Lecture 4 Econometric Foundations Stephen P Ryan Olin
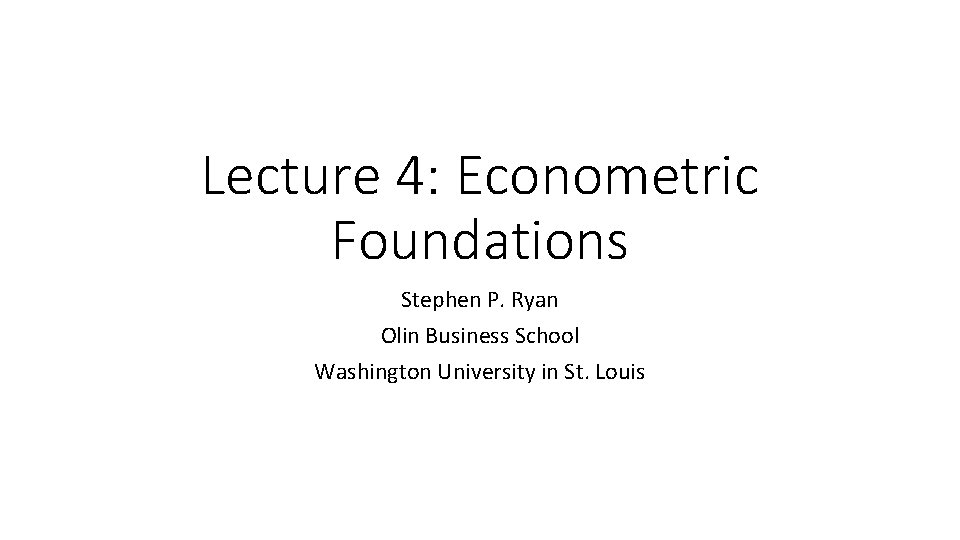
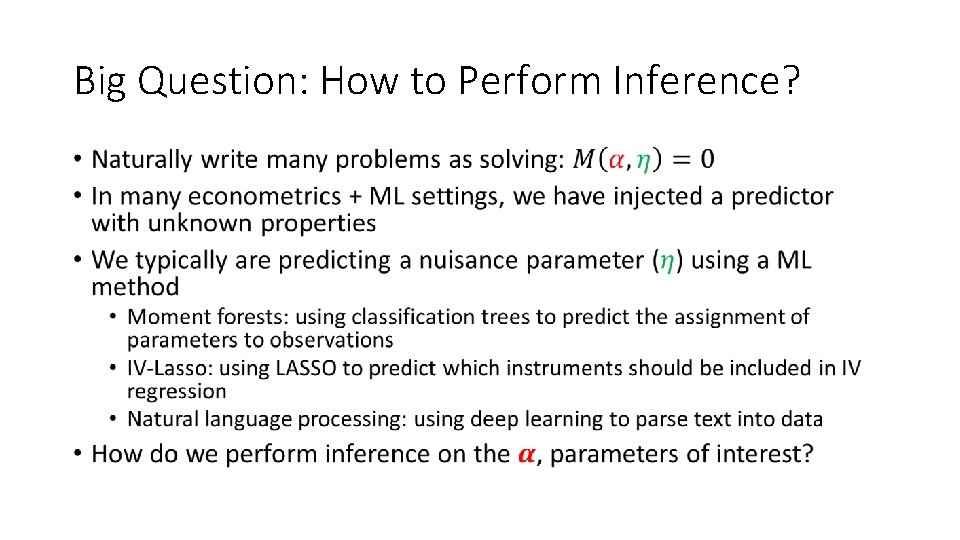
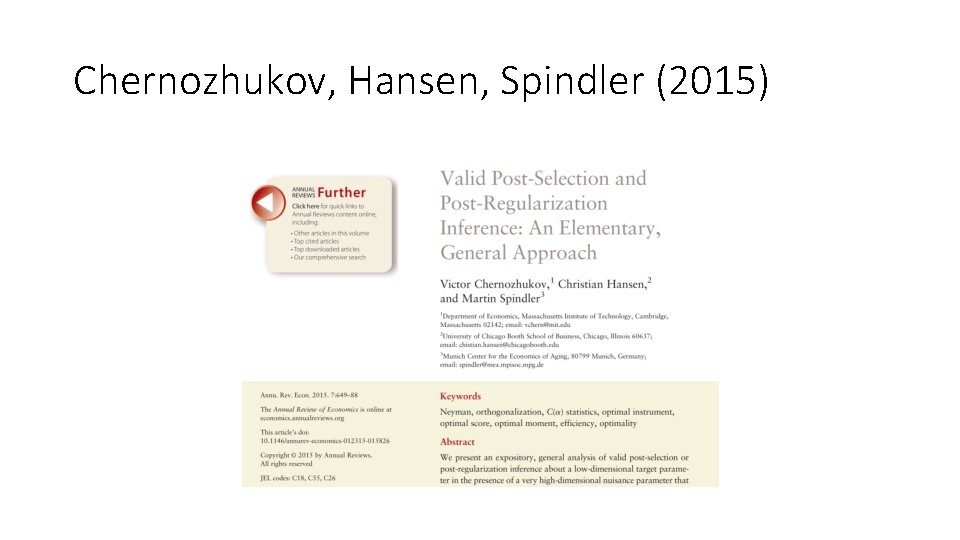
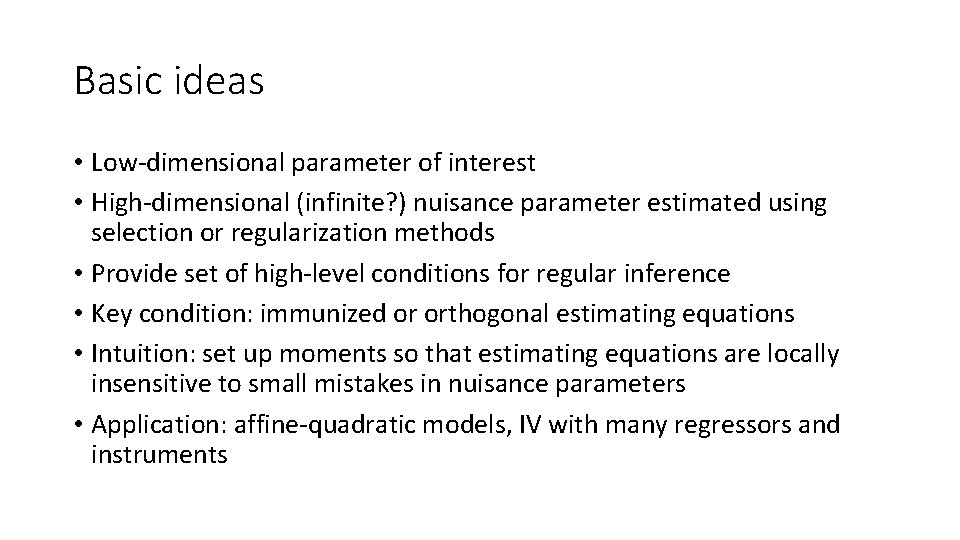
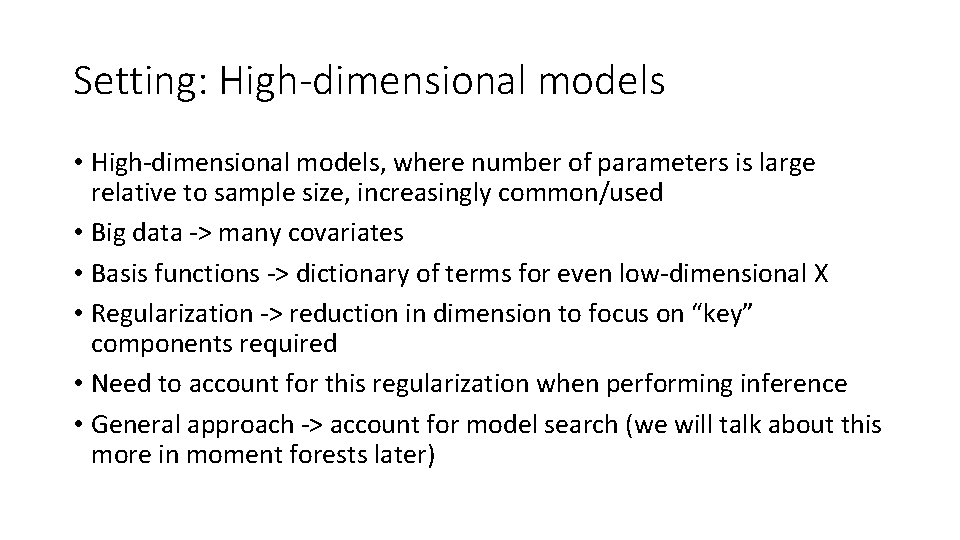
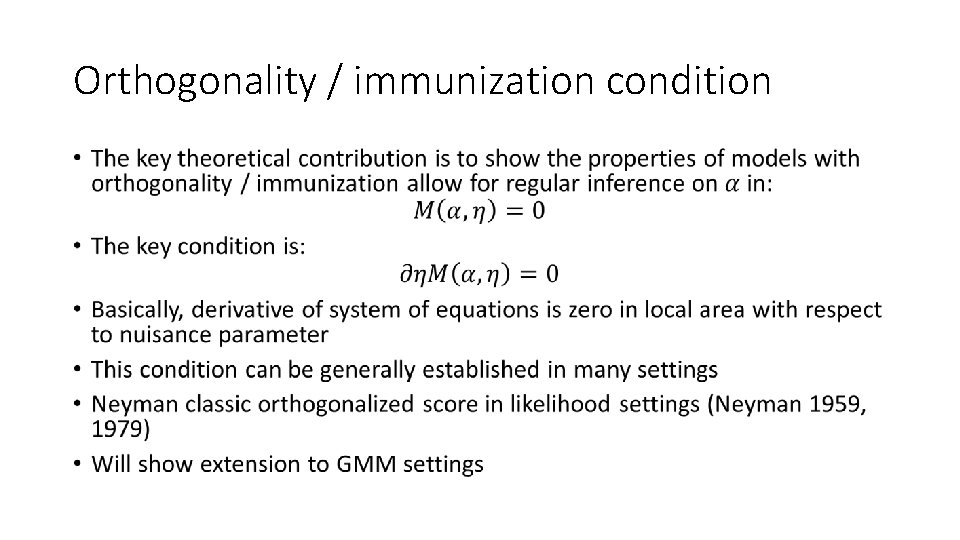
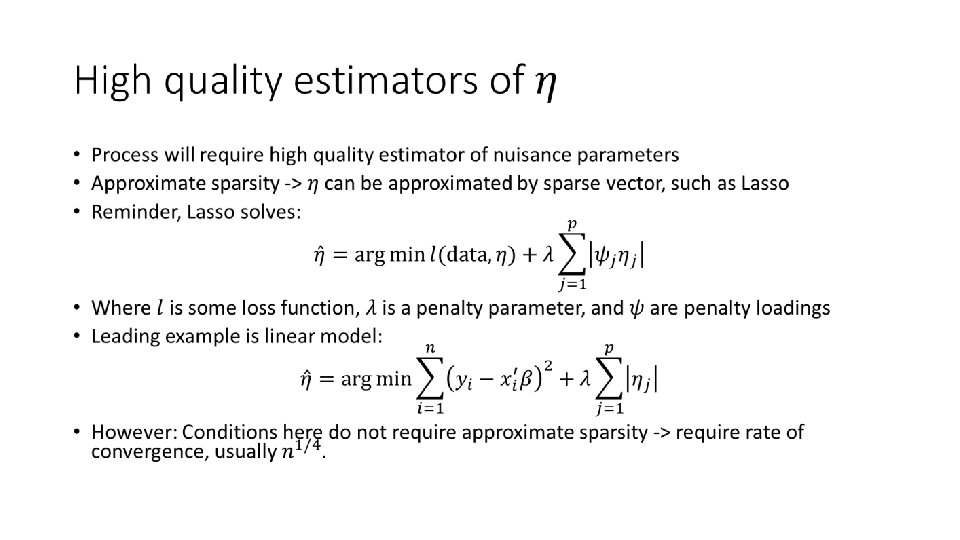
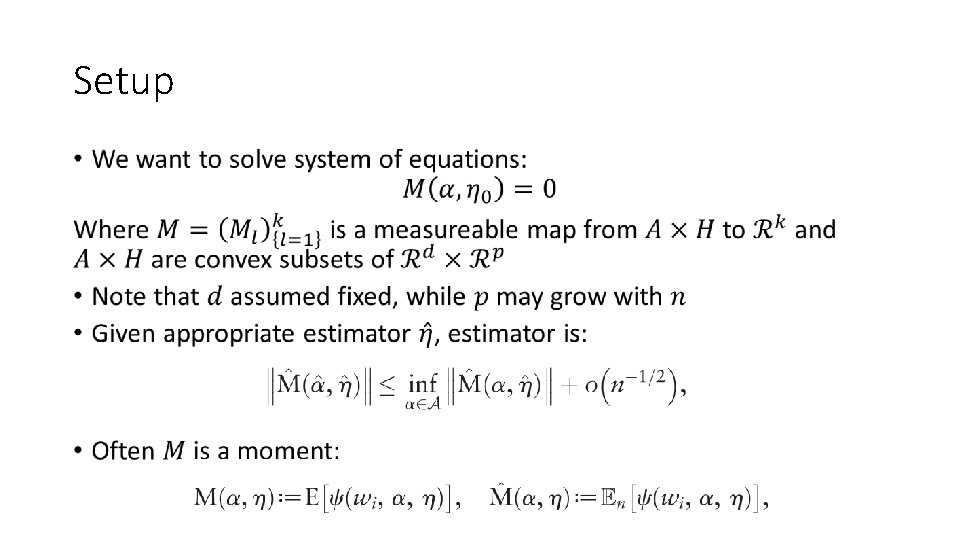
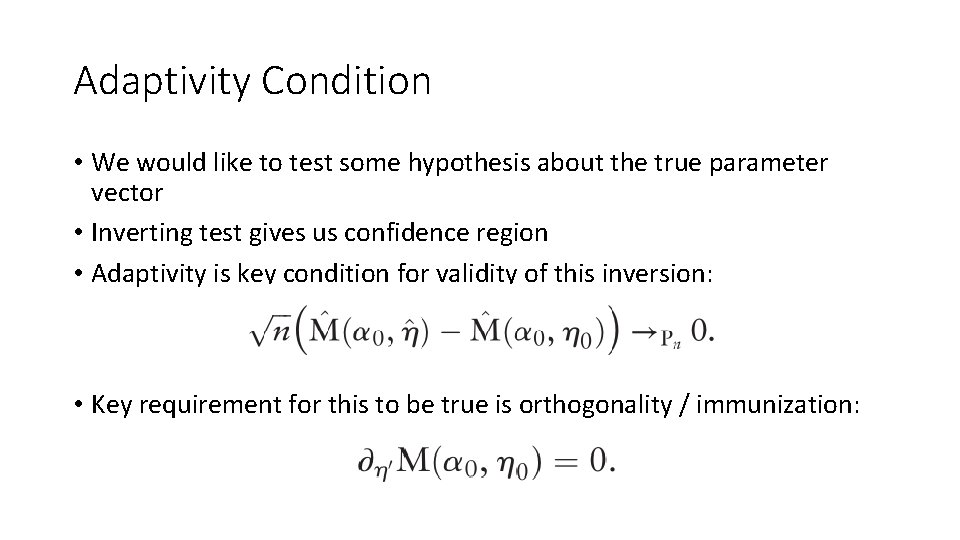
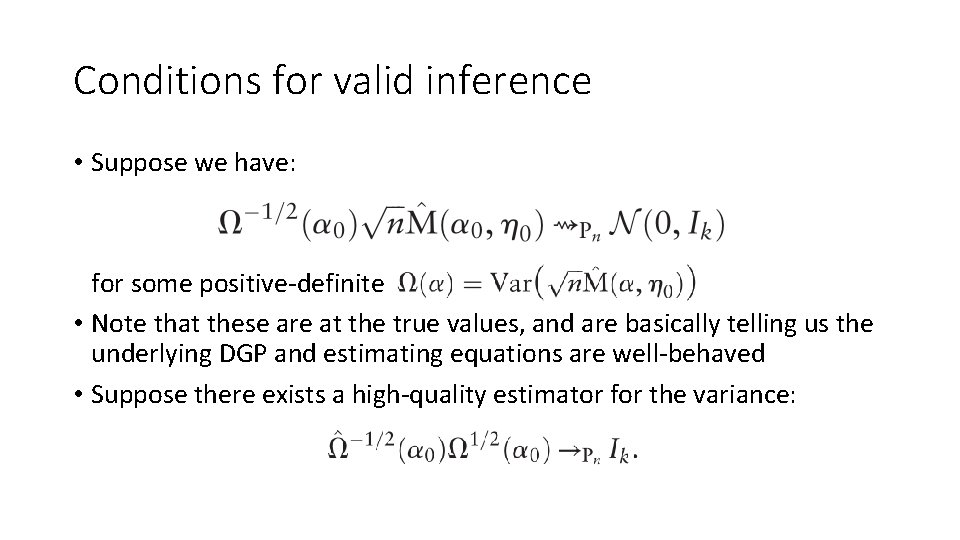
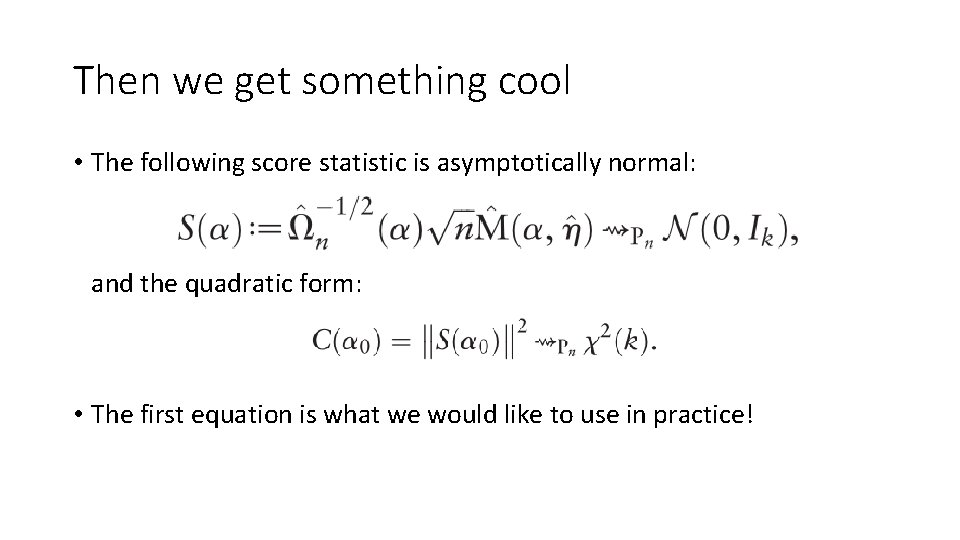
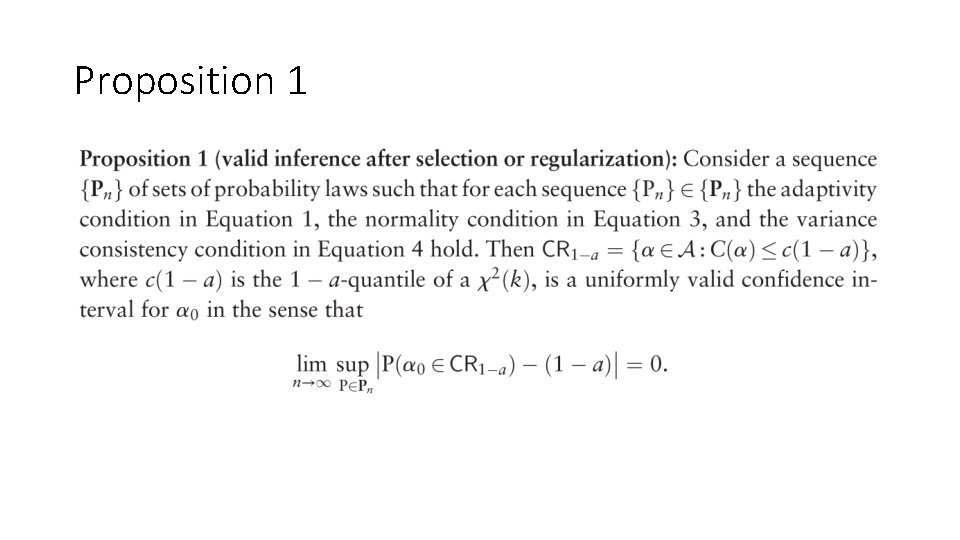
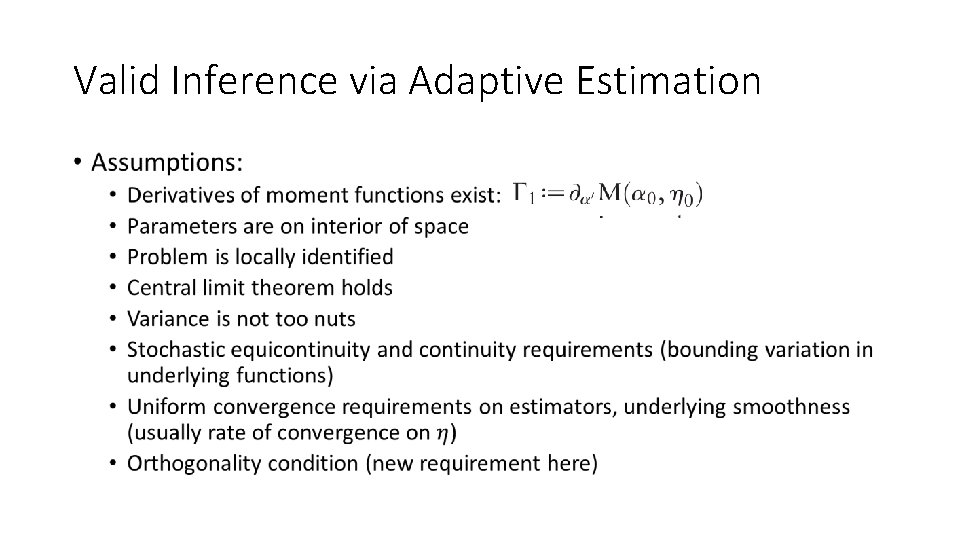
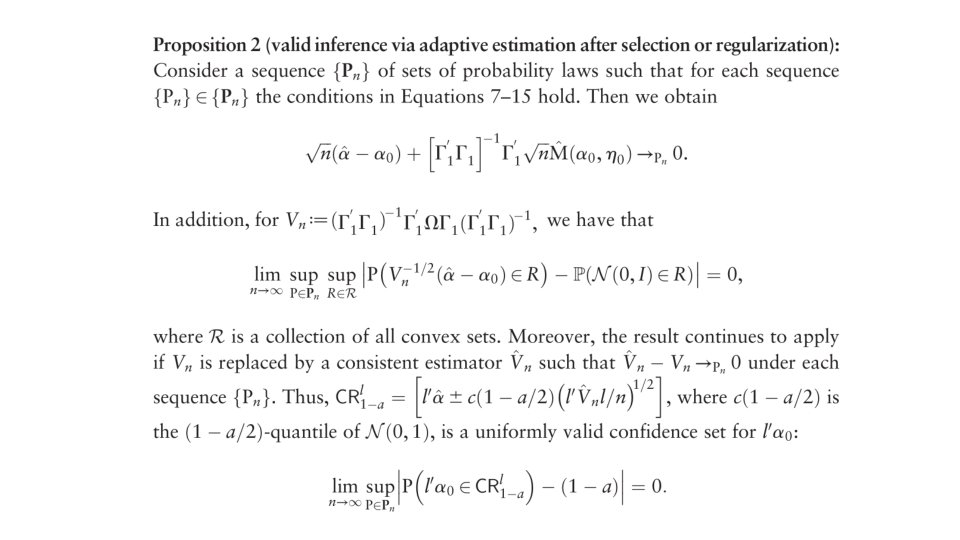
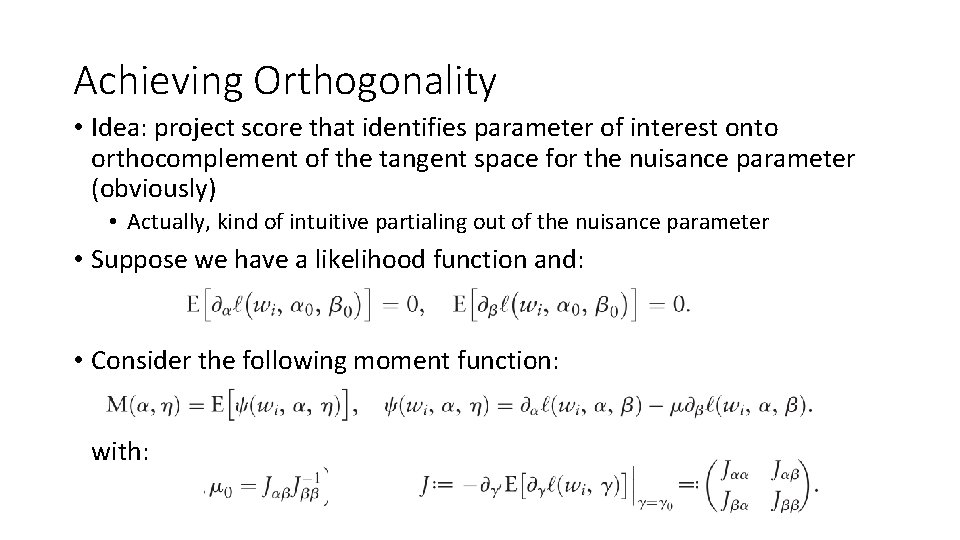
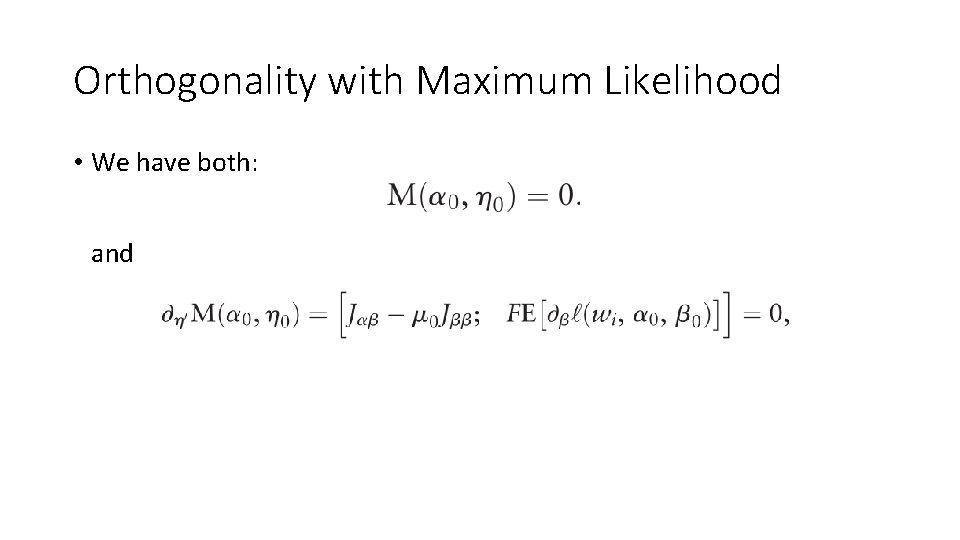
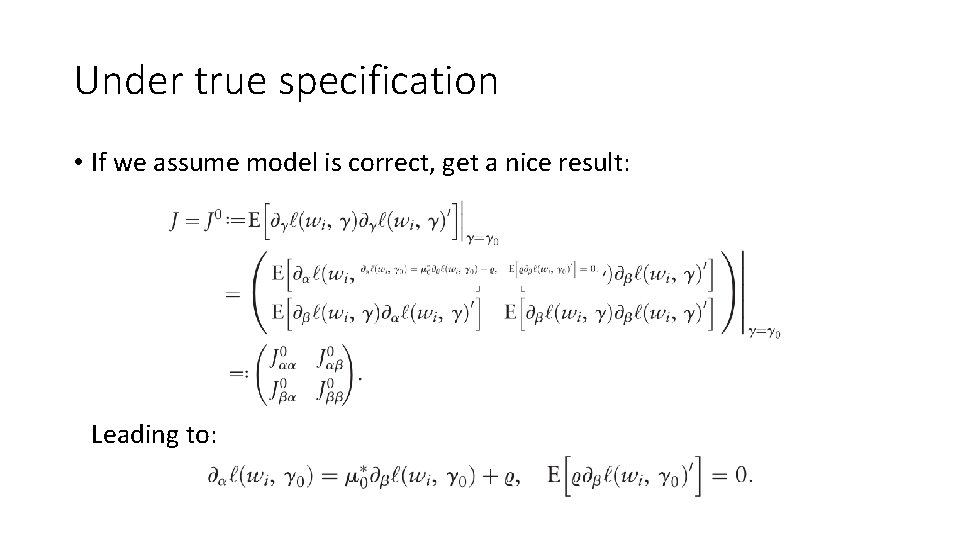
![Lemma 1 [Neyman’s Orthogonalization] Lemma 1 [Neyman’s Orthogonalization]](https://slidetodoc.com/presentation_image_h/f2d7ed3fc53342cb2161c703cb4558e5/image-18.jpg)
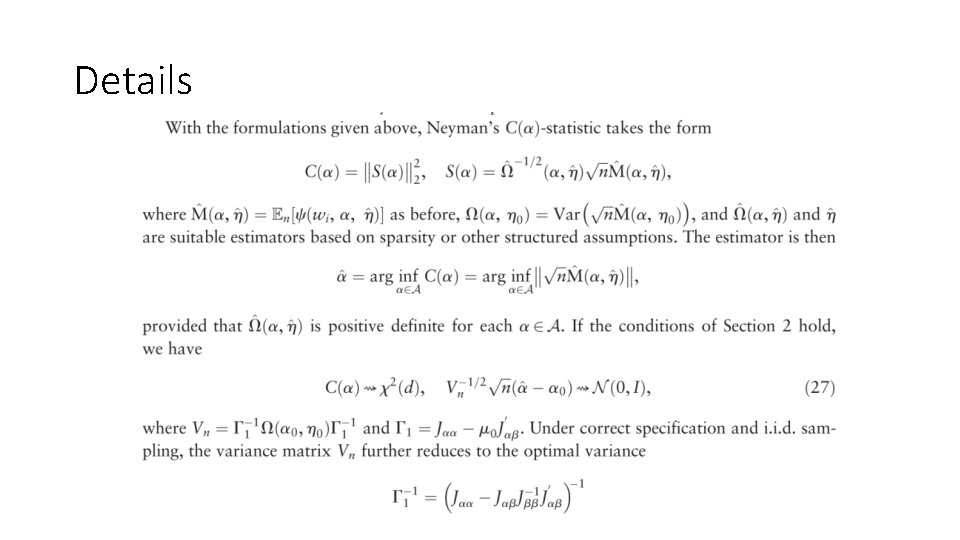
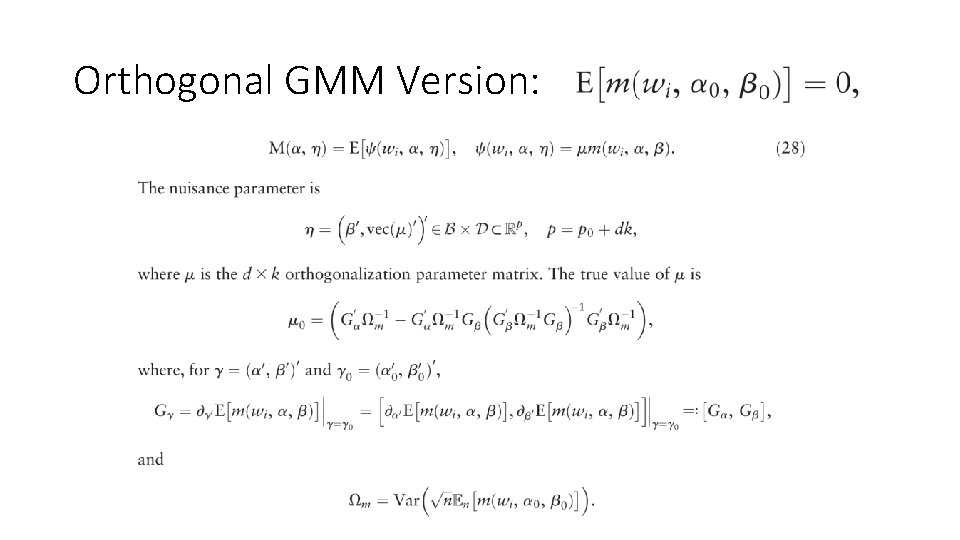
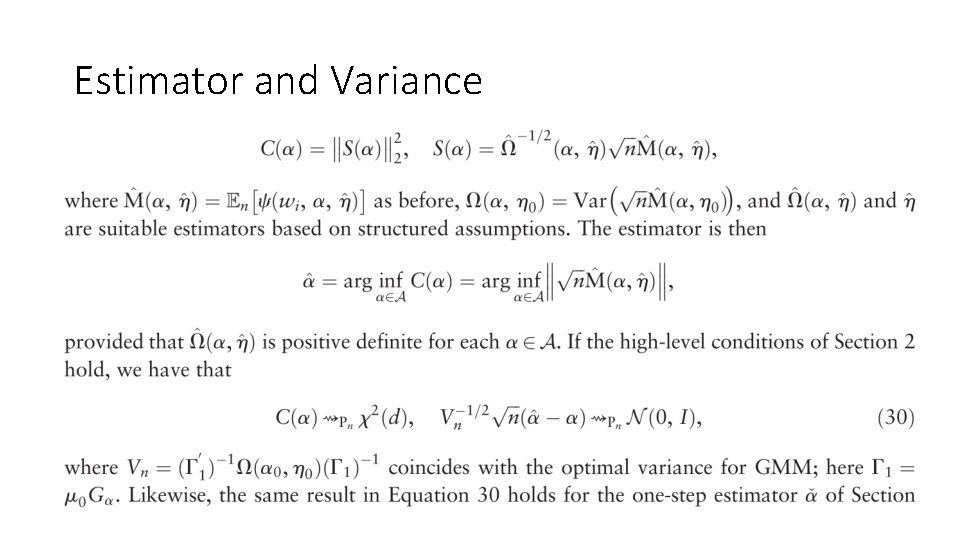
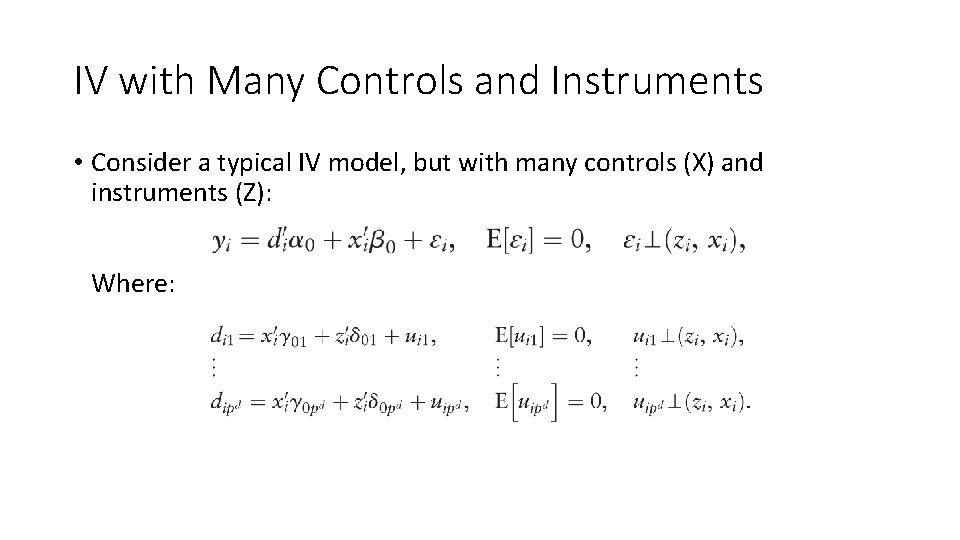
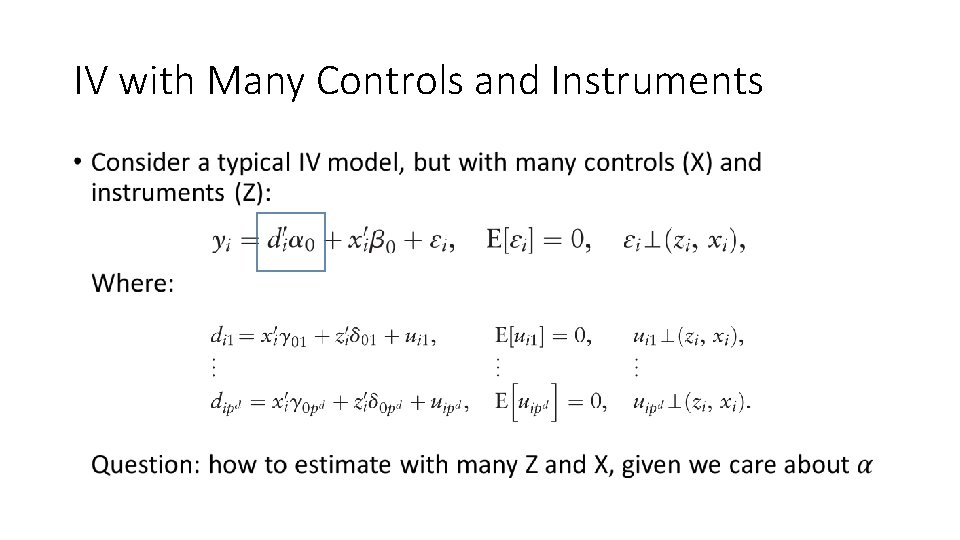
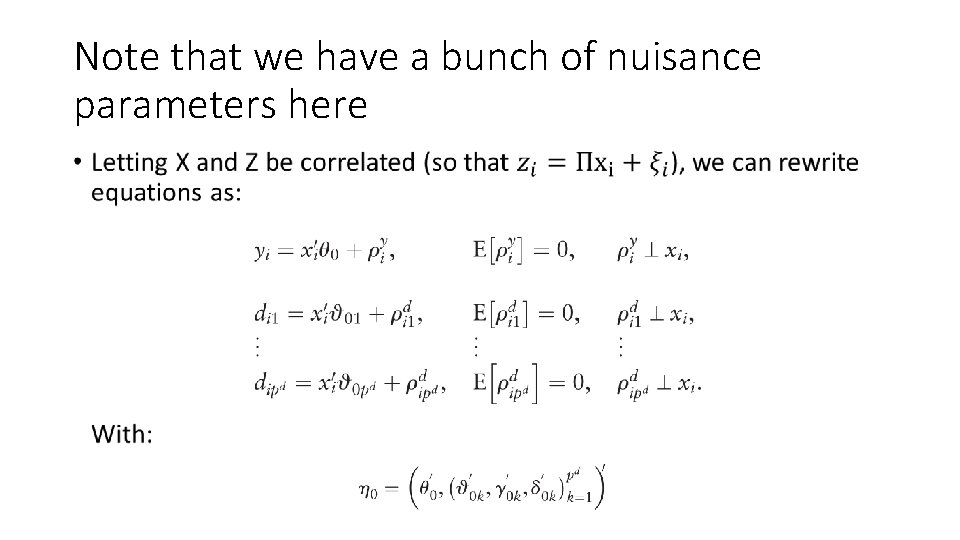
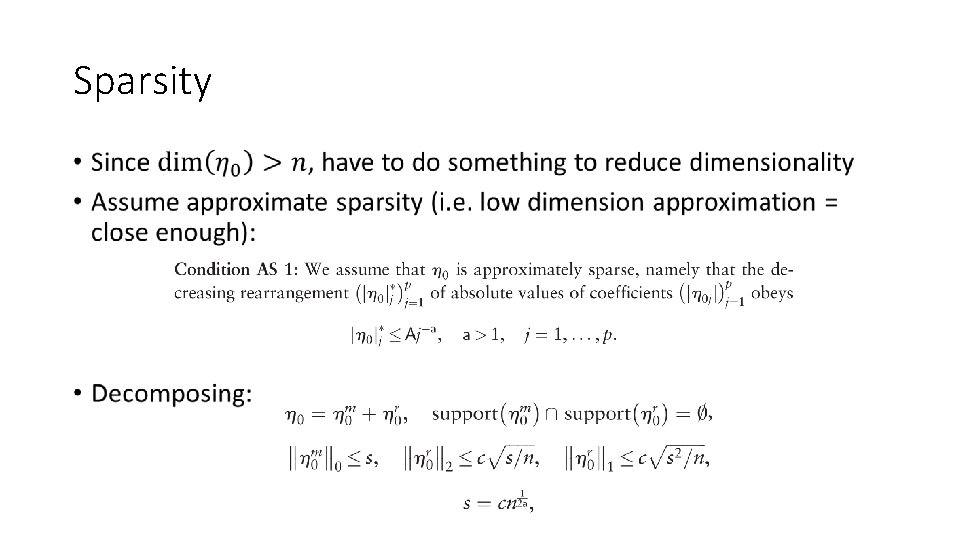
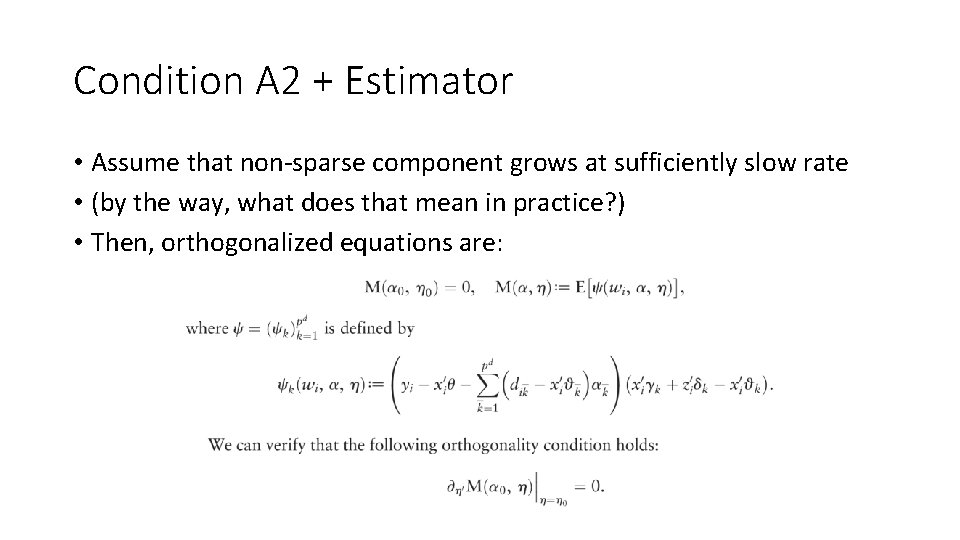
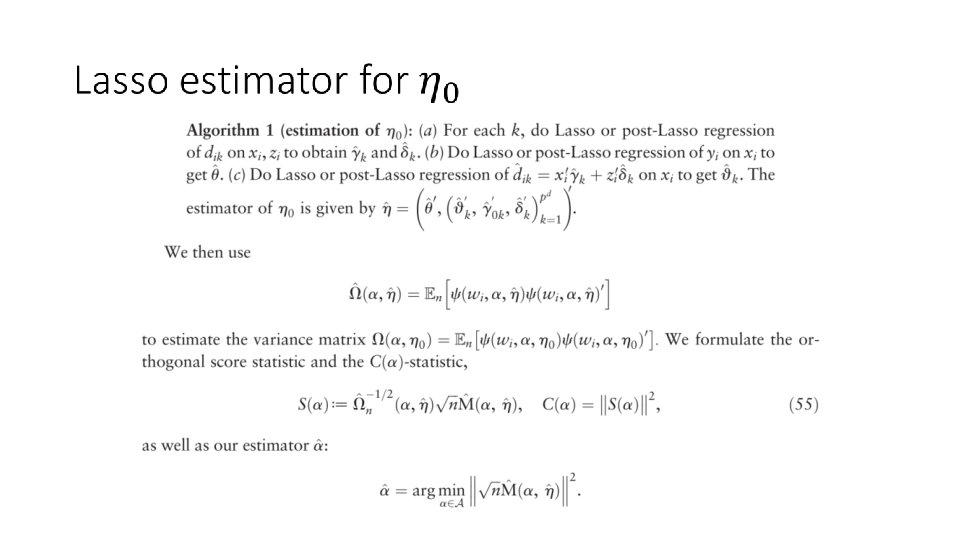
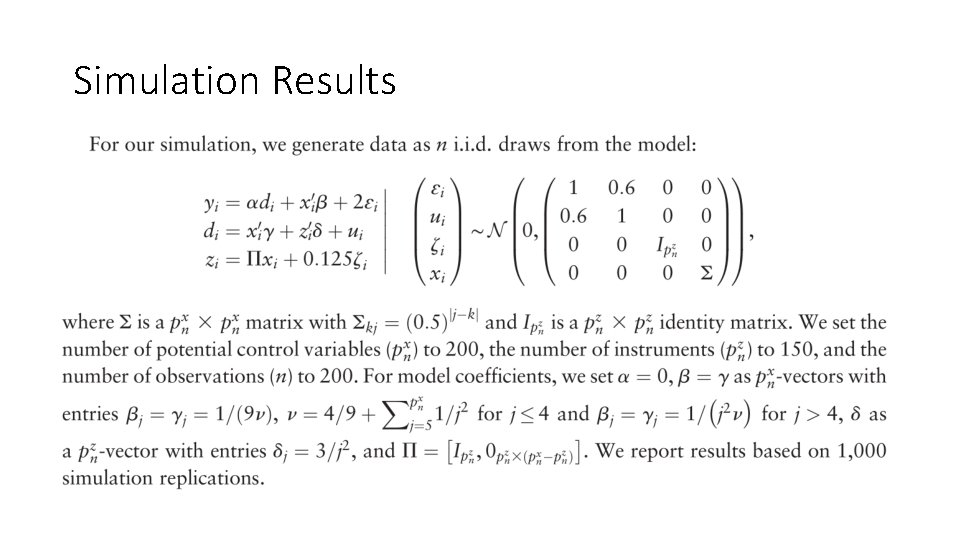
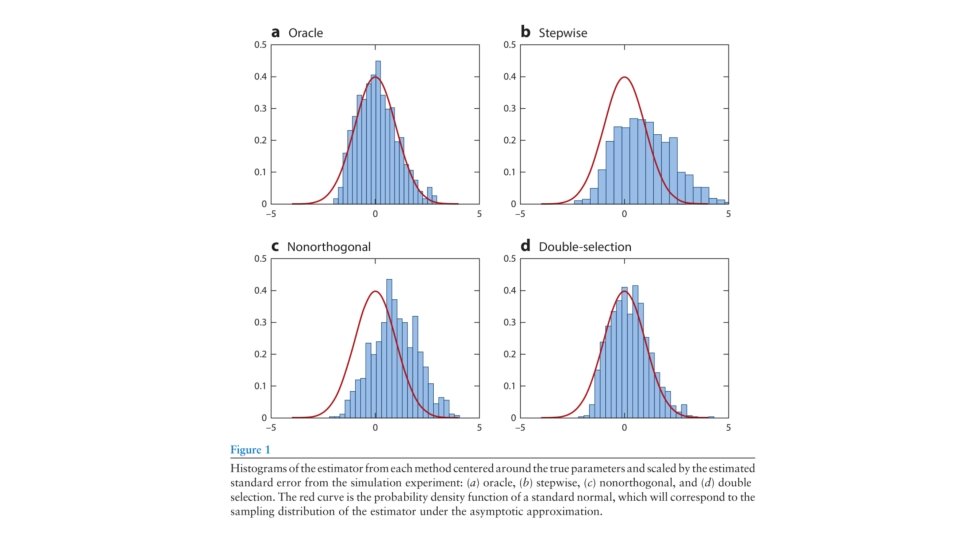
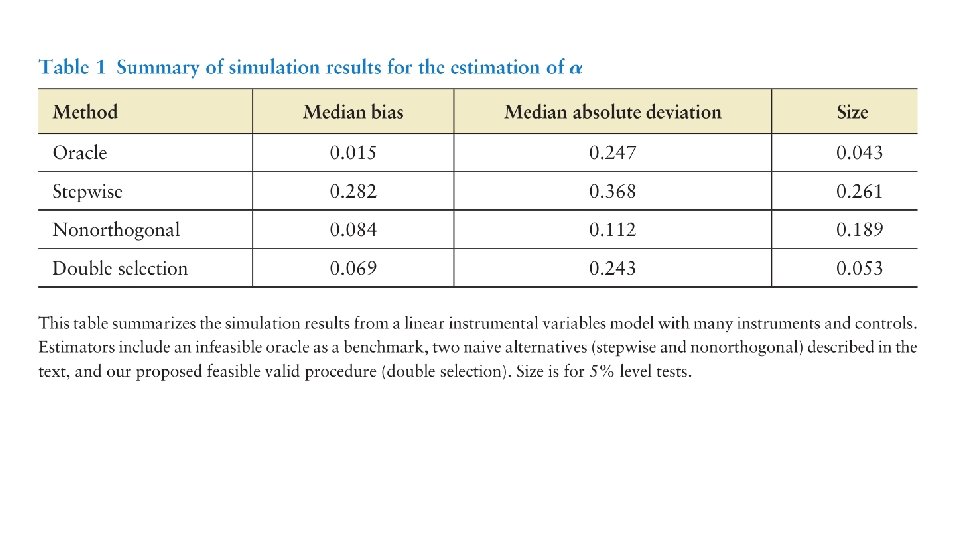
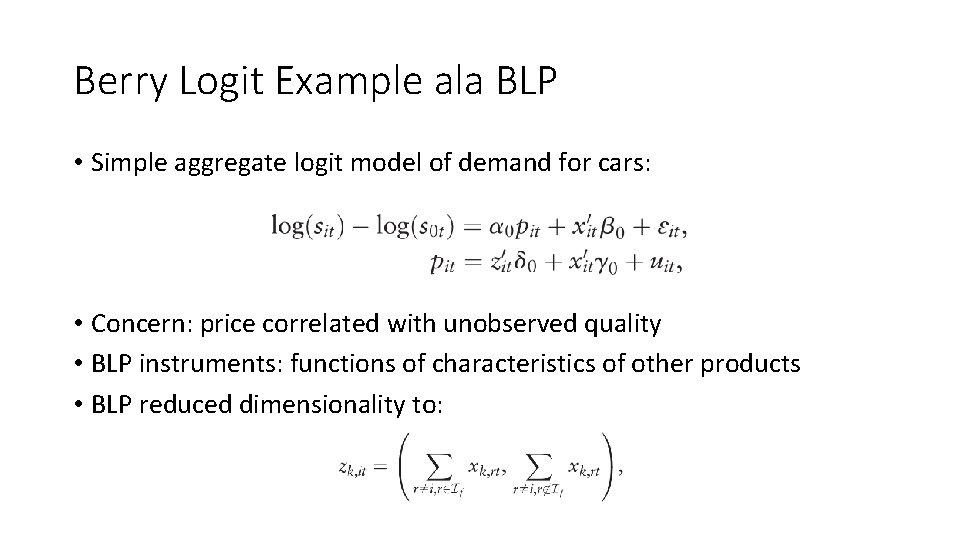
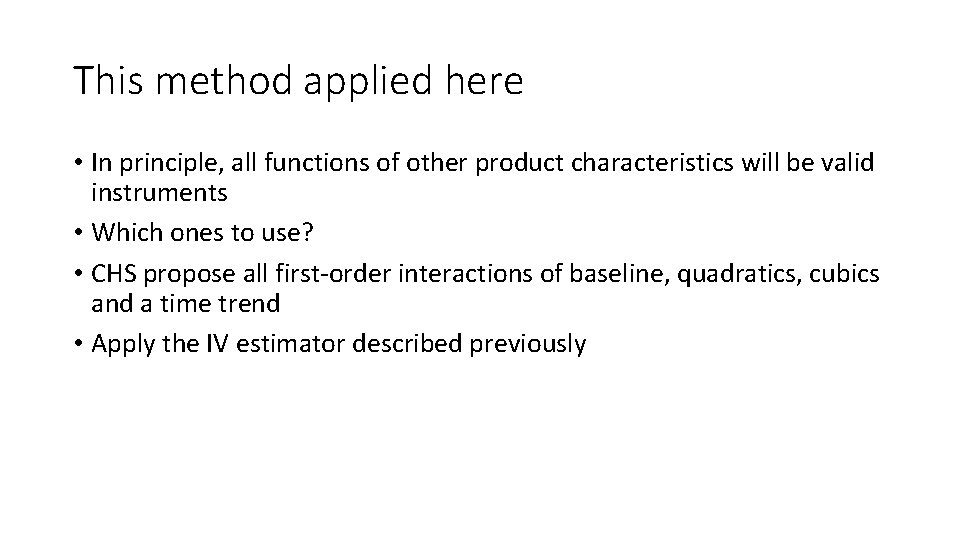
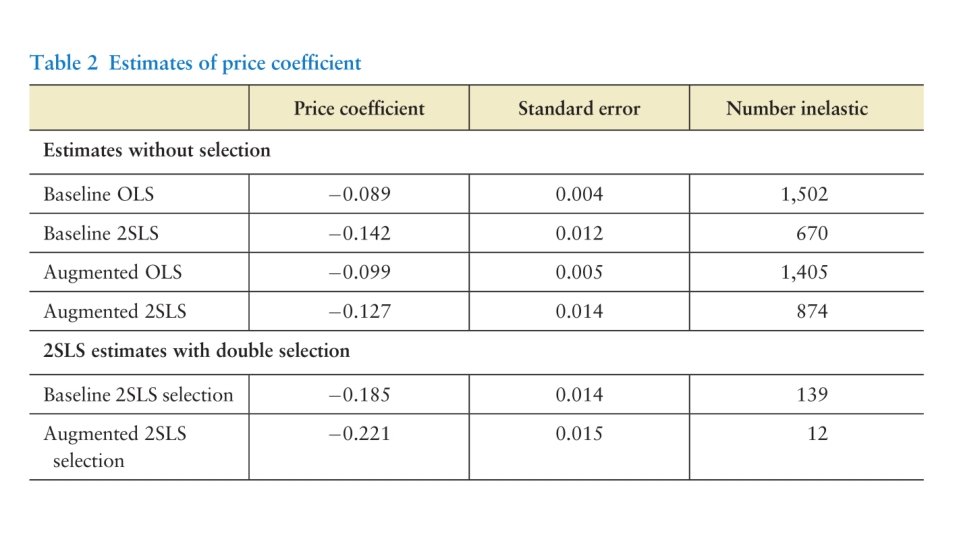
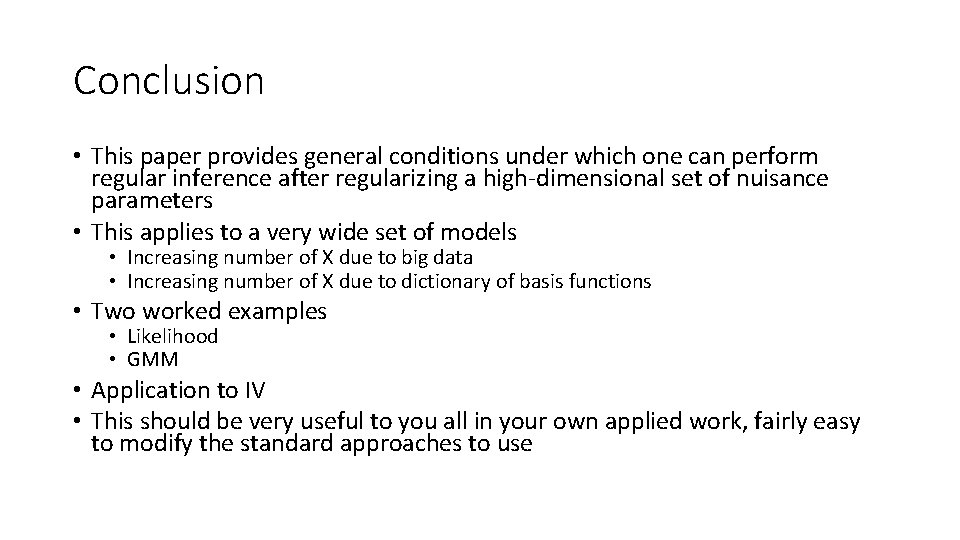
- Slides: 34
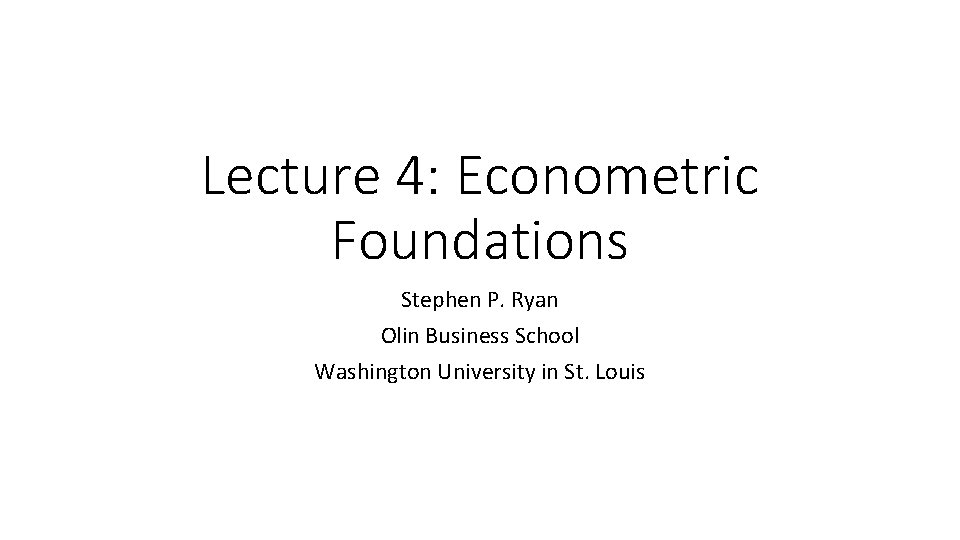
Lecture 4: Econometric Foundations Stephen P. Ryan Olin Business School Washington University in St. Louis
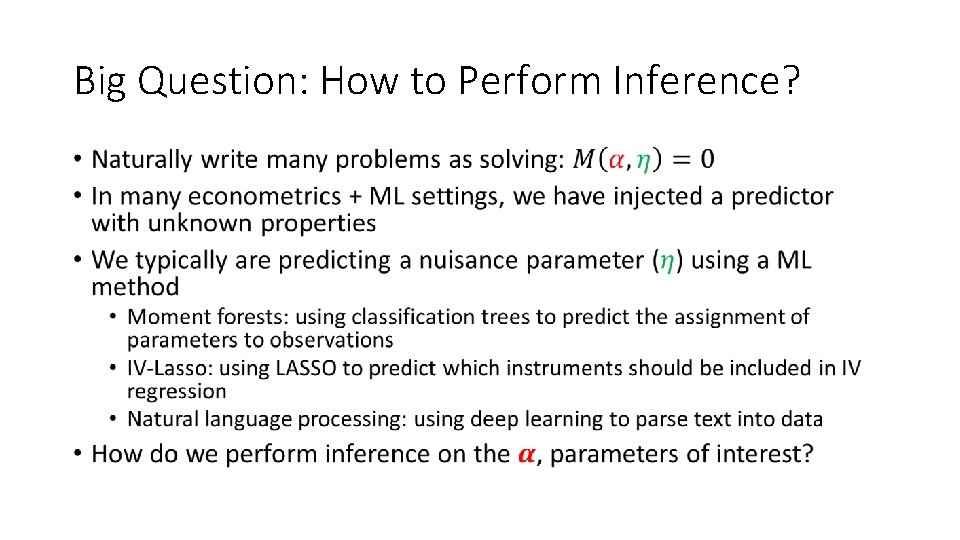
Big Question: How to Perform Inference? •
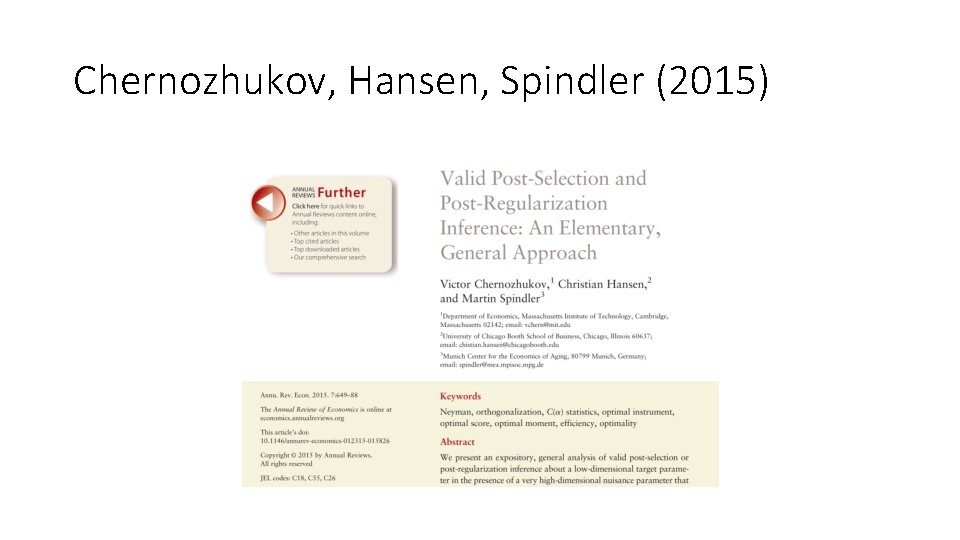
Chernozhukov, Hansen, Spindler (2015)
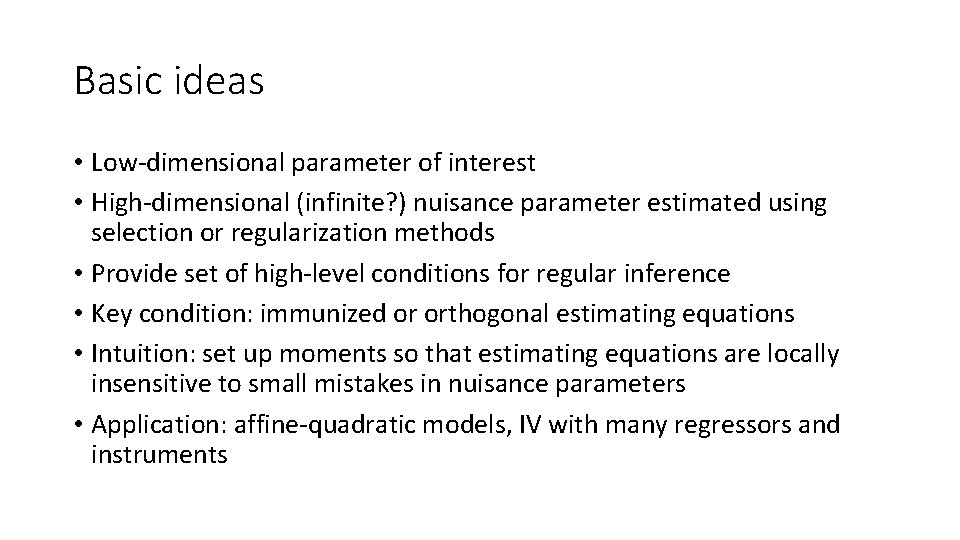
Basic ideas • Low-dimensional parameter of interest • High-dimensional (infinite? ) nuisance parameter estimated using selection or regularization methods • Provide set of high-level conditions for regular inference • Key condition: immunized or orthogonal estimating equations • Intuition: set up moments so that estimating equations are locally insensitive to small mistakes in nuisance parameters • Application: affine-quadratic models, IV with many regressors and instruments
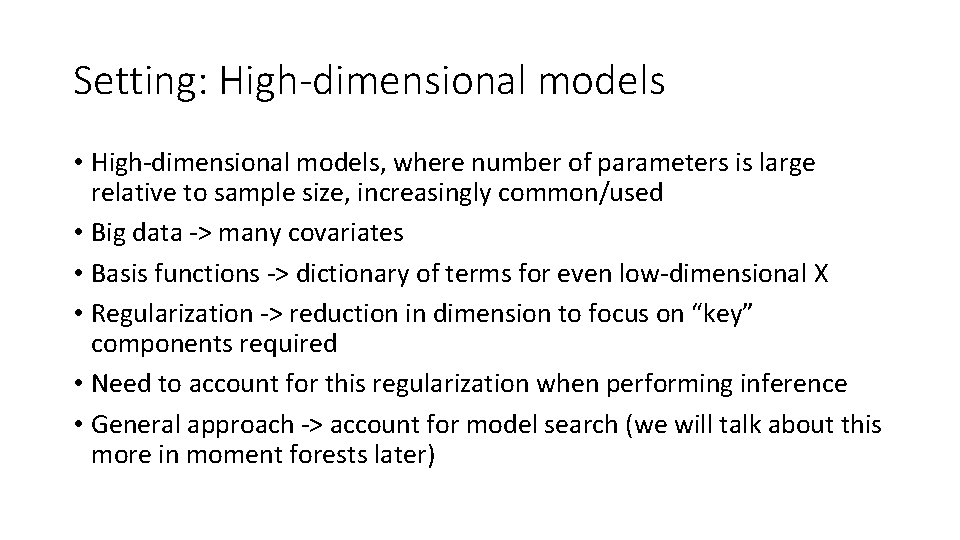
Setting: High-dimensional models • High-dimensional models, where number of parameters is large relative to sample size, increasingly common/used • Big data -> many covariates • Basis functions -> dictionary of terms for even low-dimensional X • Regularization -> reduction in dimension to focus on “key” components required • Need to account for this regularization when performing inference • General approach -> account for model search (we will talk about this more in moment forests later)
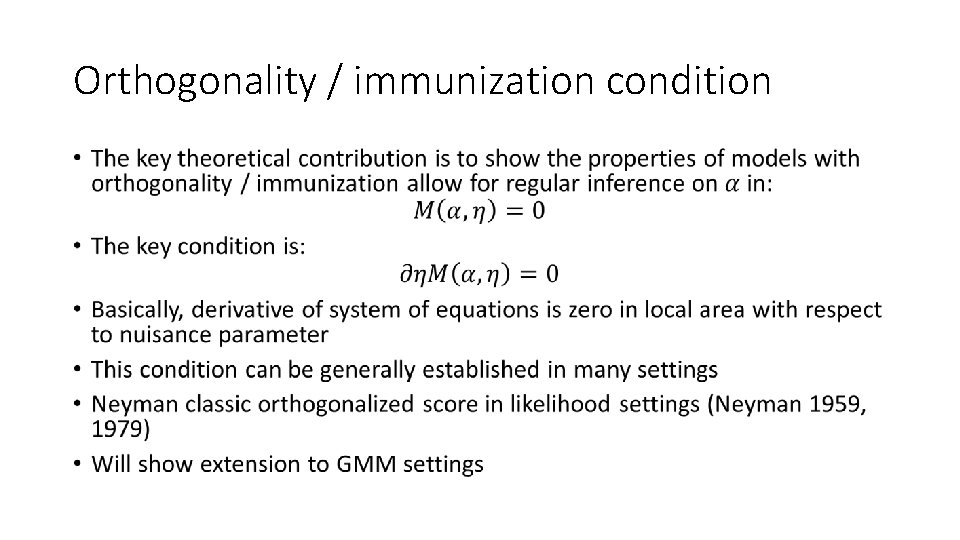
Orthogonality / immunization condition •
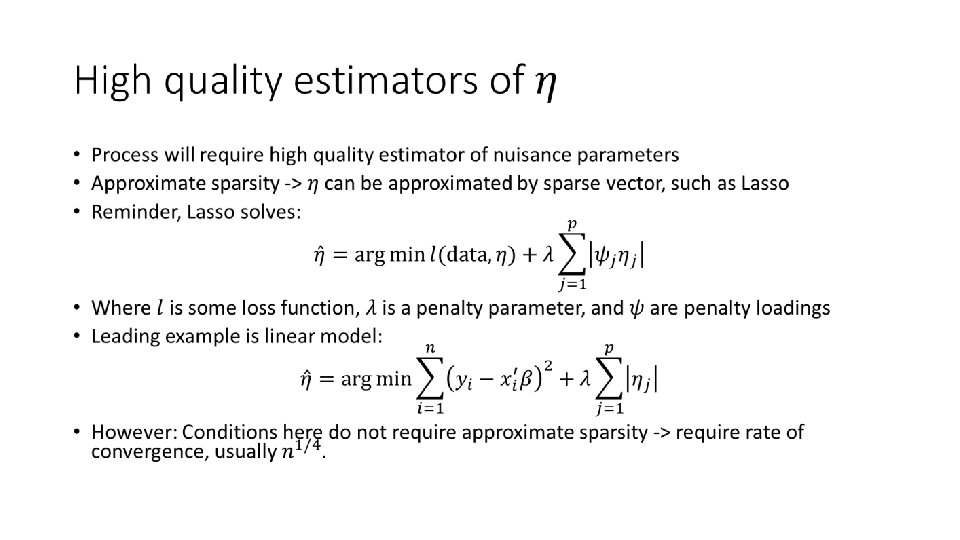
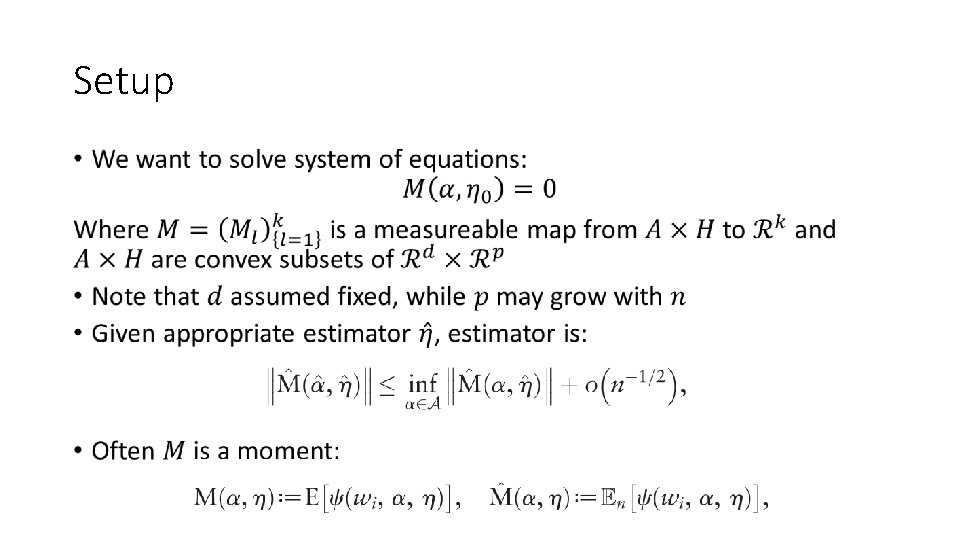
Setup •
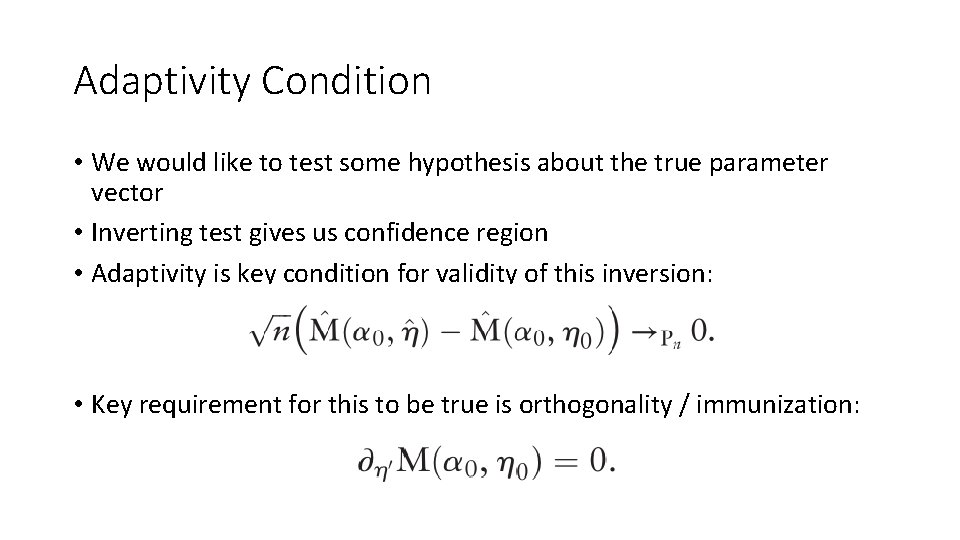
Adaptivity Condition • We would like to test some hypothesis about the true parameter vector • Inverting test gives us confidence region • Adaptivity is key condition for validity of this inversion: • Key requirement for this to be true is orthogonality / immunization:
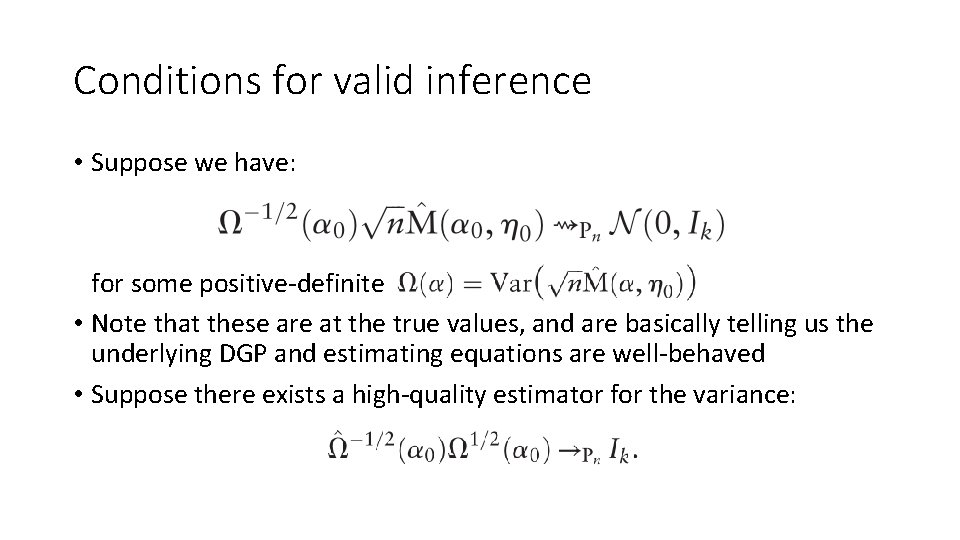
Conditions for valid inference • Suppose we have: for some positive-definite • Note that these are at the true values, and are basically telling us the underlying DGP and estimating equations are well-behaved • Suppose there exists a high-quality estimator for the variance:
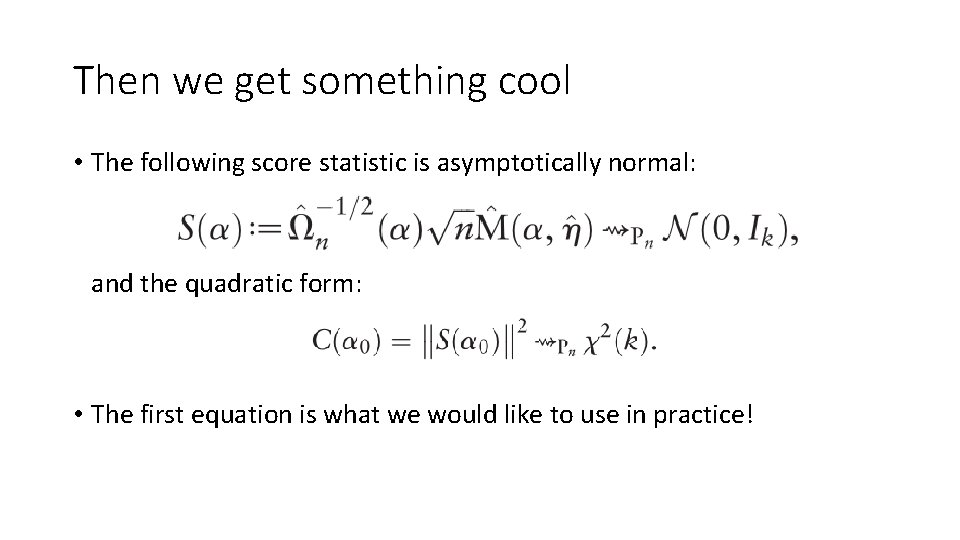
Then we get something cool • The following score statistic is asymptotically normal: and the quadratic form: • The first equation is what we would like to use in practice!
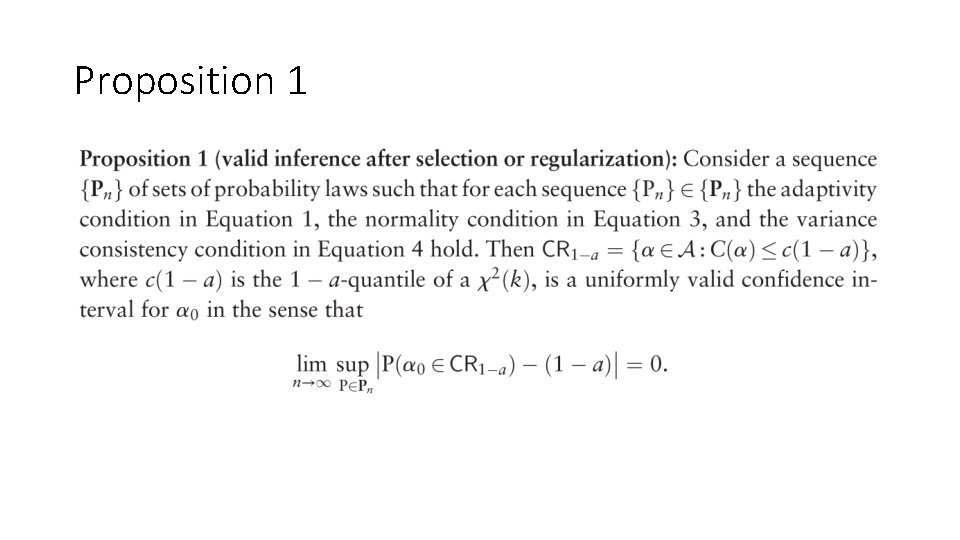
Proposition 1
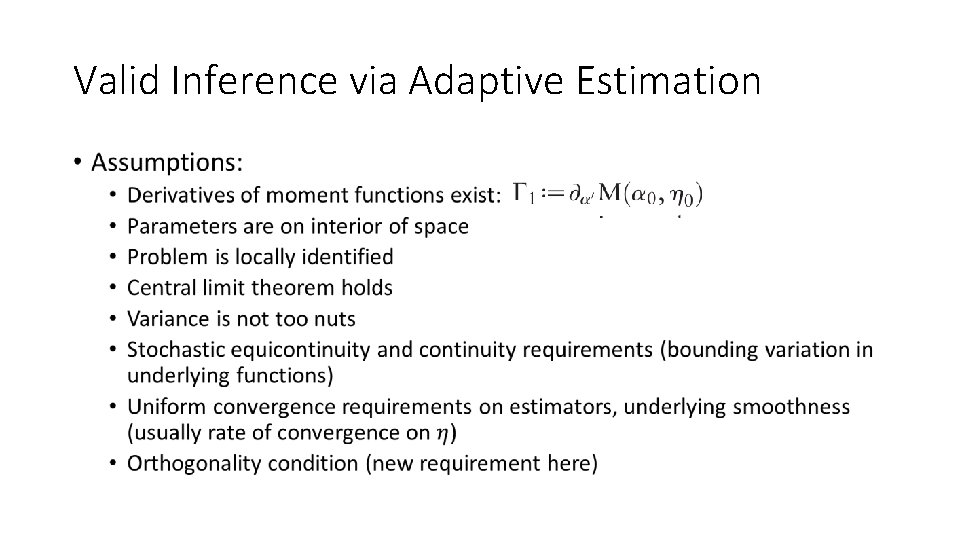
Valid Inference via Adaptive Estimation •
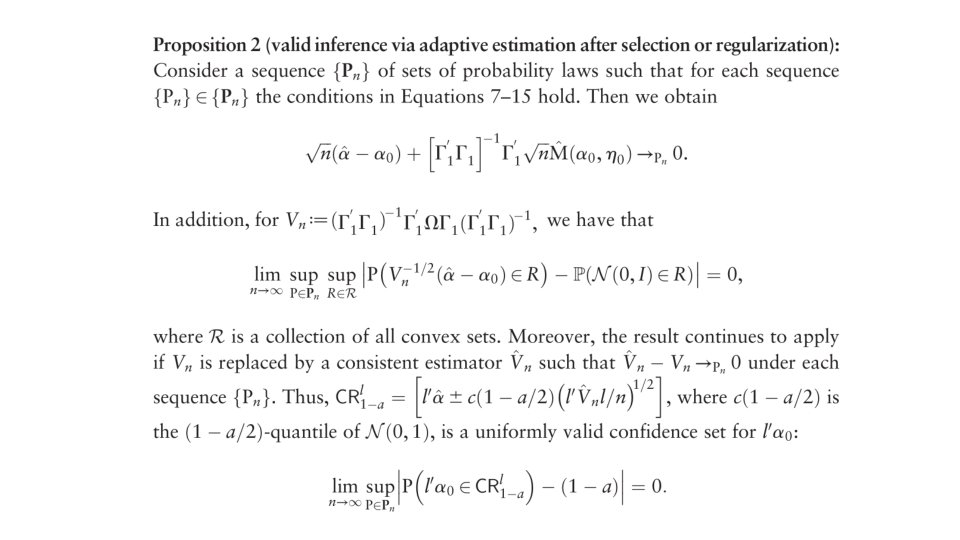
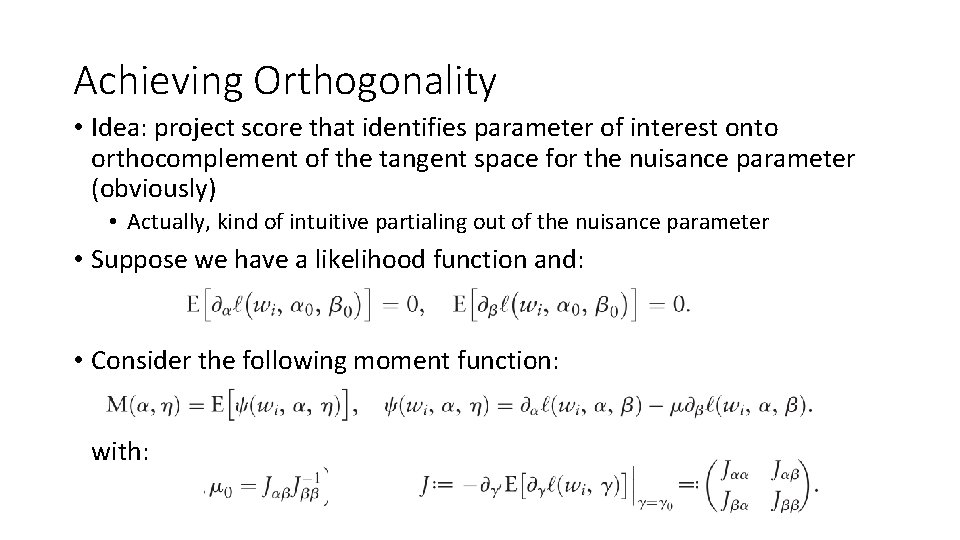
Achieving Orthogonality • Idea: project score that identifies parameter of interest onto orthocomplement of the tangent space for the nuisance parameter (obviously) • Actually, kind of intuitive partialing out of the nuisance parameter • Suppose we have a likelihood function and: • Consider the following moment function: with:
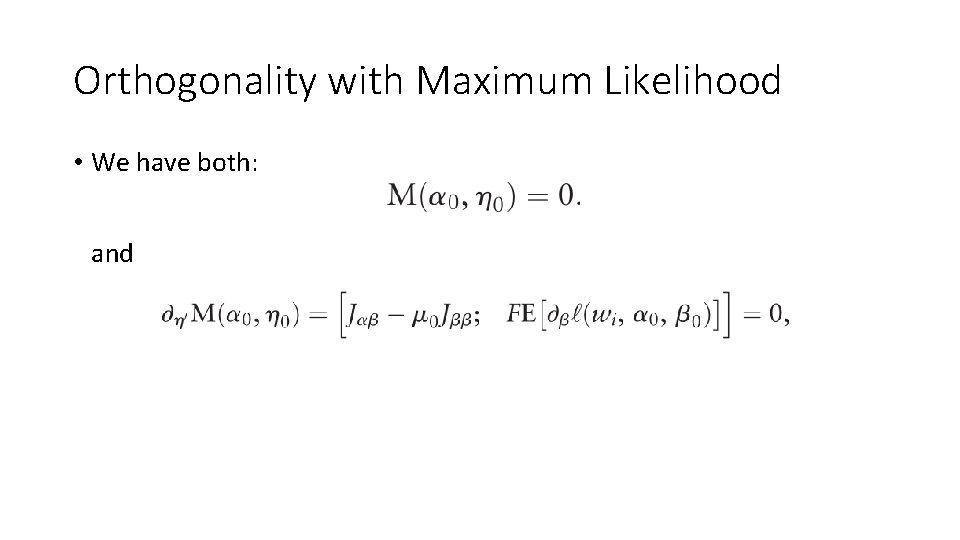
Orthogonality with Maximum Likelihood • We have both: and
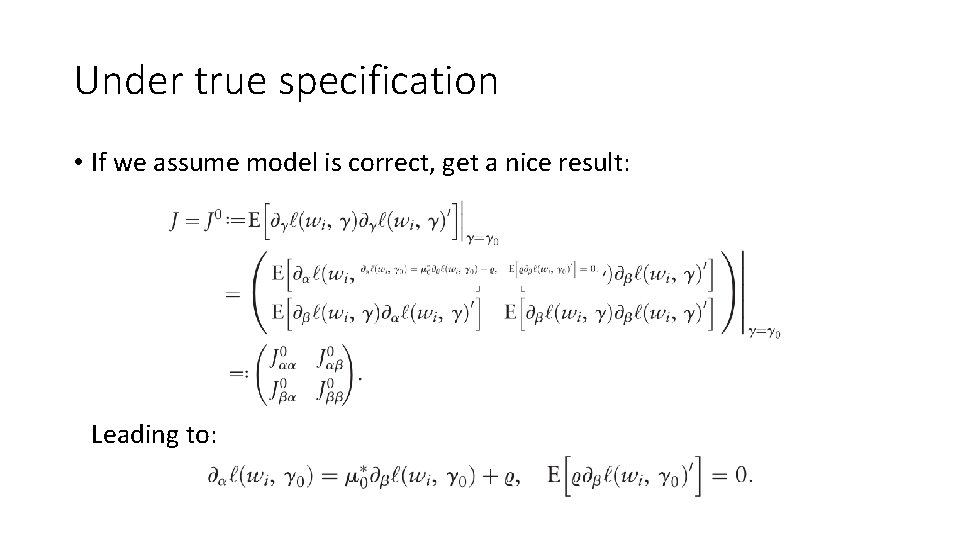
Under true specification • If we assume model is correct, get a nice result: Leading to:
![Lemma 1 Neymans Orthogonalization Lemma 1 [Neyman’s Orthogonalization]](https://slidetodoc.com/presentation_image_h/f2d7ed3fc53342cb2161c703cb4558e5/image-18.jpg)
Lemma 1 [Neyman’s Orthogonalization]
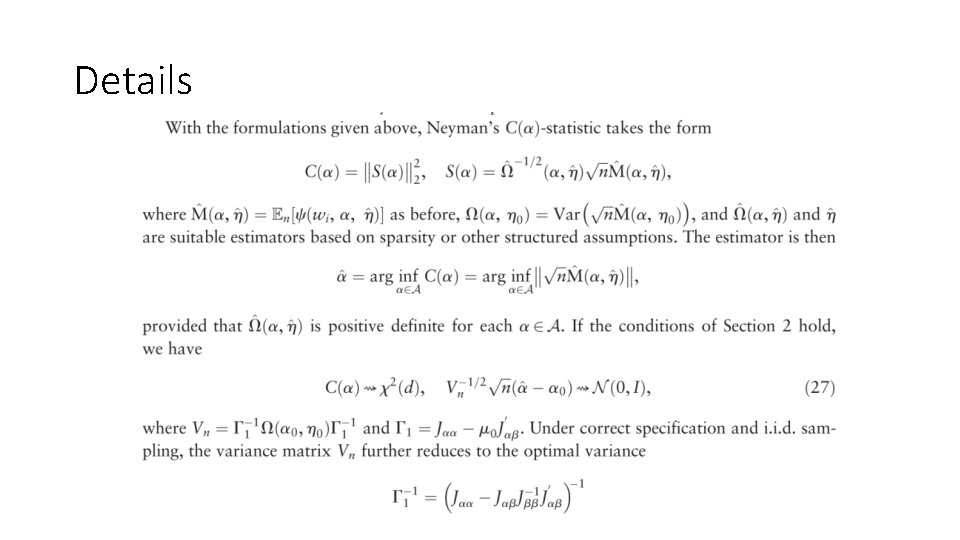
Details
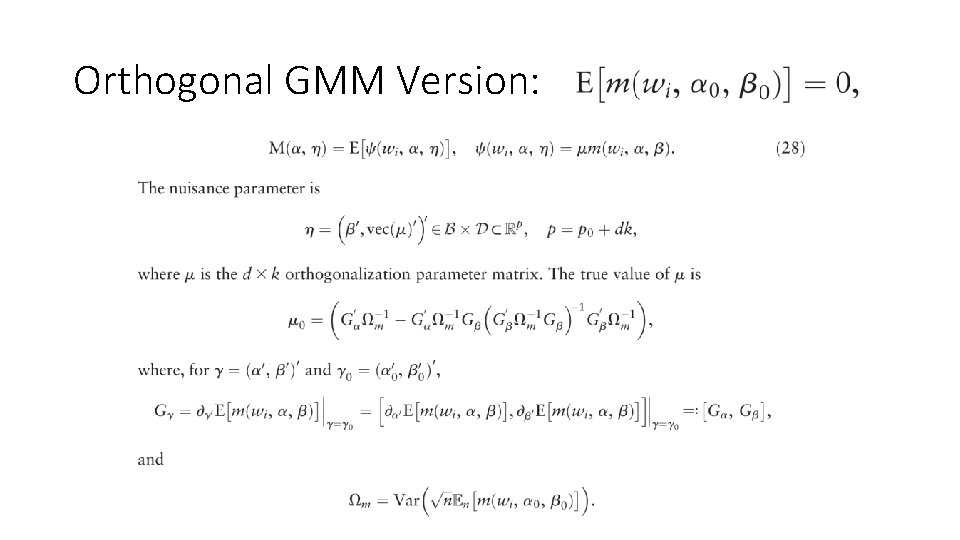
Orthogonal GMM Version:
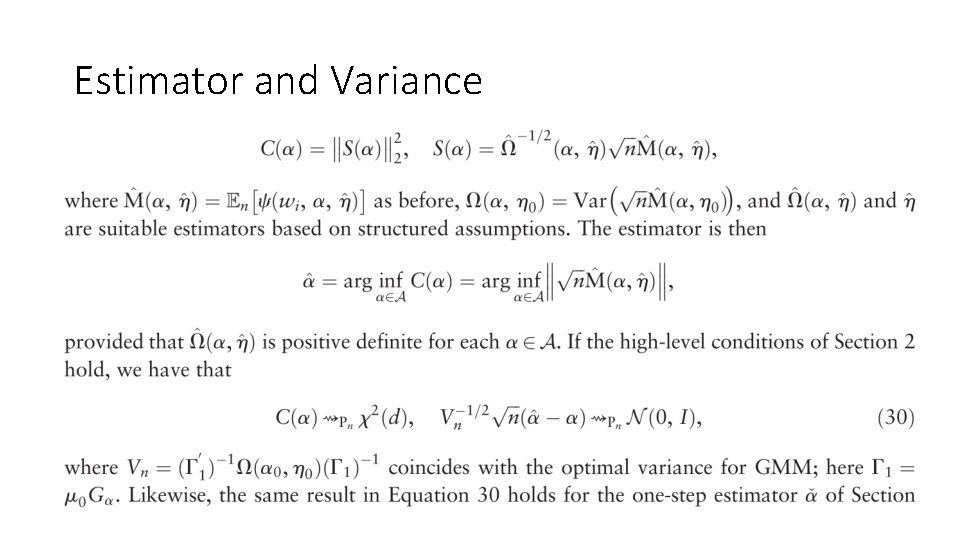
Estimator and Variance
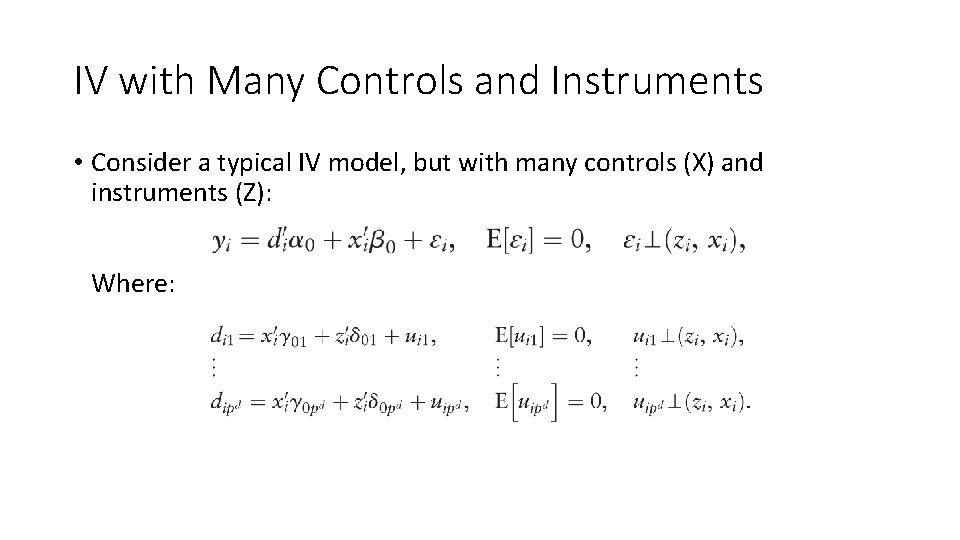
IV with Many Controls and Instruments • Consider a typical IV model, but with many controls (X) and instruments (Z): Where:
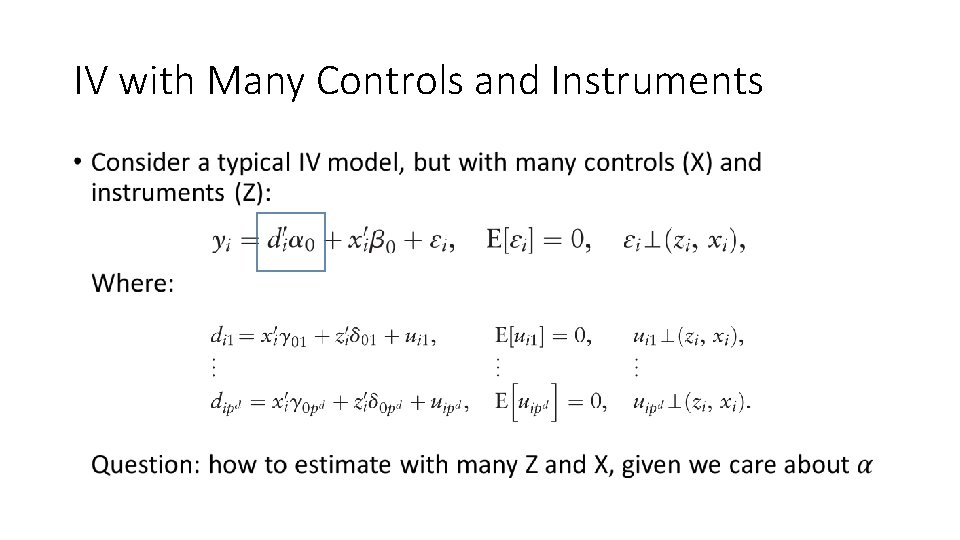
IV with Many Controls and Instruments •
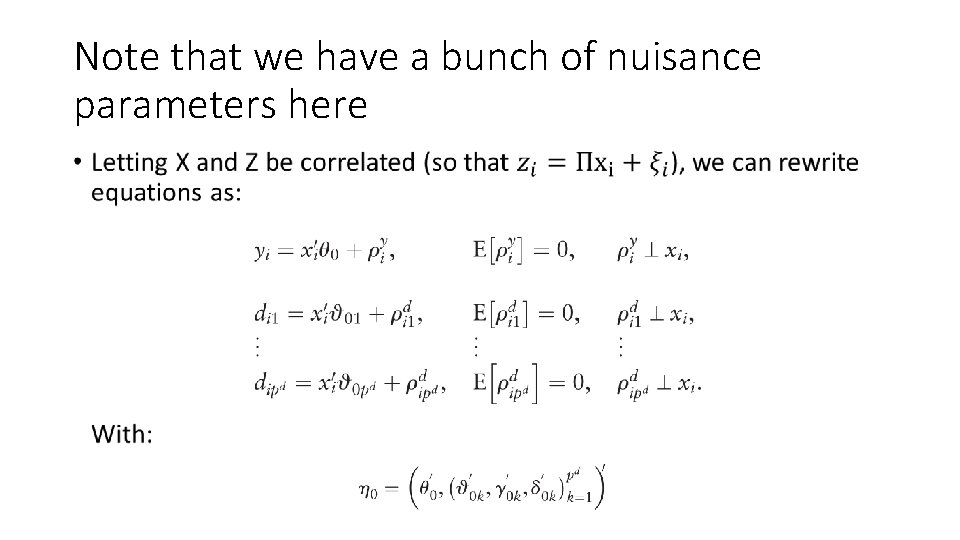
Note that we have a bunch of nuisance parameters here •
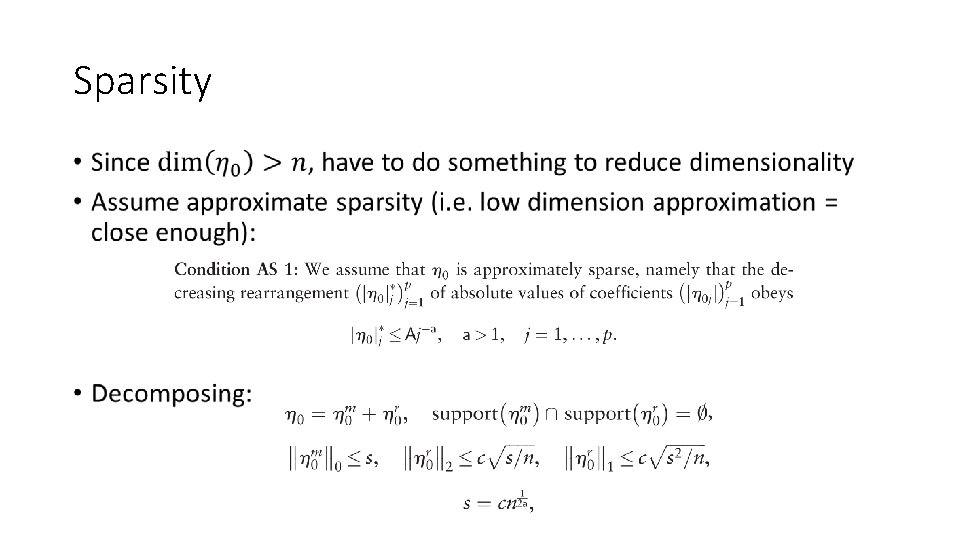
Sparsity •
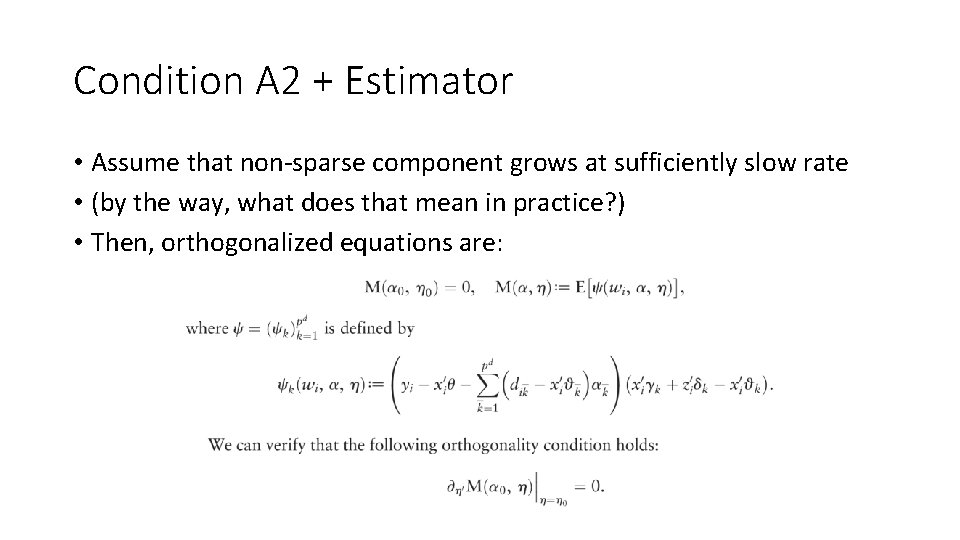
Condition A 2 + Estimator • Assume that non-sparse component grows at sufficiently slow rate • (by the way, what does that mean in practice? ) • Then, orthogonalized equations are:
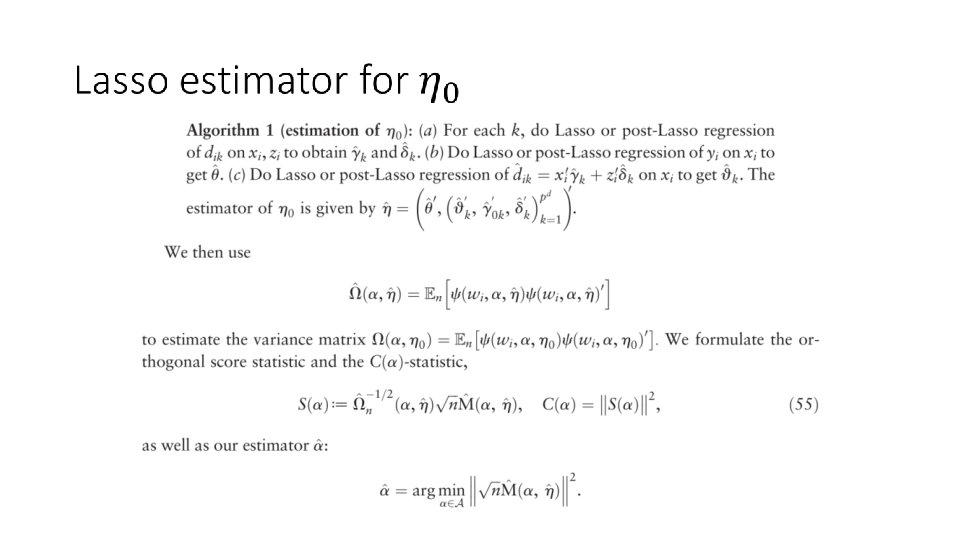
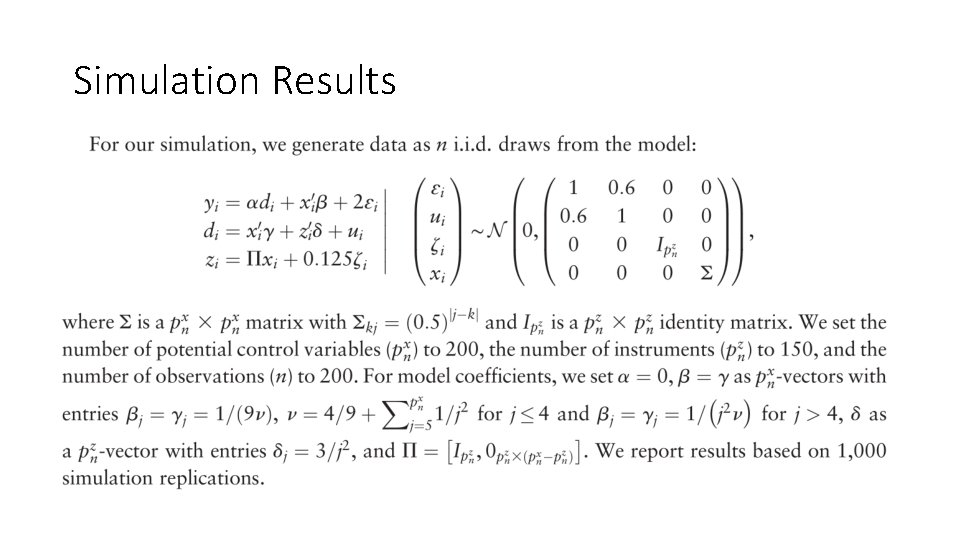
Simulation Results
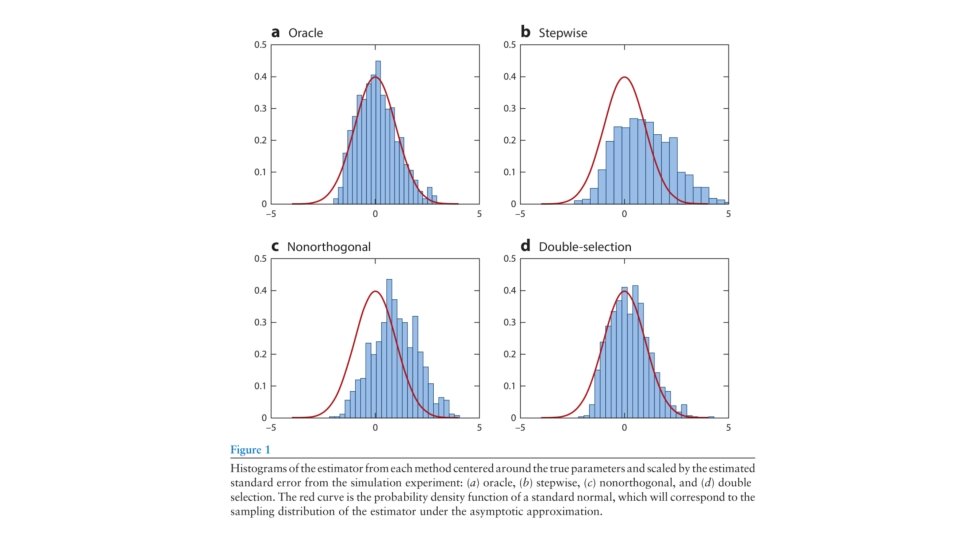
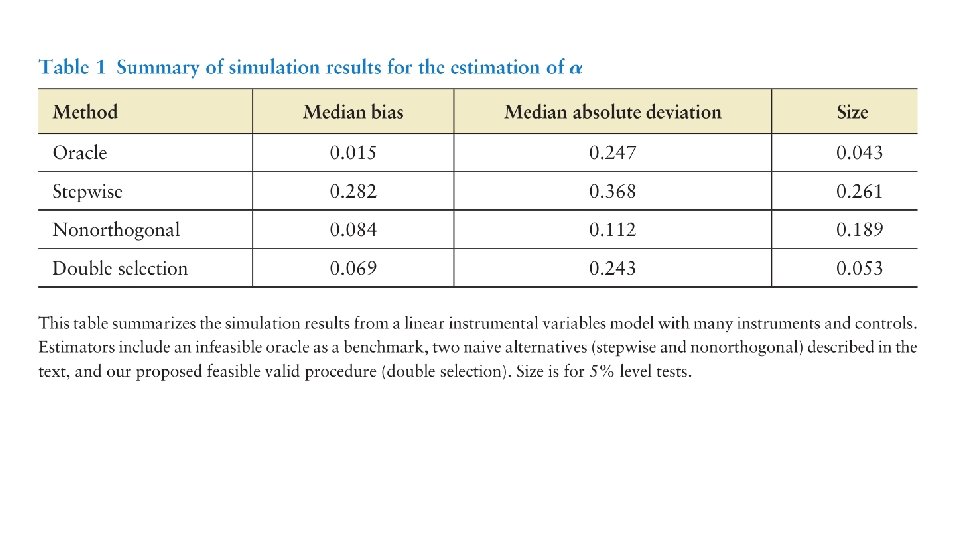
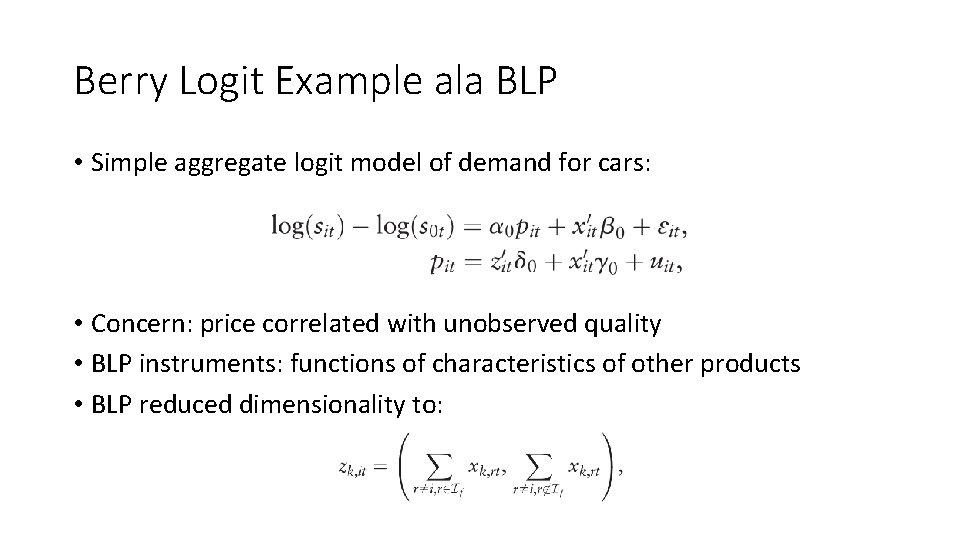
Berry Logit Example ala BLP • Simple aggregate logit model of demand for cars: • Concern: price correlated with unobserved quality • BLP instruments: functions of characteristics of other products • BLP reduced dimensionality to:
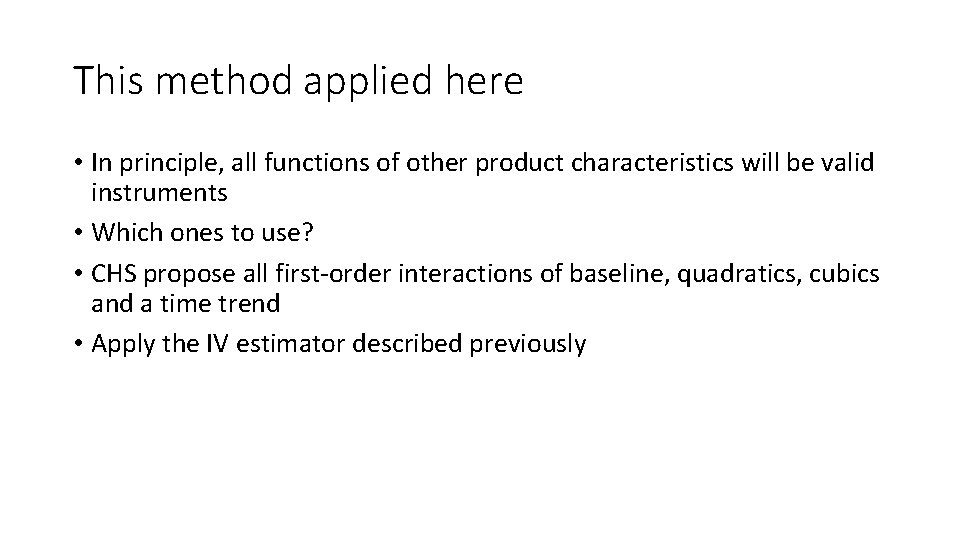
This method applied here • In principle, all functions of other product characteristics will be valid instruments • Which ones to use? • CHS propose all first-order interactions of baseline, quadratics, cubics and a time trend • Apply the IV estimator described previously
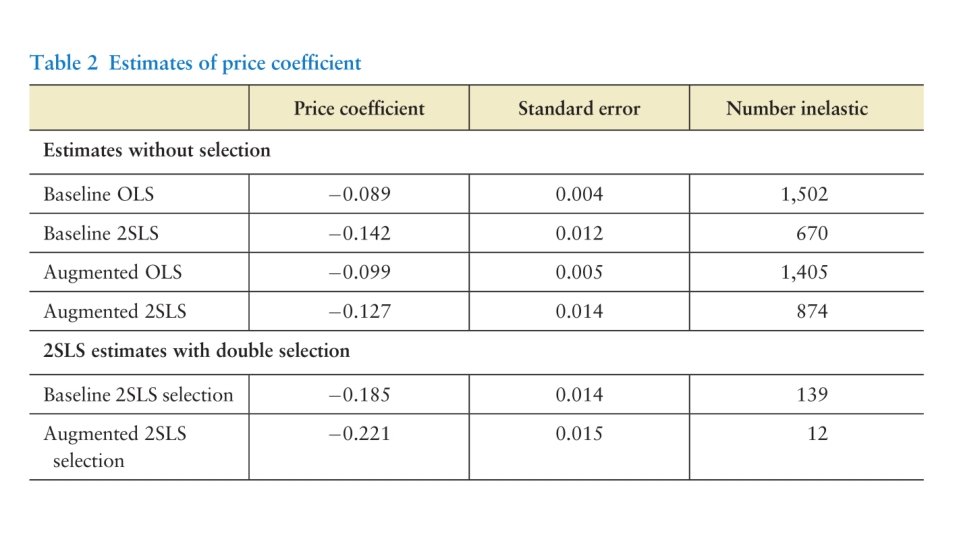
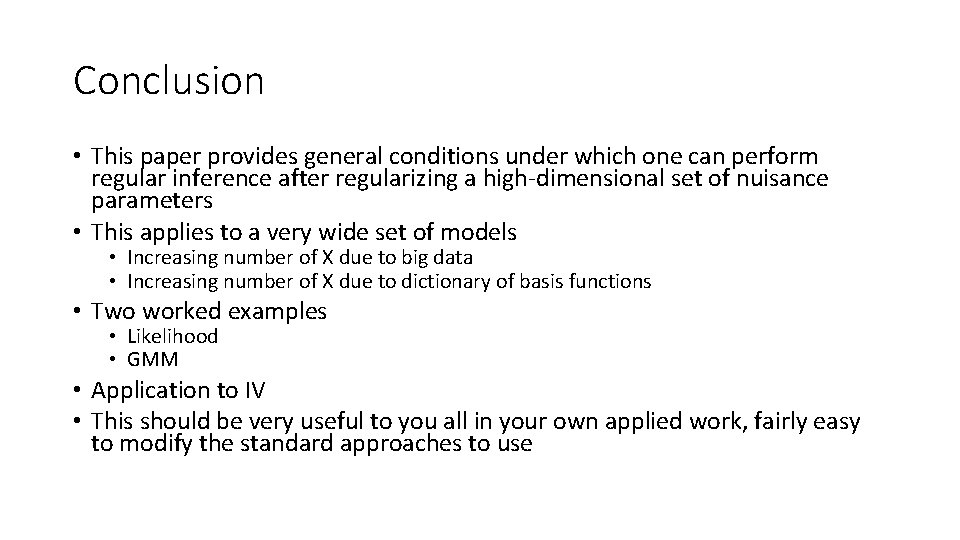
Conclusion • This paper provides general conditions under which one can perform regular inference after regularizing a high-dimensional set of nuisance parameters • This applies to a very wide set of models • Increasing number of X due to big data • Increasing number of X due to dictionary of basis functions • Two worked examples • Likelihood • GMM • Application to IV • This should be very useful to you all in your own applied work, fairly easy to modify the standard approaches to use
Methodology of econometrics
Stephen p ryan
Fr olin mayfield
Zubi olin
Lena olin sabina
Erik olin wright sınıf kuramı
Mina olin siin kokkuvõte
01:640:244 lecture notes - lecture 15: plat, idah, farad
Pencounters
Mcit 592
Foundations of group behavior
Software architecture foundations theory and practice
Secure foundations
The "great awakening" laid the social foundations for the
Idempotent law example
Sigma simplicity
Aohs foundations of anatomy and physiology 1
Aohs foundations of anatomy and physiology 1
Philosophical foundations of the american revolution
Foundations of faith elder cook
Six sigma foundations
Foundations of cybersecurity northeastern
Foundation of information system in business
Clinical supervision foundations ii
Sefel training
Foundations of individual behavior
Software architecture foundations theory and practice
Different foundations of curriculum
18734 cmu
Foundations of clinical research applications to practice
The generic term for the guest room sheets and pillowcases
New foundations home for children
Preschool learning foundations
Foundations of organization structure
Perennialism curriculum trends