JPMTAnalogue Signal Processor PMT analogue signal processor in
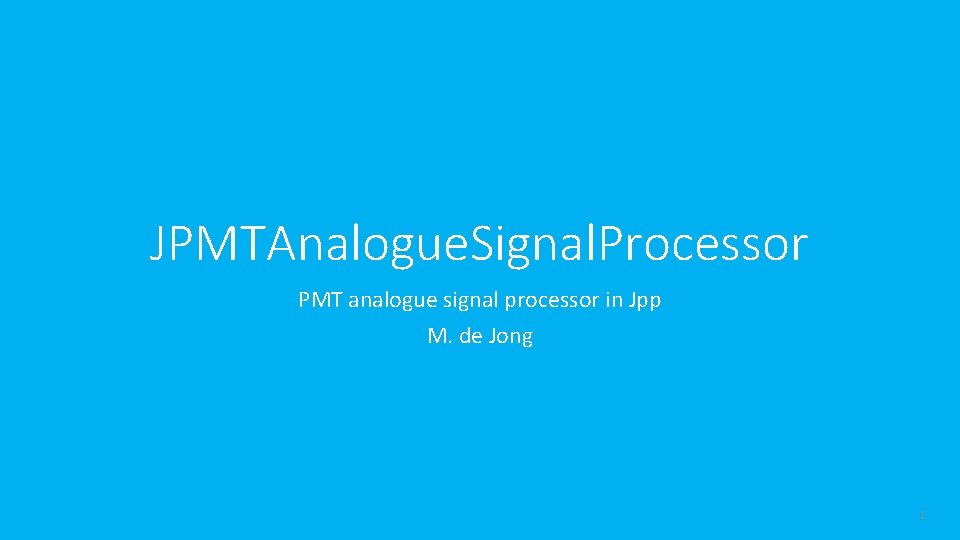
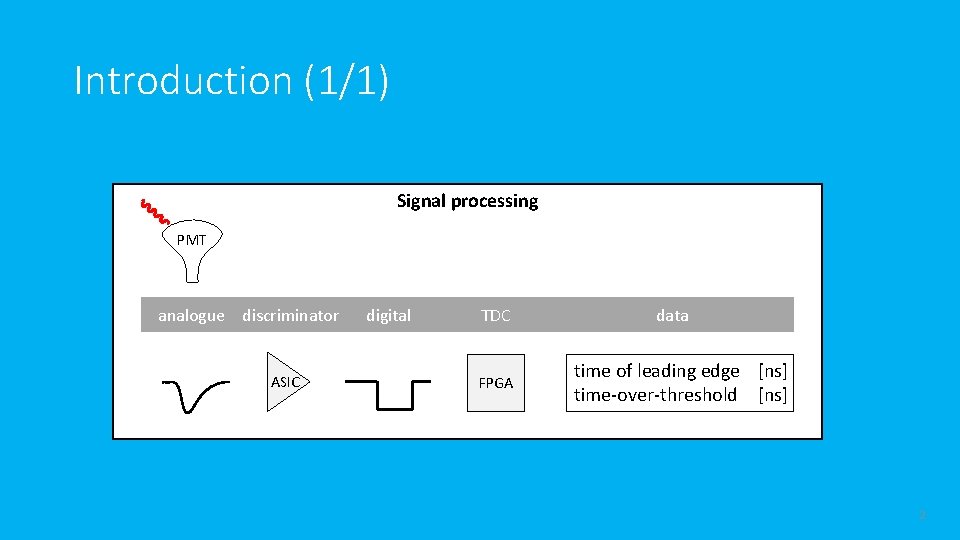
![Introduction (2/3) amplitude [p. e. ] definition gain spread threshold time-over-threshold time 3 Introduction (2/3) amplitude [p. e. ] definition gain spread threshold time-over-threshold time 3](https://slidetodoc.com/presentation_image_h2/3f4618d58ce64b4fb3418e93e8fbedc4/image-3.jpg)
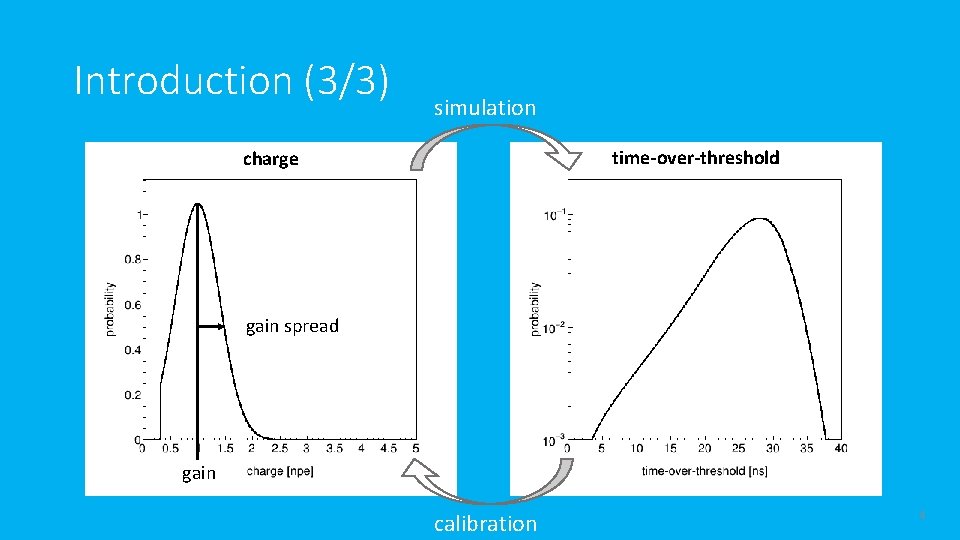
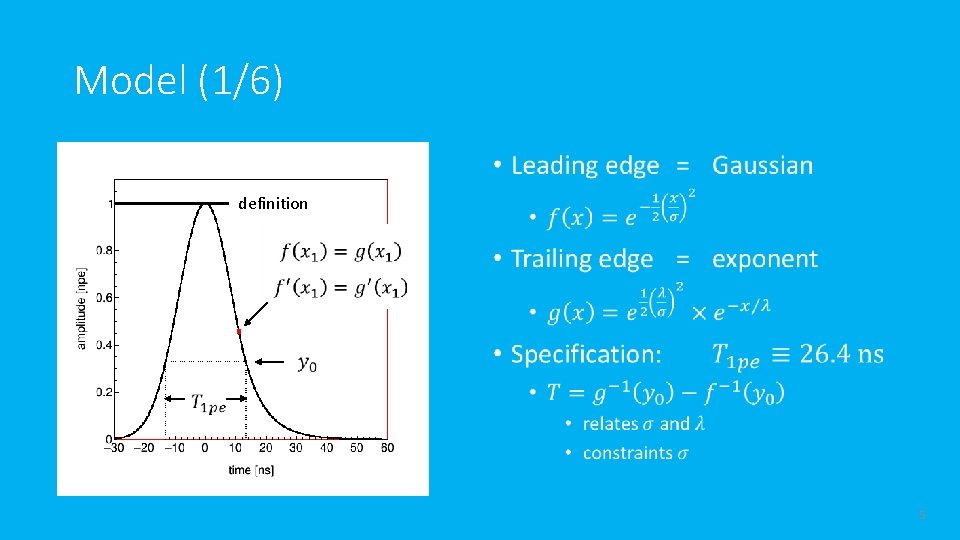
![Model (2/6) 2 number of hits [a. u. ] Bram Schermer 1 (Nikhef) 3 Model (2/6) 2 number of hits [a. u. ] Bram Schermer 1 (Nikhef) 3](https://slidetodoc.com/presentation_image_h2/3f4618d58ce64b4fb3418e93e8fbedc4/image-6.jpg)
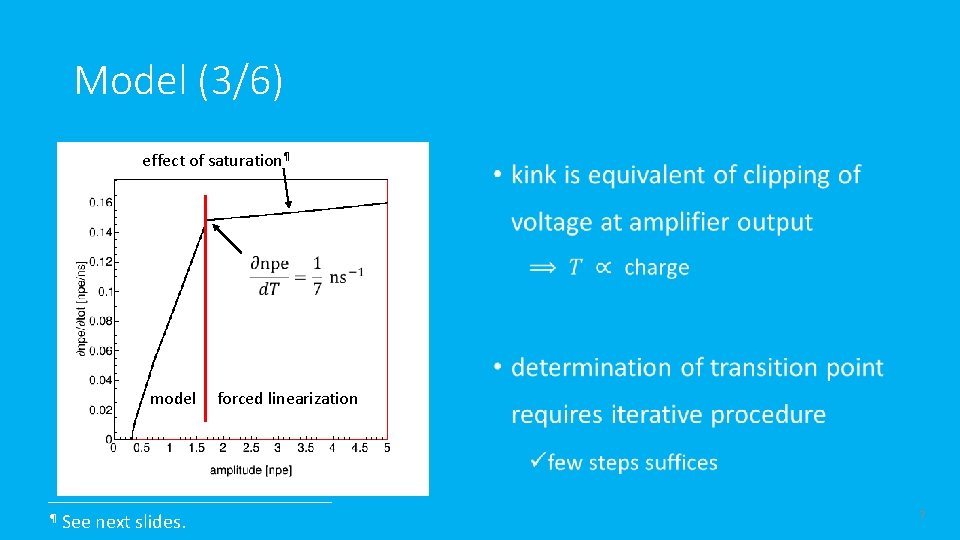
![Model (4/6) number of hits [a. u. ] Model for given number of photo-electrons Model (4/6) number of hits [a. u. ] Model for given number of photo-electrons](https://slidetodoc.com/presentation_image_h2/3f4618d58ce64b4fb3418e93e8fbedc4/image-8.jpg)
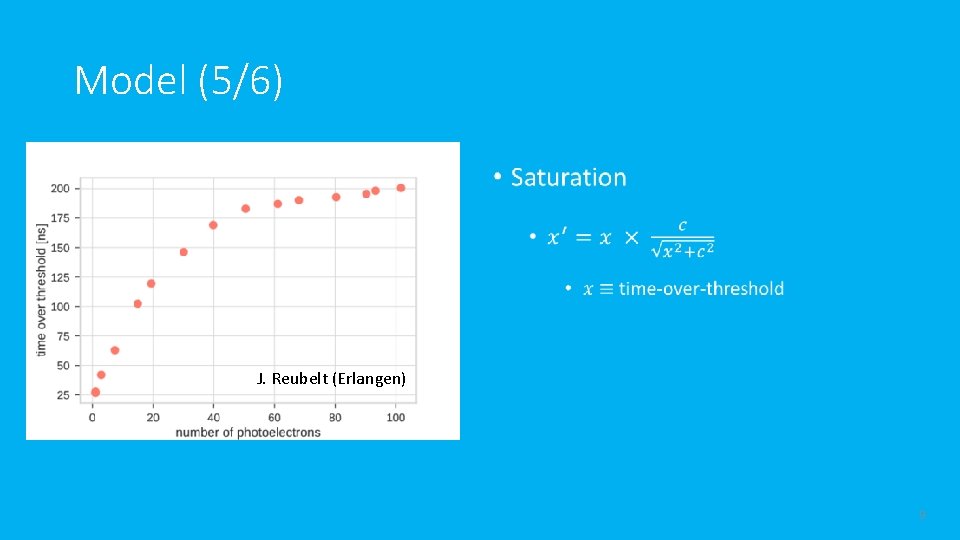
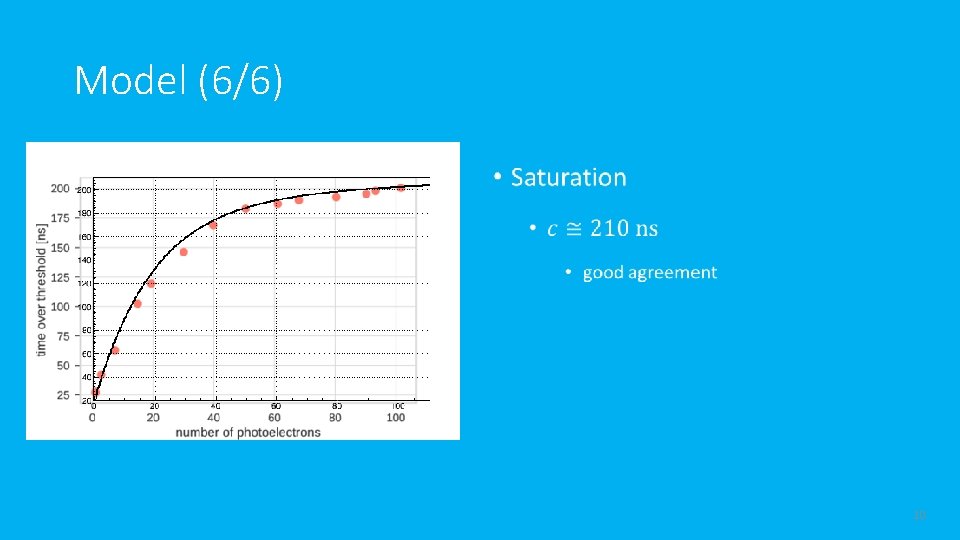
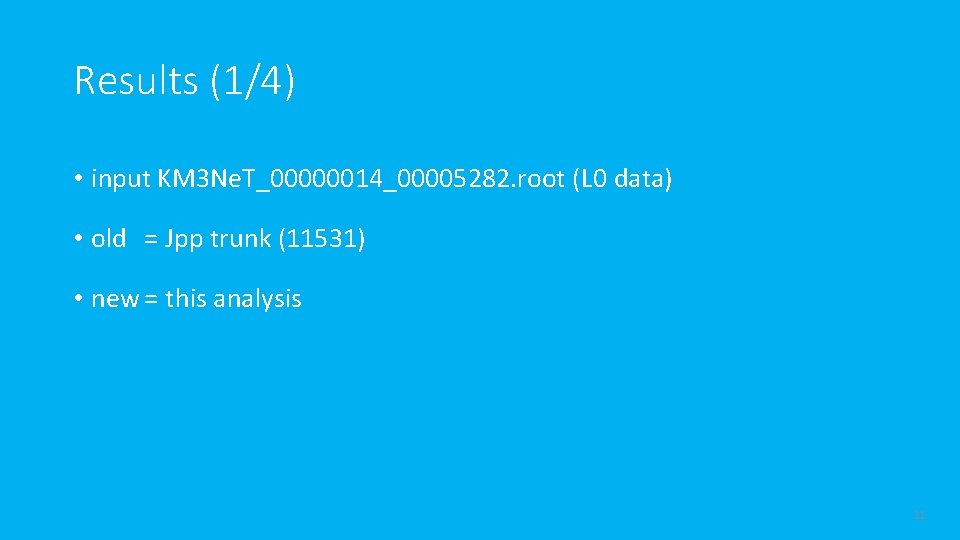
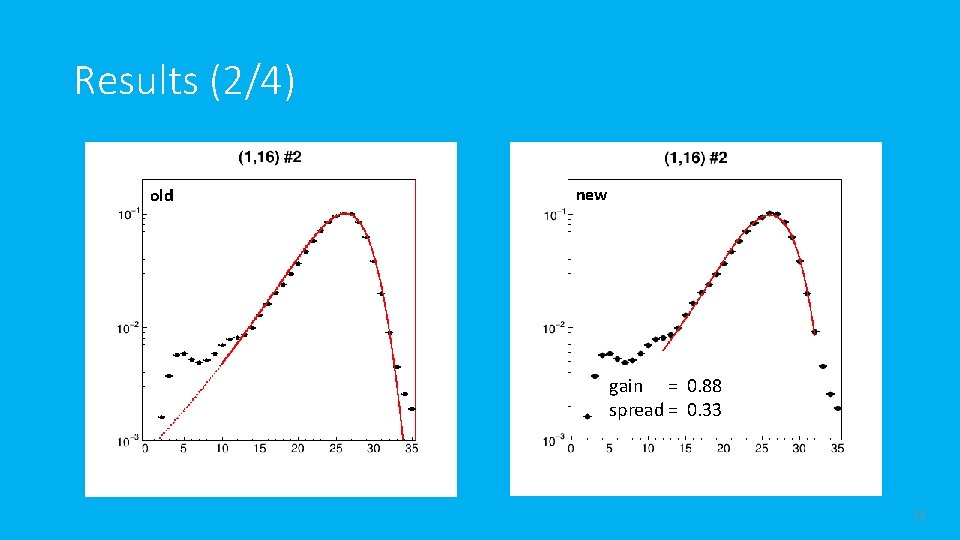
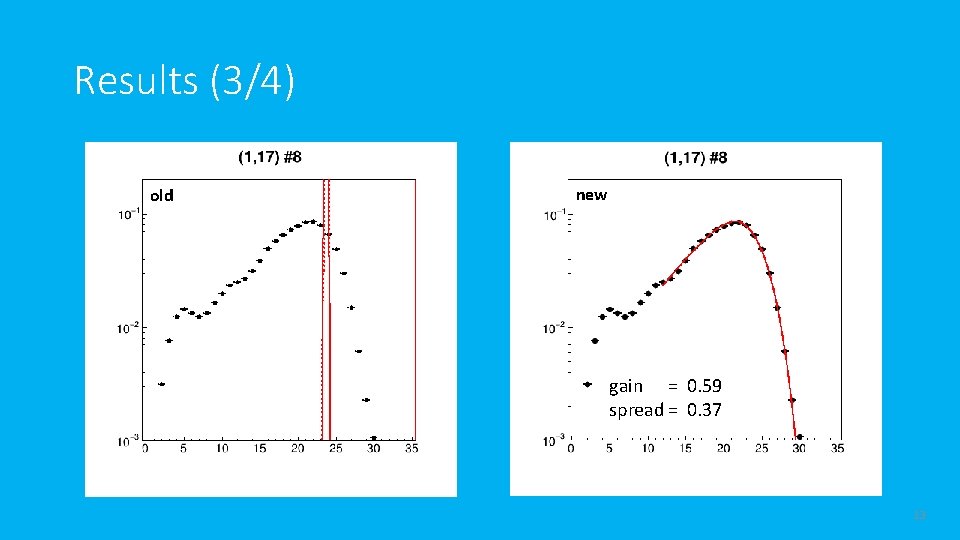
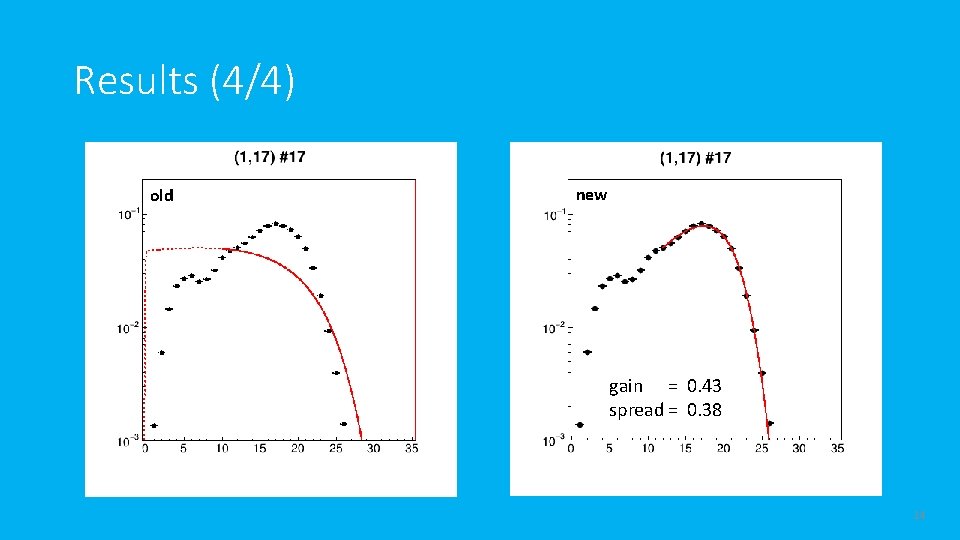
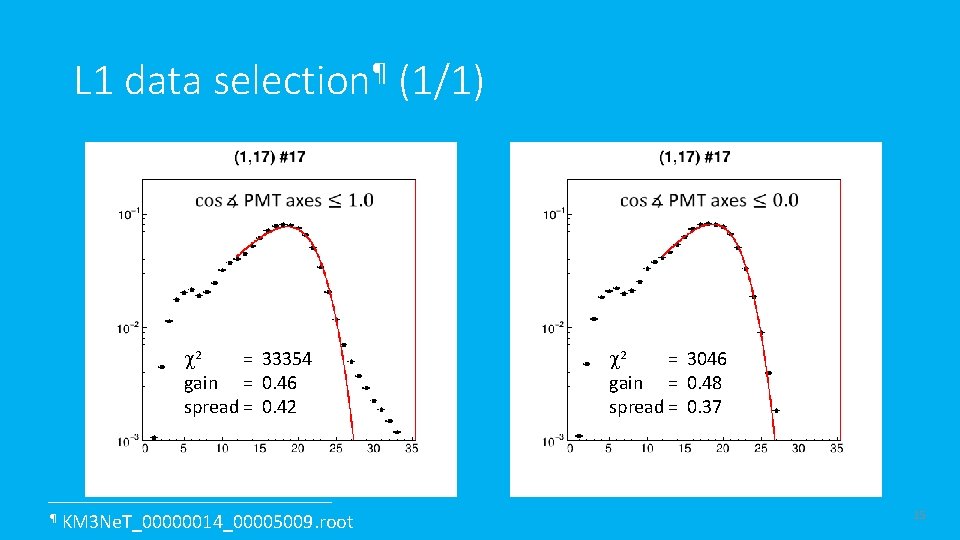
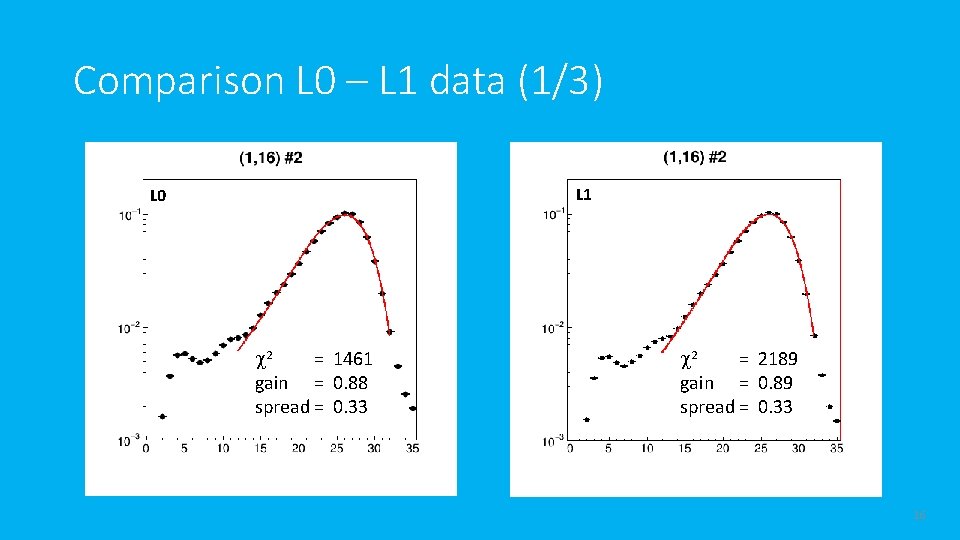
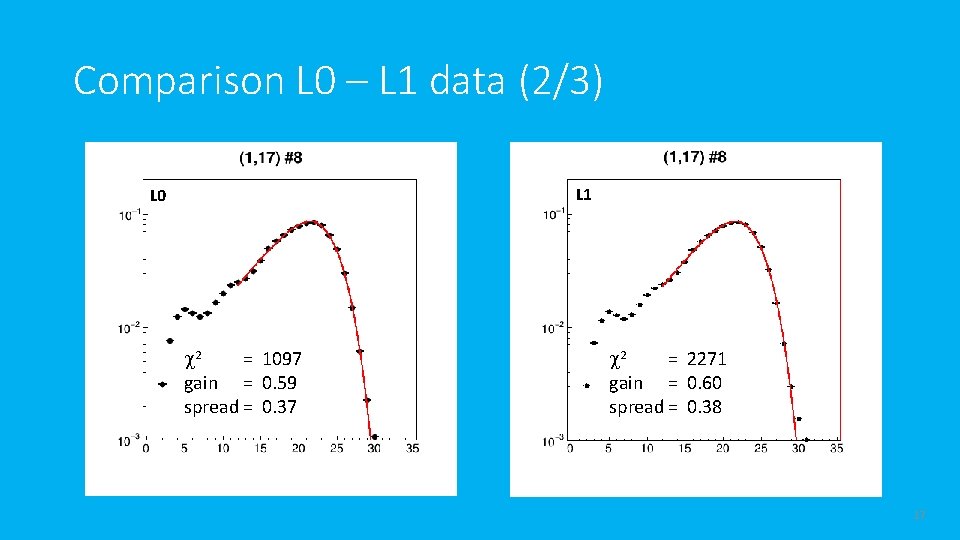
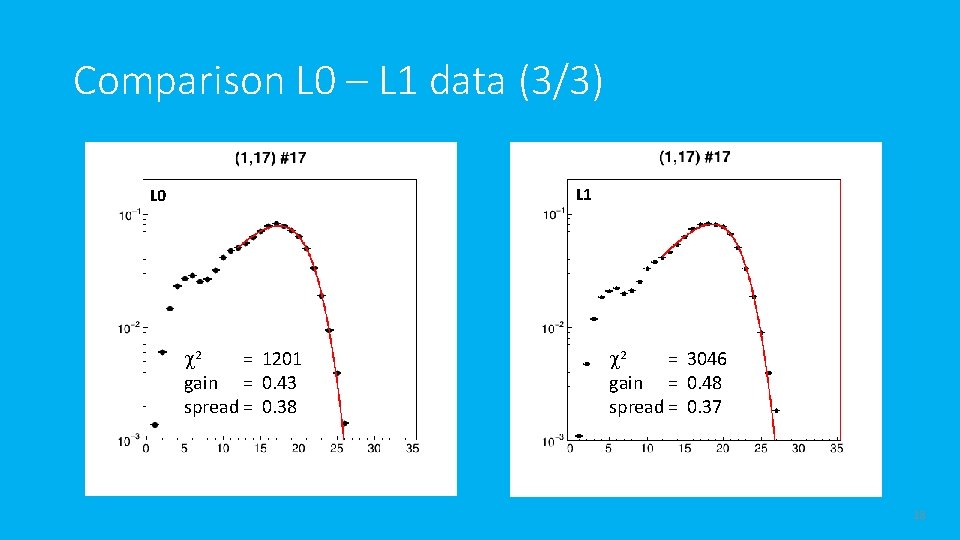
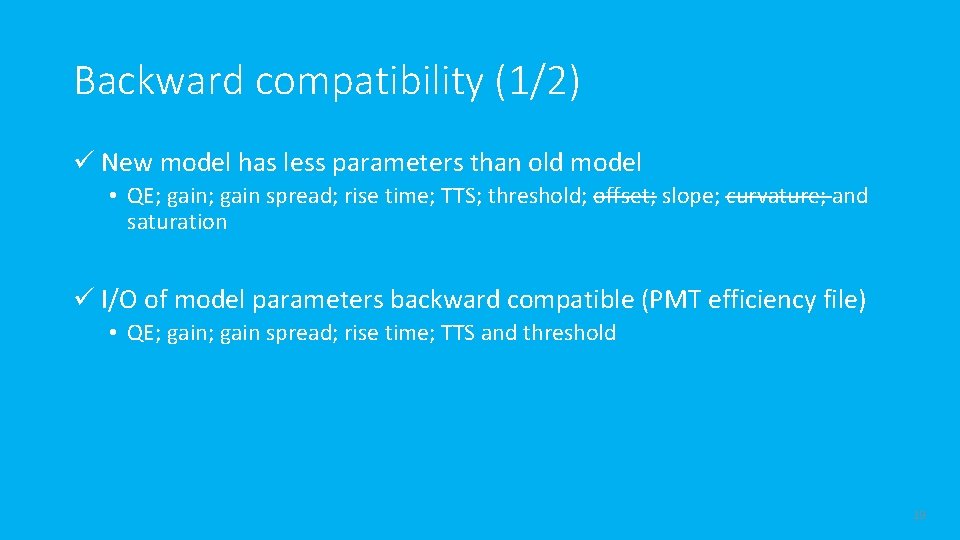
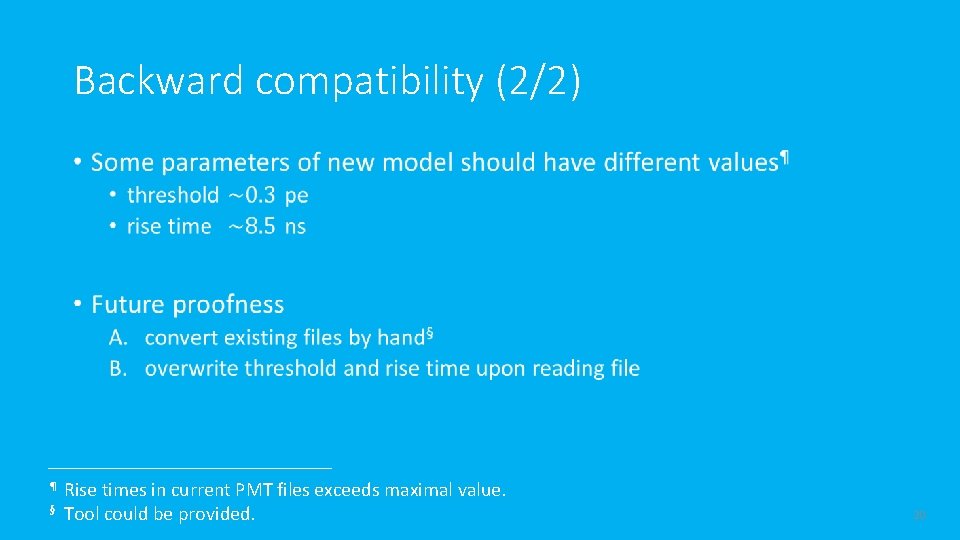
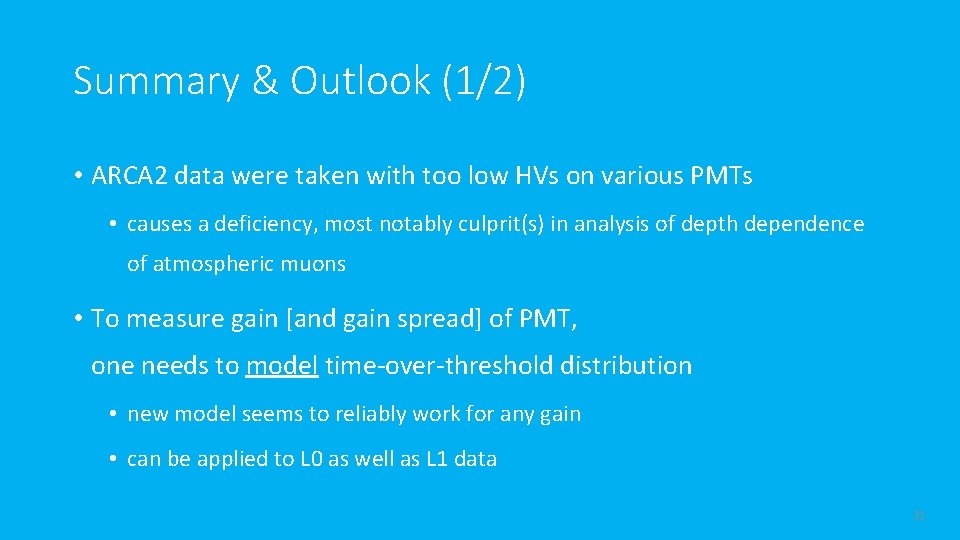
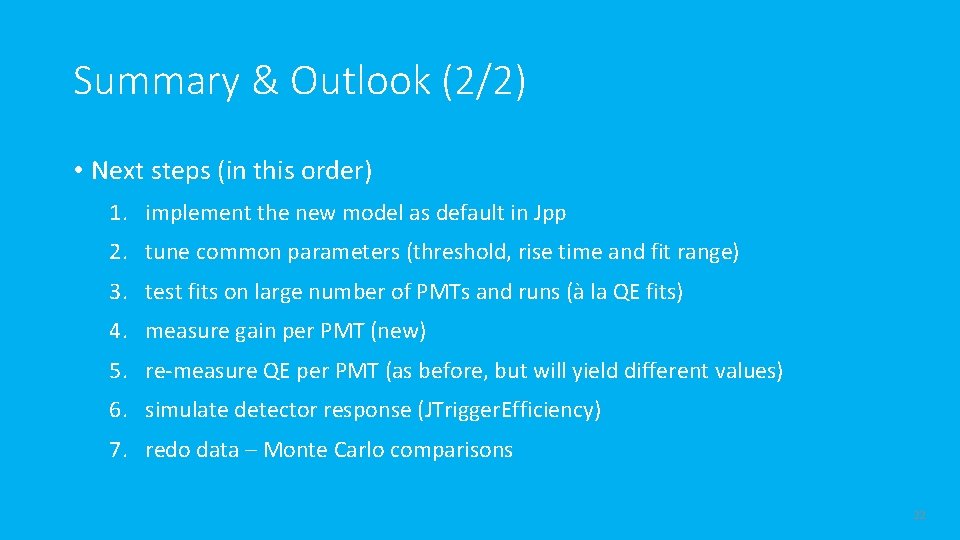
- Slides: 22
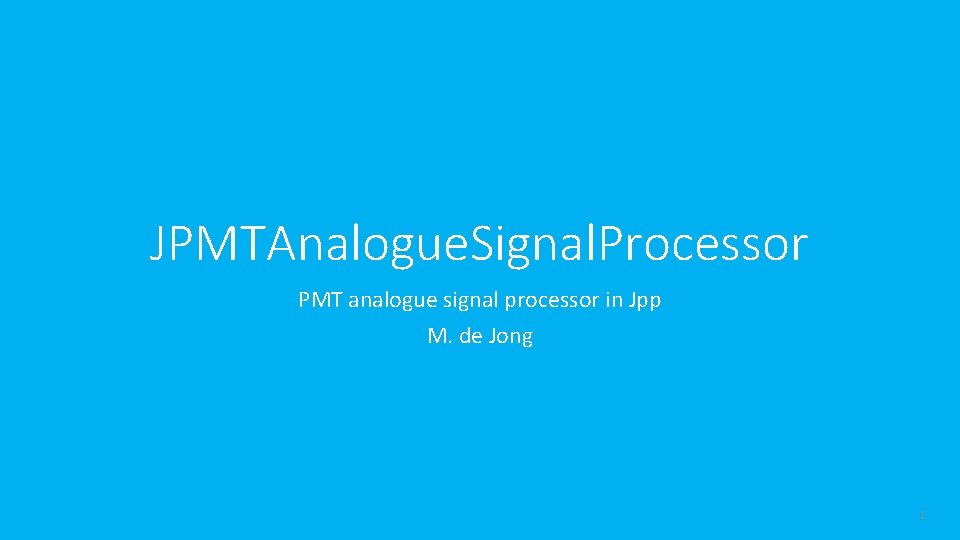
JPMTAnalogue. Signal. Processor PMT analogue signal processor in Jpp M. de Jong 1
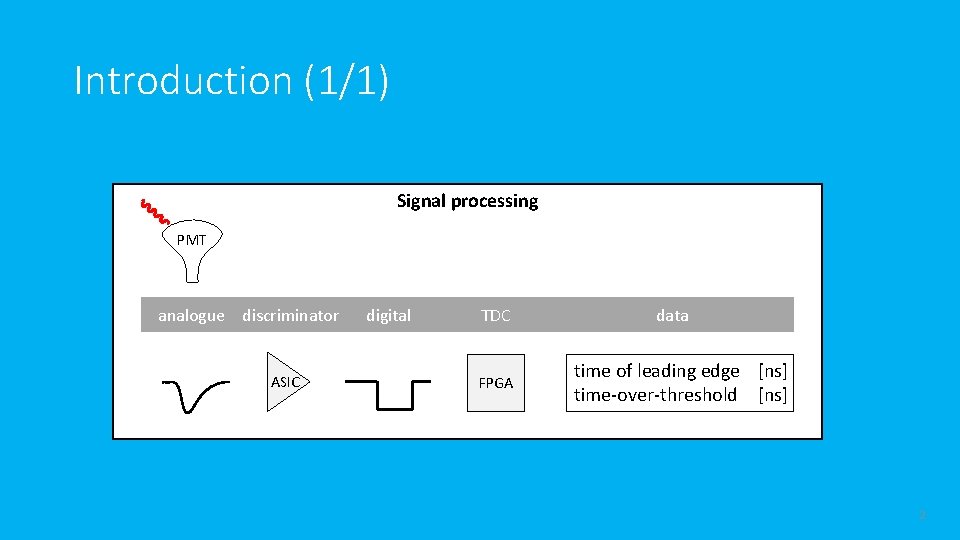
Introduction (1/1) Signal processing PMT analogue discriminator ASIC digital TDC FPGA data time of leading edge [ns] time-over-threshold [ns] 2
![Introduction 23 amplitude p e definition gain spread threshold timeoverthreshold time 3 Introduction (2/3) amplitude [p. e. ] definition gain spread threshold time-over-threshold time 3](https://slidetodoc.com/presentation_image_h2/3f4618d58ce64b4fb3418e93e8fbedc4/image-3.jpg)
Introduction (2/3) amplitude [p. e. ] definition gain spread threshold time-over-threshold time 3
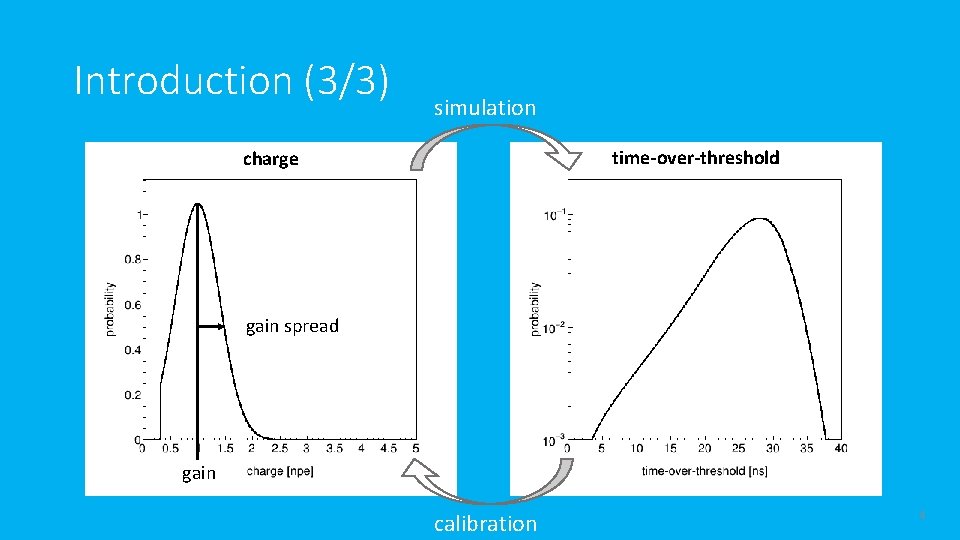
Introduction (3/3) simulation time-over-threshold charge gain spread gain calibration 4
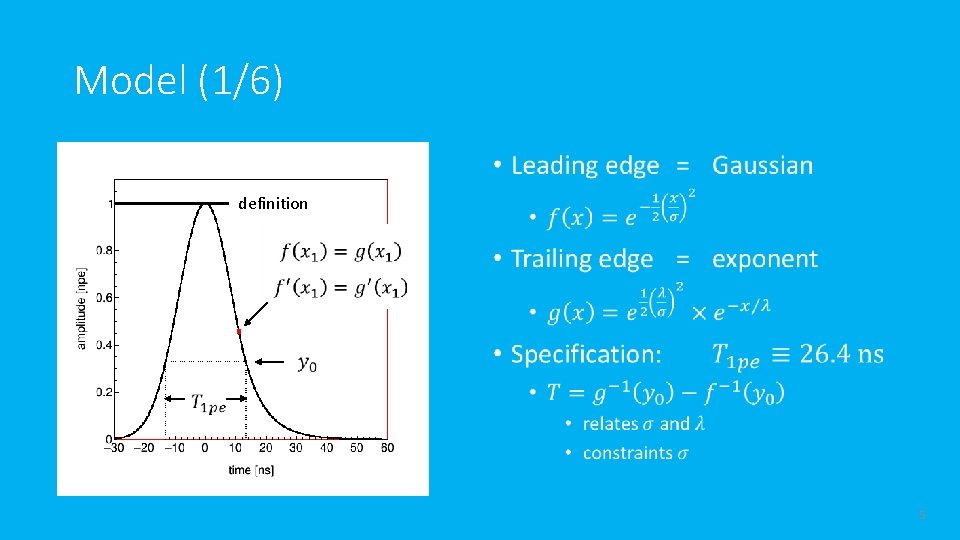
Model (1/6) • definition 5
![Model 26 2 number of hits a u Bram Schermer 1 Nikhef 3 Model (2/6) 2 number of hits [a. u. ] Bram Schermer 1 (Nikhef) 3](https://slidetodoc.com/presentation_image_h2/3f4618d58ce64b4fb3418e93e8fbedc4/image-6.jpg)
Model (2/6) 2 number of hits [a. u. ] Bram Schermer 1 (Nikhef) 3 4 5 peak 1 2 3 ns 27 35 42 4 5 6 49 56 62 6 time over threshold [ns] 6
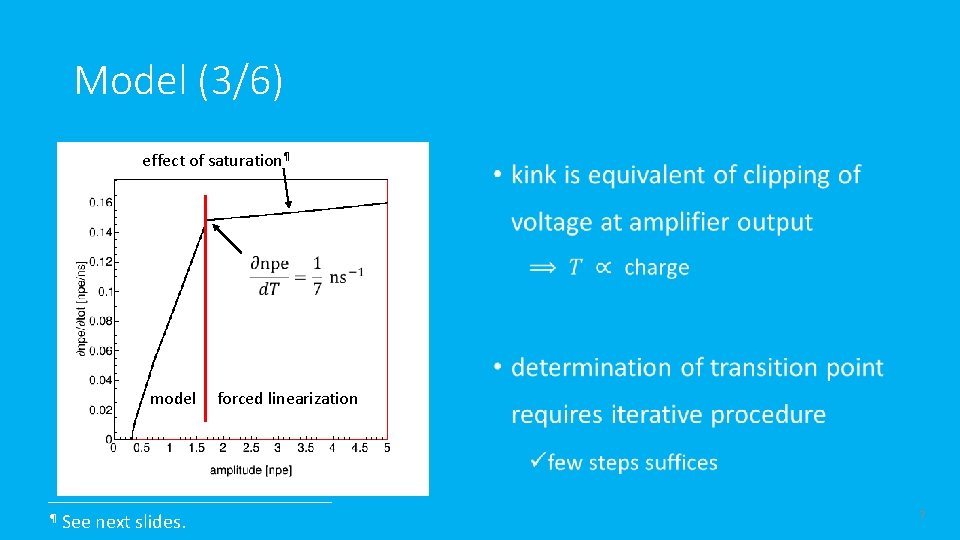
Model (3/6) effect of saturation¶ model ¶ See next slides. • forced linearization 7
![Model 46 number of hits a u Model for given number of photoelectrons Model (4/6) number of hits [a. u. ] Model for given number of photo-electrons](https://slidetodoc.com/presentation_image_h2/3f4618d58ce64b4fb3418e93e8fbedc4/image-8.jpg)
Model (4/6) number of hits [a. u. ] Model for given number of photo-electrons time over threshold [ns] 8
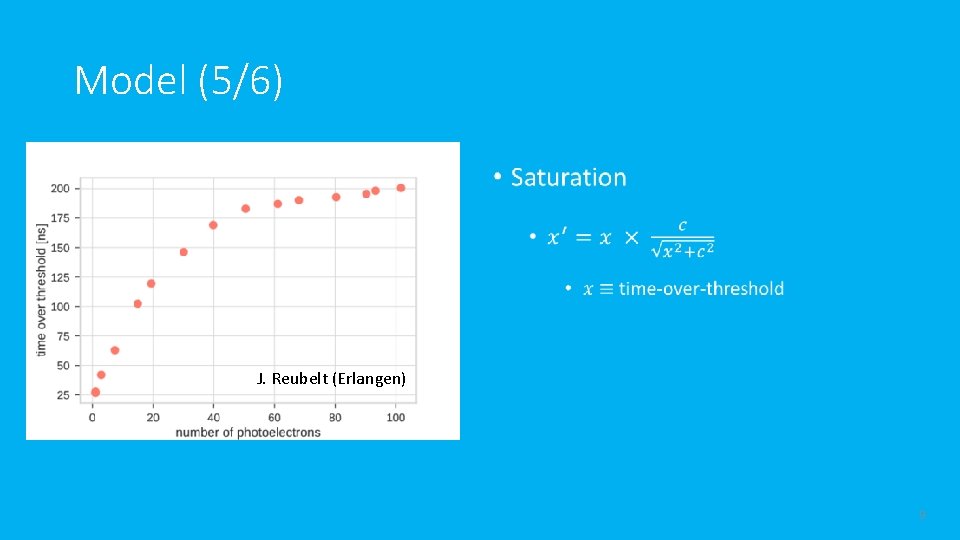
Model (5/6) • J. Reubelt (Erlangen) 9
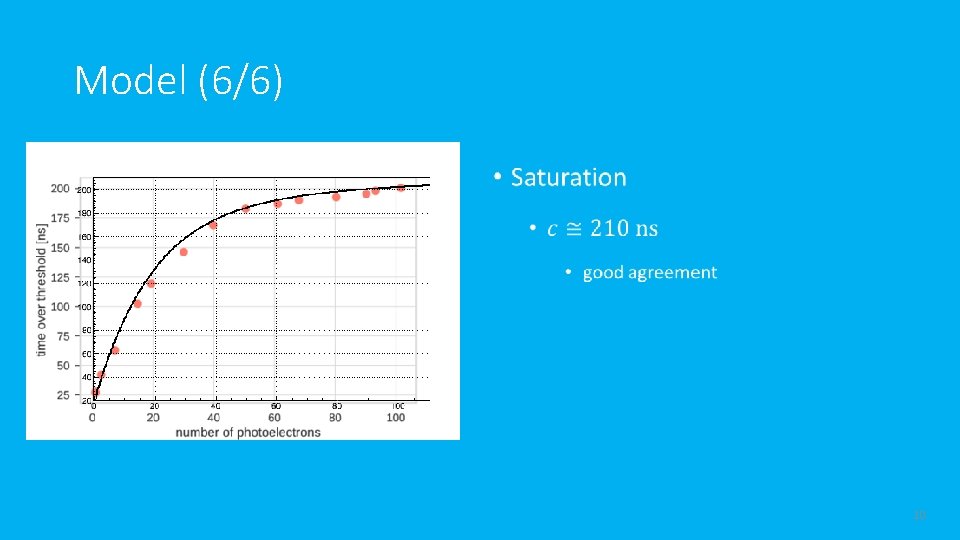
Model (6/6) • 10
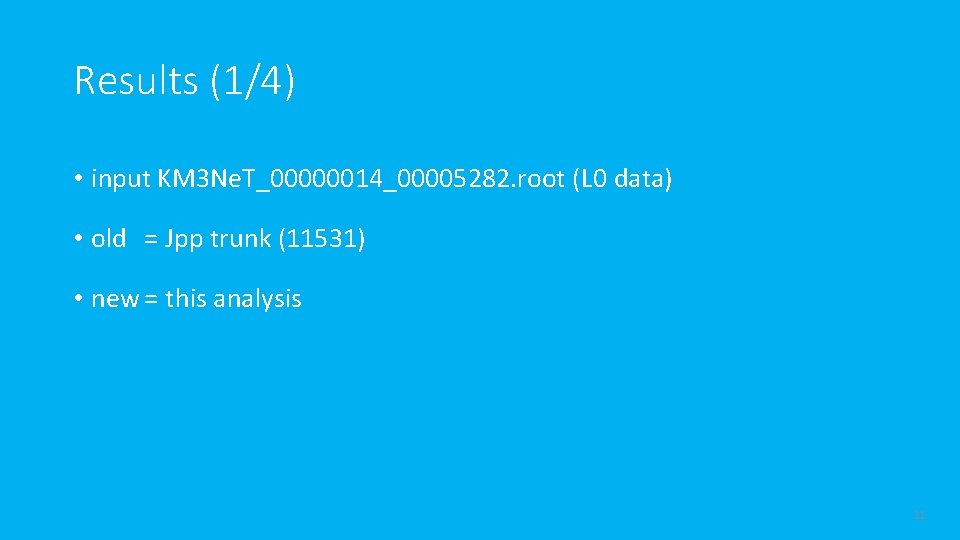
Results (1/4) • input KM 3 Ne. T_00000014_00005282. root (L 0 data) • old = Jpp trunk (11531) • new = this analysis 11
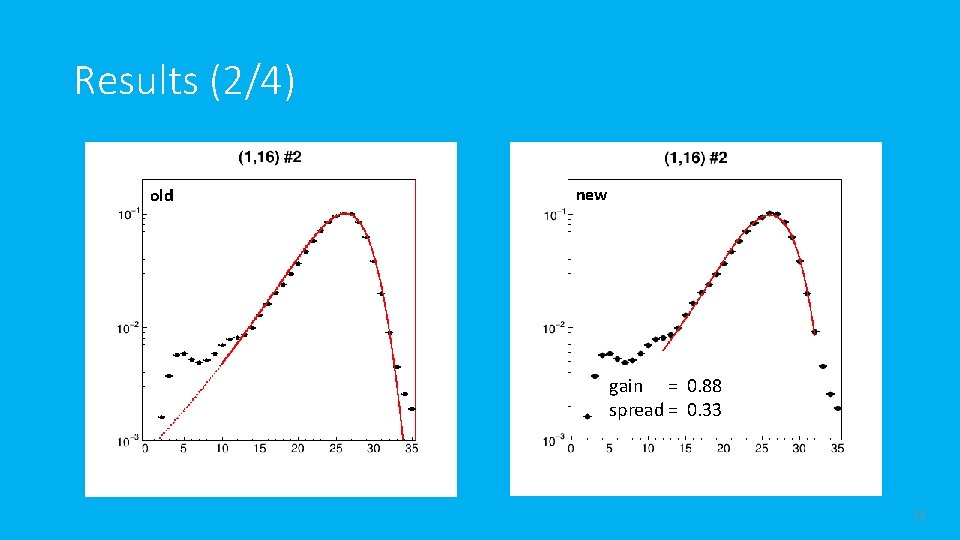
Results (2/4) old new gain = 0. 88 spread = 0. 33 12
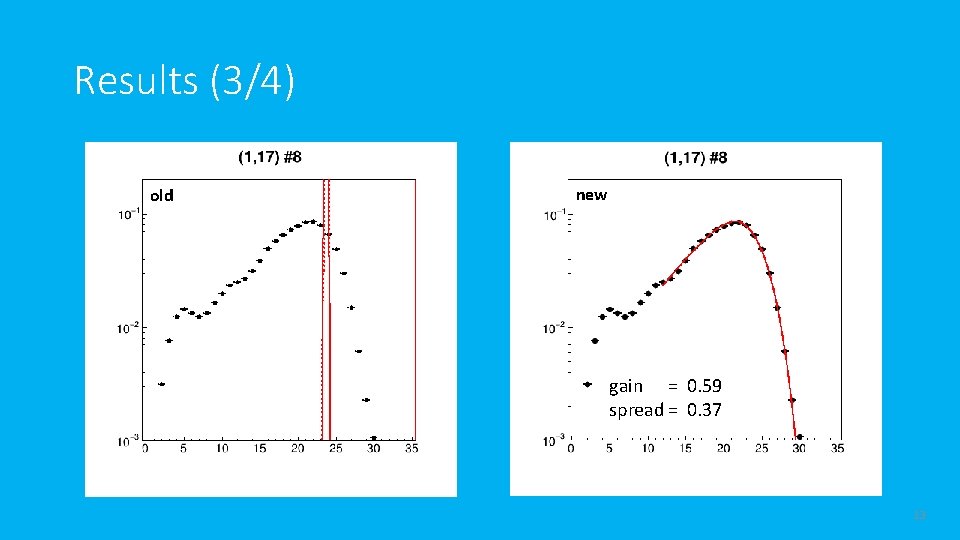
Results (3/4) old new gain = 0. 59 spread = 0. 37 13
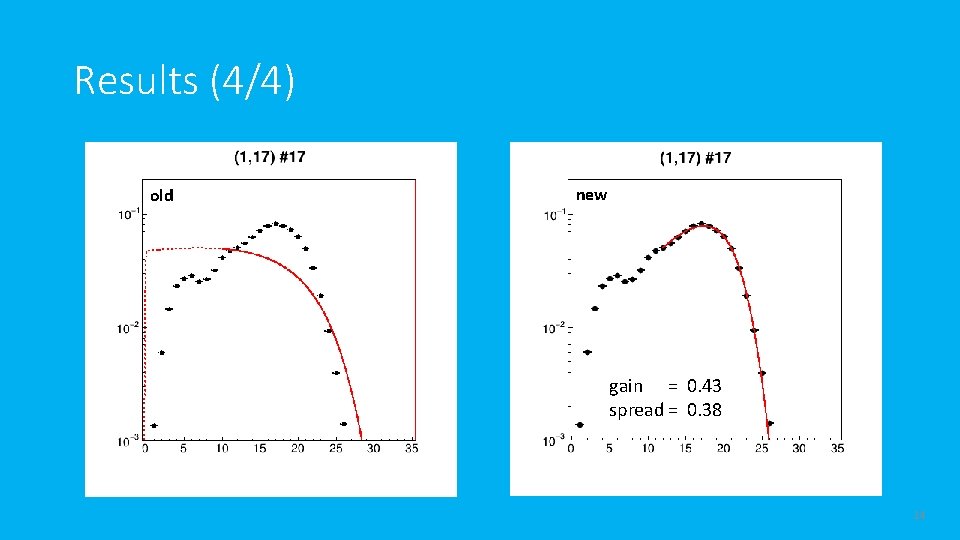
Results (4/4) old new gain = 0. 43 spread = 0. 38 14
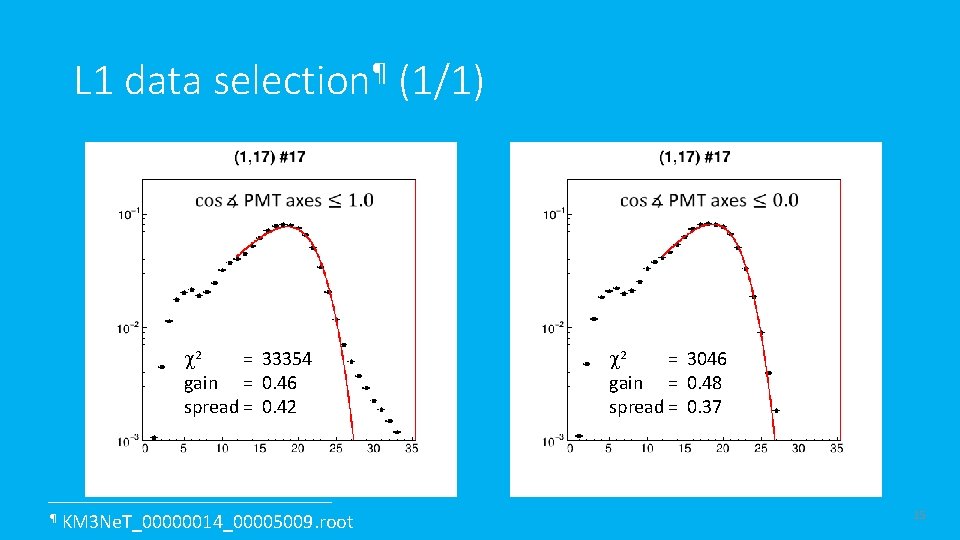
L 1 data selection¶ (1/1) c 2 = 33354 gain = 0. 46 spread = 0. 42 ¶ KM 3 Ne. T_00000014_00005009. root c 2 = 3046 gain = 0. 48 spread = 0. 37 15
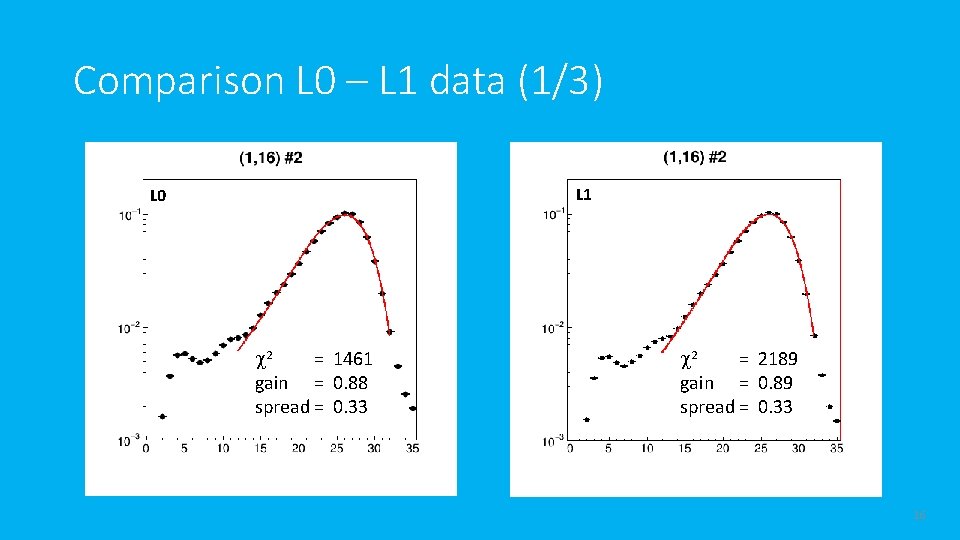
Comparison L 0 – L 1 data (1/3) L 1 L 0 c 2 = 1461 gain = 0. 88 spread = 0. 33 c 2 = 2189 gain = 0. 89 spread = 0. 33 16
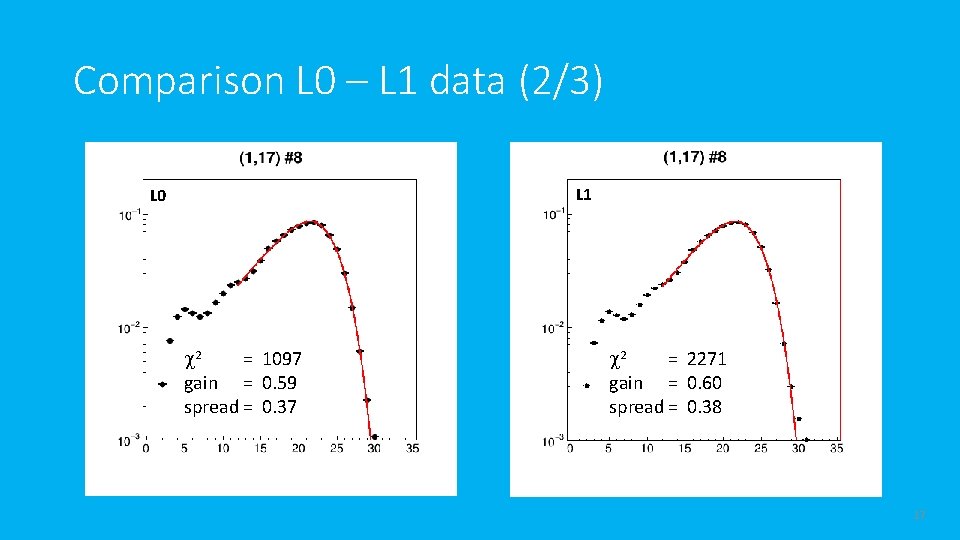
Comparison L 0 – L 1 data (2/3) L 1 L 0 c 2 = 1097 gain = 0. 59 spread = 0. 37 c 2 = 2271 gain = 0. 60 spread = 0. 38 17
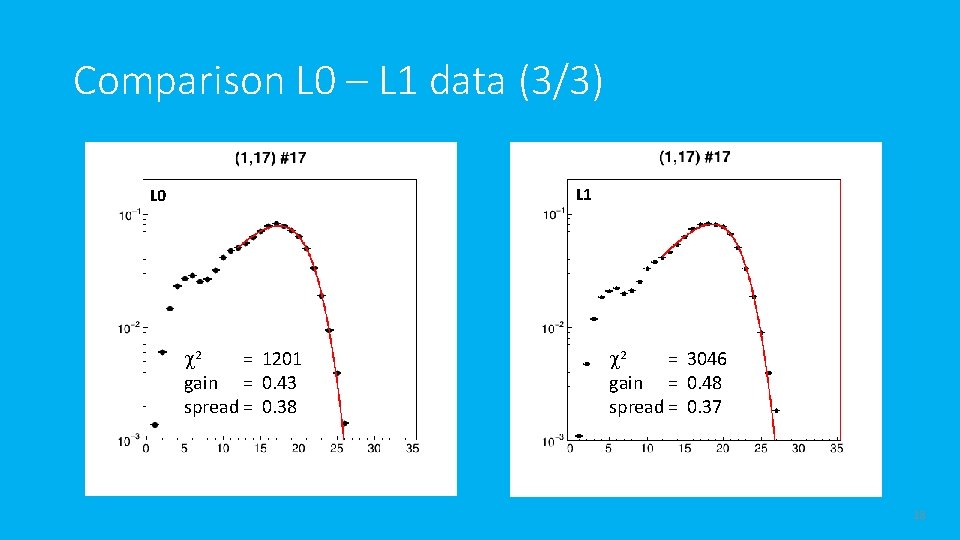
Comparison L 0 – L 1 data (3/3) L 1 L 0 c 2 = 1201 gain = 0. 43 spread = 0. 38 c 2 = 3046 gain = 0. 48 spread = 0. 37 18
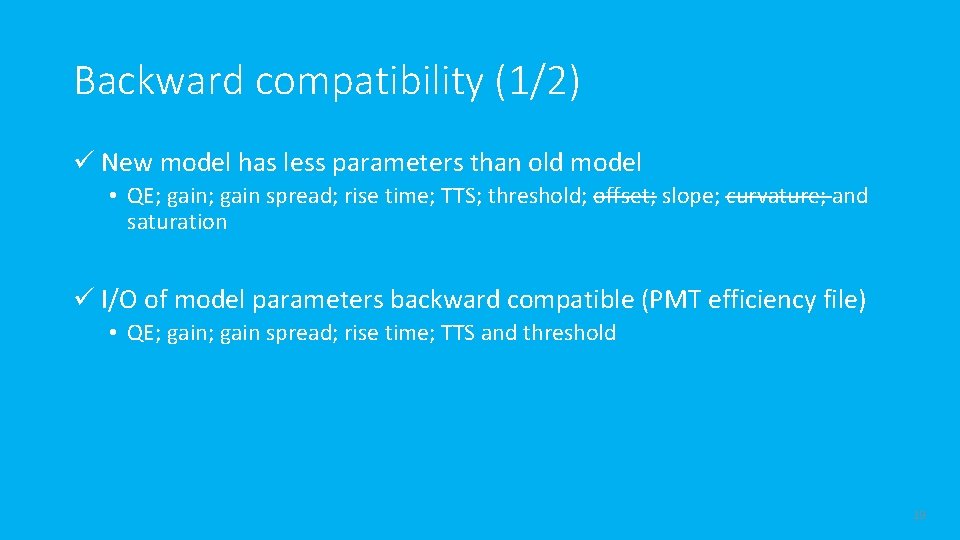
Backward compatibility (1/2) ü New model has less parameters than old model • QE; gain spread; rise time; TTS; threshold; offset; slope; curvature; and saturation ü I/O of model parameters backward compatible (PMT efficiency file) • QE; gain spread; rise time; TTS and threshold 19
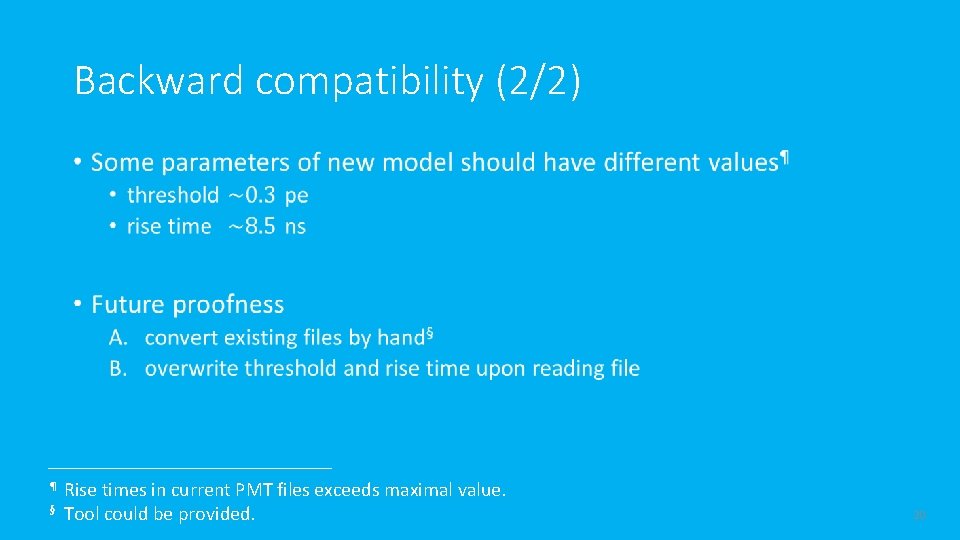
Backward compatibility (2/2) • ¶ § Rise times in current PMT files exceeds maximal value. Tool could be provided. 20
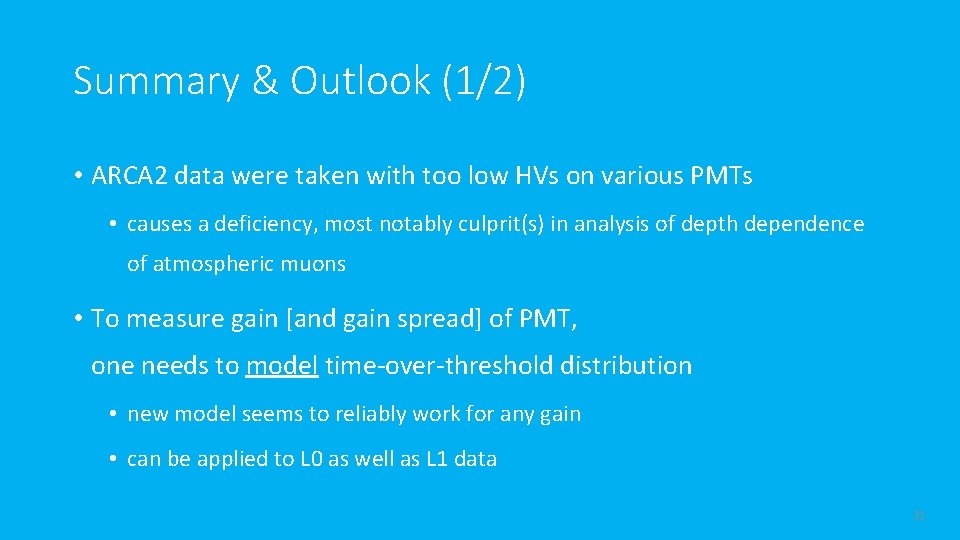
Summary & Outlook (1/2) • ARCA 2 data were taken with too low HVs on various PMTs • causes a deficiency, most notably culprit(s) in analysis of depth dependence of atmospheric muons • To measure gain [and gain spread] of PMT, one needs to model time-over-threshold distribution • new model seems to reliably work for any gain • can be applied to L 0 as well as L 1 data 21
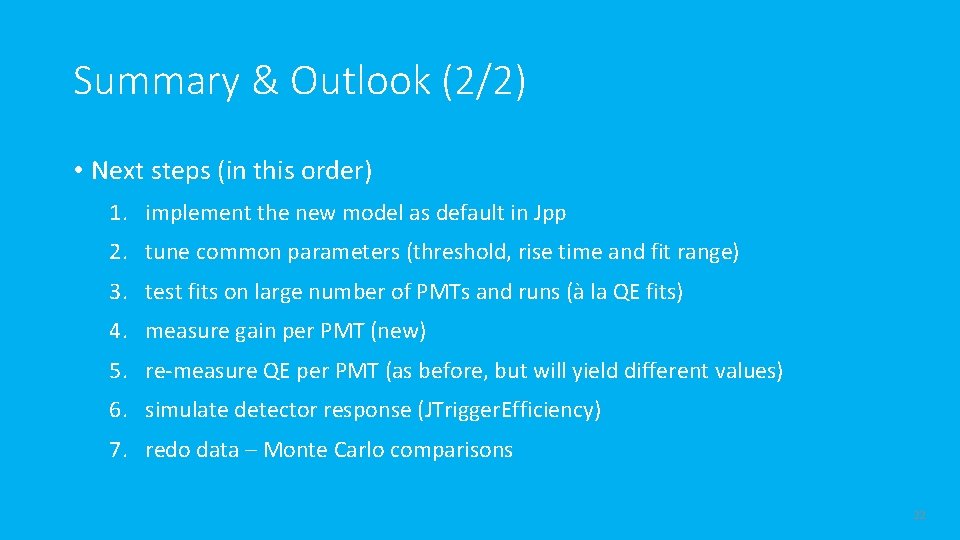
Summary & Outlook (2/2) • Next steps (in this order) 1. implement the new model as default in Jpp 2. tune common parameters (threshold, rise time and fit range) 3. test fits on large number of PMTs and runs (à la QE fits) 4. measure gain per PMT (new) 5. re-measure QE per PMT (as before, but will yield different values) 6. simulate detector response (JTrigger. Efficiency) 7. redo data – Monte Carlo comparisons 22
Analogue studies are used when researchers ____.
Torque is rotational analogue of
Status digital analogue
Analog vs digital
Sadq h10
Analog and digital signals in computer networking
Analogue and digital electronics
Analogue chapter 3
Types of adcs
Analogue door phone
Analogue and digital images
Writing time in analogue form
Risc
Digital signal processor
Dsp algorithms and architecture notes
Pmt posyandu
Pmt calibration
Suntrust olb mtg pmt
Most maynard operation sequence technique
Cr cd pmt
Pmt
Sk pmt
Pmt protection plan primary life/dis/iu