Multilevel Models 4 Sociology 8811 Class 26 Copyright
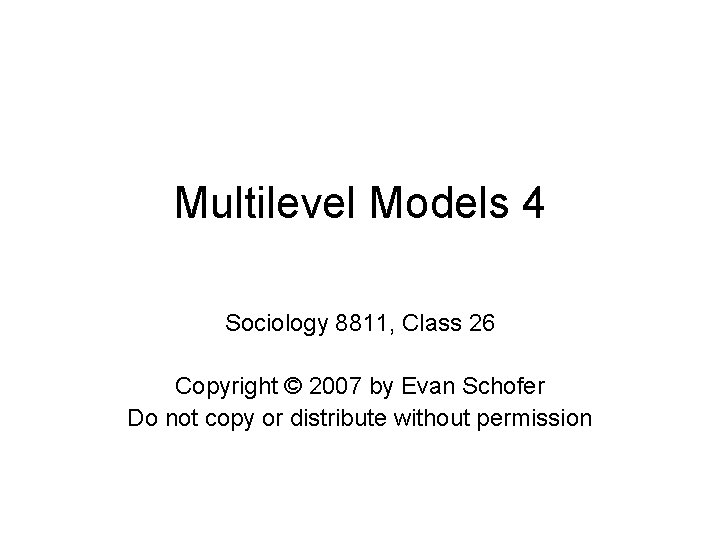
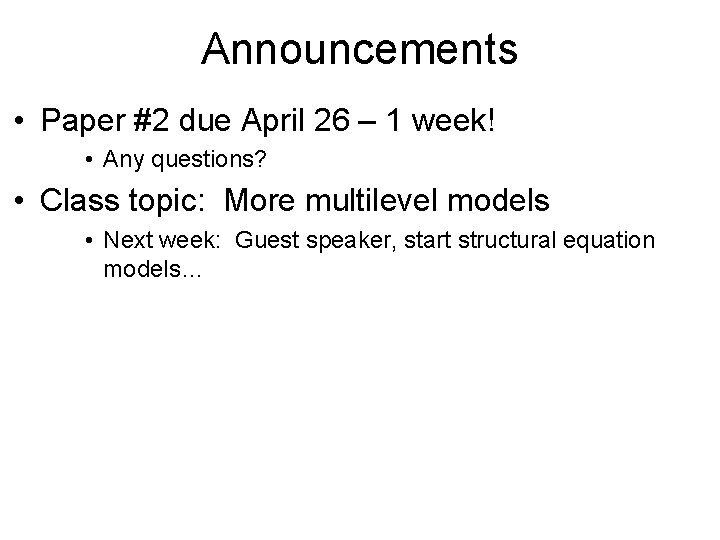
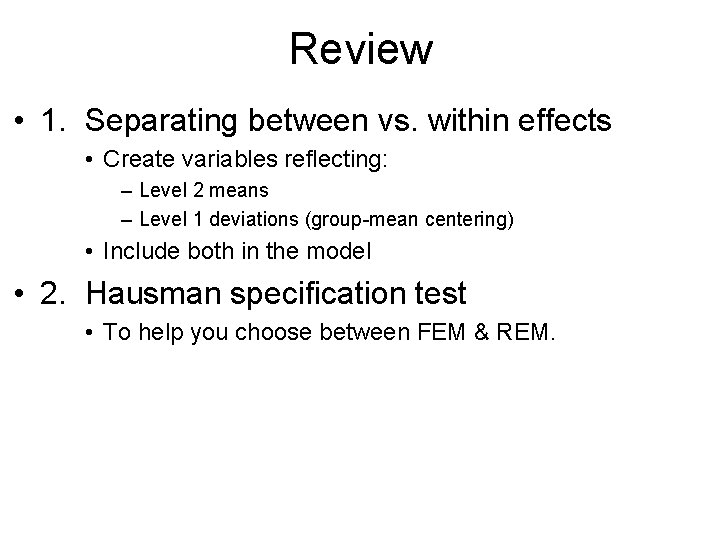
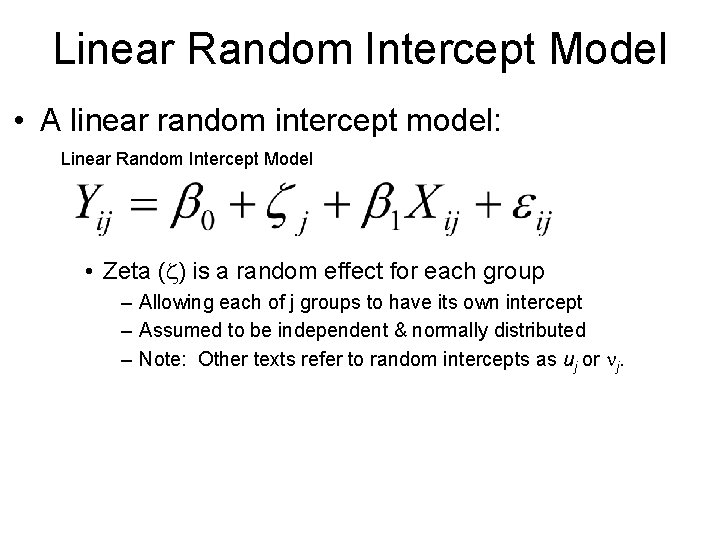
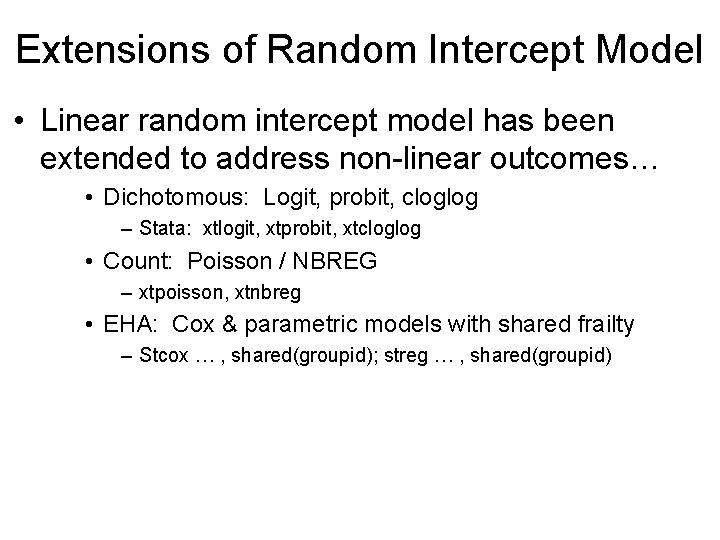
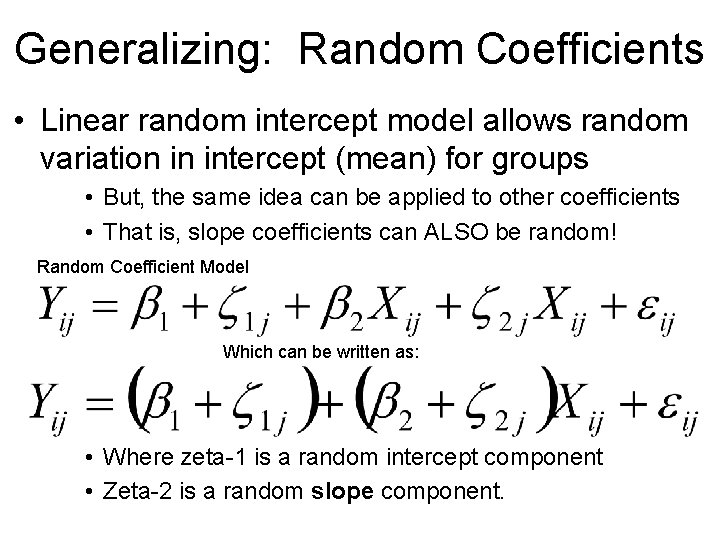
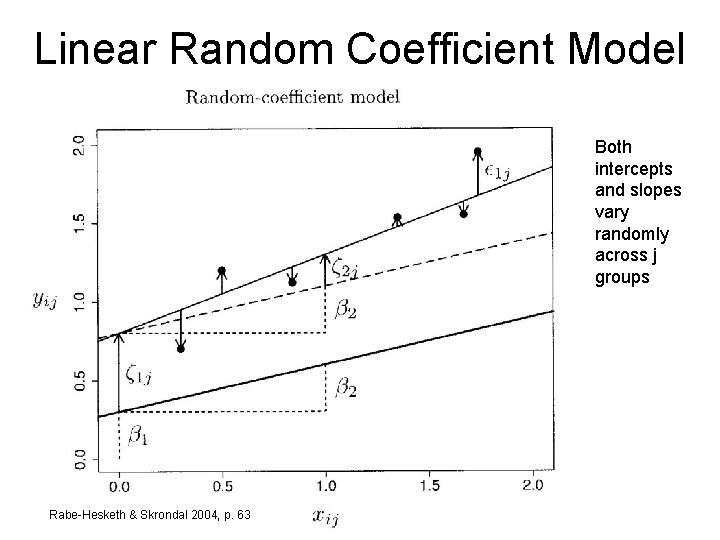
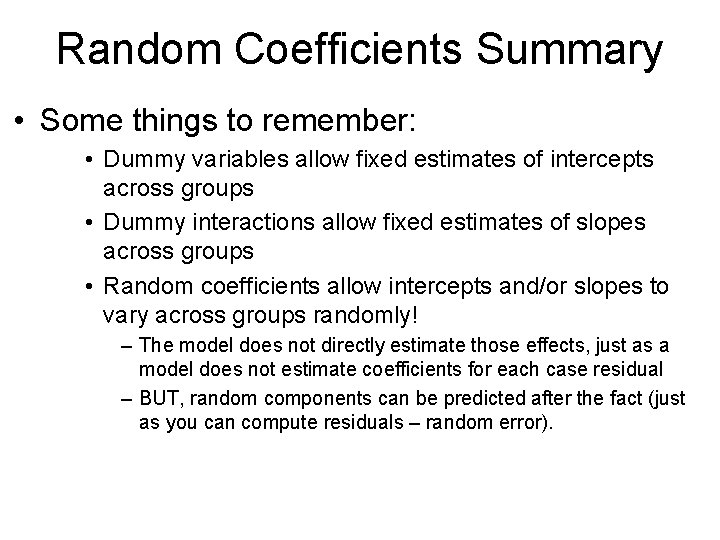
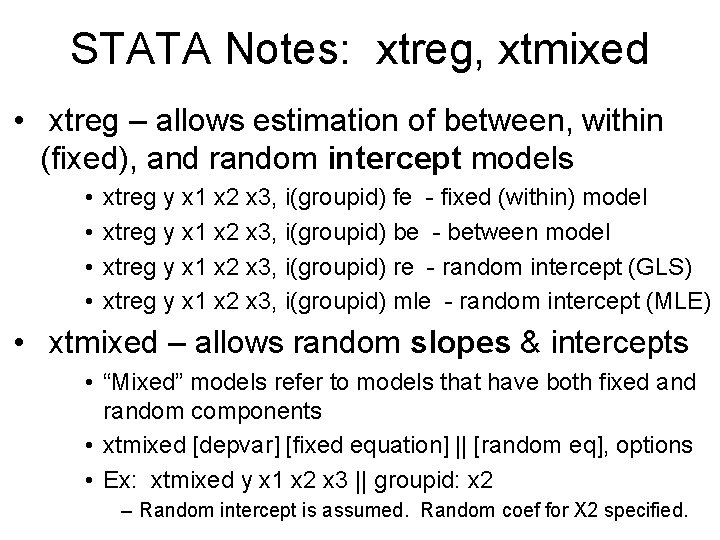
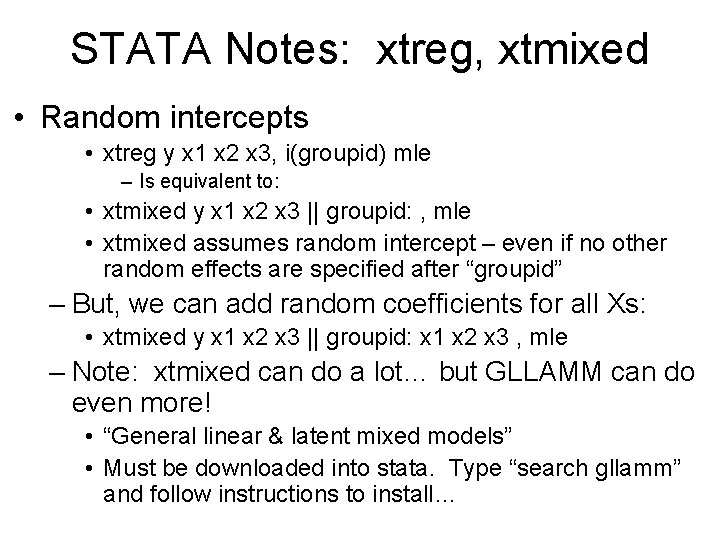
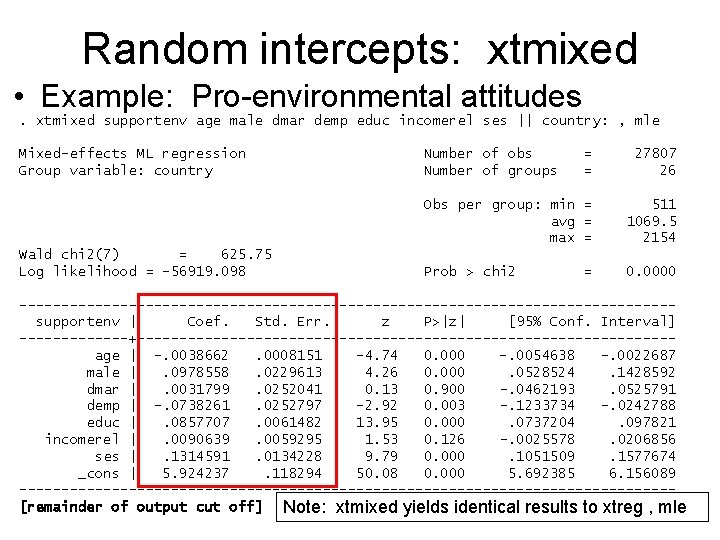
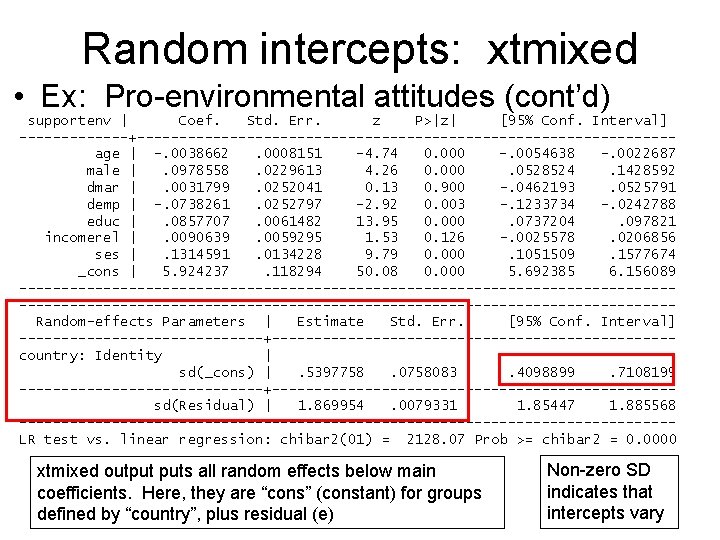
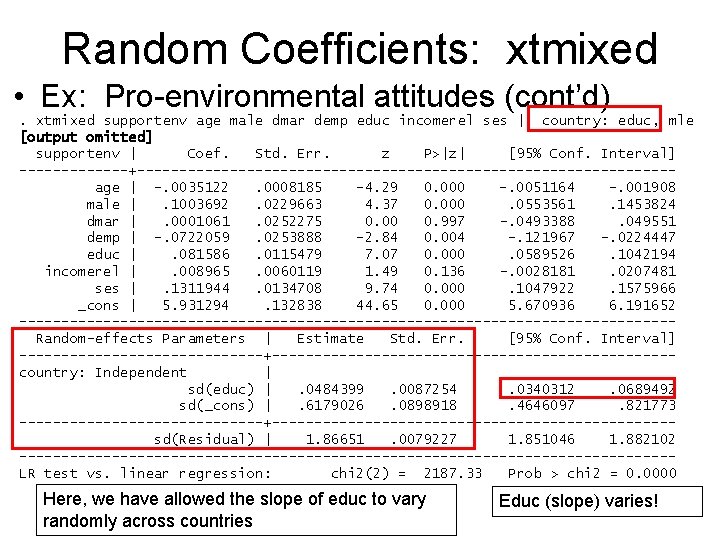
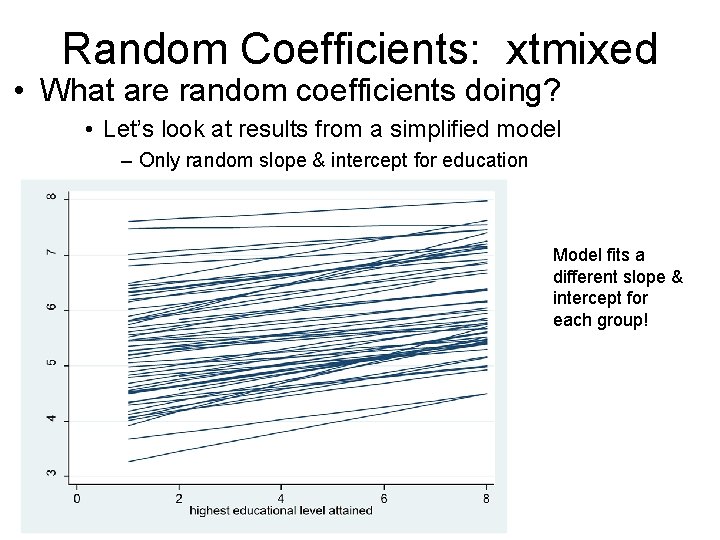
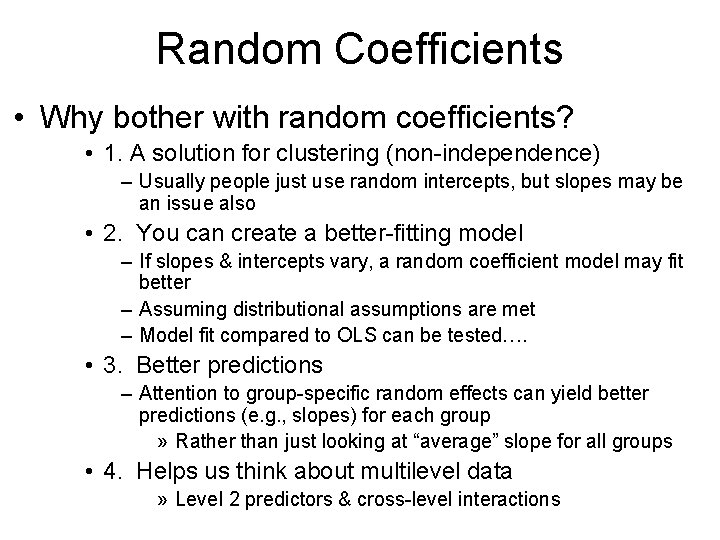
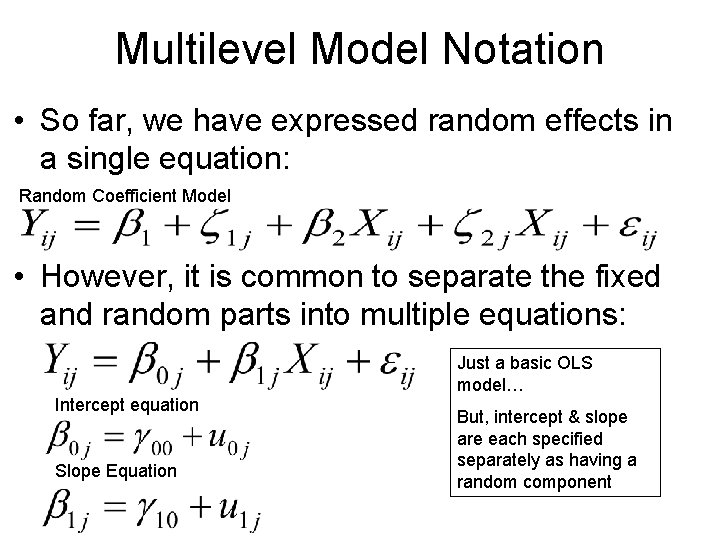
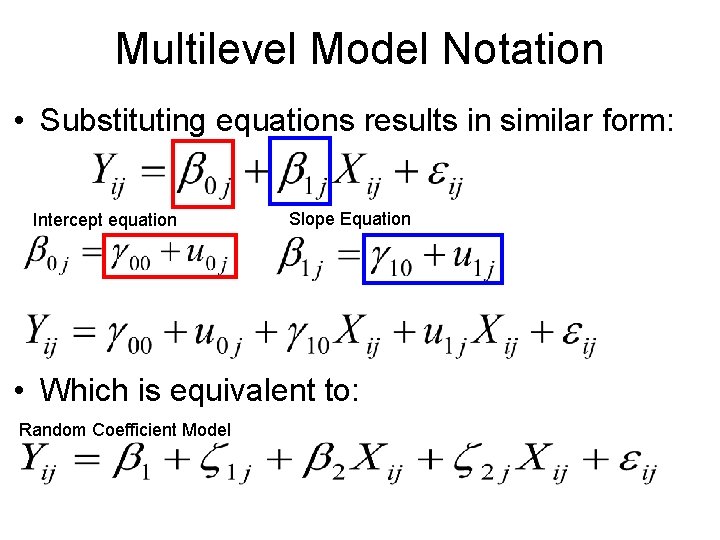
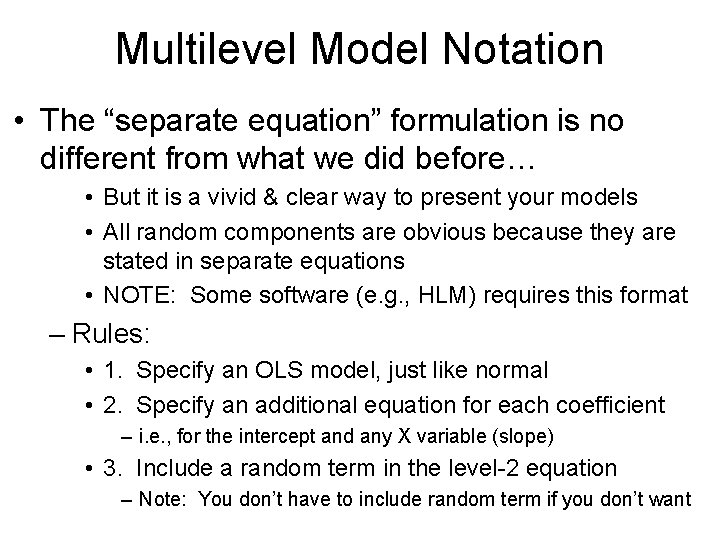
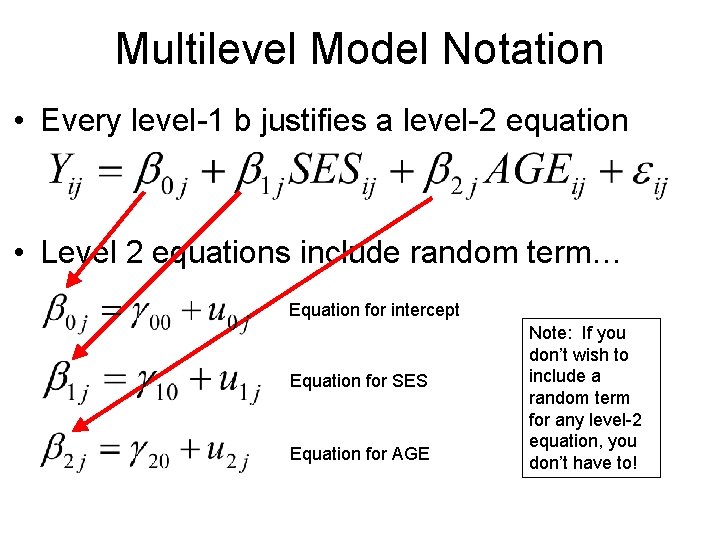
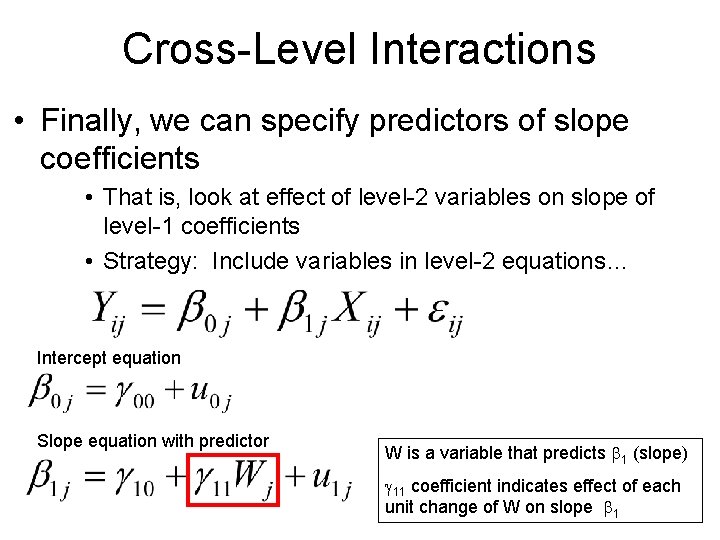
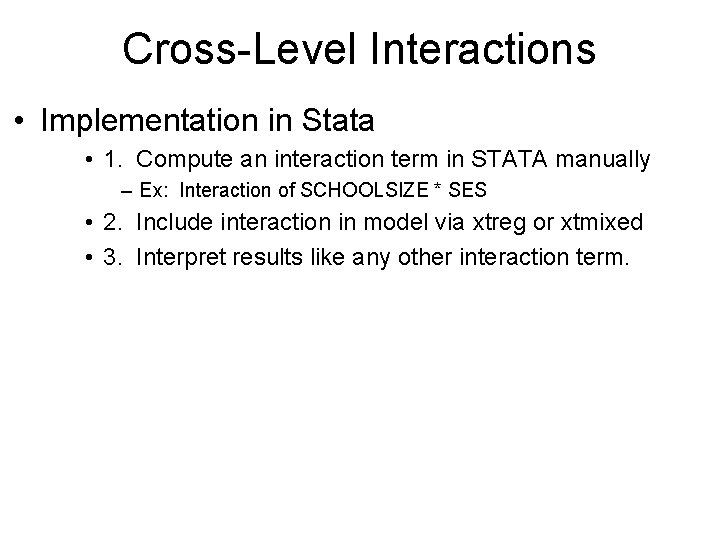
- Slides: 21
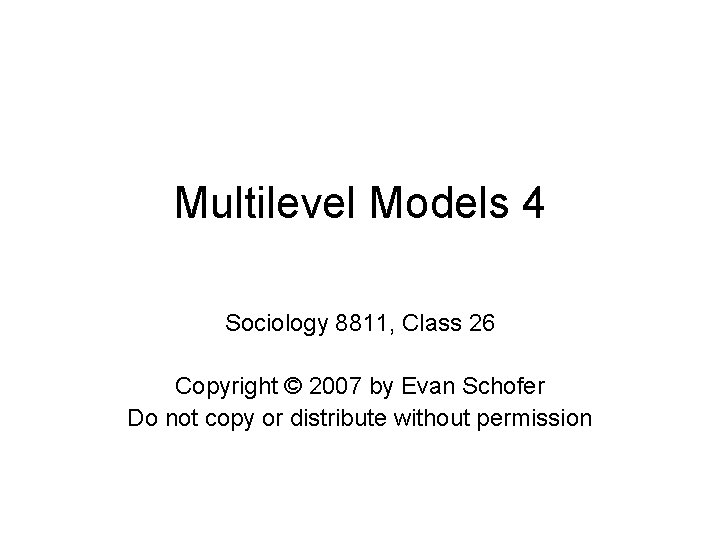
Multilevel Models 4 Sociology 8811, Class 26 Copyright © 2007 by Evan Schofer Do not copy or distribute without permission
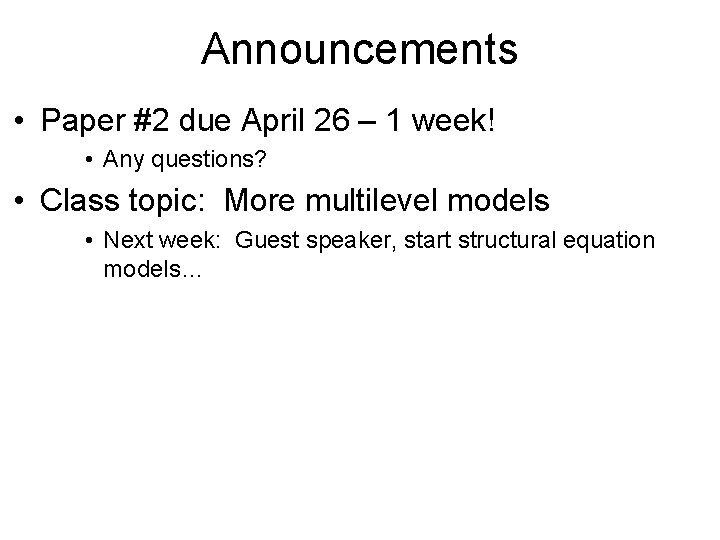
Announcements • Paper #2 due April 26 – 1 week! • Any questions? • Class topic: More multilevel models • Next week: Guest speaker, start structural equation models…
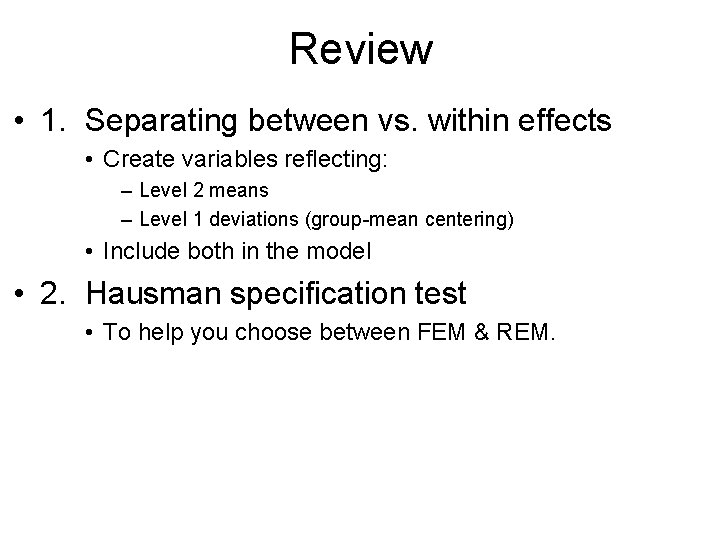
Review • 1. Separating between vs. within effects • Create variables reflecting: – Level 2 means – Level 1 deviations (group-mean centering) • Include both in the model • 2. Hausman specification test • To help you choose between FEM & REM.
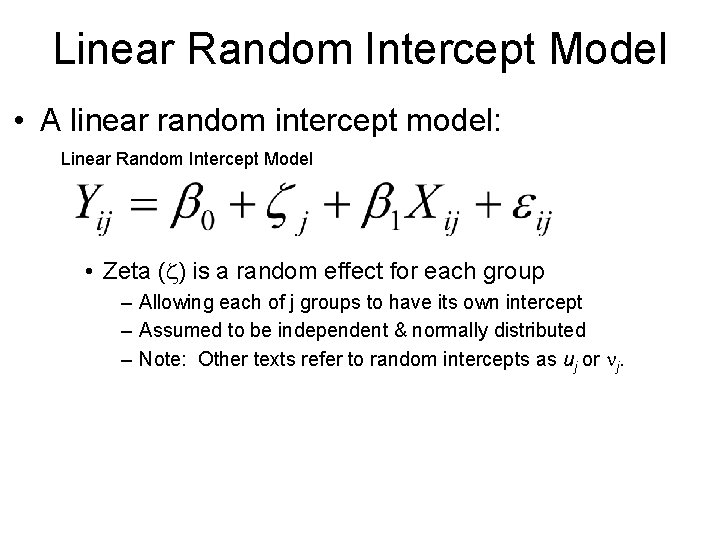
Linear Random Intercept Model • A linear random intercept model: Linear Random Intercept Model • Zeta (z) is a random effect for each group – Allowing each of j groups to have its own intercept – Assumed to be independent & normally distributed – Note: Other texts refer to random intercepts as uj or nj.
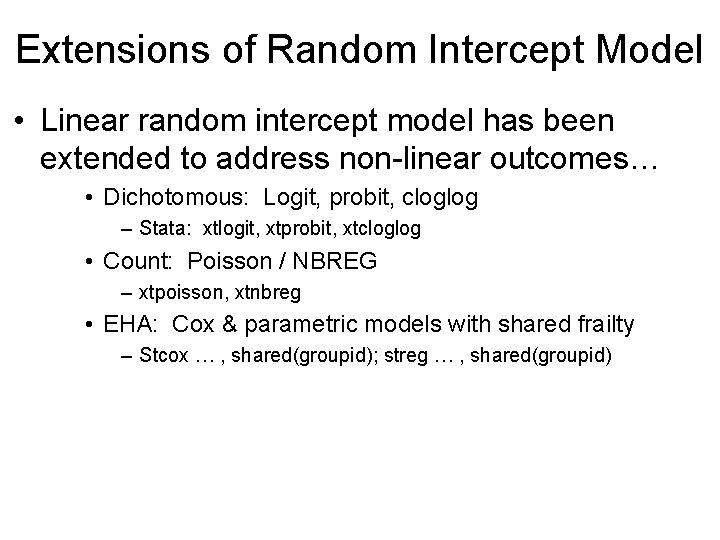
Extensions of Random Intercept Model • Linear random intercept model has been extended to address non-linear outcomes… • Dichotomous: Logit, probit, cloglog – Stata: xtlogit, xtprobit, xtcloglog • Count: Poisson / NBREG – xtpoisson, xtnbreg • EHA: Cox & parametric models with shared frailty – Stcox … , shared(groupid); streg … , shared(groupid)
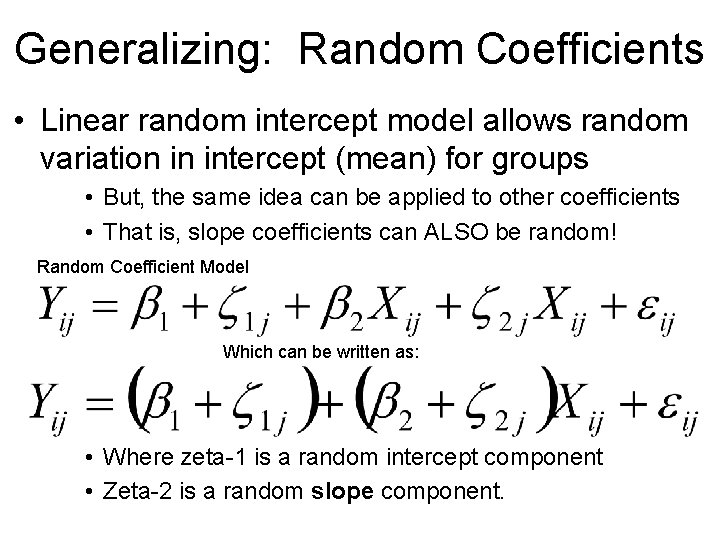
Generalizing: Random Coefficients • Linear random intercept model allows random variation in intercept (mean) for groups • But, the same idea can be applied to other coefficients • That is, slope coefficients can ALSO be random! Random Coefficient Model Which can be written as: • Where zeta-1 is a random intercept component • Zeta-2 is a random slope component.
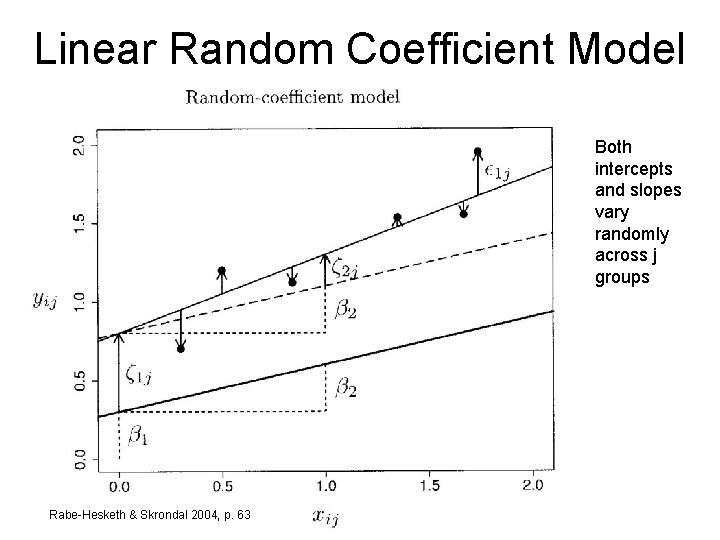
Linear Random Coefficient Model Both intercepts and slopes vary randomly across j groups Rabe-Hesketh & Skrondal 2004, p. 63
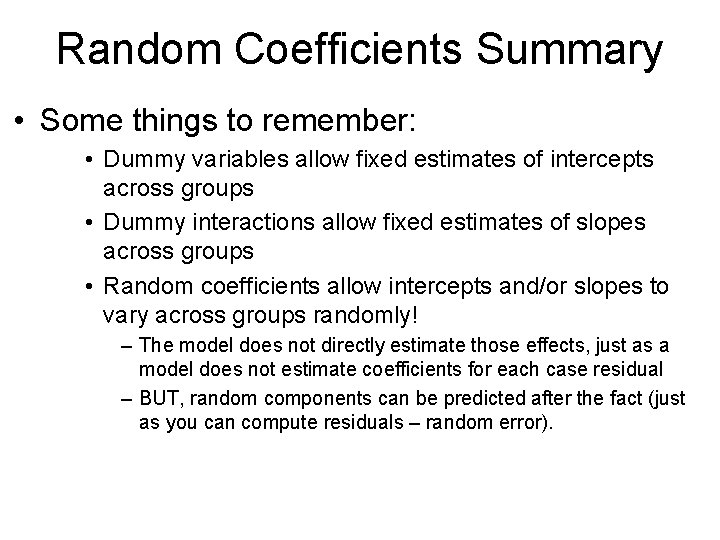
Random Coefficients Summary • Some things to remember: • Dummy variables allow fixed estimates of intercepts across groups • Dummy interactions allow fixed estimates of slopes across groups • Random coefficients allow intercepts and/or slopes to vary across groups randomly! – The model does not directly estimate those effects, just as a model does not estimate coefficients for each case residual – BUT, random components can be predicted after the fact (just as you can compute residuals – random error).
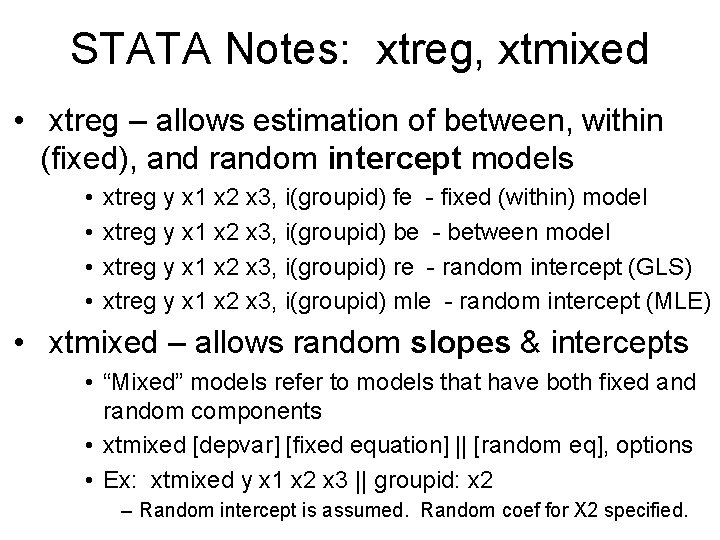
STATA Notes: xtreg, xtmixed • xtreg – allows estimation of between, within (fixed), and random intercept models • • xtreg y x 1 x 2 x 3, i(groupid) fe - fixed (within) model xtreg y x 1 x 2 x 3, i(groupid) be - between model xtreg y x 1 x 2 x 3, i(groupid) re - random intercept (GLS) xtreg y x 1 x 2 x 3, i(groupid) mle - random intercept (MLE) • xtmixed – allows random slopes & intercepts • “Mixed” models refer to models that have both fixed and random components • xtmixed [depvar] [fixed equation] || [random eq], options • Ex: xtmixed y x 1 x 2 x 3 || groupid: x 2 – Random intercept is assumed. Random coef for X 2 specified.
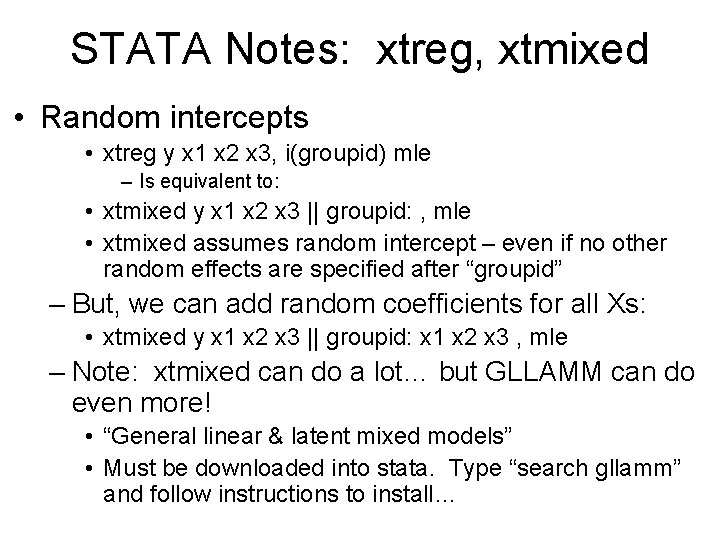
STATA Notes: xtreg, xtmixed • Random intercepts • xtreg y x 1 x 2 x 3, i(groupid) mle – Is equivalent to: • xtmixed y x 1 x 2 x 3 || groupid: , mle • xtmixed assumes random intercept – even if no other random effects are specified after “groupid” – But, we can add random coefficients for all Xs: • xtmixed y x 1 x 2 x 3 || groupid: x 1 x 2 x 3 , mle – Note: xtmixed can do a lot… but GLLAMM can do even more! • “General linear & latent mixed models” • Must be downloaded into stata. Type “search gllamm” and follow instructions to install…
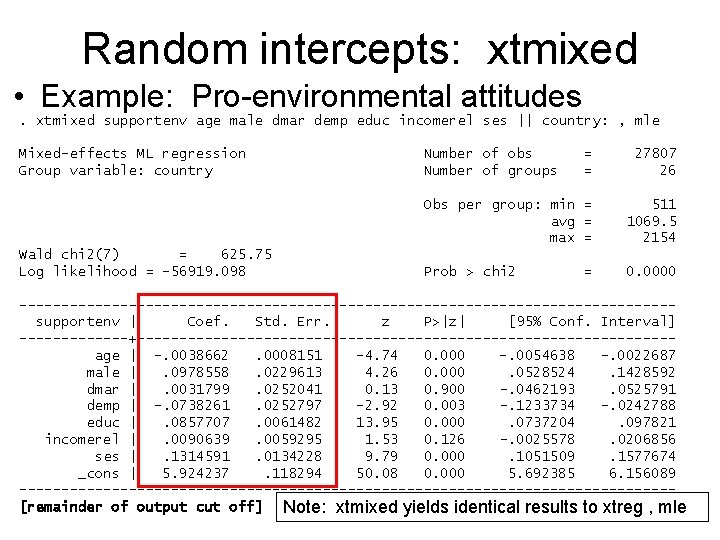
Random intercepts: xtmixed • Example: Pro-environmental attitudes. xtmixed supportenv age male dmar demp educ incomerel ses || country: , mle Mixed-effects ML regression Group variable: country Wald chi 2(7) = 625. 75 Log likelihood = -56919. 098 Number of obs Number of groups = = 27807 26 Obs per group: min = avg = max = 511 1069. 5 2154 Prob > chi 2 0. 0000 = ---------------------------------------supportenv | Coef. Std. Err. z P>|z| [95% Conf. Interval] -------+--------------------------------age | -. 0038662. 0008151 -4. 74 0. 000 -. 0054638 -. 0022687 male |. 0978558. 0229613 4. 26 0. 000. 0528524. 1428592 dmar |. 0031799. 0252041 0. 13 0. 900 -. 0462193. 0525791 demp | -. 0738261. 0252797 -2. 92 0. 003 -. 1233734 -. 0242788 educ |. 0857707. 0061482 13. 95 0. 000. 0737204. 097821 incomerel |. 0090639. 0059295 1. 53 0. 126 -. 0025578. 0206856 ses |. 1314591. 0134228 9. 79 0. 000. 1051509. 1577674 _cons | 5. 924237. 118294 50. 08 0. 000 5. 692385 6. 156089 ---------------------------------------[remainder of output cut off] Note: xtmixed yields identical results to xtreg , mle
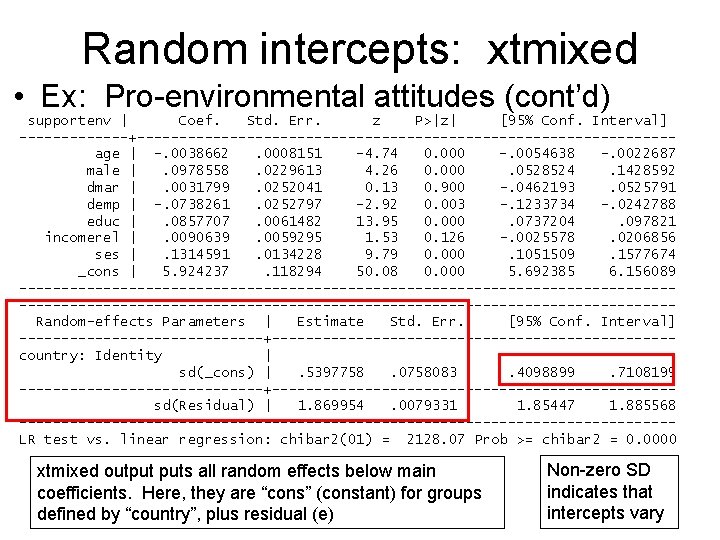
Random intercepts: xtmixed • Ex: Pro-environmental attitudes (cont’d) supportenv | Coef. Std. Err. z P>|z| [95% Conf. Interval] -------+--------------------------------age | -. 0038662. 0008151 -4. 74 0. 000 -. 0054638 -. 0022687 male |. 0978558. 0229613 4. 26 0. 000. 0528524. 1428592 dmar |. 0031799. 0252041 0. 13 0. 900 -. 0462193. 0525791 demp | -. 0738261. 0252797 -2. 92 0. 003 -. 1233734 -. 0242788 educ |. 0857707. 0061482 13. 95 0. 000. 0737204. 097821 incomerel |. 0090639. 0059295 1. 53 0. 126 -. 0025578. 0206856 ses |. 1314591. 0134228 9. 79 0. 000. 1051509. 1577674 _cons | 5. 924237. 118294 50. 08 0. 000 5. 692385 6. 156089 -----------------------------------------------------------------------------Random-effects Parameters | Estimate Std. Err. [95% Conf. Interval] ---------------+------------------------country: Identity | sd(_cons) |. 5397758. 0758083. 4098899. 7108199 ---------------+------------------------sd(Residual) | 1. 869954. 0079331 1. 85447 1. 885568 ---------------------------------------LR test vs. linear regression: chibar 2(01) = 2128. 07 Prob >= chibar 2 = 0. 0000 xtmixed output puts all random effects below main coefficients. Here, they are “cons” (constant) for groups defined by “country”, plus residual (e) Non-zero SD indicates that intercepts vary
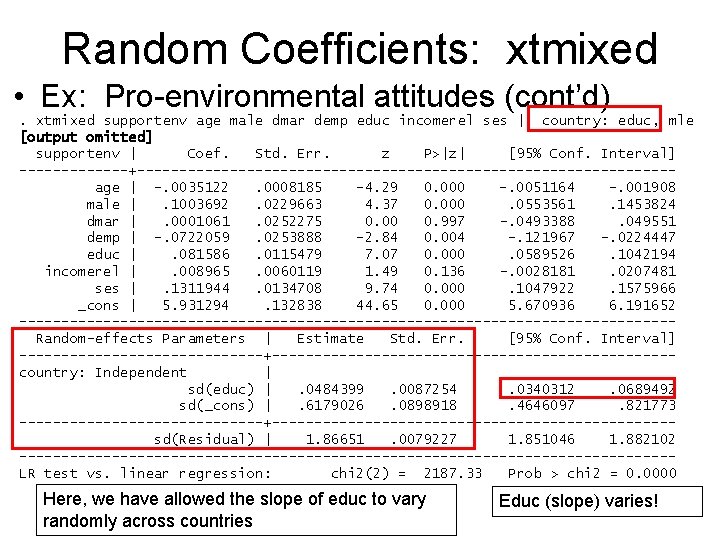
Random Coefficients: xtmixed • Ex: Pro-environmental attitudes (cont’d). xtmixed supportenv age male dmar demp educ incomerel ses || country: educ, mle [output omitted] supportenv | Coef. Std. Err. z P>|z| [95% Conf. Interval] -------+--------------------------------age | -. 0035122. 0008185 -4. 29 0. 000 -. 0051164 -. 001908 male |. 1003692. 0229663 4. 37 0. 000. 0553561. 1453824 dmar |. 0001061. 0252275 0. 00 0. 997 -. 0493388. 049551 demp | -. 0722059. 0253888 -2. 84 0. 004 -. 121967 -. 0224447 educ |. 081586. 0115479 7. 07 0. 000. 0589526. 1042194 incomerel |. 008965. 0060119 1. 49 0. 136 -. 0028181. 0207481 ses |. 1311944. 0134708 9. 74 0. 000. 1047922. 1575966 _cons | 5. 931294. 132838 44. 65 0. 000 5. 670936 6. 191652 ---------------------------------------Random-effects Parameters | Estimate Std. Err. [95% Conf. Interval] ---------------+------------------------country: Independent | sd(educ) |. 0484399. 0087254. 0340312. 0689492 sd(_cons) |. 6179026. 0898918. 4646097. 821773 ---------------+------------------------sd(Residual) | 1. 86651. 0079227 1. 851046 1. 882102 ---------------------------------------LR test vs. linear regression: chi 2(2) = 2187. 33 Prob > chi 2 = 0. 0000 Here, we have allowed the slope of educ to vary randomly across countries Educ (slope) varies!
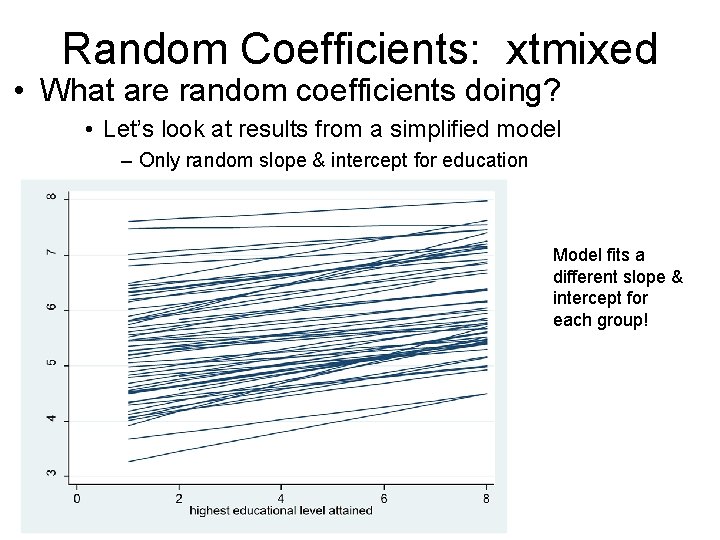
Random Coefficients: xtmixed • What are random coefficients doing? • Let’s look at results from a simplified model – Only random slope & intercept for education Model fits a different slope & intercept for each group!
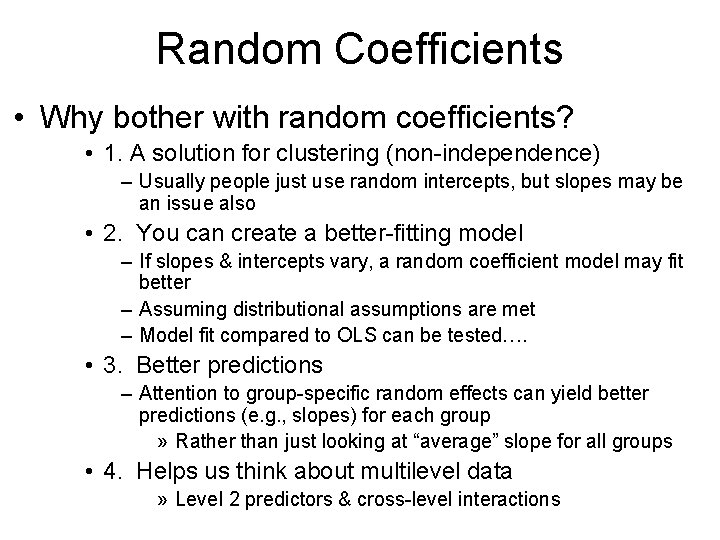
Random Coefficients • Why bother with random coefficients? • 1. A solution for clustering (non-independence) – Usually people just use random intercepts, but slopes may be an issue also • 2. You can create a better-fitting model – If slopes & intercepts vary, a random coefficient model may fit better – Assuming distributional assumptions are met – Model fit compared to OLS can be tested…. • 3. Better predictions – Attention to group-specific random effects can yield better predictions (e. g. , slopes) for each group » Rather than just looking at “average” slope for all groups • 4. Helps us think about multilevel data » Level 2 predictors & cross-level interactions
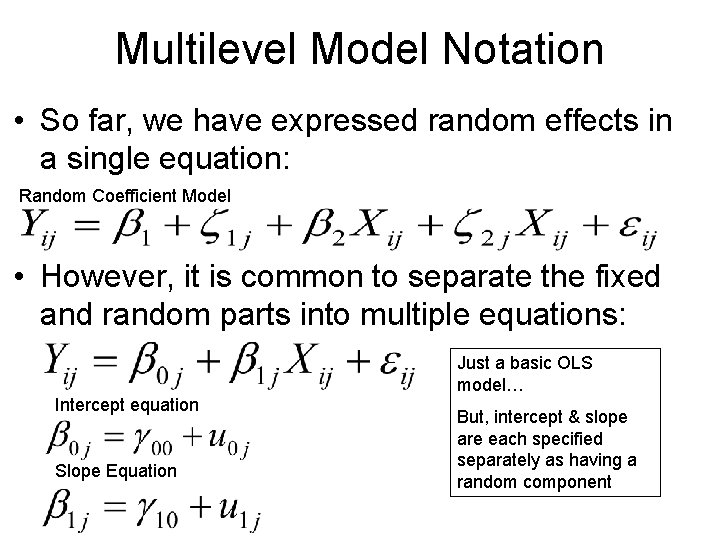
Multilevel Model Notation • So far, we have expressed random effects in a single equation: Random Coefficient Model • However, it is common to separate the fixed and random parts into multiple equations: Just a basic OLS model… Intercept equation Slope Equation But, intercept & slope are each specified separately as having a random component
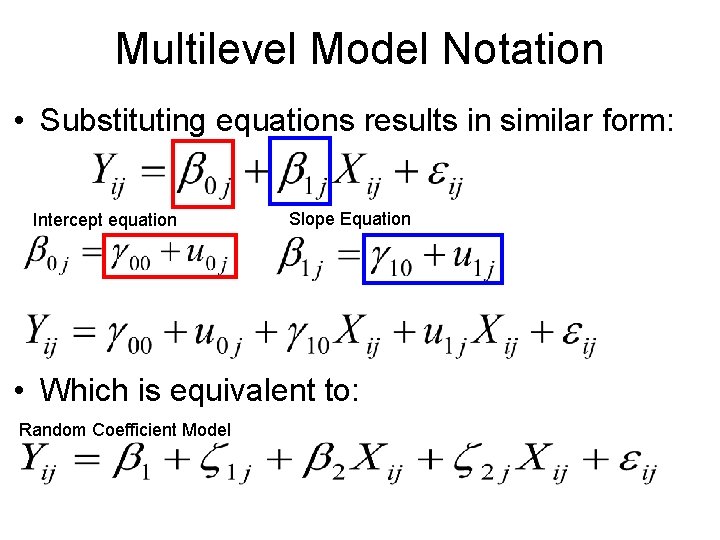
Multilevel Model Notation • Substituting equations results in similar form: Intercept equation Slope Equation • Which is equivalent to: Random Coefficient Model
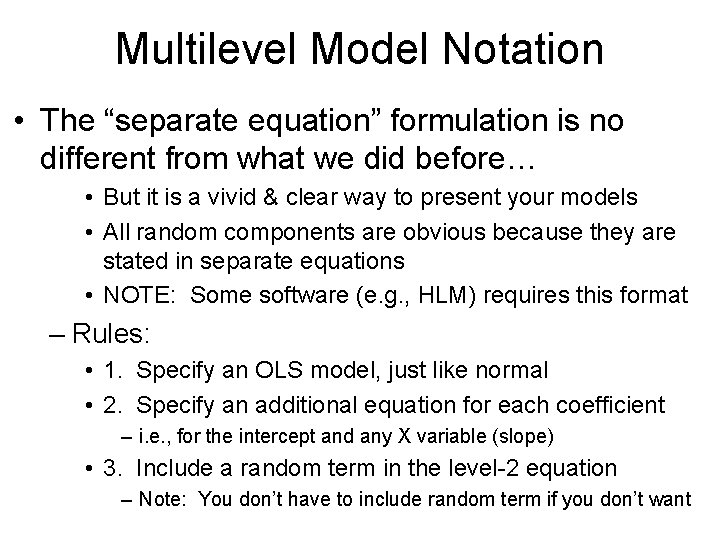
Multilevel Model Notation • The “separate equation” formulation is no different from what we did before… • But it is a vivid & clear way to present your models • All random components are obvious because they are stated in separate equations • NOTE: Some software (e. g. , HLM) requires this format – Rules: • 1. Specify an OLS model, just like normal • 2. Specify an additional equation for each coefficient – i. e. , for the intercept and any X variable (slope) • 3. Include a random term in the level-2 equation – Note: You don’t have to include random term if you don’t want
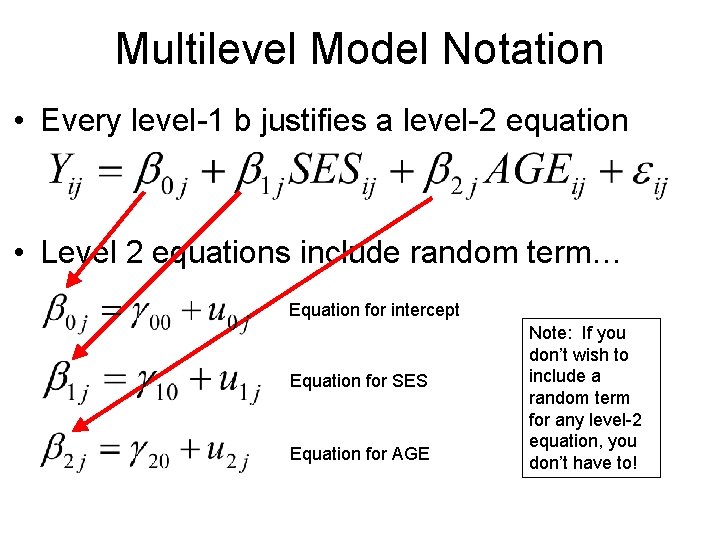
Multilevel Model Notation • Every level-1 b justifies a level-2 equation • Level 2 equations include random term… Equation for intercept Equation for SES Equation for AGE Note: If you don’t wish to include a random term for any level-2 equation, you don’t have to!
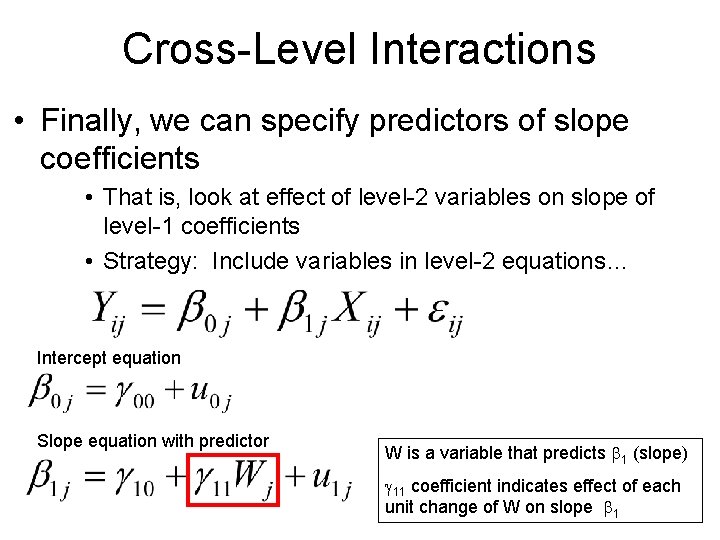
Cross-Level Interactions • Finally, we can specify predictors of slope coefficients • That is, look at effect of level-2 variables on slope of level-1 coefficients • Strategy: Include variables in level-2 equations… Intercept equation Slope equation with predictor W is a variable that predicts b 1 (slope) g 11 coefficient indicates effect of each unit change of W on slope b 1
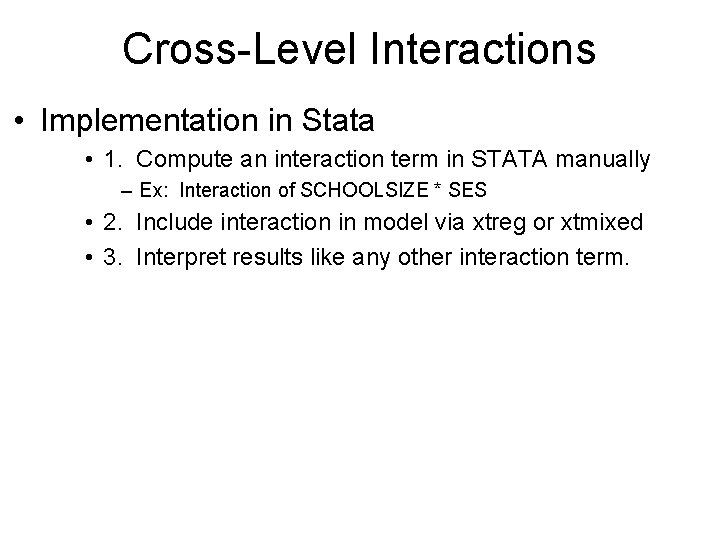
Cross-Level Interactions • Implementation in Stata • 1. Compute an interaction term in STATA manually – Ex: Interaction of SCHOOLSIZE * SES • 2. Include interaction in model via xtreg or xtmixed • 3. Interpret results like any other interaction term.
Advanced regression and multilevel models
Multi level security example
Difference between modal and semi modal verbs
Multilevel page table
Teaching multilevel esl classes
Multilevel feedback queue
Multilevel model equation example
Multilevel model equation example
Multilevel paging in os
Multilevel bus architecture in embedded systems
Multilevel page tables
Process virtual address space
Multilevel modeling in spss
Mfq scheduling
Multilevel indexing
Miller indices visualizer
Multilevel nand gate
Inheritance adalah
Multilevel instruction
Contoh multilevel inheritance
Multiplexing and spreading
Multilevel instruction