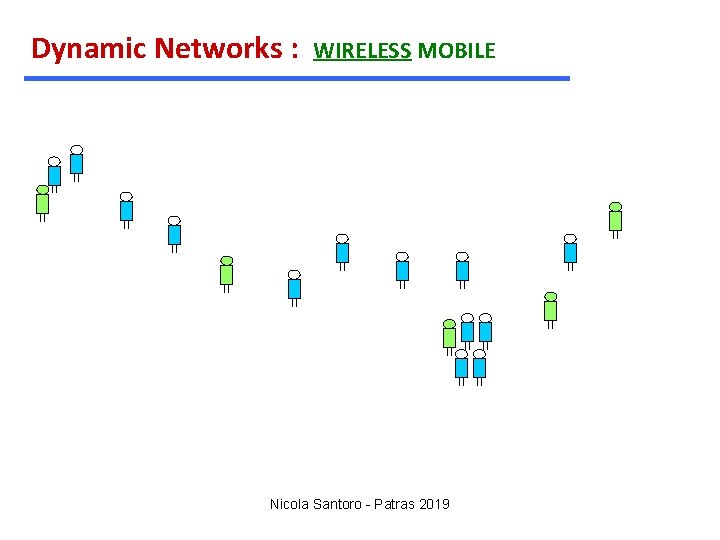
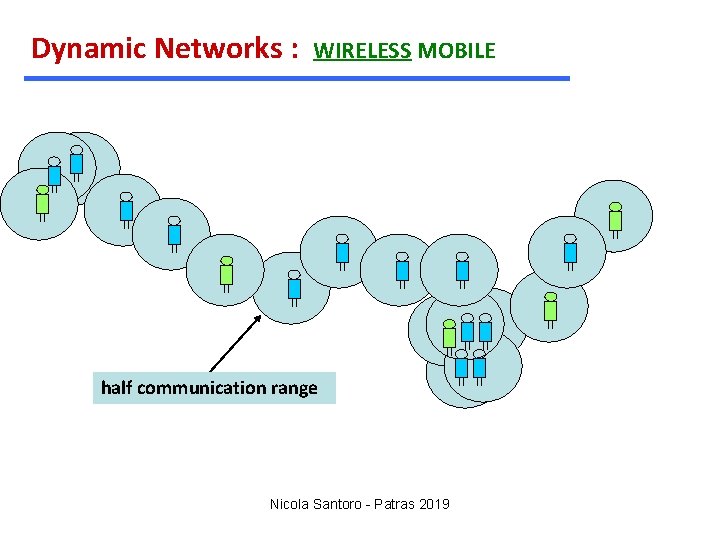
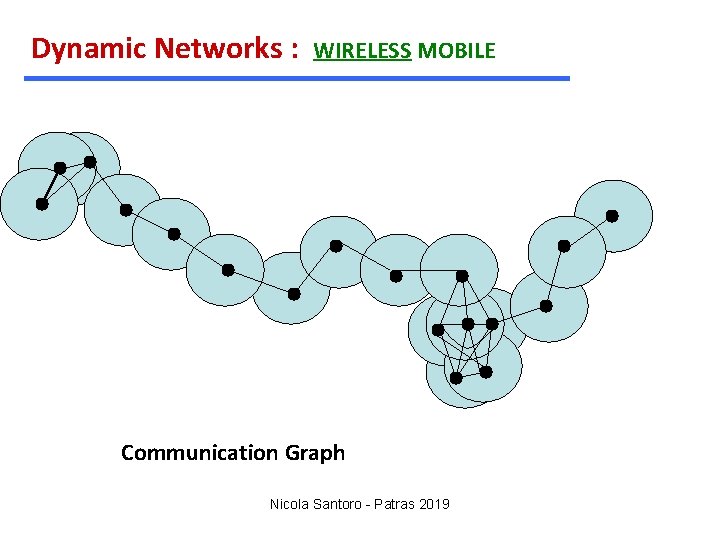
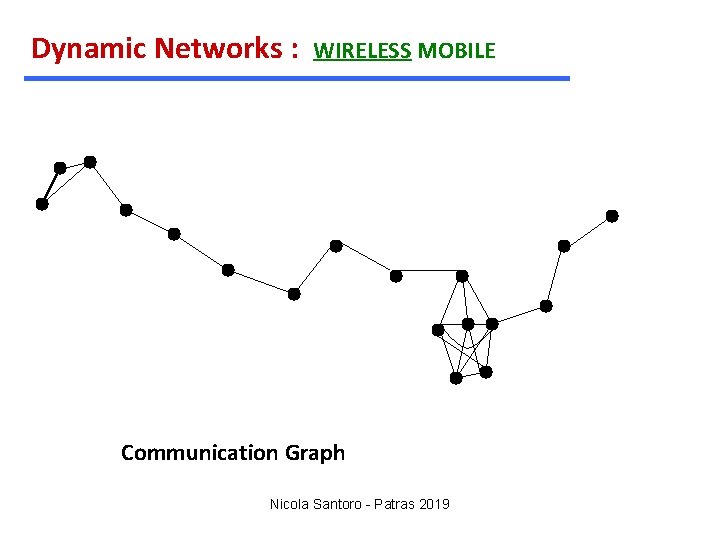
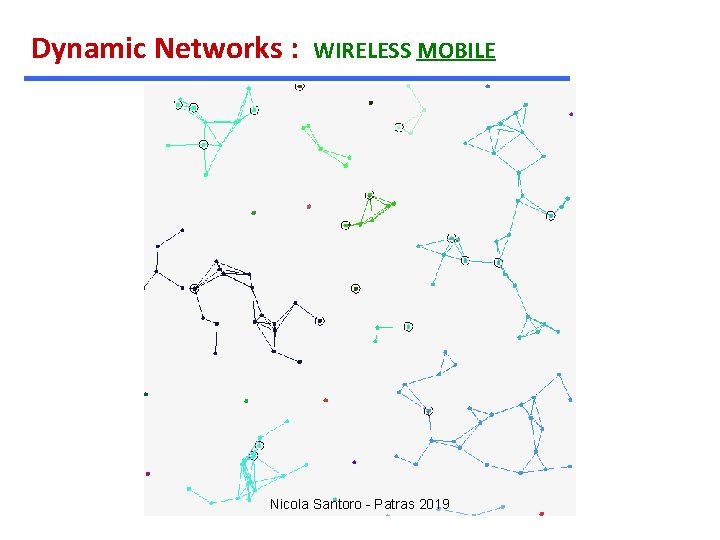
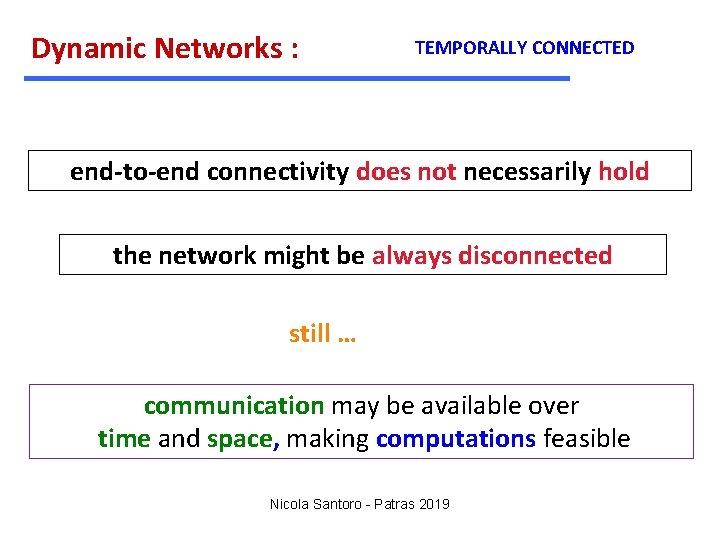
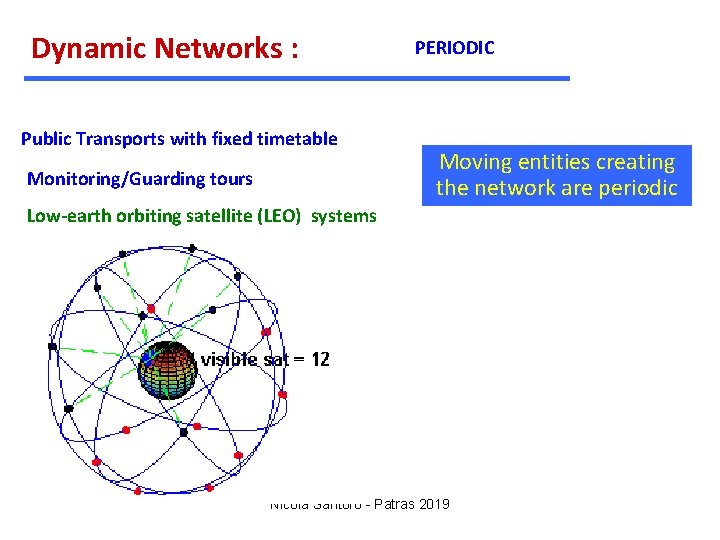
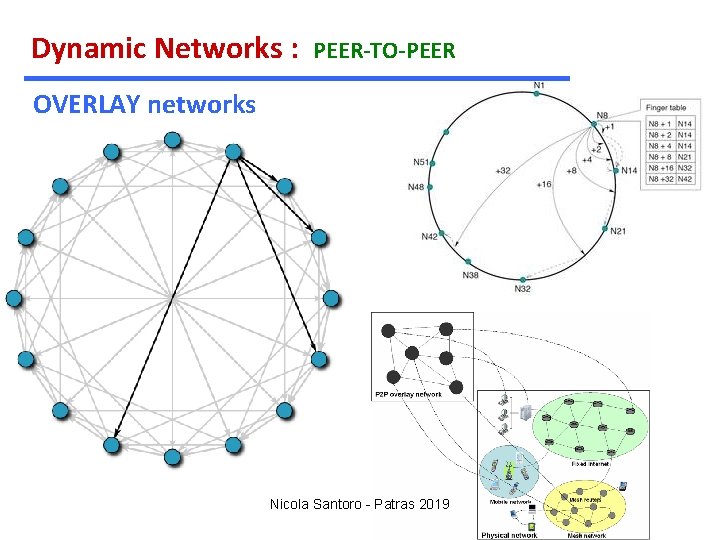
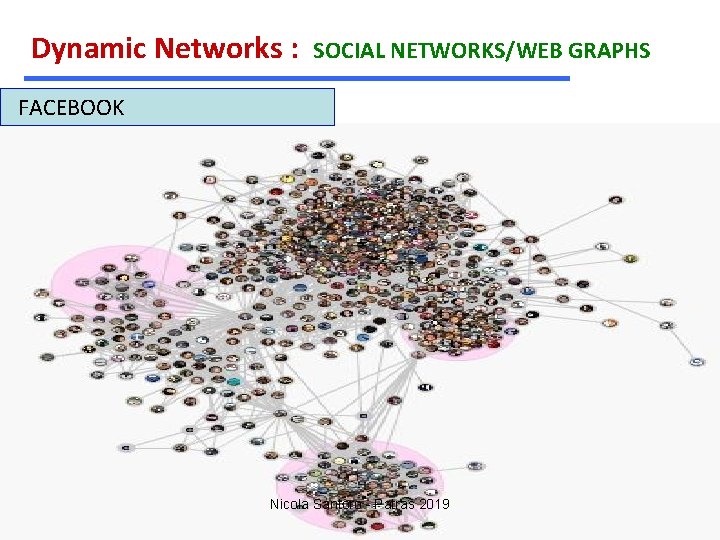
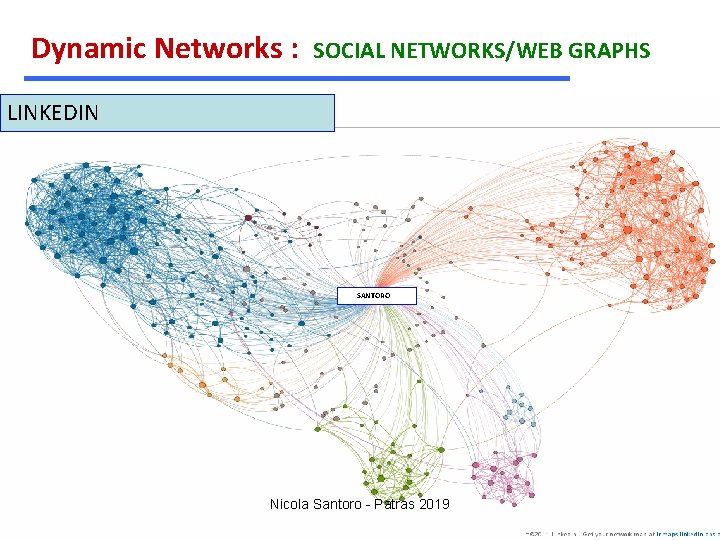
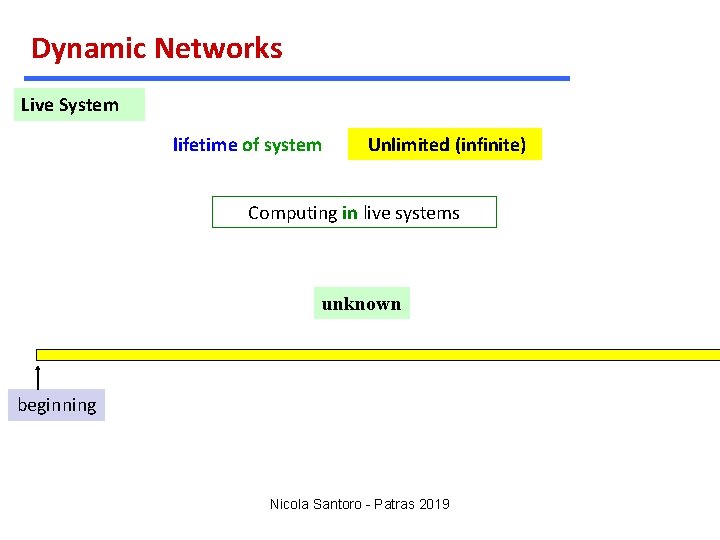
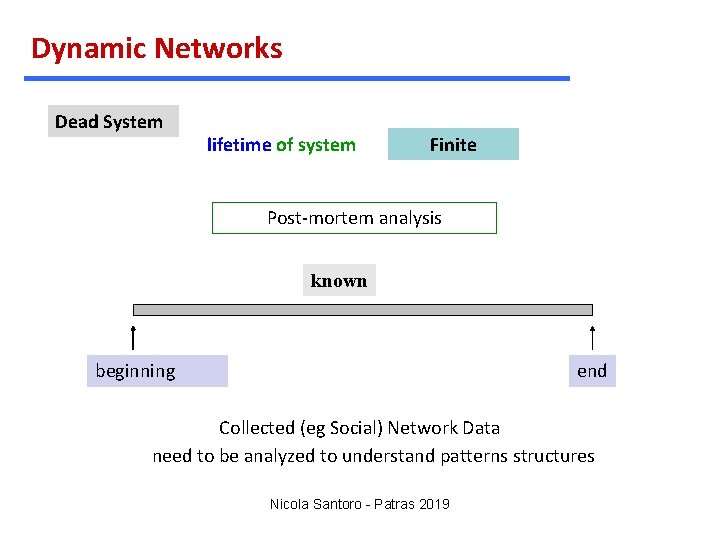
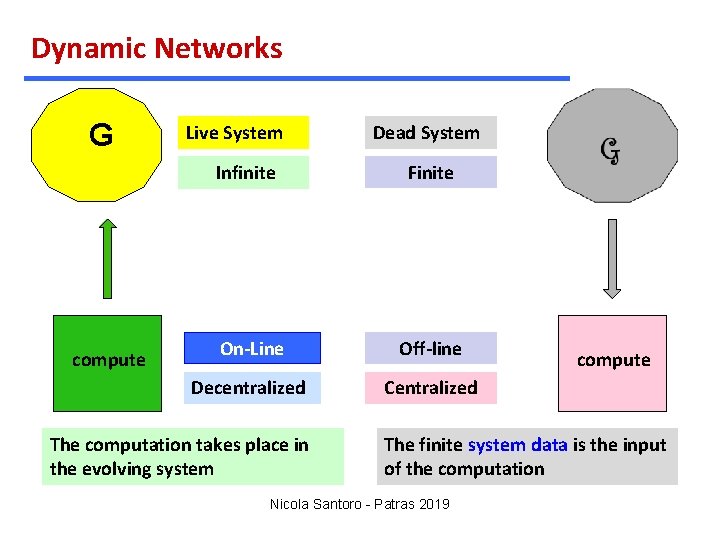
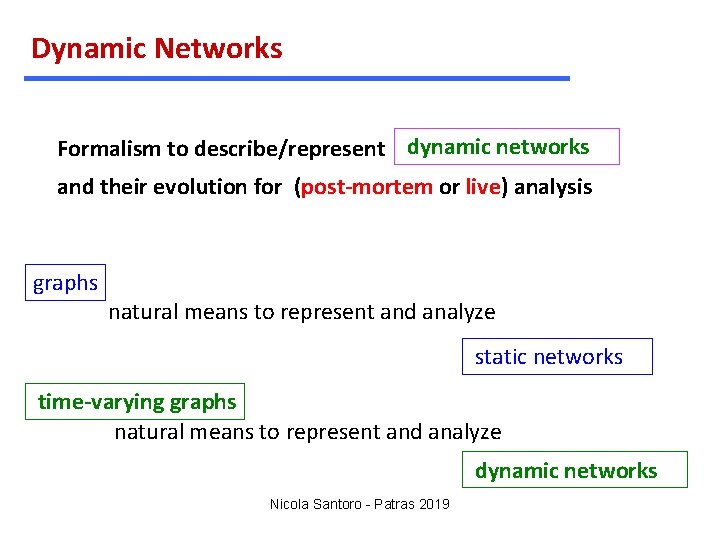
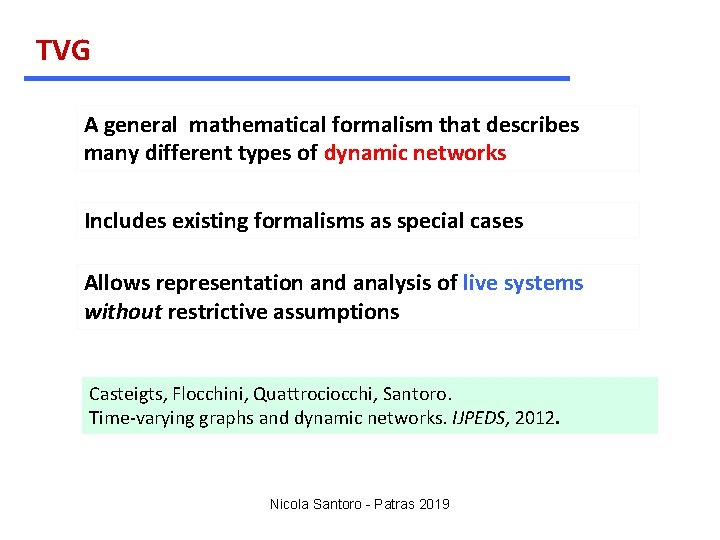
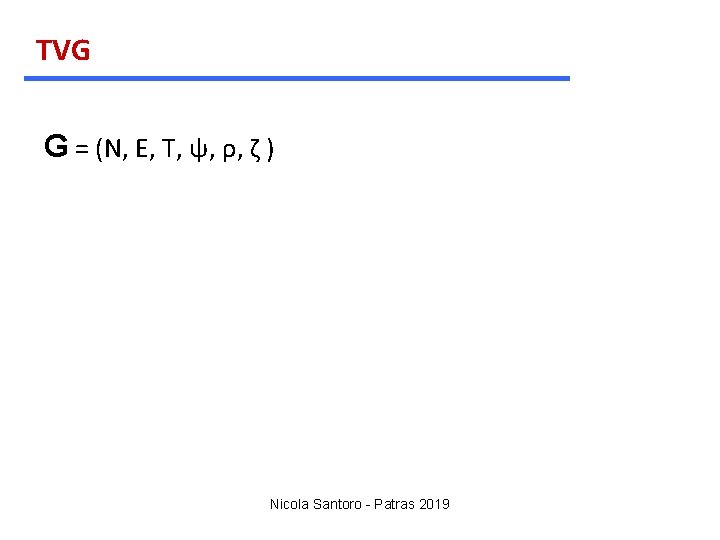
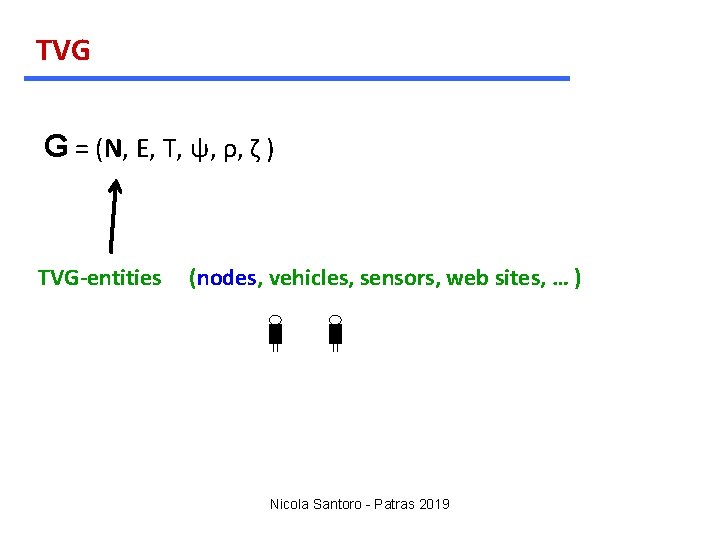
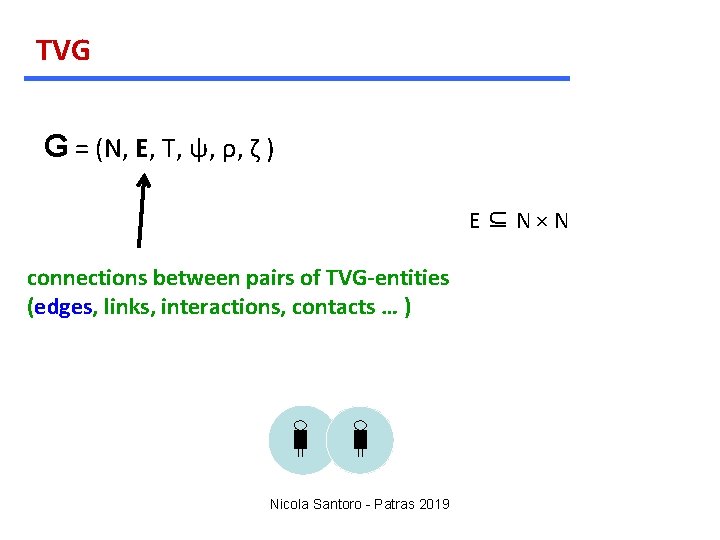
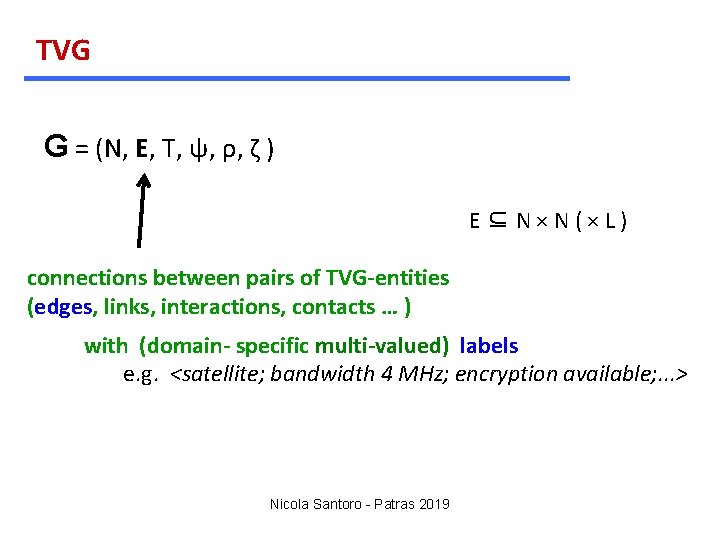
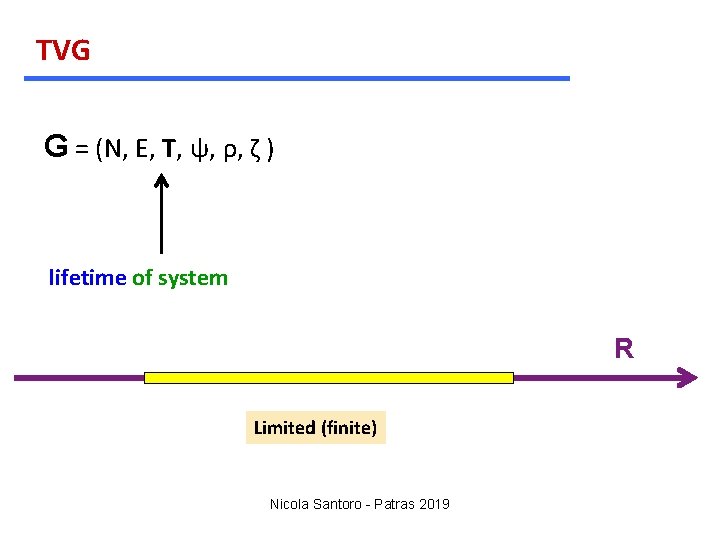
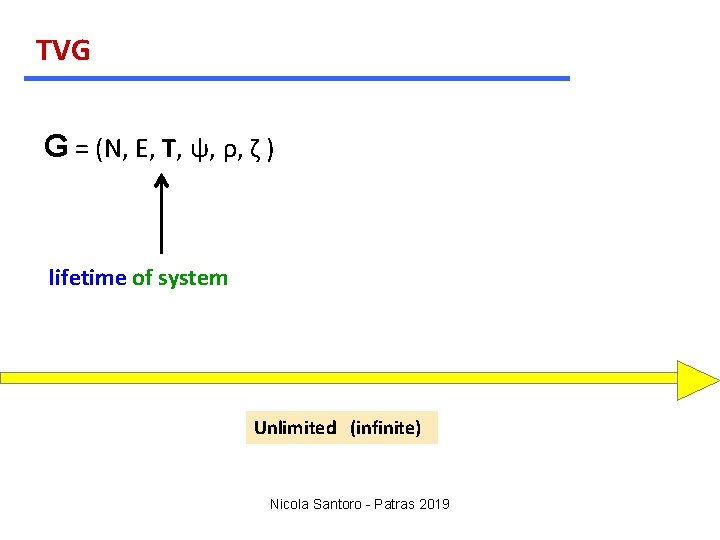
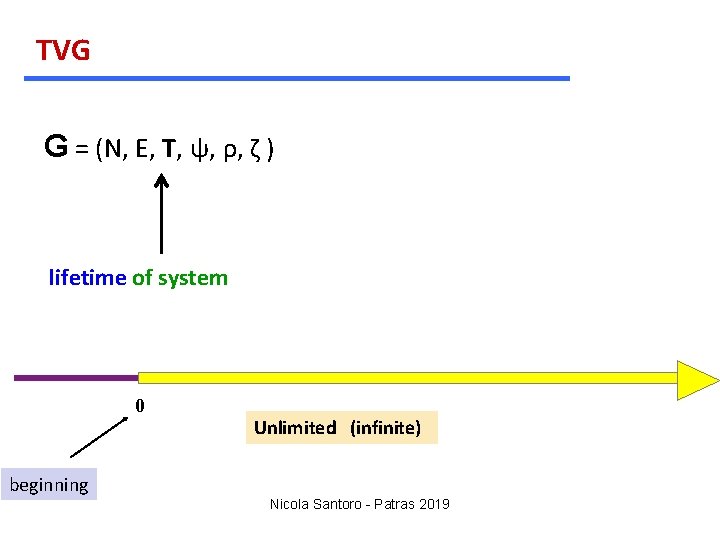
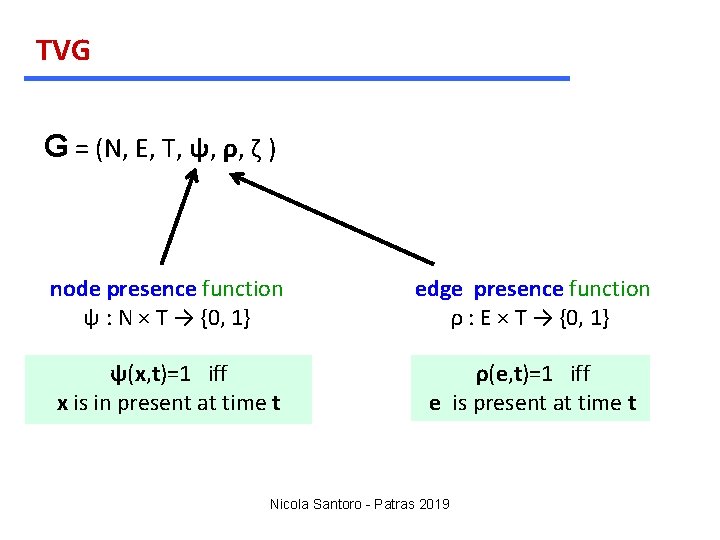
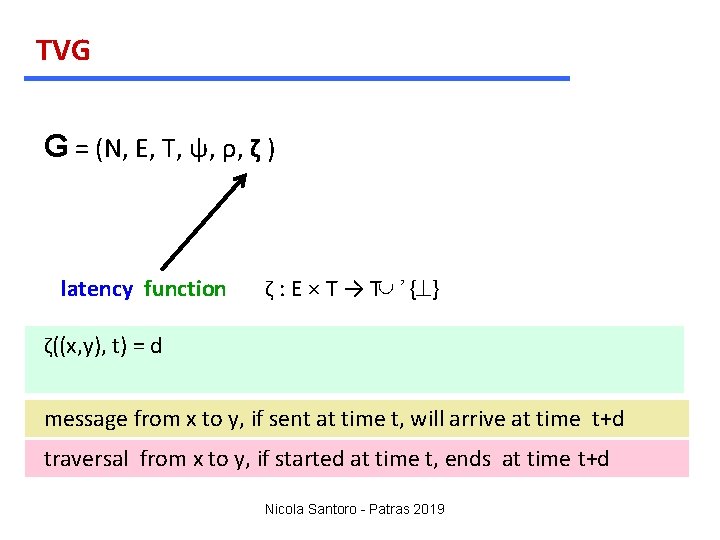
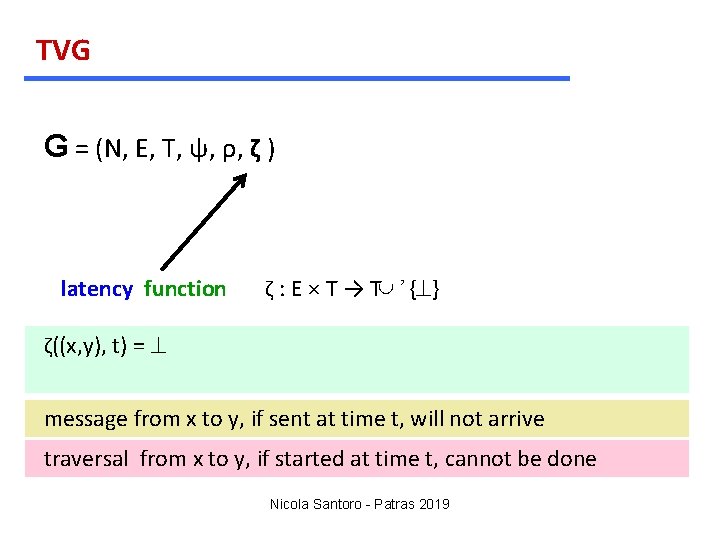
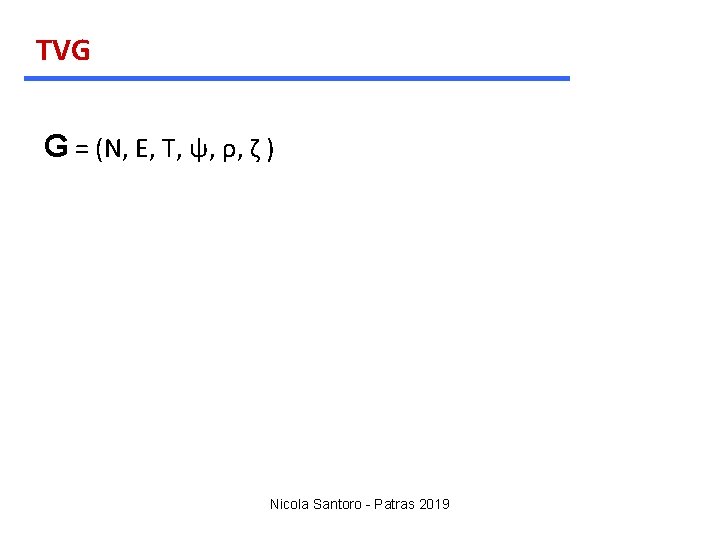
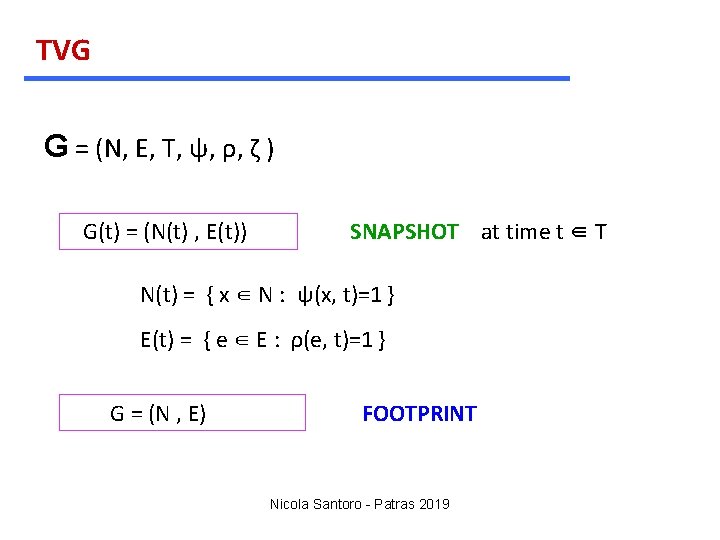
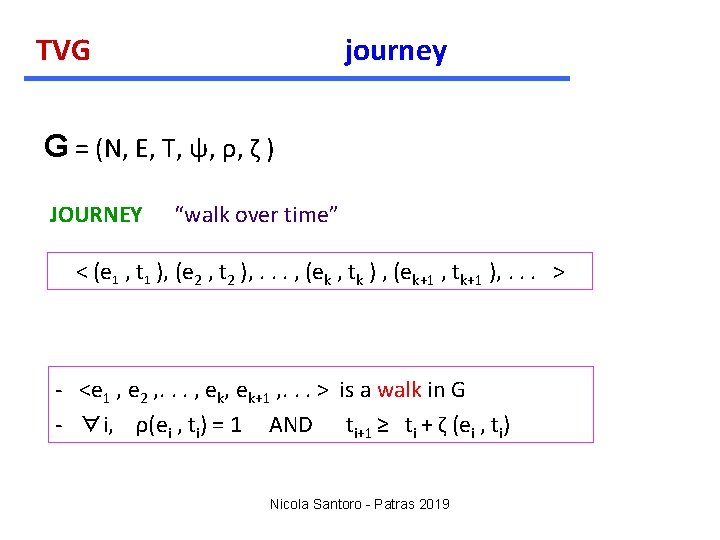
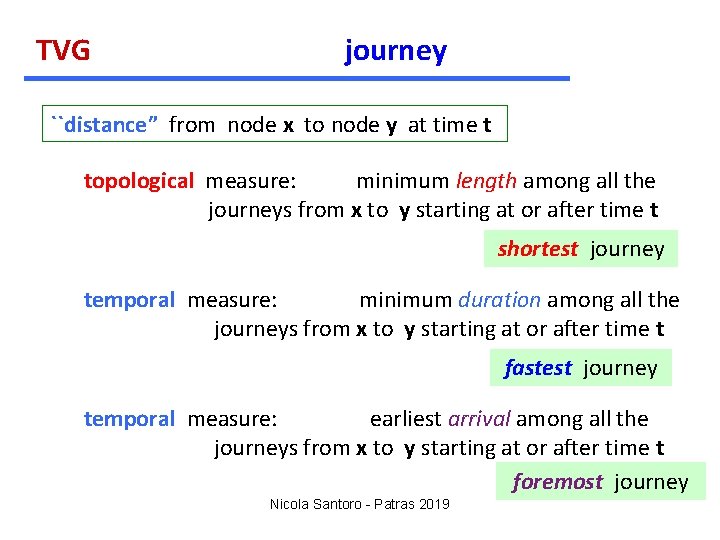
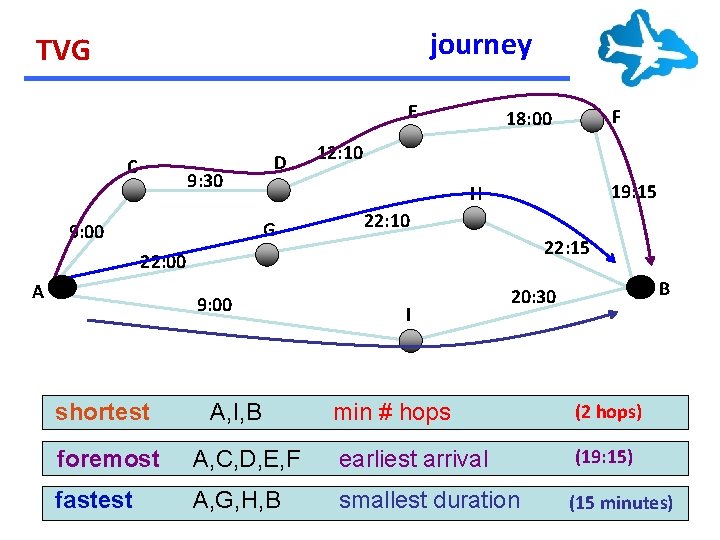
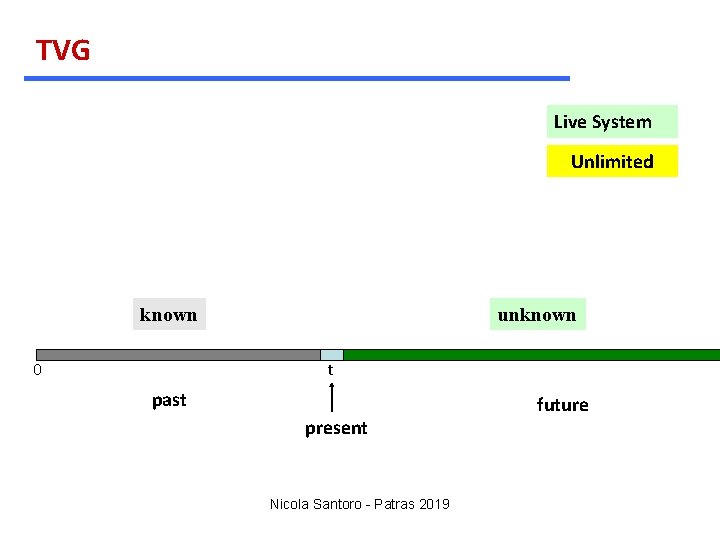
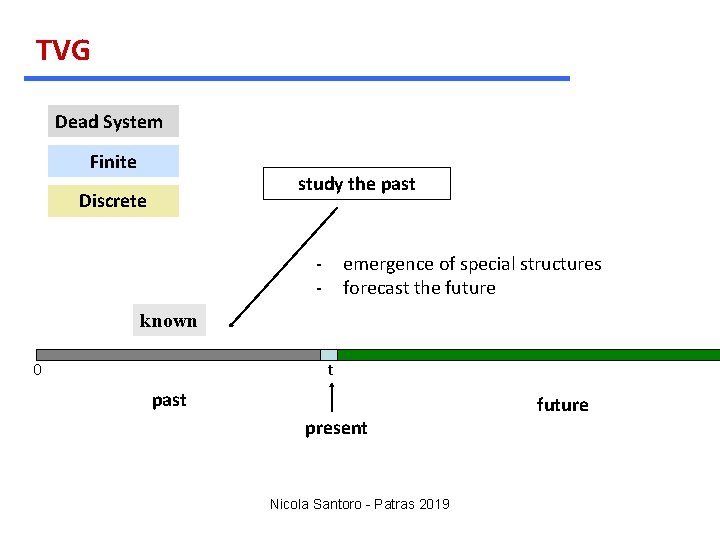
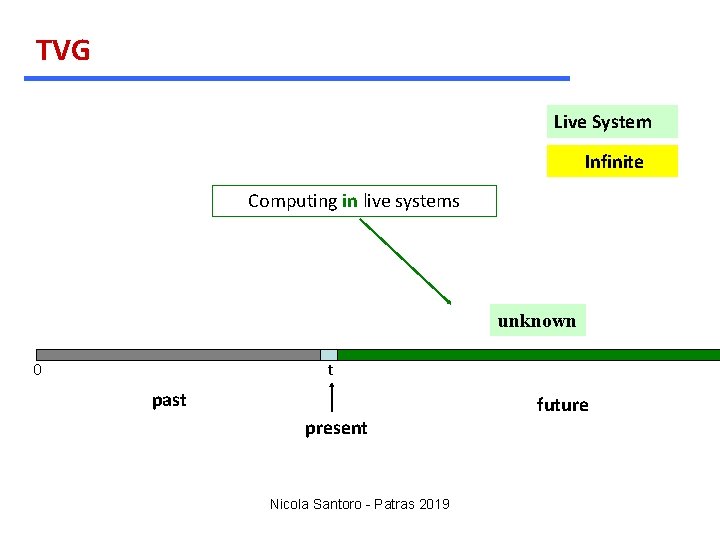
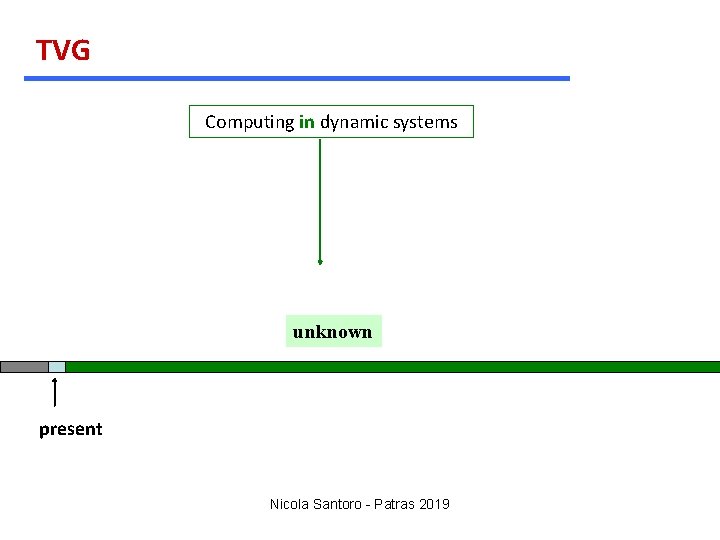
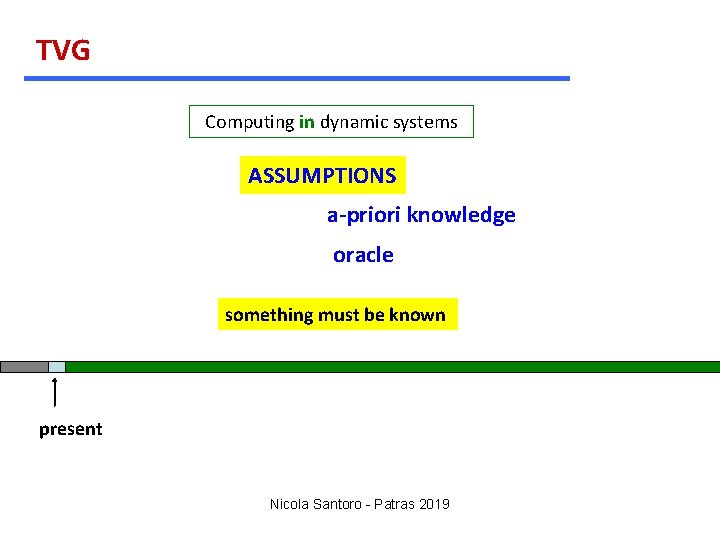
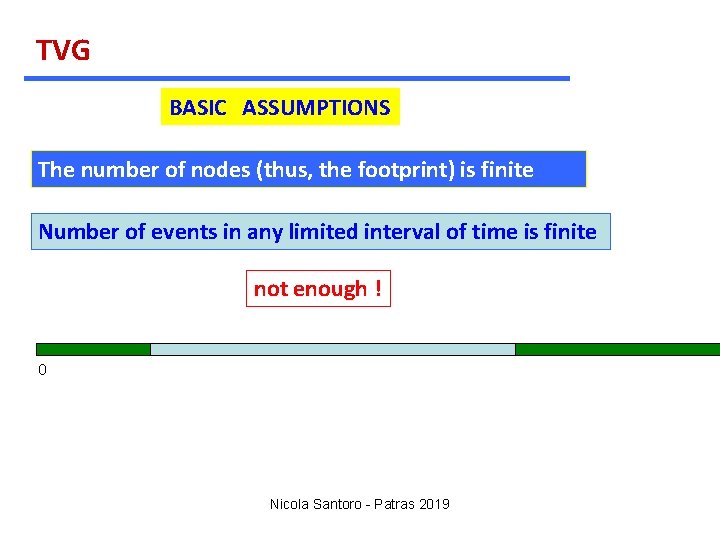
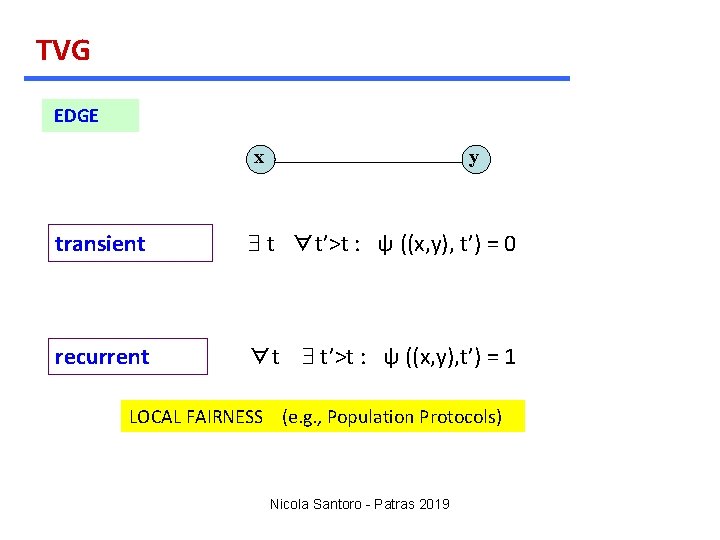
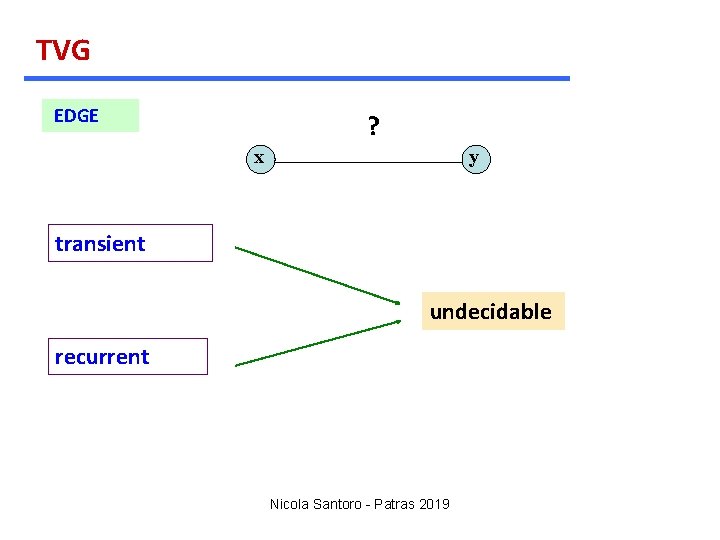
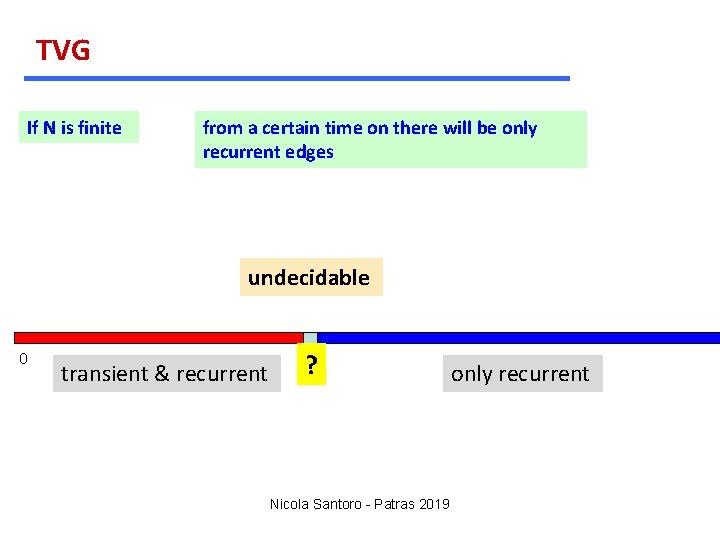
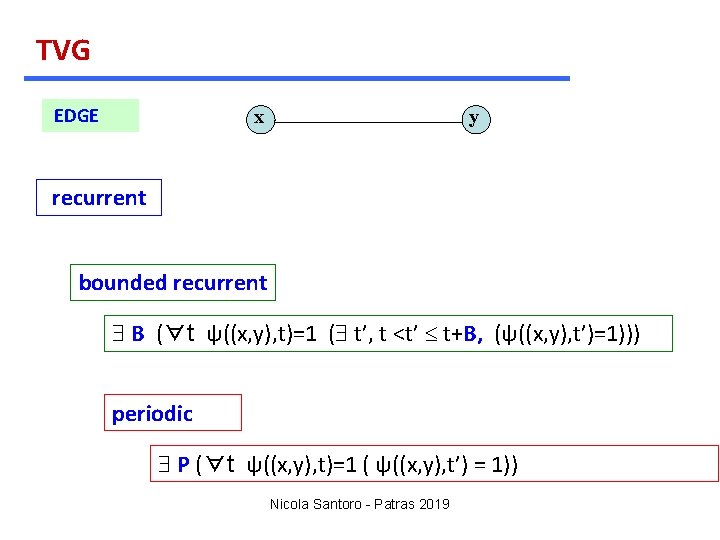
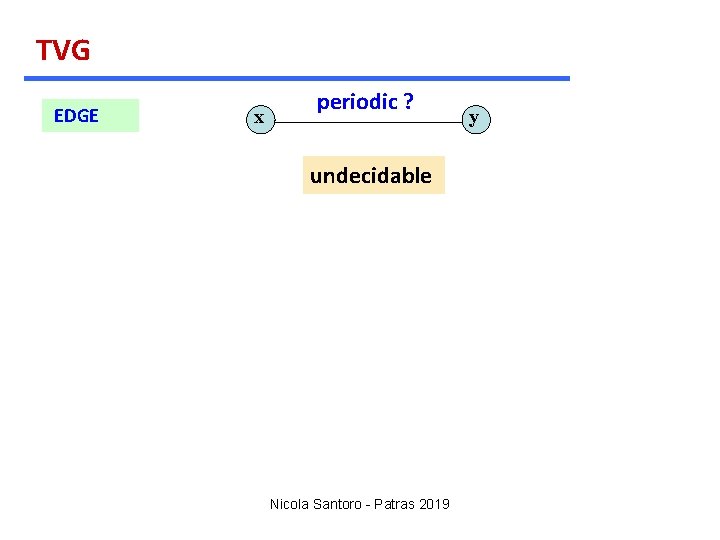
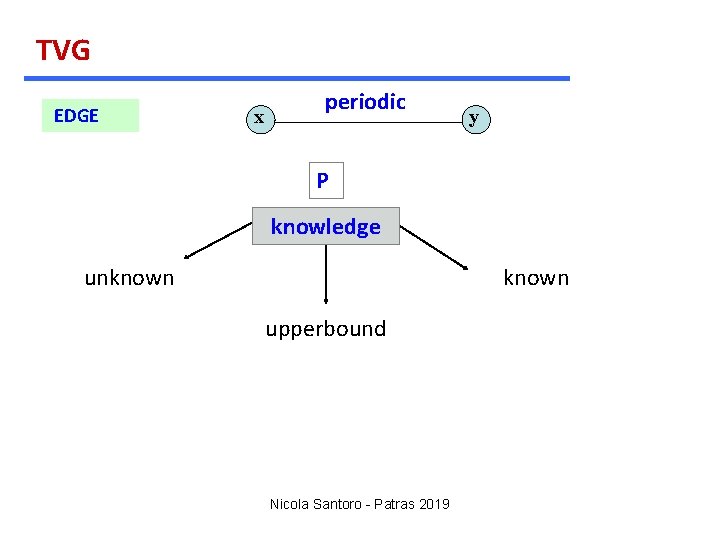
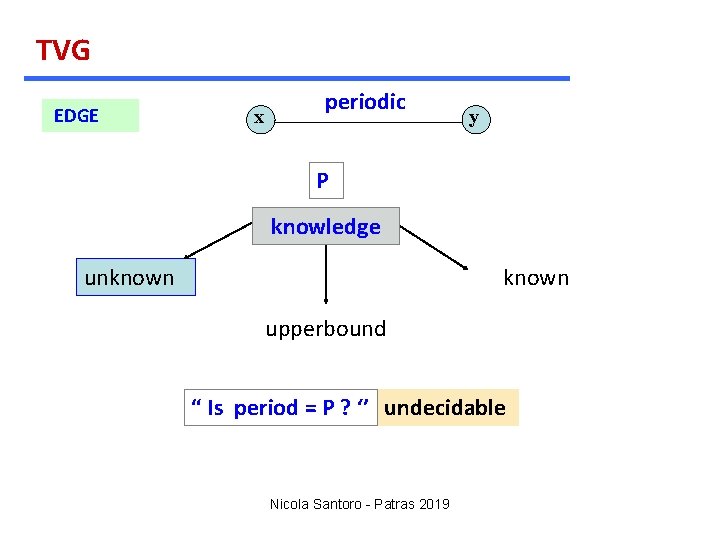
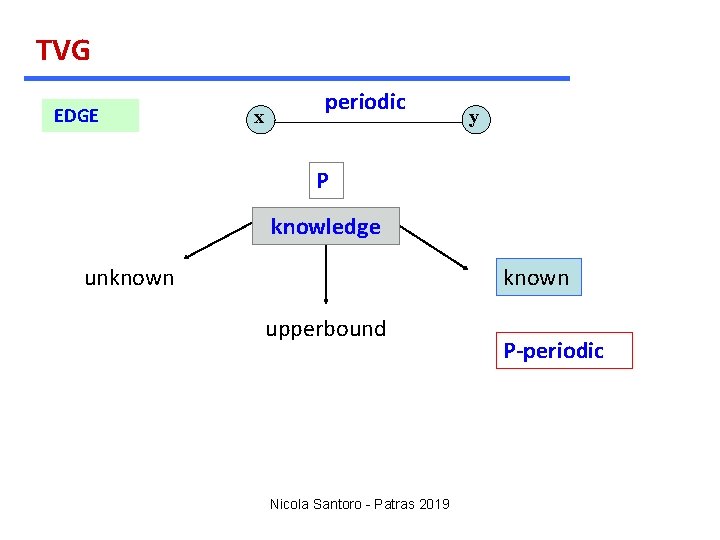
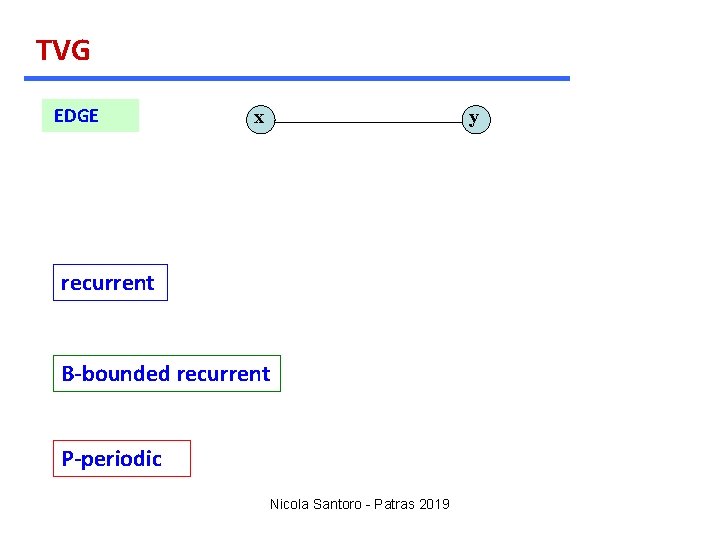
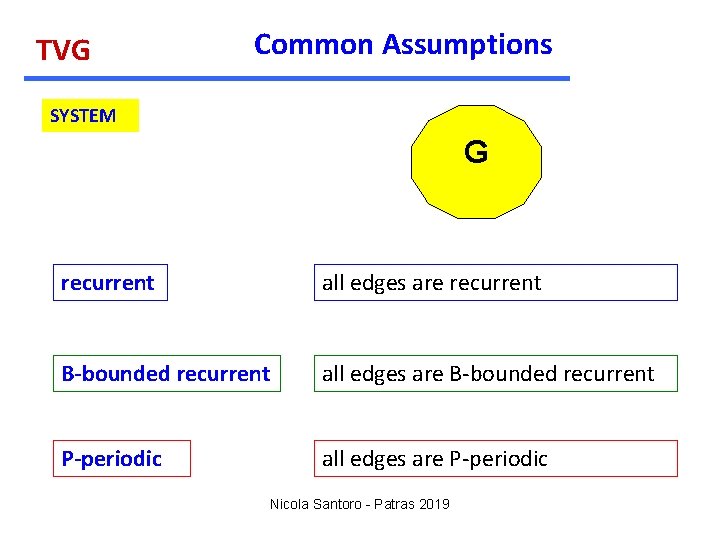
![TVG SYSTEMS CLASSES PROBLEMS computational power G[recurrent P[recurrent] ] B-bounded recurrent G[bounded] P-periodic G[periodic] TVG SYSTEMS CLASSES PROBLEMS computational power G[recurrent P[recurrent] ] B-bounded recurrent G[bounded] P-periodic G[periodic]](https://slidetodoc.com/presentation_image_h/20f0eb14b4e952b4479cc35f50d28925/image-56.jpg)
![TVG SYSTEMS PROBLEMS Foremost. Bcast ≺ Shortest. Bcast ≺ Fastest. Bcast BROADCAST recurrent P[recurrent] TVG SYSTEMS PROBLEMS Foremost. Bcast ≺ Shortest. Bcast ≺ Fastest. Bcast BROADCAST recurrent P[recurrent]](https://slidetodoc.com/presentation_image_h/20f0eb14b4e952b4479cc35f50d28925/image-57.jpg)
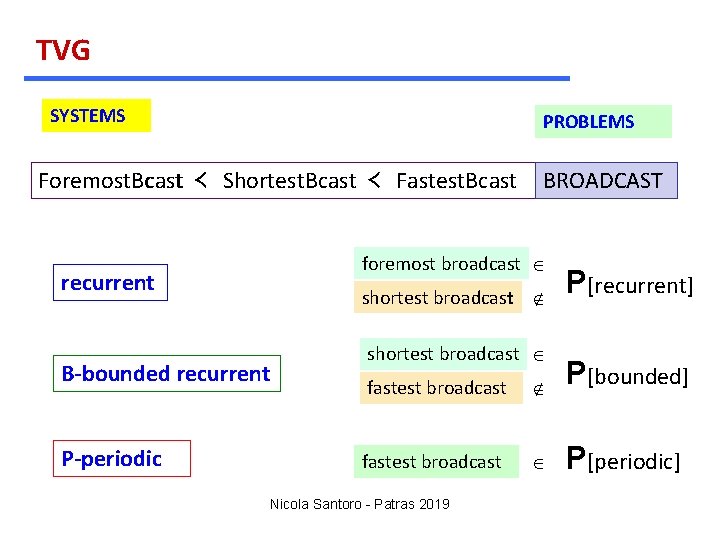
![TVG Foremost. Bcast ≺ Shortest. Bcast ≺ Fastest. Bcast P[periodic] periodic Í/ P[bounded] Í/ TVG Foremost. Bcast ≺ Shortest. Bcast ≺ Fastest. Bcast P[periodic] periodic Í/ P[bounded] Í/](https://slidetodoc.com/presentation_image_h/20f0eb14b4e952b4479cc35f50d28925/image-59.jpg)
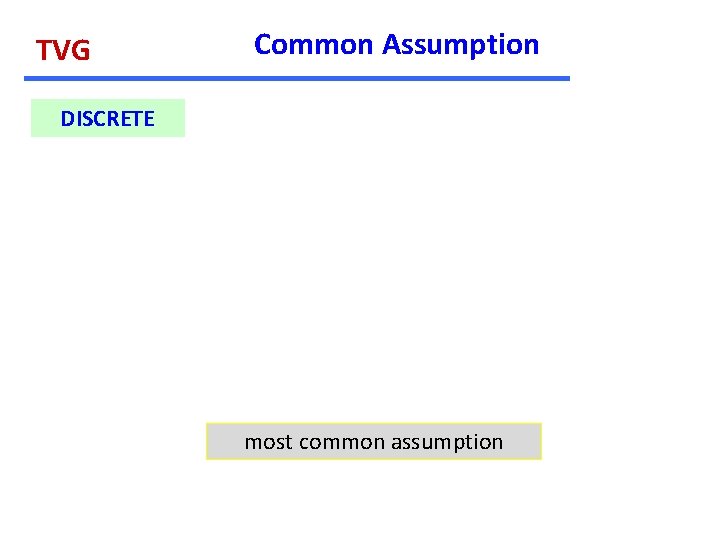
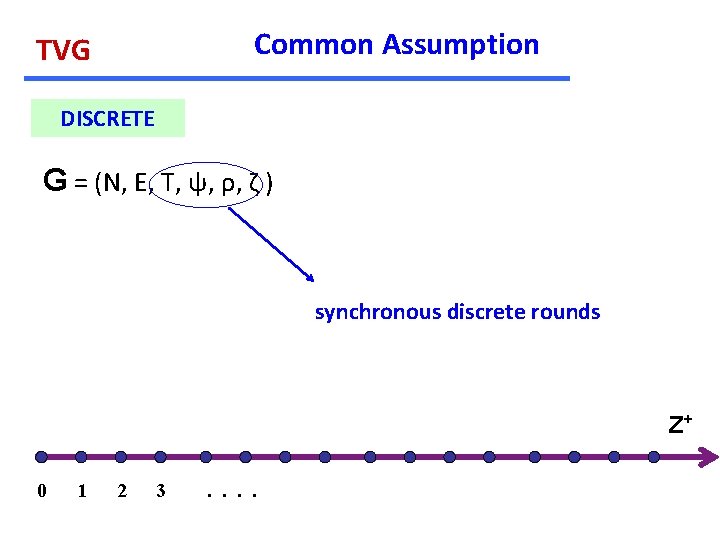
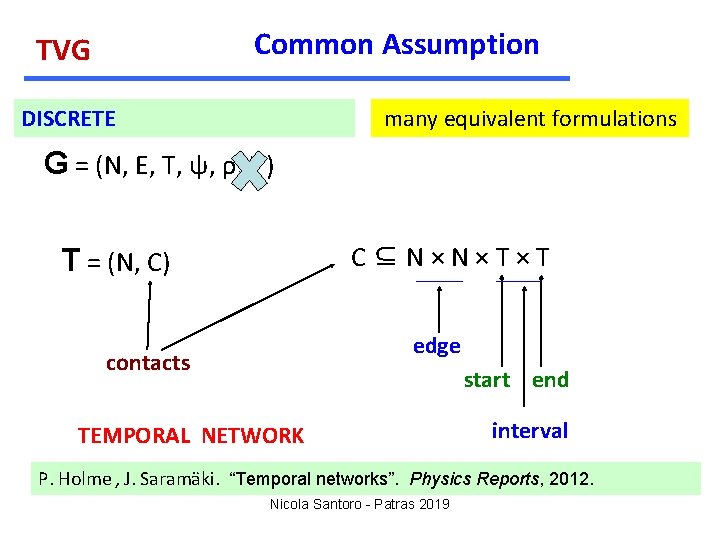
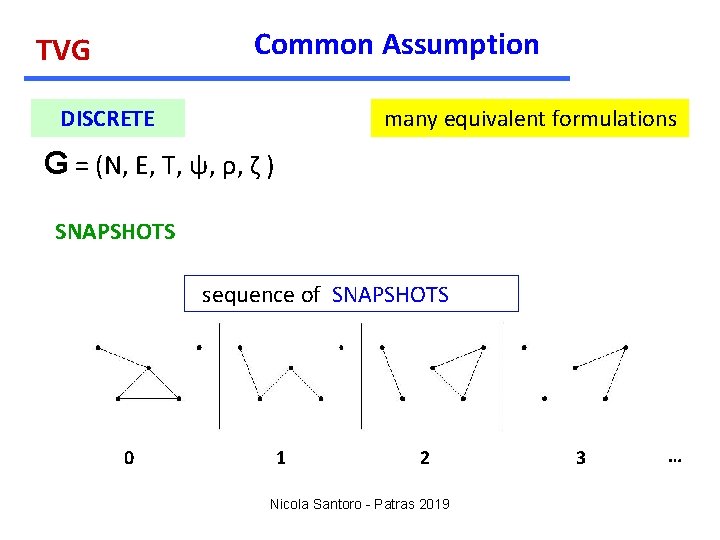
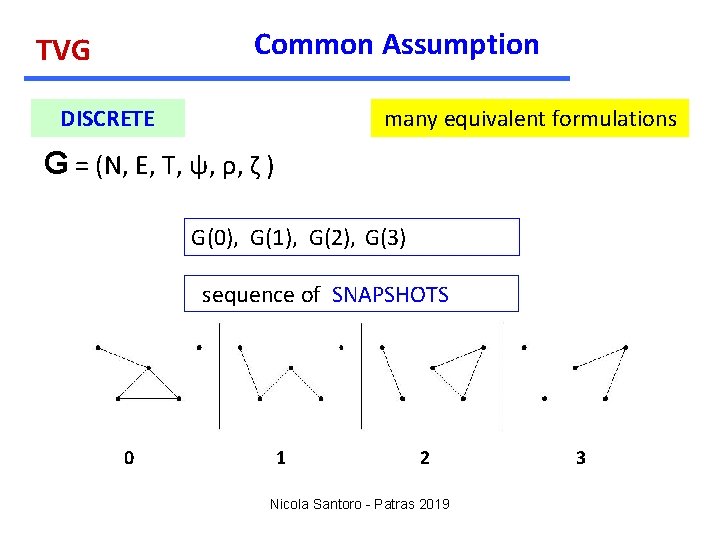
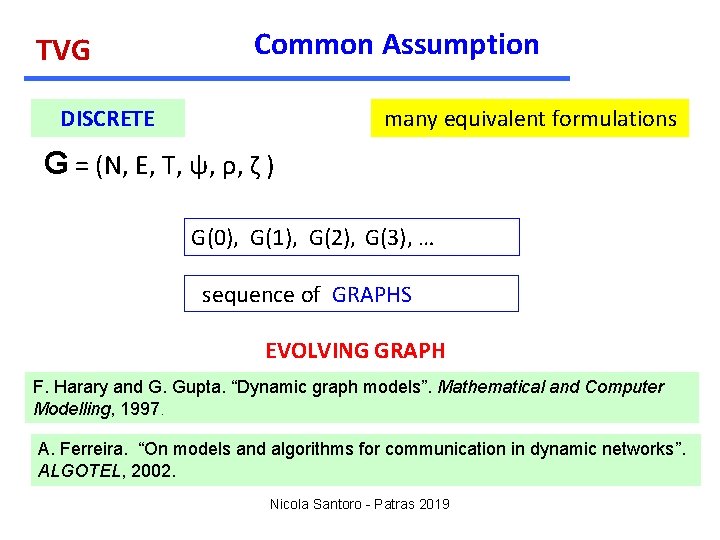
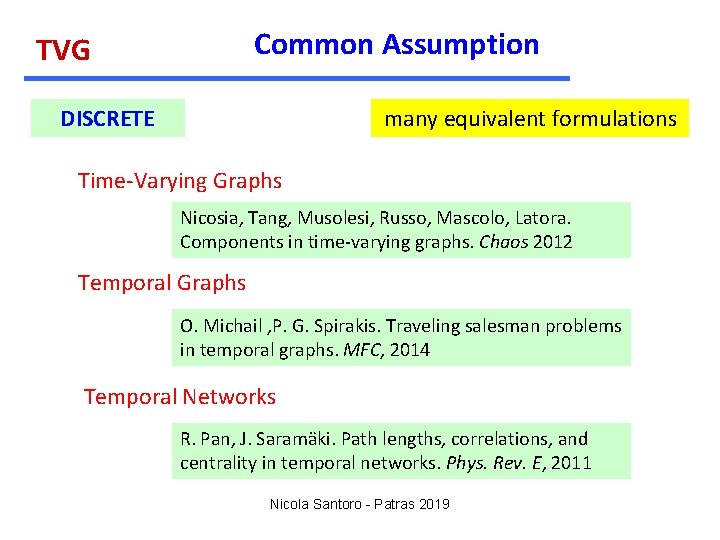
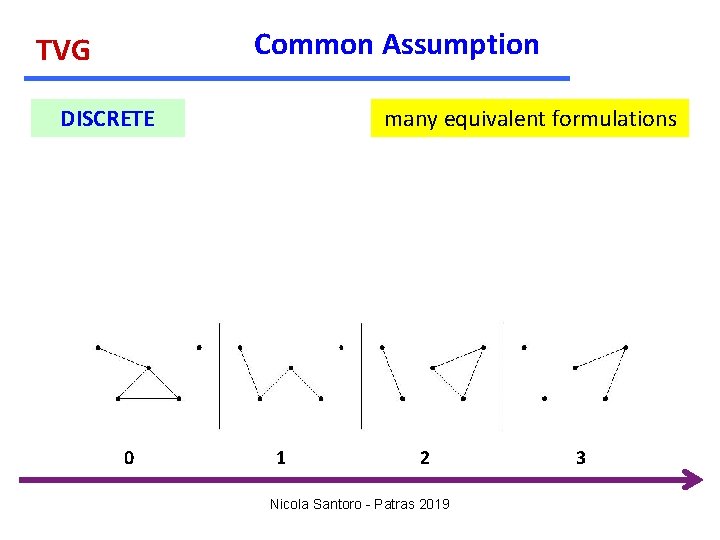
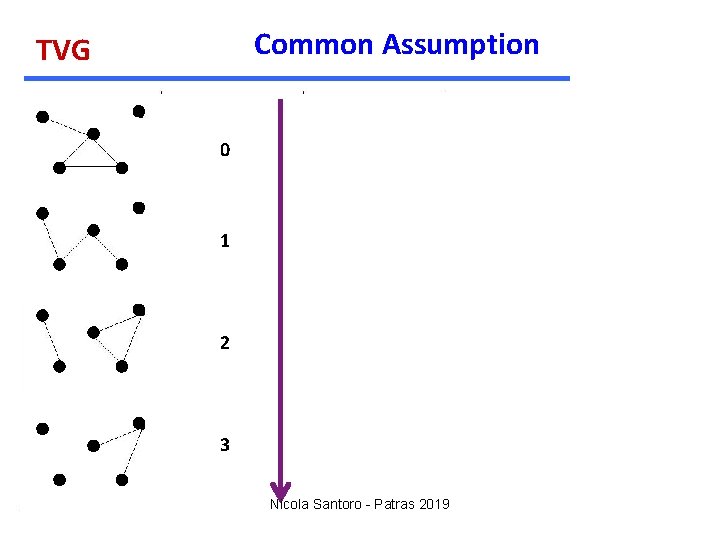
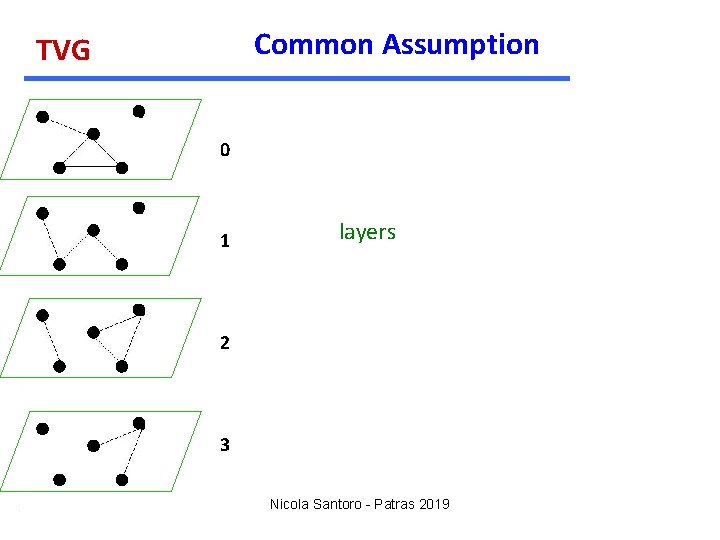
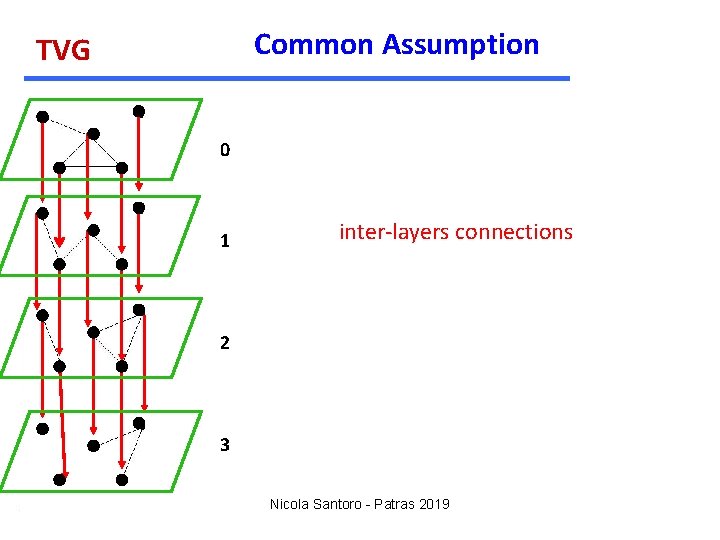
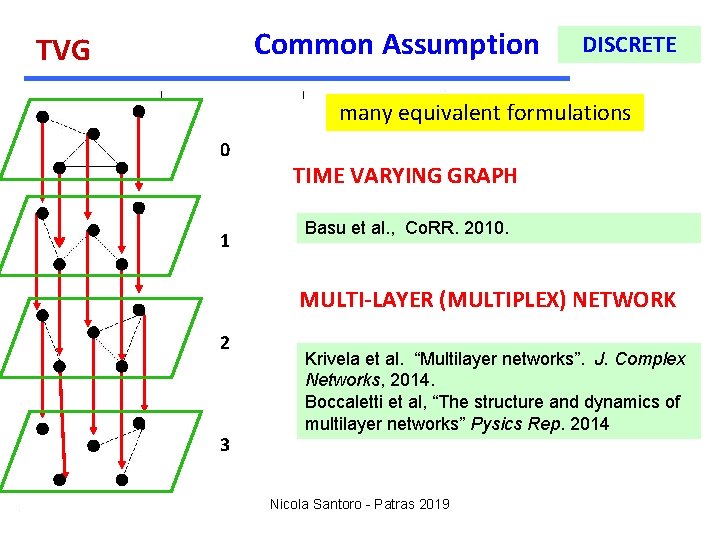
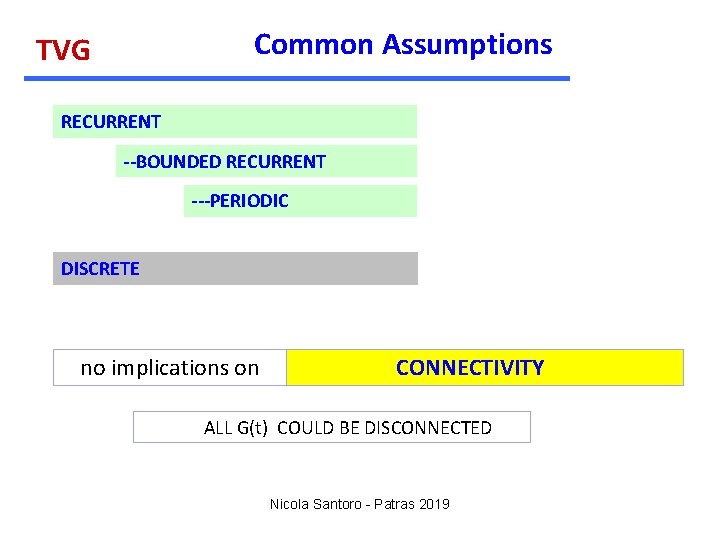
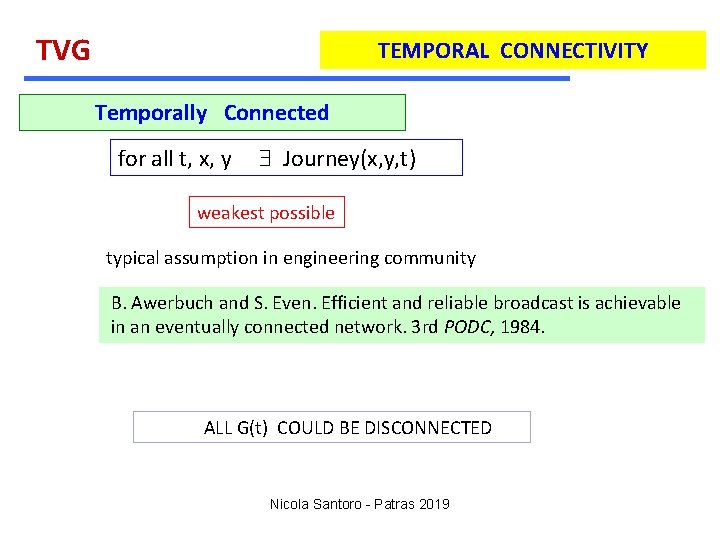
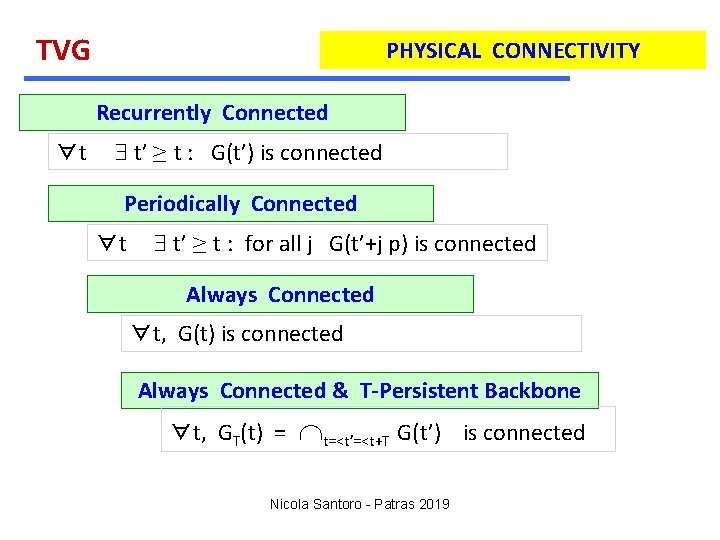
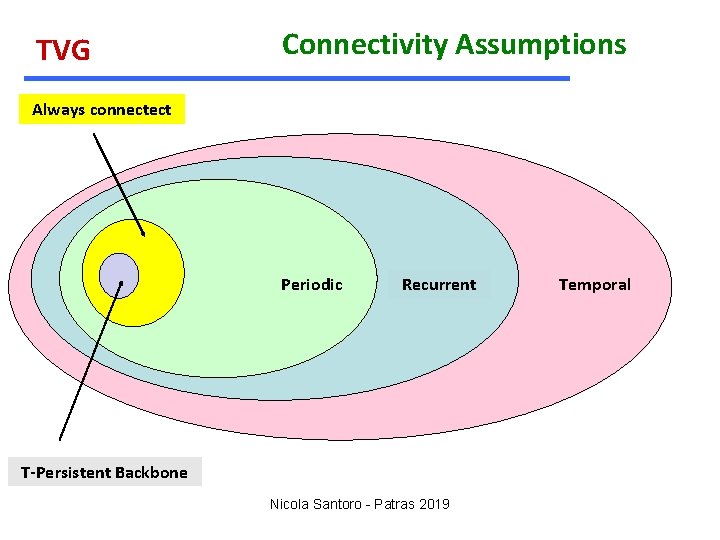
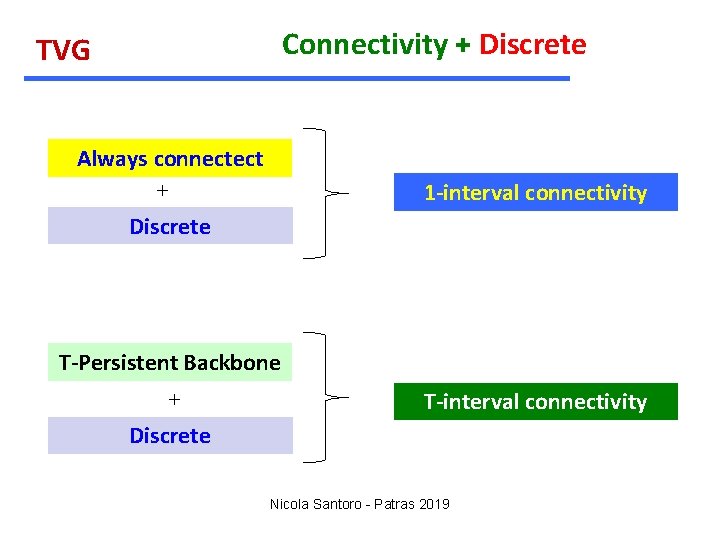
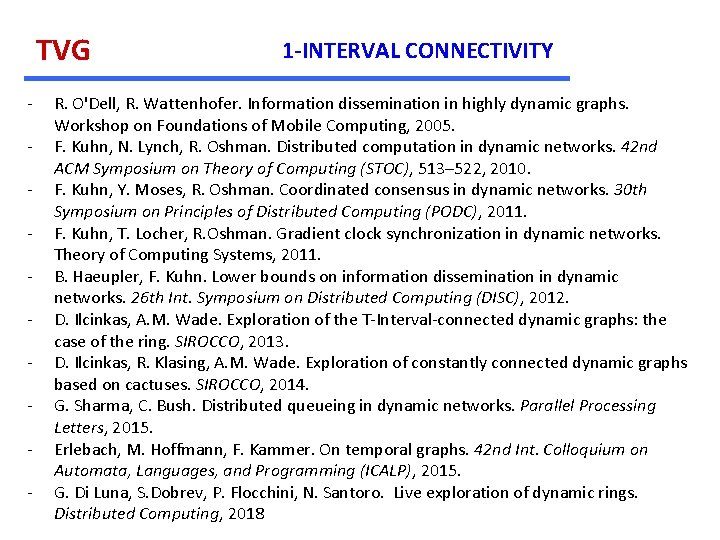
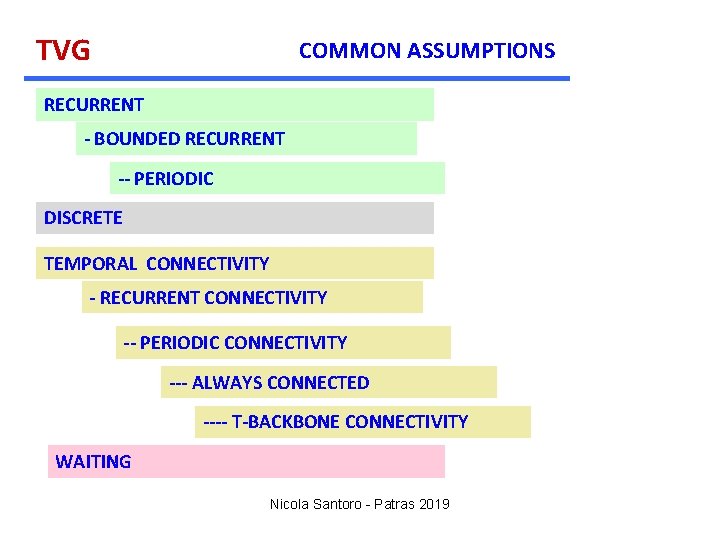
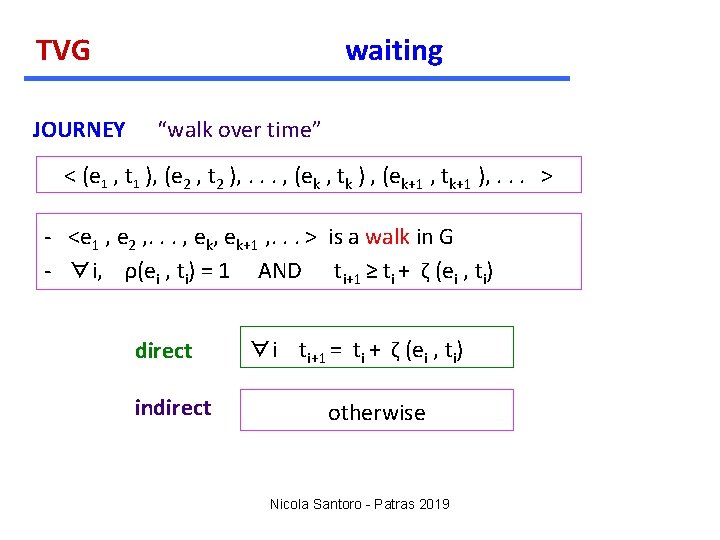
![TVG waiting A mobile agent E [1, 3) Traverse path [A, E, D] t=0 TVG waiting A mobile agent E [1, 3) Traverse path [A, E, D] t=0](https://slidetodoc.com/presentation_image_h/20f0eb14b4e952b4479cc35f50d28925/image-80.jpg)
![TVG waiting A E [1, 3) Traverse path [A, E, D] t=1 WAIT at TVG waiting A E [1, 3) Traverse path [A, E, D] t=1 WAIT at](https://slidetodoc.com/presentation_image_h/20f0eb14b4e952b4479cc35f50d28925/image-81.jpg)
![Time-Varying Graph: waiting A E [1, 3) Traverse path [A, E, D] t=2 t=3 Time-Varying Graph: waiting A E [1, 3) Traverse path [A, E, D] t=2 t=3](https://slidetodoc.com/presentation_image_h/20f0eb14b4e952b4479cc35f50d28925/image-82.jpg)
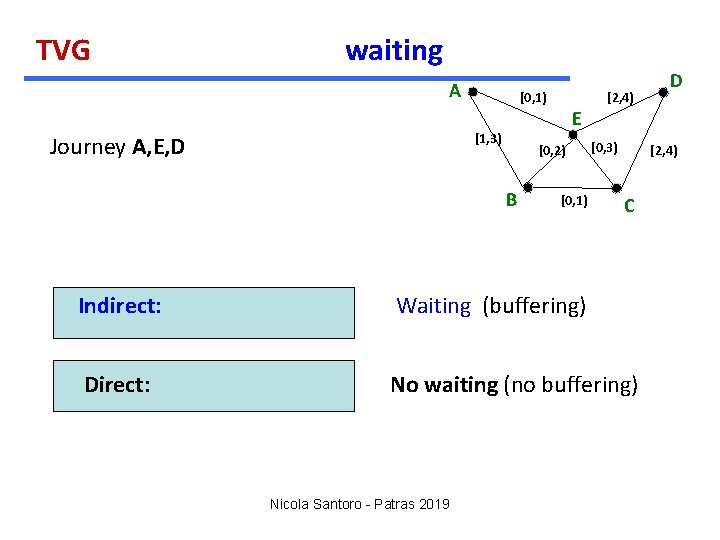
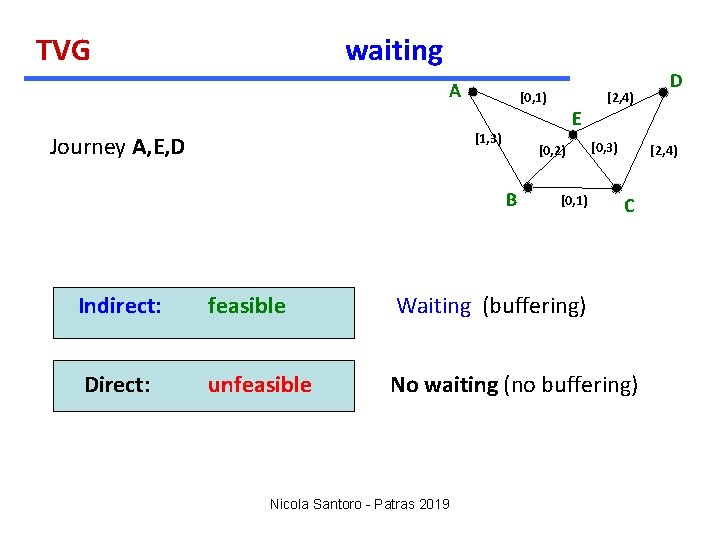
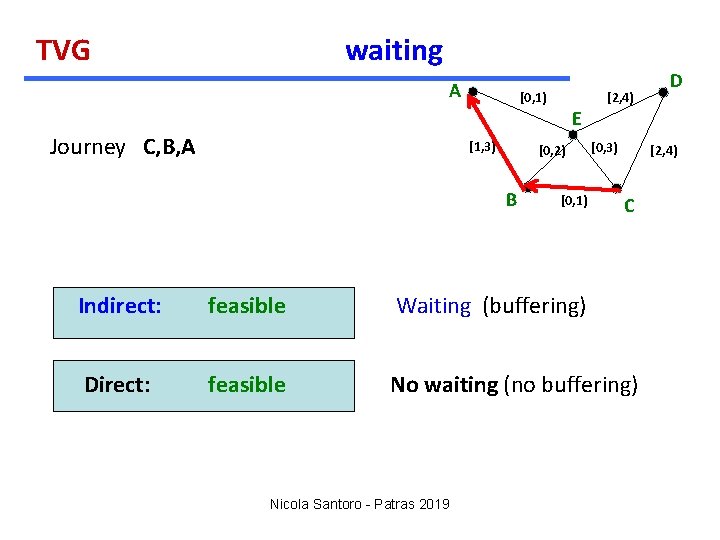
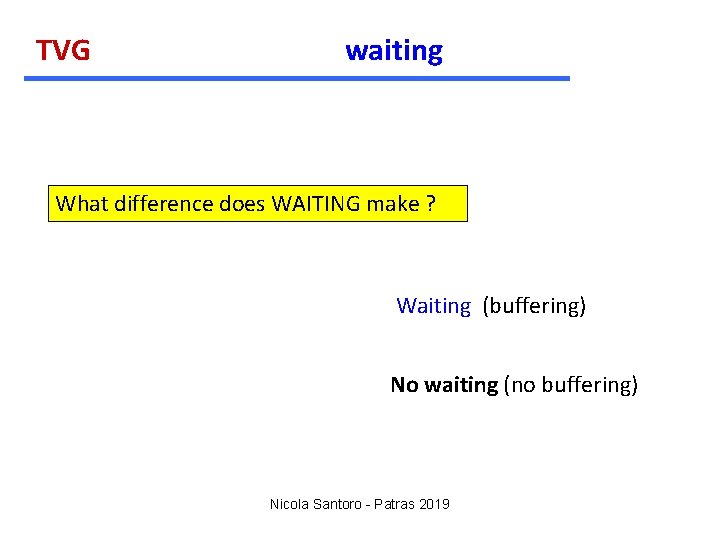
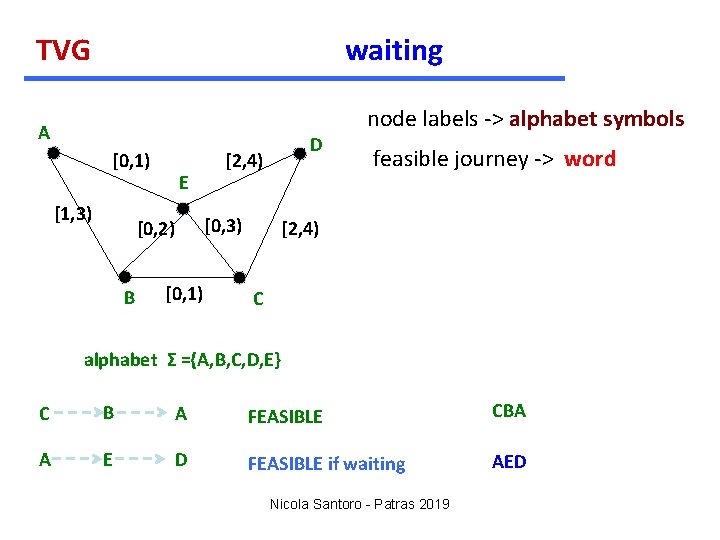
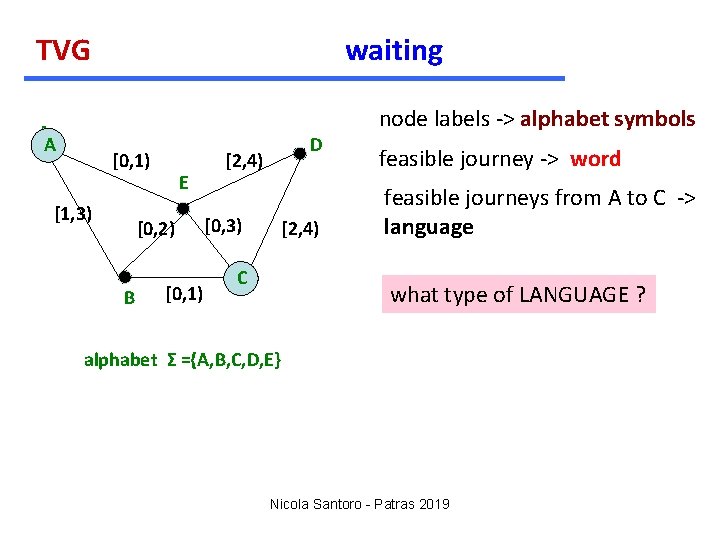
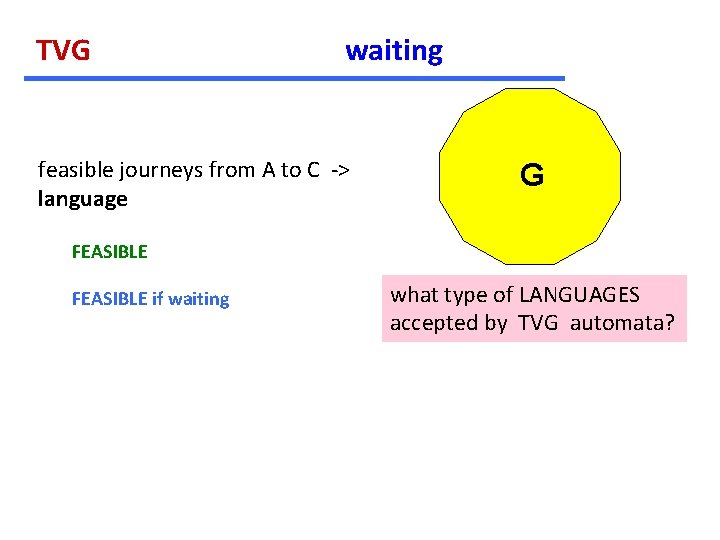
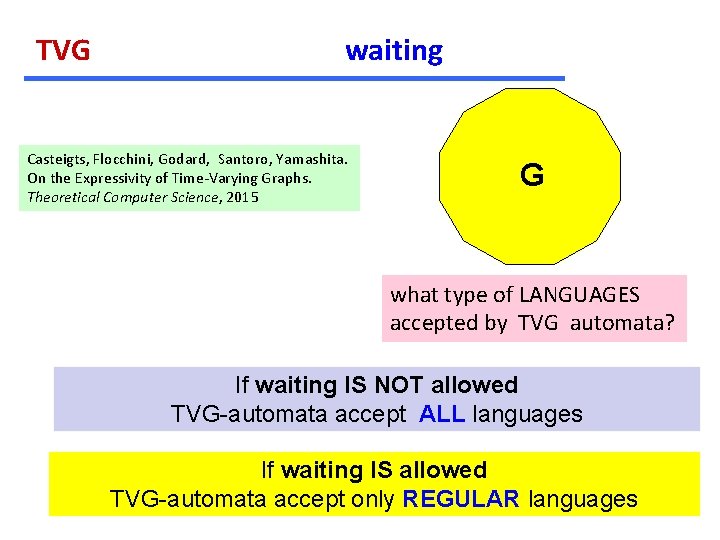
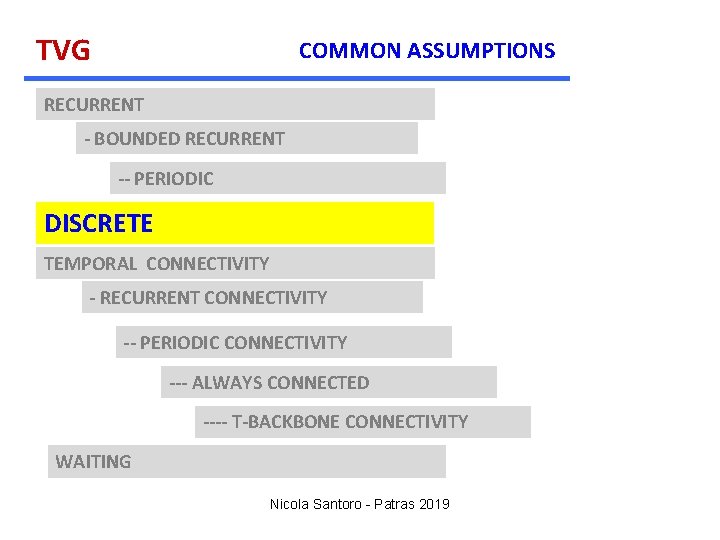
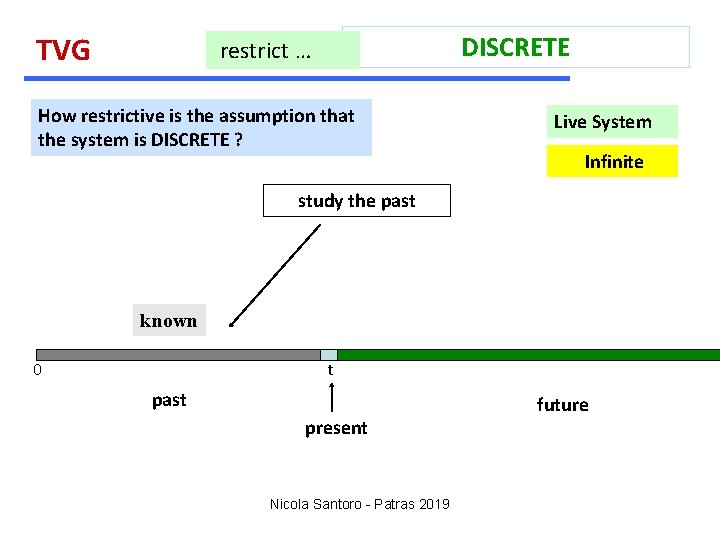
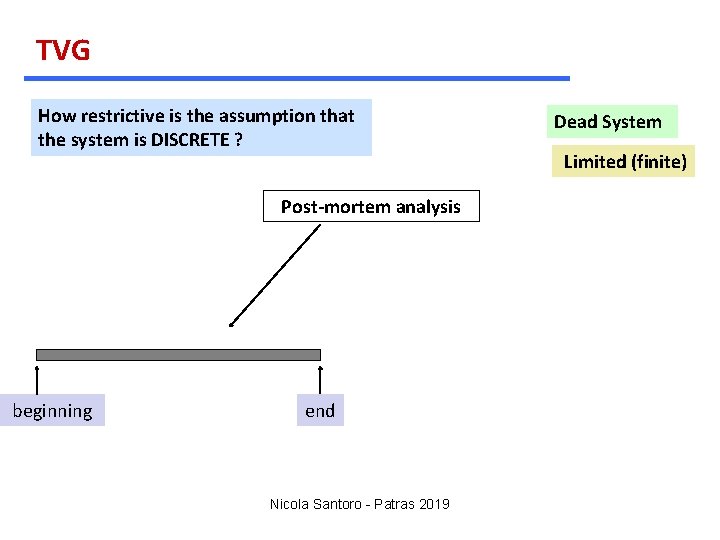
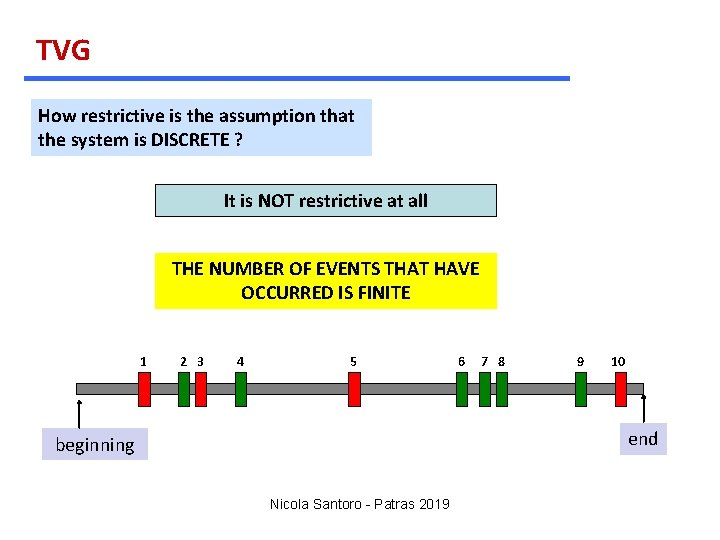
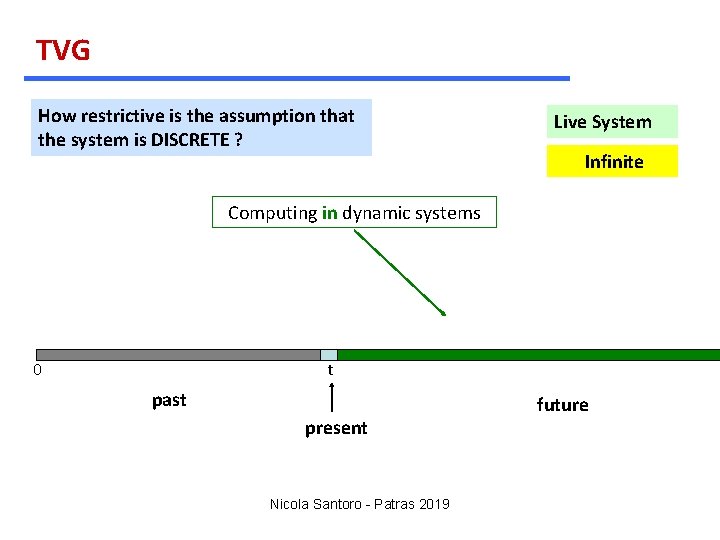
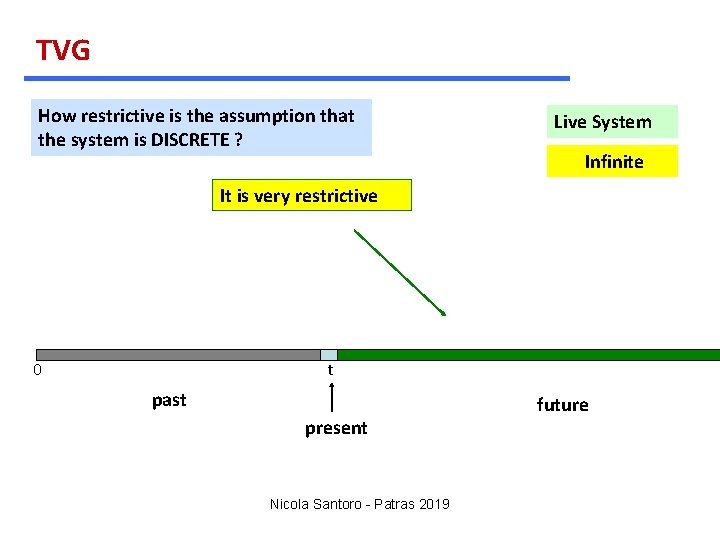
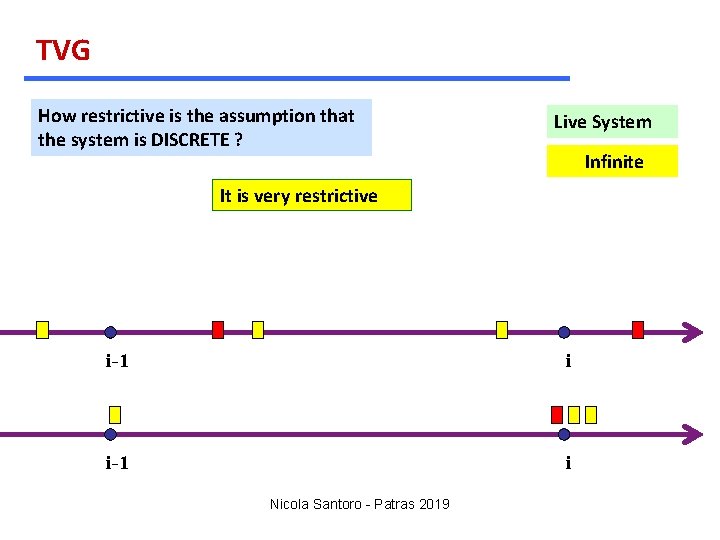
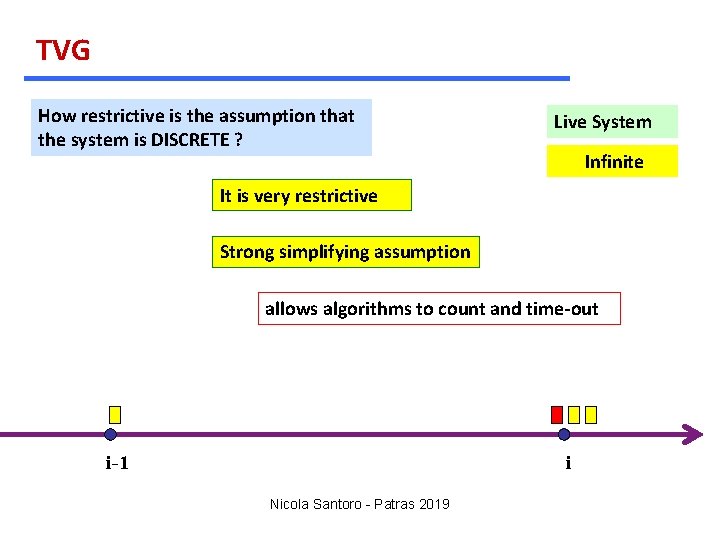
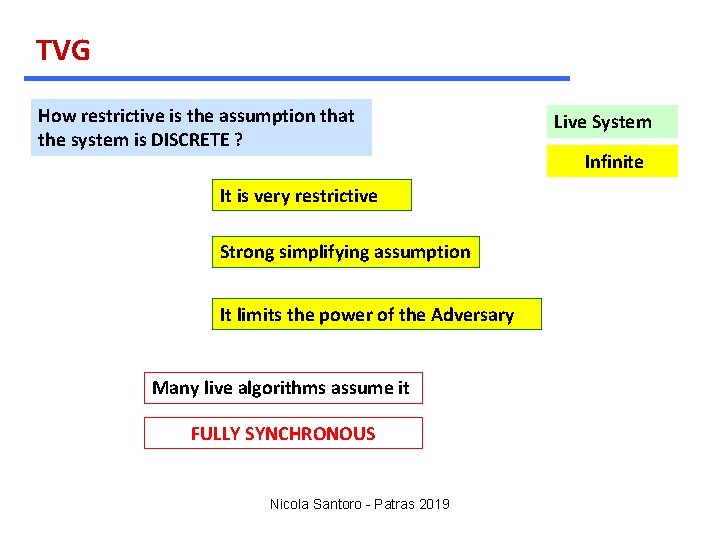
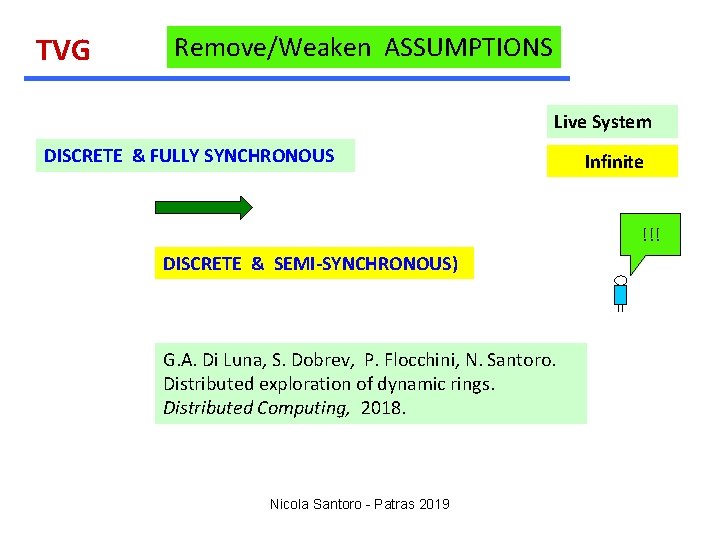
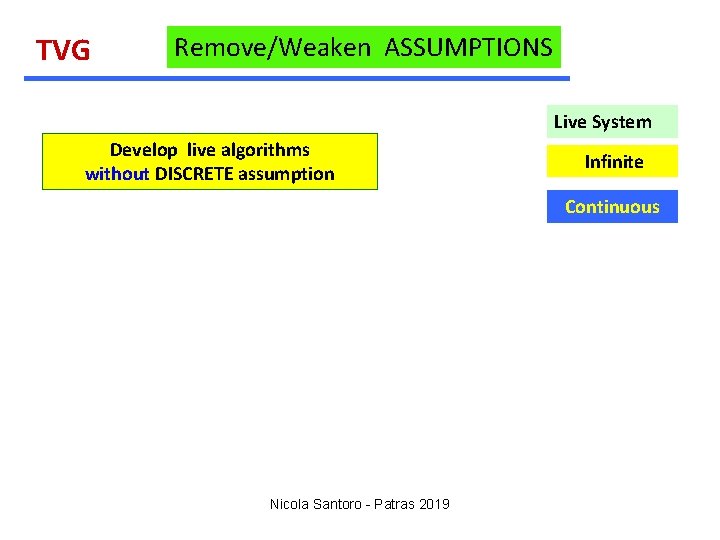
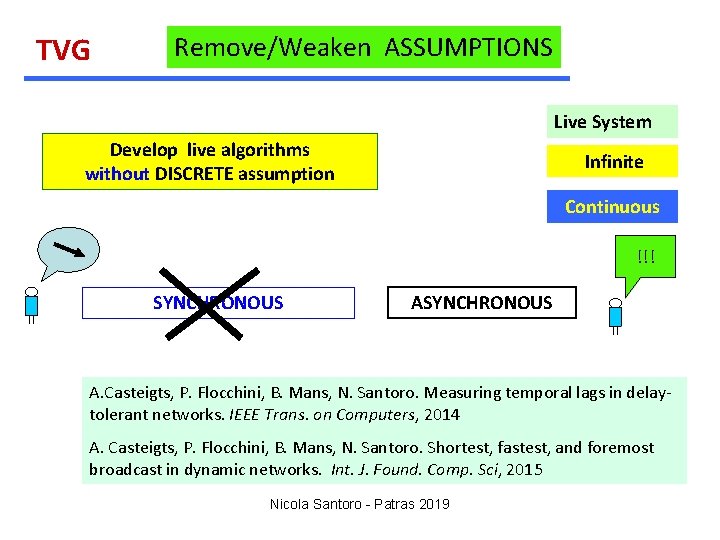
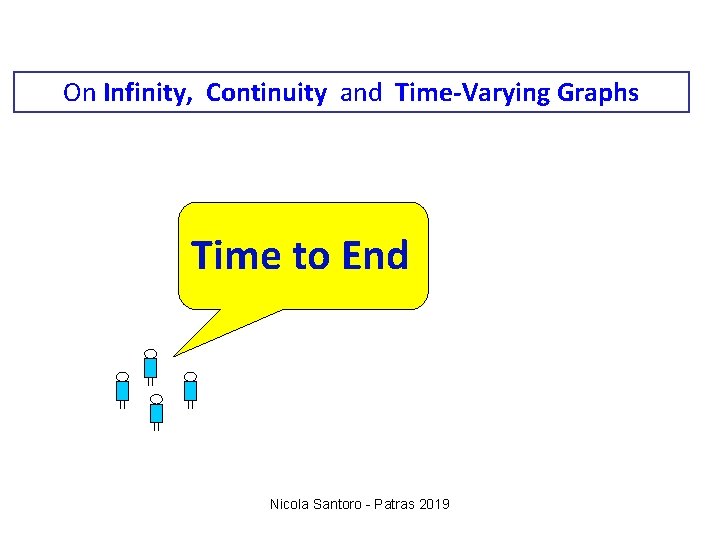
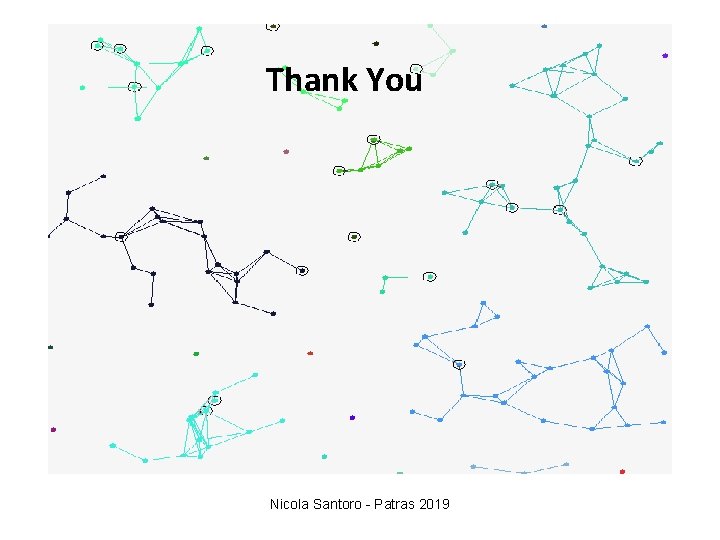
- Slides: 104
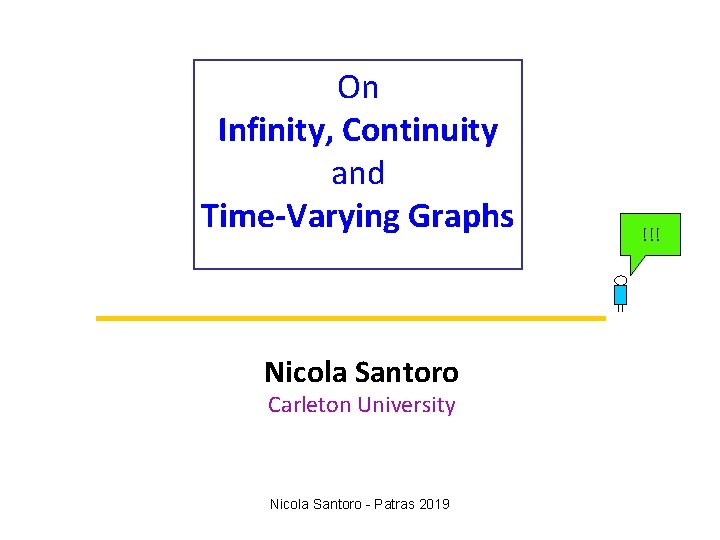
On Infinity, Continuity and Time-Varying Graphs Nicola Santoro Carleton University Nicola Santoro - Patras 2019 !!!
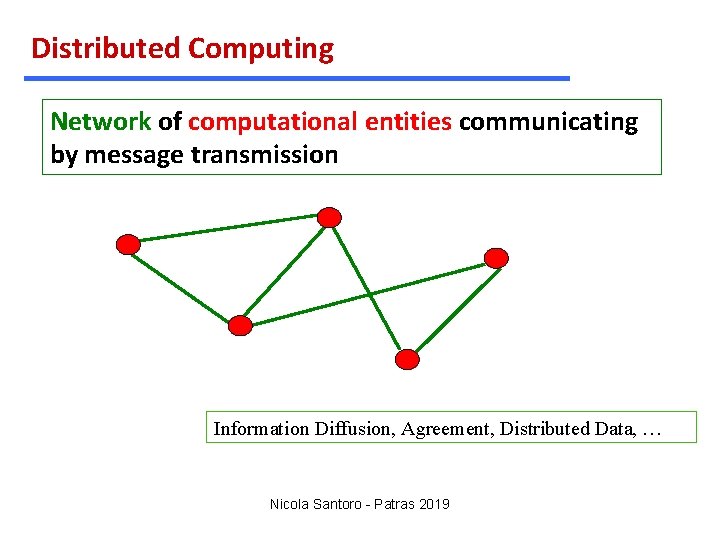
Distributed Computing Network of computational entities communicating by message transmission Information Diffusion, Agreement, Distributed Data, … Nicola Santoro - Patras 2019
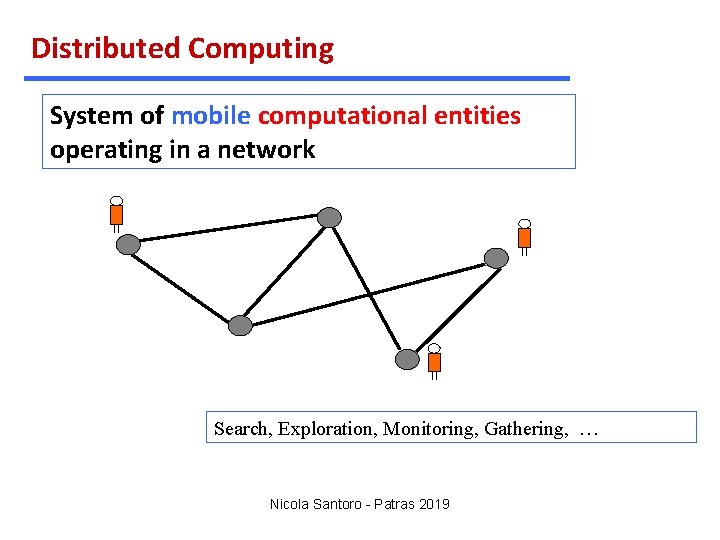
Distributed Computing System of mobile computational entities operating in a network Search, Exploration, Monitoring, Gathering, … Nicola Santoro - Patras 2019
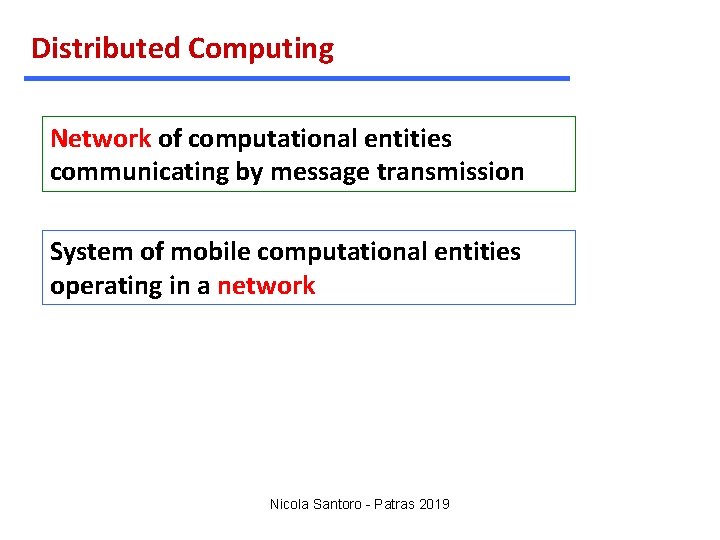
Distributed Computing Network of computational entities communicating by message transmission System of mobile computational entities operating in a network Nicola Santoro - Patras 2019
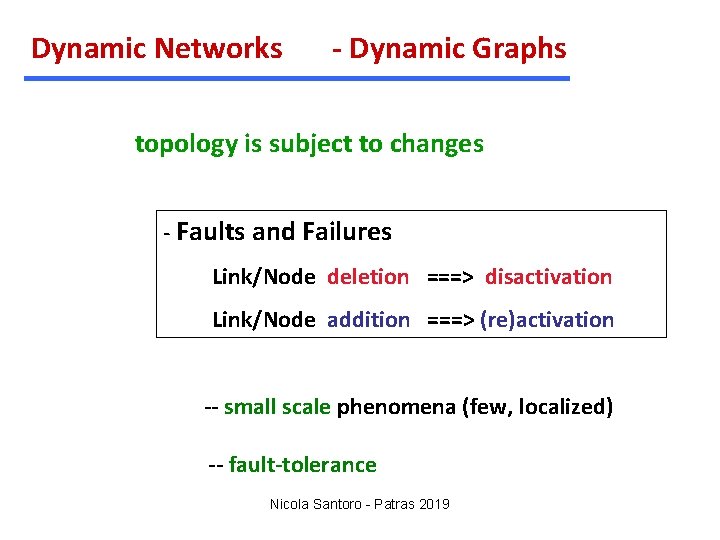
Dynamic Networks - Dynamic Graphs topology is subject to changes - Faults and Failures Link/Node deletion ===> disactivation Link/Node addition ===> (re)activation -- small scale phenomena (few, localized) -- fault-tolerance Nicola Santoro - Patras 2019
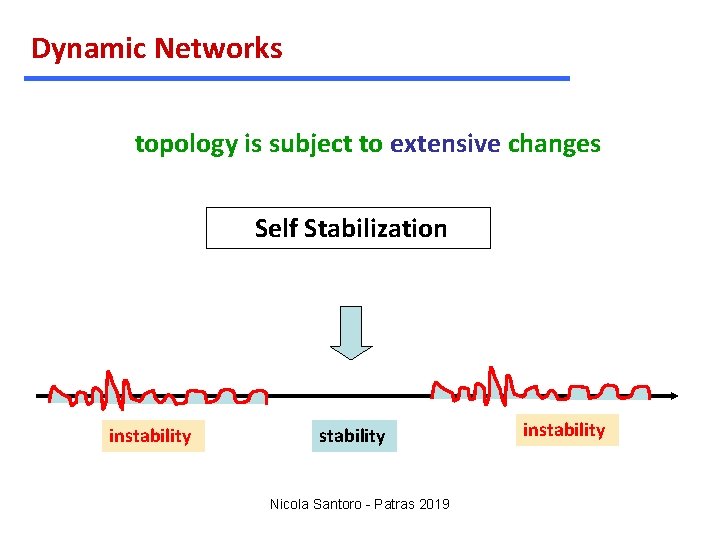
Dynamic Networks topology is subject to extensive changes Self Stabilization instability Nicola Santoro - Patras 2019 instability
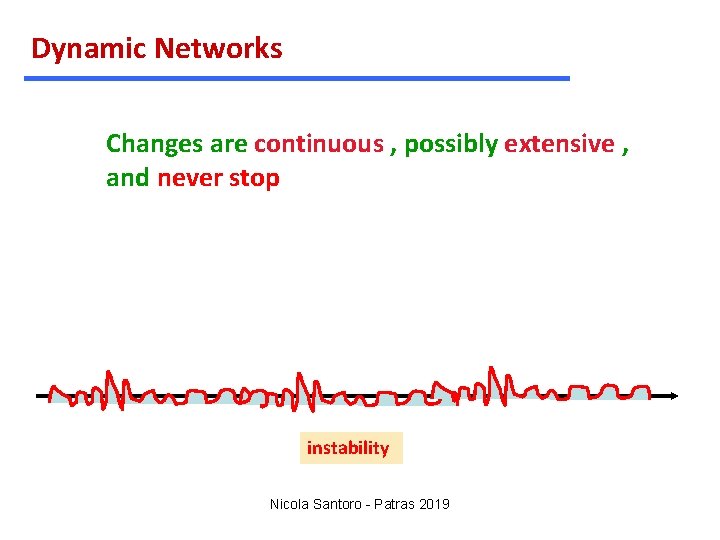
Dynamic Networks Changes are continuous , possibly extensive , and never stop instability Nicola Santoro - Patras 2019
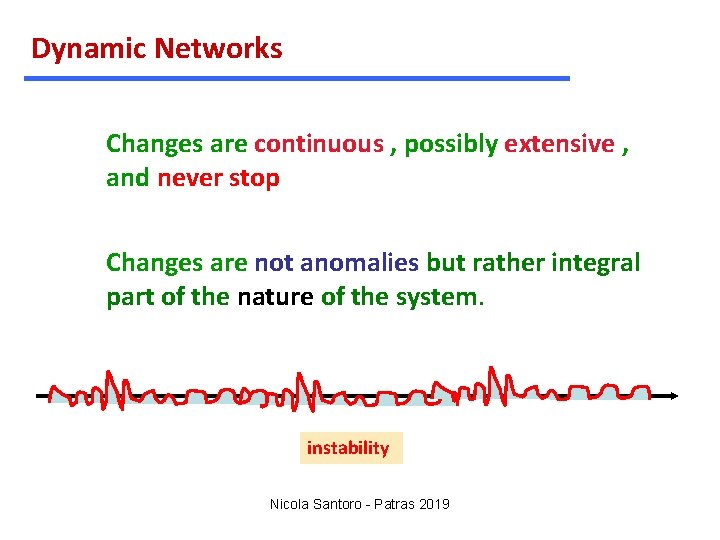
Dynamic Networks Changes are continuous , possibly extensive , and never stop Changes are not anomalies but rather integral part of the nature of the system. instability Nicola Santoro - Patras 2019
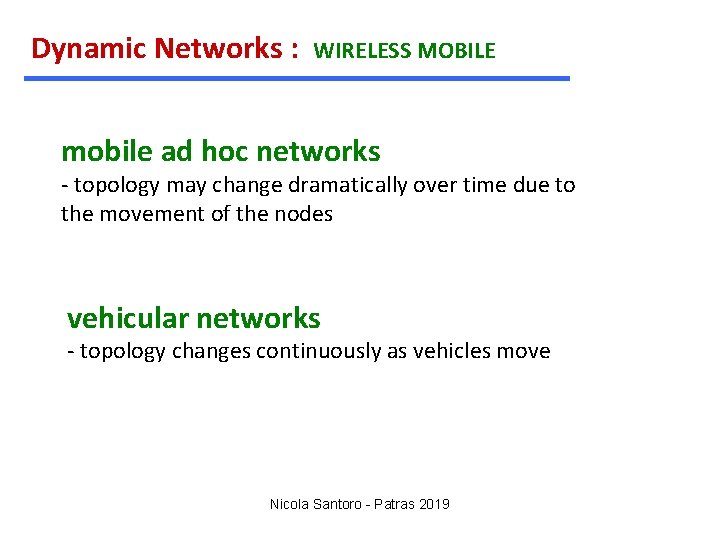
Dynamic Networks : WIRELESS MOBILE mobile ad hoc networks - topology may change dramatically over time due to the movement of the nodes vehicular networks - topology changes continuously as vehicles move Nicola Santoro - Patras 2019
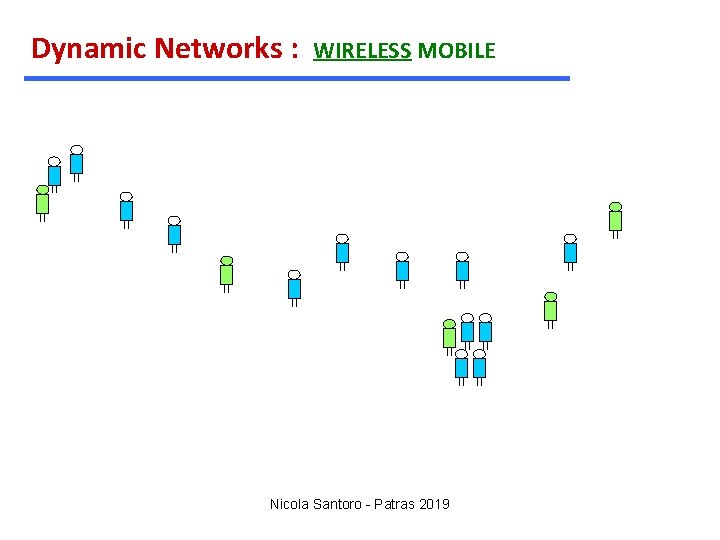
Dynamic Networks : WIRELESS MOBILE Nicola Santoro - Patras 2019
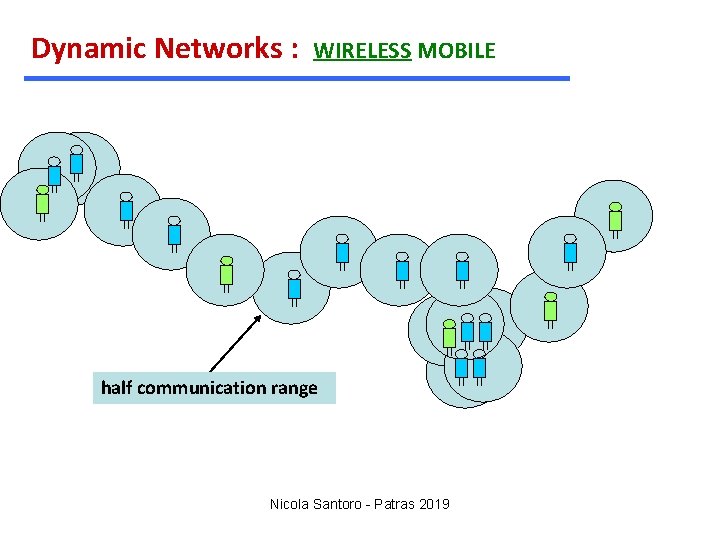
Dynamic Networks : WIRELESS MOBILE half communication range Nicola Santoro - Patras 2019
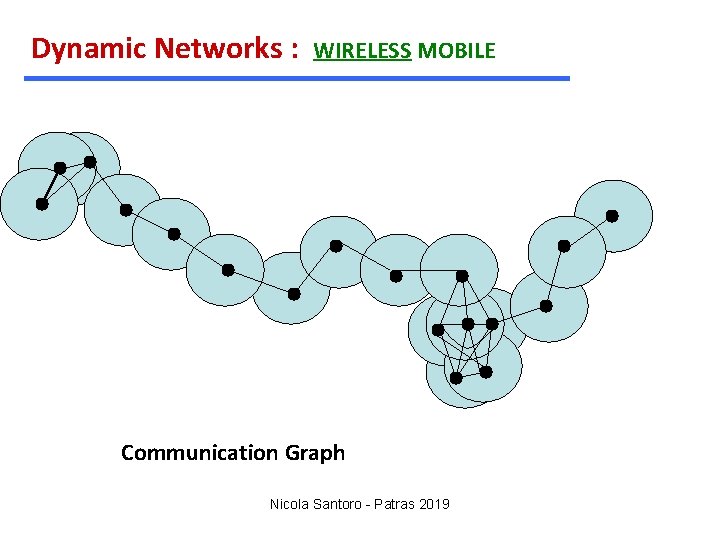
Dynamic Networks : WIRELESS MOBILE Communication Graph Nicola Santoro - Patras 2019
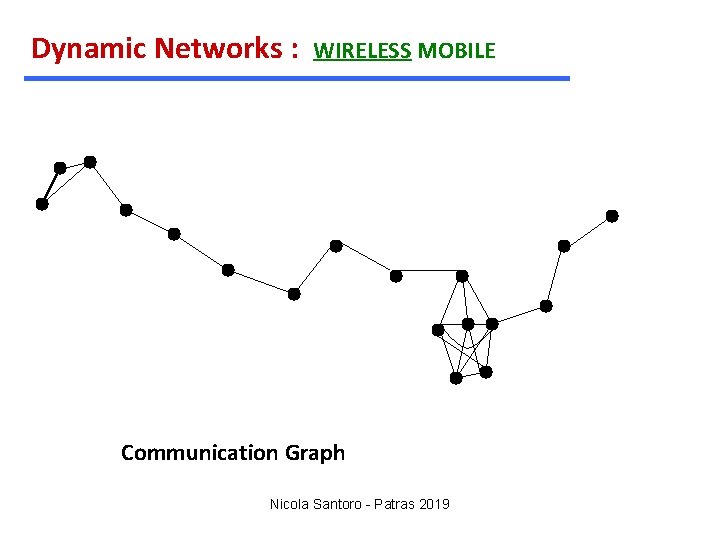
Dynamic Networks : WIRELESS MOBILE Communication Graph Nicola Santoro - Patras 2019
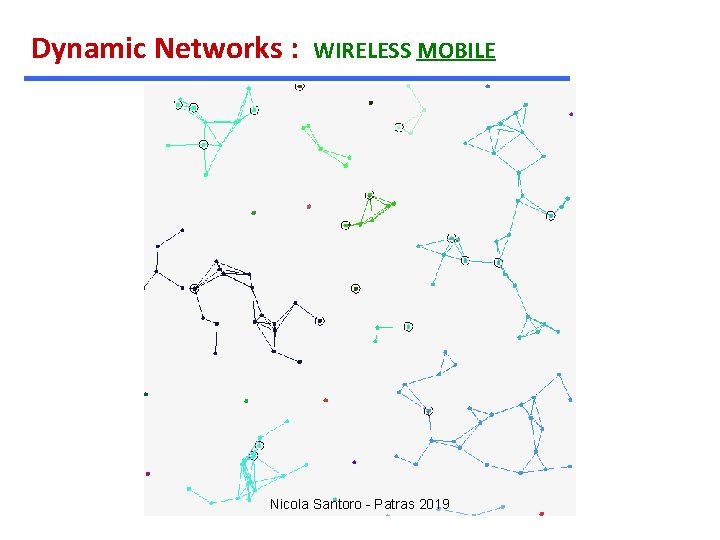
Dynamic Networks : WIRELESS MOBILE Nicola Santoro - Patras 2019
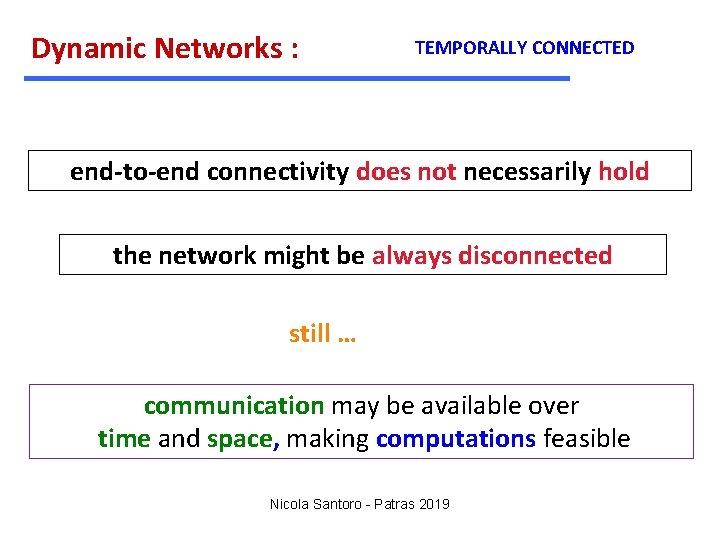
Dynamic Networks : TEMPORALLY CONNECTED end-to-end connectivity does not necessarily hold the network might be always disconnected still … communication may be available over time and space, making computations feasible Nicola Santoro - Patras 2019
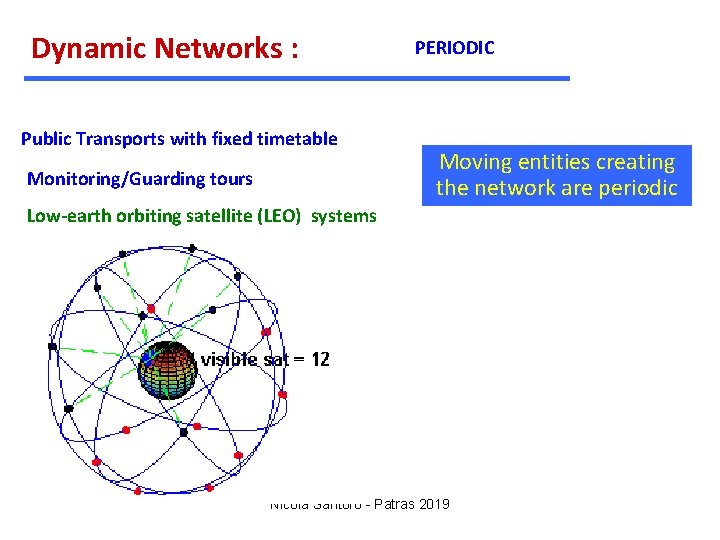
Dynamic Networks : Public Transports with fixed timetable Monitoring/Guarding tours PERIODIC Moving entities creating the network are periodic Low-earth orbiting satellite (LEO) systems NYC Santoro transit -(MTA) Nicola Patras 2019
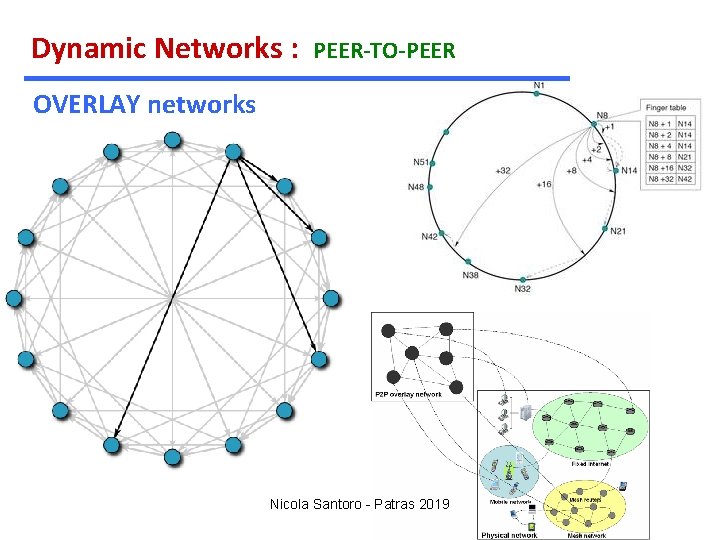
Dynamic Networks : PEER-TO-PEER OVERLAY networks Nicola Santoro - Patras 2019
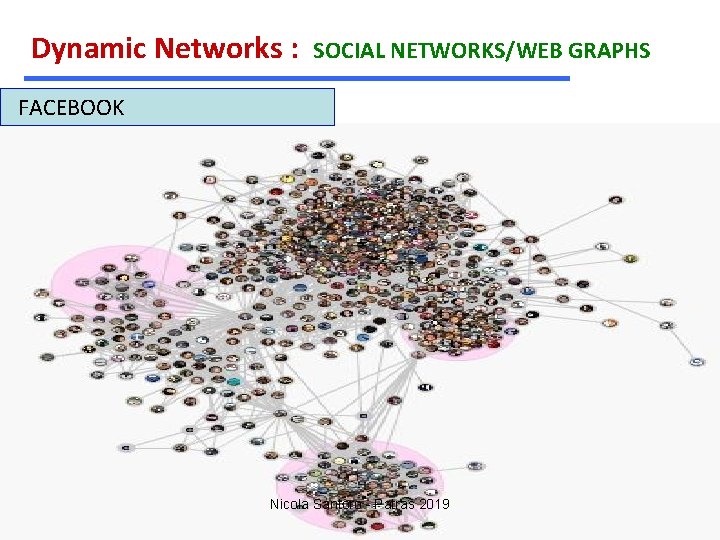
Dynamic Networks : SOCIAL NETWORKS/WEB GRAPHS FACEBOOK Nicola Santoro - Patras 2019
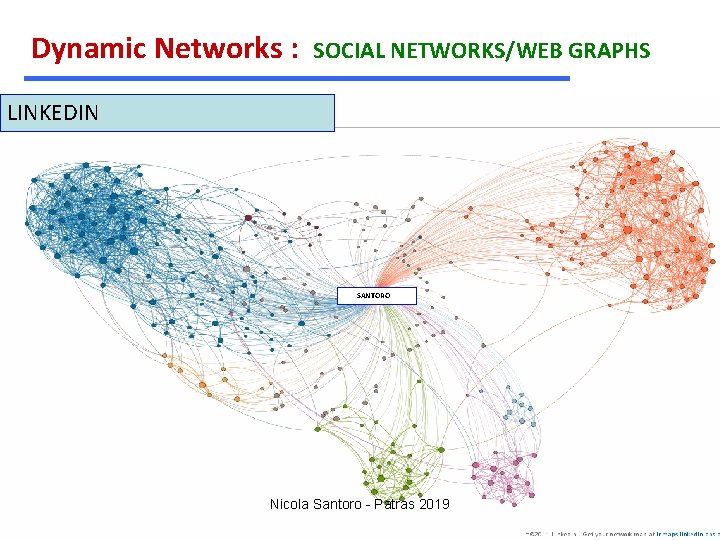
Dynamic Networks : SOCIAL NETWORKS/WEB GRAPHS LINKEDIN SANTORO Nicola Santoro - Patras 2019
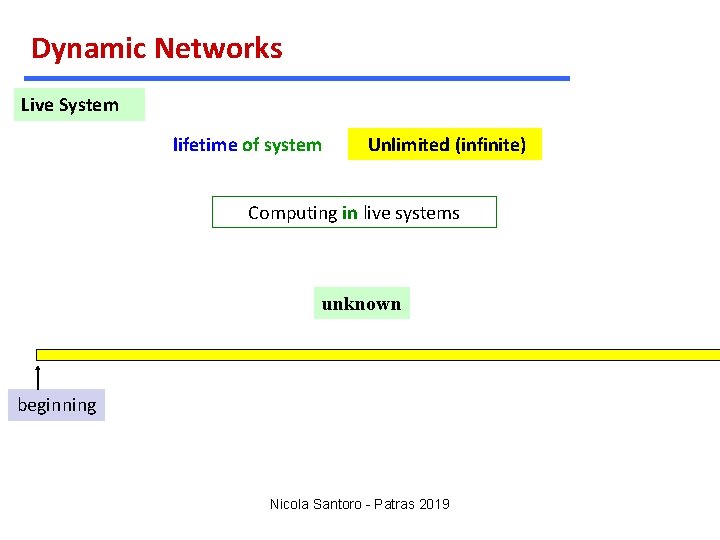
Dynamic Networks Live System lifetime of system Unlimited (infinite) Computing in live systems unknown beginning Nicola Santoro - Patras 2019
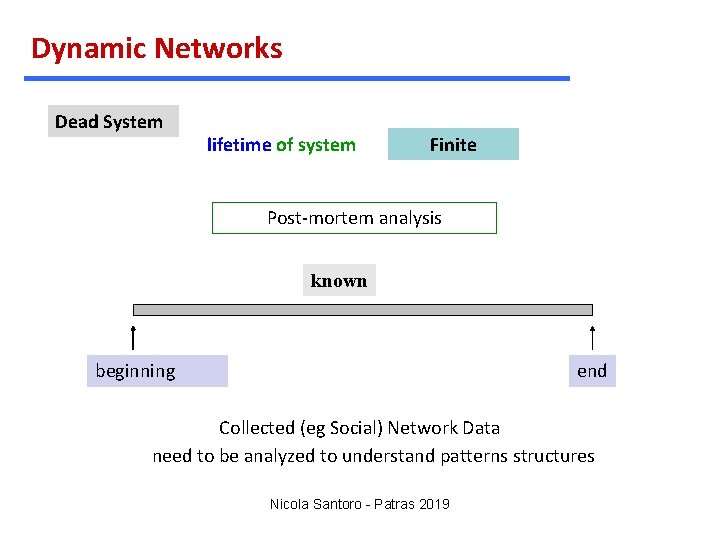
Dynamic Networks Dead System lifetime of system Finite Post-mortem analysis known beginning end Collected (eg Social) Network Data need to be analyzed to understand patterns structures Nicola Santoro - Patras 2019
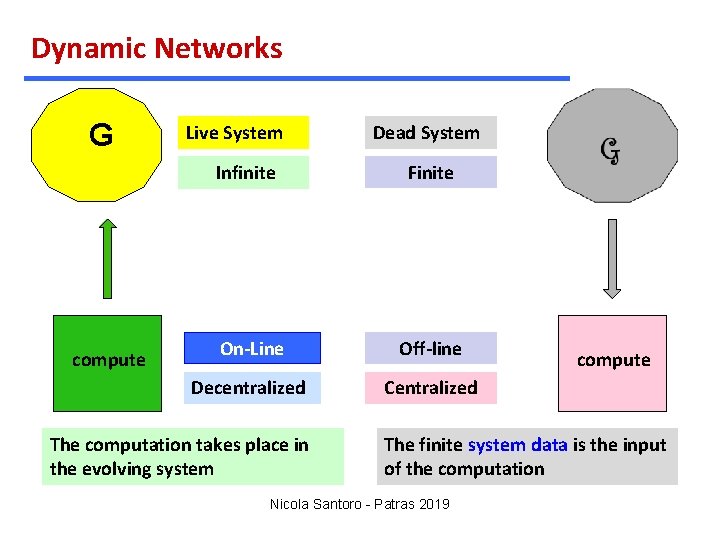
Dynamic Networks G Live System Infinite compute Dead System Finite On-Line Off-line Decentralized Centralized The computation takes place in the evolving system compute The finite system data is the input of the computation Nicola Santoro - Patras 2019
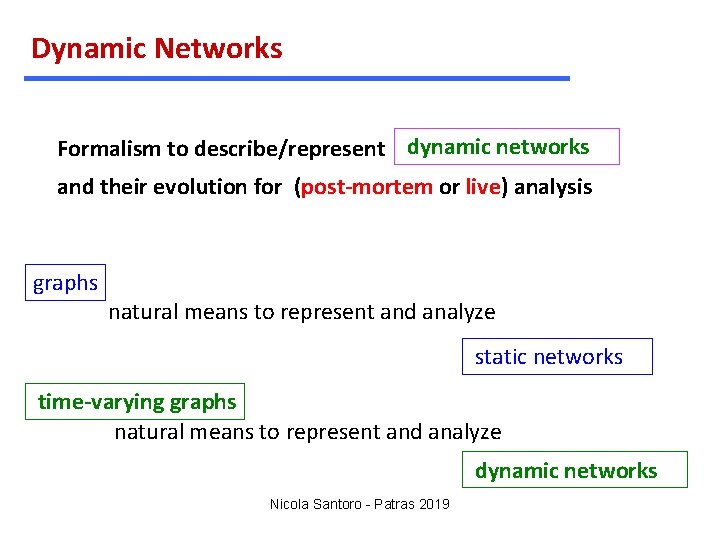
Dynamic Networks Formalism to describe/represent dynamic networks and their evolution for (post-mortem or live) analysis graphs natural means to represent and analyze static networks time-varying graphs natural means to represent and analyze dynamic networks Nicola Santoro - Patras 2019
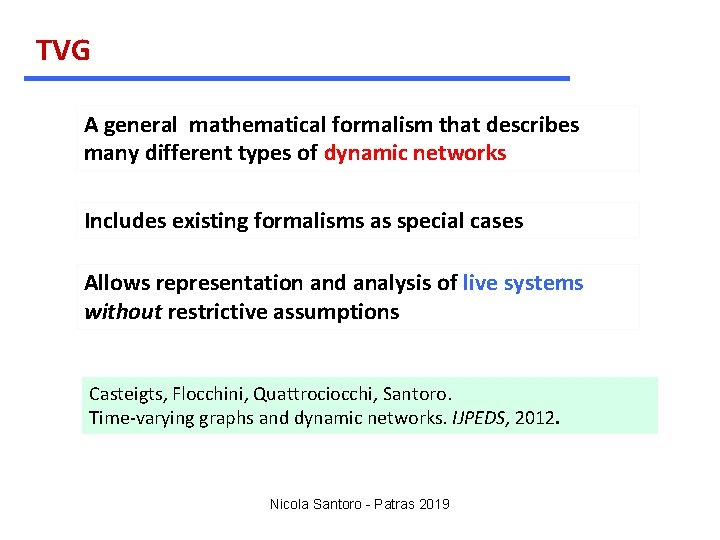
TVG A general mathematical formalism that describes many different types of dynamic networks Includes existing formalisms as special cases Allows representation and analysis of live systems without restrictive assumptions Casteigts, Flocchini, Quattrociocchi, Santoro. Time-varying graphs and dynamic networks. IJPEDS, 2012. Nicola Santoro - Patras 2019
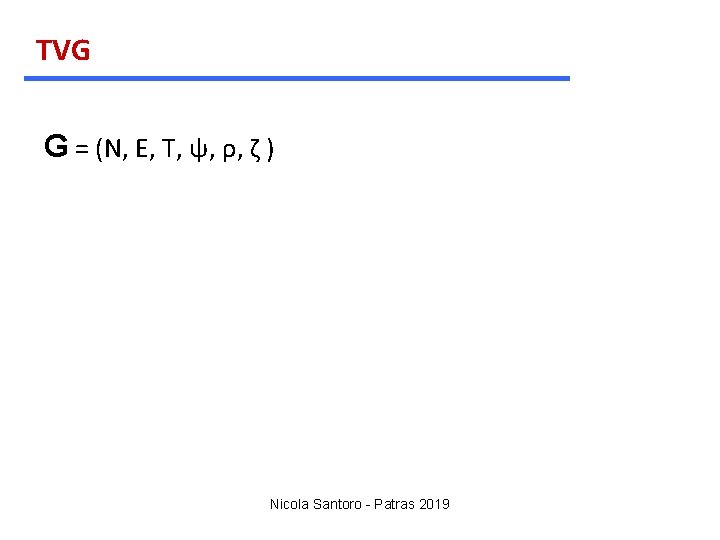
TVG G = (N, E, T, ψ, ρ, ζ ) Nicola Santoro - Patras 2019
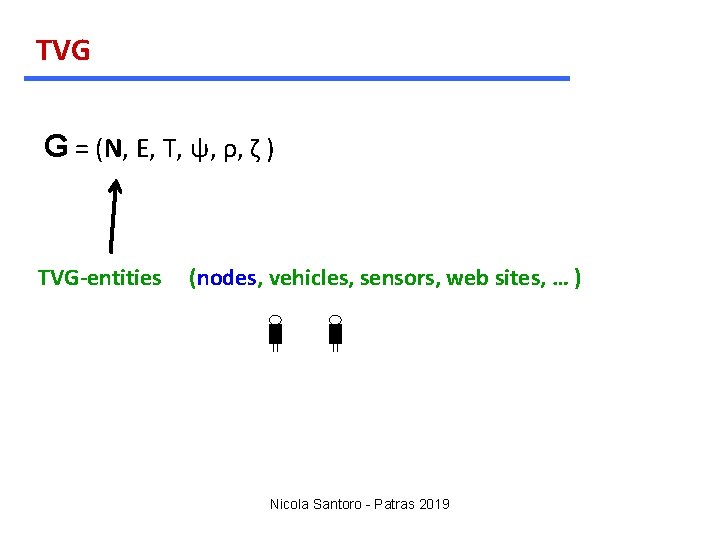
TVG G = (N, E, T, ψ, ρ, ζ ) TVG-entities (nodes, vehicles, sensors, web sites, … ) Nicola Santoro - Patras 2019
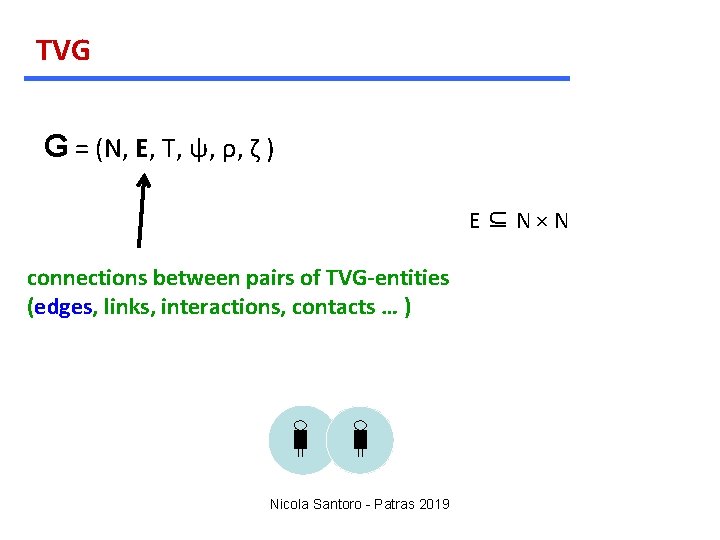
TVG G = (N, E, T, ψ, ρ, ζ ) E⊆N×N connections between pairs of TVG-entities (edges, links, interactions, contacts … ) Nicola Santoro - Patras 2019
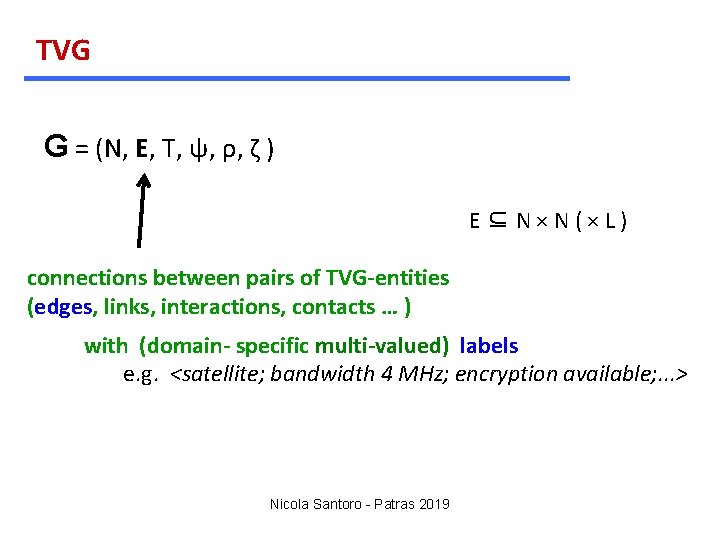
TVG G = (N, E, T, ψ, ρ, ζ ) E⊆N×N(×L) connections between pairs of TVG-entities (edges, links, interactions, contacts … ) with (domain- specific multi-valued) labels e. g. <satellite; bandwidth 4 MHz; encryption available; . . . > Nicola Santoro - Patras 2019
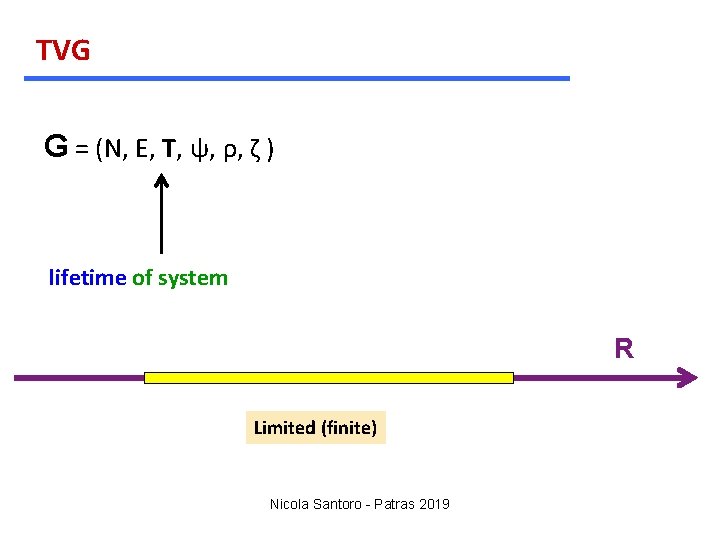
TVG G = (N, E, T, ψ, ρ, ζ ) lifetime of system R Limited (finite) Nicola Santoro - Patras 2019
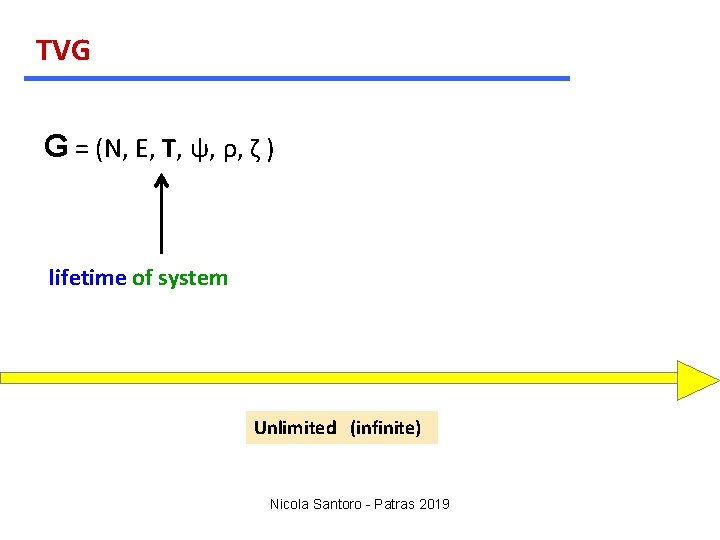
TVG G = (N, E, T, ψ, ρ, ζ ) lifetime of system Unlimited (infinite) Nicola Santoro - Patras 2019
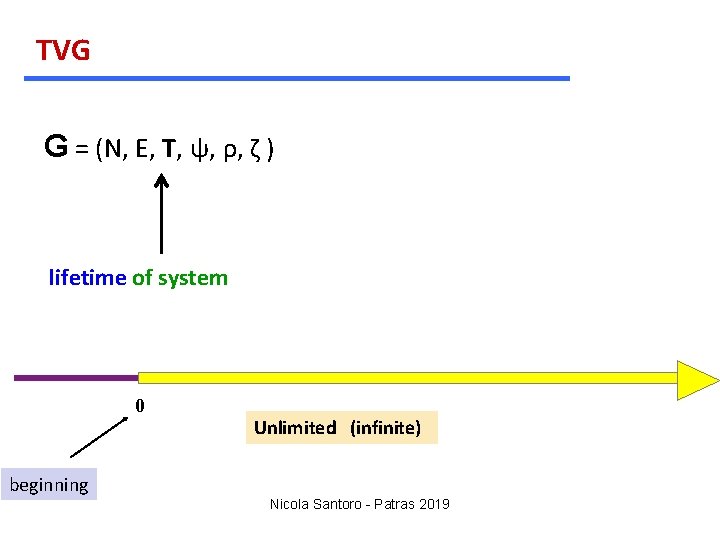
TVG G = (N, E, T, ψ, ρ, ζ ) lifetime of system 0 Unlimited (infinite) beginning Nicola Santoro - Patras 2019
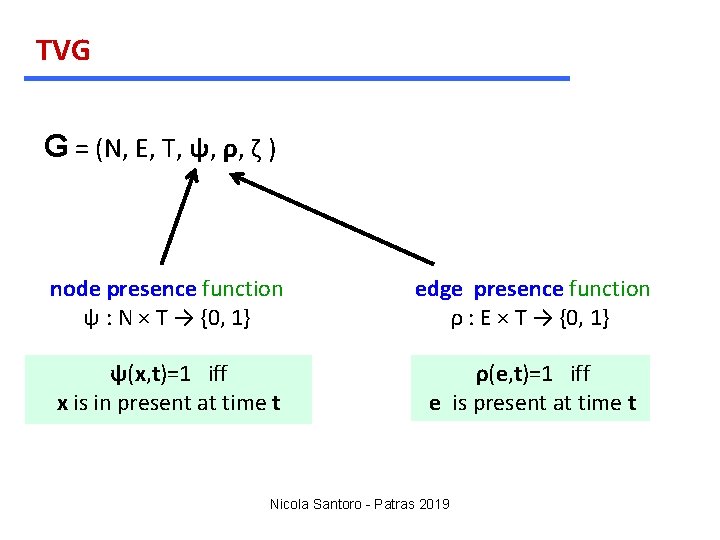
TVG G = (N, E, T, ψ, ρ, ζ ) node presence function ψ : N × T → {0, 1} edge presence function ρ : E × T → {0, 1} ψ(x, t)=1 iff x is in present at time t ρ(e, t)=1 iff e is present at time t Nicola Santoro - Patras 2019
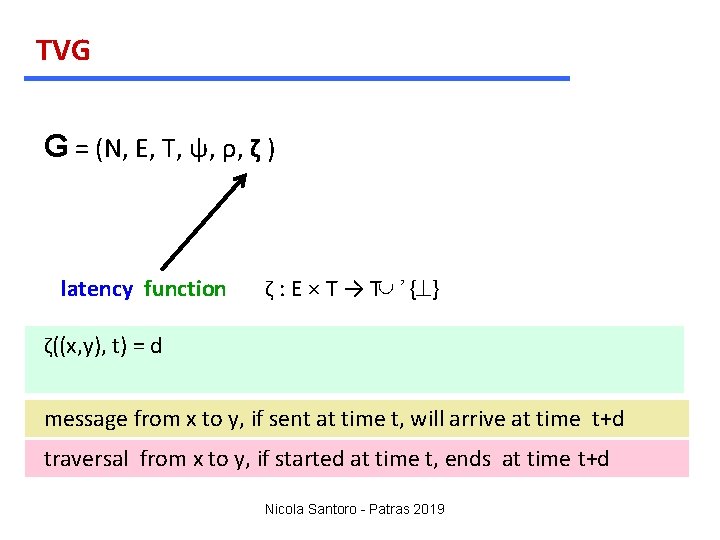
TVG G = (N, E, T, ψ, ρ, ζ ) ζ: E×T→T ˛Ç latency function {^} ζ((x, y), t) = d message from x to y, if sent at time t, will arrive at time t+d traversal from x to y, if started at time t, ends at time t+d Nicola Santoro - Patras 2019
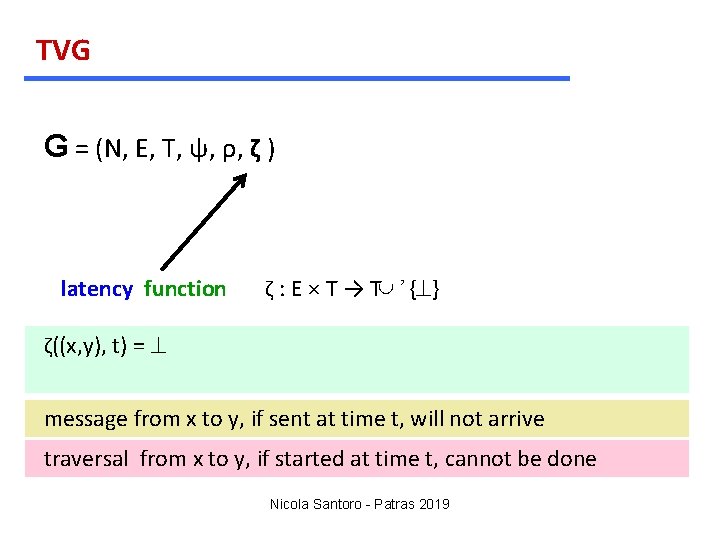
TVG G = (N, E, T, ψ, ρ, ζ ) ζ: E×T→T ˛Ç latency function {^} ζ((x, y), t) = ^ message from x to y, if sent at time t, will not arrive traversal from x to y, if started at time t, cannot be done Nicola Santoro - Patras 2019
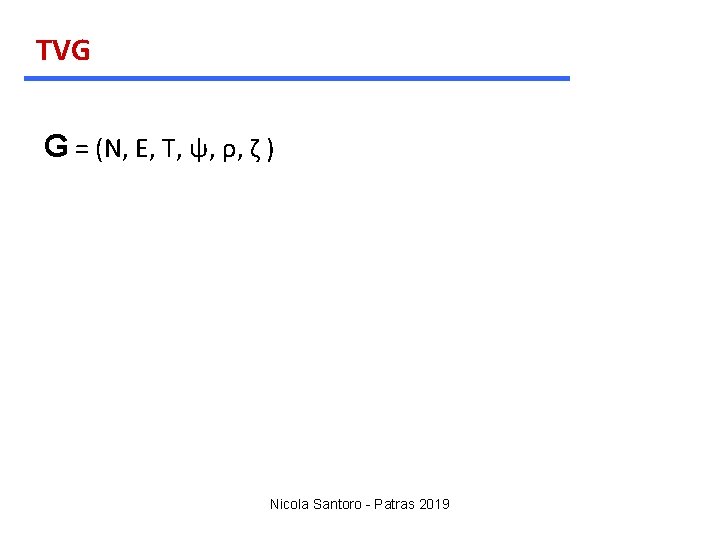
TVG G = (N, E, T, ψ, ρ, ζ ) Nicola Santoro - Patras 2019
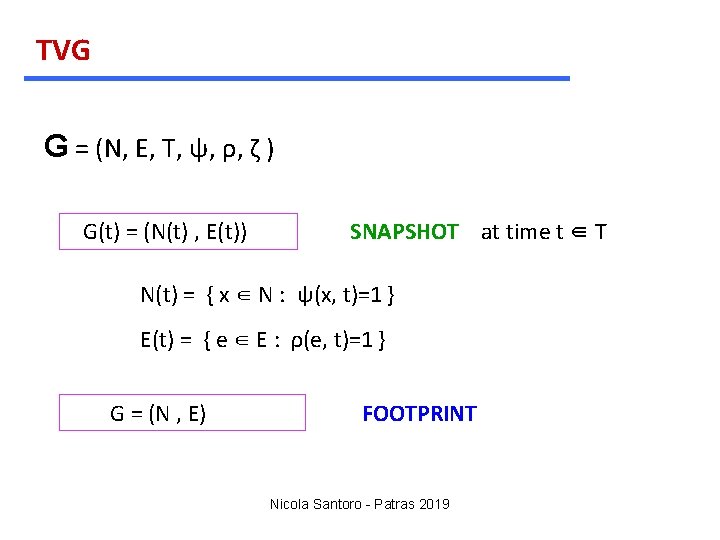
TVG G = (N, E, T, ψ, ρ, ζ ) G(t) = (N(t) , E(t)) SNAPSHOT at time t ∈ T N(t) = { x ∈ N : ψ(x, t)=1 } E(t) = { e ∈ E : ρ(e, t)=1 } G = (N , E) FOOTPRINT Nicola Santoro - Patras 2019
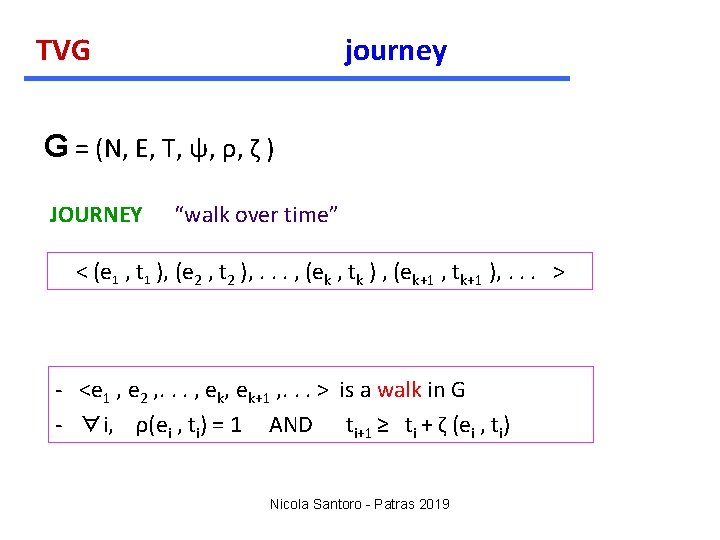
TVG journey G = (N, E, T, ψ, ρ, ζ ) JOURNEY “walk over time” < (e 1 , t 1 ), (e 2 , t 2 ), . . . , (ek , tk ) , (ek+1 , tk+1 ), . . . > - <e 1 , e 2 , . . . , ek+1 , . . . > is a walk in G - ∀i, ρ(ei , ti) = 1 AND ti+1 ≥ ti + ζ (ei , ti) Nicola Santoro - Patras 2019
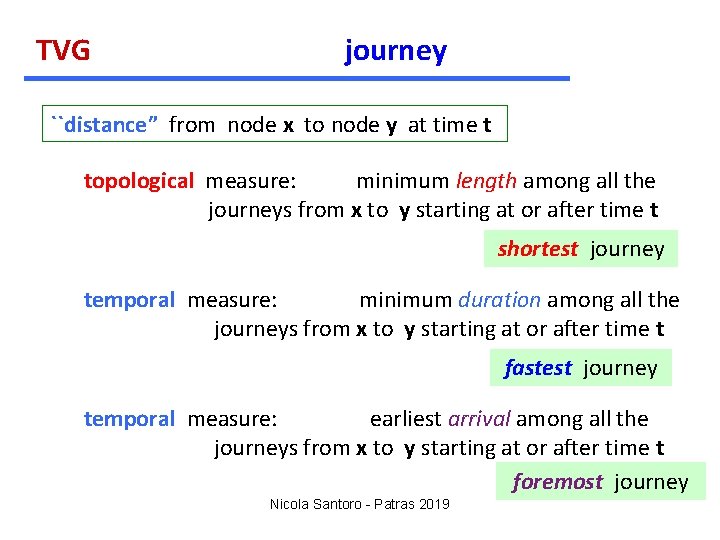
TVG journey ``distance” from node x to node y at time t topological measure: minimum length among all the journeys from x to y starting at or after time t shortest journey temporal measure: minimum duration among all the journeys from x to y starting at or after time t fastest journey temporal measure: earliest arrival among all the journeys from x to y starting at or after time t foremost journey Nicola Santoro - Patras 2019
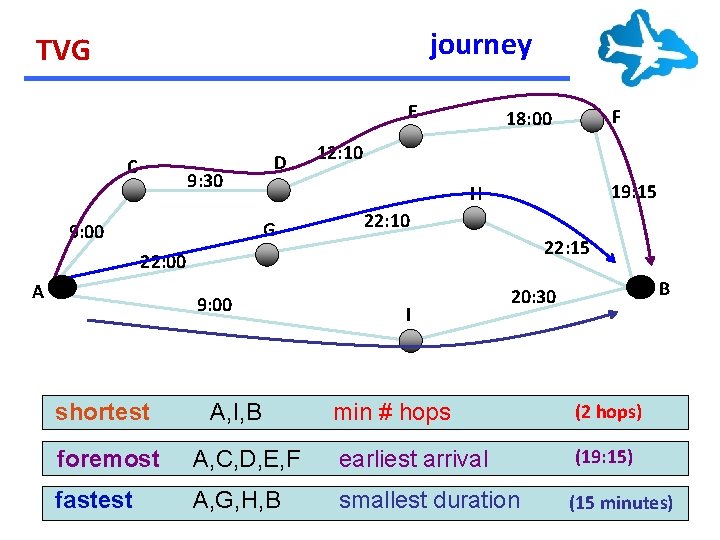
journey TVG E C 9: 30 D 12: 10 19: 15 H G 9: 00 22: 15 22: 00 A 9: 00 shortest F 18: 00 A, I, B I B 20: 30 min # hops (2 hops) earliest arrival (19: 15) foremost A, C, D, E, F fastest A, G, H, BNicola. Santoro smallest duration - Patras 2019 (15 minutes)
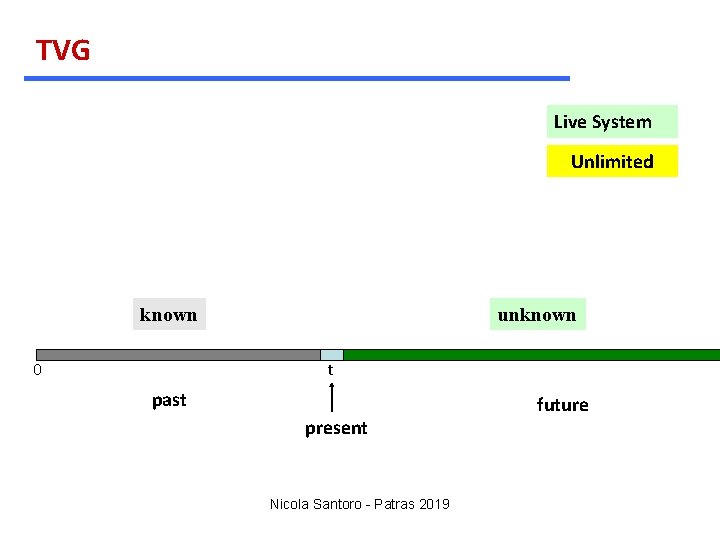
TVG Live System Unlimited known 0 unknown t past present Nicola Santoro - Patras 2019 future
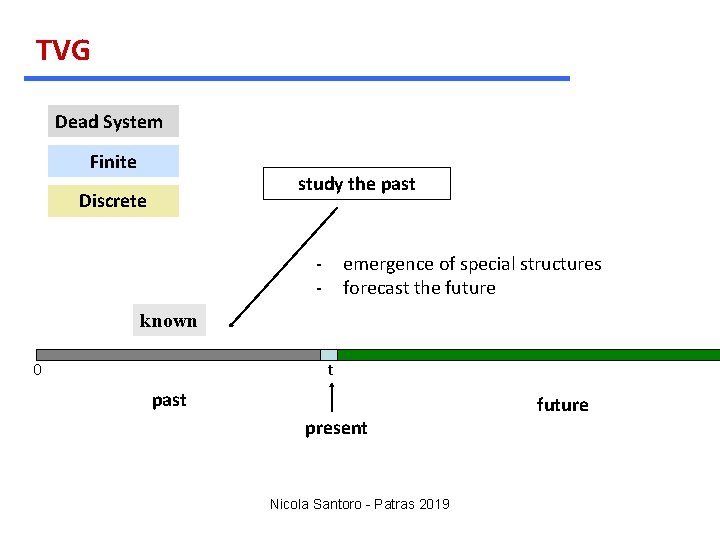
TVG Dead System Finite study the past Discrete - emergence of special structures forecast the future known 0 t past present Nicola Santoro - Patras 2019 future
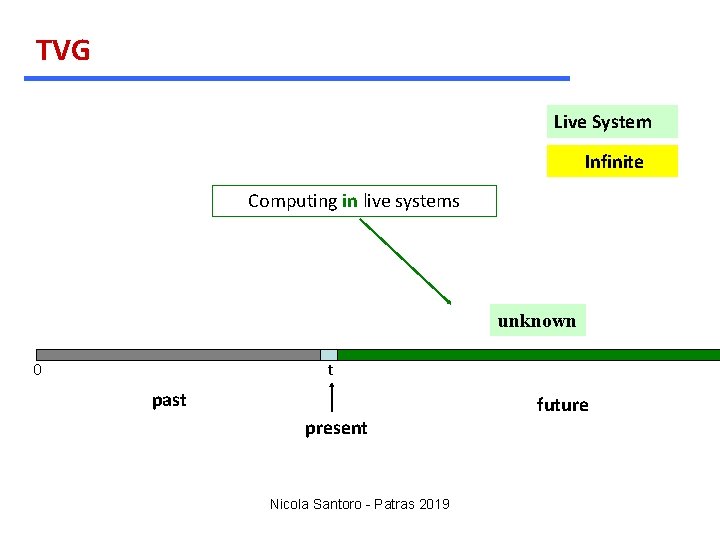
TVG Live System Infinite Computing in live systems unknown 0 t past present Nicola Santoro - Patras 2019 future
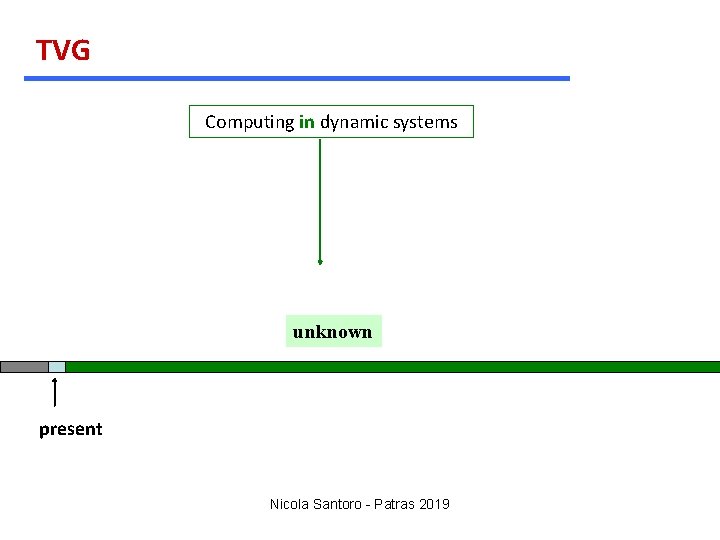
TVG Computing in dynamic systems unknown present Nicola Santoro - Patras 2019
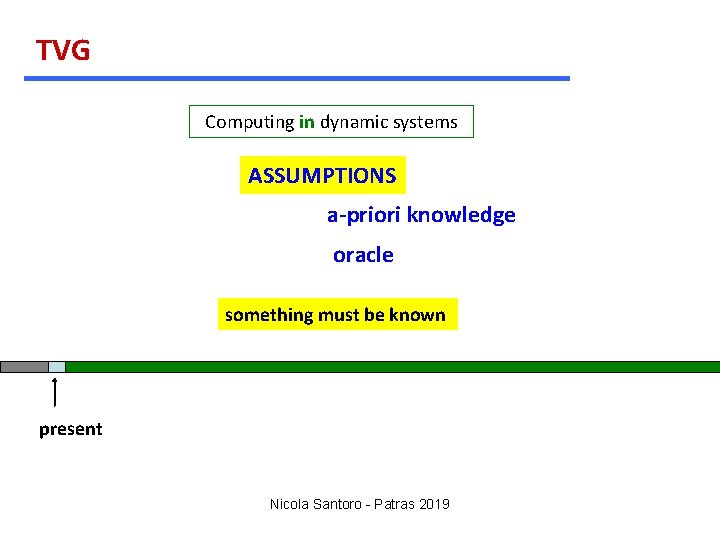
TVG Computing in dynamic systems ASSUMPTIONS a-priori knowledge oracle something must be known present Nicola Santoro - Patras 2019
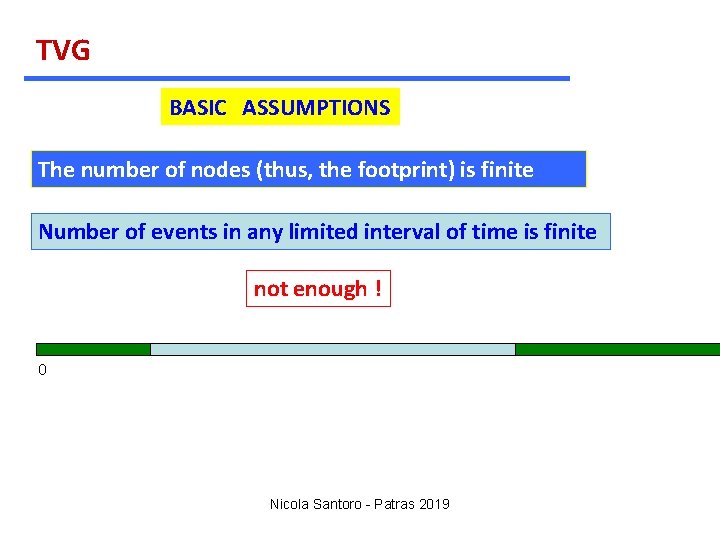
TVG BASIC ASSUMPTIONS The number of nodes (thus, the footprint) is finite Number of events in any limited interval of time is finite not enough ! 0 Nicola Santoro - Patras 2019
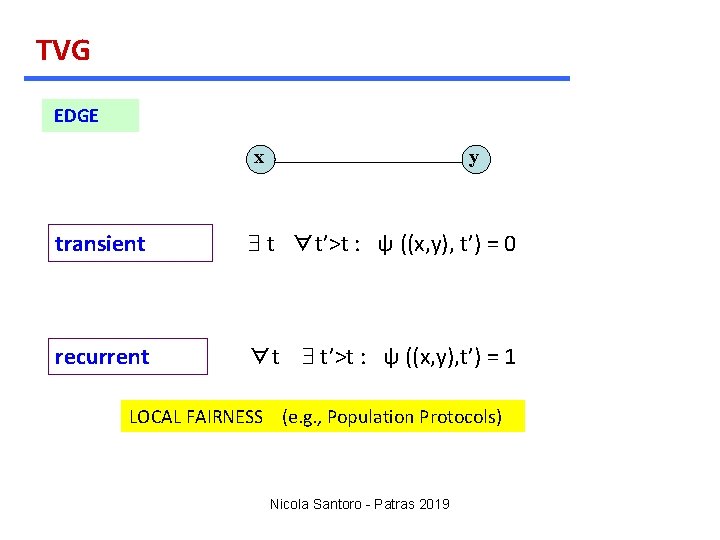
TVG EDGE x y transient $ t ∀t’>t : ψ ((x, y), t’) = 0 recurrent ∀t $ t’>t : ψ ((x, y), t’) = 1 LOCAL FAIRNESS (e. g. , Population Protocols) Nicola Santoro - Patras 2019
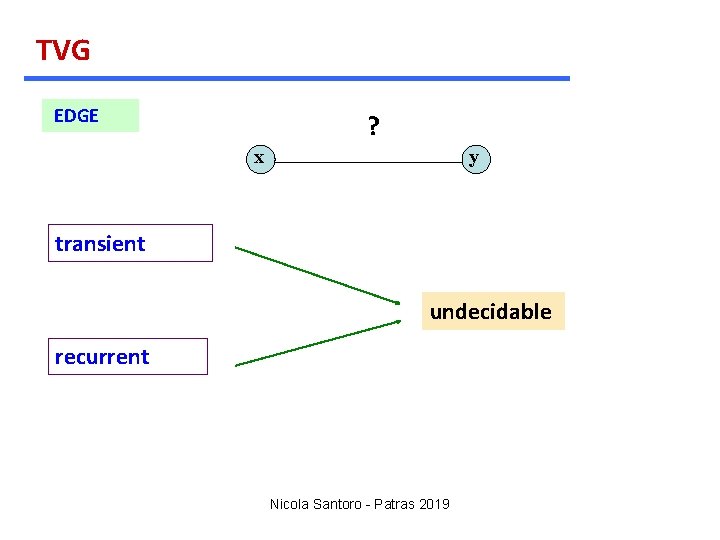
TVG EDGE ? x y transient undecidable recurrent Nicola Santoro - Patras 2019
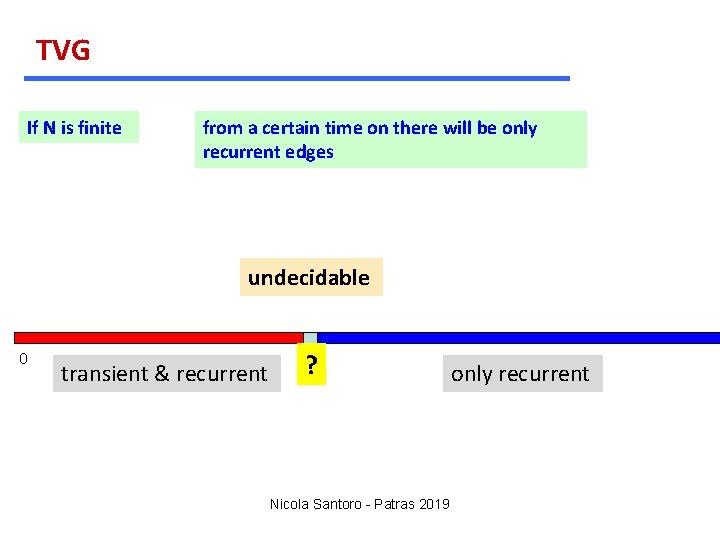
TVG If N is finite from a certain time on there will be only recurrent edges undecidable 0 transient & recurrent ? t Nicola Santoro - Patras 2019 only recurrent
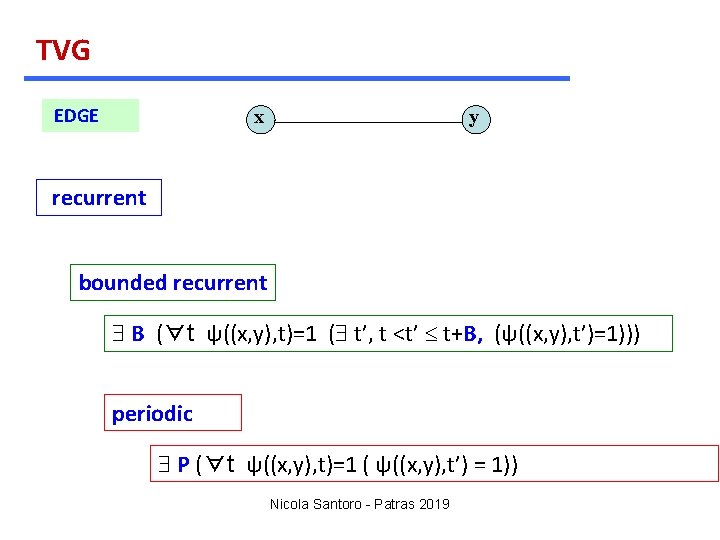
TVG EDGE x y recurrent bounded recurrent $ B (∀t ψ((x, y), t)=1 ($ t’, t <t’ £ t+B, (ψ((x, y), t’)=1))) periodic $ P (∀t ψ((x, y), t)=1 ( ψ((x, y), t’) = 1)) Nicola Santoro - Patras 2019
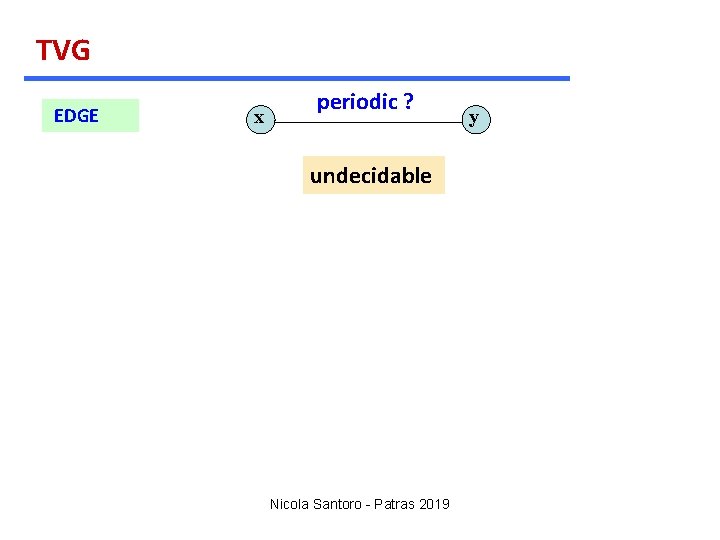
TVG EDGE x periodic ? undecidable Nicola Santoro - Patras 2019 y
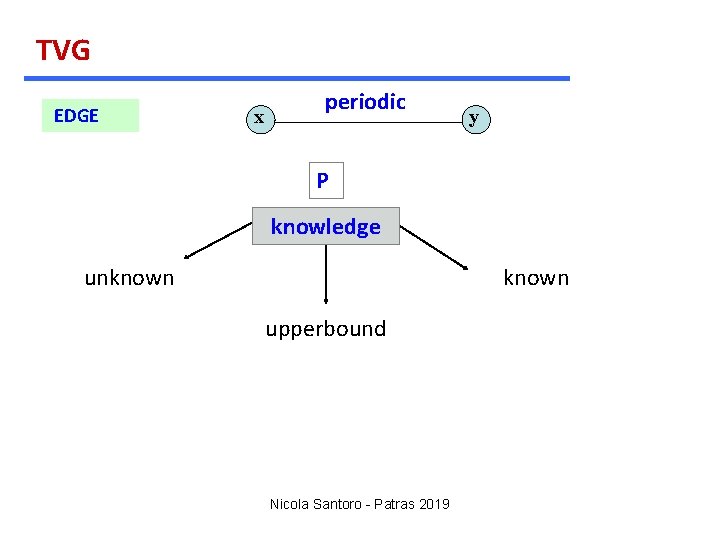
TVG EDGE x periodic y P knowledge unknown upperbound Nicola Santoro - Patras 2019
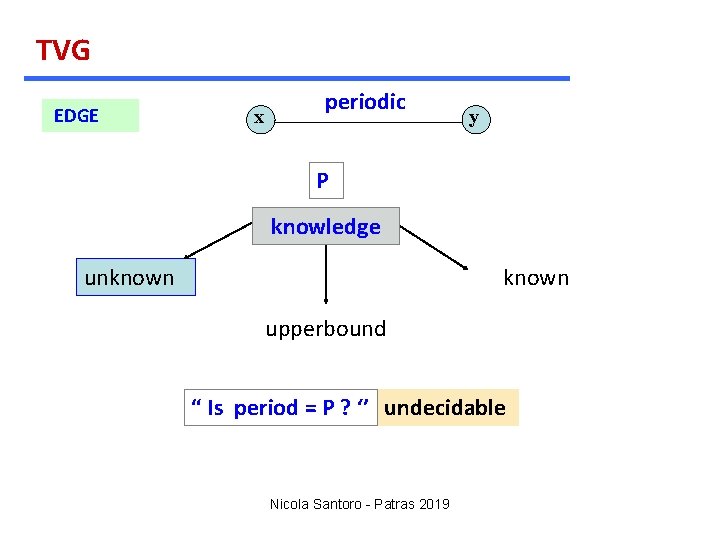
TVG EDGE x periodic y P knowledge unknown upperbound “ Is period = P ? ‘’ undecidable Nicola Santoro - Patras 2019
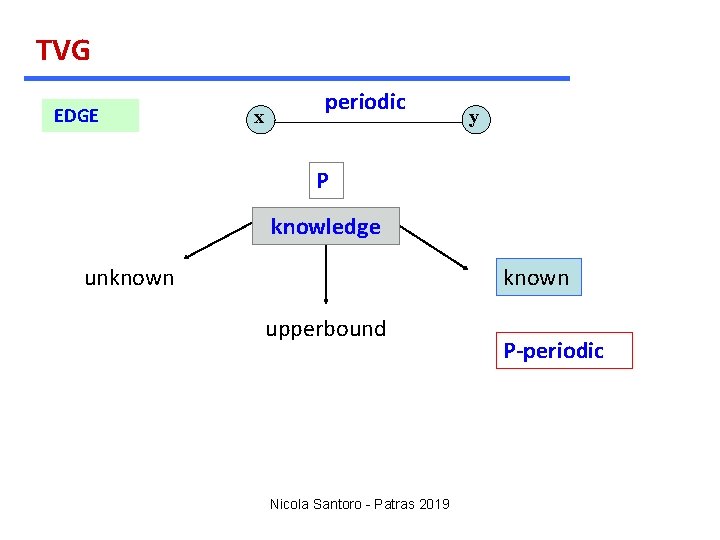
TVG EDGE x periodic y P knowledge unknown upperbound Nicola Santoro - Patras 2019 P-periodic
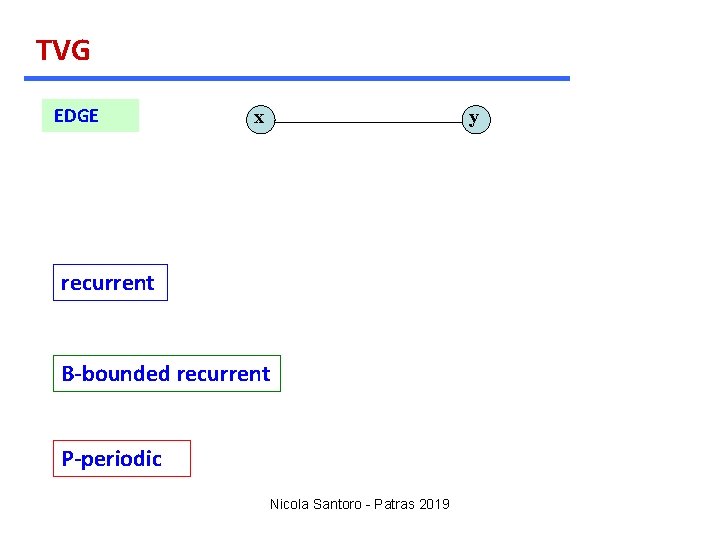
TVG EDGE x y recurrent B-bounded recurrent P-periodic Nicola Santoro - Patras 2019
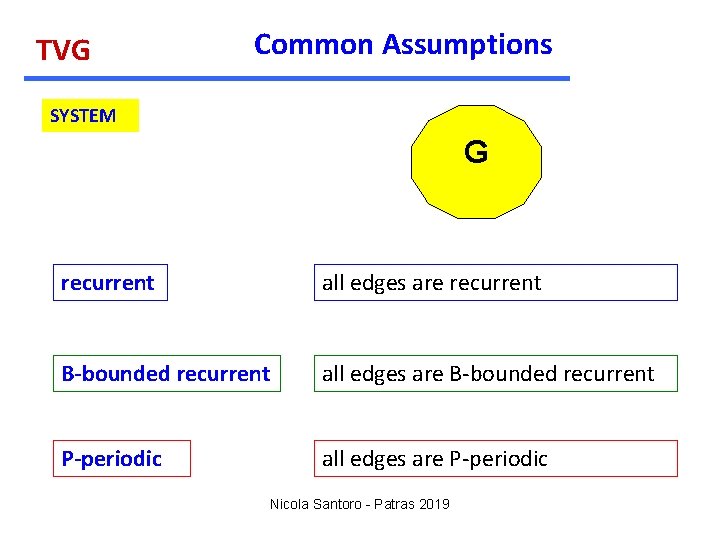
TVG Common Assumptions SYSTEM G recurrent all edges are recurrent B-bounded recurrent all edges are B-bounded recurrent P-periodic all edges are P-periodic Nicola Santoro - Patras 2019
![TVG SYSTEMS CLASSES PROBLEMS computational power Grecurrent Precurrent Bbounded recurrent Gbounded Pperiodic Gperiodic TVG SYSTEMS CLASSES PROBLEMS computational power G[recurrent P[recurrent] ] B-bounded recurrent G[bounded] P-periodic G[periodic]](https://slidetodoc.com/presentation_image_h/20f0eb14b4e952b4479cc35f50d28925/image-56.jpg)
TVG SYSTEMS CLASSES PROBLEMS computational power G[recurrent P[recurrent] ] B-bounded recurrent G[bounded] P-periodic G[periodic] P[periodic] Nicola Santoro - Patras 2019
![TVG SYSTEMS PROBLEMS Foremost Bcast Shortest Bcast Fastest Bcast BROADCAST recurrent Precurrent TVG SYSTEMS PROBLEMS Foremost. Bcast ≺ Shortest. Bcast ≺ Fastest. Bcast BROADCAST recurrent P[recurrent]](https://slidetodoc.com/presentation_image_h/20f0eb14b4e952b4479cc35f50d28925/image-57.jpg)
TVG SYSTEMS PROBLEMS Foremost. Bcast ≺ Shortest. Bcast ≺ Fastest. Bcast BROADCAST recurrent P[recurrent] B-bounded recurrent P[bounded] P-periodic P[periodic] Nicola Santoro - Patras 2019
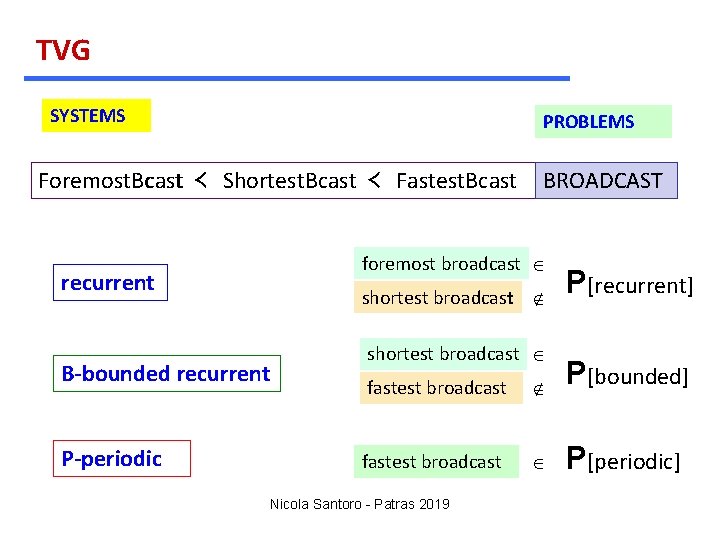
TVG SYSTEMS PROBLEMS Foremost. Bcast ≺ Shortest. Bcast ≺ Fastest. Bcast foremost broadcast Î recurrent shortest broadcast Ï B-bounded recurrent P-periodic BROADCAST shortest broadcast Î P[recurrent] fastest broadcast Ï P[bounded] fastest broadcast Î P[periodic] Nicola Santoro - Patras 2019
![TVG Foremost Bcast Shortest Bcast Fastest Bcast Pperiodic periodic Í Pbounded Í TVG Foremost. Bcast ≺ Shortest. Bcast ≺ Fastest. Bcast P[periodic] periodic Í/ P[bounded] Í/](https://slidetodoc.com/presentation_image_h/20f0eb14b4e952b4479cc35f50d28925/image-59.jpg)
TVG Foremost. Bcast ≺ Shortest. Bcast ≺ Fastest. Bcast P[periodic] periodic Í/ P[bounded] Í/ P[recurrent] ‹ bounded ‹ recurrent PROBLEMS SYSTEMS A. Casteigts, P. Flocchini, B. Mans, N. Santoro. Measuring temporal lags in delaytolerant networks. IEEE Trans. on Computers, 2014 A. Casteigts, P. Flocchini, B. Mans, N. Santoro. Shortest, fastest, and foremost broadcast in dynamic networks. J. Found. Comp. Nicola. Int. Santoro - Patras 2019 Sci, 2015
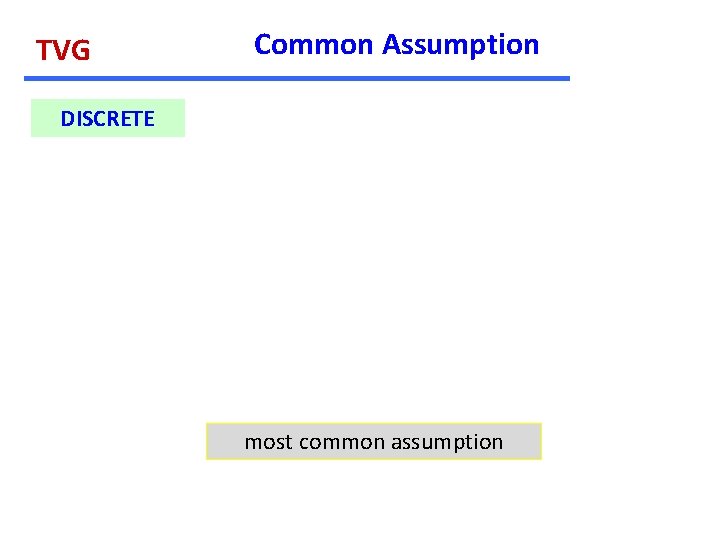
TVG Common Assumption DISCRETE most common assumption
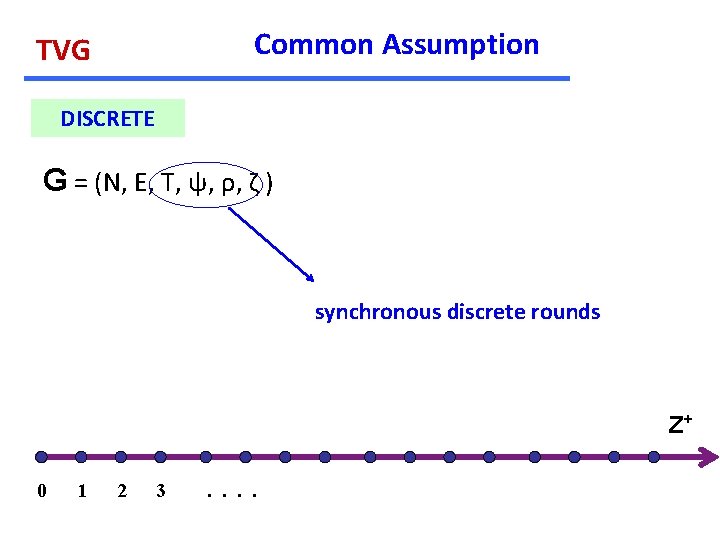
Common Assumption TVG DISCRETE G = (N, E, T, ψ, ρ, ζ ) synchronous discrete rounds Z+ 0 1 2 3 . .
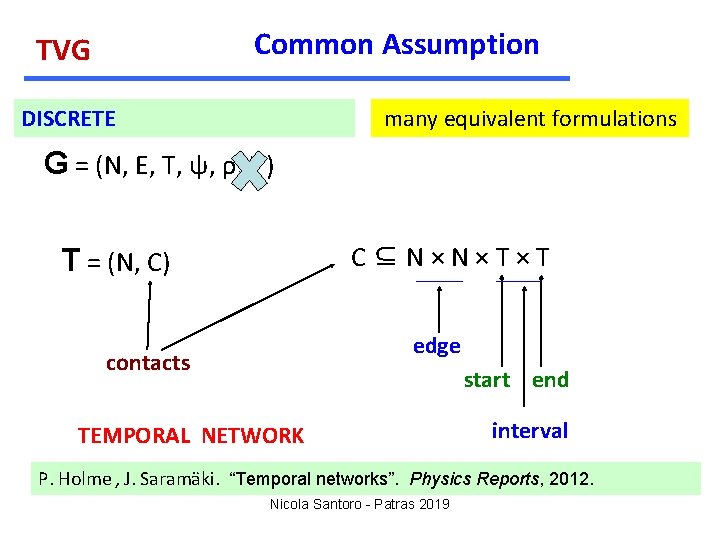
Common Assumption TVG DISCRETE many equivalent formulations G = (N, E, T, ψ, ρ, ζ ) C⊆N×N×T×T T = (N, C) edge contacts start end TEMPORAL NETWORK interval P. Holme , J. Saramäki. “Temporal networks”. Physics Reports, 2012. Nicola Santoro - Patras 2019
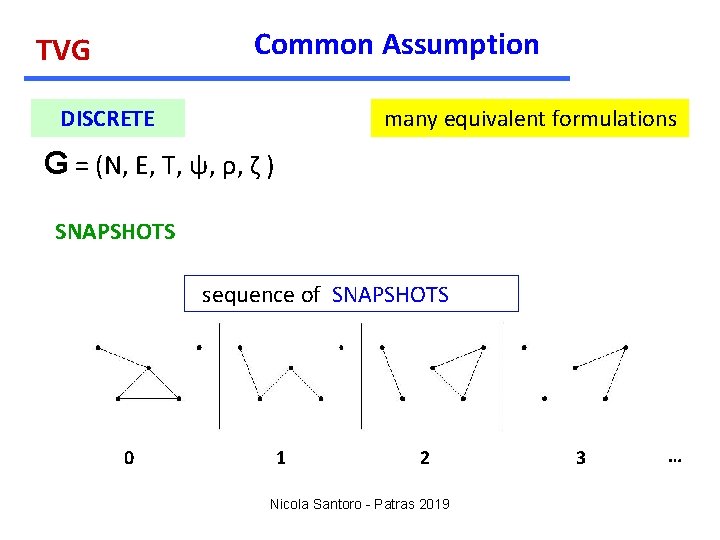
Common Assumption TVG DISCRETE many equivalent formulations G = (N, E, T, ψ, ρ, ζ ) SNAPSHOTS sequence of SNAPSHOTS 0 1 2 Nicola Santoro - Patras 2019 3 …
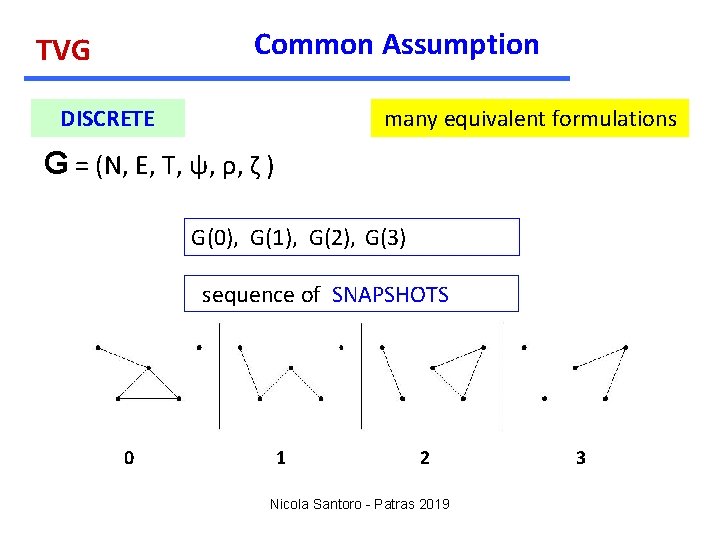
Common Assumption TVG DISCRETE many equivalent formulations G = (N, E, T, ψ, ρ, ζ ) G(0), G(1), G(2), G(3) sequence of SNAPSHOTS 0 1 2 Nicola Santoro - Patras 2019 3
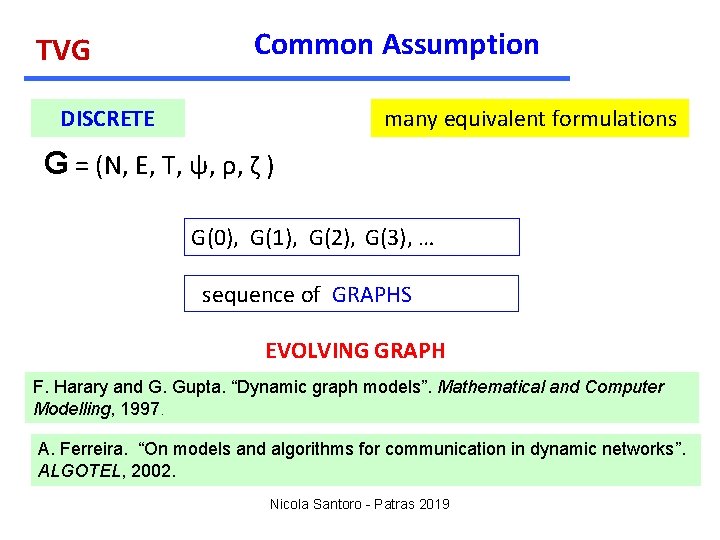
TVG Common Assumption DISCRETE many equivalent formulations G = (N, E, T, ψ, ρ, ζ ) G(0), G(1), G(2), G(3), … sequence of GRAPHS EVOLVING GRAPH F. Harary and G. Gupta. “Dynamic graph models”. Mathematical and Computer Modelling, 1997. A. Ferreira. “On models and algorithms for communication in dynamic networks”. ALGOTEL, 2002. Nicola Santoro - Patras 2019
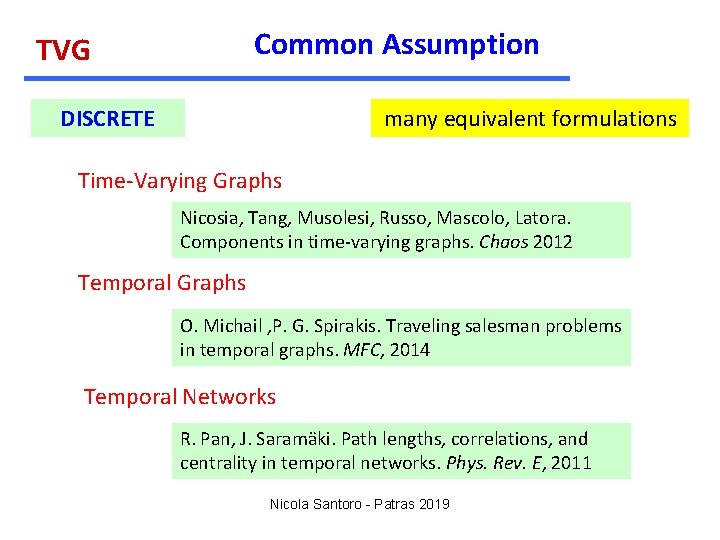
Common Assumption TVG DISCRETE many equivalent formulations Time-Varying Graphs Nicosia, Tang, Musolesi, Russo, Mascolo, Latora. Components in time-varying graphs. Chaos 2012 Temporal Graphs O. Michail , P. G. Spirakis. Traveling salesman problems in temporal graphs. MFC, 2014 Temporal Networks R. Pan, J. Sarama ki. Path lengths, correlations, and centrality in temporal networks. Phys. Rev. E, 2011 Nicola Santoro - Patras 2019
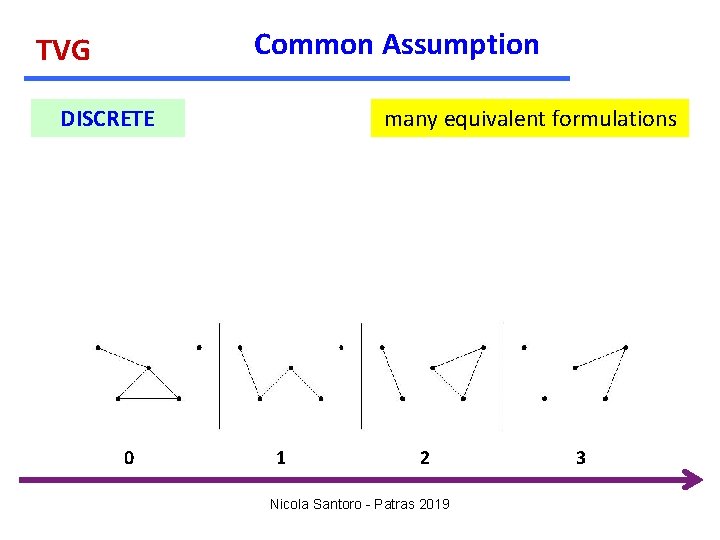
Common Assumption TVG DISCRETE 0 many equivalent formulations 1 2 Nicola Santoro - Patras 2019 3
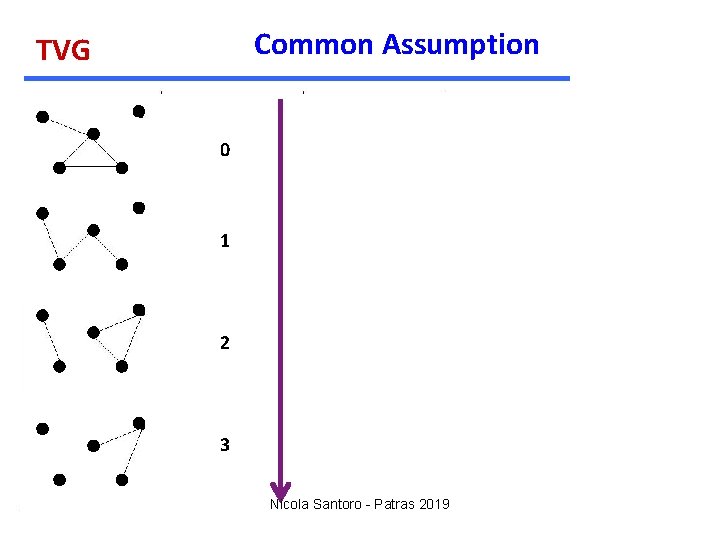
Common Assumption TVG 0 1 2 3 Nicola Santoro - Patras 2019
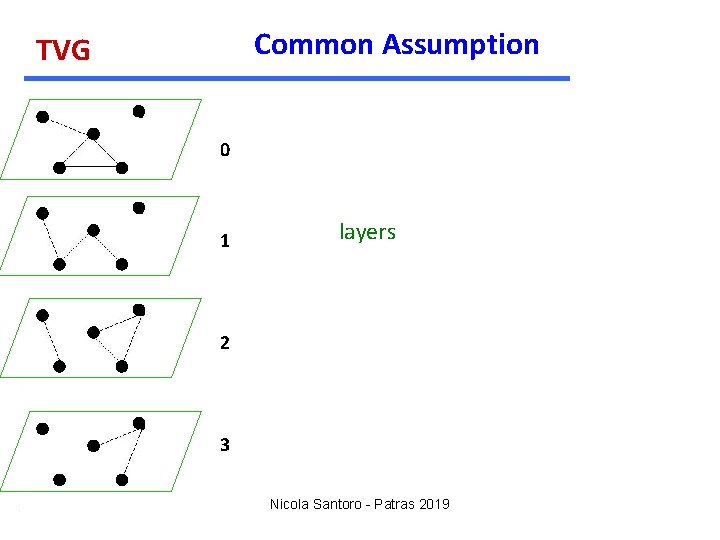
Common Assumption TVG 0 1 layers 2 3 Nicola Santoro - Patras 2019
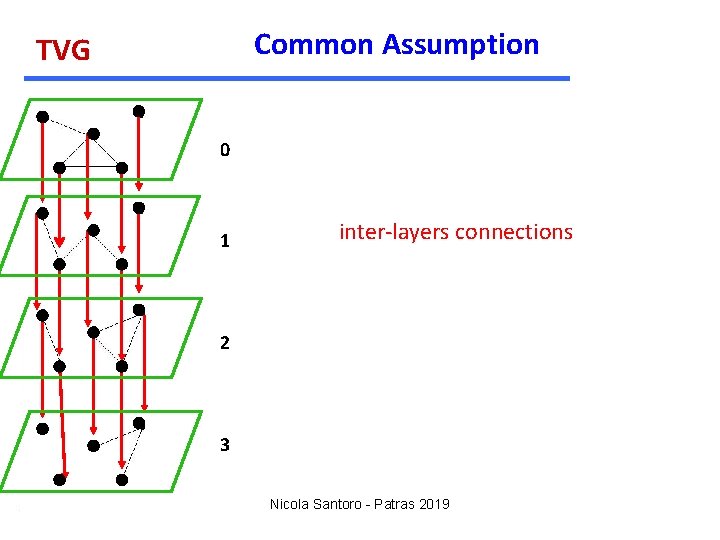
Common Assumption TVG 0 1 inter-layers connections 2 3 Nicola Santoro - Patras 2019
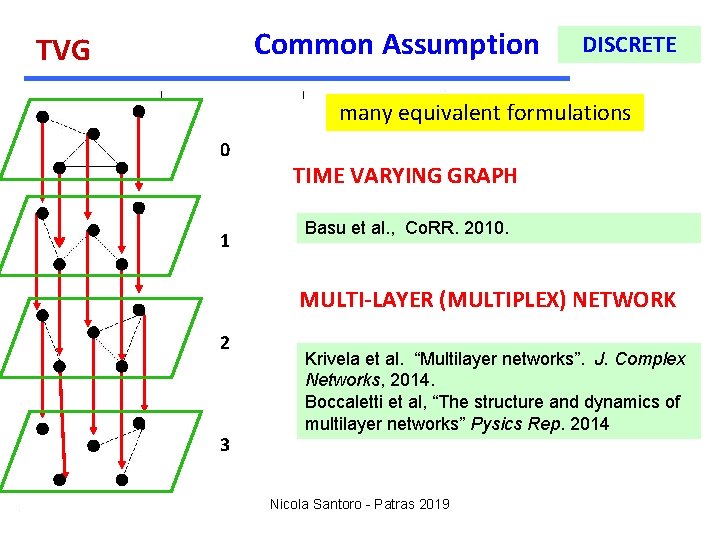
Common Assumption TVG DISCRETE many equivalent formulations 0 1 TIME VARYING GRAPH Basu et al. , Co. RR. 2010. MULTI-LAYER (MULTIPLEX) NETWORK 2 3 Krivela et al. “Multilayer networks”. J. Complex Networks, 2014. Boccaletti et al, “The structure and dynamics of multilayer networks” Pysics Rep. 2014 Nicola Santoro - Patras 2019
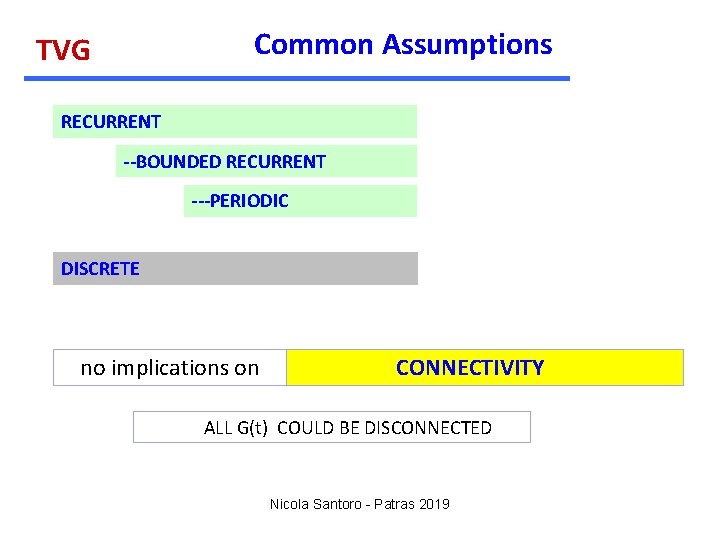
Common Assumptions TVG RECURRENT --BOUNDED RECURRENT ---PERIODIC DISCRETE no implications on CONNECTIVITY ALL G(t) COULD BE DISCONNECTED Nicola Santoro - Patras 2019
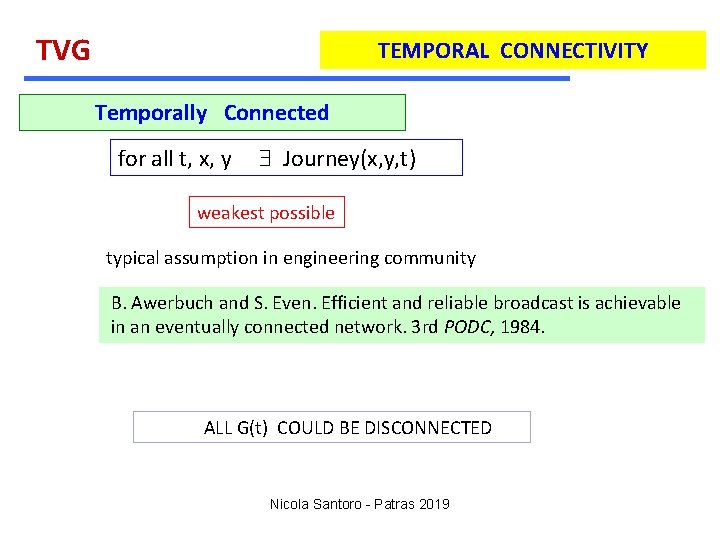
TVG TEMPORAL CONNECTIVITY Temporally Connected for all t, x, y $ Journey(x, y, t) weakest possible typical assumption in engineering community B. Awerbuch and S. Even. Efficient and reliable broadcast is achievable in an eventually connected network. 3 rd PODC, 1984. ALL G(t) COULD BE DISCONNECTED Nicola Santoro - Patras 2019
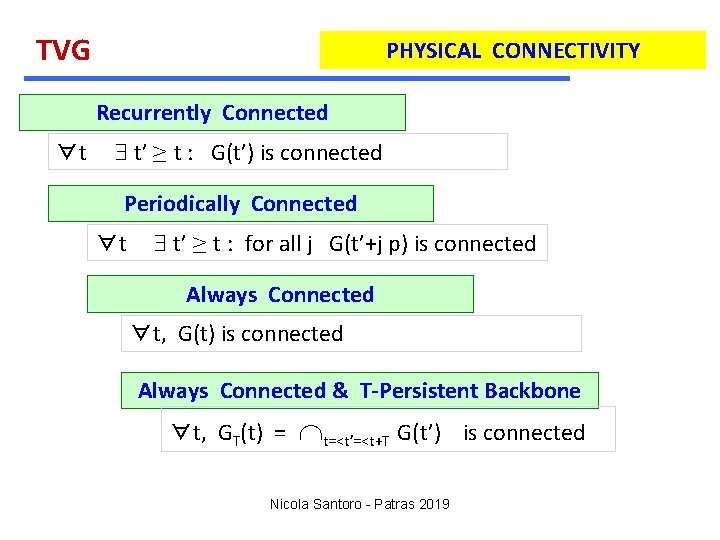
TVG PHYSICAL CONNECTIVITY Recurrently Connected ∀t $ t’ ≥ t : G(t’) is connected Periodically Connected ∀t $ t’ ≥ t : for all j G(t’+j p) is connected Always Connected ∀t, G(t) is connected Always Connected & T-Persistent Backbone ∀t, GT(t) = Çt=<t’=<t+T G(t’) Nicola Santoro - Patras 2019 is connected
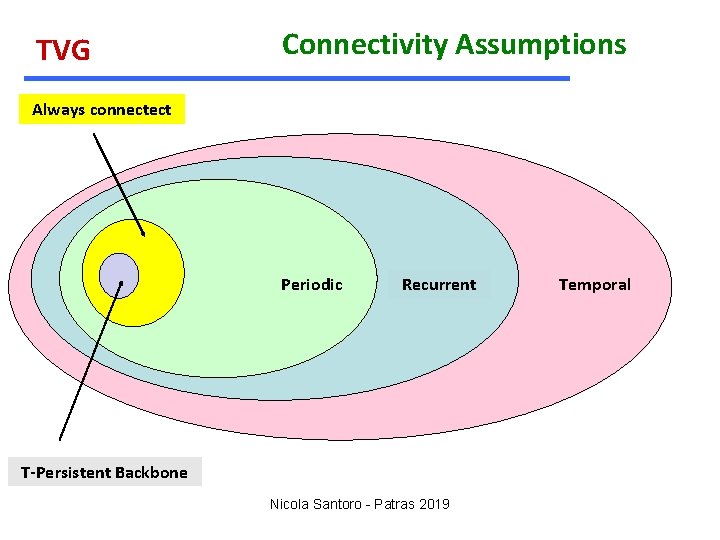
TVG Connectivity Assumptions Always connectect Periodic Recurrent T-Persistent Always+Backbone Nicola Santoro - Patras 2019 Temporal
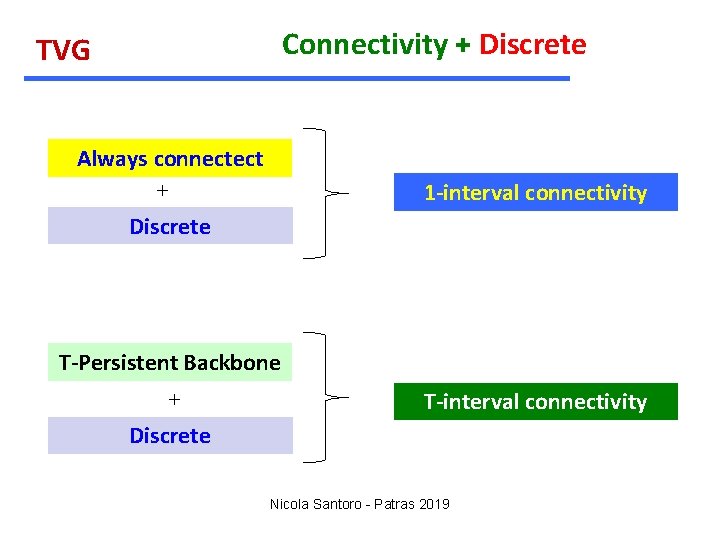
Connectivity + Discrete TVG Always connectect 1 -interval connectivity + Discrete T-Persistent Backbone + T-interval connectivity Discrete Nicola Santoro - Patras 2019
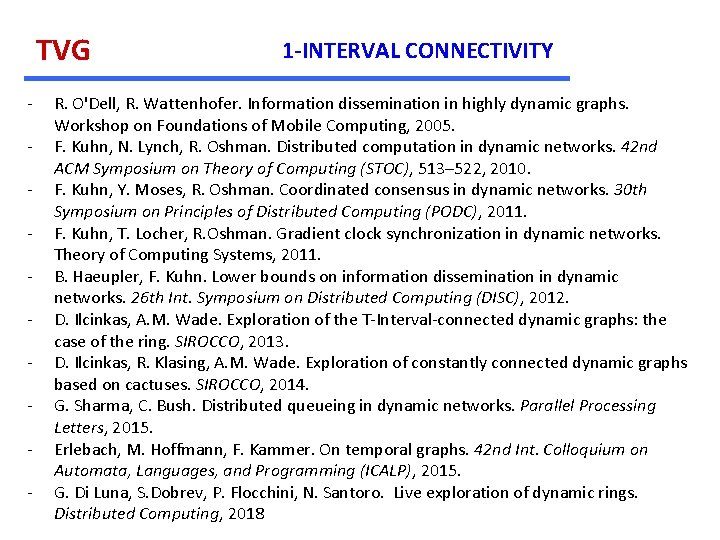
TVG - 1 -INTERVAL CONNECTIVITY R. O'Dell, R. Wattenhofer. Information dissemination in highly dynamic graphs. Workshop on Foundations of Mobile Computing, 2005. F. Kuhn, N. Lynch, R. Oshman. Distributed computation in dynamic networks. 42 nd ACM Symposium on Theory of Computing (STOC), 513– 522, 2010. F. Kuhn, Y. Moses, R. Oshman. Coordinated consensus in dynamic networks. 30 th Symposium on Principles of Distributed Computing (PODC), 2011. F. Kuhn, T. Locher, R. Oshman. Gradient clock synchronization in dynamic networks. Theory of Computing Systems, 2011. B. Haeupler, F. Kuhn. Lower bounds on information dissemination in dynamic networks. 26 th Int. Symposium on Distributed Computing (DISC), 2012. D. Ilcinkas, A. M. Wade. Exploration of the T-Interval-connected dynamic graphs: the case of the ring. SIROCCO, 2013. D. Ilcinkas, R. Klasing, A. M. Wade. Exploration of constantly connected dynamic graphs based on cactuses. SIROCCO, 2014. G. Sharma, C. Bush. Distributed queueing in dynamic networks. Parallel Processing Letters, 2015. Erlebach, M. Hoffmann, F. Kammer. On temporal graphs. 42 nd Int. Colloquium on Automata, Languages, and Programming (ICALP), 2015. G. Di Luna, S. Dobrev, P. Flocchini, N. Santoro. Live exploration of dynamic rings. Distributed Computing, 2018
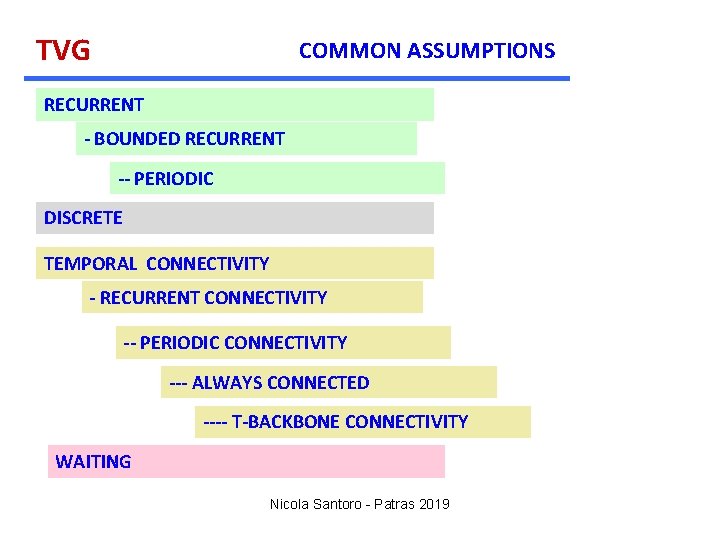
TVG COMMON ASSUMPTIONS RECURRENT - BOUNDED RECURRENT -- PERIODIC DISCRETE TEMPORAL CONNECTIVITY - RECURRENT CONNECTIVITY -- PERIODIC CONNECTIVITY --- ALWAYS CONNECTED ---- T-BACKBONE CONNECTIVITY WAITING Nicola Santoro - Patras 2019
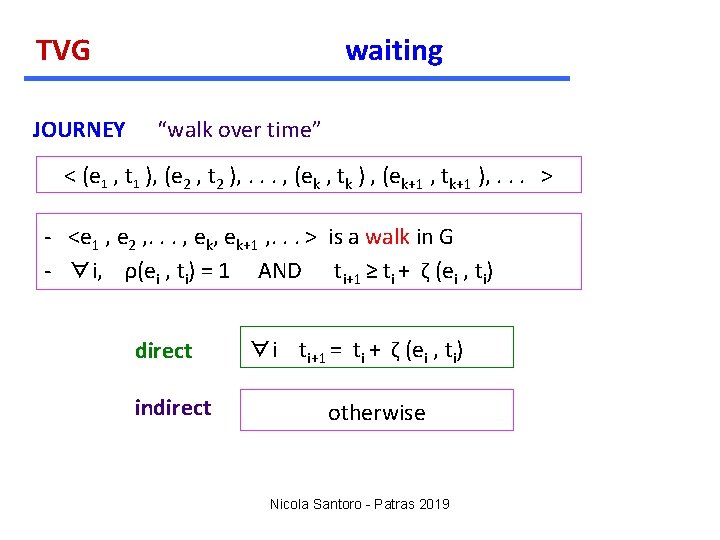
TVG JOURNEY waiting “walk over time” < (e 1 , t 1 ), (e 2 , t 2 ), . . . , (ek , tk ) , (ek+1 , tk+1 ), . . . > - <e 1 , e 2 , . . . , ek+1 , . . . > is a walk in G - ∀i, ρ(ei , ti) = 1 AND ti+1 ≥ ti + ζ (ei , ti) direct indirect ∀i ti+1 = ti + ζ (ei , ti) otherwise Nicola Santoro - Patras 2019
![TVG waiting A mobile agent E 1 3 Traverse path A E D t0 TVG waiting A mobile agent E [1, 3) Traverse path [A, E, D] t=0](https://slidetodoc.com/presentation_image_h/20f0eb14b4e952b4479cc35f50d28925/image-80.jpg)
TVG waiting A mobile agent E [1, 3) Traverse path [A, E, D] t=0 D E B [0, 2) B A C Nicola Santoro - Patras 2019 [2, 4) [0, 1) D [0, 1) [0, 3) [2, 4) C
![TVG waiting A E 1 3 Traverse path A E D t1 WAIT at TVG waiting A E [1, 3) Traverse path [A, E, D] t=1 WAIT at](https://slidetodoc.com/presentation_image_h/20f0eb14b4e952b4479cc35f50d28925/image-81.jpg)
TVG waiting A E [1, 3) Traverse path [A, E, D] t=1 WAIT at E D E B [0, 2) B A C Nicola Santoro - Patras 2019 [2, 4) [0, 1) D [0, 1) [0, 3) [2, 4) C
![TimeVarying Graph waiting A E 1 3 Traverse path A E D t2 t3 Time-Varying Graph: waiting A E [1, 3) Traverse path [A, E, D] t=2 t=3](https://slidetodoc.com/presentation_image_h/20f0eb14b4e952b4479cc35f50d28925/image-82.jpg)
Time-Varying Graph: waiting A E [1, 3) Traverse path [A, E, D] t=2 t=3 D E B [0, 2) B A C Nicola Santoro - Patras 2019 [2, 4) [0, 1) D [0, 1) [0, 3) [2, 4) C
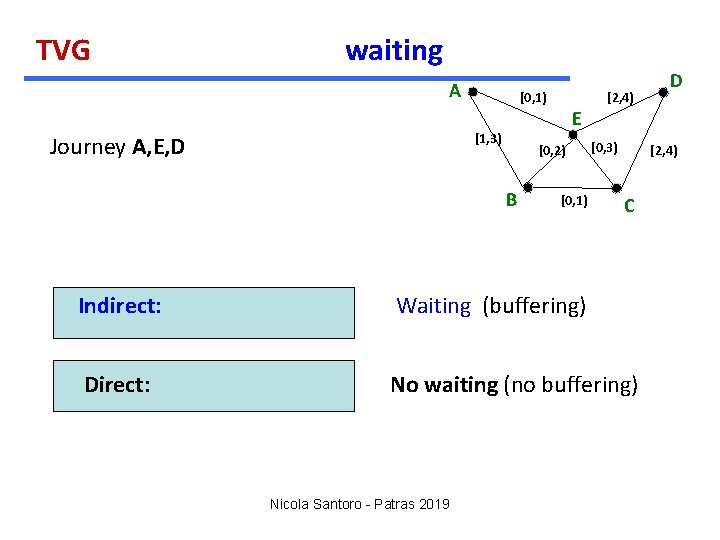
TVG waiting A E [1, 3) Journey A, E, D [2, 4) [0, 1) [0, 2) B [0, 1) [0, 3) [2, 4) C Indirect: Waiting (buffering) Direct: No waiting (no buffering) Nicola Santoro - Patras 2019 D
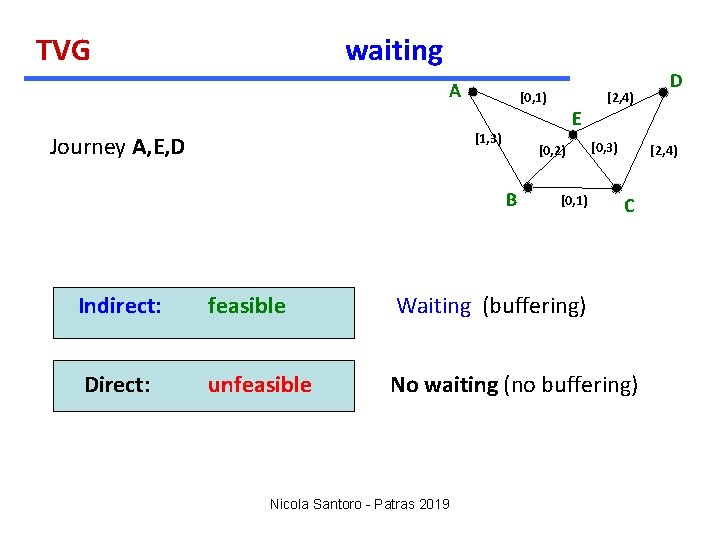
TVG waiting A E [1, 3) Journey A, E, D [2, 4) [0, 1) [0, 2) B [0, 1) [0, 3) [2, 4) C Indirect: feasible Waiting (buffering) Direct: unfeasible No waiting (no buffering) Nicola Santoro - Patras 2019 D
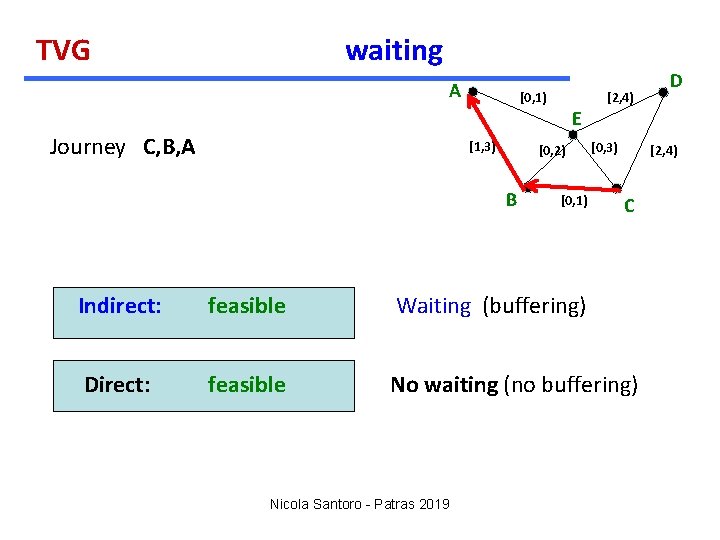
TVG waiting A [2, 4) [0, 1) D E Journey C, B, A [1, 3) [0, 2) B [0, 1) [0, 3) [2, 4) C Indirect: feasible Waiting (buffering) Direct: feasible No waiting (no buffering) Nicola Santoro - Patras 2019
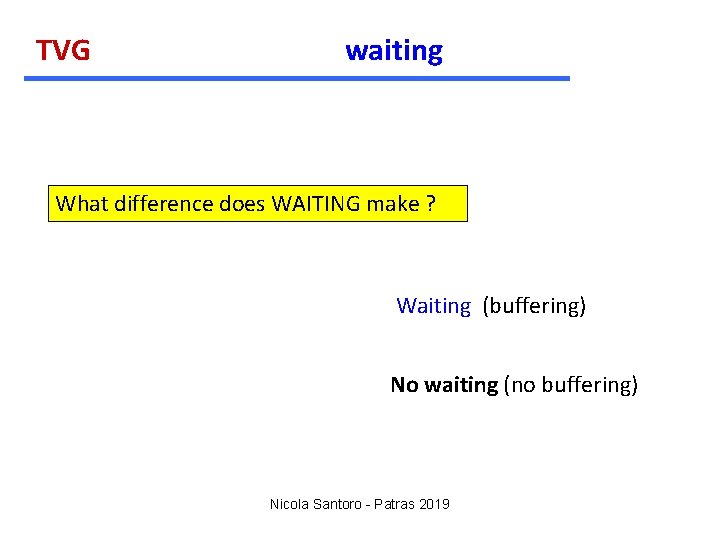
TVG waiting What difference does WAITING make ? Waiting (buffering) No waiting (no buffering) Nicola Santoro - Patras 2019
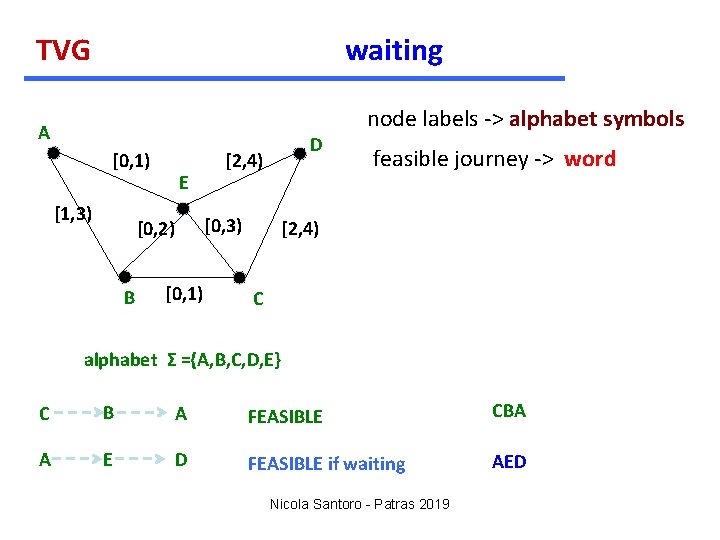
TVG waiting A [0, 1) [1, 3) E [2, 4) [0, 3) [0, 2) B D [0, 1) node labels -> alphabet symbols feasible journey -> word [2, 4) C alphabet Σ ={A, B, C, D, E} C B A FEASIBLE CBA A E D FEASIBLE if waiting AED Nicola Santoro - Patras 2019
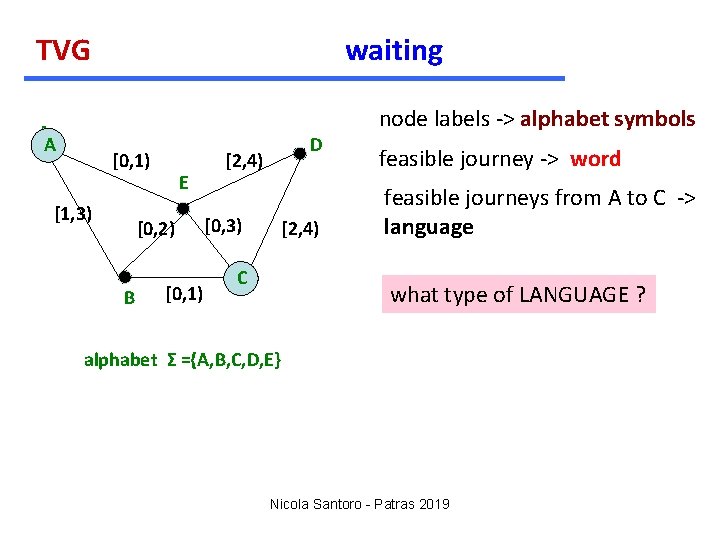
TVG A A waiting [0, 1) [1, 3) E [0, 2) B [0, 1) D [2, 4) [0, 3) [2, 4) C node labels -> alphabet symbols feasible journey -> word feasible journeys from A to C -> language what type of LANGUAGE ? alphabet Σ ={A, B, C, D, E} Nicola Santoro - Patras 2019
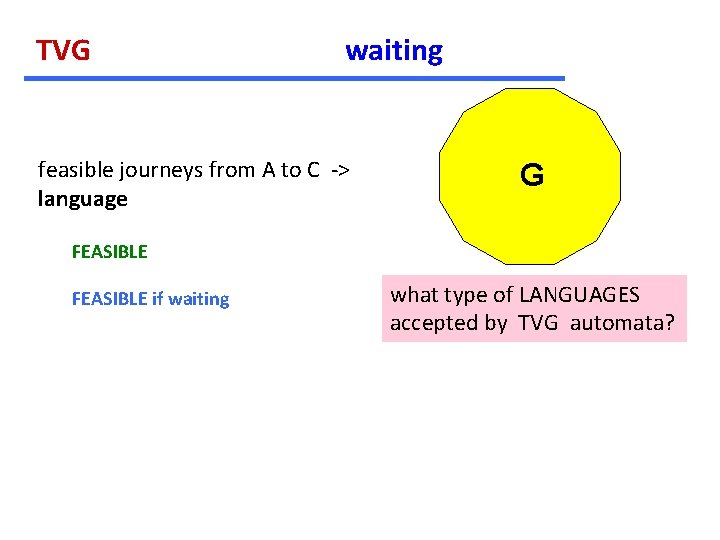
TVG waiting feasible journeys from A to C -> language G FEASIBLE if waiting what type of LANGUAGES accepted by TVG automata?
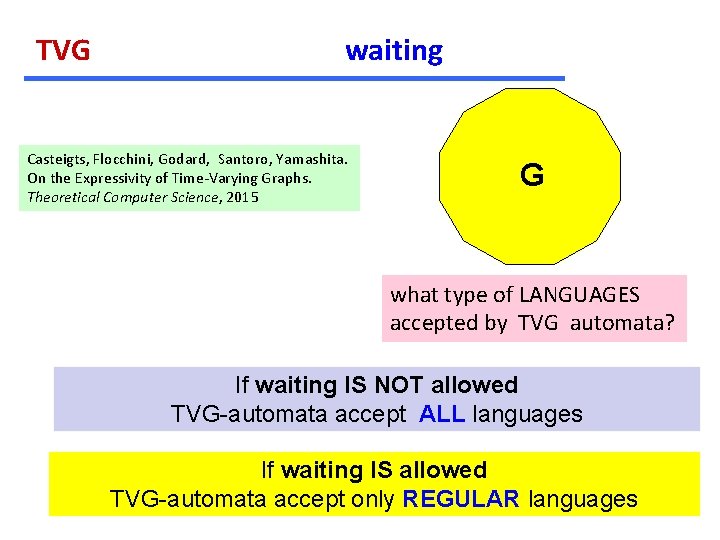
TVG waiting Casteigts, Flocchini, Godard, Santoro, Yamashita. On the Expressivity of Time-Varying Graphs. Theoretical Computer Science, 2015 G what type of LANGUAGES accepted by TVG automata? If waiting IS NOT allowed TVG-automata accept ALL languages If waiting IS allowed TVG-automata accept only REGULAR languages
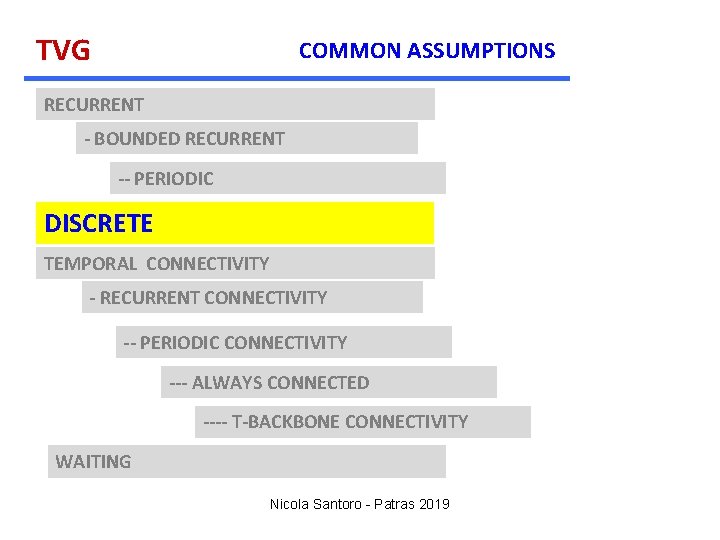
TVG COMMON ASSUMPTIONS RECURRENT - BOUNDED RECURRENT -- PERIODIC DISCRETE TEMPORAL CONNECTIVITY - RECURRENT CONNECTIVITY -- PERIODIC CONNECTIVITY --- ALWAYS CONNECTED ---- T-BACKBONE CONNECTIVITY WAITING Nicola Santoro - Patras 2019
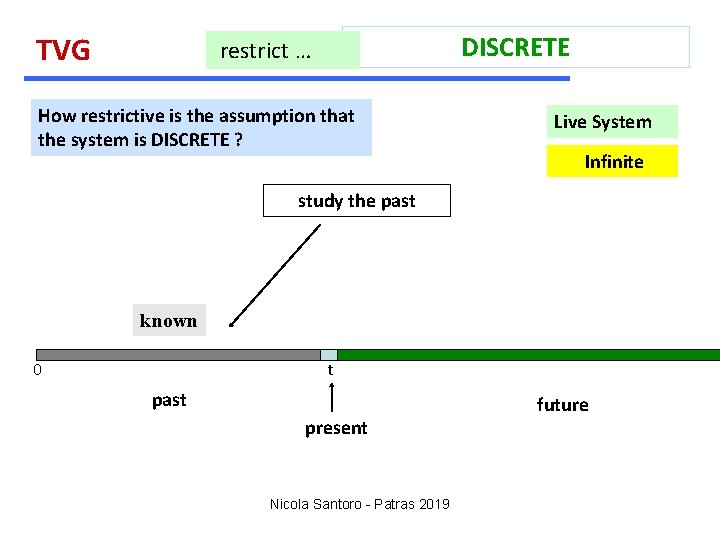
TVG DISCRETE restrict … How restrictive is the assumption that the system is DISCRETE ? Live System Infinite study the past known 0 t past present Nicola Santoro - Patras 2019 future
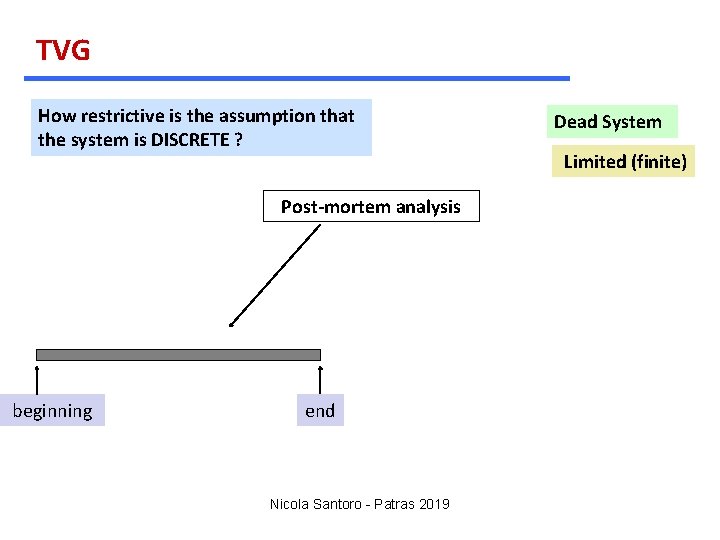
TVG How restrictive is the assumption that the system is DISCRETE ? Post-mortem analysis beginning end Nicola Santoro - Patras 2019 Dead System Limited (finite)
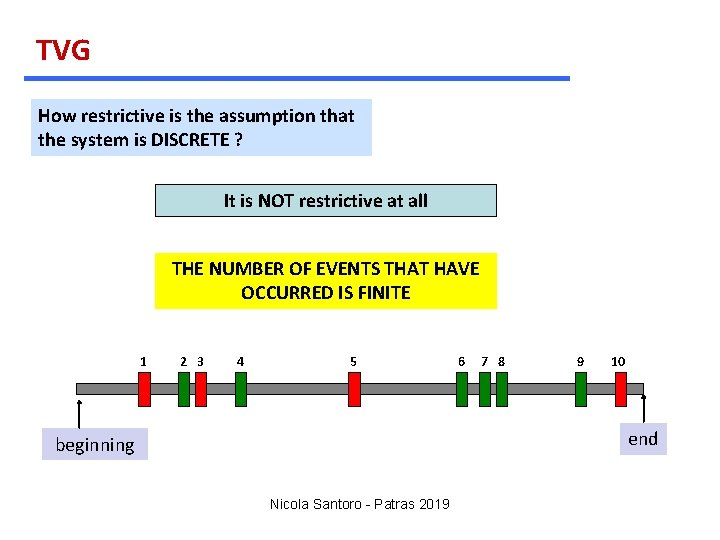
TVG How restrictive is the assumption that the system is DISCRETE ? It is NOT restrictive at all THE NUMBER OF EVENTS THAT HAVE OCCURRED IS FINITE 1 2 3 4 5 6 7 8 9 10 end beginning Nicola Santoro - Patras 2019
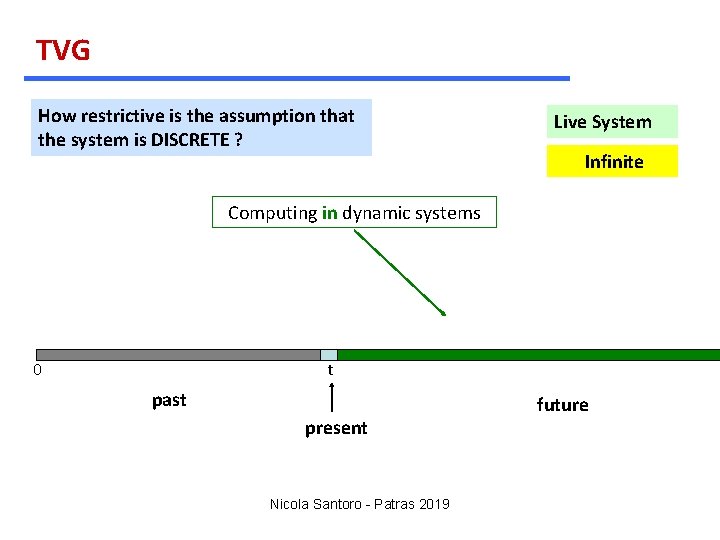
TVG How restrictive is the assumption that the system is DISCRETE ? Live System Infinite Computing in dynamic systems 0 t past present Nicola Santoro - Patras 2019 future
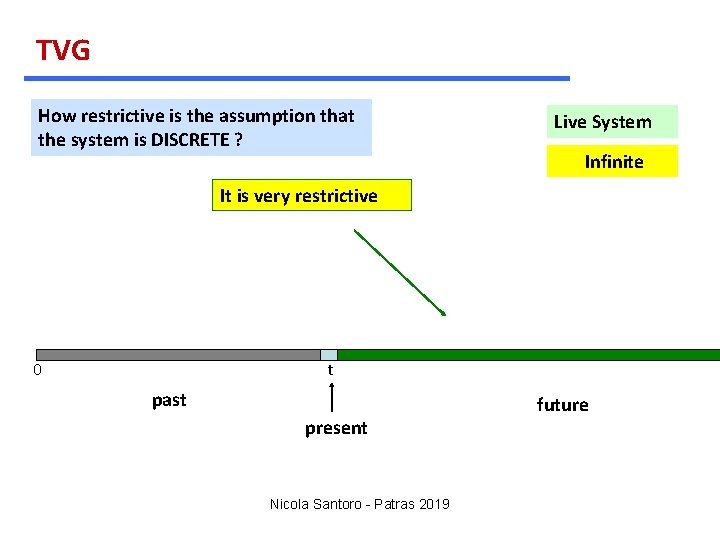
TVG How restrictive is the assumption that the system is DISCRETE ? Live System Infinite It is very restrictive 0 t past present Nicola Santoro - Patras 2019 future
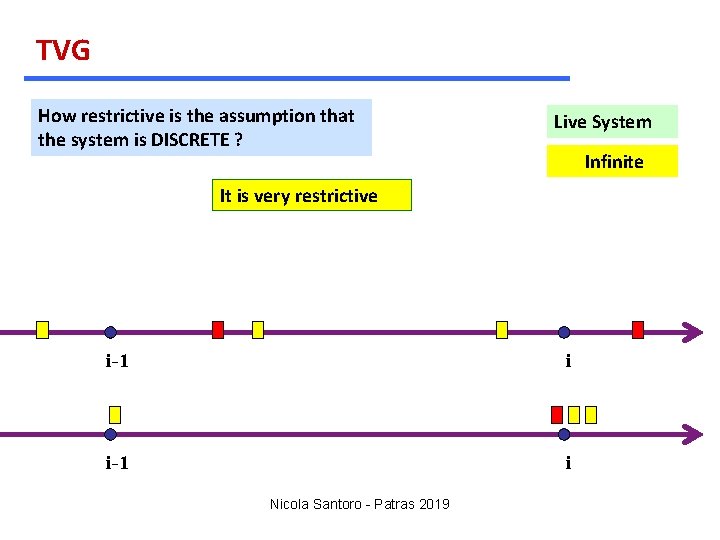
TVG How restrictive is the assumption that the system is DISCRETE ? Live System Infinite It is very restrictive i-1 i Nicola Santoro - Patras 2019
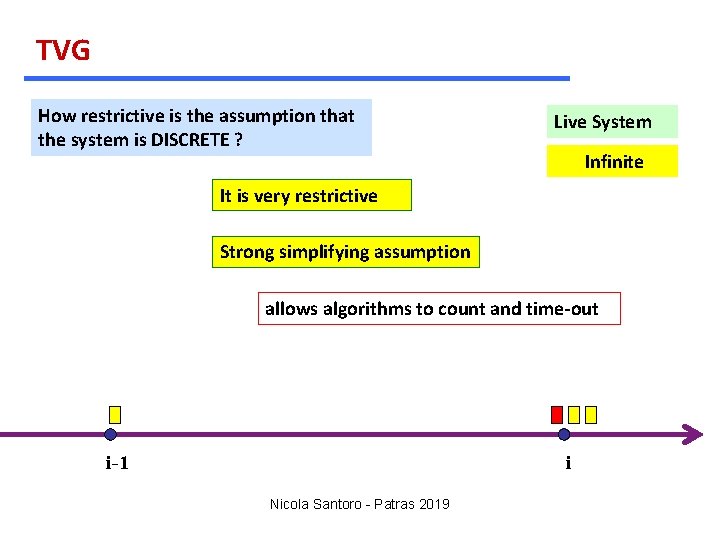
TVG How restrictive is the assumption that the system is DISCRETE ? Live System Infinite It is very restrictive Strong simplifying assumption allows algorithms to count and time-out i-1 i Nicola Santoro - Patras 2019
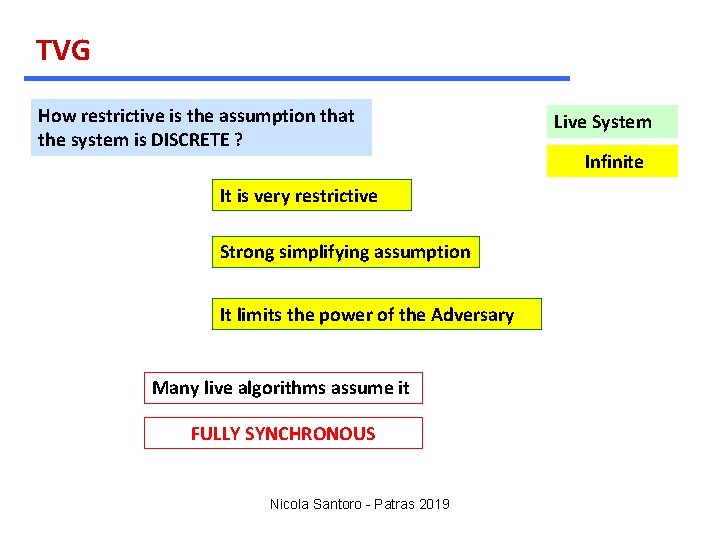
TVG How restrictive is the assumption that the system is DISCRETE ? It is very restrictive Strong simplifying assumption It limits the power of the Adversary Many live algorithms assume it FULLY SYNCHRONOUS Nicola Santoro - Patras 2019 Live System Infinite
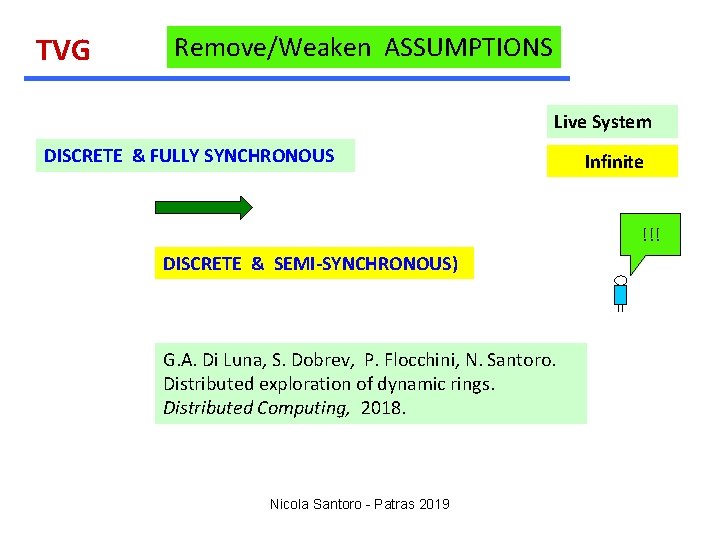
TVG Remove/Weaken ASSUMPTIONS Live System DISCRETE & FULLY SYNCHRONOUS Infinite !!! DISCRETE & SEMI-SYNCHRONOUS) G. A. Di Luna, S. Dobrev, P. Flocchini, N. Santoro. Distributed exploration of dynamic rings. Distributed Computing, 2018. Nicola Santoro - Patras 2019
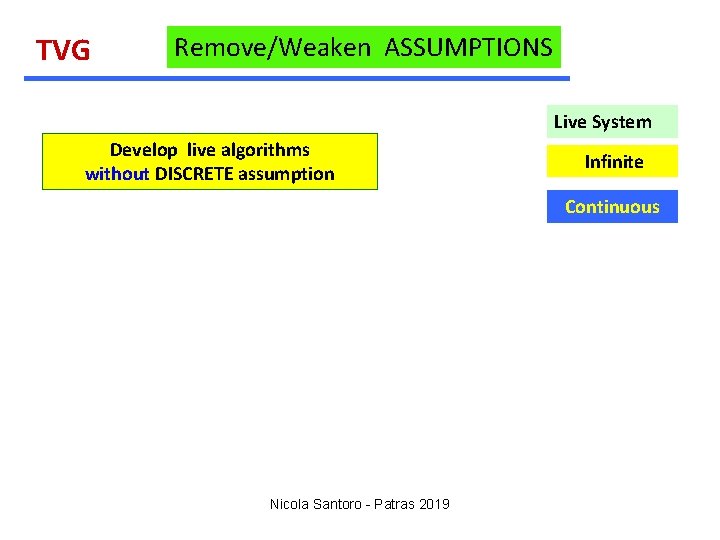
TVG Remove/Weaken ASSUMPTIONS Live System Develop live algorithms without DISCRETE assumption Infinite Continuous Nicola Santoro - Patras 2019
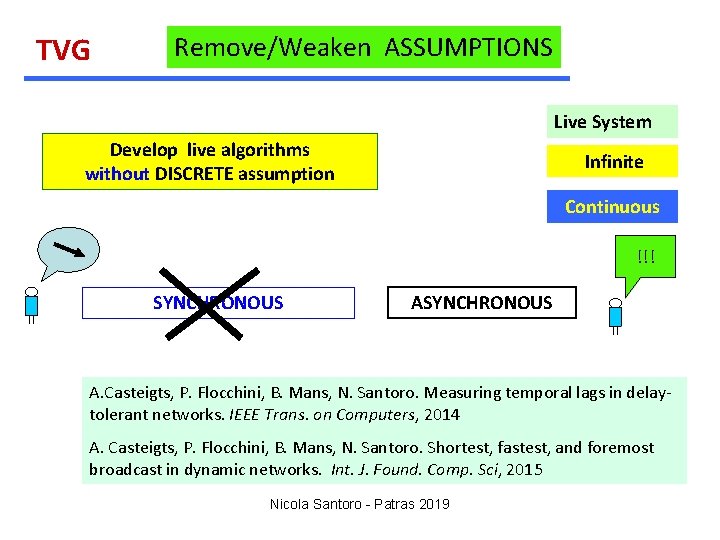
TVG Remove/Weaken ASSUMPTIONS Live System Develop live algorithms without DISCRETE assumption Infinite Continuous !!! SYNCHRONOUS A. Casteigts, P. Flocchini, B. Mans, N. Santoro. Measuring temporal lags in delaytolerant networks. IEEE Trans. on Computers, 2014 A. Casteigts, P. Flocchini, B. Mans, N. Santoro. Shortest, fastest, and foremost broadcast in dynamic networks. Int. J. Found. Comp. Sci, 2015 Nicola Santoro - Patras 2019
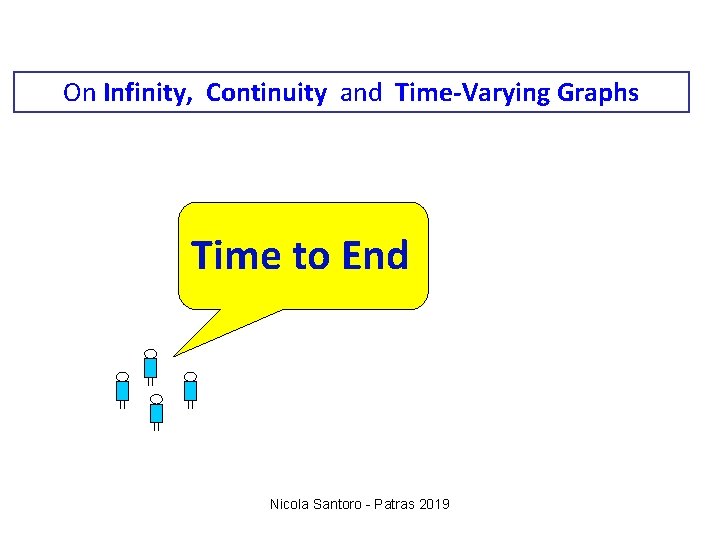
On Infinity, Continuity and Time-Varying Graphs Time to End Nicola Santoro - Patras 2019
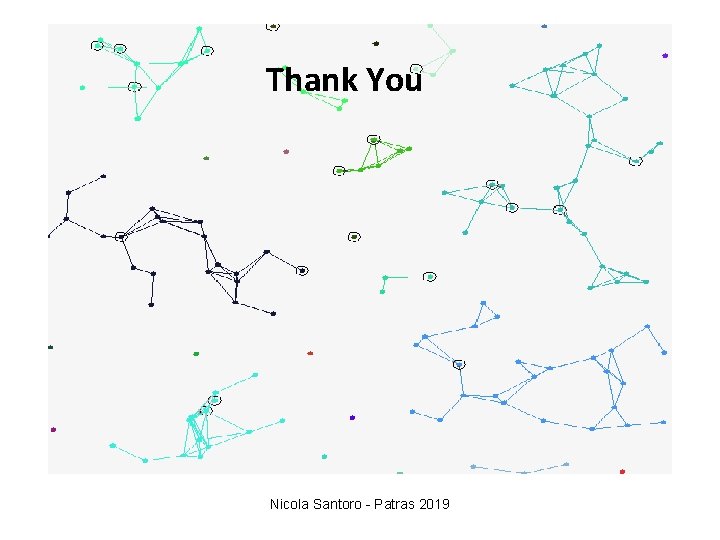
Thank You Nicola Santoro - Patras 2019
Fabrizio santoro
Kari santoro
Samantha santoro
Sec asymptotes
Absolute continuity implies uniform continuity
State testing and testability tips
Graphs that enlighten and graphs that deceive
Speed and velocity
5-3 practice polynomial functions
Steamboat willie apush
Count to infinity problem solution
To infinity and beyond latin
Limits at infinity horizontal asymptotes
Limits involving infinity asymptotes
Activity 3 to infinity and beyond
Nicola carozza
Nicola licata
Nicola cezzi
Nicola allen knutsford
Angie verona nude
Nicola yoon książki
Nicola bacchetta
Reiseberufe
Nicola sheldon
Nicola cezzi
Nicola de bellis
Nicola tyas
Recogniseble
Nicola niglio
Nicola holt uwe
Nicola riley
Nicola lugaresi
Andrea di nicola
Sifat silvika kayu
Nicola cezzi
Nicola.szx
Rocco de nicola
Summary of superhuman by nicola yoon
Nicola aitchison
Nicola cezzi
Nicola kershaw
Flat coding frame
Nicola morabito
Nicola cezzi
Nicola cusano
Nicola giovanni cezzi
Nicola cezzi
Nicola hogan
Liceo scientifico nicola sensale nocera inferiore
Nicola marchant
Nicola cezzi
Phylosophy
Nicola cantore
University of kansas
Nicola bowman
Nicola tyas sex
Nicola benge
Casqe
Coding ingegneria
Nicola cigliano
Nicola cezzi
52nd lpsc
Nicola cezzi
Nicola cezzi
Nicola gale
Don nicola solla
Brent clinical commissioning group
Nicola cezzi
Nicola cezzi
Nicola cezzi
Nicola bowman
Limits calculator
Hilbert's infinite hotel
G/4
When can we use l'hopital's rule
Sigma notation word problems
Vsauce counting past infinity
Infinity-data.xlsx
Geometric series convergence
Count to infinity problem
Count to infinity problem
Limits at infinity definition
Interval notation for infinity
Limits at infinity definition
How to determine infinite limits
Complex gsm
Lens sign convention
Tora
Infinity in greek
Trans cr(en)2f2 point group
Infinity next
1 to infinity
Infinity middle school calendar
Hris best practices
Check point infinity
Infinity symbol
Infinite concept
Infinity vx
Infinite energy incorporated srl
Infinity media company
Sc421 - given product cannot be purchased infinity
Alteryx certification
Limits at infinity horizontal asymptotes
Anthony infinity 090
Image formation by convex lens