Nonlocal Means NLM Filter for Trim Statics Yunsong
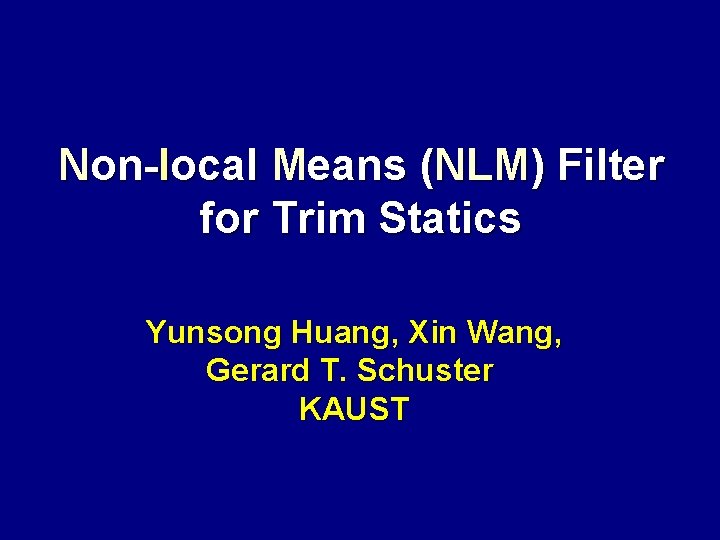
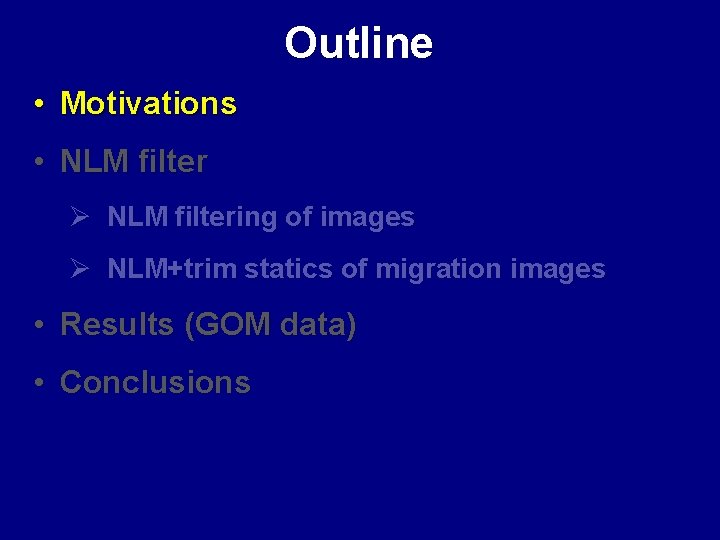
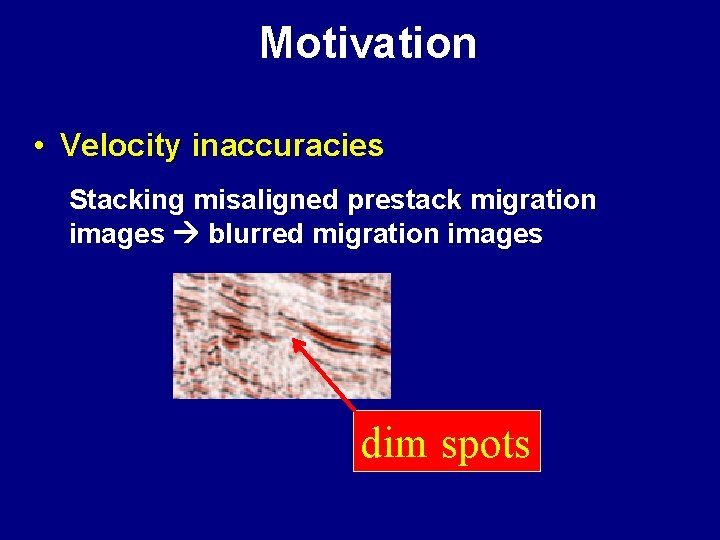
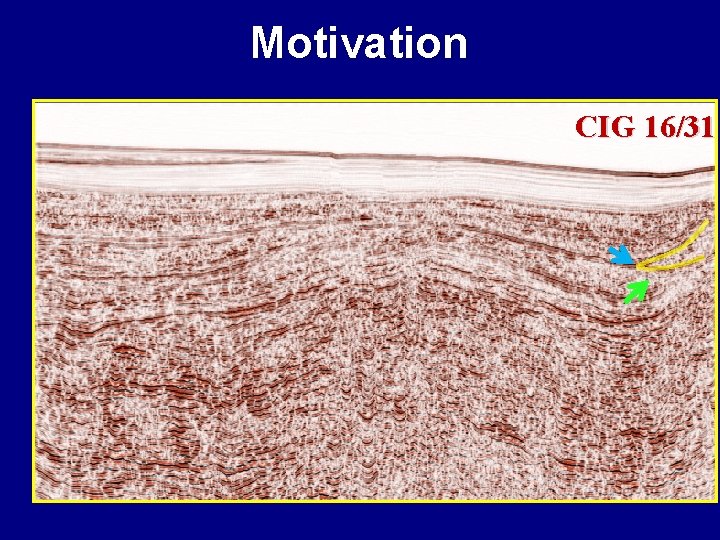
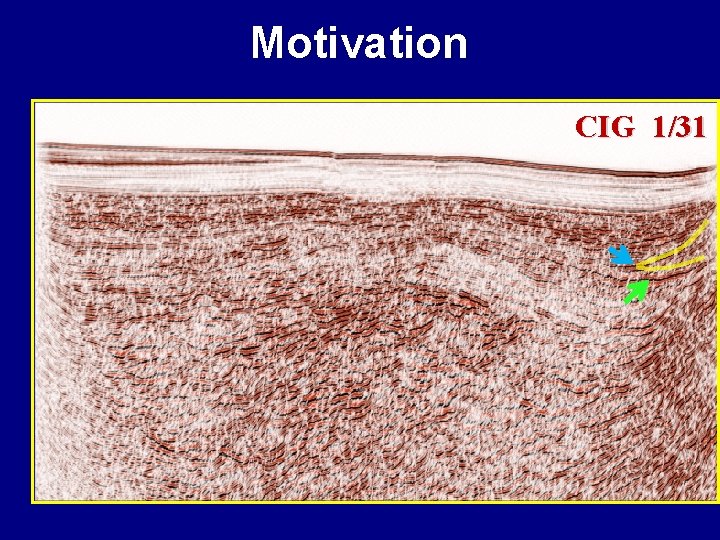
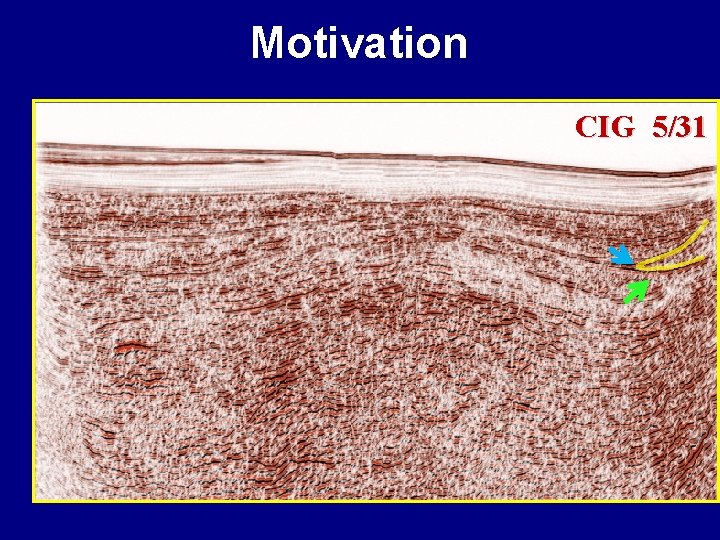
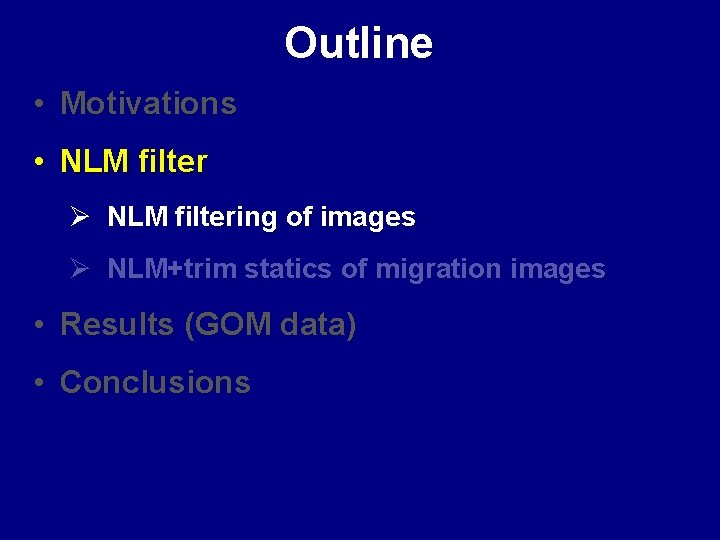
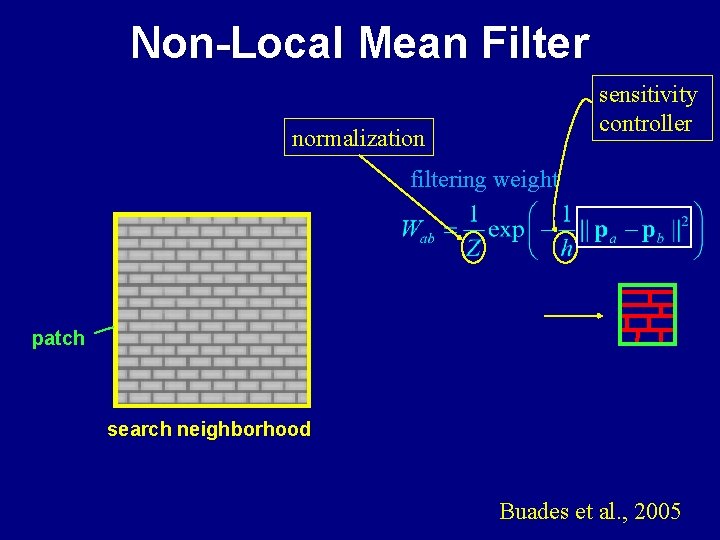
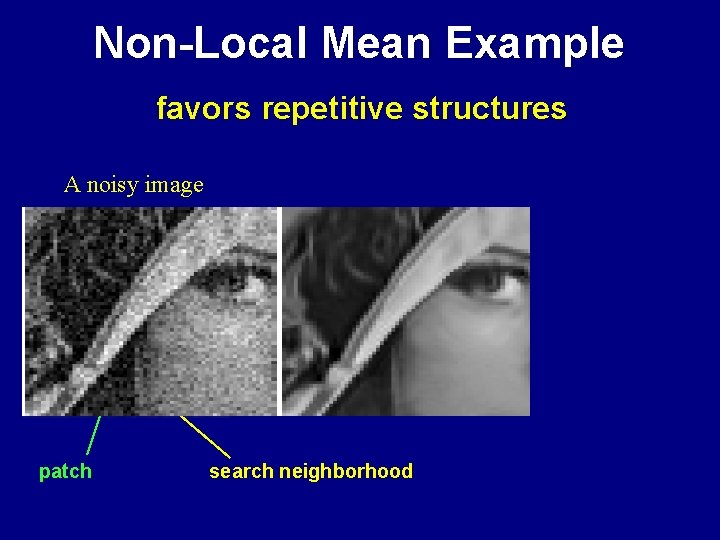
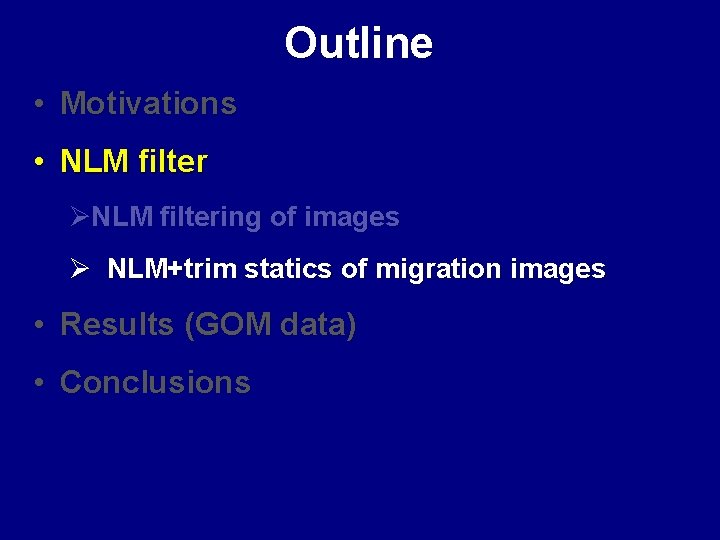
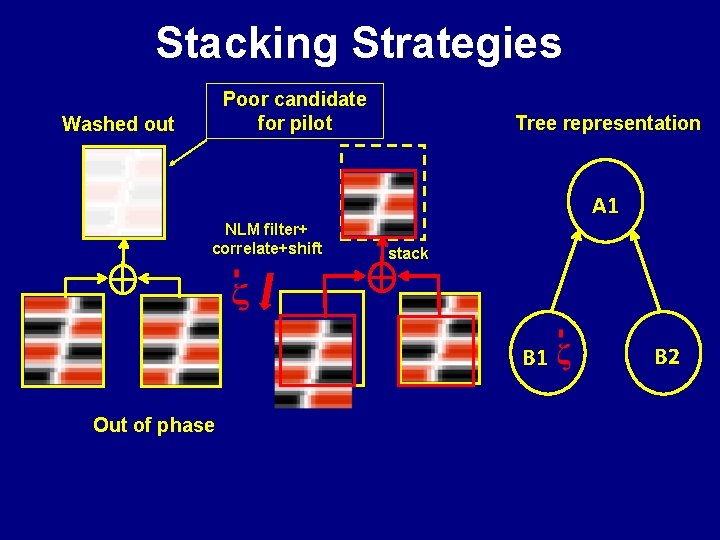
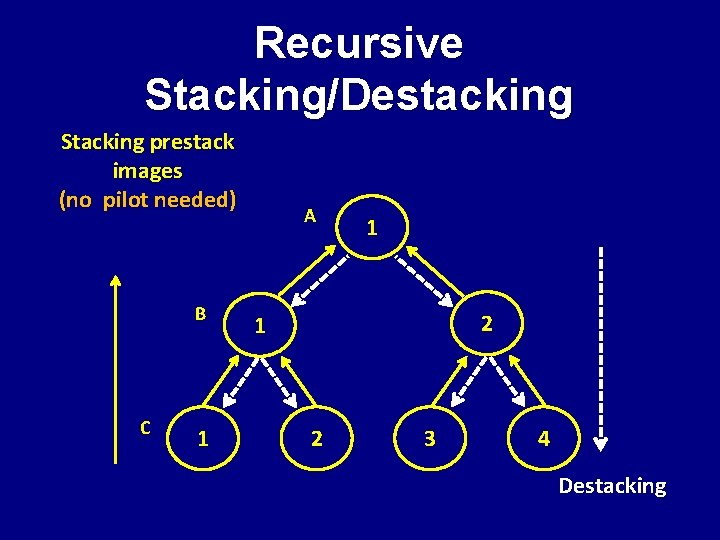
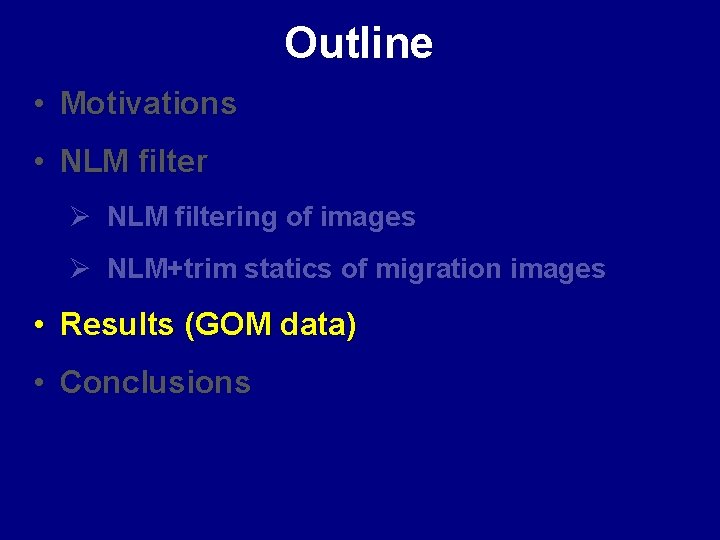
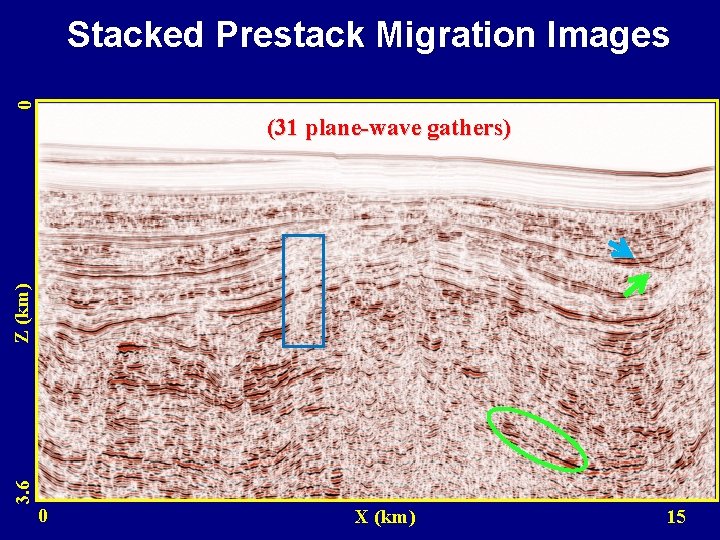
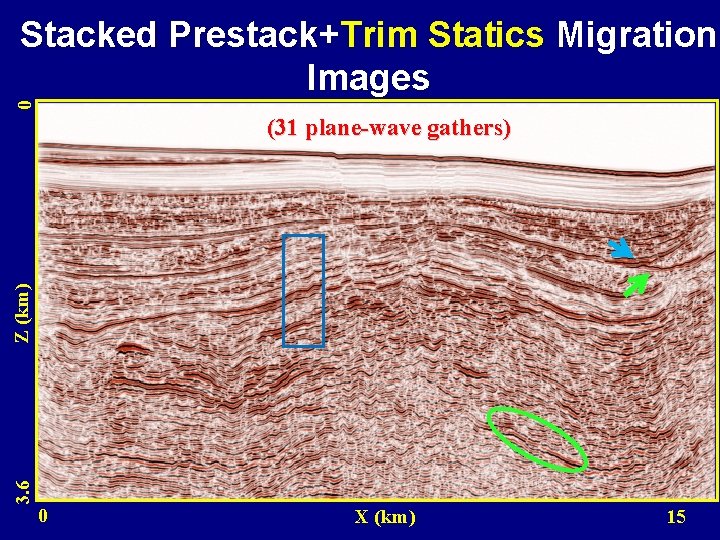
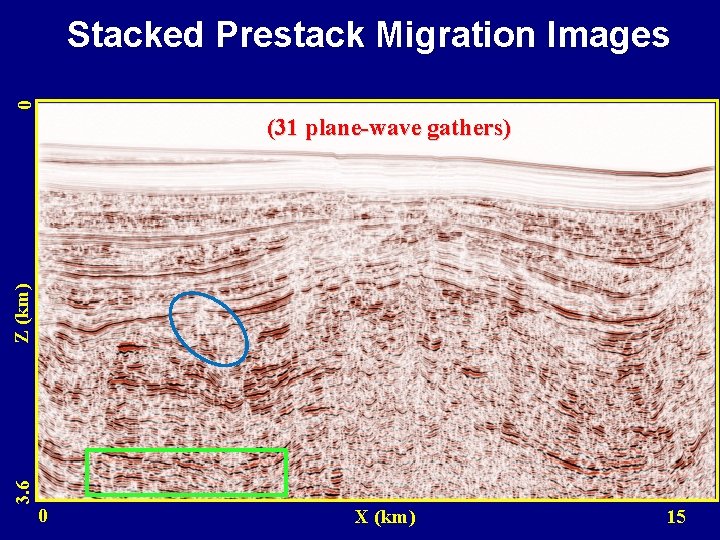
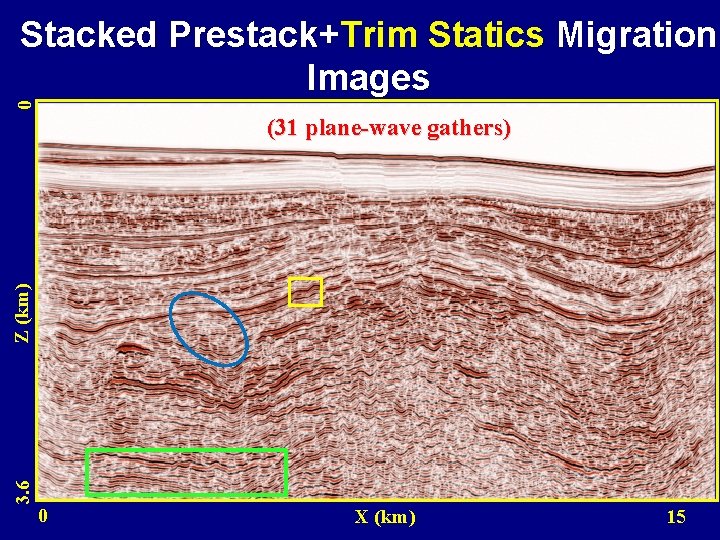
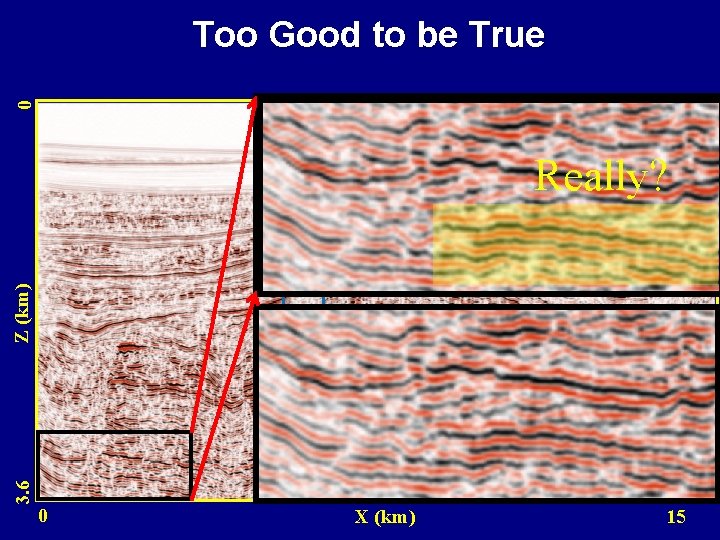
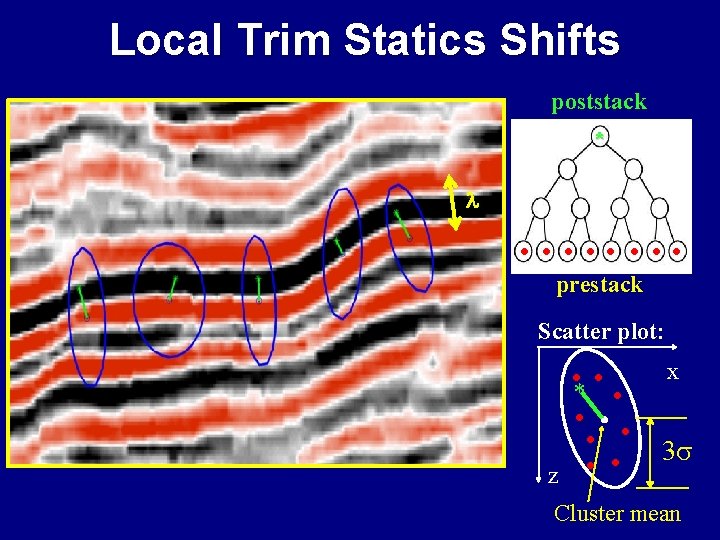
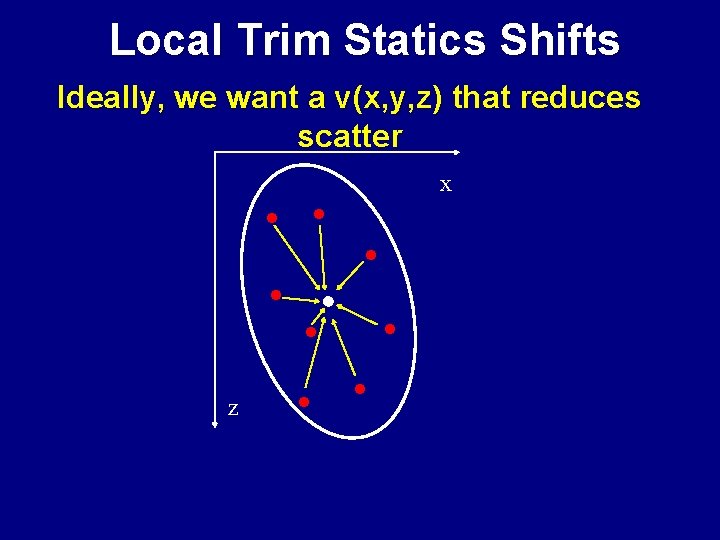
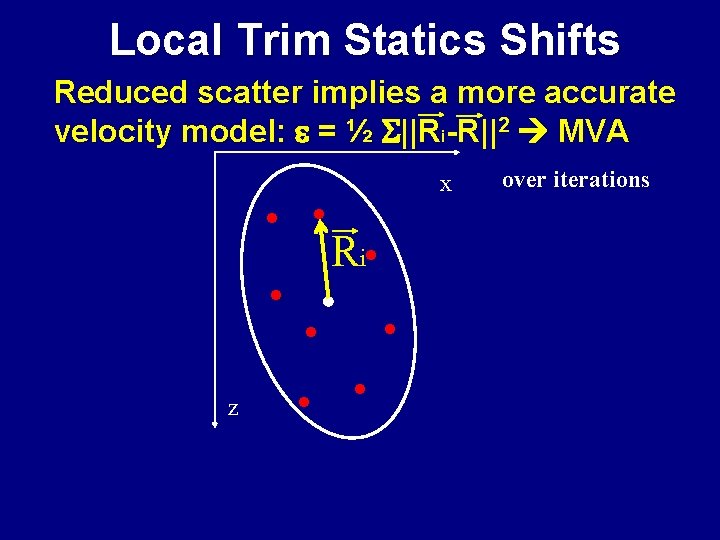
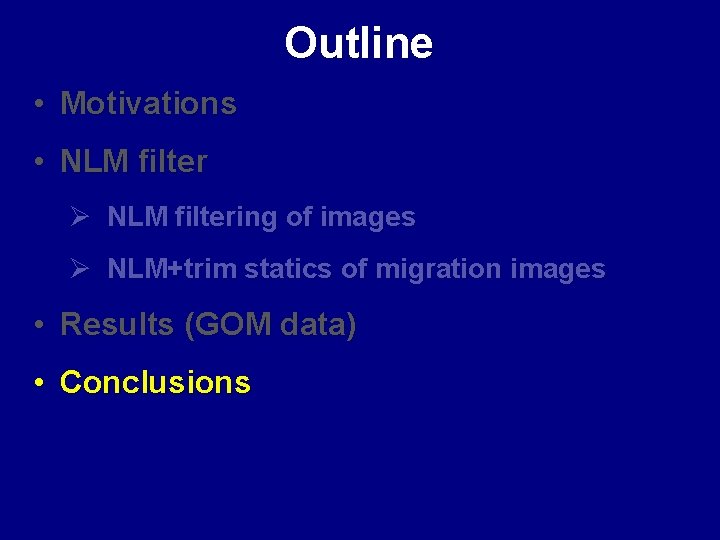
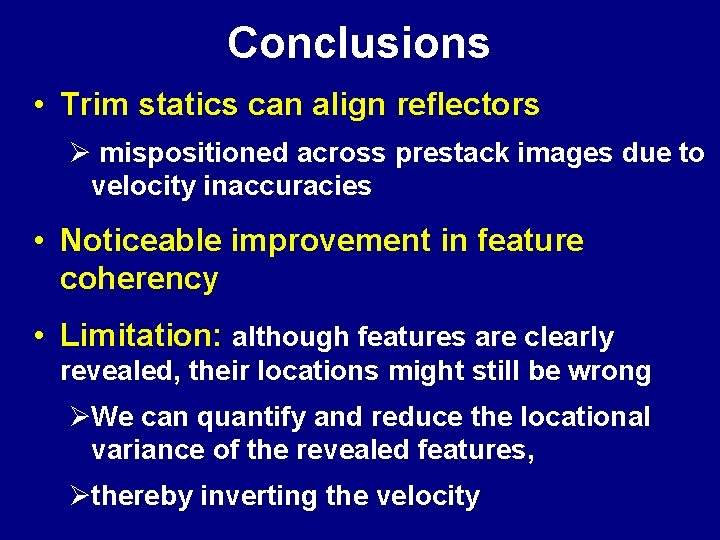
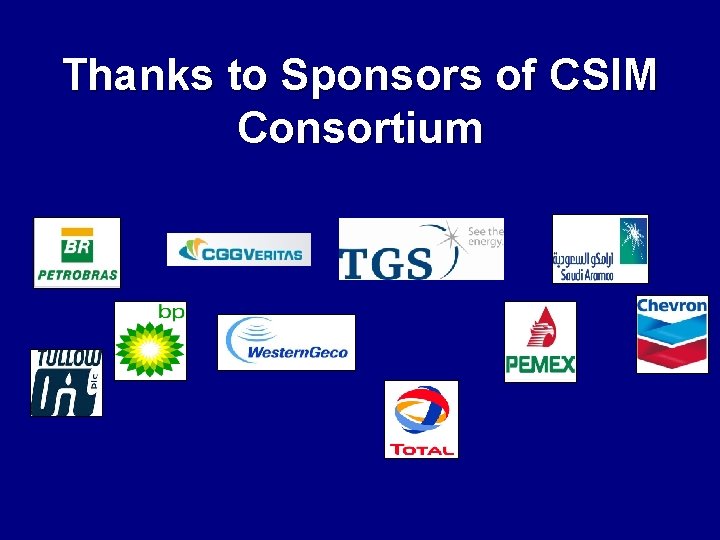
- Slides: 24
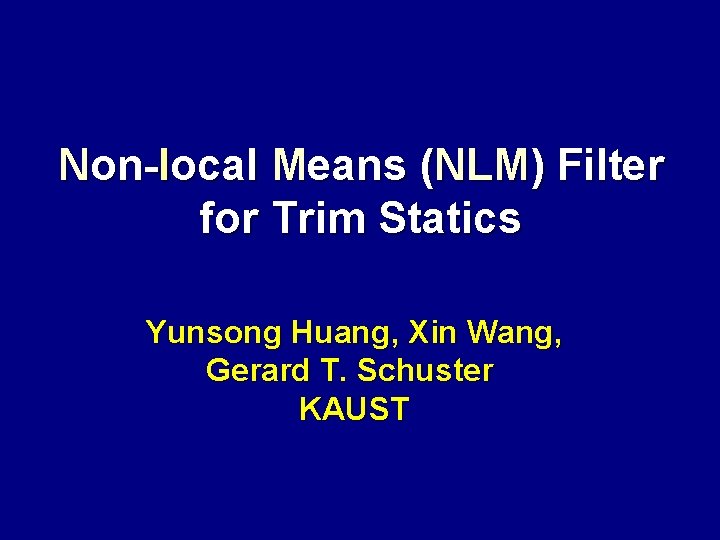
Non-local Means (NLM) Filter for Trim Statics Yunsong Huang, Xin Wang, Gerard T. Schuster KAUST
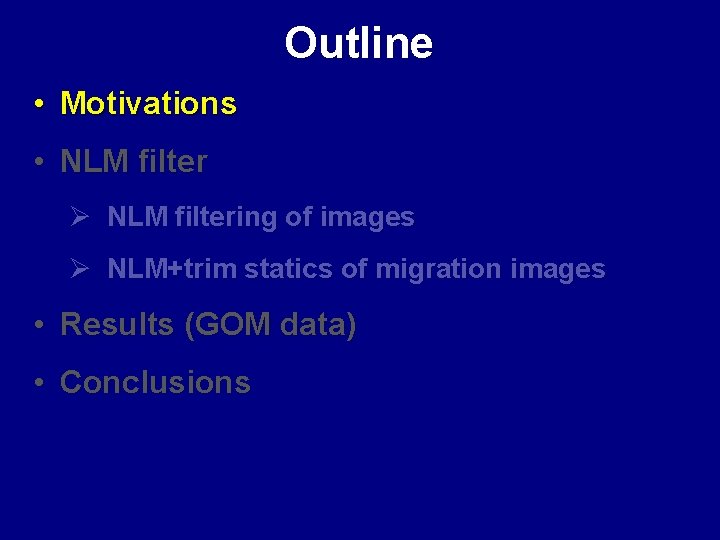
Outline • Motivations • NLM filter Ø NLM filtering of images Ø NLM+trim statics of migration images • Results (GOM data) • Conclusions
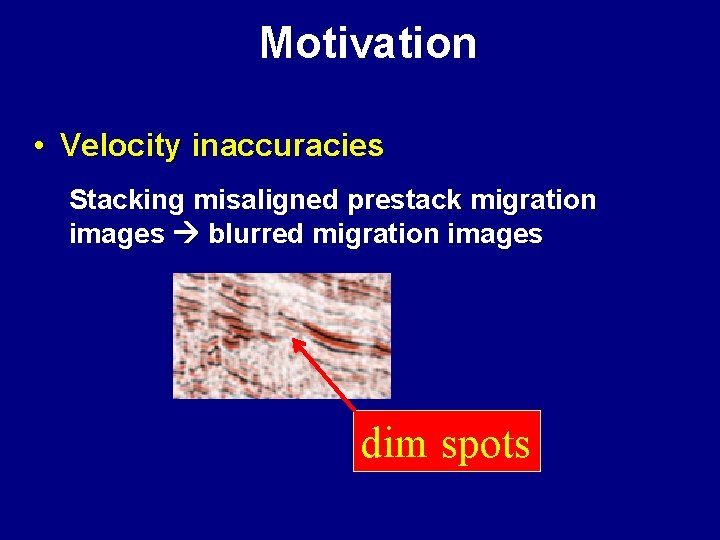
Motivation • Velocity inaccuracies Stacking misaligned prestack migration images blurred migration images dim spots
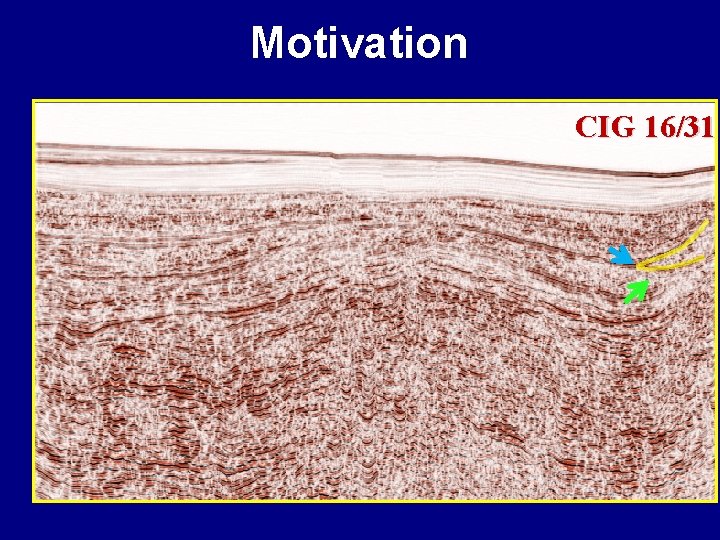
Motivation CIG 16/31
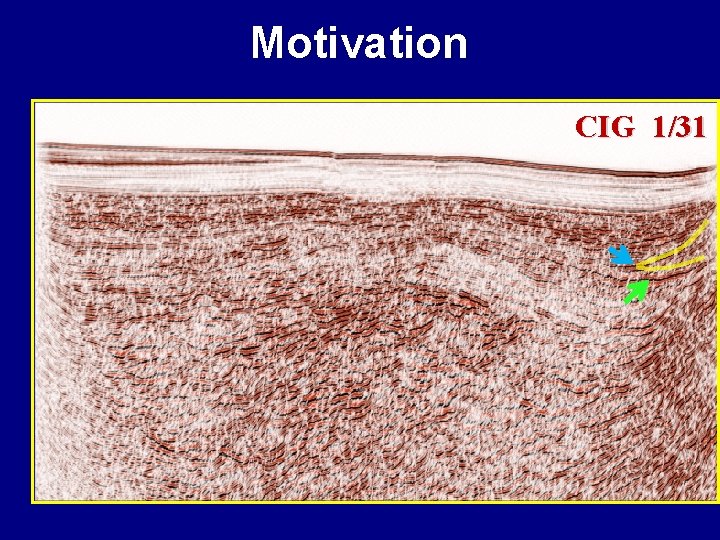
Motivation CIG 1/31
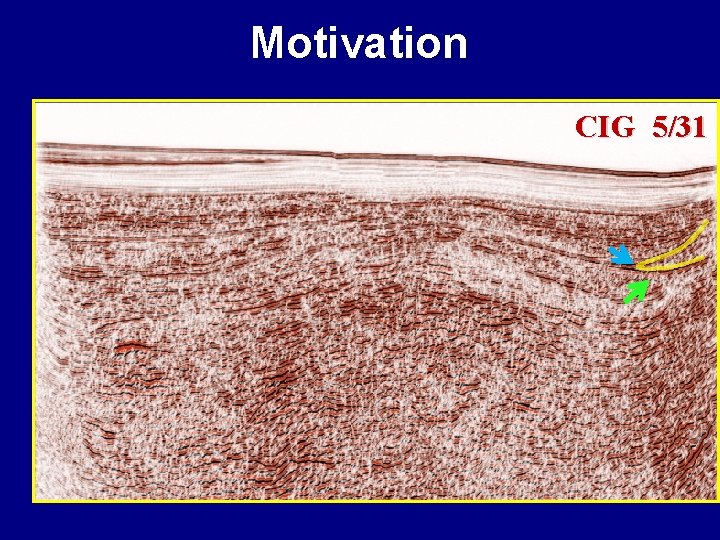
Motivation CIG 5/31
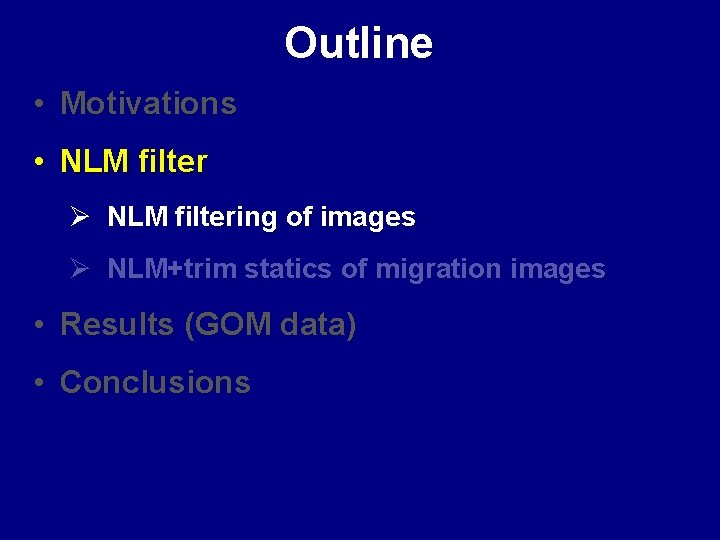
Outline • Motivations • NLM filter Ø NLM filtering of images Ø NLM+trim statics of migration images • Results (GOM data) • Conclusions
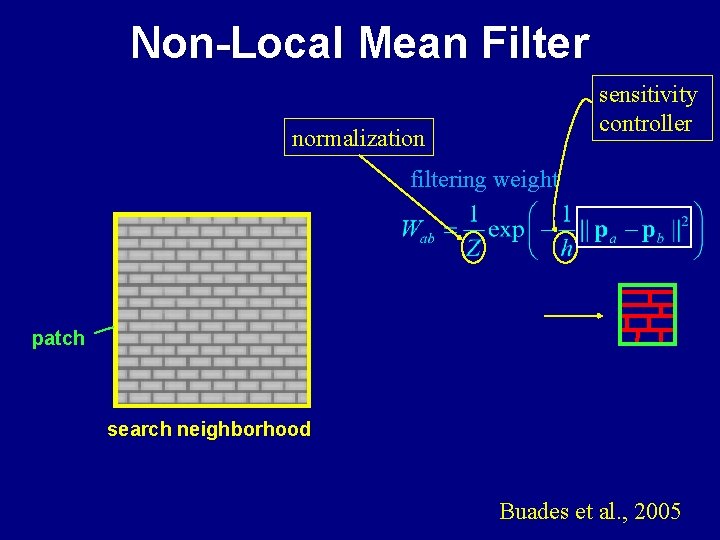
Non-Local Mean Filter sensitivity controller normalization filtering weight pb patch pa search neighborhood Buades et al. , 2005
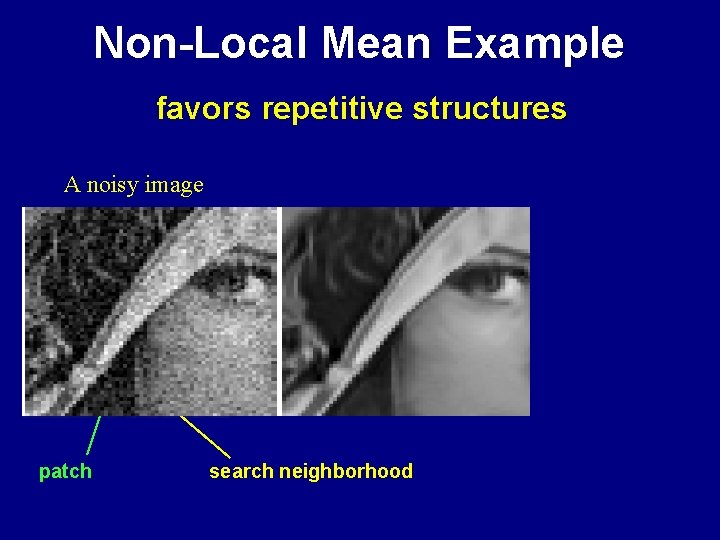
Non-Local Mean Example favors repetitive structures A noisy image patch Endo-filtered by NLM search neighborhood filtering weights
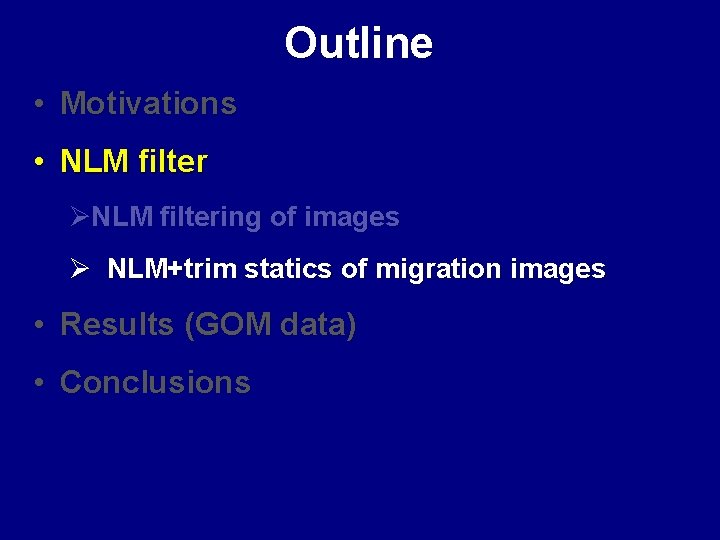
Outline • Motivations • NLM filter ØNLM filtering of images Ø NLM+trim statics of migration images • Results (GOM data) • Conclusions
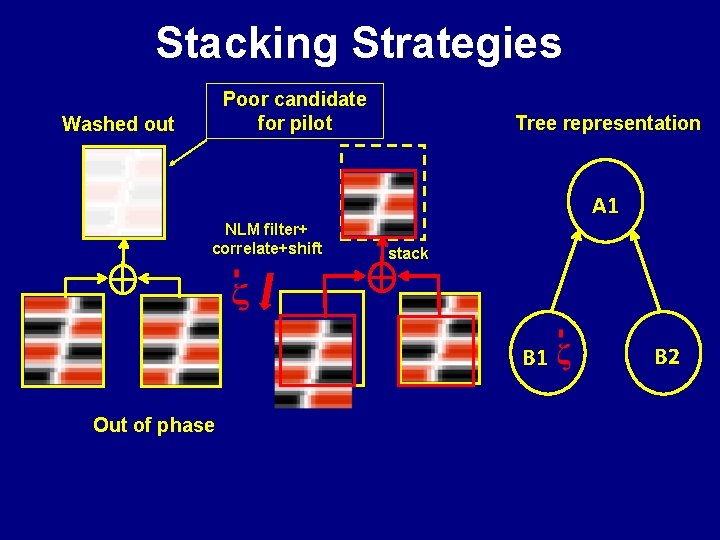
Stacking Strategies Poor candidate for pilot Washed out Tree representation A 1 NLM filter+ correlate+shift stack B 1 Out of phase B 2
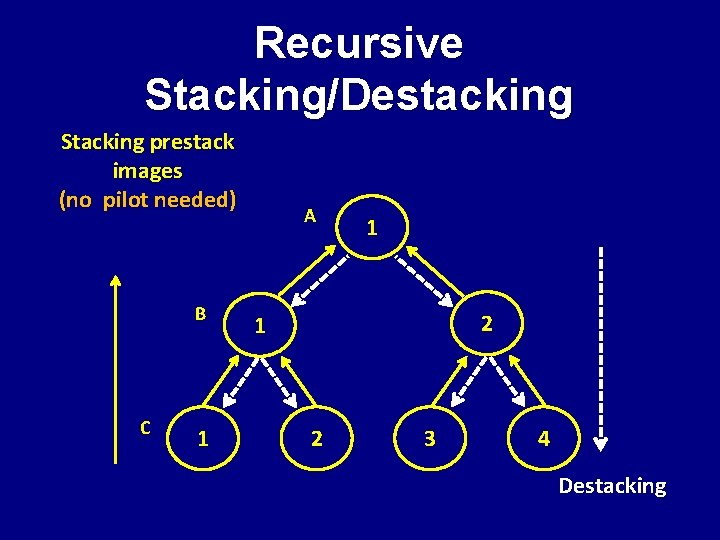
Recursive Stacking/Destacking Stacking prestack images (no pilot needed) B C 1 A 1 2 3 4 Destacking
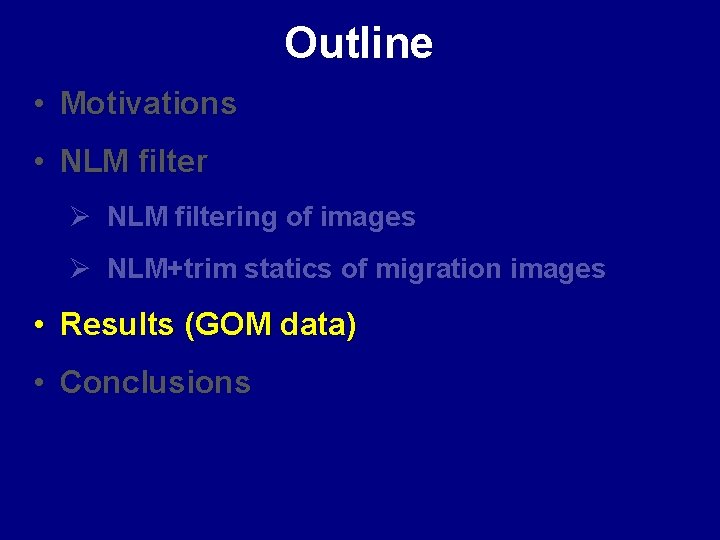
Outline • Motivations • NLM filter Ø NLM filtering of images Ø NLM+trim statics of migration images • Results (GOM data) • Conclusions
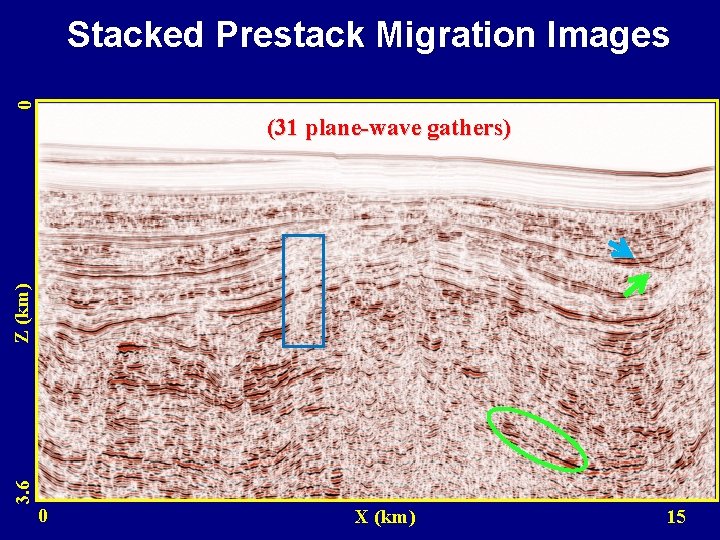
0 Stacked Prestack Migration Images 3. 6 Z (km) (31 plane-wave gathers) 0 X (km) 15
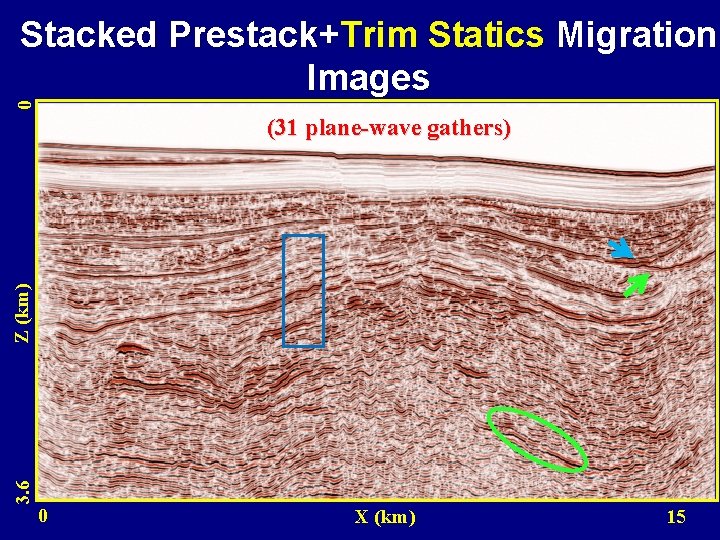
0 Stacked Prestack+Trim Statics Migration Images 3. 6 Z (km) (31 plane-wave gathers) 0 X (km) 15
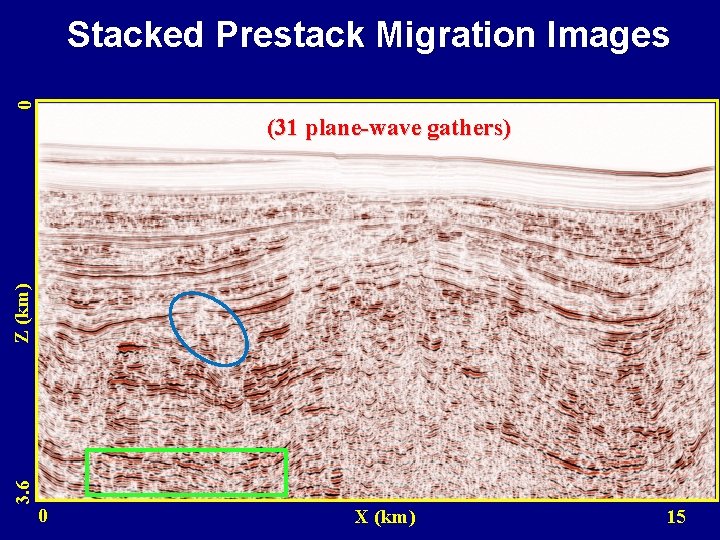
0 Stacked Prestack Migration Images 3. 6 Z (km) (31 plane-wave gathers) 0 X (km) 15
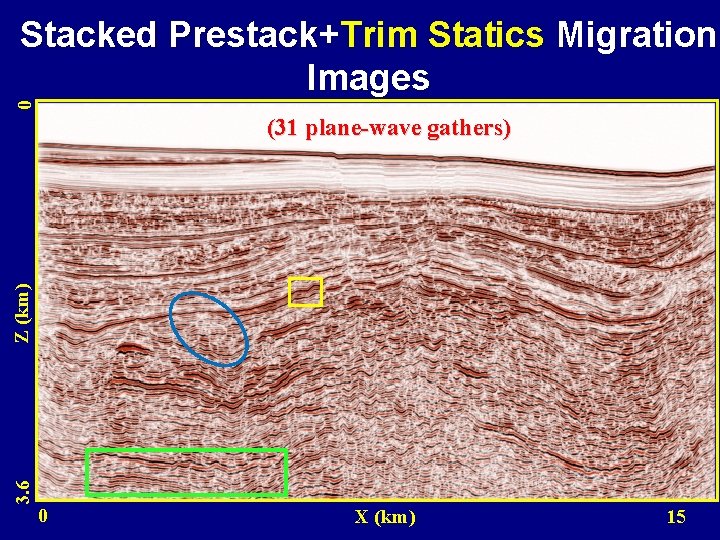
0 Stacked Prestack+Trim Statics Migration Images 3. 6 Z (km) (31 plane-wave gathers) 0 X (km) 15
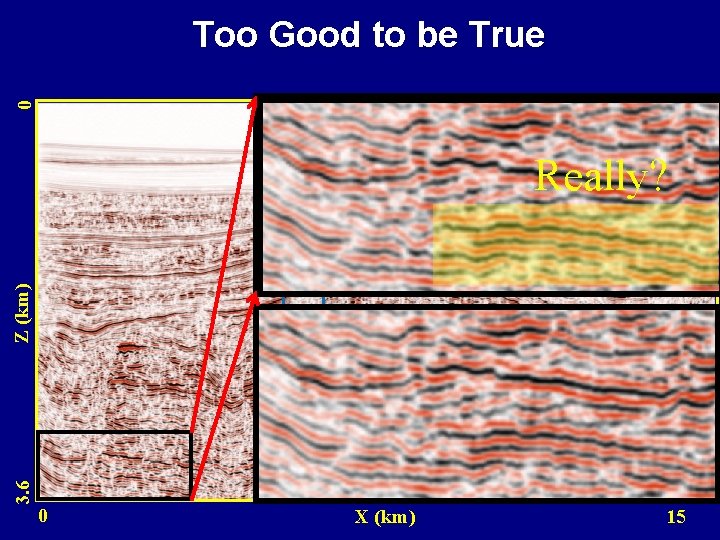
0 Too Good to be True (31 plane-wave gathers) 3. 6 Z (km) Really? 0 X (km) 15
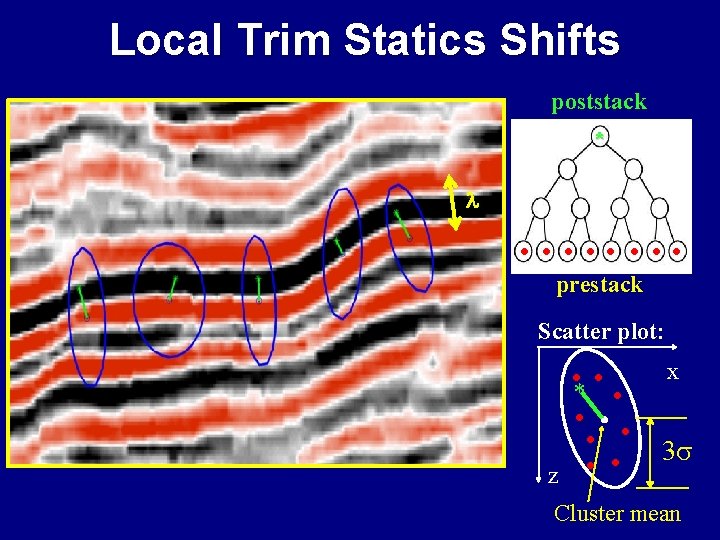
Local Trim Statics Shifts poststack * l . . . . prestack Scatter plot: . *. . . . z x 3 s Cluster mean
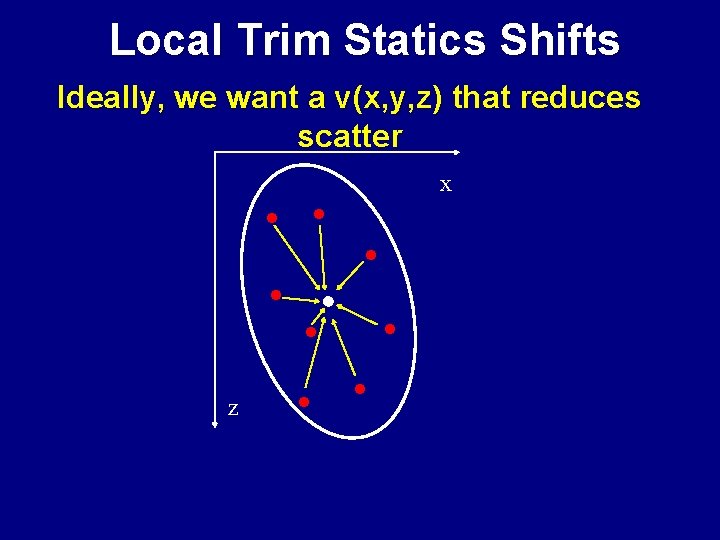
Local Trim Statics Shifts Ideally, we want a v(x, y, z) that reduces scatter z . . . . x
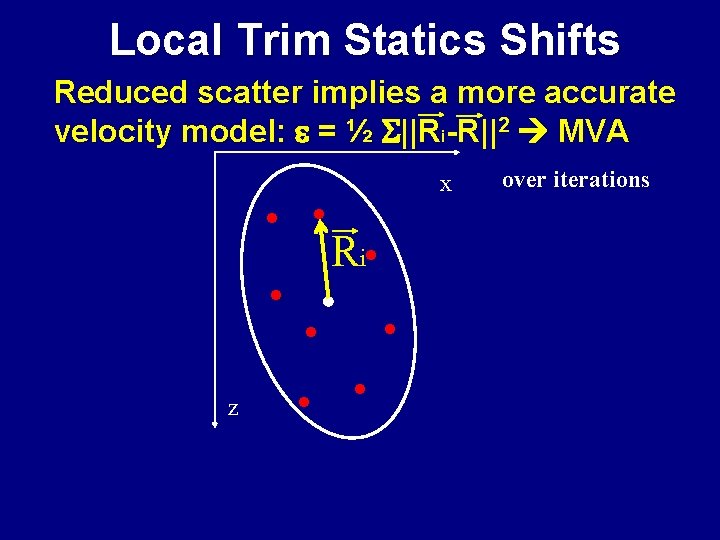
Local Trim Statics Shifts Reduced scatter implies a more accurate velocity model: e = ½ S||Ri-R||2 MVA . . R. . . i z x over iterations
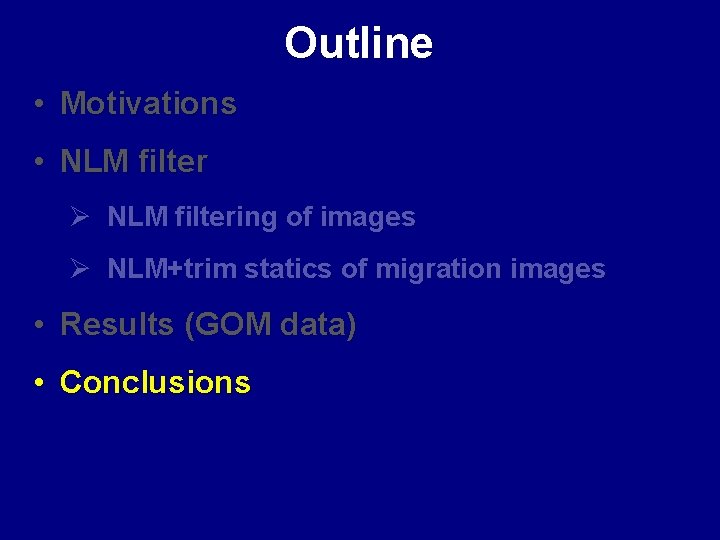
Outline • Motivations • NLM filter Ø NLM filtering of images Ø NLM+trim statics of migration images • Results (GOM data) • Conclusions
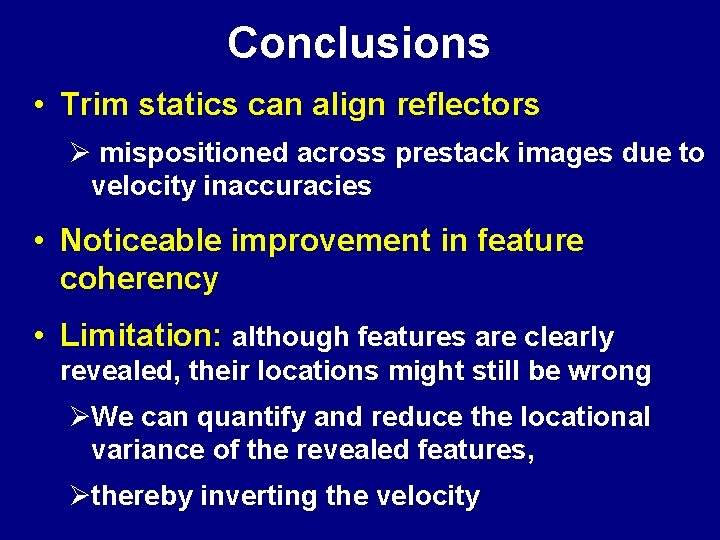
Conclusions • Trim statics can align reflectors Ø mispositioned across prestack images due to velocity inaccuracies • Noticeable improvement in feature coherency • Limitation: although features are clearly revealed, their locations might still be wrong ØWe can quantify and reduce the locational variance of the revealed features, Øthereby inverting the velocity
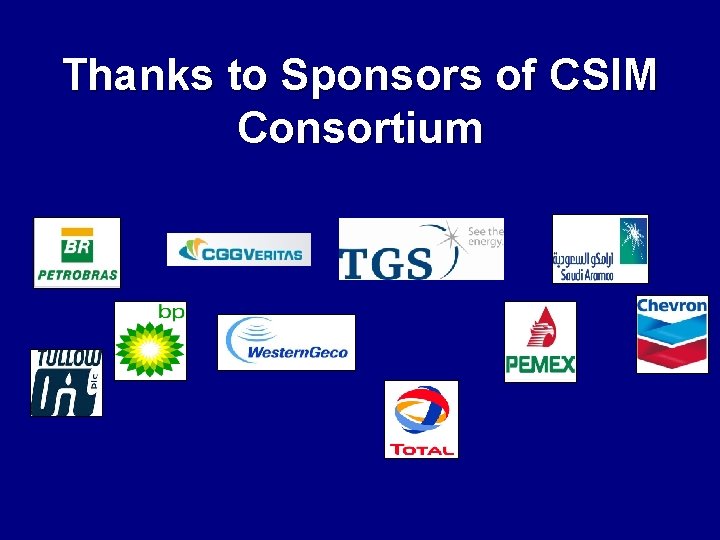
Thanks to Sponsors of CSIM Consortium
Perbedaan rapid sand filter dan slow sand filter
Blt method will convert analog filter to dt filter that has
Difference between rapid sand filter and slow sand filter
Nlm.nih.gov
Ghr.nlm.nih
Raslard def
Pubmed.ncbi.nlm.nih.gov pub
Bookshelf nlm
Ghr.nlm.nih.gov
Http://ghr.nlm.nih.gov/
Nlm
Spin quantum number of nitrogen
Ncbi.nlm.nih.gov
Kongre kütüphanesi sınıflama sistemi
Torsten iversen
Herramienta blast
Nlm min side
Nlm 10 english
Similar
Trim stata
Zfs vmware
Trim broda
Screencastify meaning
Trim and heel
Zfs structure