HSRP 734 Advanced Statistical Methods July 17 2008
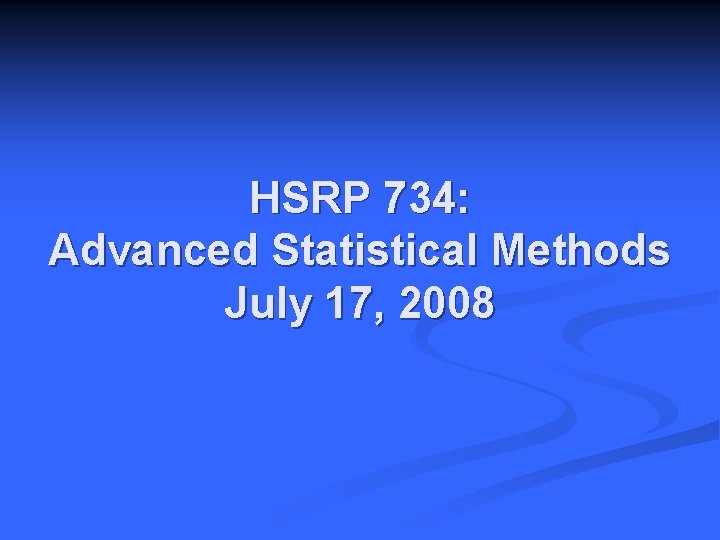
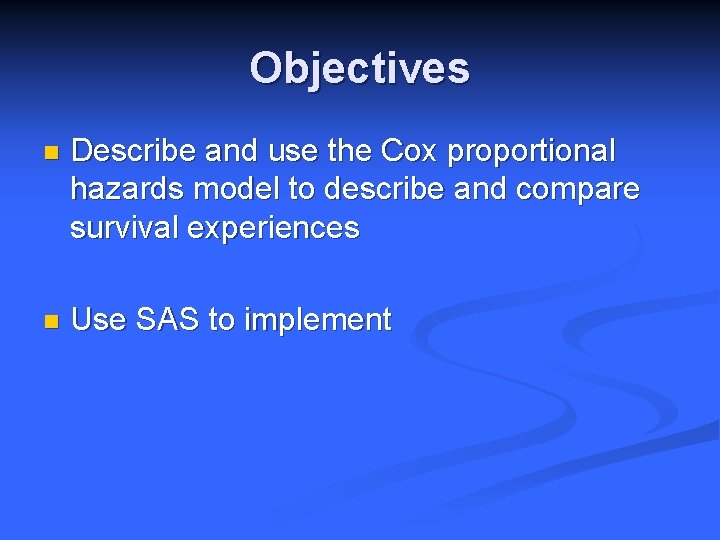
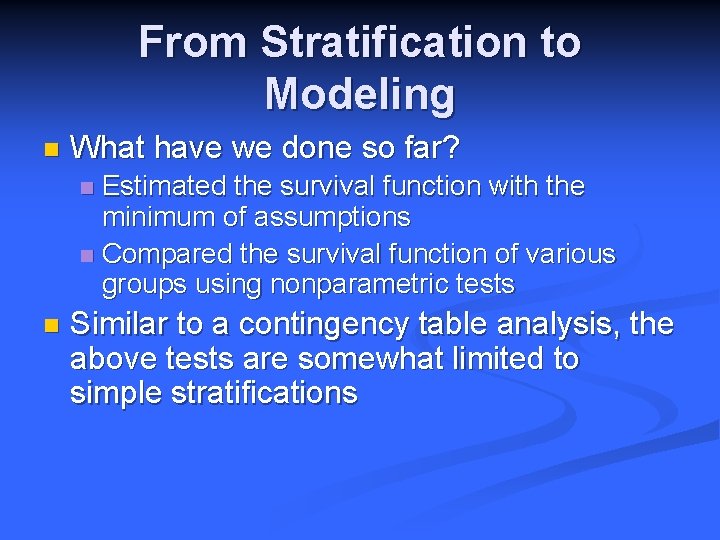
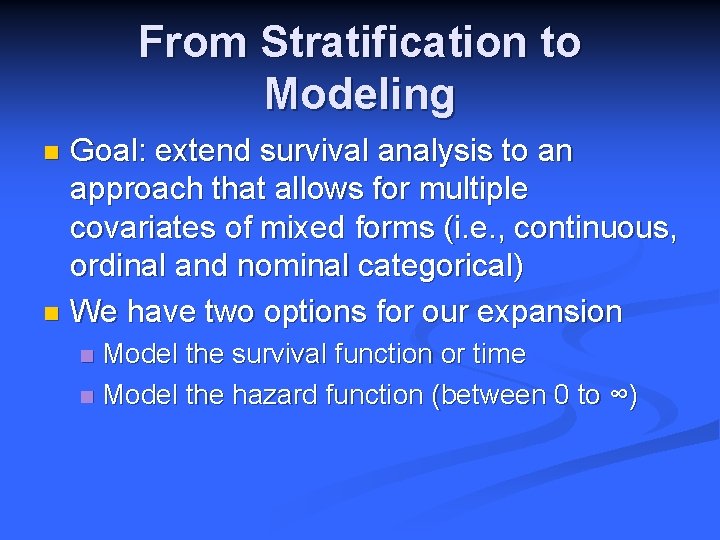
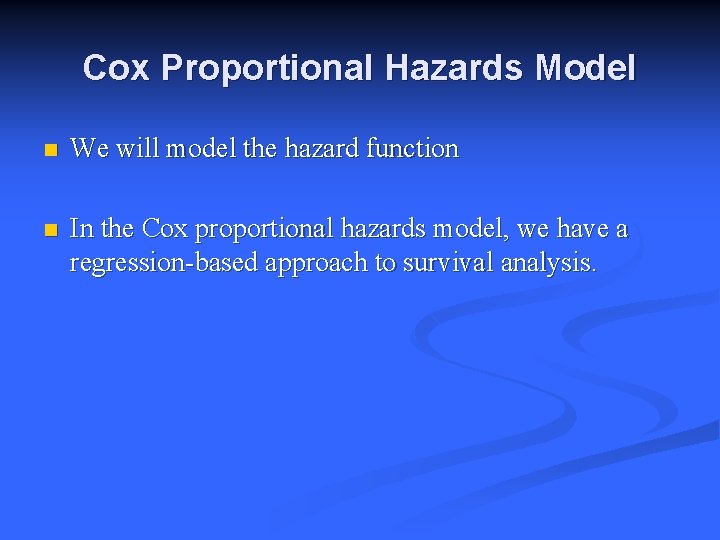
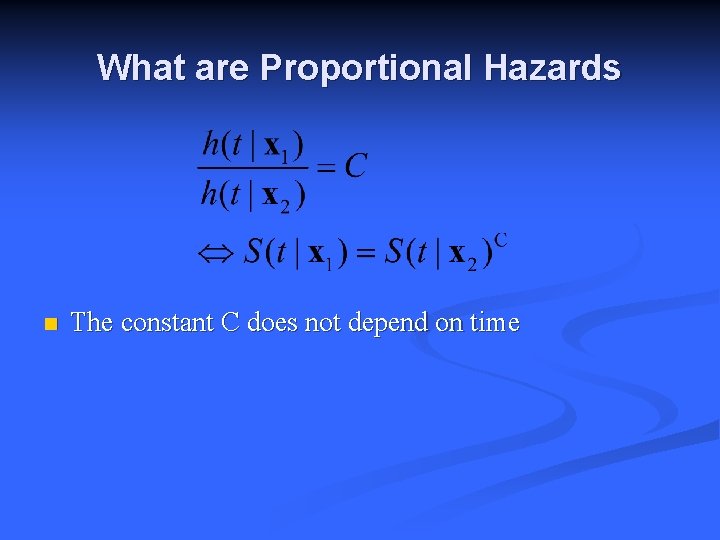
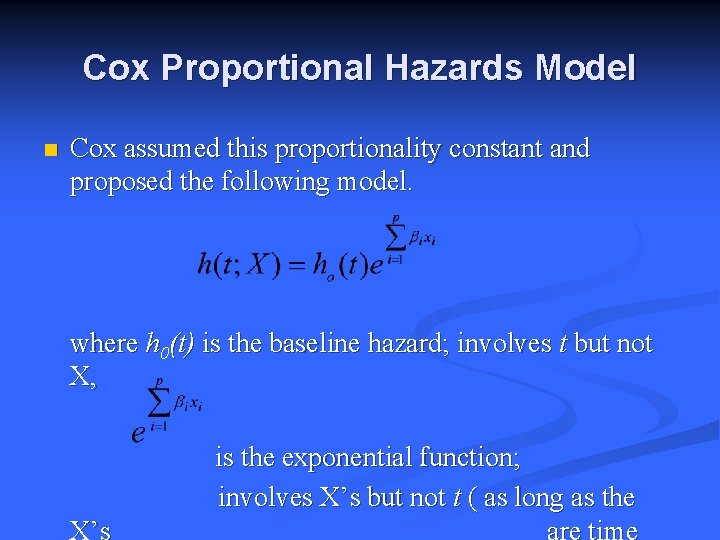
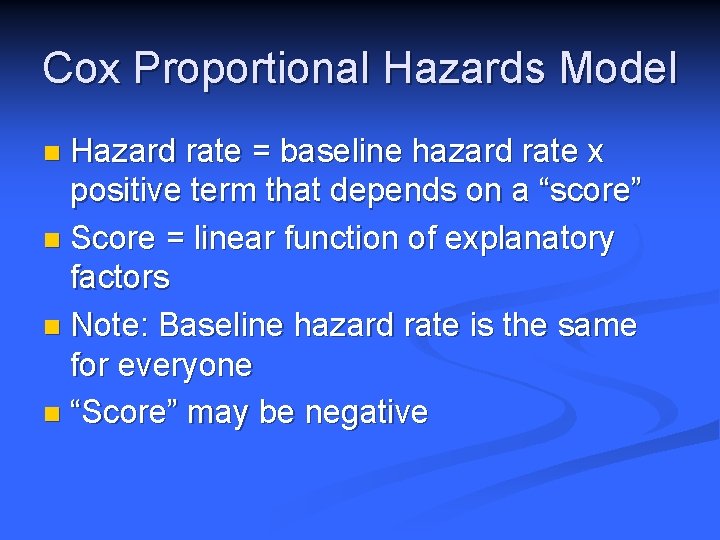
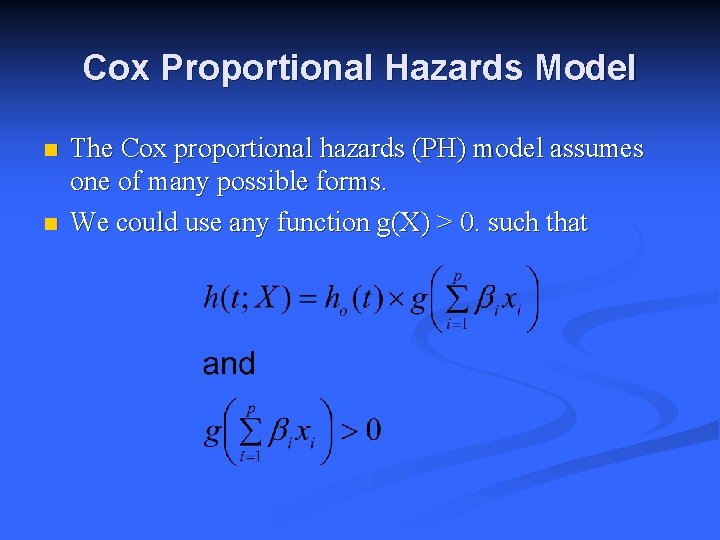
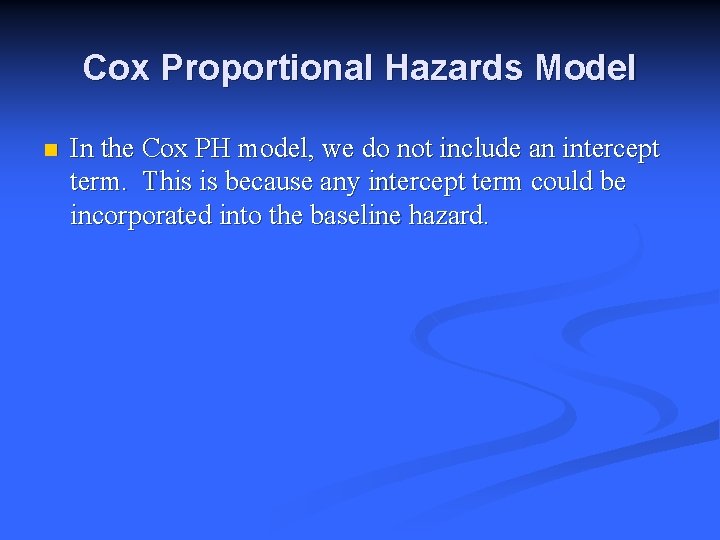
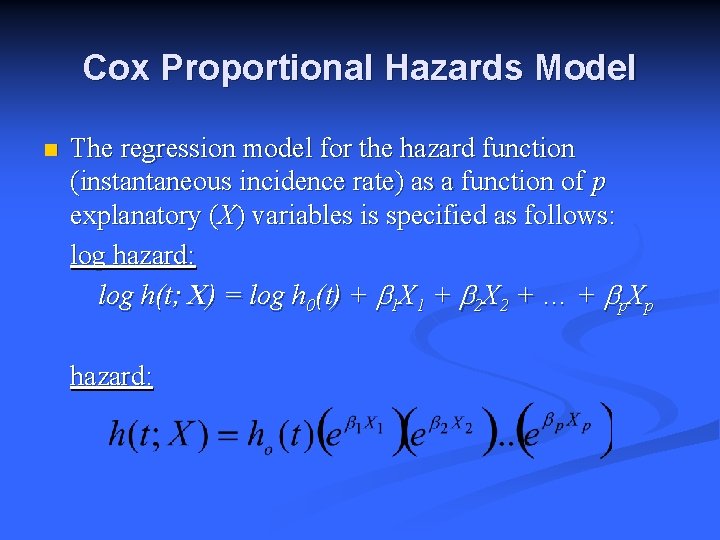
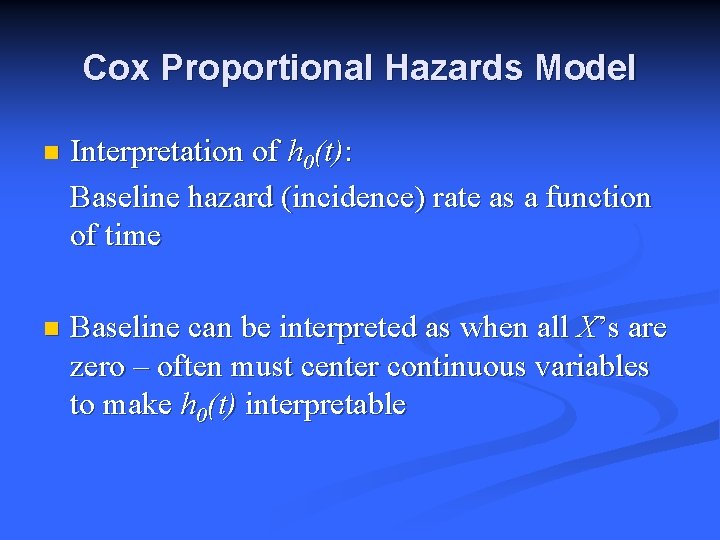
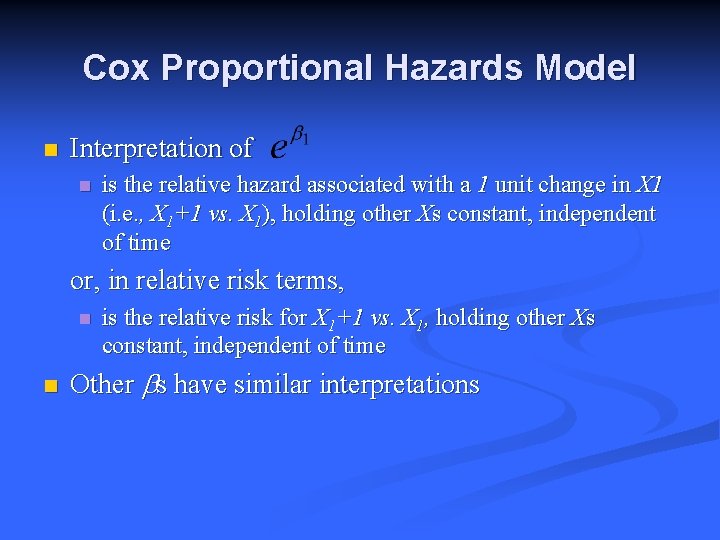
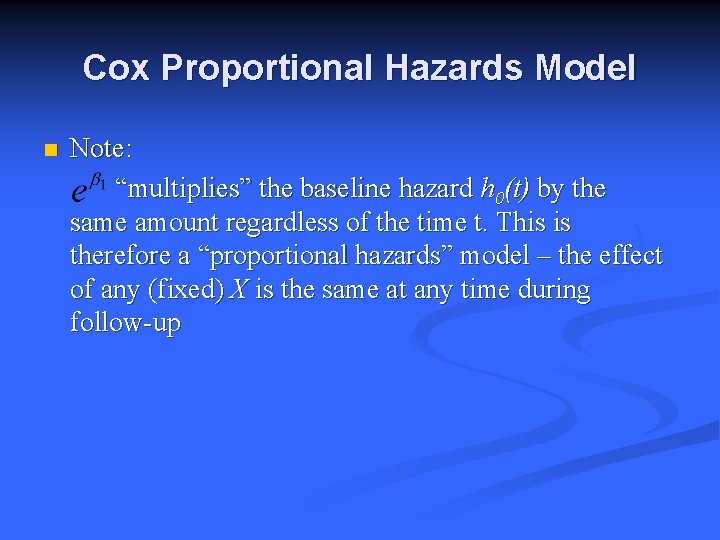
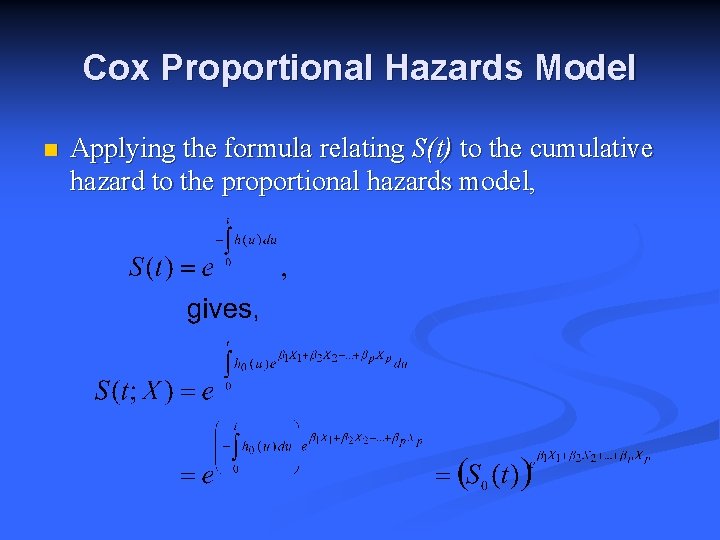
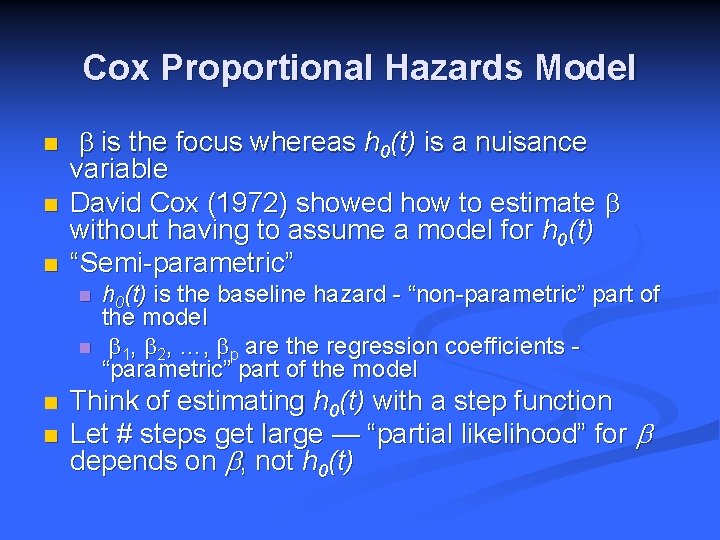
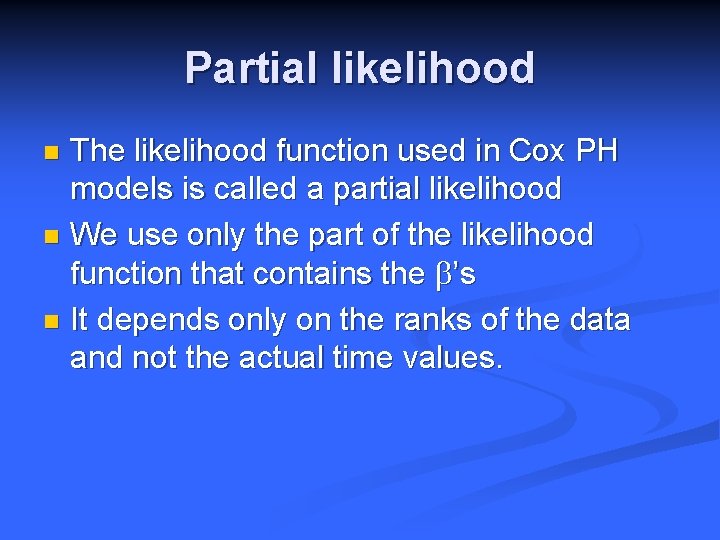
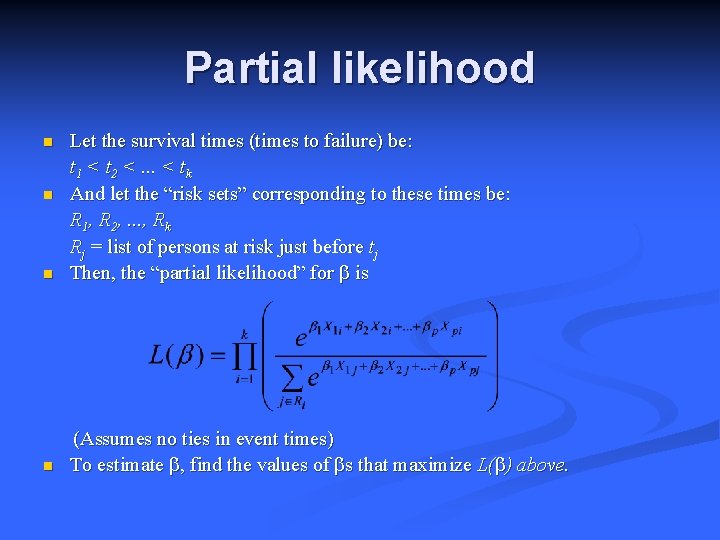
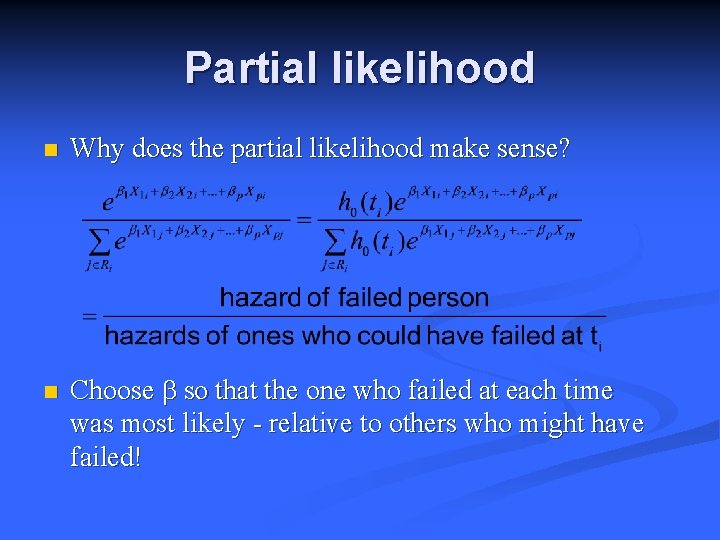
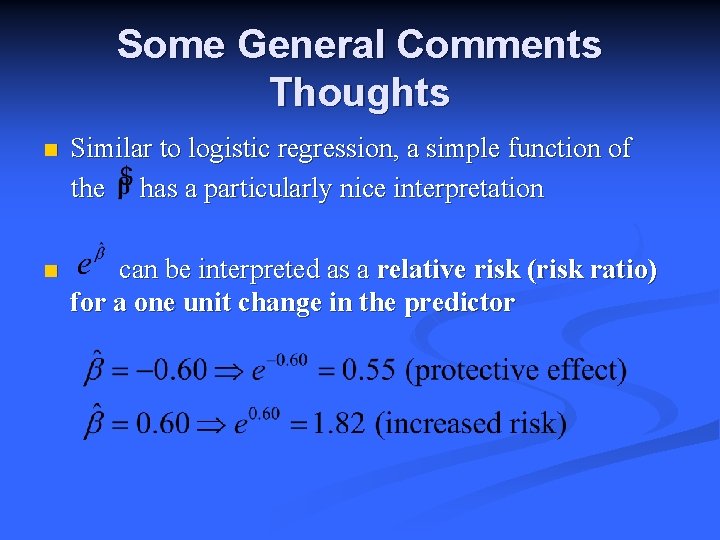
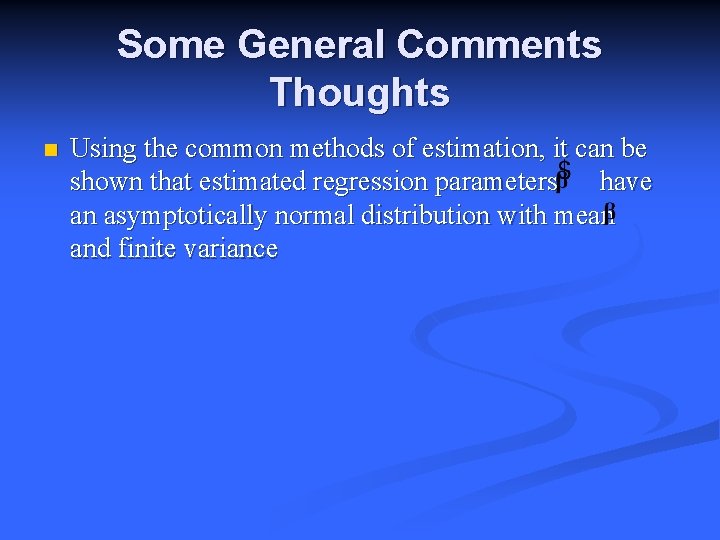
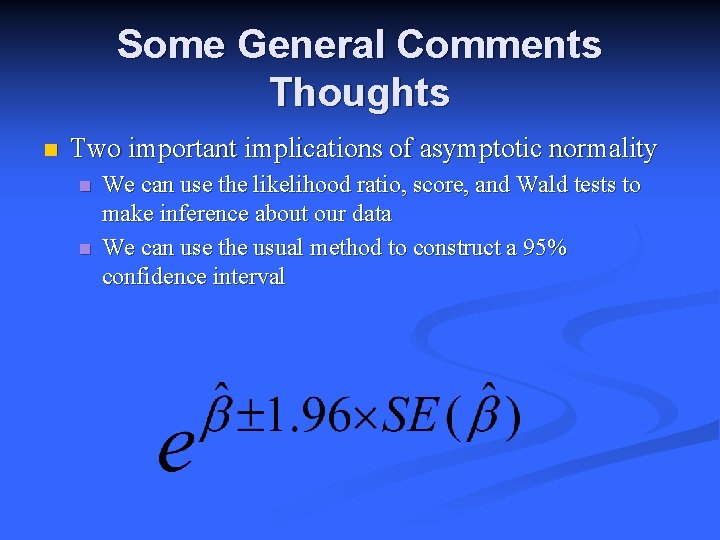
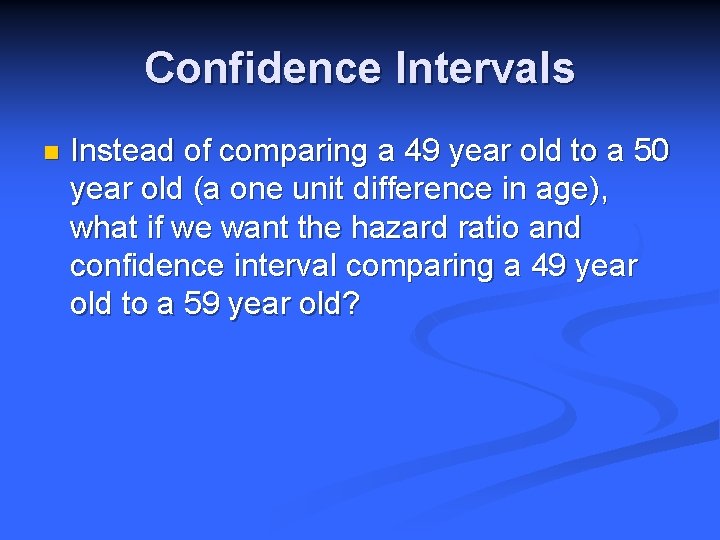
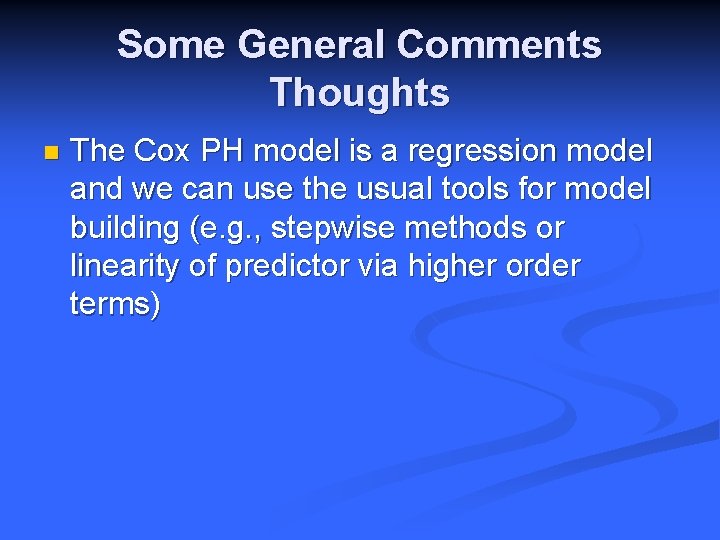
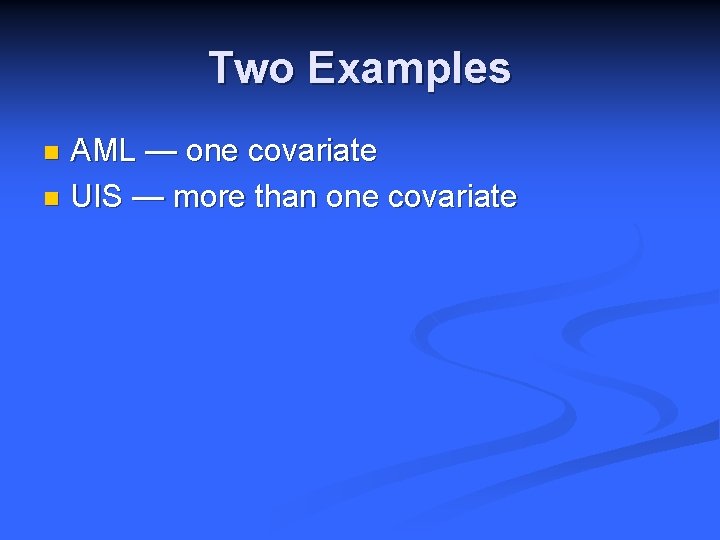
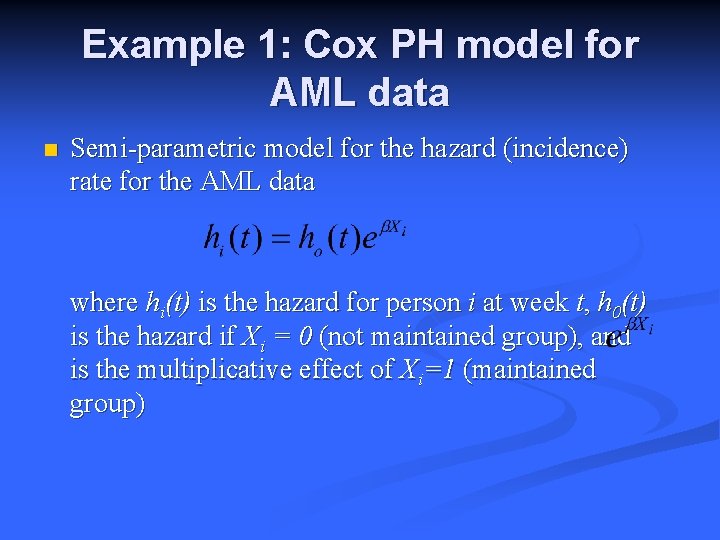
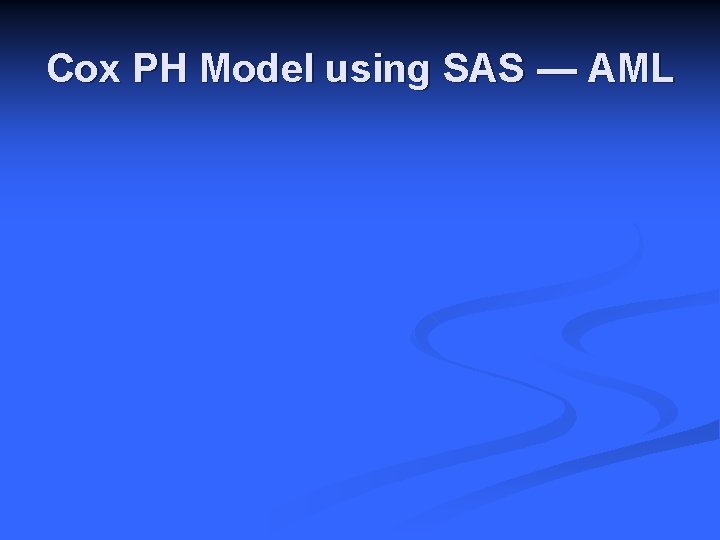
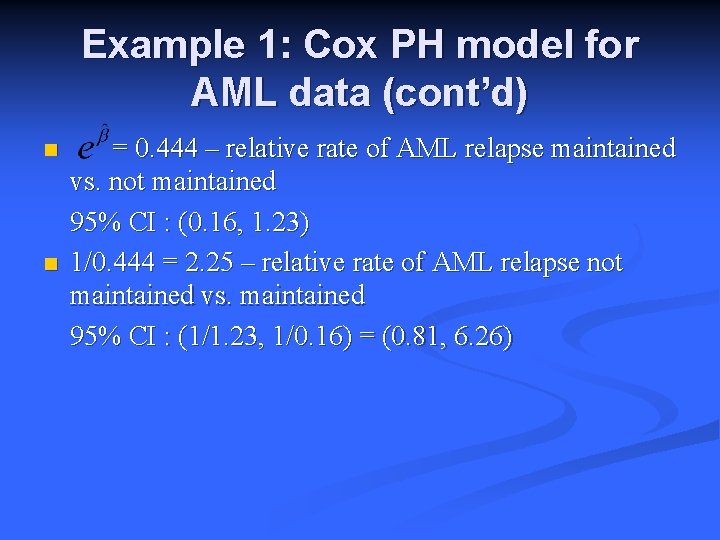
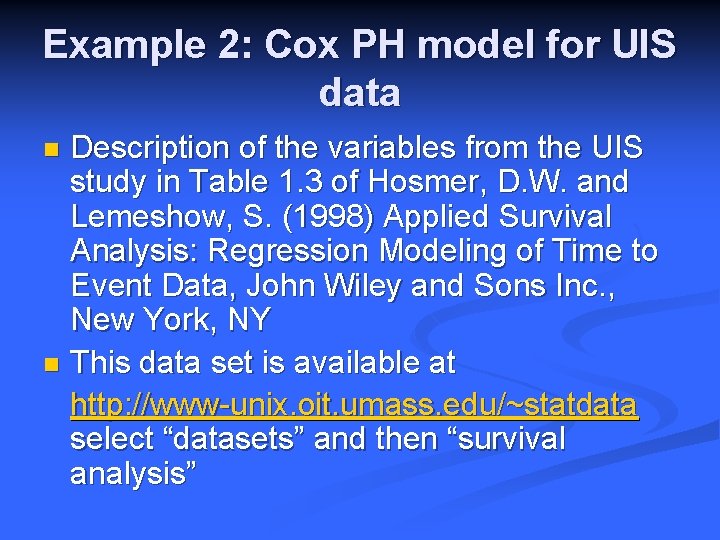
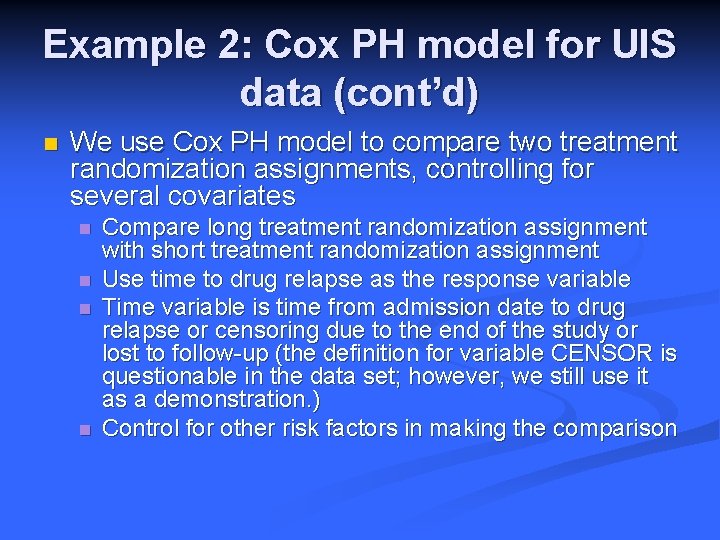
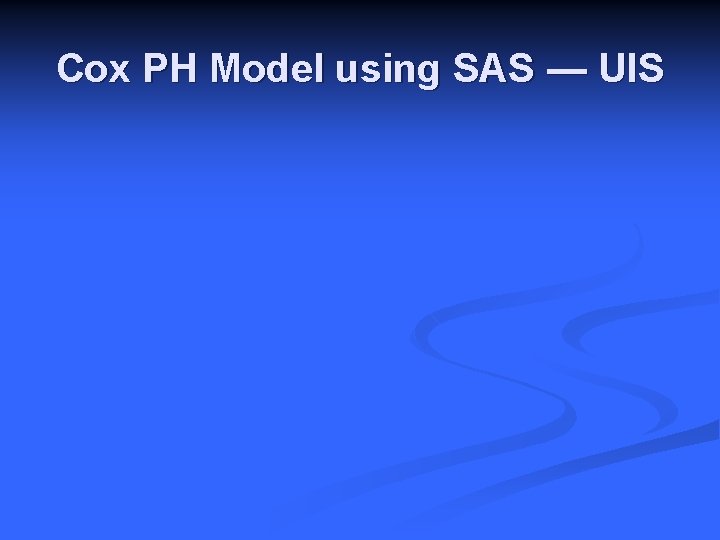
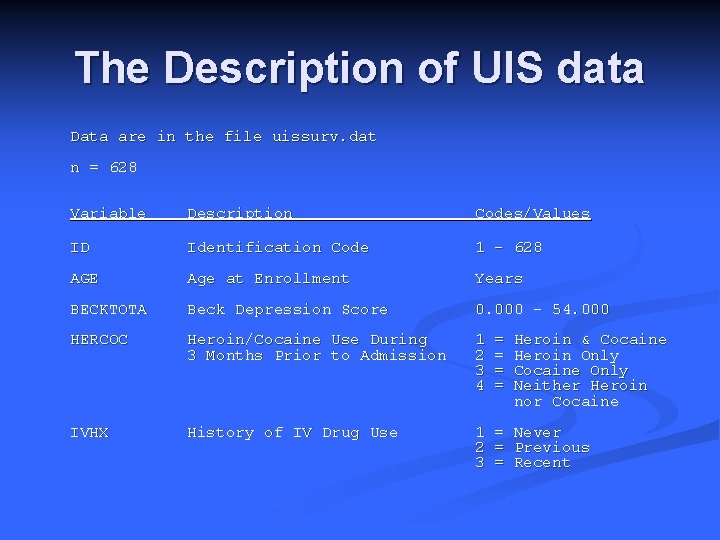
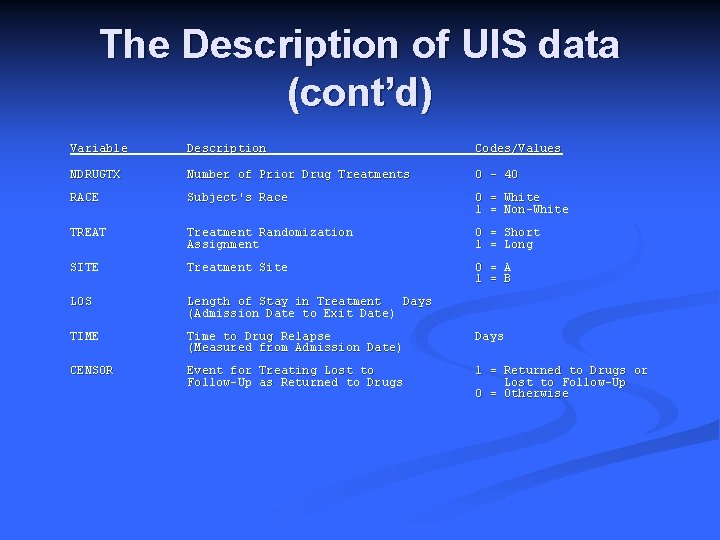
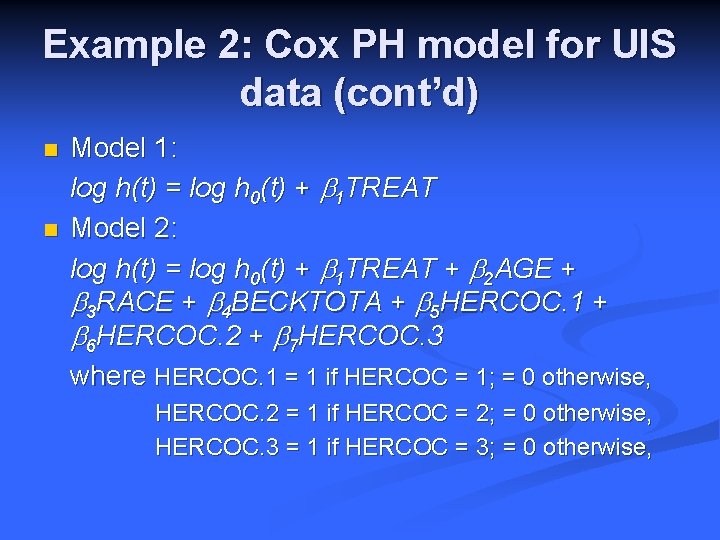
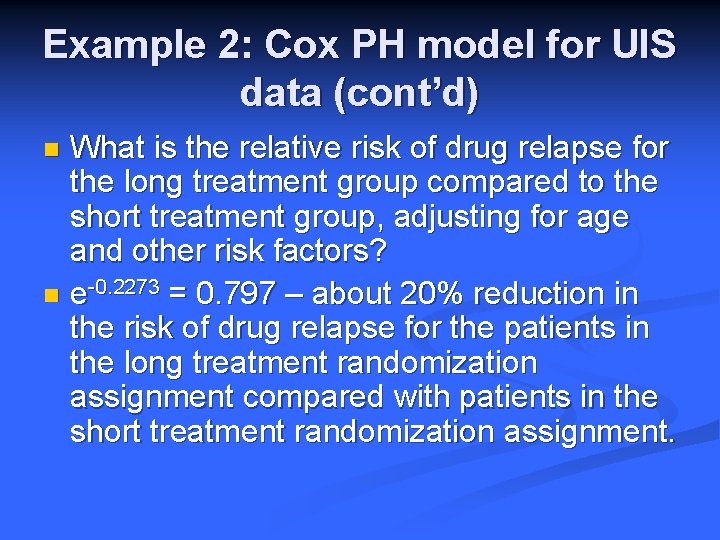
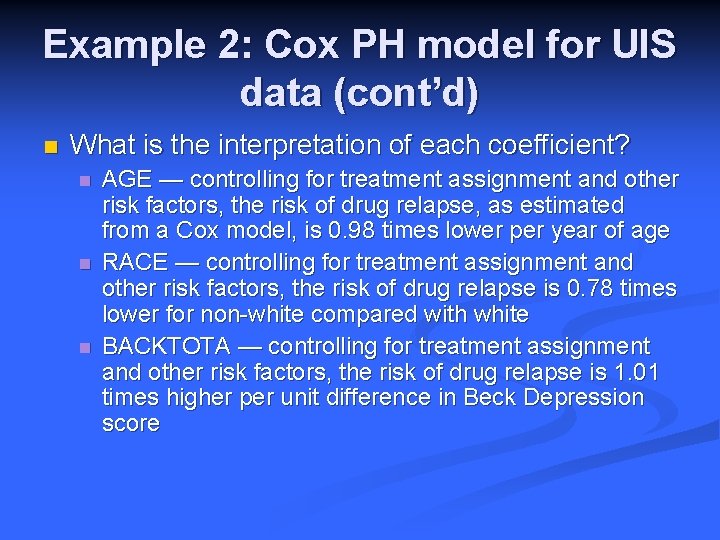
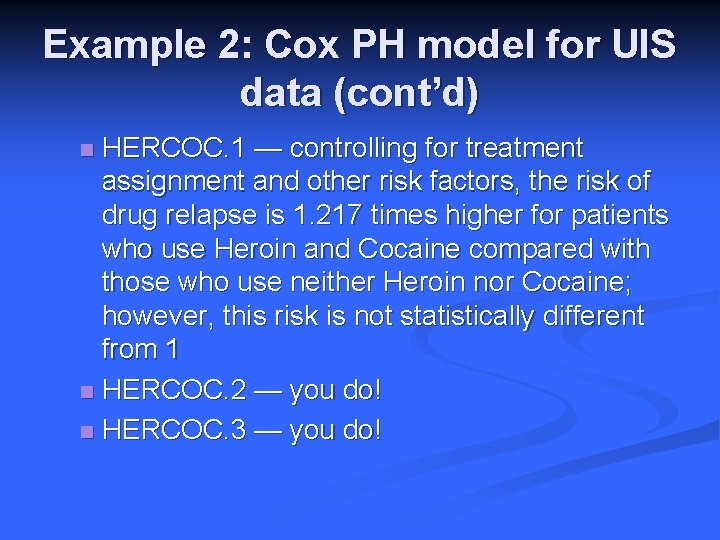
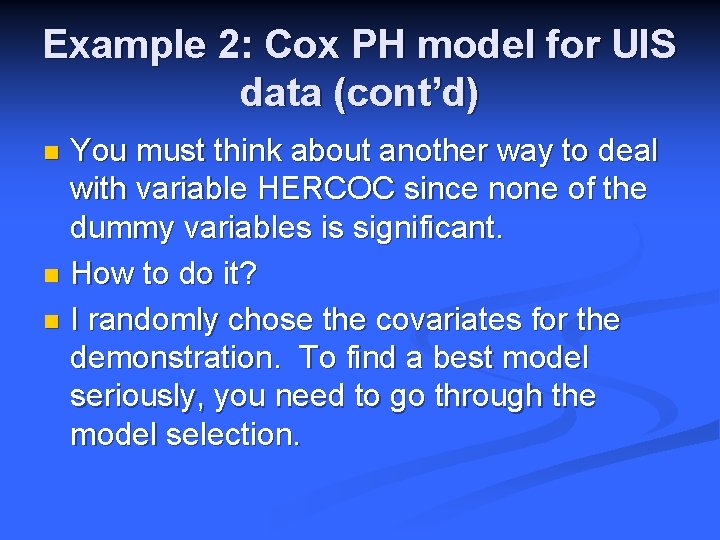
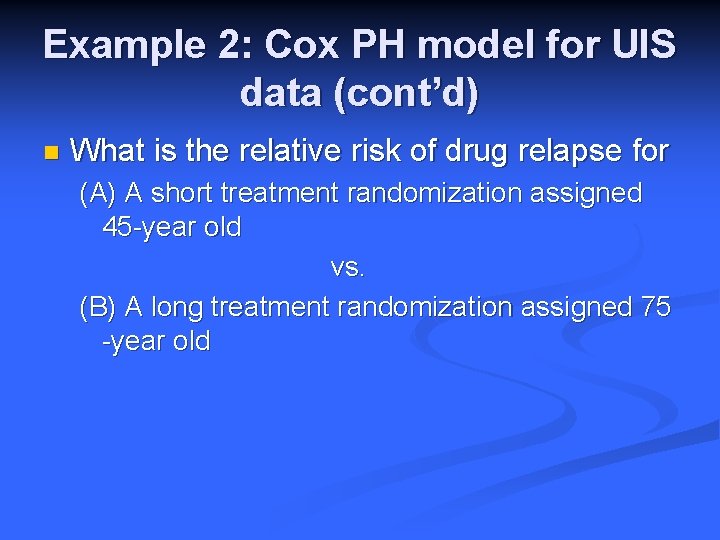
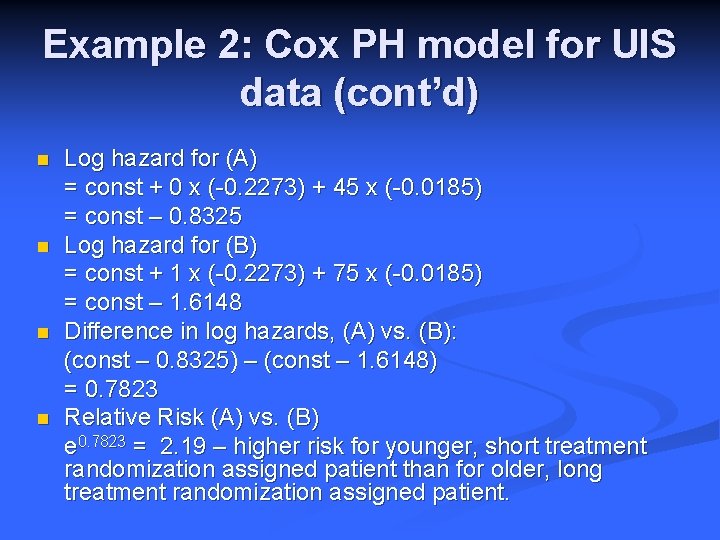
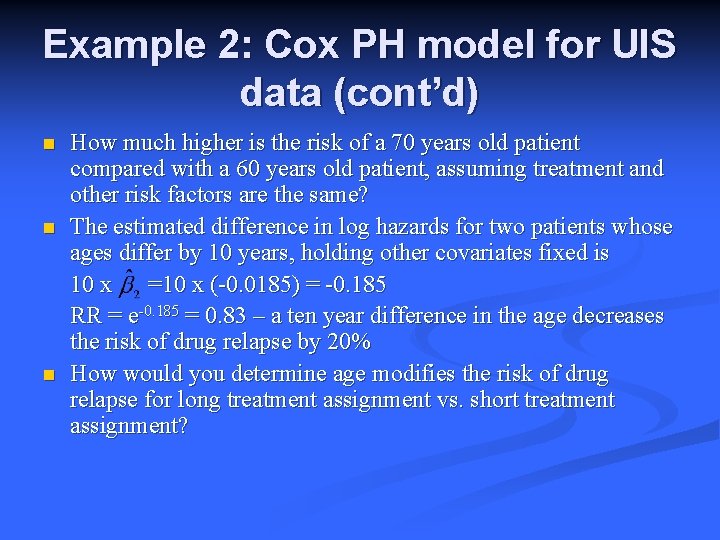
- Slides: 41
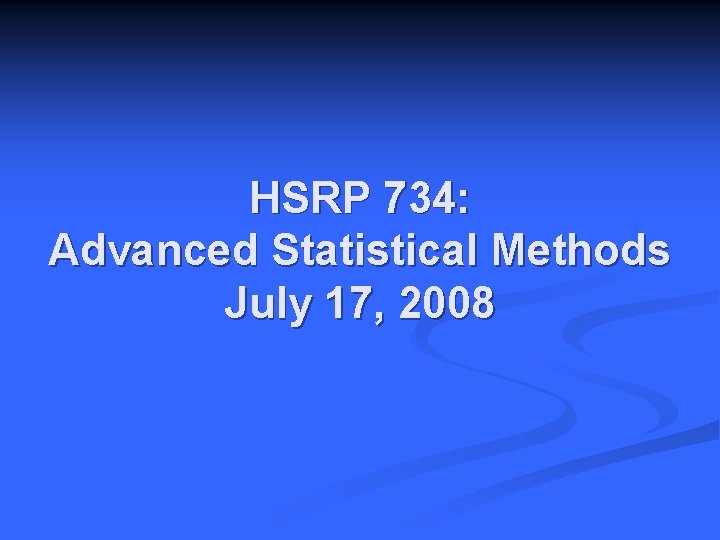
HSRP 734: Advanced Statistical Methods July 17, 2008
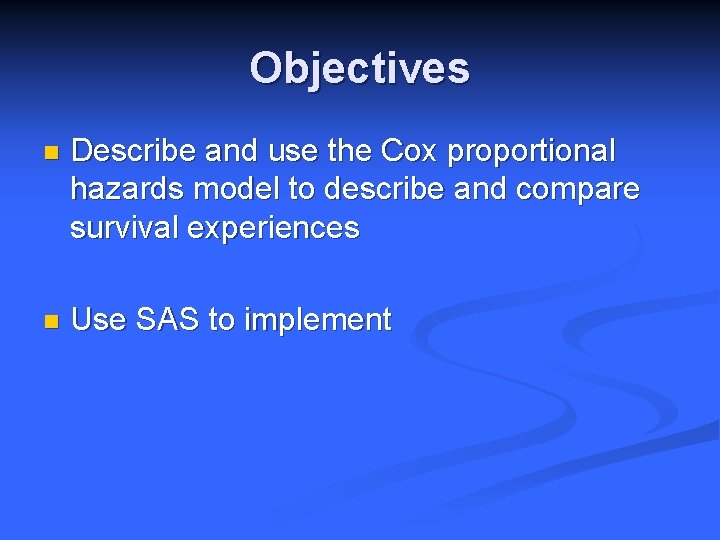
Objectives n Describe and use the Cox proportional hazards model to describe and compare survival experiences n Use SAS to implement
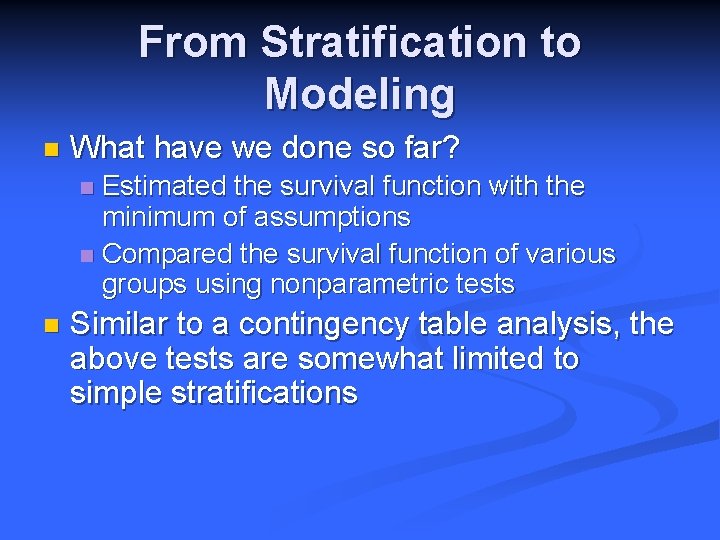
From Stratification to Modeling n What have we done so far? Estimated the survival function with the minimum of assumptions n Compared the survival function of various groups using nonparametric tests n n Similar to a contingency table analysis, the above tests are somewhat limited to simple stratifications
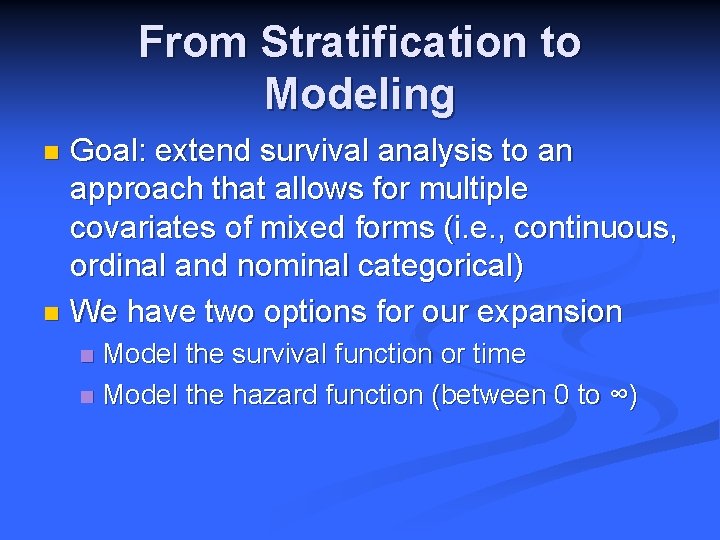
From Stratification to Modeling Goal: extend survival analysis to an approach that allows for multiple covariates of mixed forms (i. e. , continuous, ordinal and nominal categorical) n We have two options for our expansion n Model the survival function or time n Model the hazard function (between 0 to ∞) n
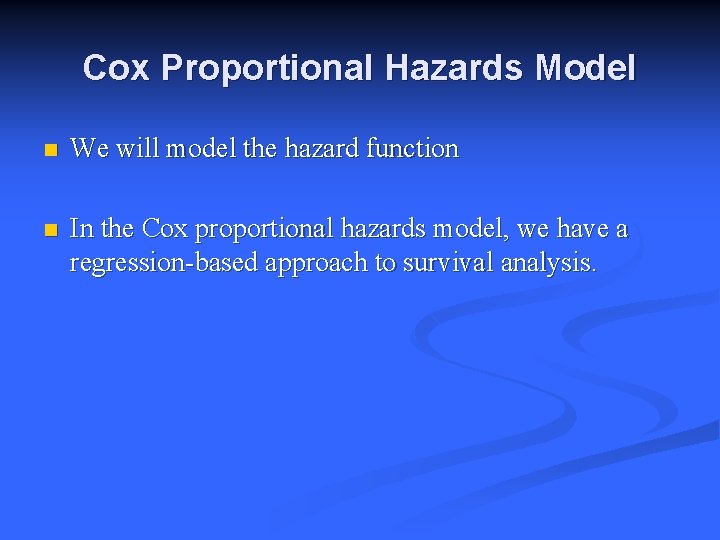
Cox Proportional Hazards Model n We will model the hazard function n In the Cox proportional hazards model, we have a regression-based approach to survival analysis.
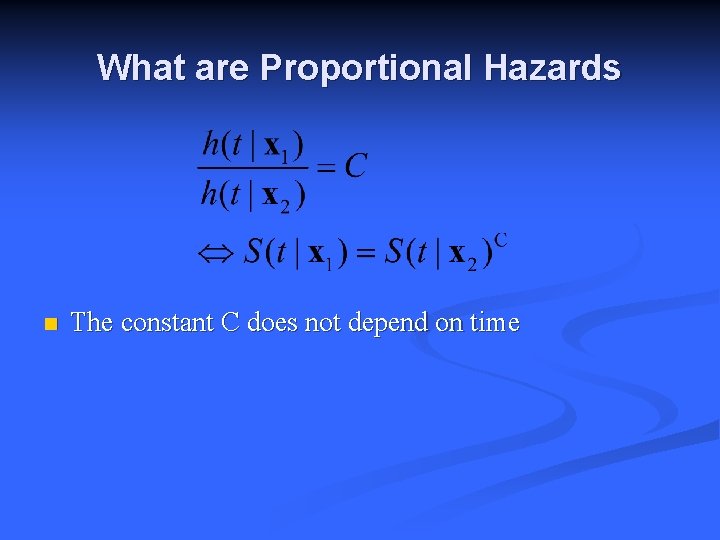
What are Proportional Hazards n The constant C does not depend on time
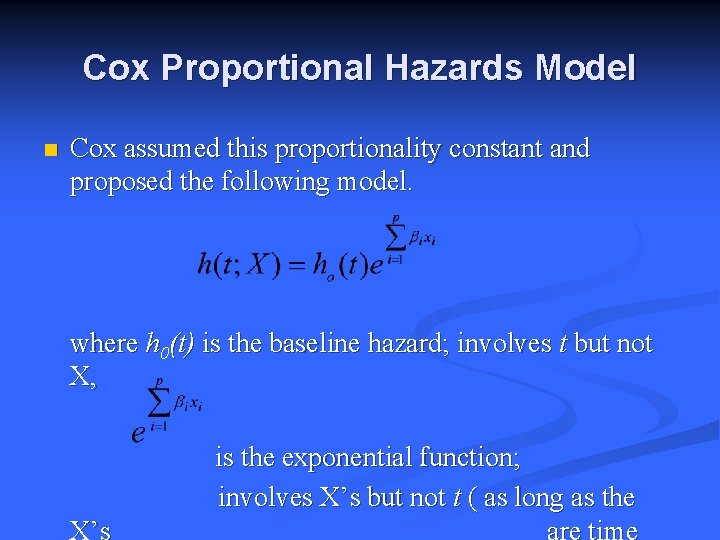
Cox Proportional Hazards Model n Cox assumed this proportionality constant and proposed the following model. where h 0(t) is the baseline hazard; involves t but not X, X’s is the exponential function; involves X’s but not t ( as long as the are time
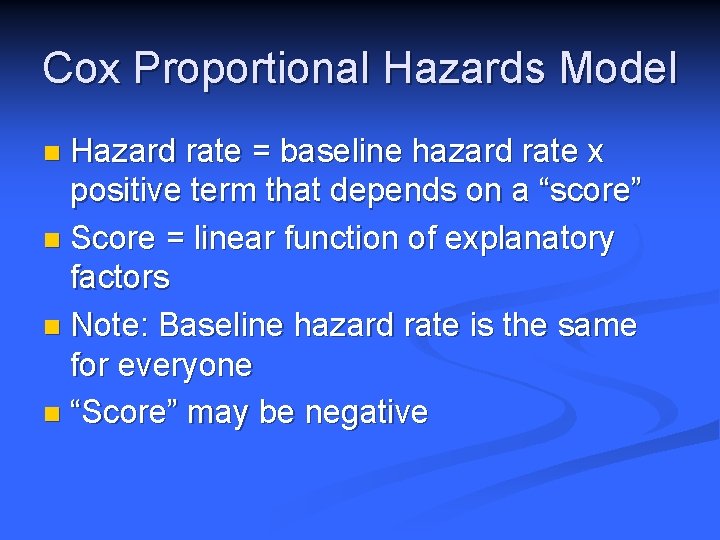
Cox Proportional Hazards Model Hazard rate = baseline hazard rate x positive term that depends on a “score” n Score = linear function of explanatory factors n Note: Baseline hazard rate is the same for everyone n “Score” may be negative n
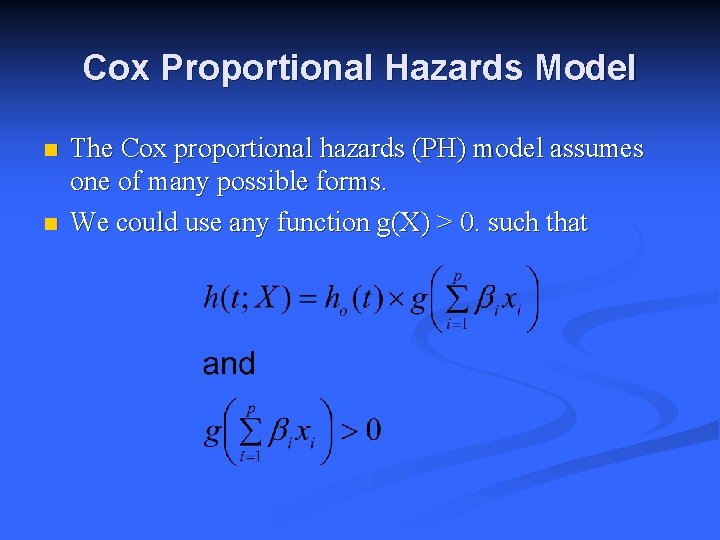
Cox Proportional Hazards Model n n The Cox proportional hazards (PH) model assumes one of many possible forms. We could use any function g(X) > 0. such that
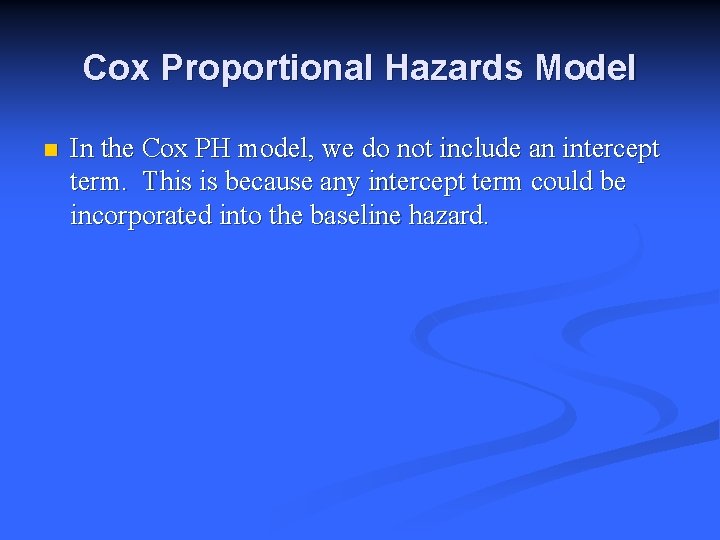
Cox Proportional Hazards Model n In the Cox PH model, we do not include an intercept term. This is because any intercept term could be incorporated into the baseline hazard.
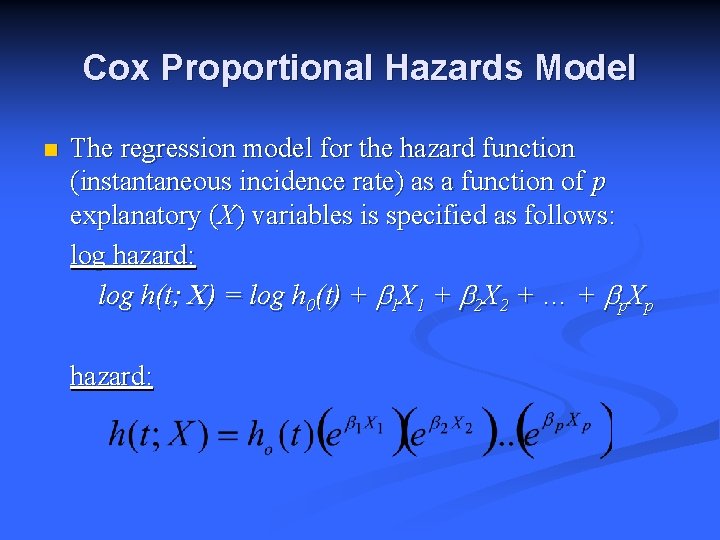
Cox Proportional Hazards Model n The regression model for the hazard function (instantaneous incidence rate) as a function of p explanatory (X) variables is specified as follows: log hazard: log h(t; X) = log h 0(t) + b 1 X 1 + b 2 X 2 + … + bp. Xp hazard:
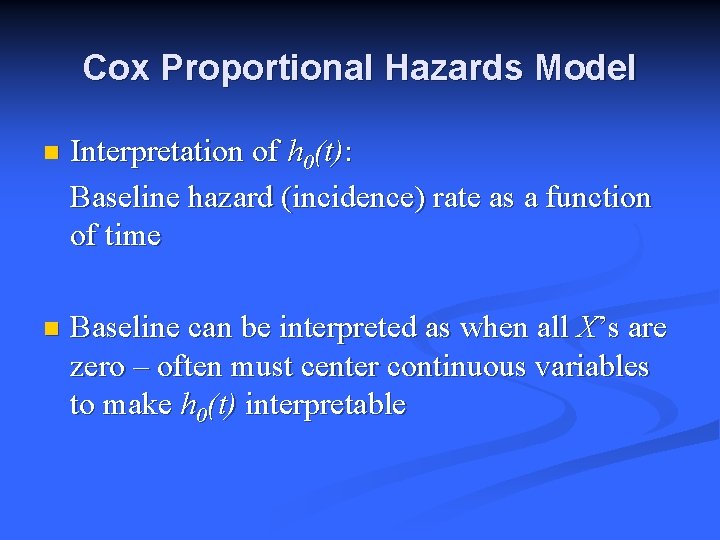
Cox Proportional Hazards Model n Interpretation of h 0(t): Baseline hazard (incidence) rate as a function of time n Baseline can be interpreted as when all X’s are zero – often must center continuous variables to make h 0(t) interpretable
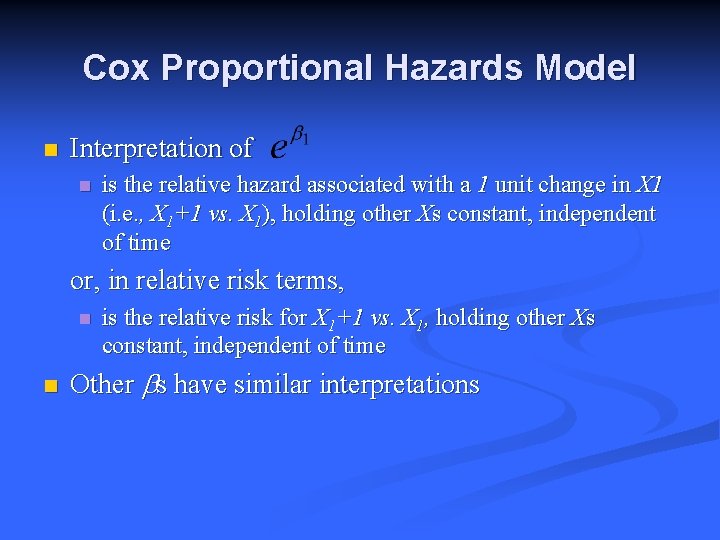
Cox Proportional Hazards Model n Interpretation of n is the relative hazard associated with a 1 unit change in X 1 (i. e. , X 1+1 vs. X 1), holding other Xs constant, independent of time or, in relative risk terms, n n is the relative risk for X 1+1 vs. X 1, holding other Xs constant, independent of time Other bs have similar interpretations
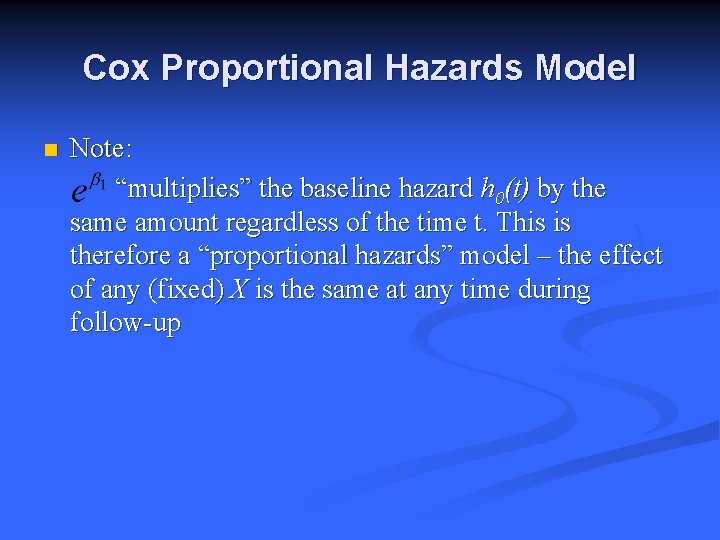
Cox Proportional Hazards Model n Note: “multiplies” the baseline hazard h 0(t) by the same amount regardless of the time t. This is therefore a “proportional hazards” model – the effect of any (fixed) X is the same at any time during follow-up
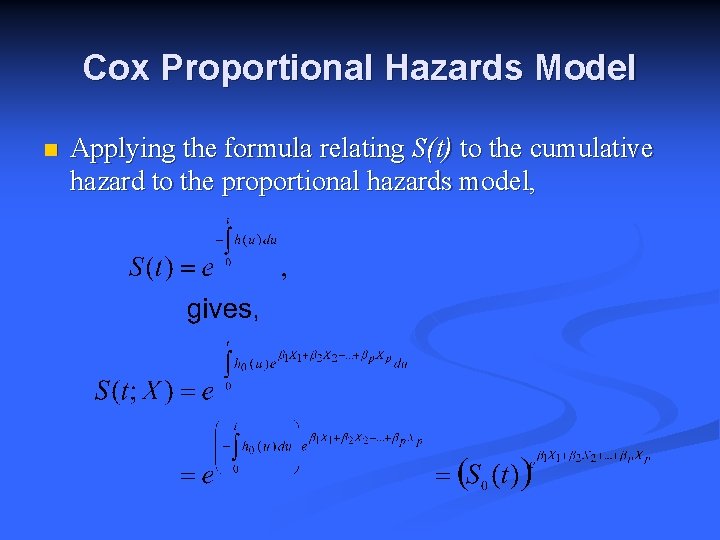
Cox Proportional Hazards Model n Applying the formula relating S(t) to the cumulative hazard to the proportional hazards model,
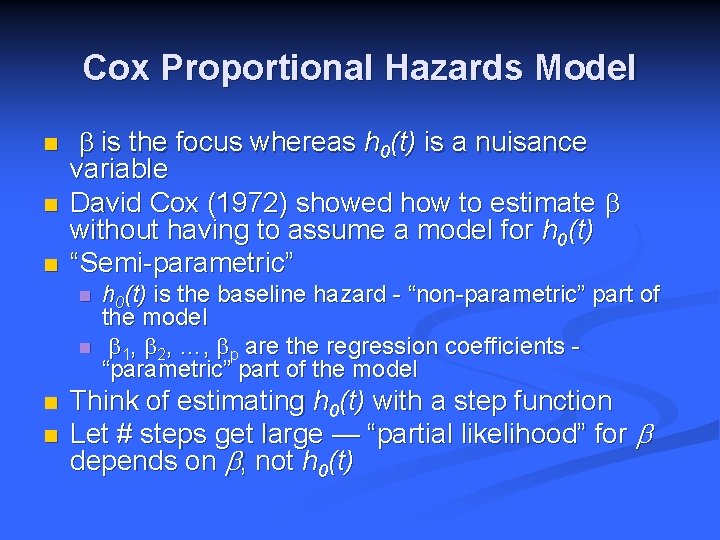
Cox Proportional Hazards Model n n n b is the focus whereas h 0(t) is a nuisance variable David Cox (1972) showed how to estimate b without having to assume a model for h 0(t) “Semi-parametric” n n h 0(t) is the baseline hazard - “non-parametric” part of the model b 1, b 2, …, bp are the regression coefficients “parametric” part of the model Think of estimating h 0(t) with a step function Let # steps get large — “partial likelihood” for b depends on b, not h 0(t)
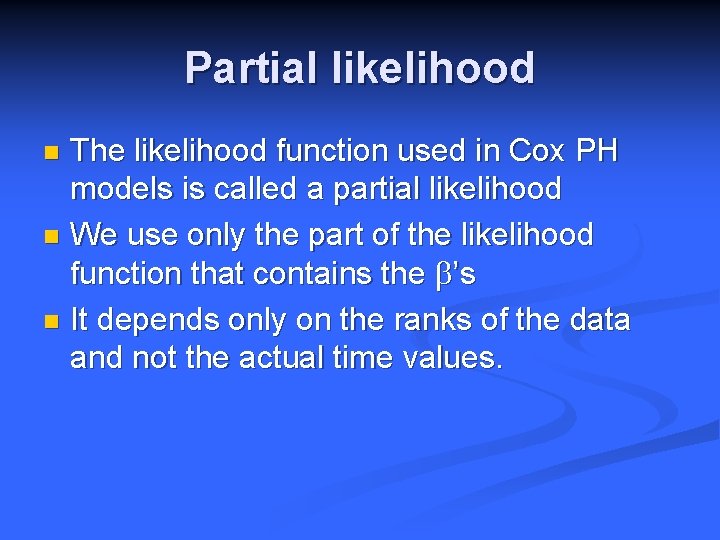
Partial likelihood The likelihood function used in Cox PH models is called a partial likelihood n We use only the part of the likelihood function that contains the b’s n It depends only on the ranks of the data and not the actual time values. n
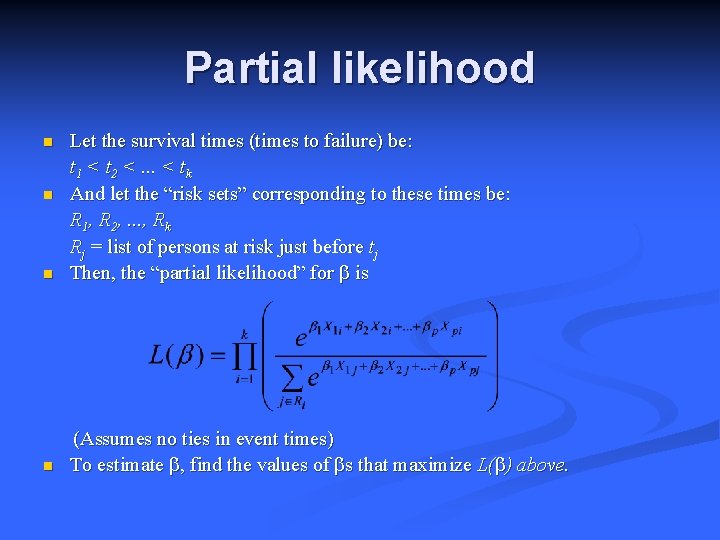
Partial likelihood n Let the survival times (times to failure) be: t 1 < t 2 <. . . < tk And let the “risk sets” corresponding to these times be: R 1, R 2, . . . , Rk Rj = list of persons at risk just before tj Then, the “partial likelihood” for b is n (Assumes no ties in event times) To estimate b, find the values of bs that maximize L(b) above. n n
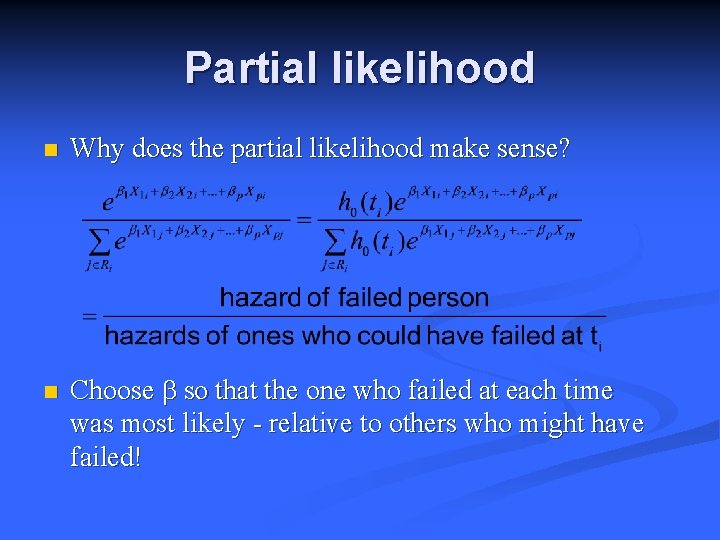
Partial likelihood n n Why does the partial likelihood make sense? Choose b so that the one who failed at each time was most likely - relative to others who might have failed!
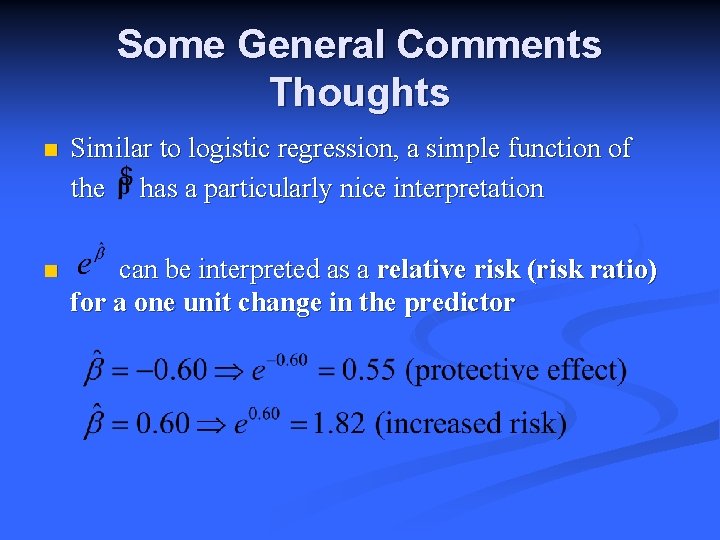
Some General Comments Thoughts n Similar to logistic regression, a simple function of the has a particularly nice interpretation n can be interpreted as a relative risk (risk ratio) for a one unit change in the predictor
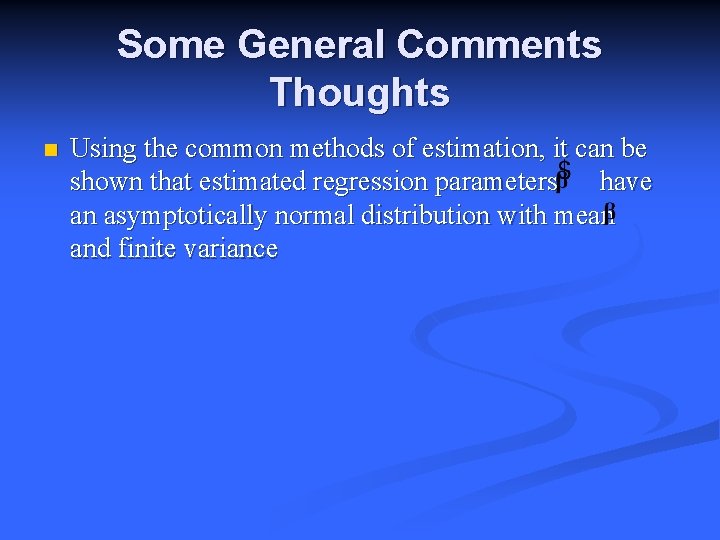
Some General Comments Thoughts n Using the common methods of estimation, it can be shown that estimated regression parameters have an asymptotically normal distribution with mean and finite variance
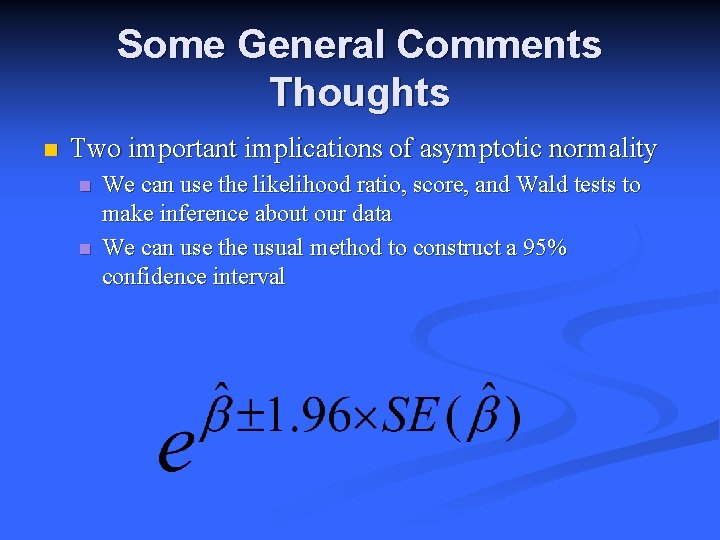
Some General Comments Thoughts n Two important implications of asymptotic normality n n We can use the likelihood ratio, score, and Wald tests to make inference about our data We can use the usual method to construct a 95% confidence interval
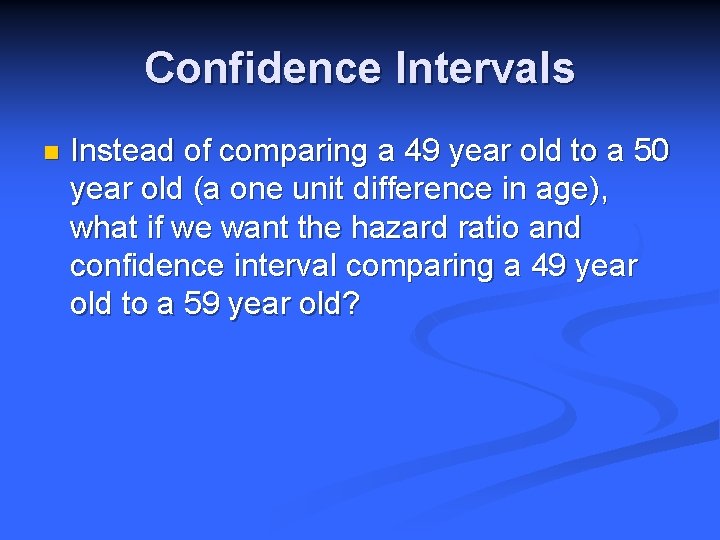
Confidence Intervals n Instead of comparing a 49 year old to a 50 year old (a one unit difference in age), what if we want the hazard ratio and confidence interval comparing a 49 year old to a 59 year old?
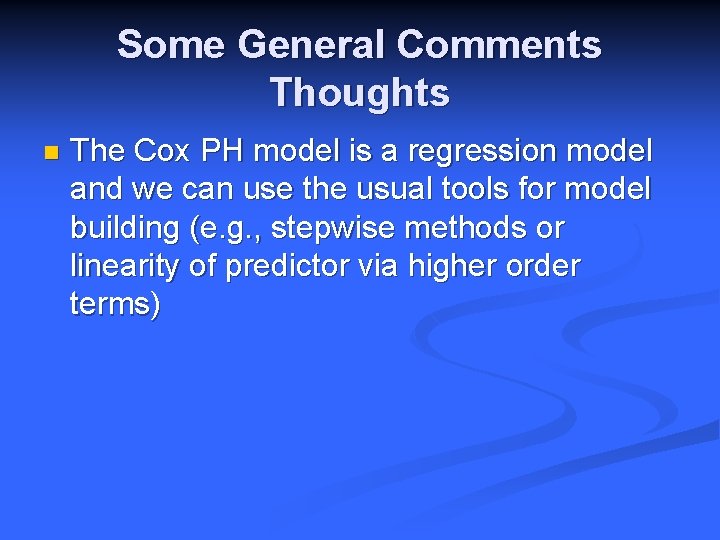
Some General Comments Thoughts n The Cox PH model is a regression model and we can use the usual tools for model building (e. g. , stepwise methods or linearity of predictor via higher order terms)
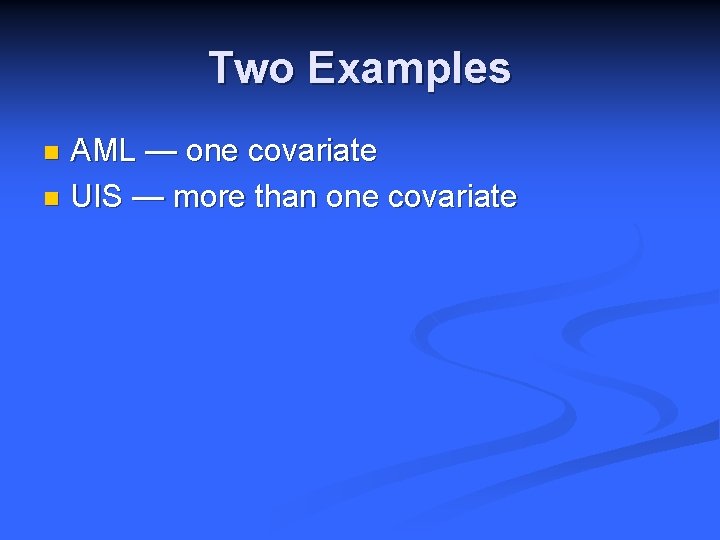
Two Examples AML — one covariate n UIS — more than one covariate n
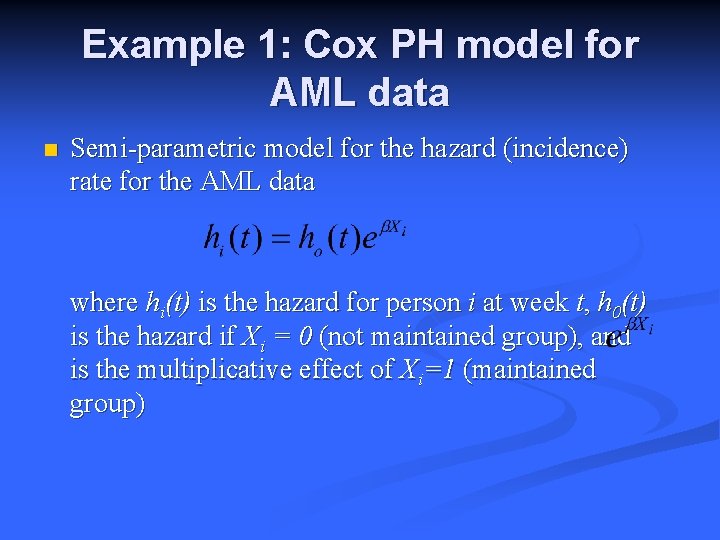
Example 1: Cox PH model for AML data n Semi-parametric model for the hazard (incidence) rate for the AML data where hi(t) is the hazard for person i at week t, h 0(t) is the hazard if Xi = 0 (not maintained group), and is the multiplicative effect of Xi=1 (maintained group)
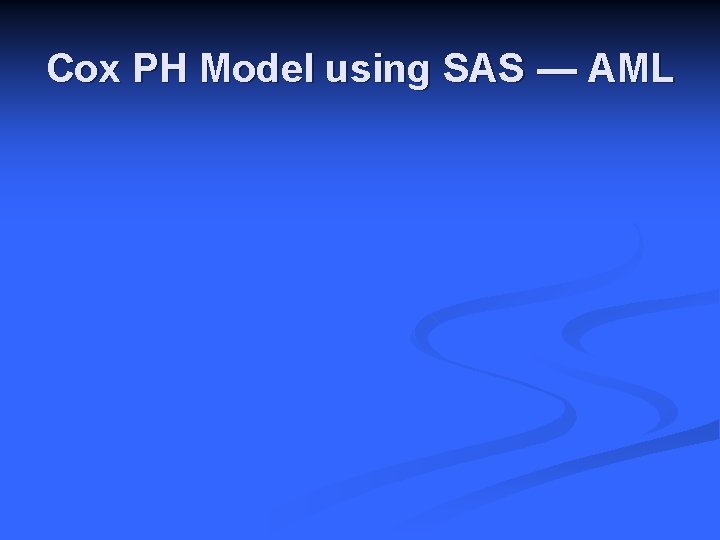
Cox PH Model using SAS — AML
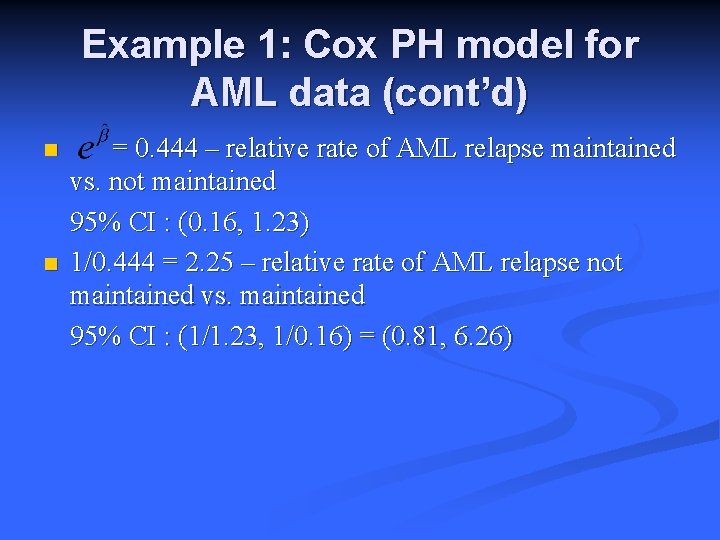
Example 1: Cox PH model for AML data (cont’d) n n = 0. 444 – relative rate of AML relapse maintained vs. not maintained 95% CI : (0. 16, 1. 23) 1/0. 444 = 2. 25 – relative rate of AML relapse not maintained vs. maintained 95% CI : (1/1. 23, 1/0. 16) = (0. 81, 6. 26)
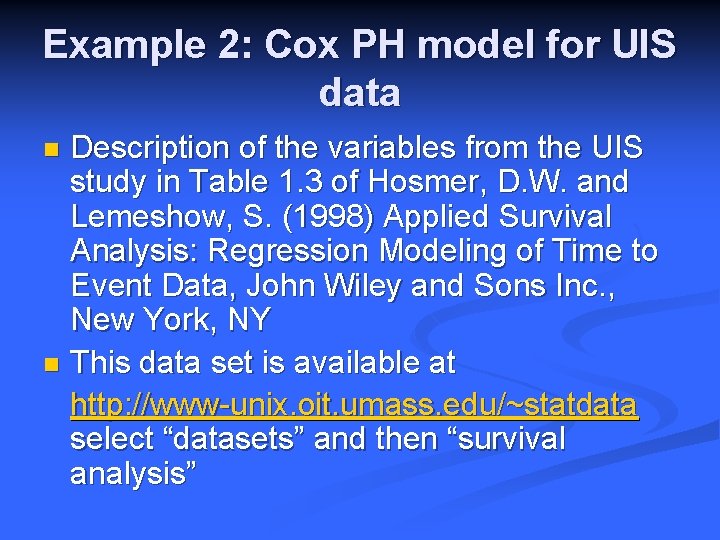
Example 2: Cox PH model for UIS data Description of the variables from the UIS study in Table 1. 3 of Hosmer, D. W. and Lemeshow, S. (1998) Applied Survival Analysis: Regression Modeling of Time to Event Data, John Wiley and Sons Inc. , New York, NY n This data set is available at http: //www-unix. oit. umass. edu/~statdata select “datasets” and then “survival analysis” n
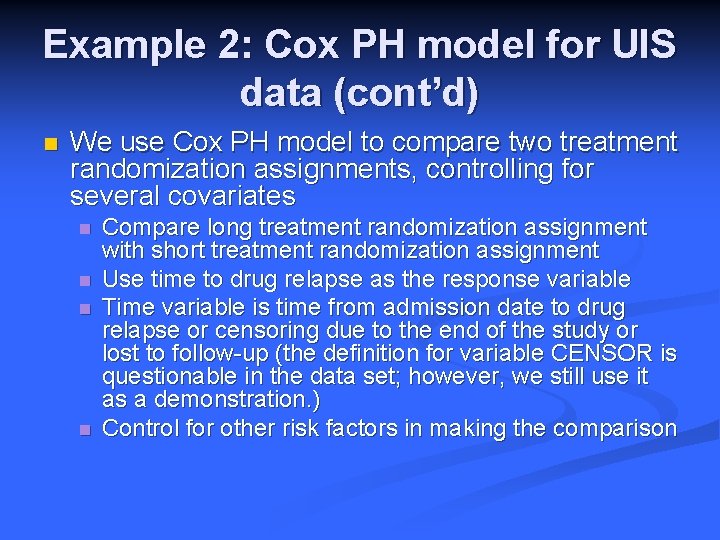
Example 2: Cox PH model for UIS data (cont’d) n We use Cox PH model to compare two treatment randomization assignments, controlling for several covariates n n Compare long treatment randomization assignment with short treatment randomization assignment Use time to drug relapse as the response variable Time variable is time from admission date to drug relapse or censoring due to the end of the study or lost to follow-up (the definition for variable CENSOR is questionable in the data set; however, we still use it as a demonstration. ) Control for other risk factors in making the comparison
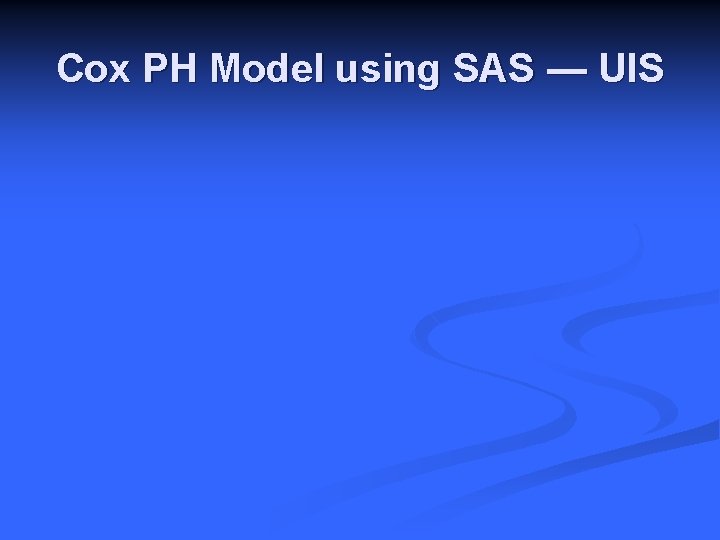
Cox PH Model using SAS — UIS
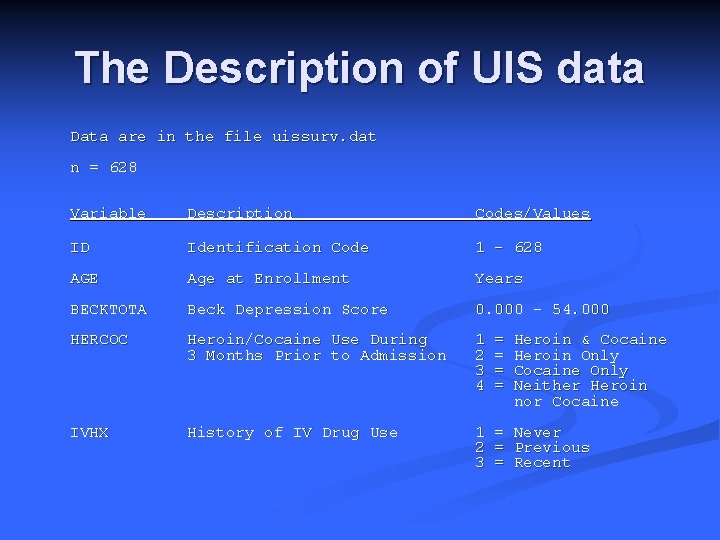
The Description of UIS data Data are in the file uissurv. dat n = 628 Variable Description Codes/Values ID Identification Code 1 - 628 AGE Age at Enrollment Years BECKTOTA Beck Depression Score 0. 000 - 54. 000 HERCOC Heroin/Cocaine Use During 3 Months Prior to Admission 1 2 3 4 = = Heroin & Cocaine Heroin Only Cocaine Only Neither Heroin nor Cocaine IVHX History of IV Drug Use 1 2 3 = = = Never Previous Recent
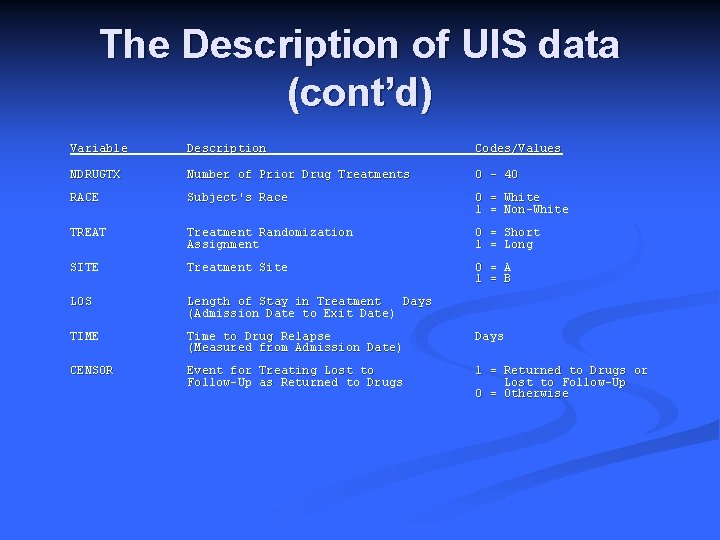
The Description of UIS data (cont’d) Variable Description Codes/Values NDRUGTX Number of Prior Drug Treatments 0 - 40 RACE Subject's Race 0 = White 1 = Non-White TREAT Treatment Randomization Assignment 0 = Short 1 = Long SITE Treatment Site 0 = A 1 = B LOS Length of Stay in Treatment Days (Admission Date to Exit Date) TIME Time to Drug Relapse (Measured from Admission Date) Days CENSOR Event for Treating Lost to Follow-Up as Returned to Drugs 1 = Returned to Drugs or Lost to Follow-Up 0 = Otherwise
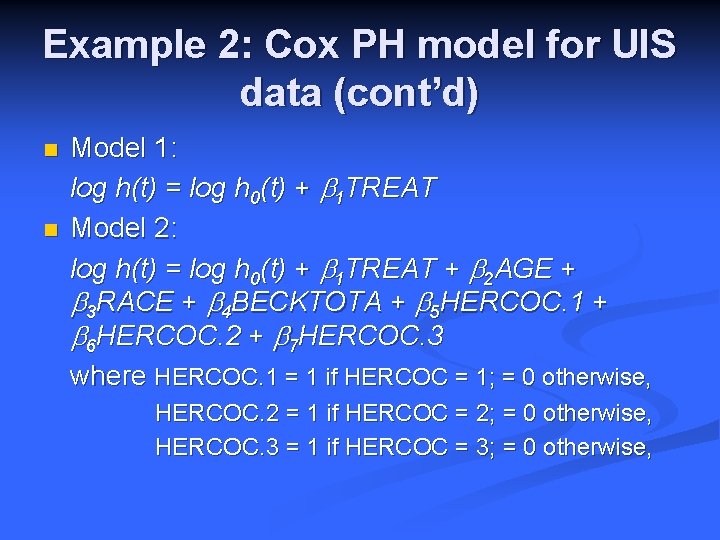
Example 2: Cox PH model for UIS data (cont’d) n n Model 1: log h(t) = log h 0(t) + b 1 TREAT Model 2: log h(t) = log h 0(t) + b 1 TREAT + b 2 AGE + b 3 RACE + b 4 BECKTOTA + b 5 HERCOC. 1 + b 6 HERCOC. 2 + b 7 HERCOC. 3 where HERCOC. 1 = 1 if HERCOC = 1; = 0 otherwise, HERCOC. 2 = 1 if HERCOC = 2; = 0 otherwise, HERCOC. 3 = 1 if HERCOC = 3; = 0 otherwise,
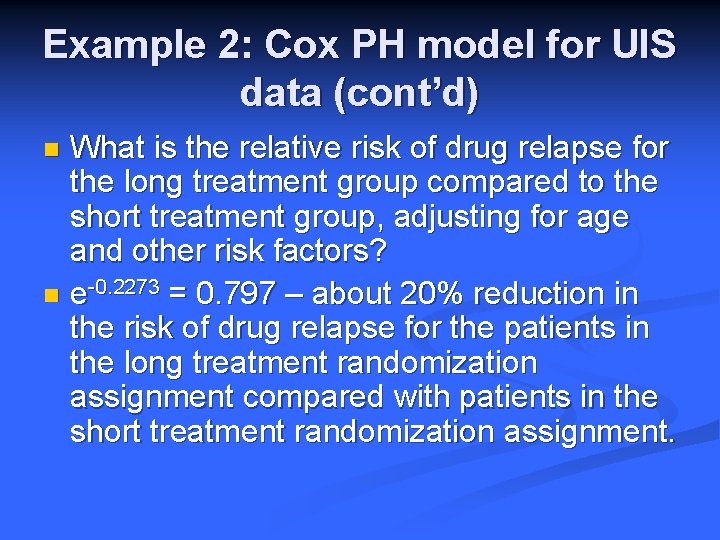
Example 2: Cox PH model for UIS data (cont’d) What is the relative risk of drug relapse for the long treatment group compared to the short treatment group, adjusting for age and other risk factors? n e-0. 2273 = 0. 797 – about 20% reduction in the risk of drug relapse for the patients in the long treatment randomization assignment compared with patients in the short treatment randomization assignment. n
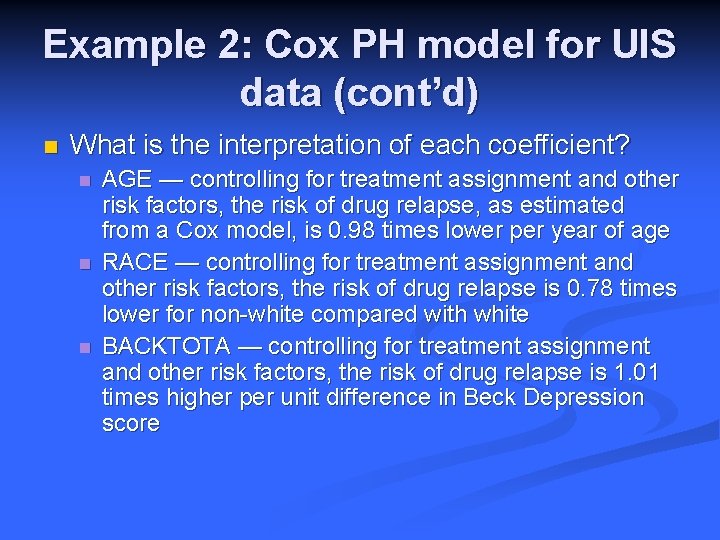
Example 2: Cox PH model for UIS data (cont’d) n What is the interpretation of each coefficient? n n n AGE — controlling for treatment assignment and other risk factors, the risk of drug relapse, as estimated from a Cox model, is 0. 98 times lower per year of age RACE — controlling for treatment assignment and other risk factors, the risk of drug relapse is 0. 78 times lower for non-white compared with white BACKTOTA — controlling for treatment assignment and other risk factors, the risk of drug relapse is 1. 01 times higher per unit difference in Beck Depression score
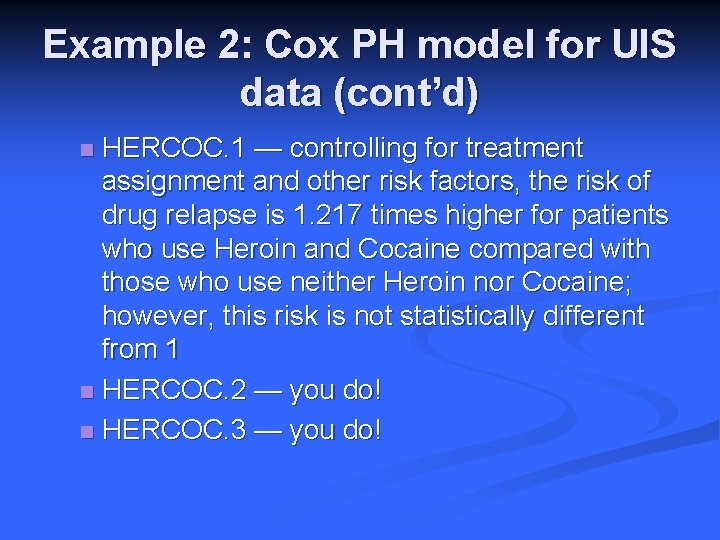
Example 2: Cox PH model for UIS data (cont’d) HERCOC. 1 — controlling for treatment assignment and other risk factors, the risk of drug relapse is 1. 217 times higher for patients who use Heroin and Cocaine compared with those who use neither Heroin nor Cocaine; however, this risk is not statistically different from 1 n HERCOC. 2 — you do! n HERCOC. 3 — you do! n
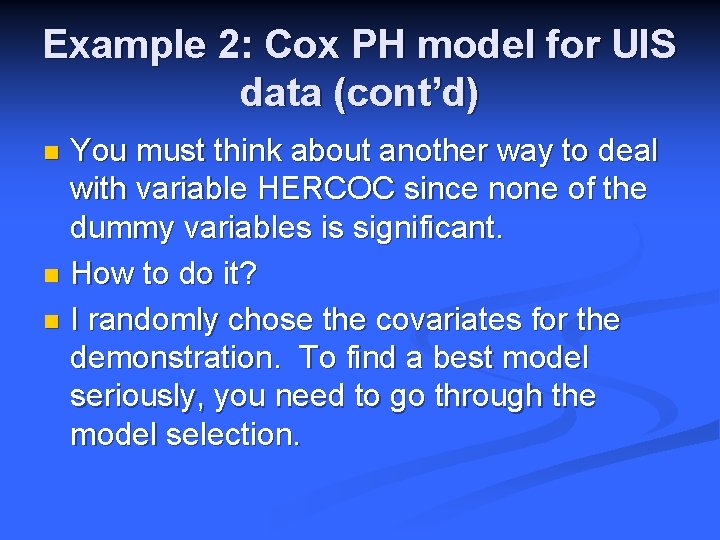
Example 2: Cox PH model for UIS data (cont’d) You must think about another way to deal with variable HERCOC since none of the dummy variables is significant. n How to do it? n I randomly chose the covariates for the demonstration. To find a best model seriously, you need to go through the model selection. n
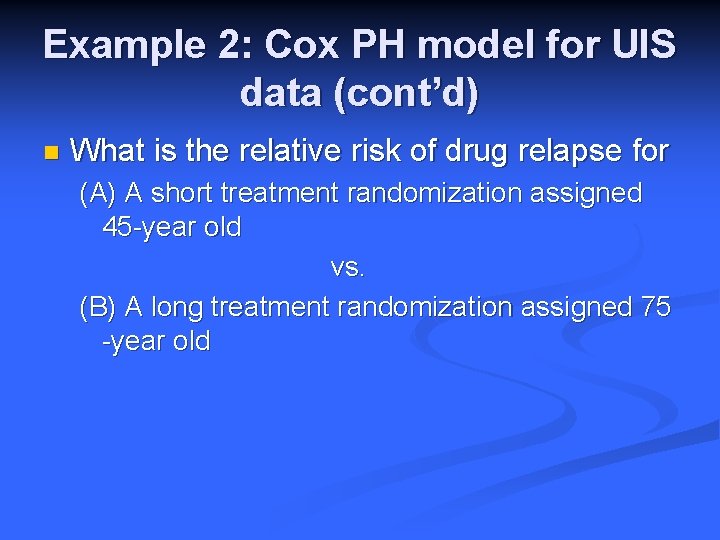
Example 2: Cox PH model for UIS data (cont’d) n What is the relative risk of drug relapse for (A) A short treatment randomization assigned 45 -year old vs. (B) A long treatment randomization assigned 75 -year old
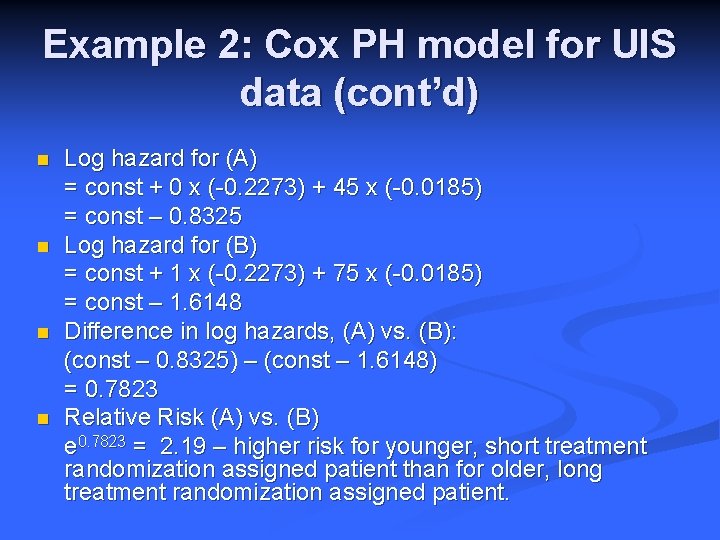
Example 2: Cox PH model for UIS data (cont’d) n n Log hazard for (A) = const + 0 x (-0. 2273) + 45 x (-0. 0185) = const – 0. 8325 Log hazard for (B) = const + 1 x (-0. 2273) + 75 x (-0. 0185) = const – 1. 6148 Difference in log hazards, (A) vs. (B): (const – 0. 8325) – (const – 1. 6148) = 0. 7823 Relative Risk (A) vs. (B) e 0. 7823 = 2. 19 – higher risk for younger, short treatment randomization assigned patient than for older, long treatment randomization assigned patient.
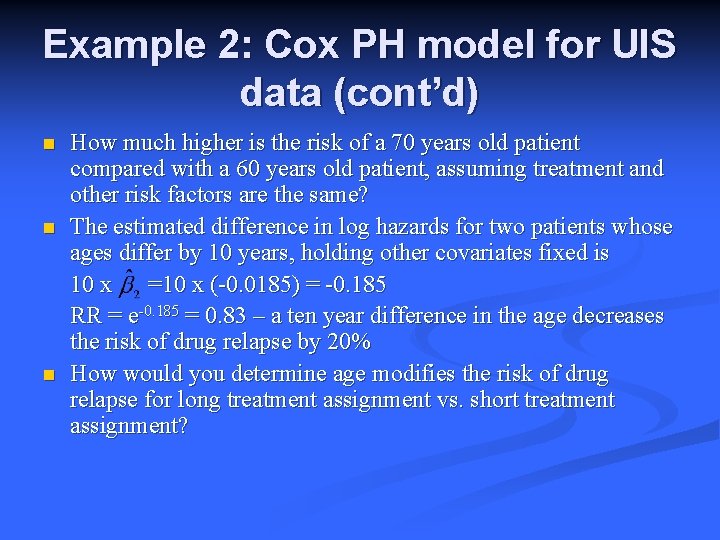
Example 2: Cox PH model for UIS data (cont’d) n n n How much higher is the risk of a 70 years old patient compared with a 60 years old patient, assuming treatment and other risk factors are the same? The estimated difference in log hazards for two patients whose ages differ by 10 years, holding other covariates fixed is 10 x =10 x (-0. 0185) = -0. 185 RR = e-0. 185 = 0. 83 – a ten year difference in the age decreases the risk of drug relapse by 20% How would you determine age modifies the risk of drug relapse for long treatment assignment vs. short treatment assignment?
Advanced and multivariate statistical methods
734-147-911
734 kc
70-734
Hsrp load balancing
First hop redundancy protocol cisco
Anycast hsrp
Hsrp troubleshooting commands
2008 2008
Statistical methods of demand forecasting
Statistical methods of demand forecasting
Branches of statics
Advanced construction methods
Advanced business research
Advanced higher modern studies essay structure
Wax pattern fabrication
July 30 2009 nasa
Missing in venice harris burdick
Malaga in july
July 16 1776
July 2 1937 amelia earhart
The cuban melodrama
Ctdssmap payment schedule july 2021
Ruth bruno
Super saturday tribal bingo july 4
On july 18 2001 a train carrying hazardous chemicals
Sources nso frenchhowell neill mit technology...
A strange day in july picture
June 22 to july 22
Poppies in july poem
Sergei korolev
2001 july 15
January february march april may june
Why are leaf yeasts more plentiful in july
July 4 sermon
What is the significance of july 4 1776 brainpop
July 1-4 1863
July 12 1776
July 10 1856
6th july 1988
2003 july 17
July 14 1789