The Twointercept Approach in Multilevel Modeling with SPSS
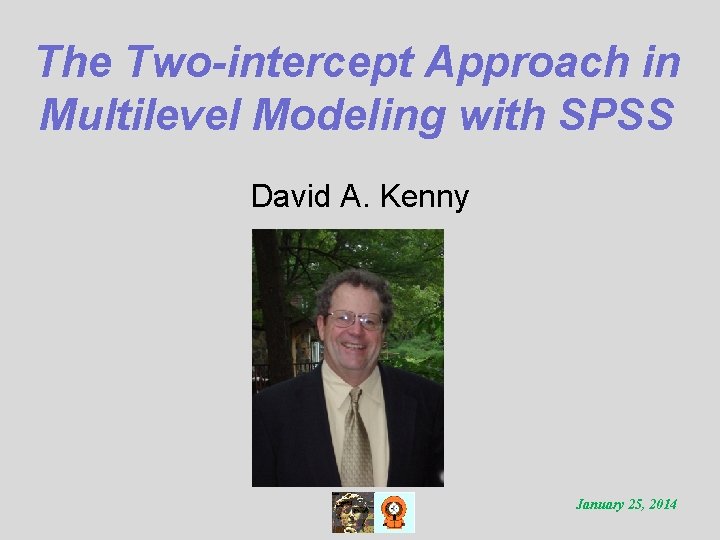
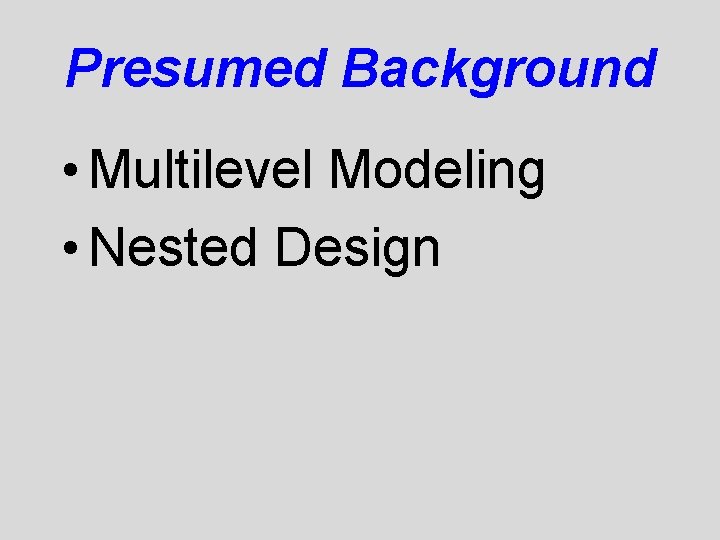
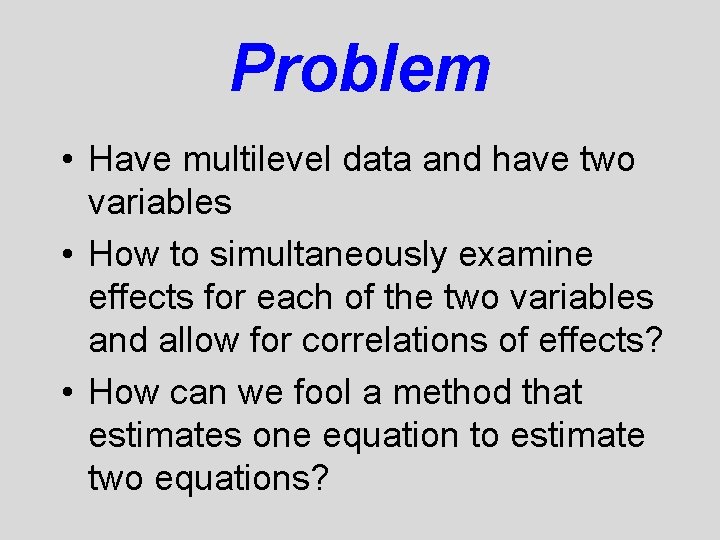
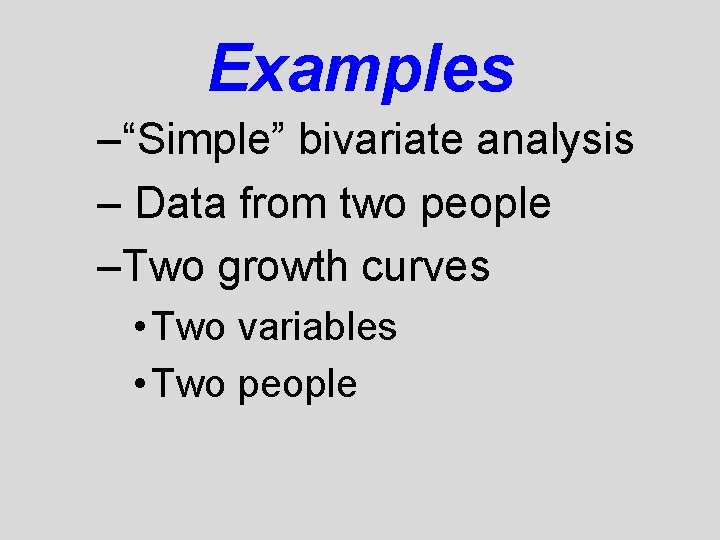
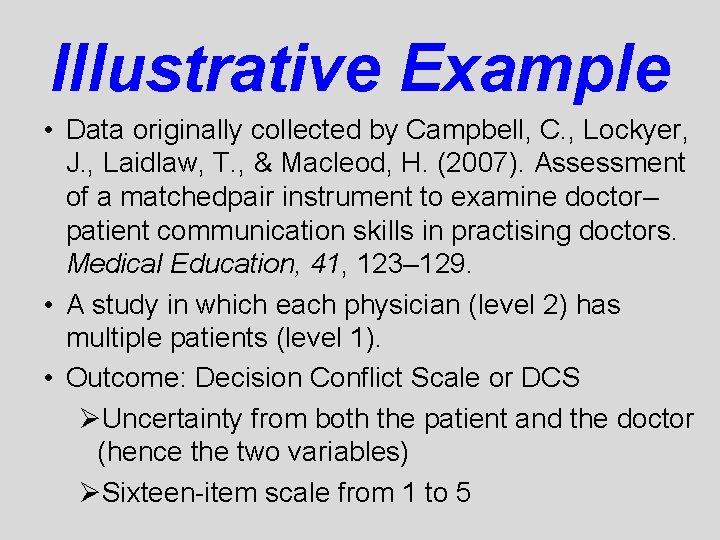
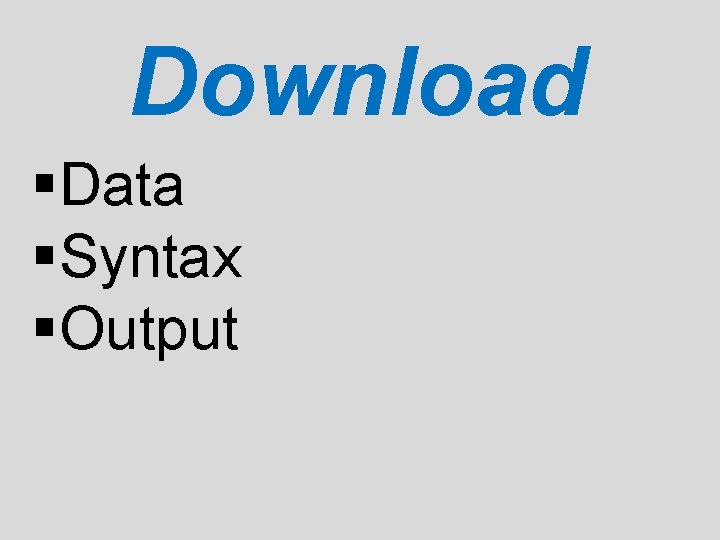
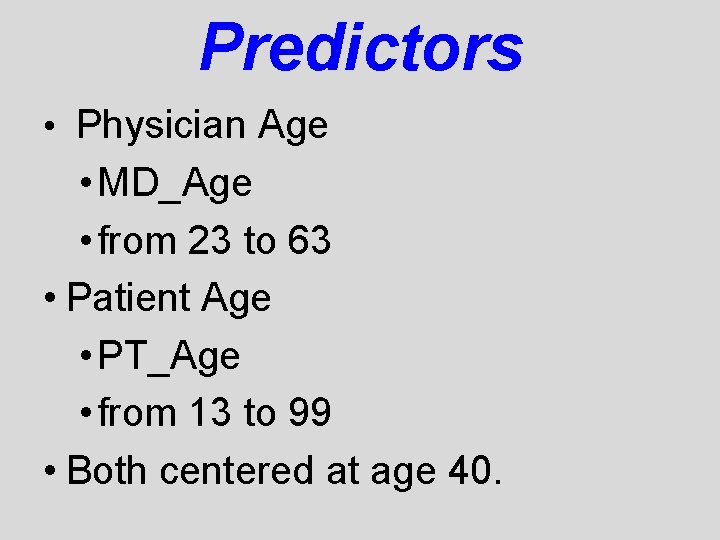
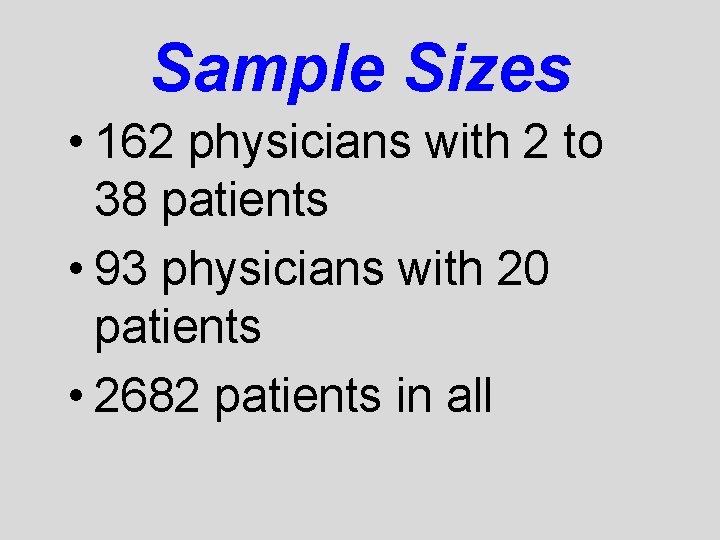
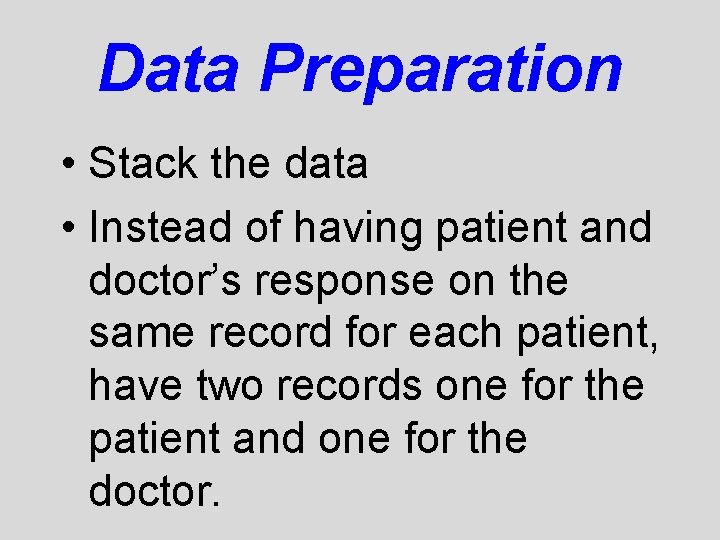
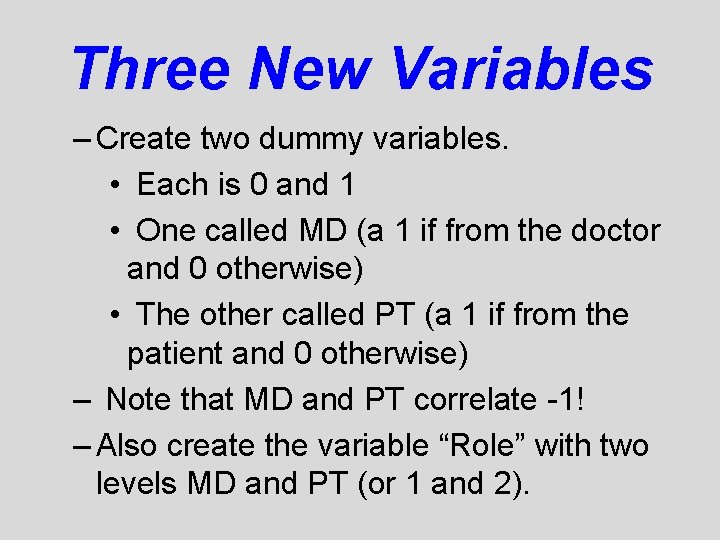
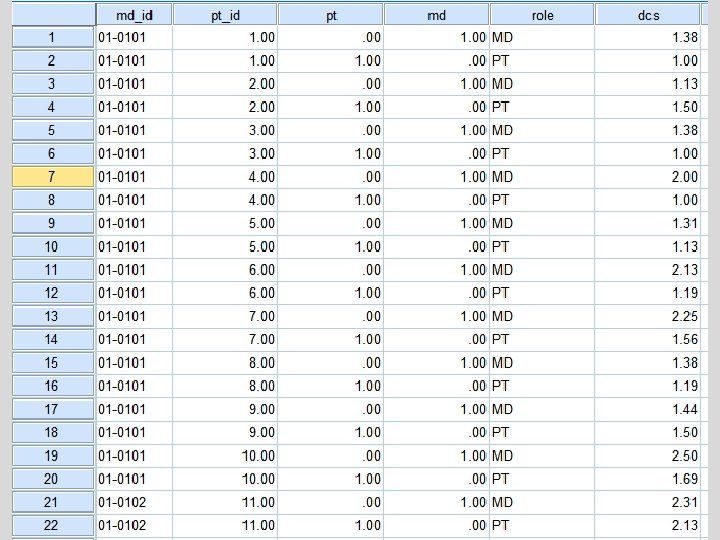
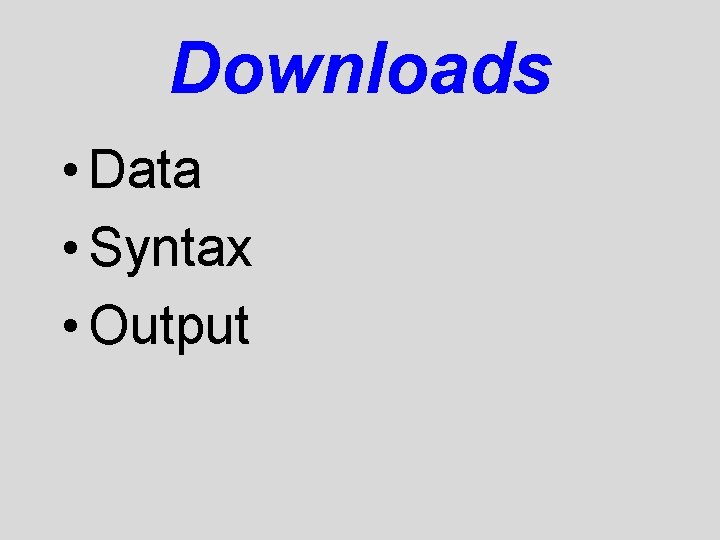
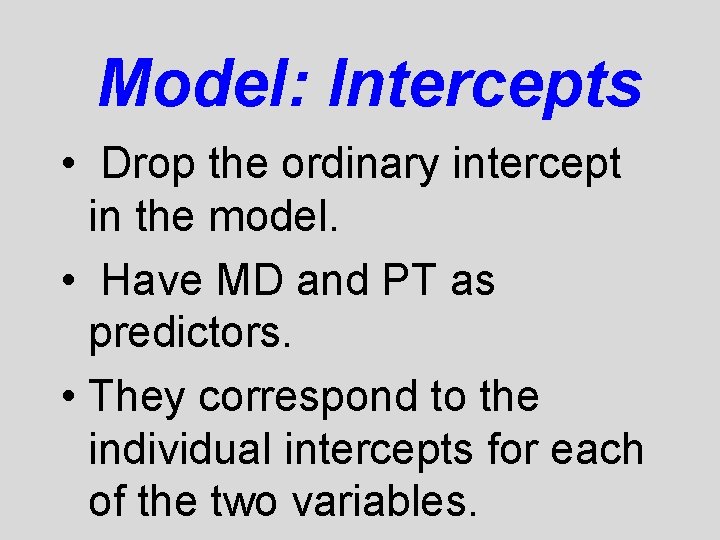
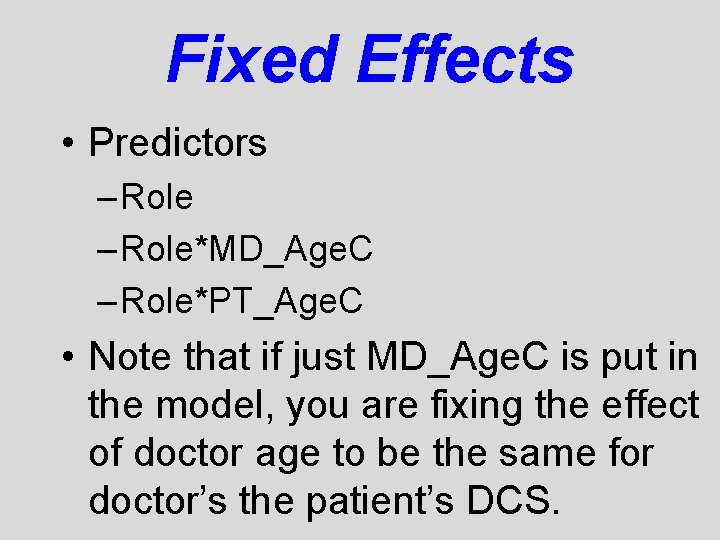
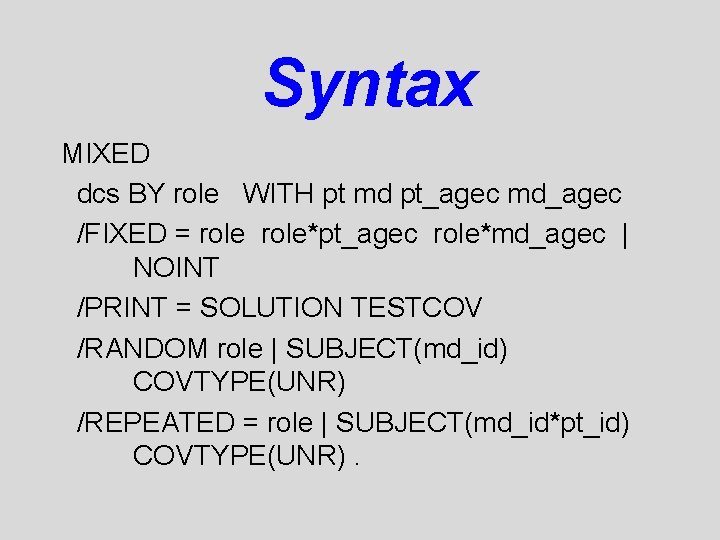
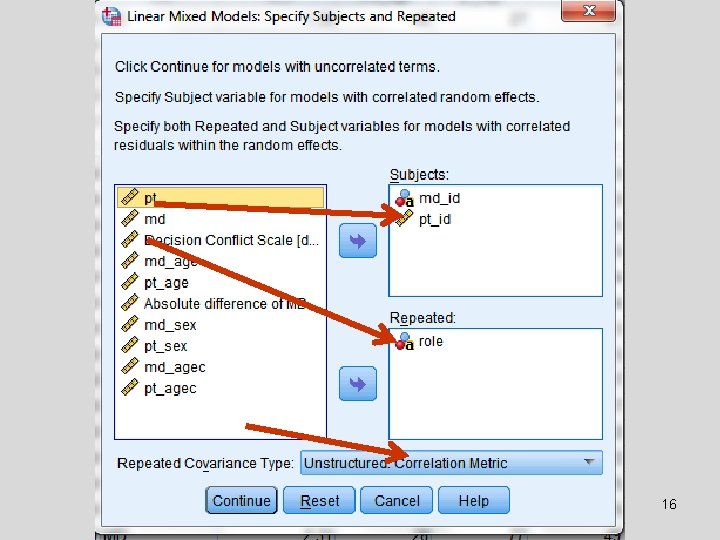
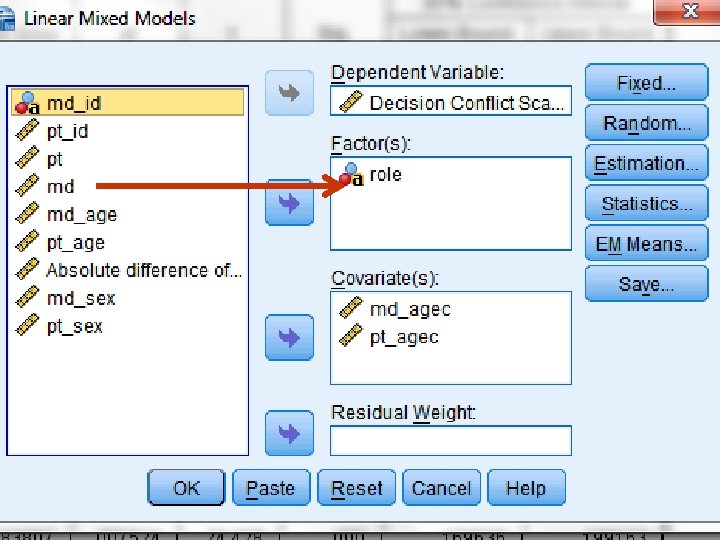
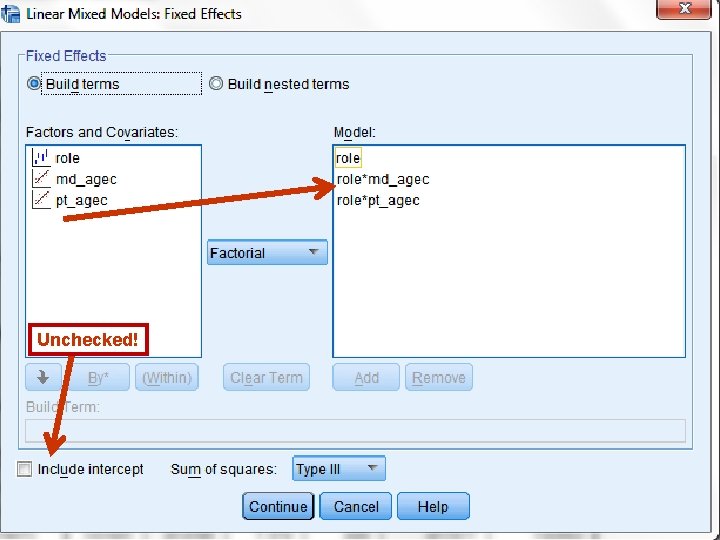
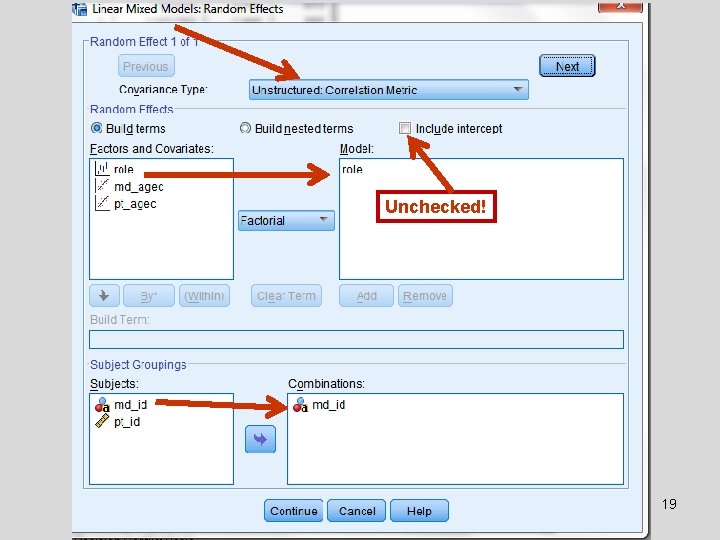
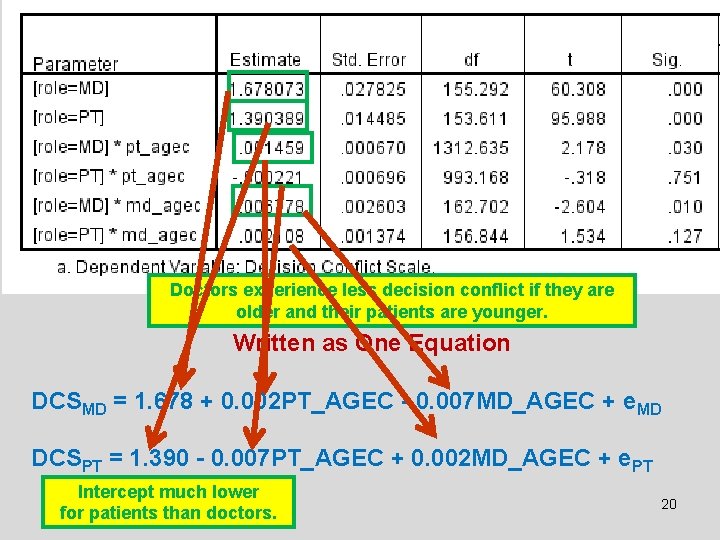
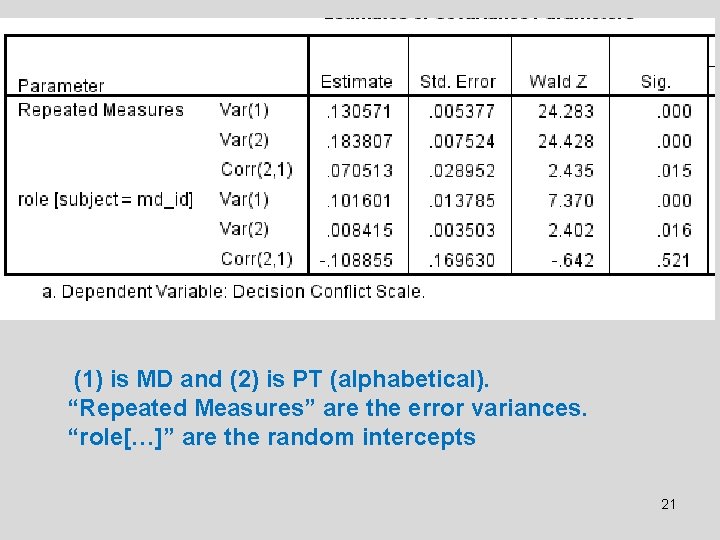
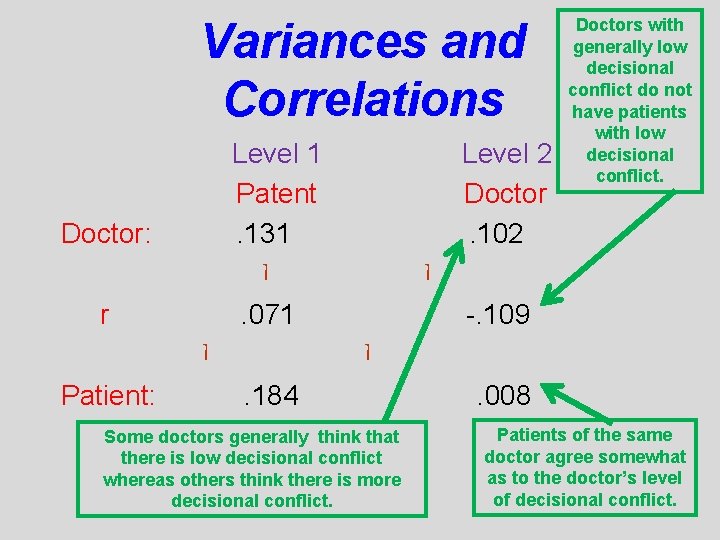
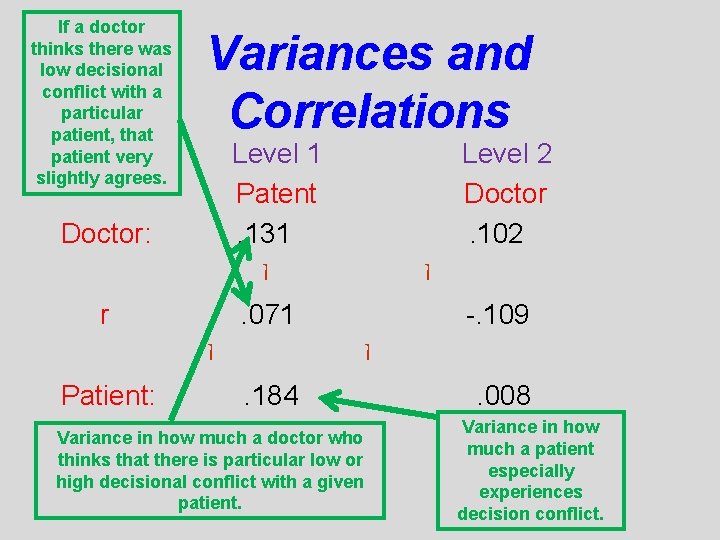
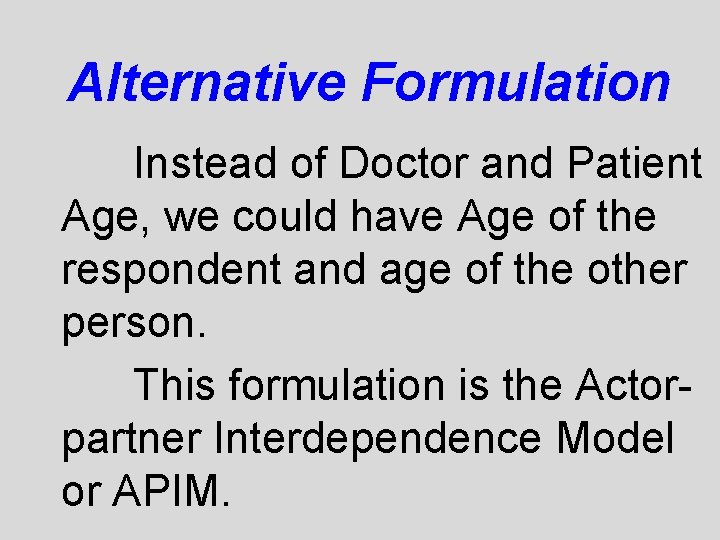
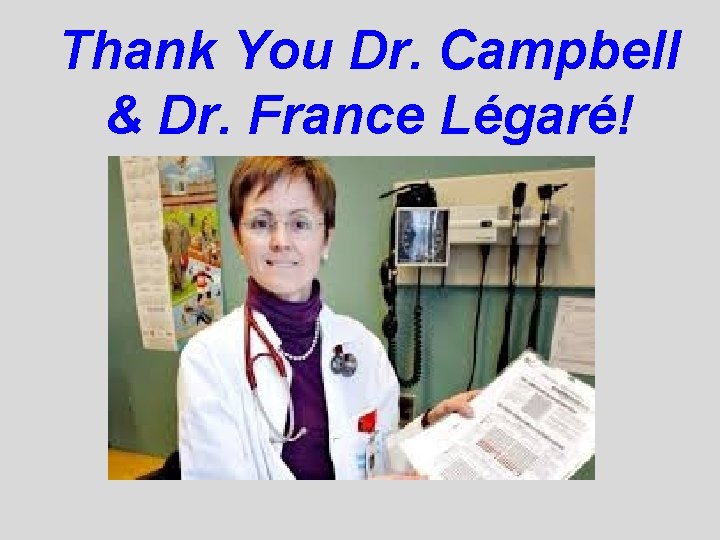
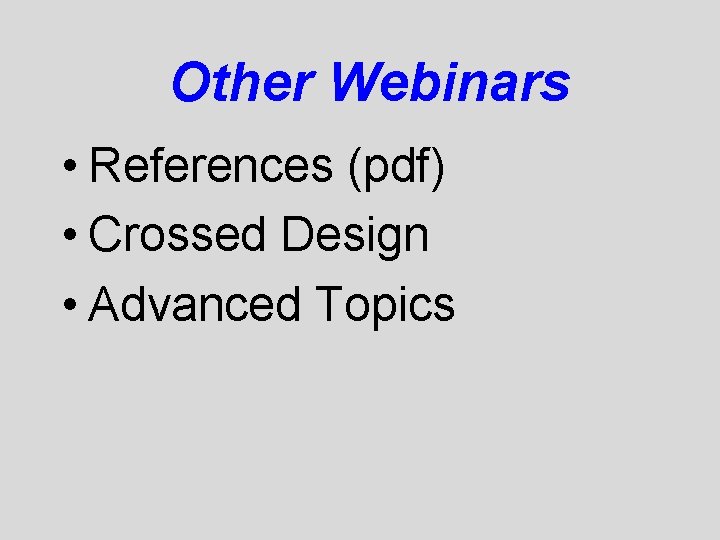
- Slides: 26
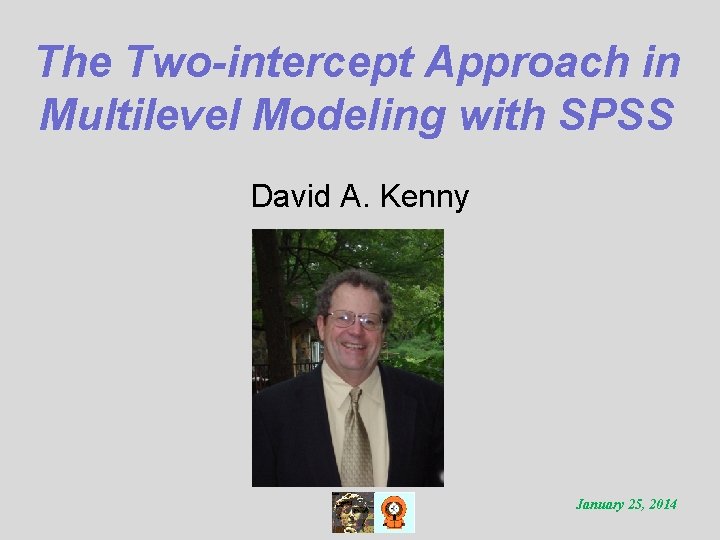
The Two-intercept Approach in Multilevel Modeling with SPSS David A. Kenny January 25, 2014
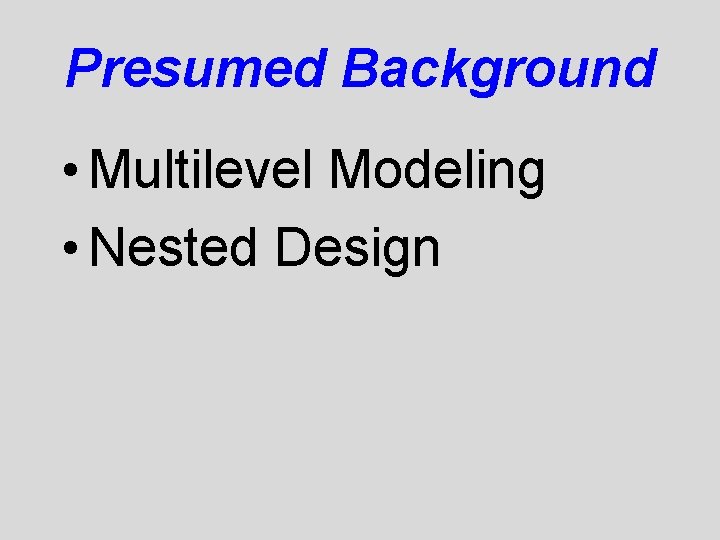
Presumed Background • Multilevel Modeling • Nested Design
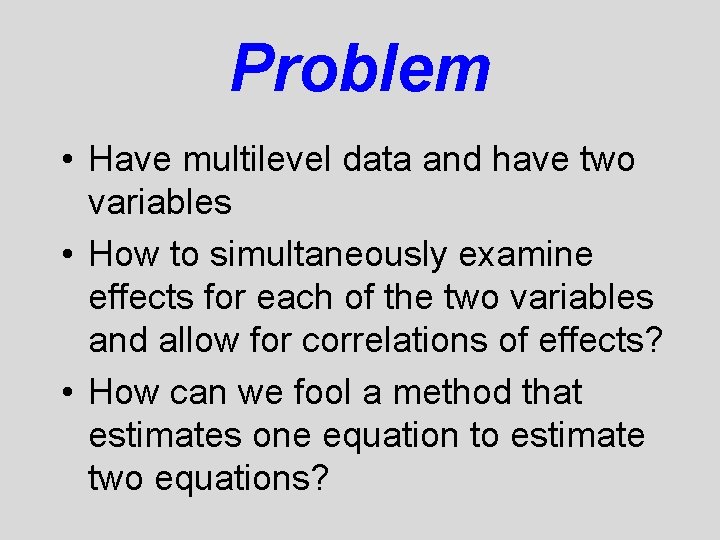
Problem • Have multilevel data and have two variables • How to simultaneously examine effects for each of the two variables and allow for correlations of effects? • How can we fool a method that estimates one equation to estimate two equations?
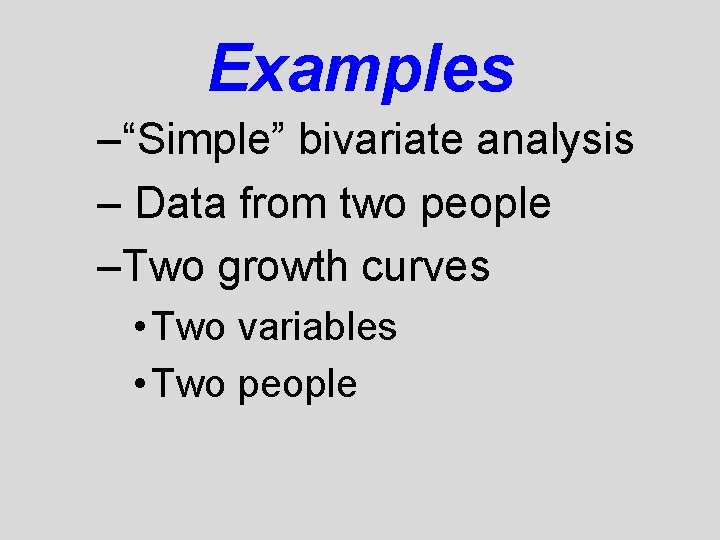
Examples –“Simple” bivariate analysis – Data from two people –Two growth curves • Two variables • Two people
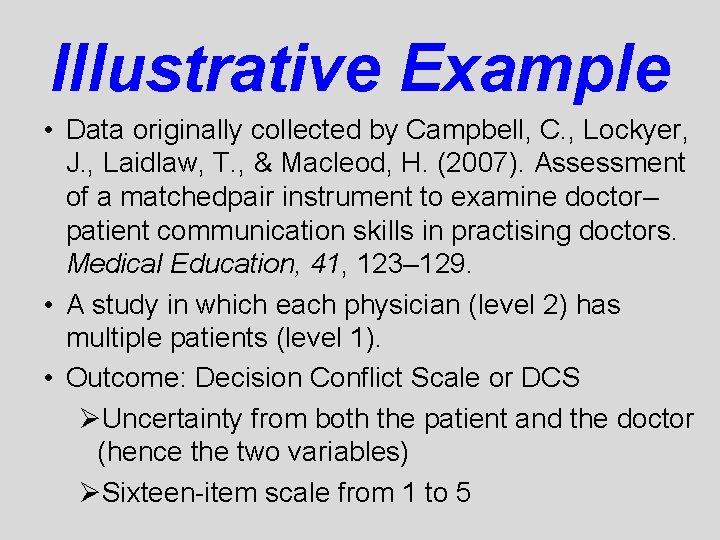
Illustrative Example • Data originally collected by Campbell, C. , Lockyer, J. , Laidlaw, T. , & Macleod, H. (2007). Assessment of a matchedpair instrument to examine doctor– patient communication skills in practising doctors. Medical Education, 41, 123– 129. • A study in which each physician (level 2) has multiple patients (level 1). • Outcome: Decision Conflict Scale or DCS ØUncertainty from both the patient and the doctor (hence the two variables) ØSixteen-item scale from 1 to 5
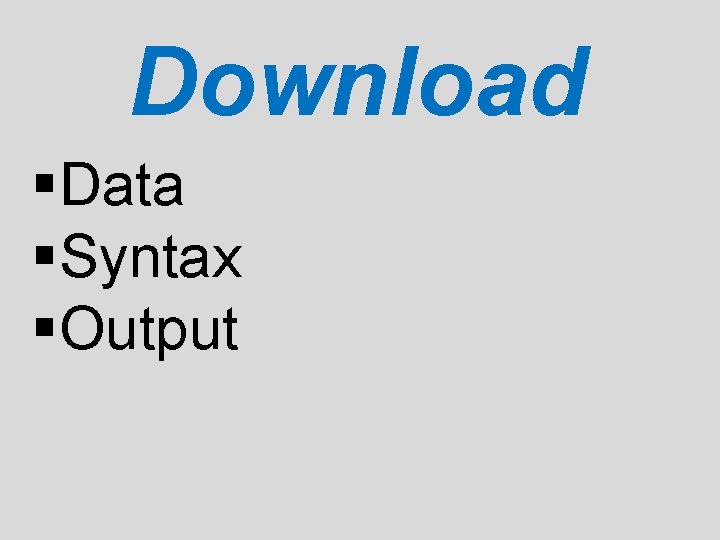
Download §Data §Syntax §Output
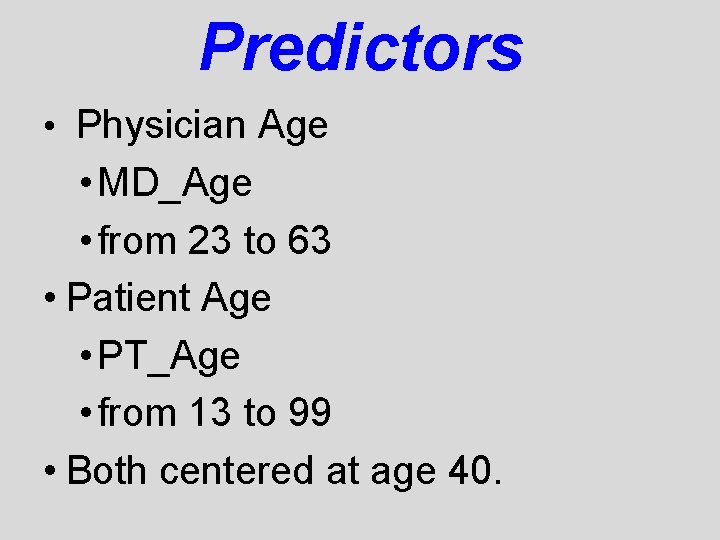
Predictors • Physician Age • MD_Age • from 23 to 63 • Patient Age • PT_Age • from 13 to 99 • Both centered at age 40.
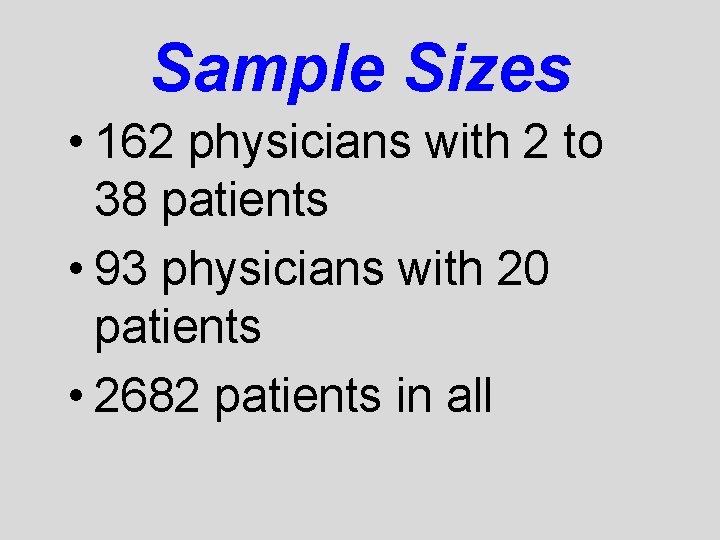
Sample Sizes • 162 physicians with 2 to 38 patients • 93 physicians with 20 patients • 2682 patients in all
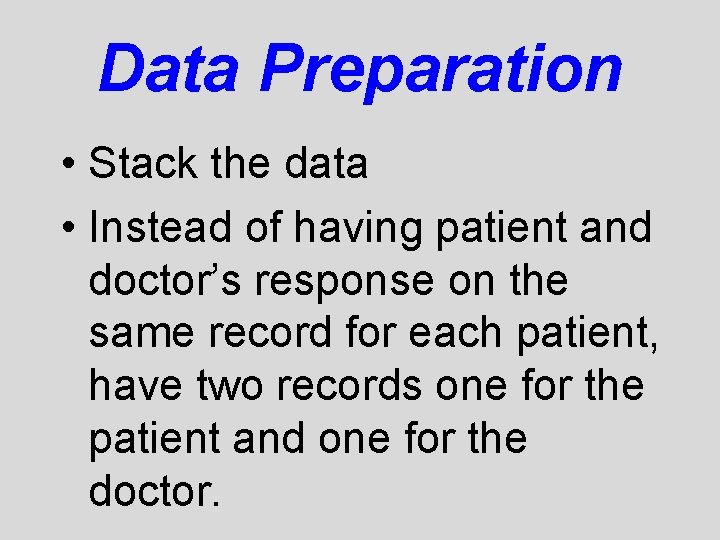
Data Preparation • Stack the data • Instead of having patient and doctor’s response on the same record for each patient, have two records one for the patient and one for the doctor.
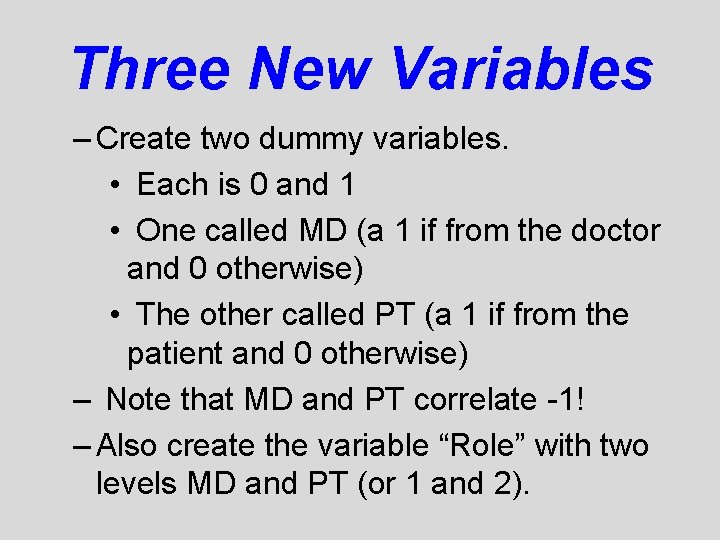
Three New Variables – Create two dummy variables. • Each is 0 and 1 • One called MD (a 1 if from the doctor and 0 otherwise) • The other called PT (a 1 if from the patient and 0 otherwise) – Note that MD and PT correlate -1! – Also create the variable “Role” with two levels MD and PT (or 1 and 2).
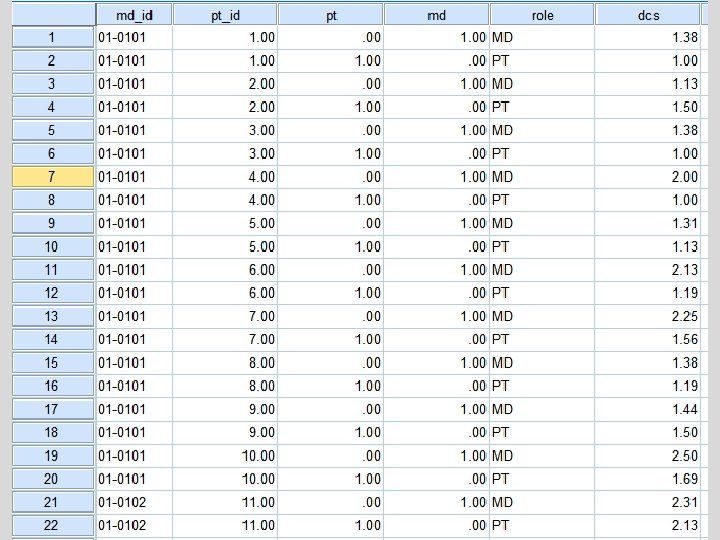
11
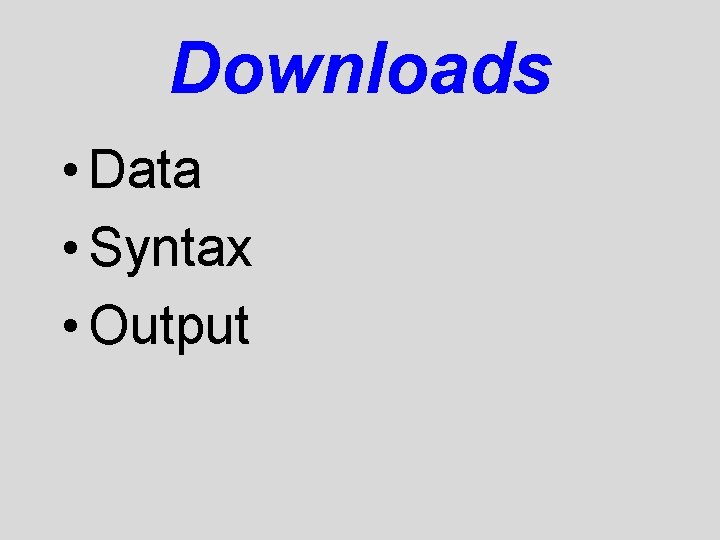
Downloads • Data • Syntax • Output
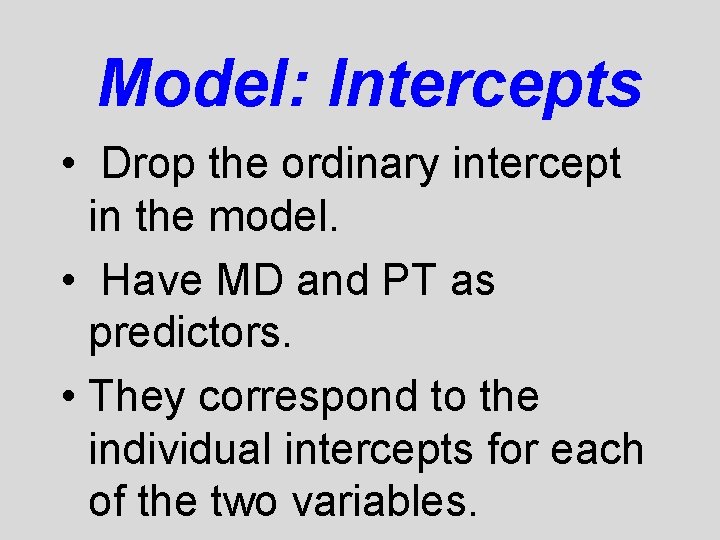
Model: Intercepts • Drop the ordinary intercept in the model. • Have MD and PT as predictors. • They correspond to the individual intercepts for each of the two variables.
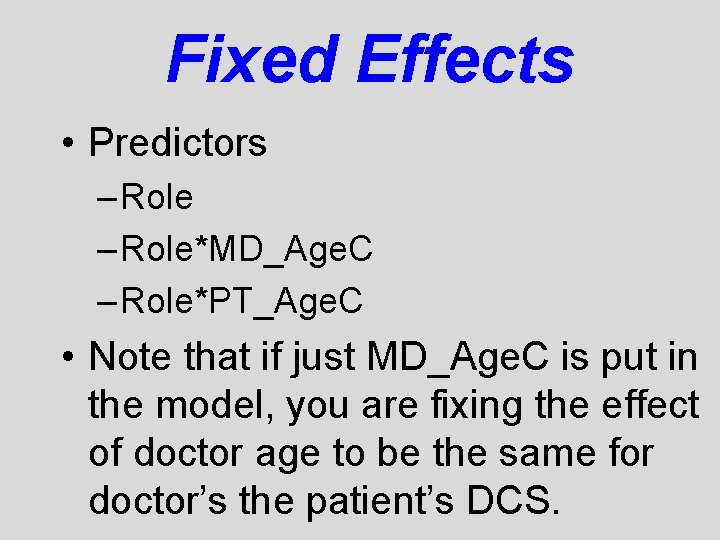
Fixed Effects • Predictors – Role*MD_Age. C – Role*PT_Age. C • Note that if just MD_Age. C is put in the model, you are fixing the effect of doctor age to be the same for doctor’s the patient’s DCS.
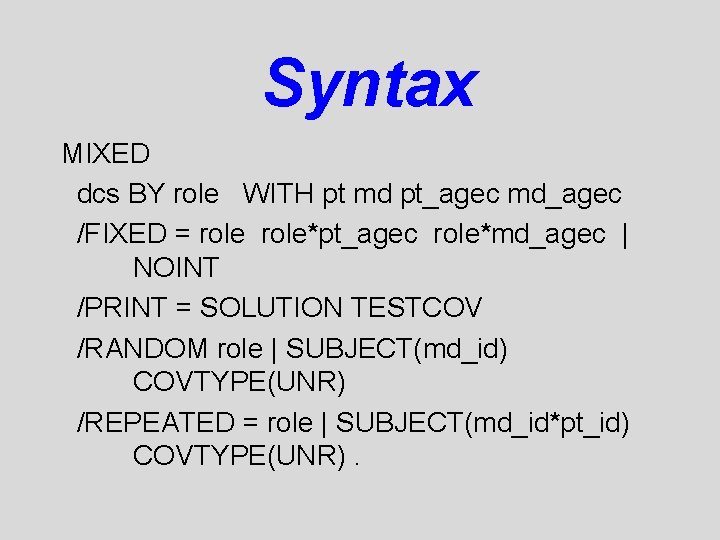
Syntax MIXED dcs BY role WITH pt md pt_agec md_agec /FIXED = role*pt_agec role*md_agec | NOINT /PRINT = SOLUTION TESTCOV /RANDOM role | SUBJECT(md_id) COVTYPE(UNR) /REPEATED = role | SUBJECT(md_id*pt_id) COVTYPE(UNR).
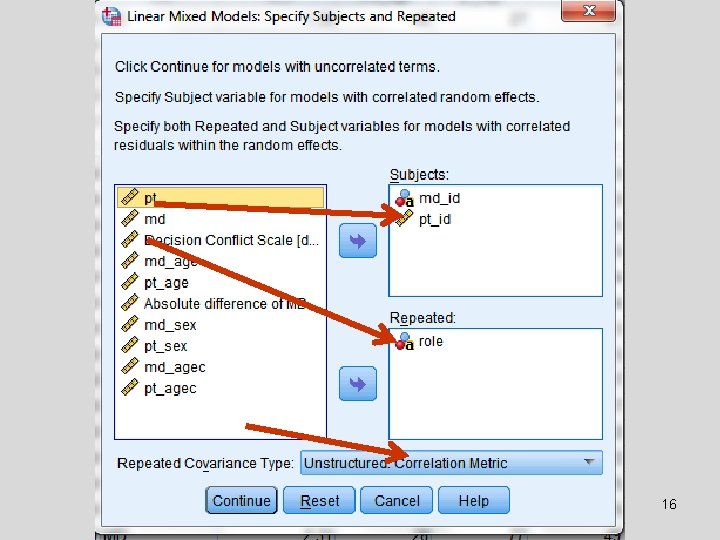
16
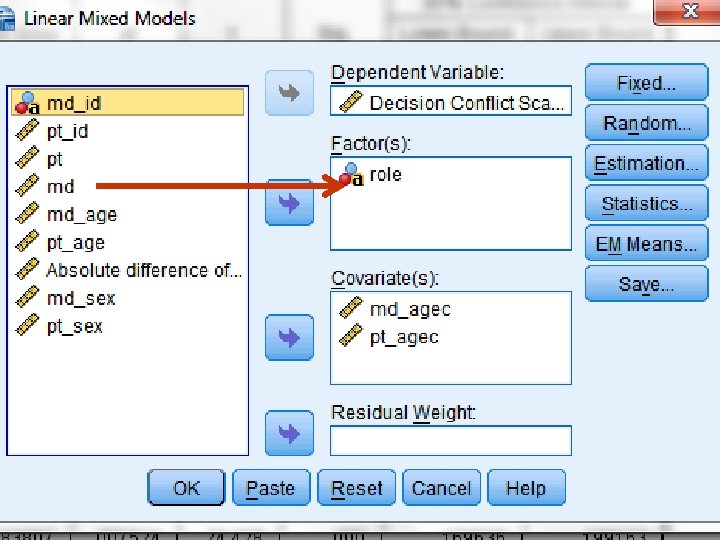
17
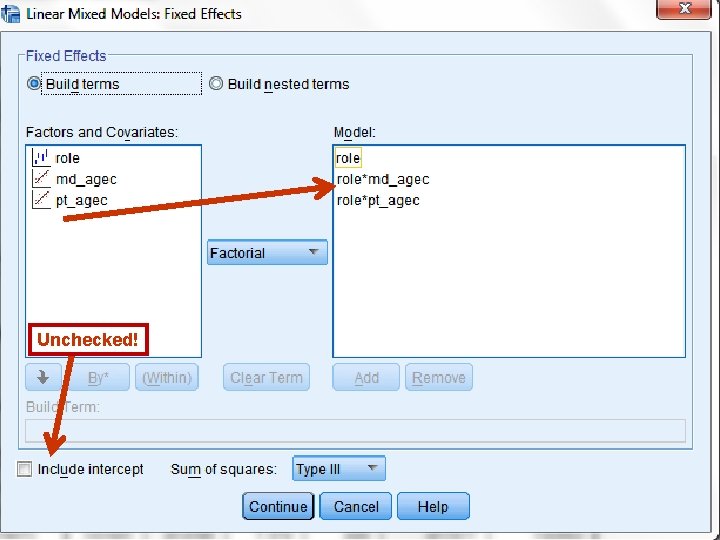
Unchecked! 18
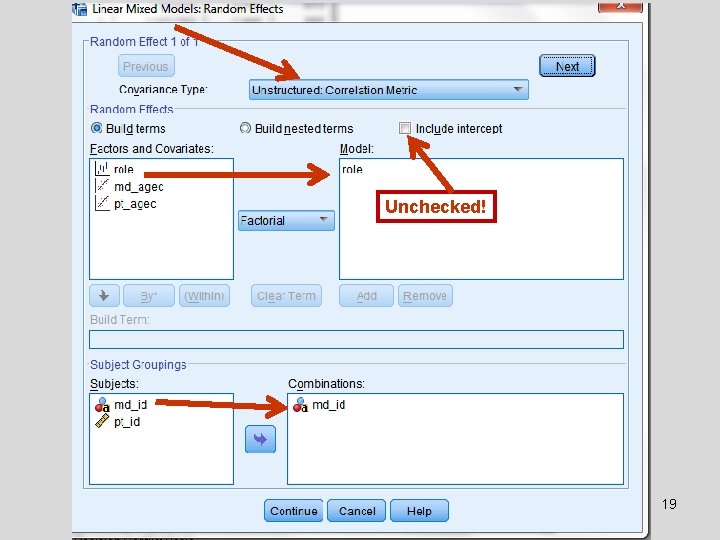
Unchecked! 19
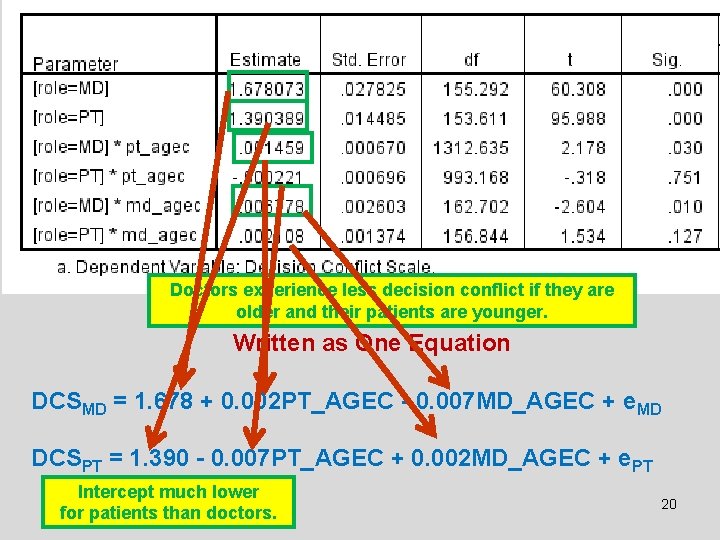
Doctors experience less decision conflict if they are older and their patients are younger. Written as One Equation DCSMD = 1. 678 + 0. 002 PT_AGEC - 0. 007 MD_AGEC + e. MD DCSPT = 1. 390 - 0. 007 PT_AGEC + 0. 002 MD_AGEC + e. PT Intercept much lower for patients than doctors. 20
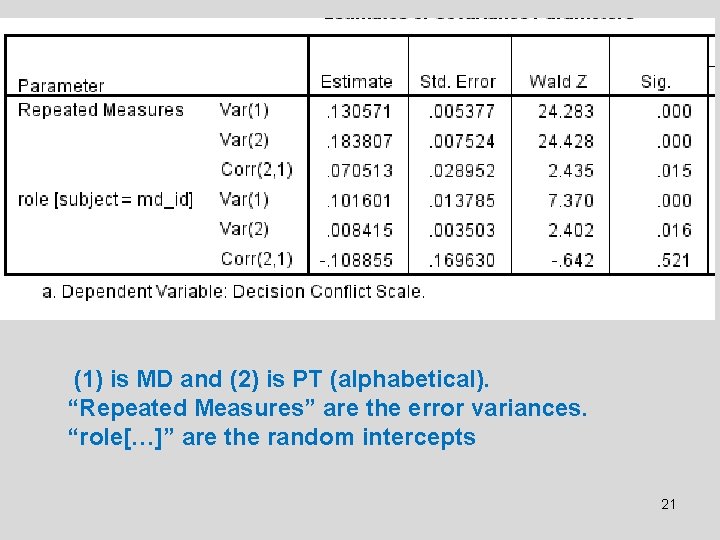
(1) is MD and (2) is PT (alphabetical). “Repeated Measures” are the error variances. “role[…]” are the random intercepts 21
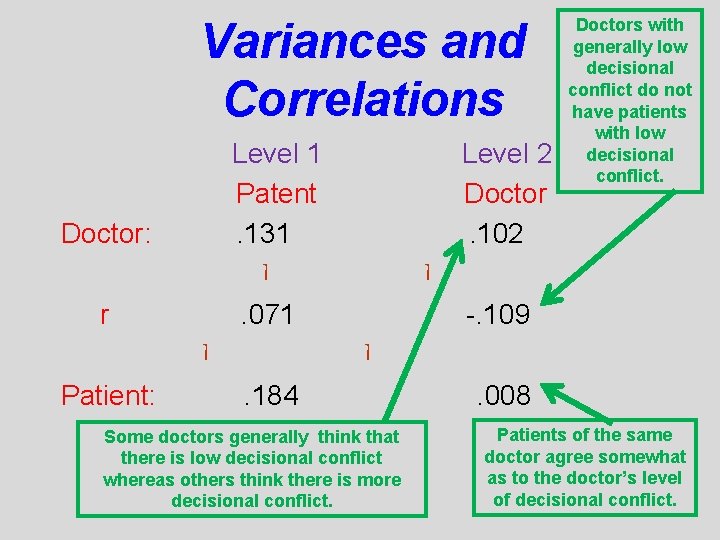
Variances and Correlations Level 1 Patent. 131 I Doctor: r I . 071 I Patient: Level 2 Doctor. 102 Doctors with generally low decisional conflict do not have patients with low decisional conflict. -. 109 I . 184 Some doctors generally think that there is low decisional conflict whereas others think there is more decisional conflict. . 008 Patients of the same doctor agree somewhat as to the doctor’s level of decisional conflict.
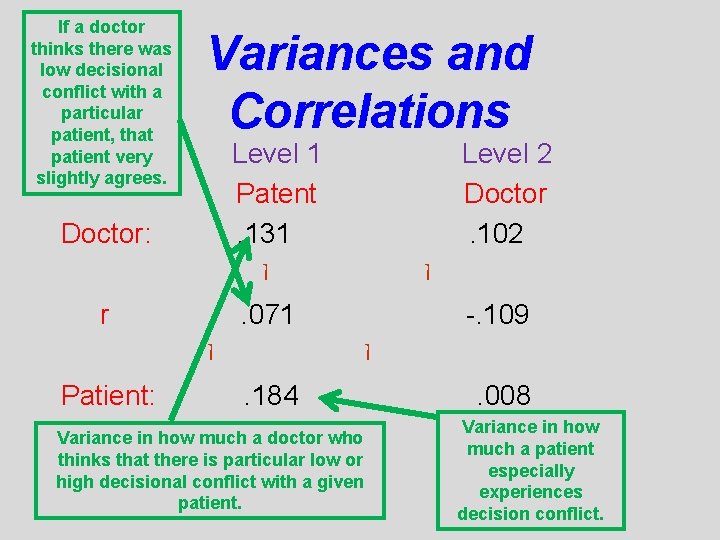
If a doctor thinks there was low decisional conflict with a particular patient, that patient very slightly agrees. Variances and Correlations Level 1 Patent. 131 I Doctor: r I . 071 I Patient: Level 2 Doctor. 102 -. 109 I. 184 Variance in how much a doctor who thinks that there is particular low or high decisional conflict with a given patient. . 008 Variance in how much a patient especially experiences decision conflict.
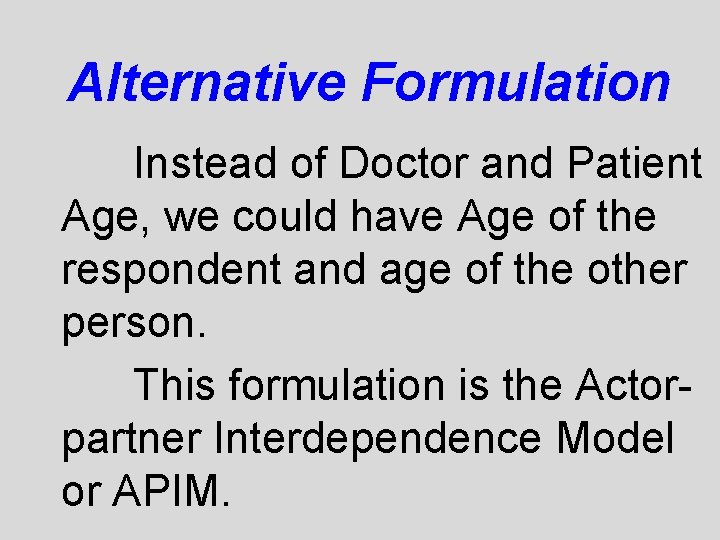
Alternative Formulation Instead of Doctor and Patient Age, we could have Age of the respondent and age of the other person. This formulation is the Actorpartner Interdependence Model or APIM.
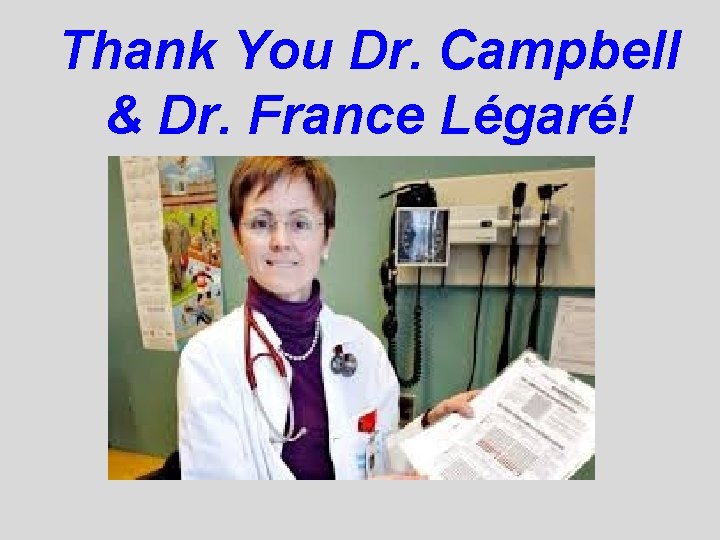
Thank You Dr. Campbell & Dr. France Légaré!
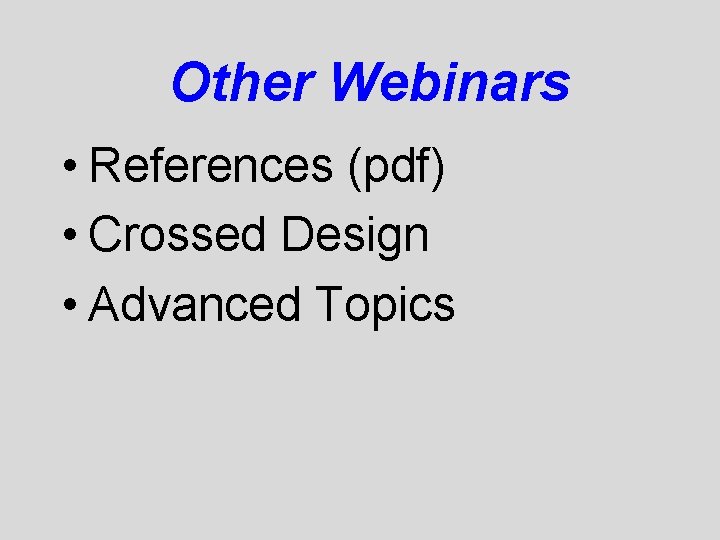
Other Webinars • References (pdf) • Crossed Design • Advanced Topics
Multilevel modeling spss
Growth curve modeling spss
Dimensional modeling vs relational modeling
Helen c. erickson
Kronecker graph
Thinking humanly: the cognitive modeling approach
Priority scheduling
Multilevel model equation example
Contoh multilevel inheritance
Mfq scheduling
Process virtual address space
Teaching multilevel esl classes
Multilevel scheme in data communication
Apa yang dimaksud dengan inheritance
Multilevel paging in os
Multiplexing and spreading
Multilevel indexing
Bandwidth utilization multiplexing and spreading
Mlfq scheduler
Sample size calculations in multilevel modelling
Advanced regression and multilevel models
Multilevel bus architecture in embedded systems
Multi level security example
Miller index visualizer
Multilevel security in database
Multilevel model equation example
Multilevel instruction