Gerald Nielson AUGMENTED REALITY Overview What is Augmented
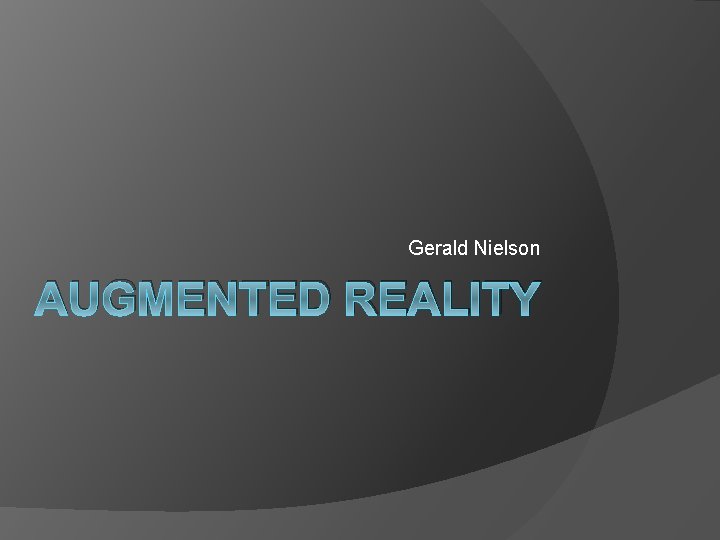
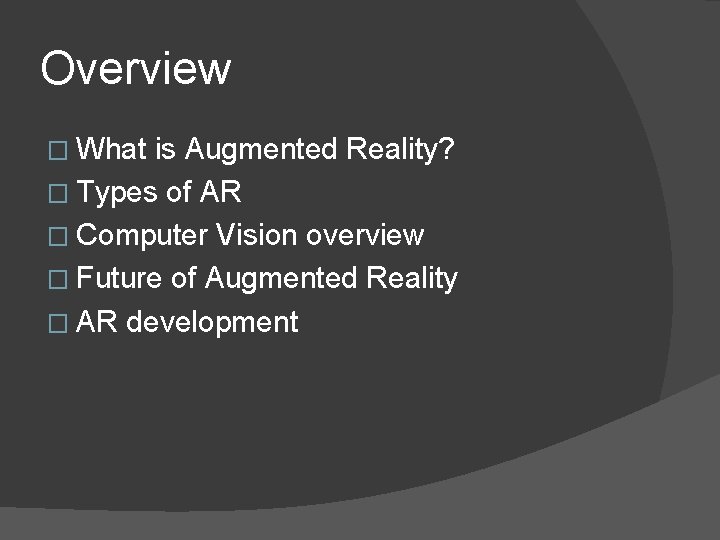
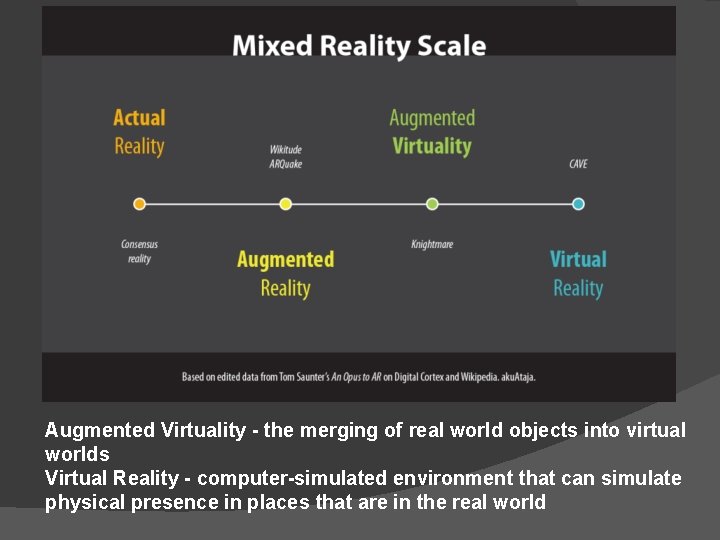
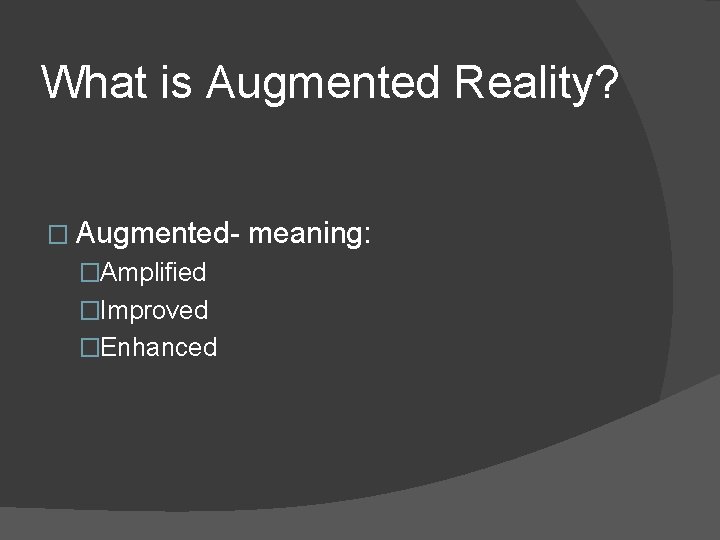
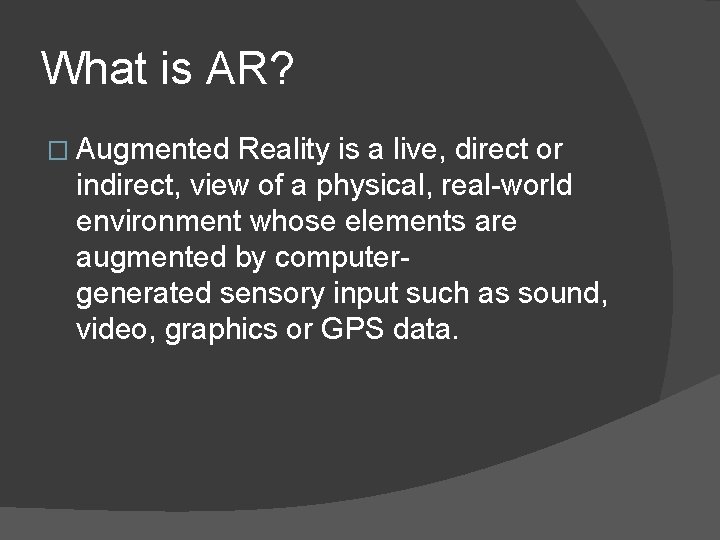
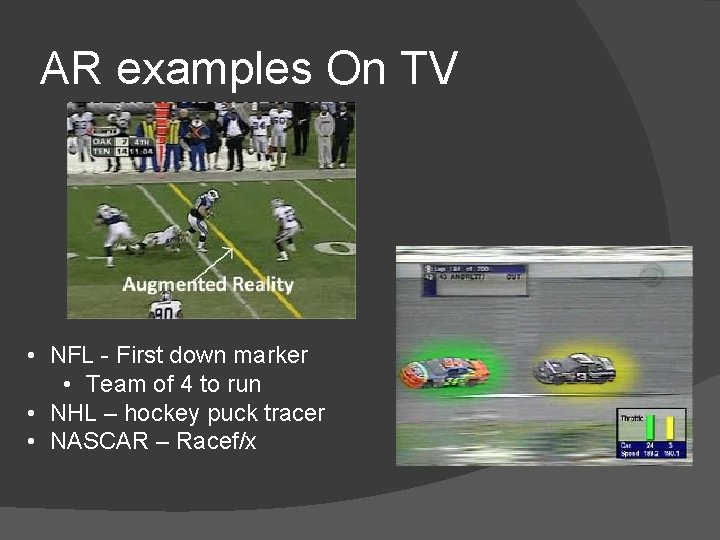
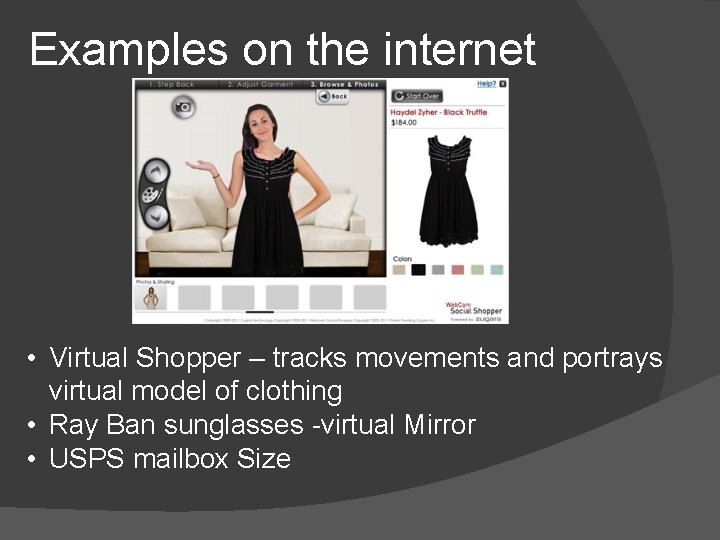
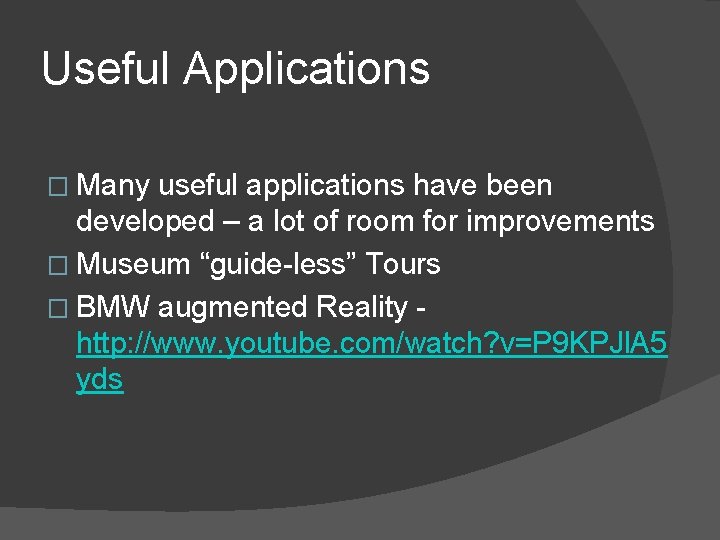
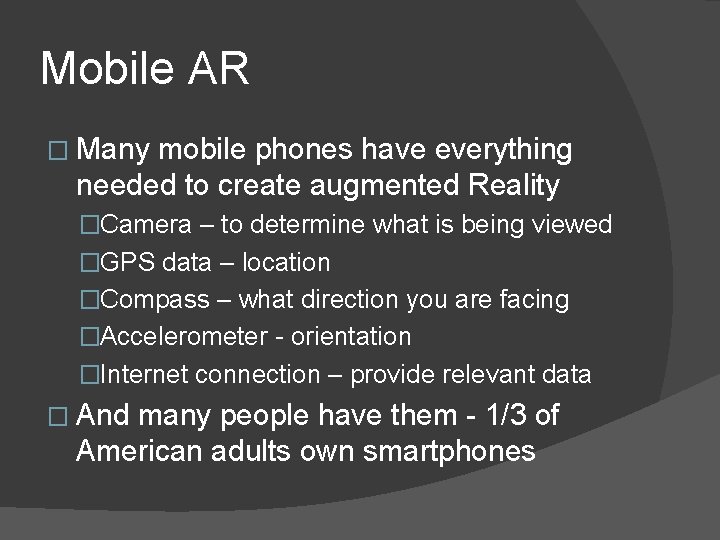
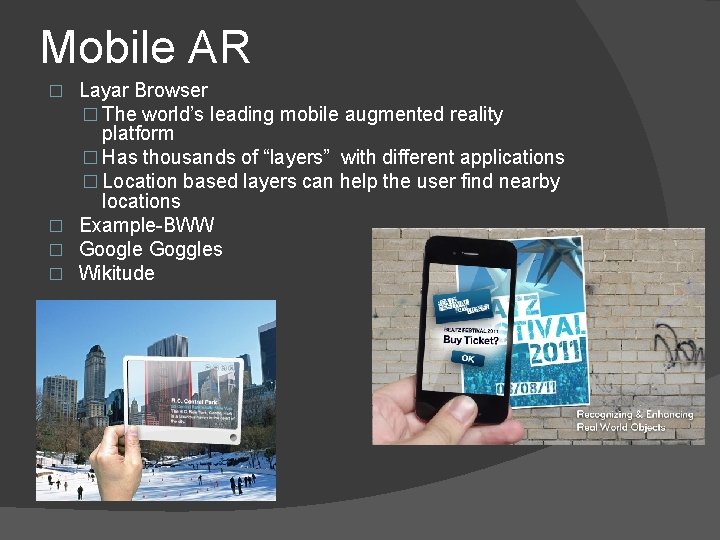
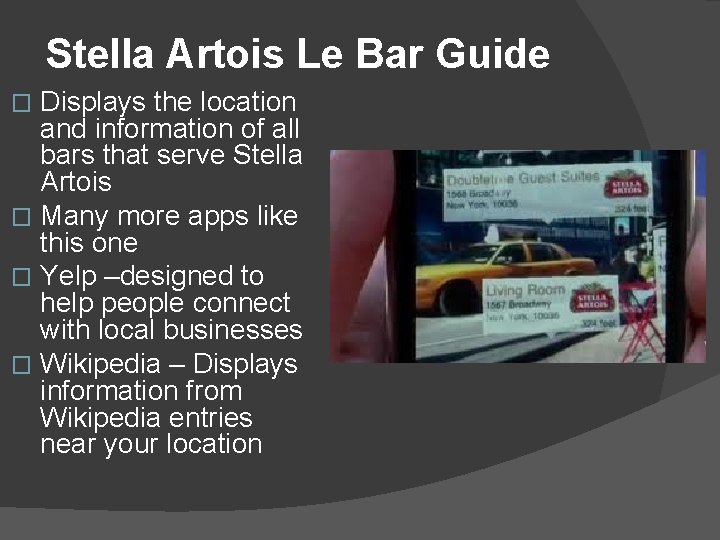
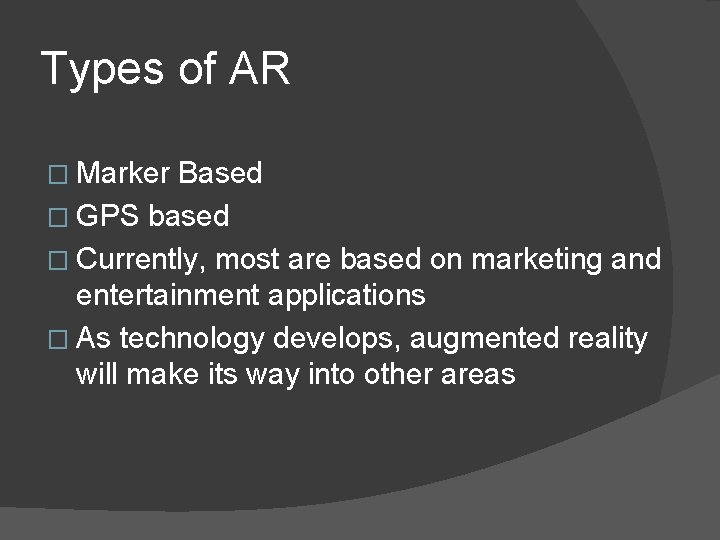
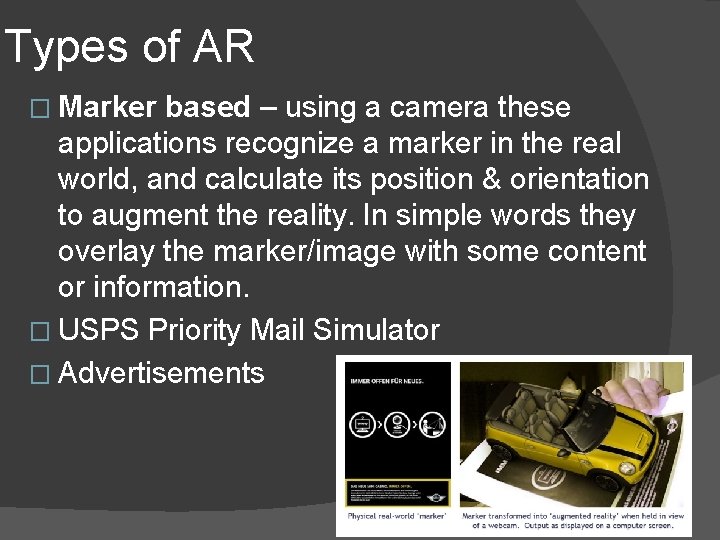
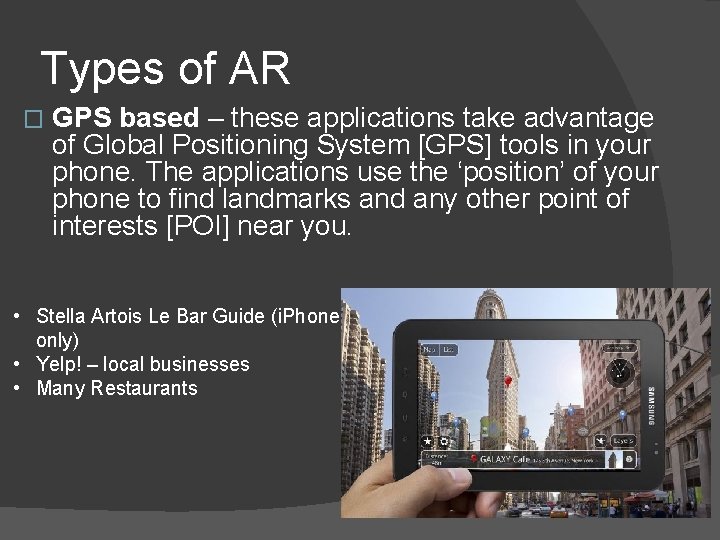
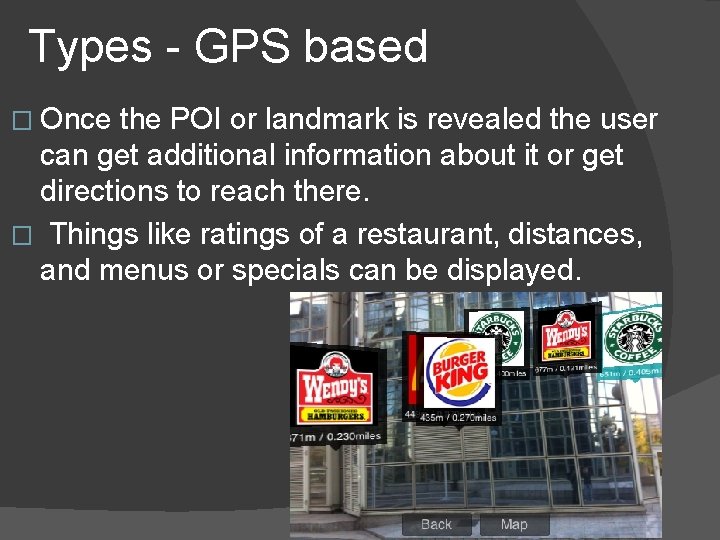
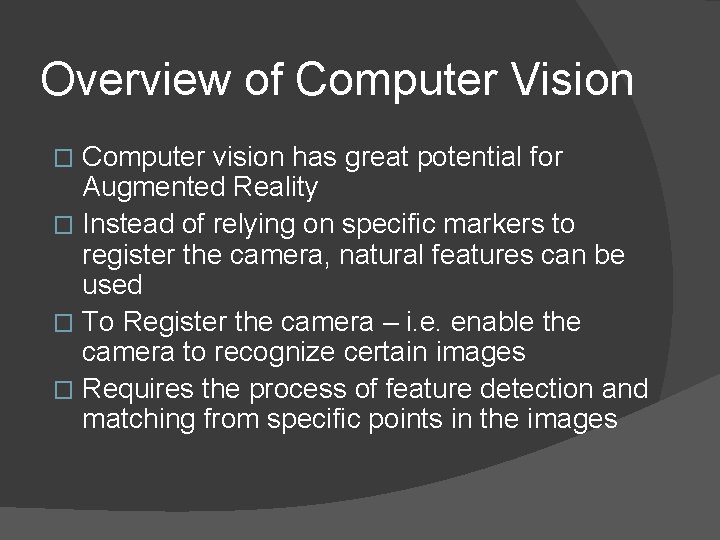
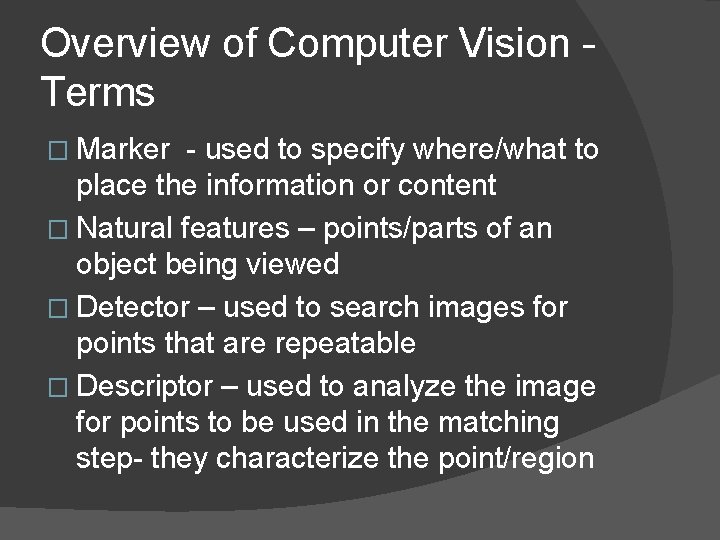
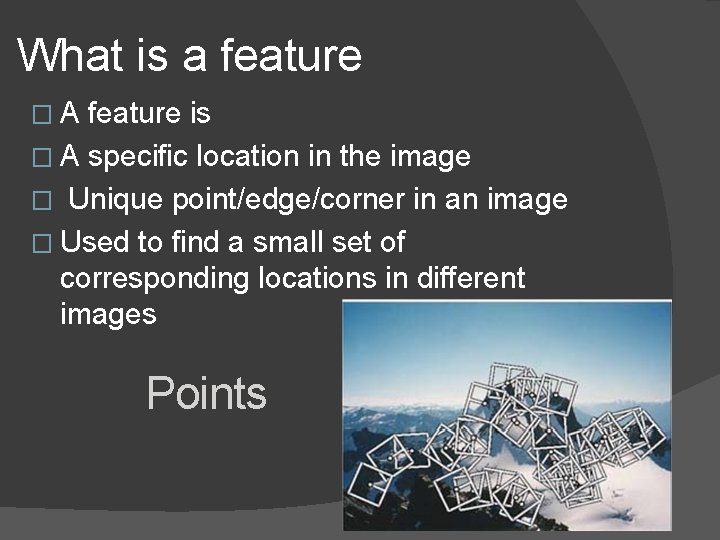
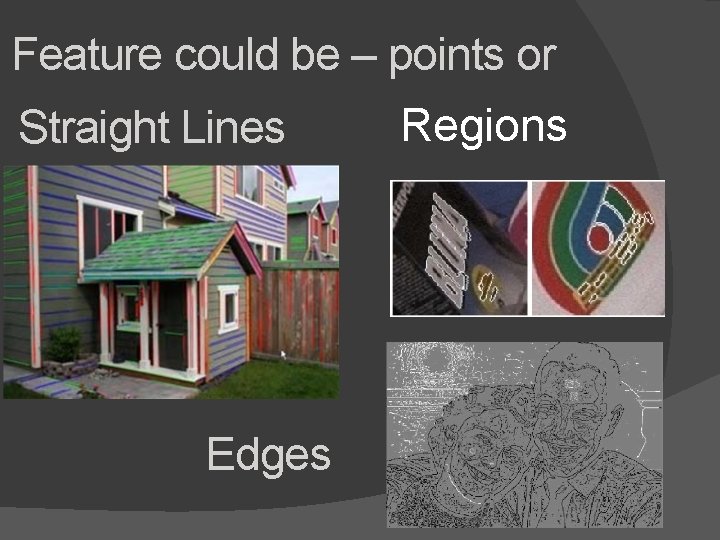
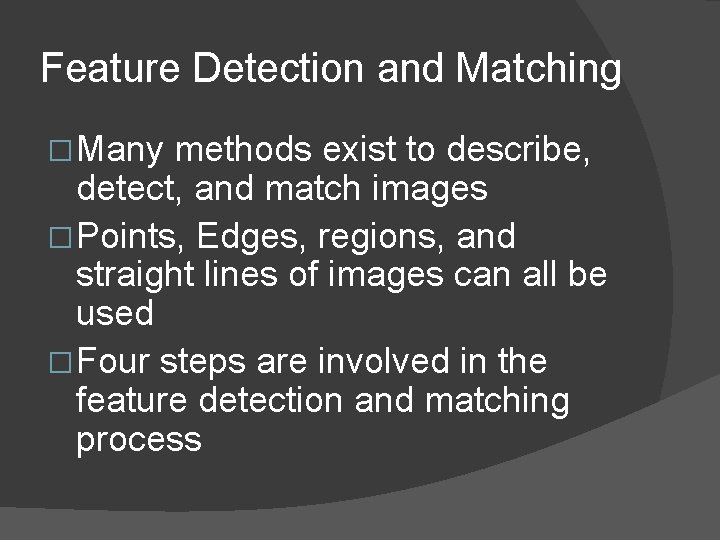
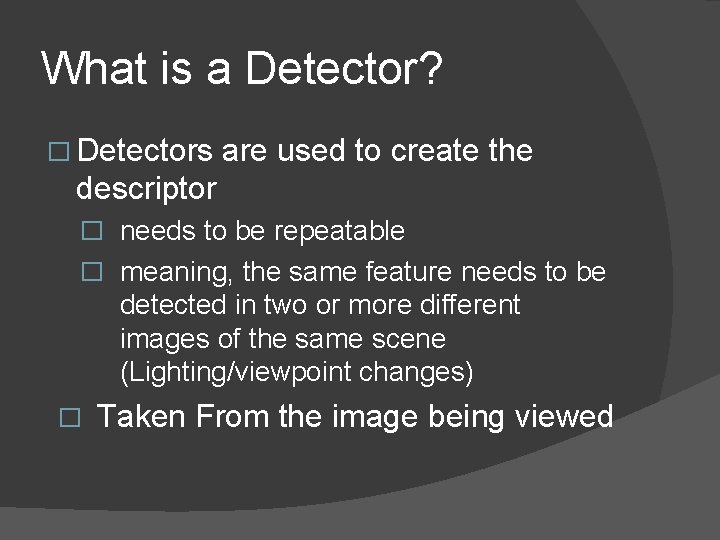
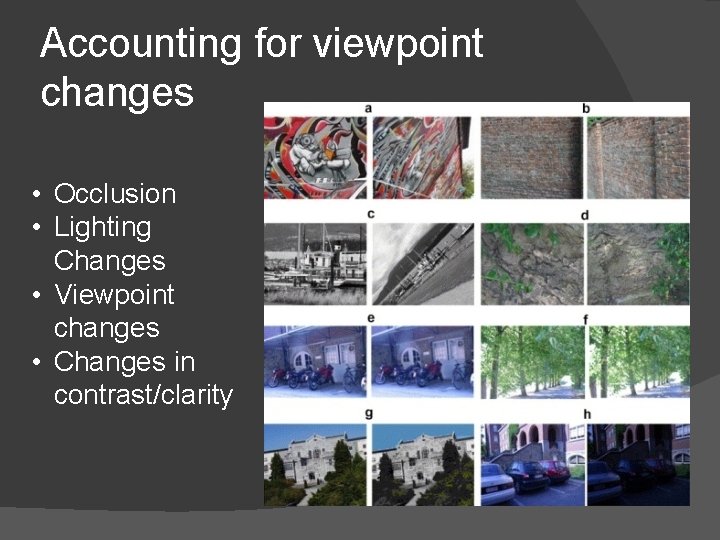
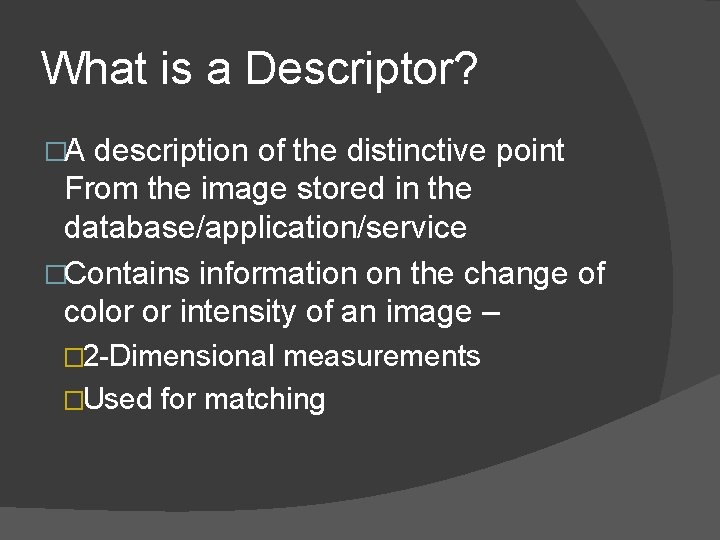
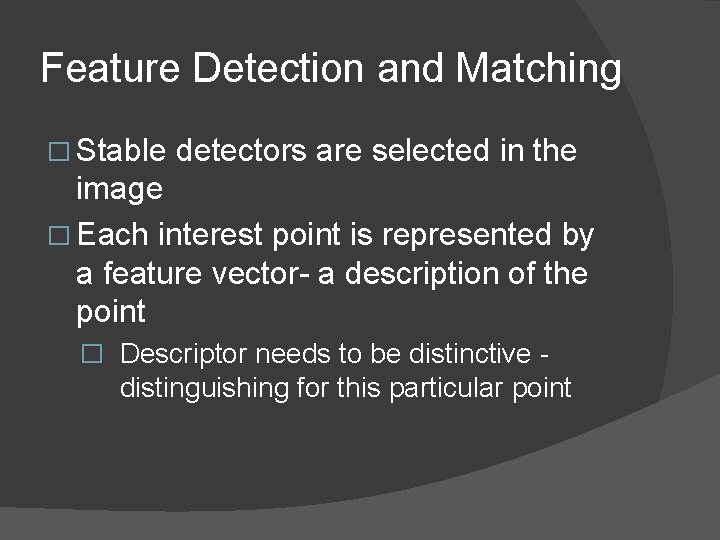
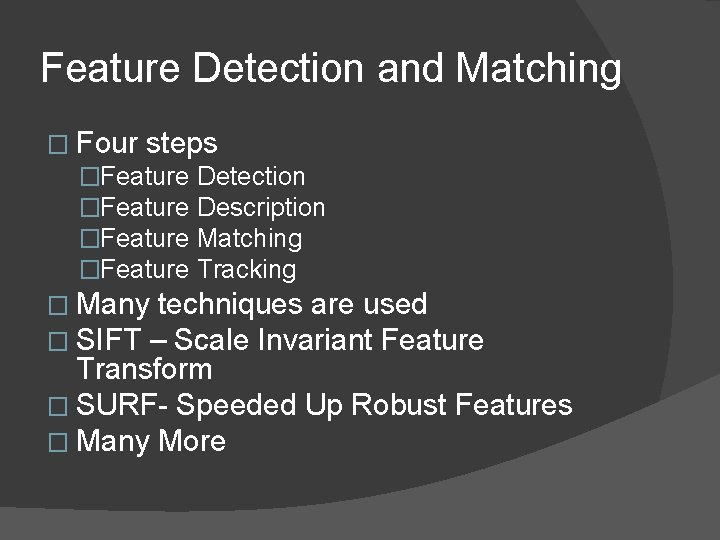
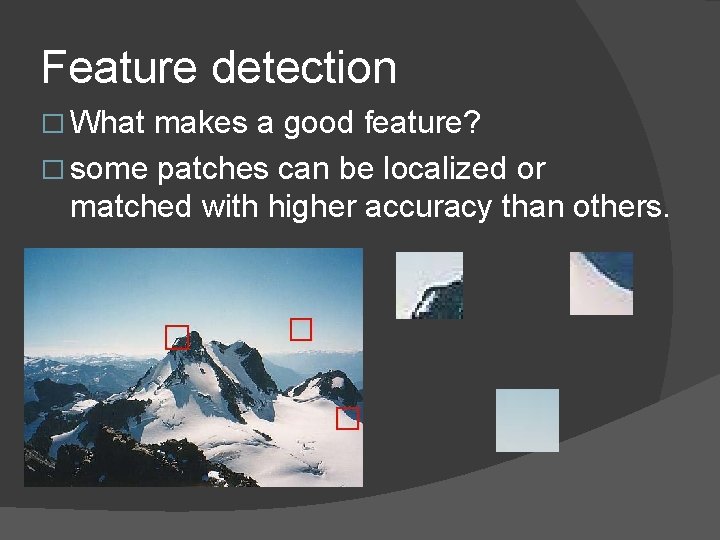
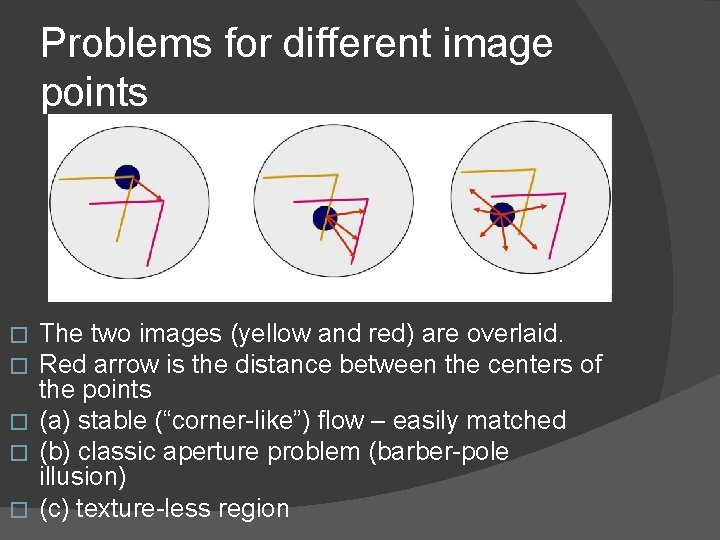
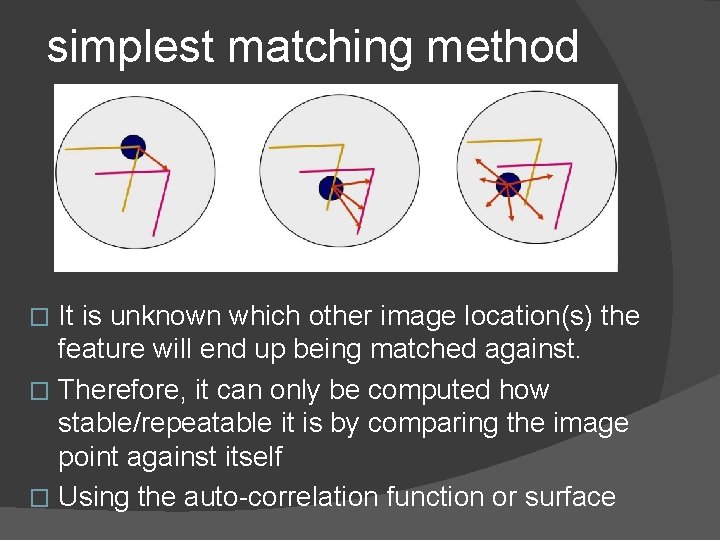
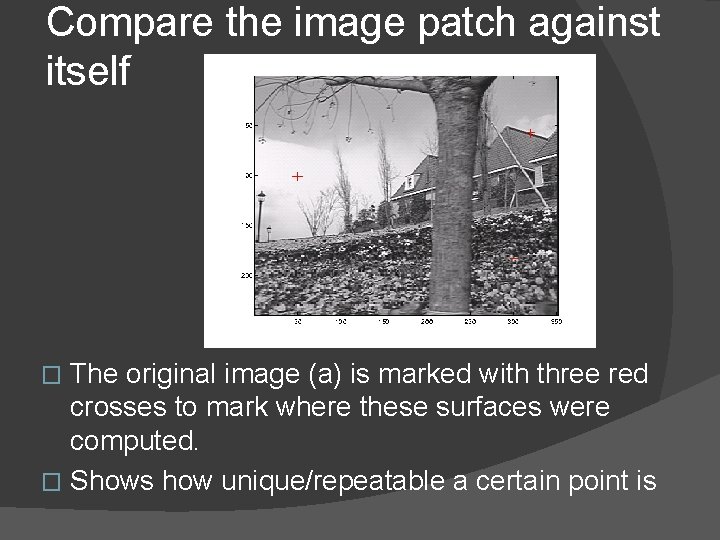
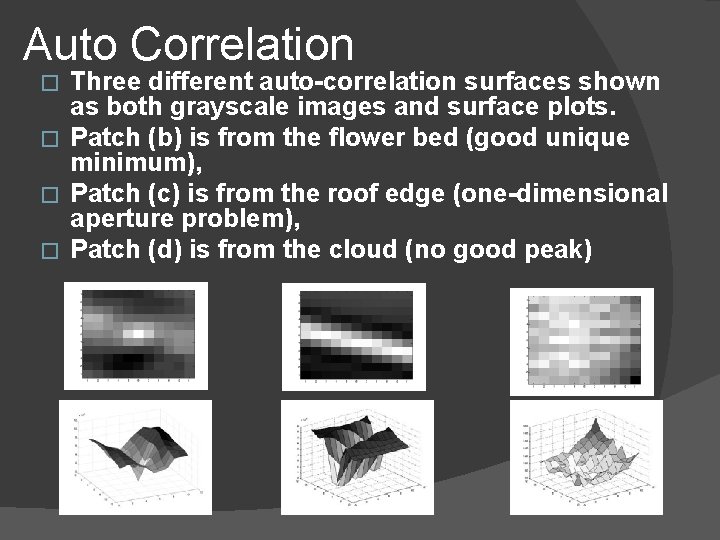
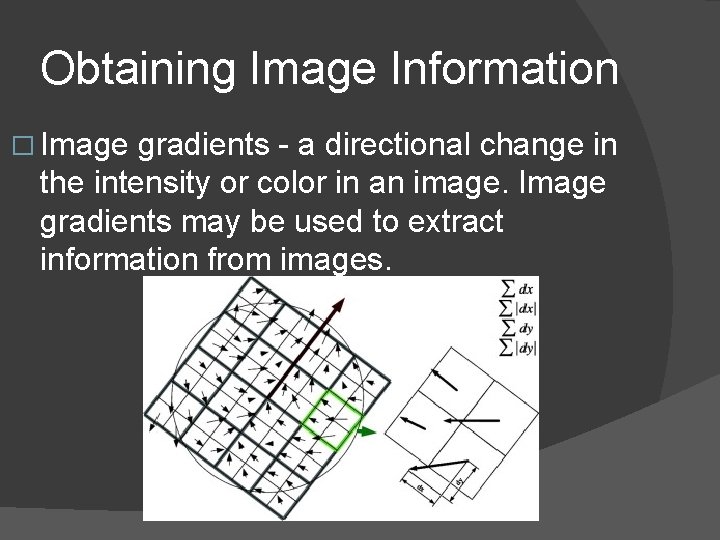
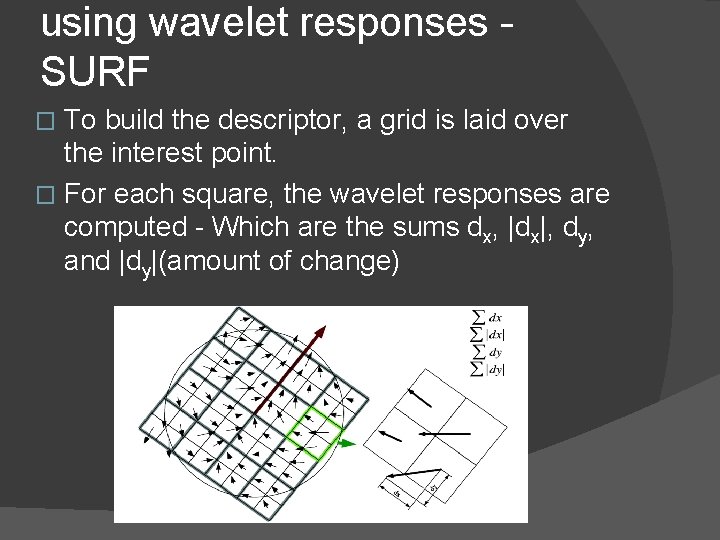
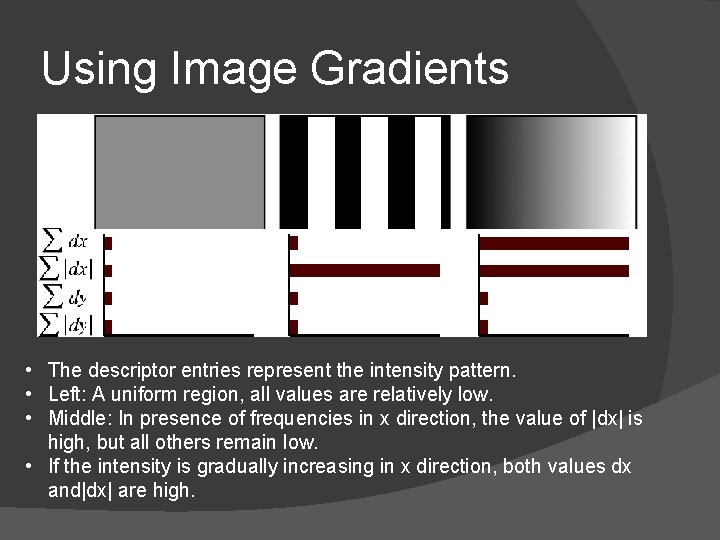
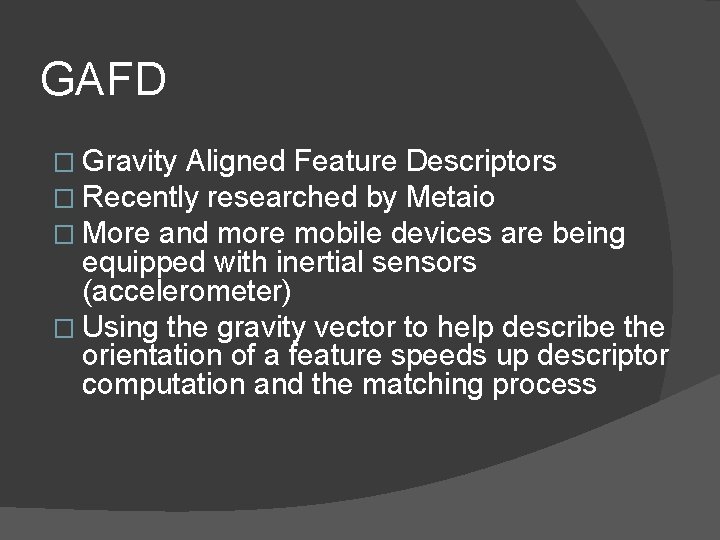
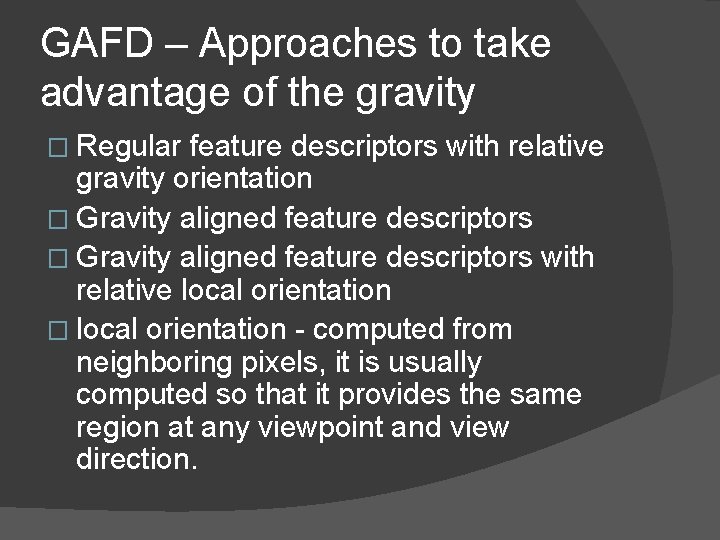
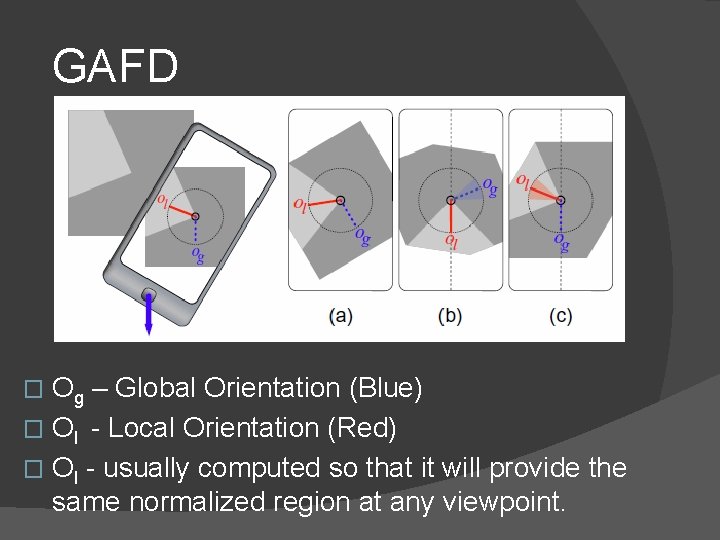
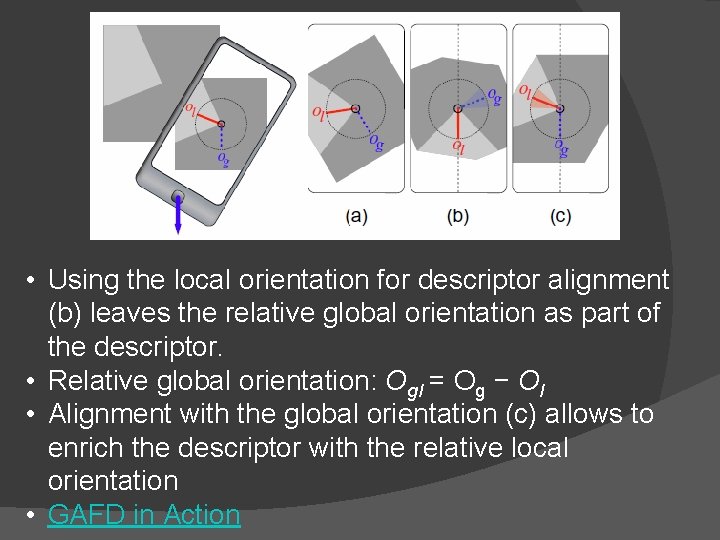
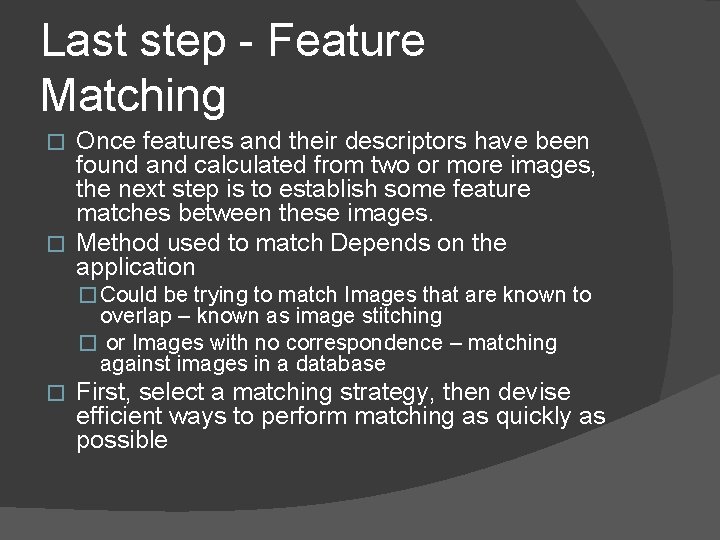
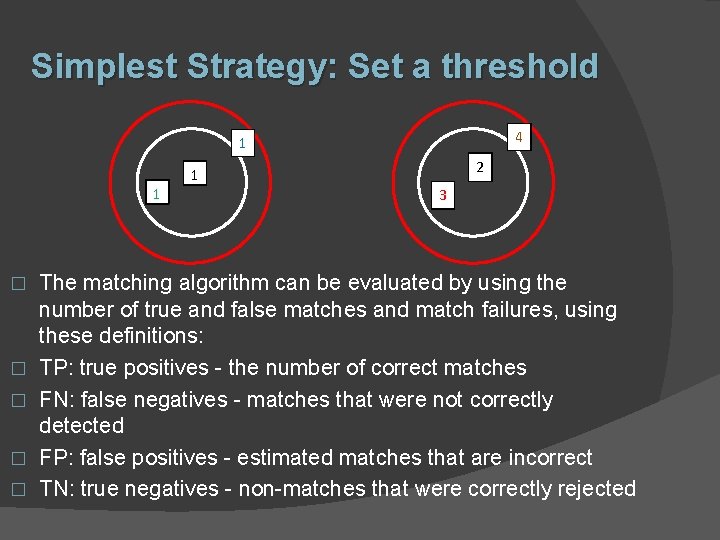
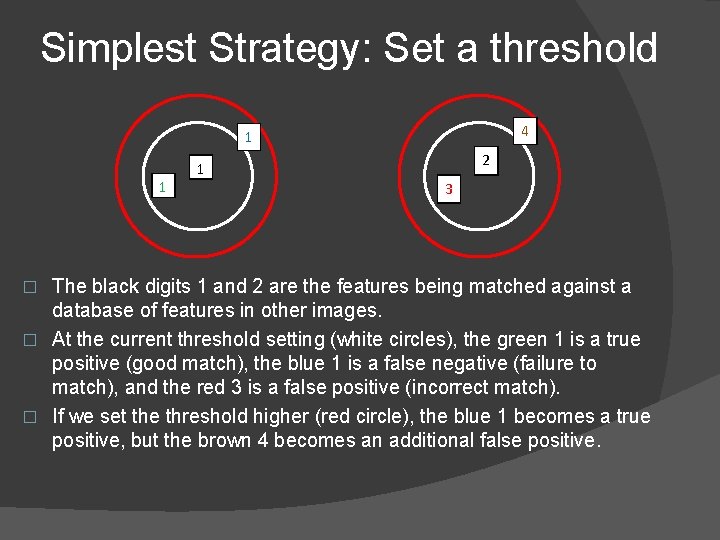
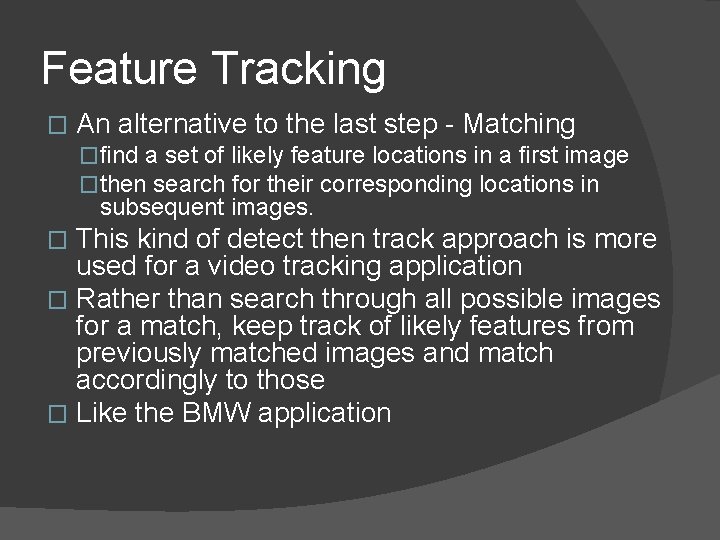
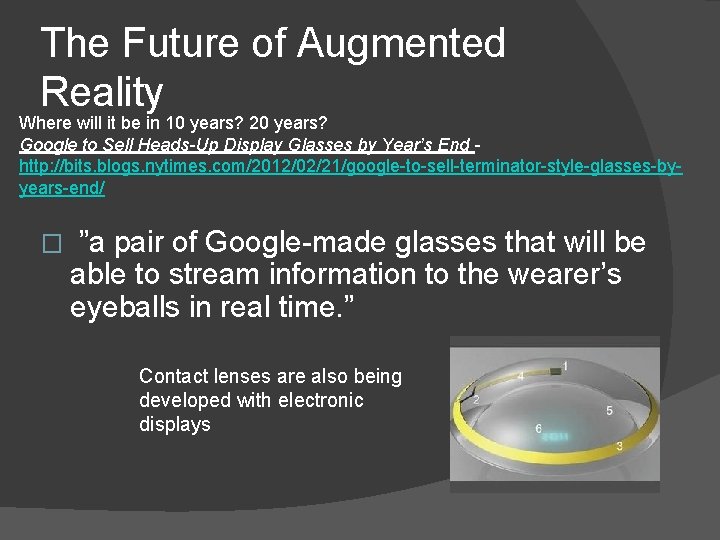
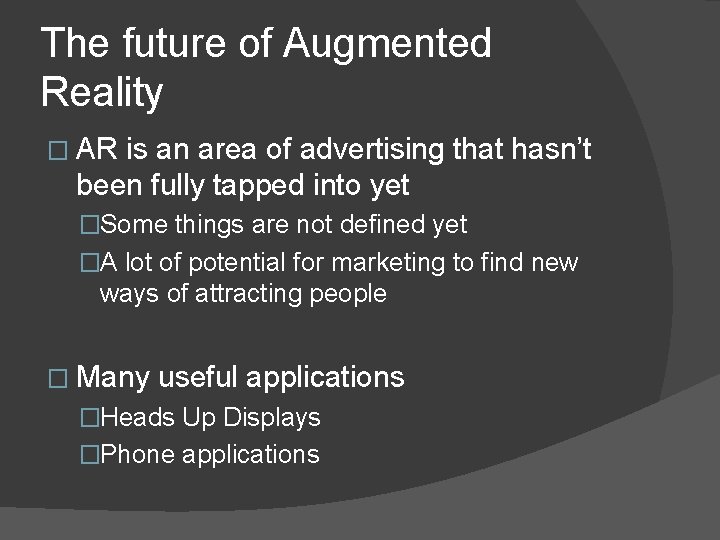
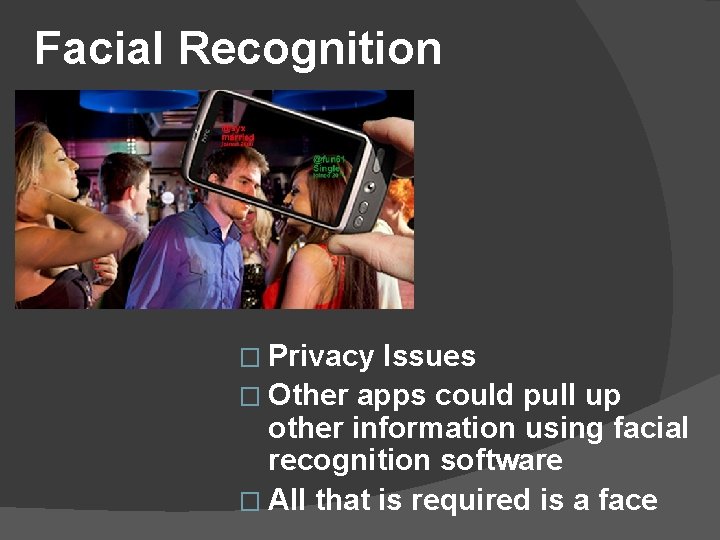
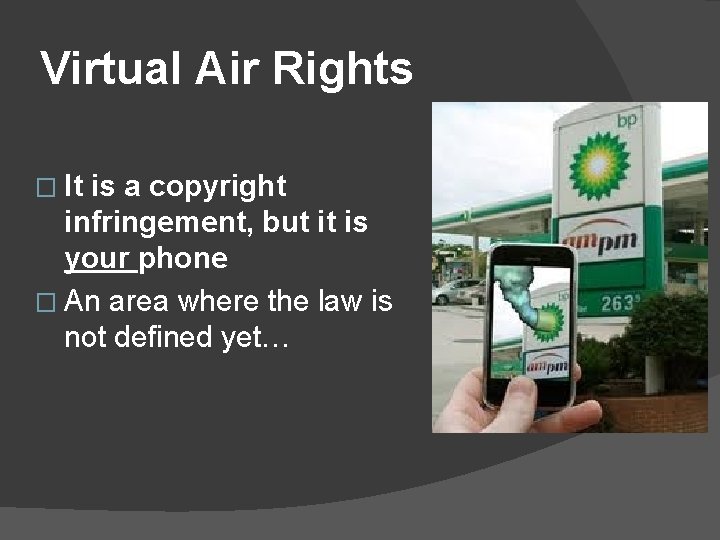
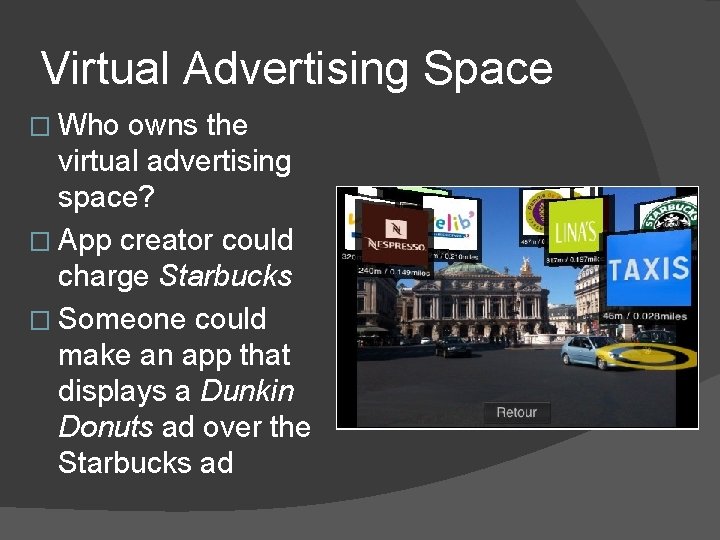
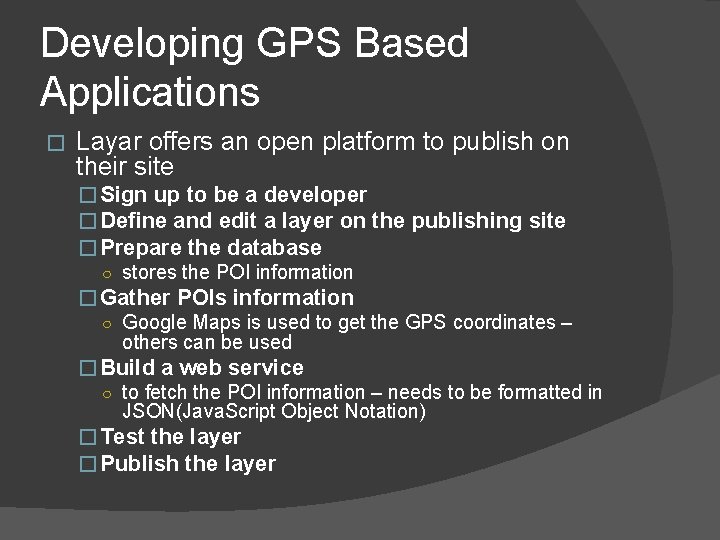
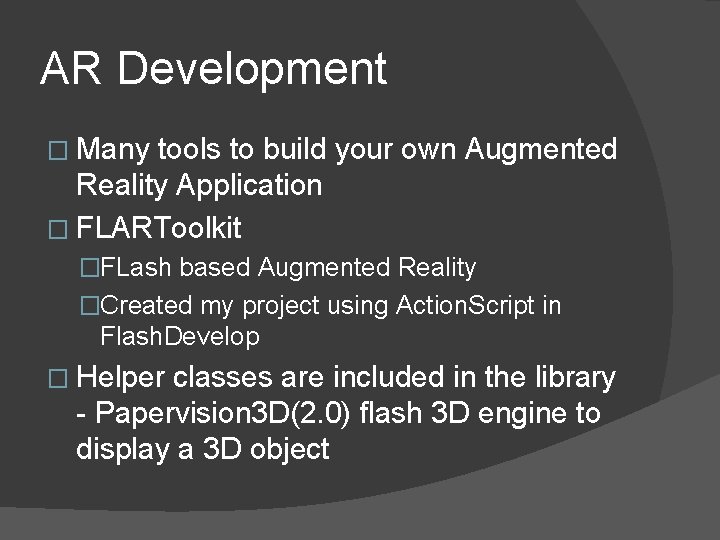
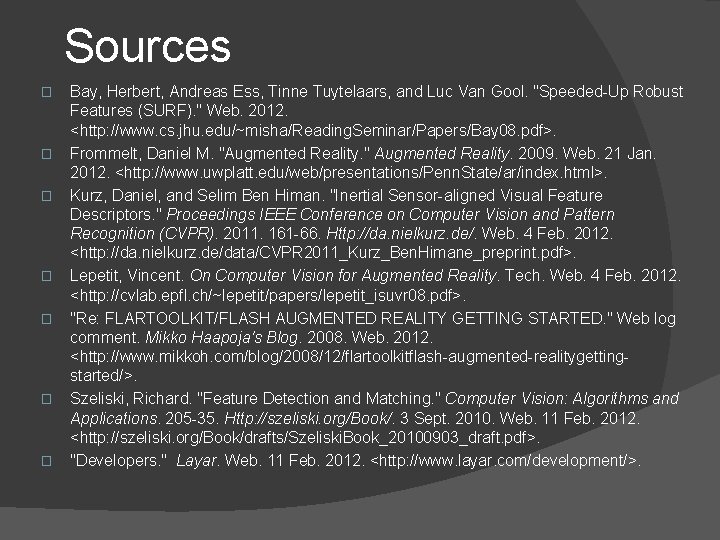
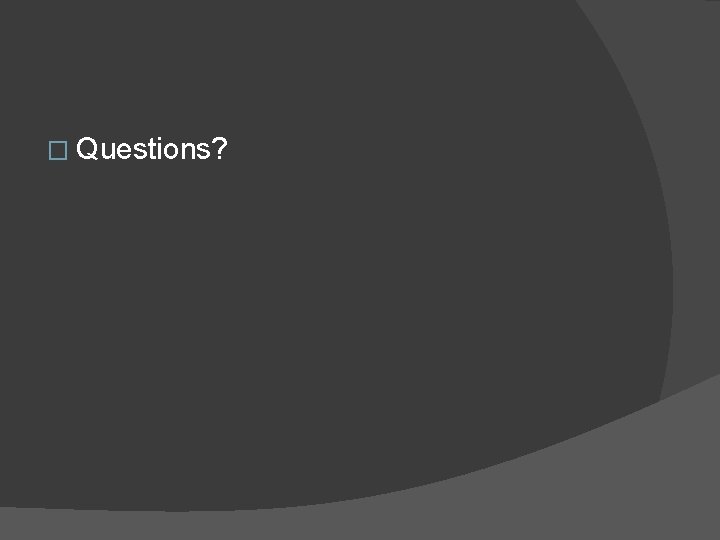
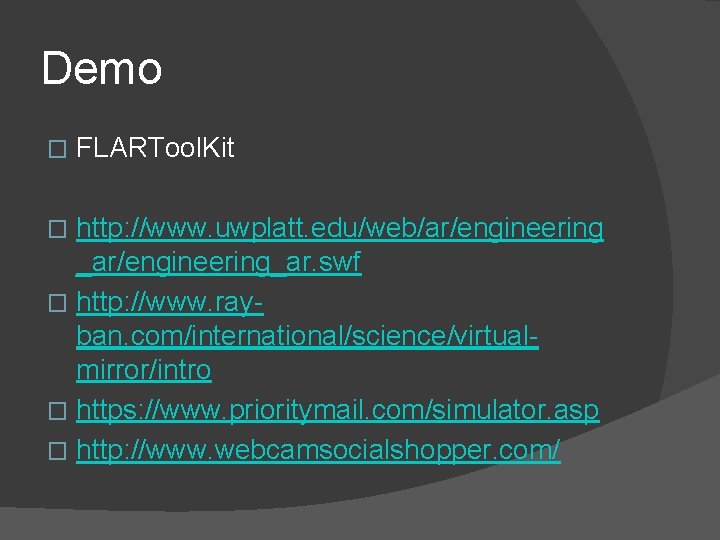
- Slides: 51
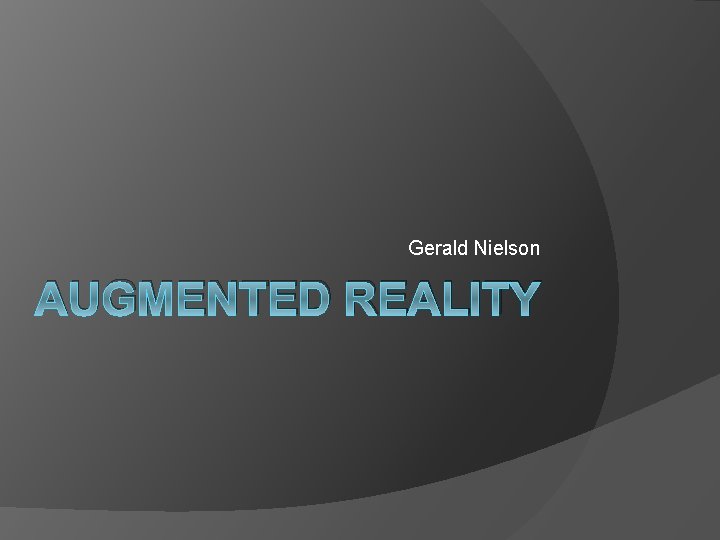
Gerald Nielson AUGMENTED REALITY
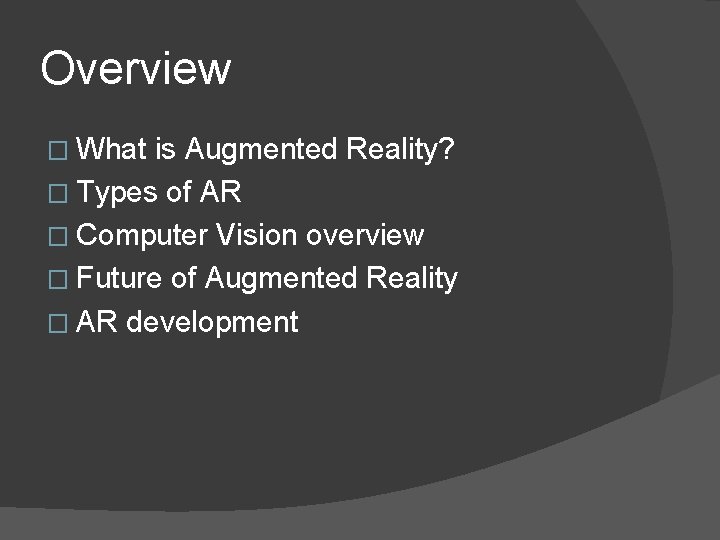
Overview � What is Augmented Reality? � Types of AR � Computer Vision overview � Future of Augmented Reality � AR development
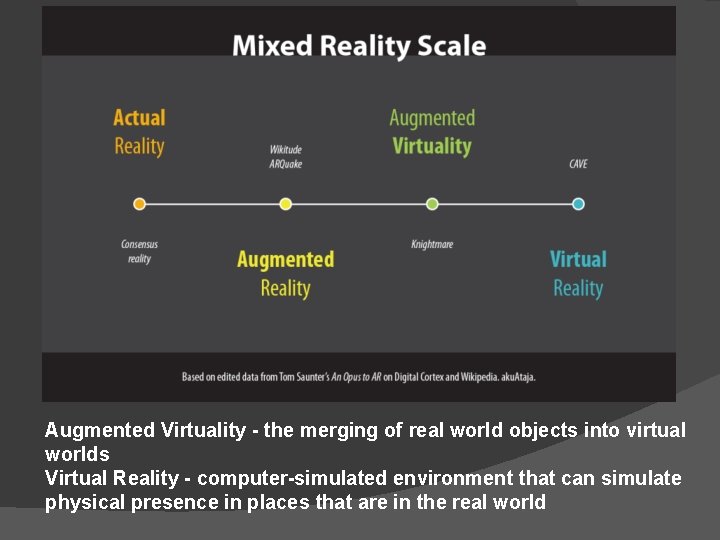
Augmented Virtuality - the merging of real world objects into virtual worlds Virtual Reality - computer-simulated environment that can simulate physical presence in places that are in the real world
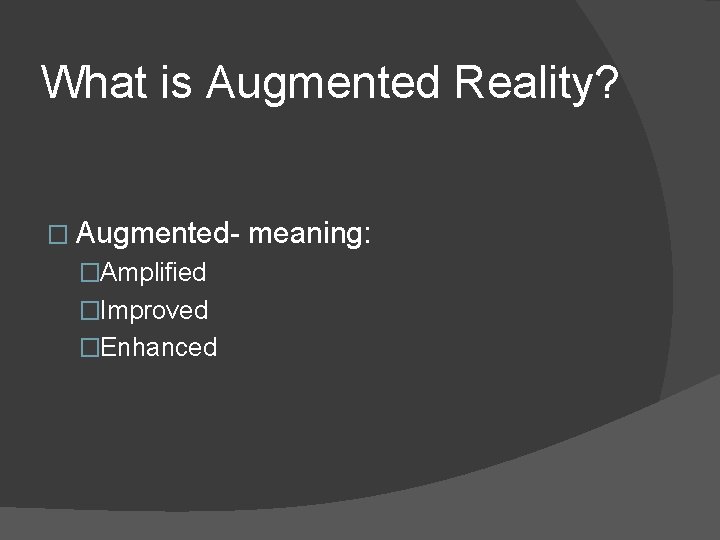
What is Augmented Reality? � Augmented�Amplified �Improved �Enhanced meaning:
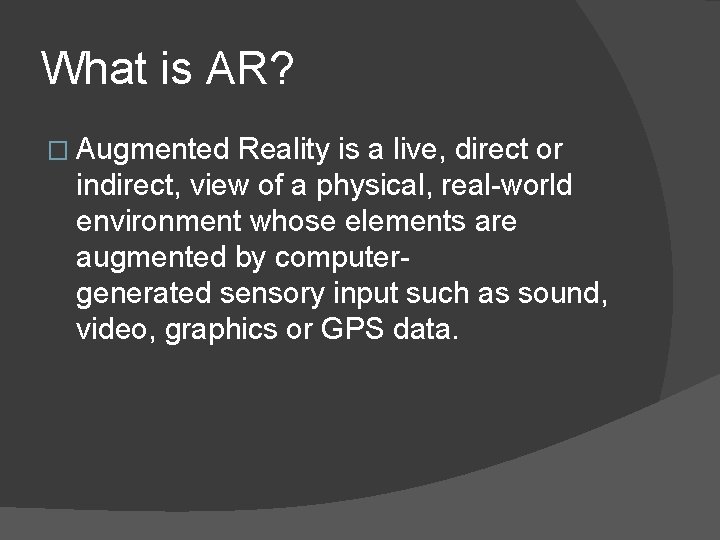
What is AR? � Augmented Reality is a live, direct or indirect, view of a physical, real-world environment whose elements are augmented by computergenerated sensory input such as sound, video, graphics or GPS data.
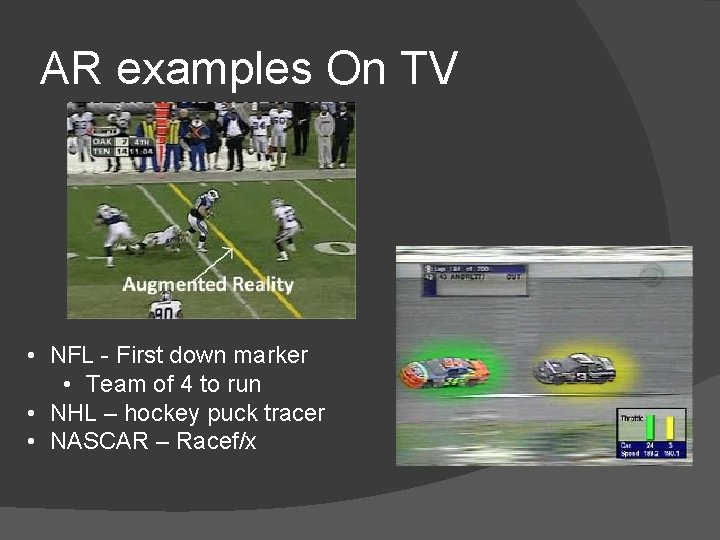
AR examples On TV • NFL - First down marker • Team of 4 to run • NHL – hockey puck tracer • NASCAR – Racef/x
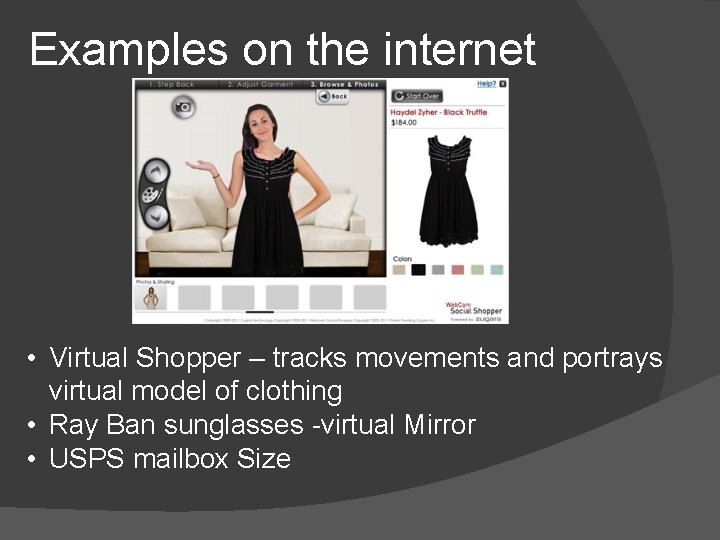
Examples on the internet • Virtual Shopper – tracks movements and portrays virtual model of clothing • Ray Ban sunglasses -virtual Mirror • USPS mailbox Size
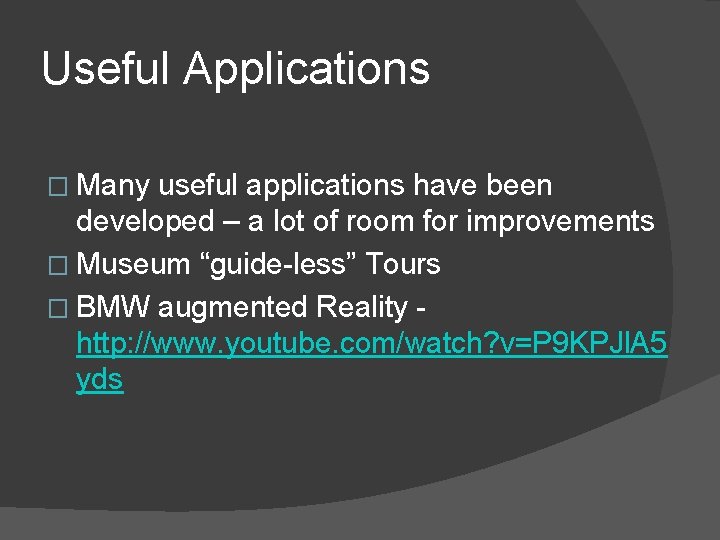
Useful Applications � Many useful applications have been developed – a lot of room for improvements � Museum “guide-less” Tours � BMW augmented Reality http: //www. youtube. com/watch? v=P 9 KPJl. A 5 yds
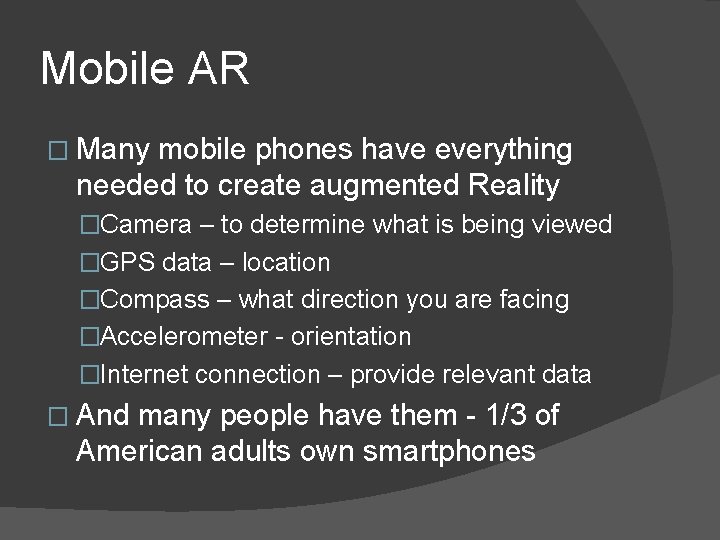
Mobile AR � Many mobile phones have everything needed to create augmented Reality �Camera – to determine what is being viewed �GPS data – location �Compass – what direction you are facing �Accelerometer - orientation �Internet connection – provide relevant data � And many people have them - 1/3 of American adults own smartphones
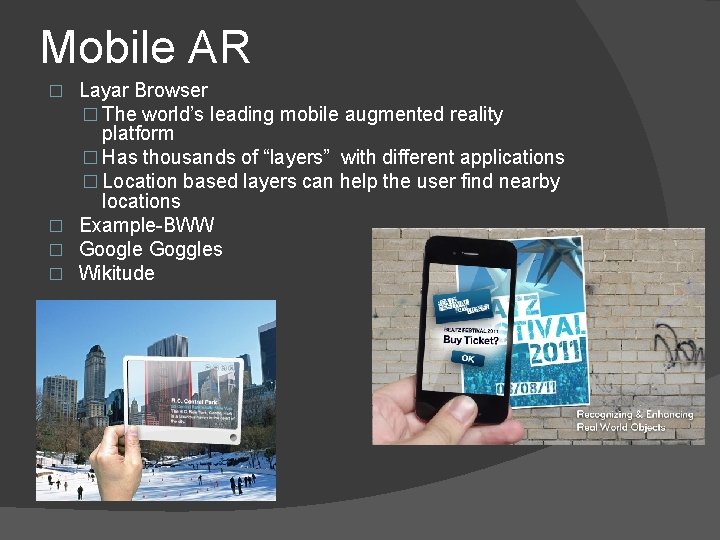
Mobile AR Layar Browser � The world’s leading mobile augmented reality platform � Has thousands of “layers” with different applications � Location based layers can help the user find nearby locations � Example-BWW � Google Goggles � Wikitude �
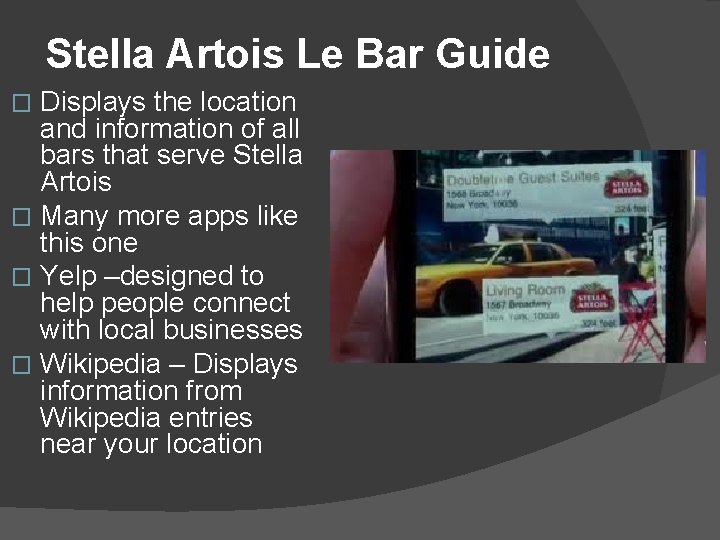
Stella Artois Le Bar Guide Displays the location and information of all bars that serve Stella Artois � Many more apps like this one � Yelp –designed to help people connect with local businesses � Wikipedia – Displays information from Wikipedia entries near your location �
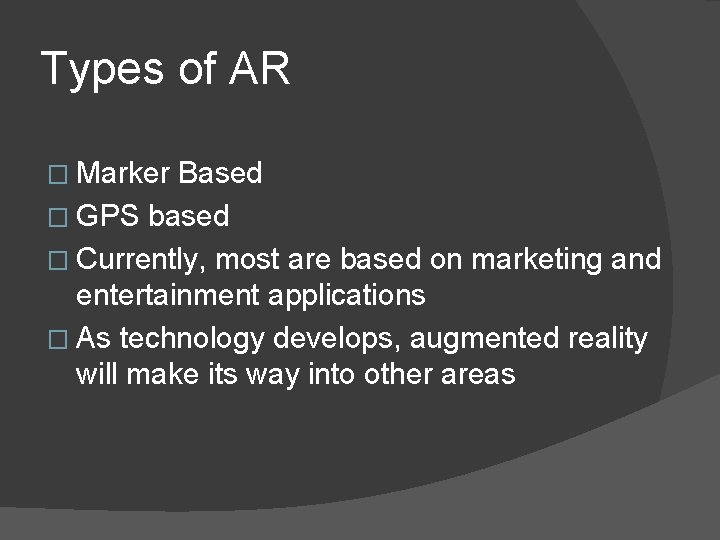
Types of AR � Marker Based � GPS based � Currently, most are based on marketing and entertainment applications � As technology develops, augmented reality will make its way into other areas
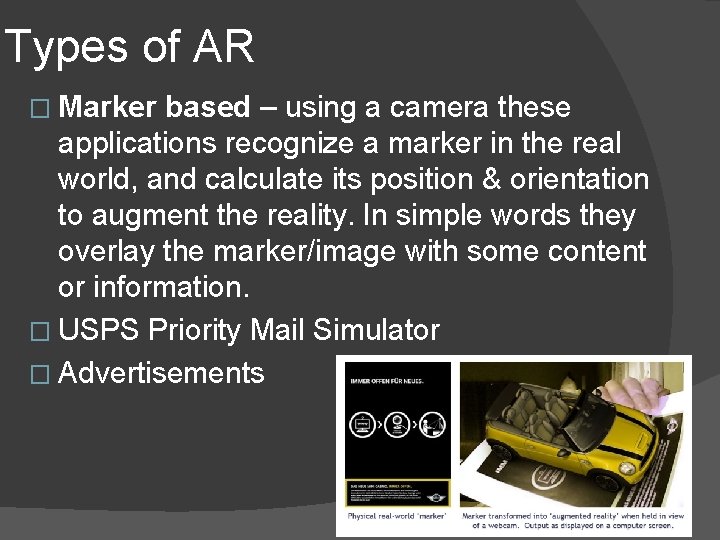
Types of AR � Marker based – using a camera these applications recognize a marker in the real world, and calculate its position & orientation to augment the reality. In simple words they overlay the marker/image with some content or information. � USPS Priority Mail Simulator � Advertisements
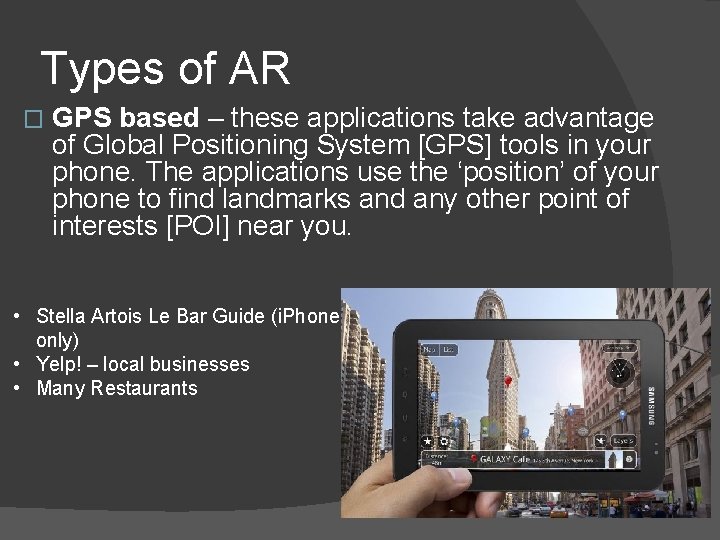
Types of AR � GPS based – these applications take advantage of Global Positioning System [GPS] tools in your phone. The applications use the ‘position’ of your phone to find landmarks and any other point of interests [POI] near you. • Stella Artois Le Bar Guide (i. Phone only) • Yelp! – local businesses • Many Restaurants
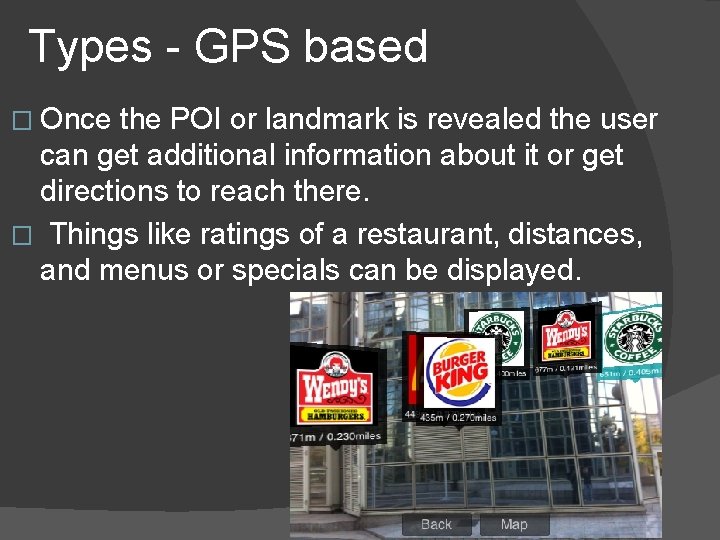
Types - GPS based � Once the POI or landmark is revealed the user can get additional information about it or get directions to reach there. � Things like ratings of a restaurant, distances, and menus or specials can be displayed.
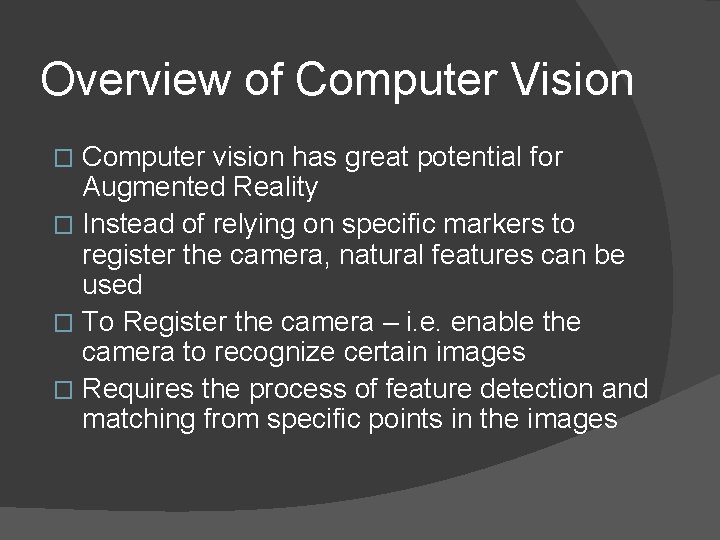
Overview of Computer Vision Computer vision has great potential for Augmented Reality � Instead of relying on specific markers to register the camera, natural features can be used � To Register the camera – i. e. enable the camera to recognize certain images � Requires the process of feature detection and matching from specific points in the images �
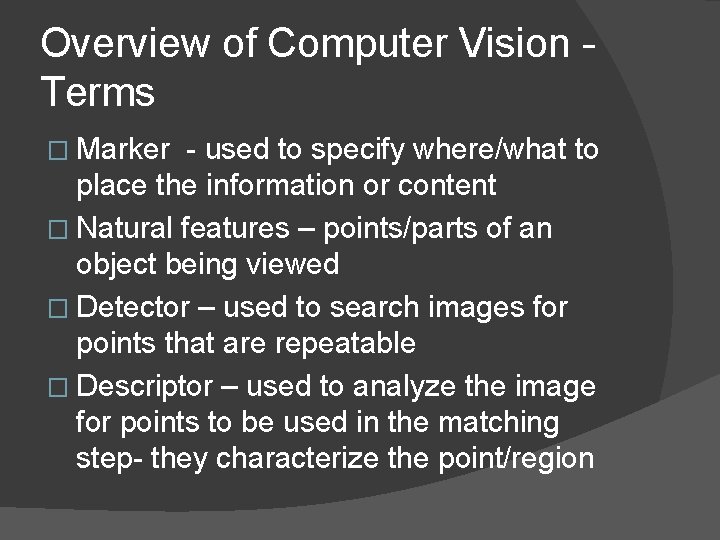
Overview of Computer Vision Terms � Marker - used to specify where/what to place the information or content � Natural features – points/parts of an object being viewed � Detector – used to search images for points that are repeatable � Descriptor – used to analyze the image for points to be used in the matching step- they characterize the point/region
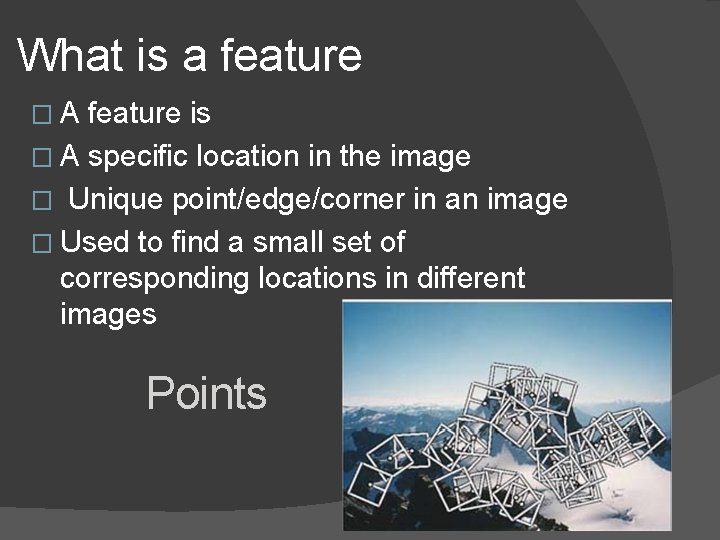
What is a feature �A feature is � A specific location in the image � Unique point/edge/corner in an image � Used to find a small set of corresponding locations in different images Points
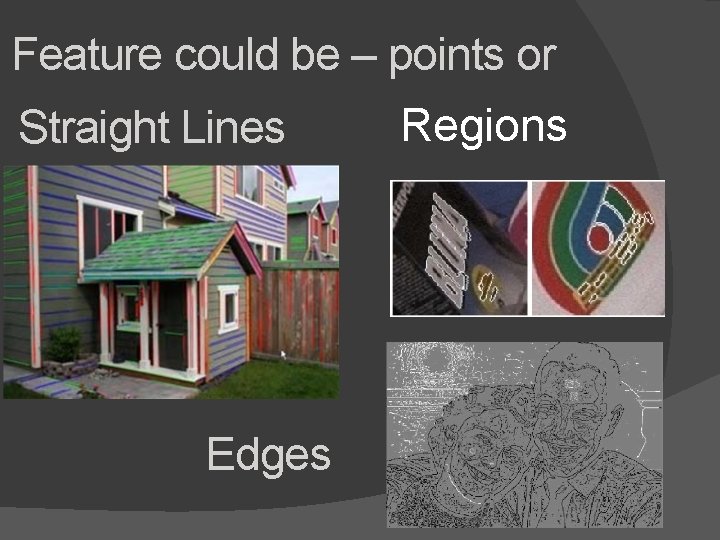
Feature could be – points or Straight Lines Edges Regions
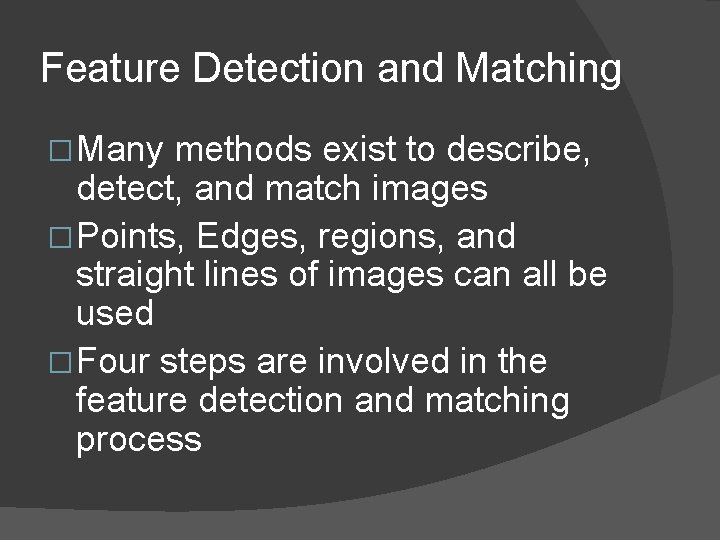
Feature Detection and Matching � Many methods exist to describe, detect, and match images � Points, Edges, regions, and straight lines of images can all be used � Four steps are involved in the feature detection and matching process
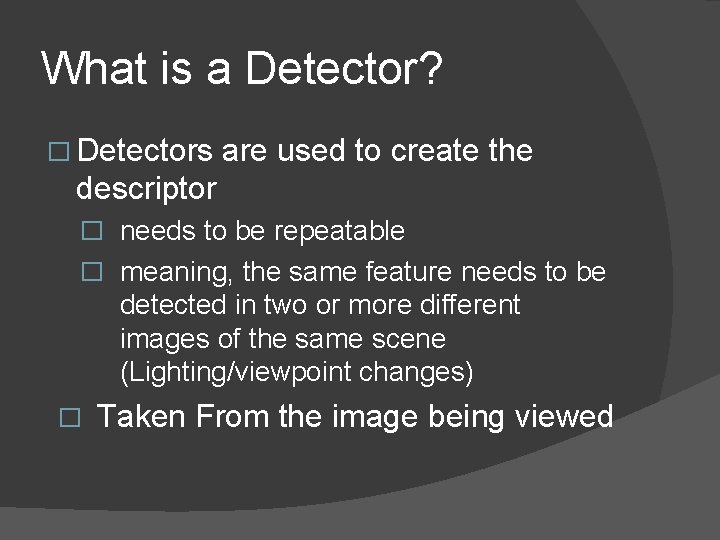
What is a Detector? � Detectors are used to create the descriptor � needs to be repeatable � meaning, the same feature needs to be detected in two or more different images of the same scene (Lighting/viewpoint changes) � Taken From the image being viewed
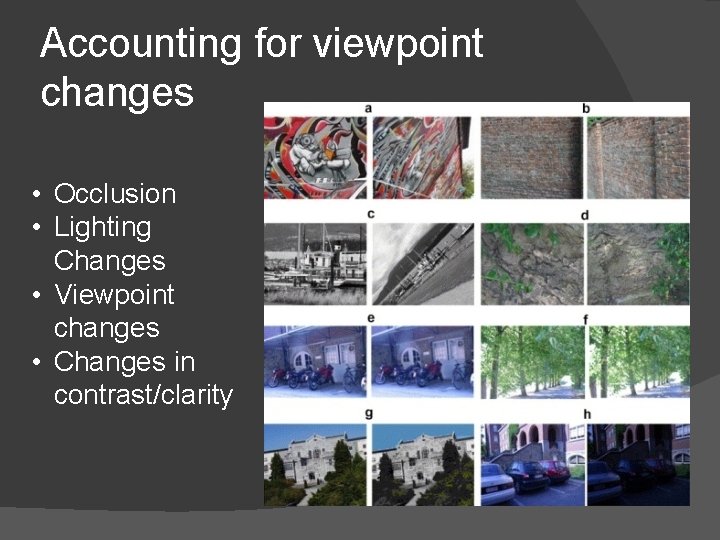
Accounting for viewpoint changes • Occlusion • Lighting Changes • Viewpoint changes • Changes in contrast/clarity
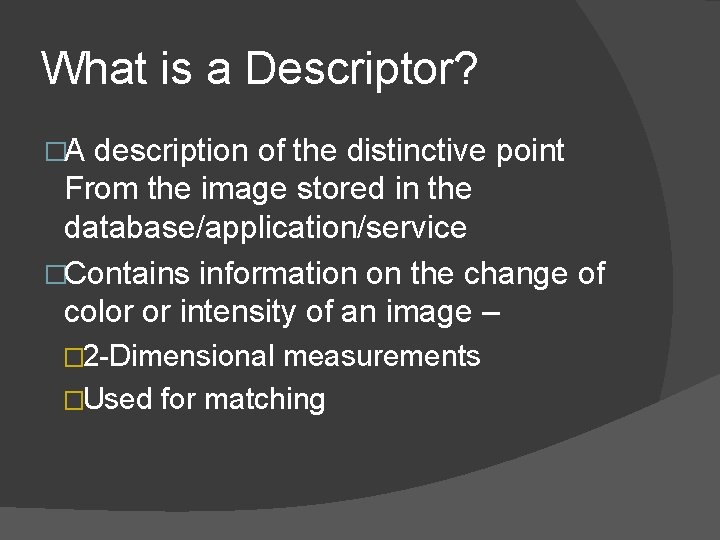
What is a Descriptor? �A description of the distinctive point From the image stored in the database/application/service �Contains information on the change of color or intensity of an image – � 2 -Dimensional measurements �Used for matching
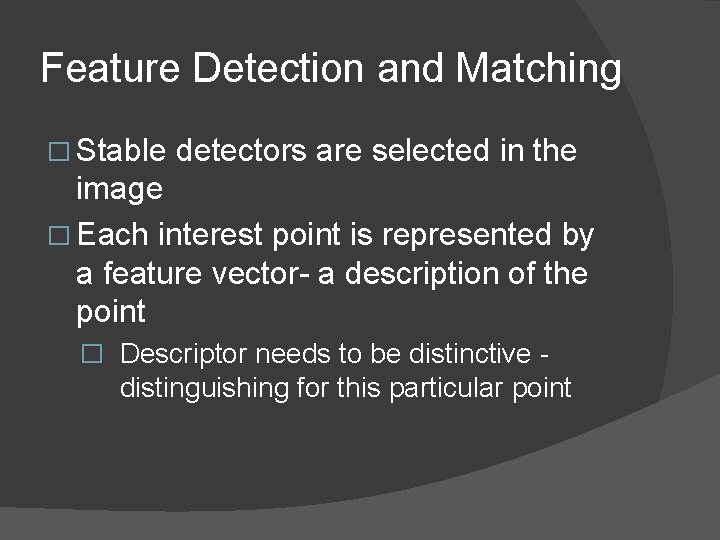
Feature Detection and Matching � Stable detectors are selected in the image � Each interest point is represented by a feature vector- a description of the point � Descriptor needs to be distinctive - distinguishing for this particular point
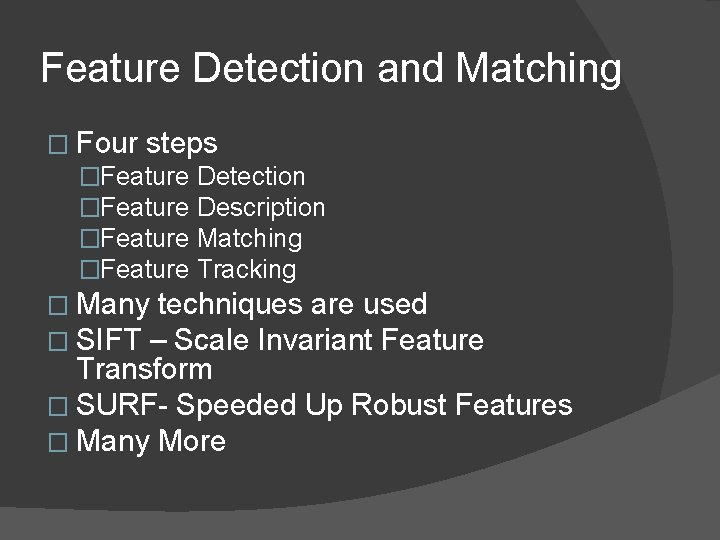
Feature Detection and Matching � Four steps �Feature Detection �Feature Description �Feature Matching �Feature Tracking � Many techniques are used � SIFT – Scale Invariant Feature Transform � SURF- Speeded Up Robust Features � Many More
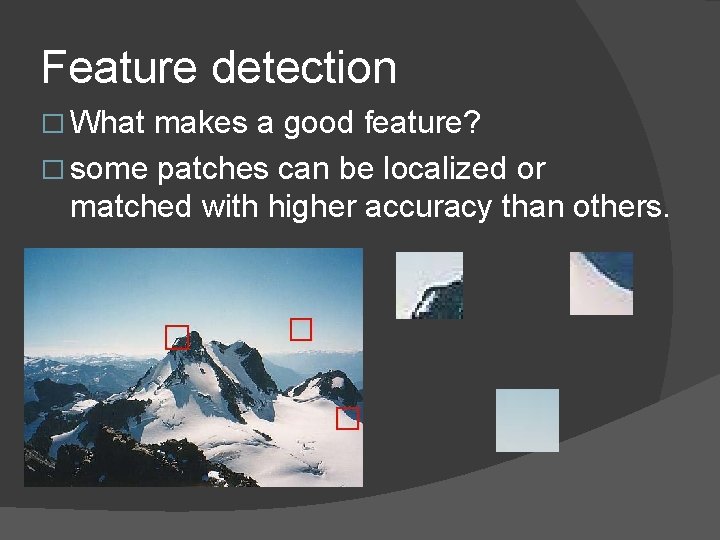
Feature detection � What makes a good feature? � some patches can be localized or matched with higher accuracy than others.
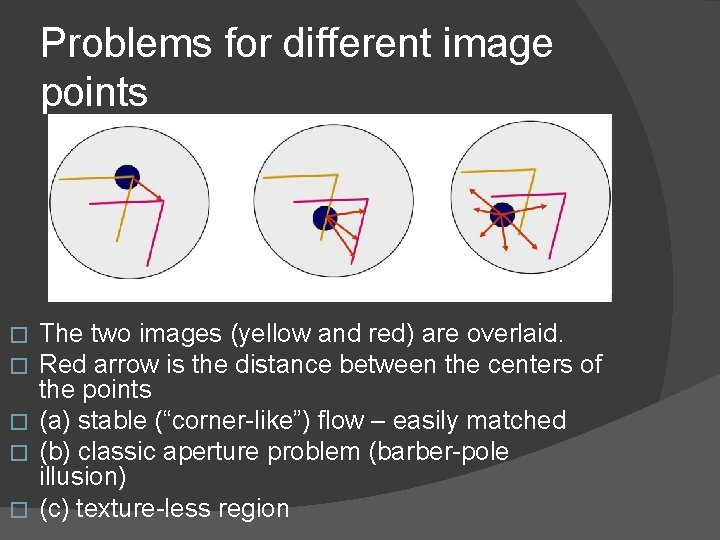
Problems for different image points The two images (yellow and red) are overlaid. Red arrow is the distance between the centers of the points � (a) stable (“corner-like”) flow – easily matched � (b) classic aperture problem (barber-pole illusion) � (c) texture-less region � �
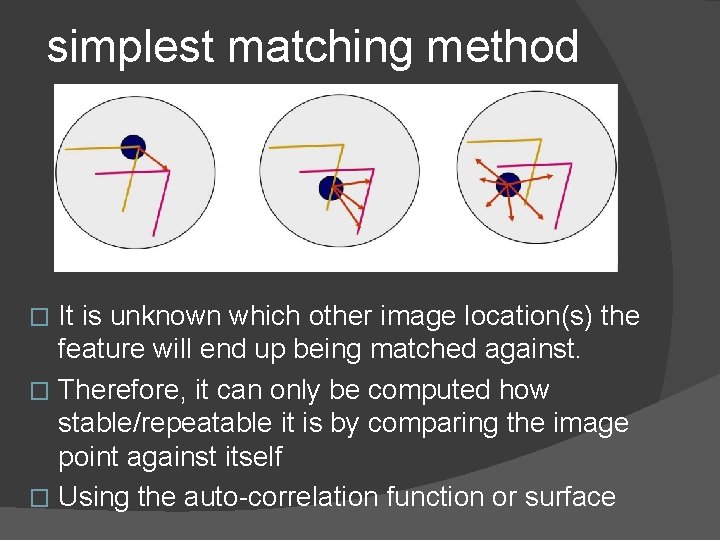
simplest matching method It is unknown which other image location(s) the feature will end up being matched against. � Therefore, it can only be computed how stable/repeatable it is by comparing the image point against itself � Using the auto-correlation function or surface �
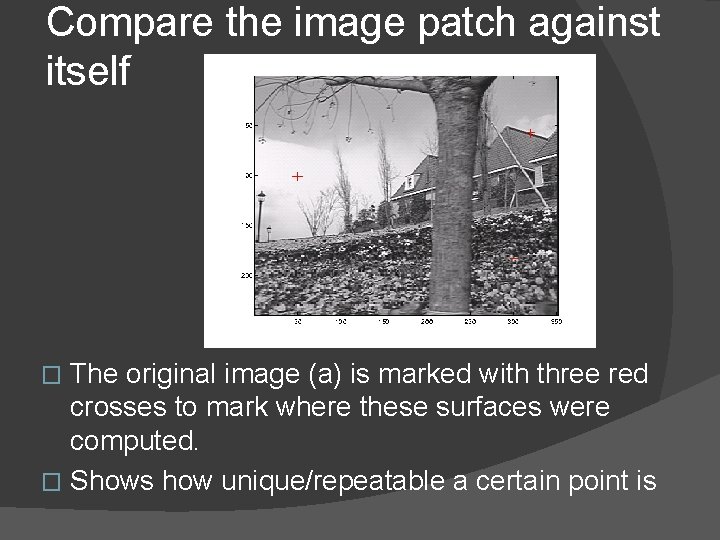
Compare the image patch against itself The original image (a) is marked with three red crosses to mark where these surfaces were computed. � Shows how unique/repeatable a certain point is �
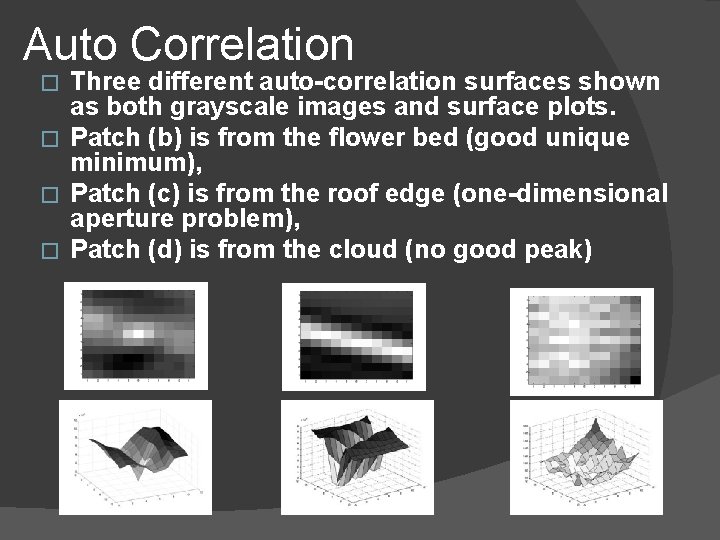
Auto Correlation Three different auto-correlation surfaces shown as both grayscale images and surface plots. � Patch (b) is from the flower bed (good unique minimum), � Patch (c) is from the roof edge (one-dimensional aperture problem), � Patch (d) is from the cloud (no good peak) �
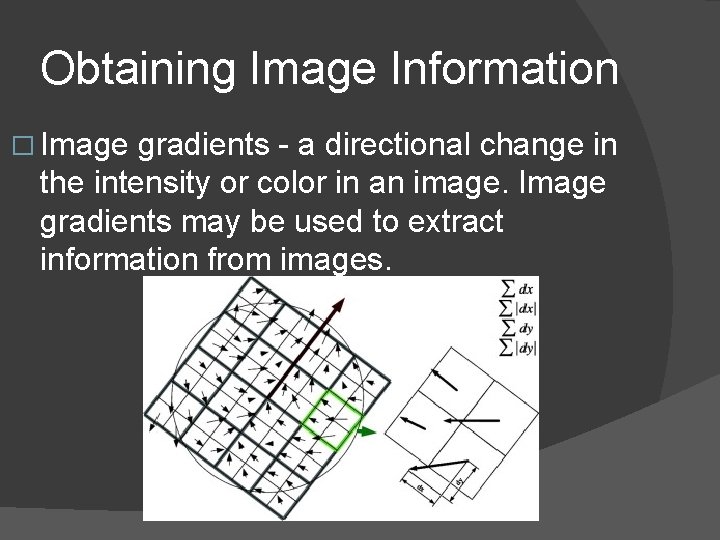
Obtaining Image Information � Image gradients - a directional change in the intensity or color in an image. Image gradients may be used to extract information from images.
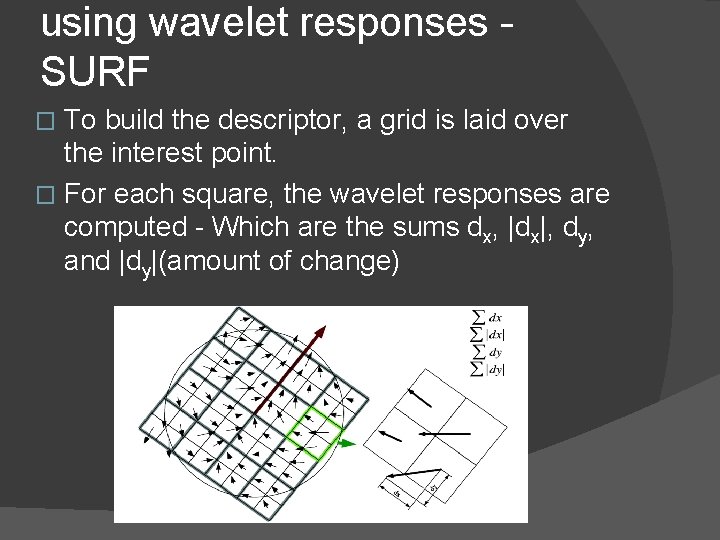
using wavelet responses SURF To build the descriptor, a grid is laid over the interest point. � For each square, the wavelet responses are computed - Which are the sums dx, |dx|, dy, and |dy|(amount of change) �
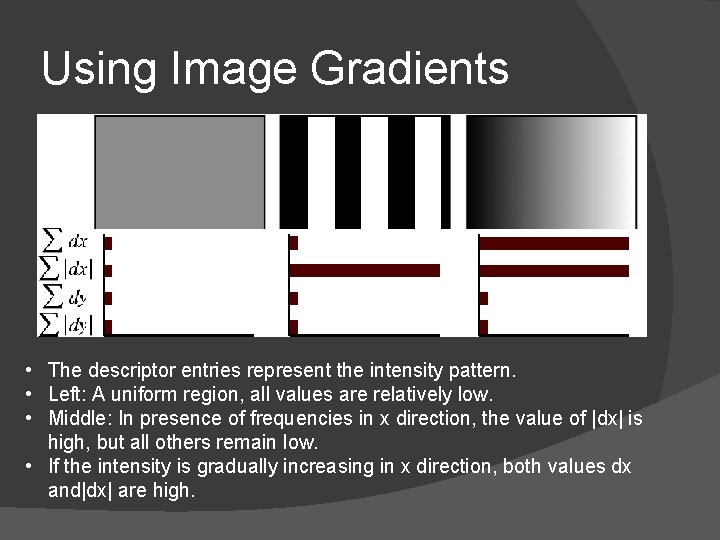
Using Image Gradients • The descriptor entries represent the intensity pattern. • Left: A uniform region, all values are relatively low. • Middle: In presence of frequencies in x direction, the value of |dx| is high, but all others remain low. • If the intensity is gradually increasing in x direction, both values dx and|dx| are high.
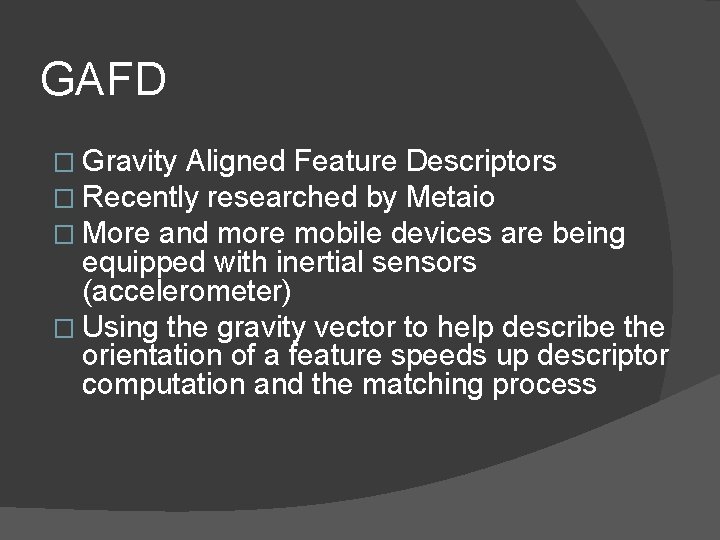
GAFD � Gravity Aligned Feature Descriptors � Recently researched by Metaio � More and more mobile devices are being equipped with inertial sensors (accelerometer) � Using the gravity vector to help describe the orientation of a feature speeds up descriptor computation and the matching process
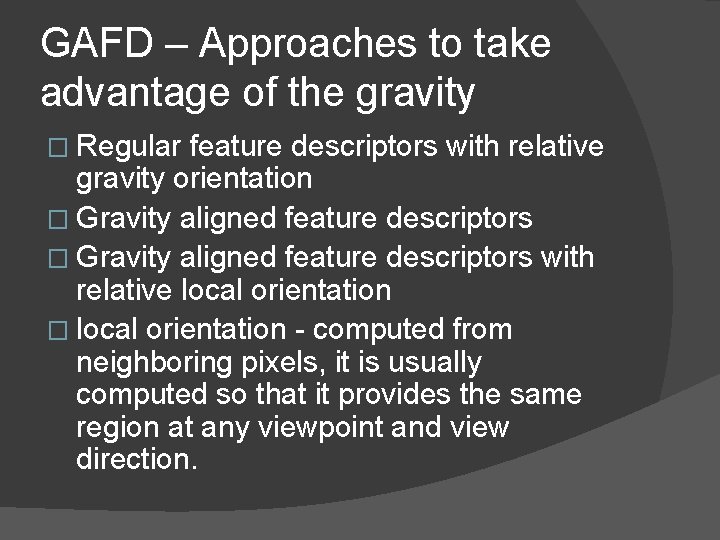
GAFD – Approaches to take advantage of the gravity � Regular feature descriptors with relative gravity orientation � Gravity aligned feature descriptors with relative local orientation � local orientation - computed from neighboring pixels, it is usually computed so that it provides the same region at any viewpoint and view direction.
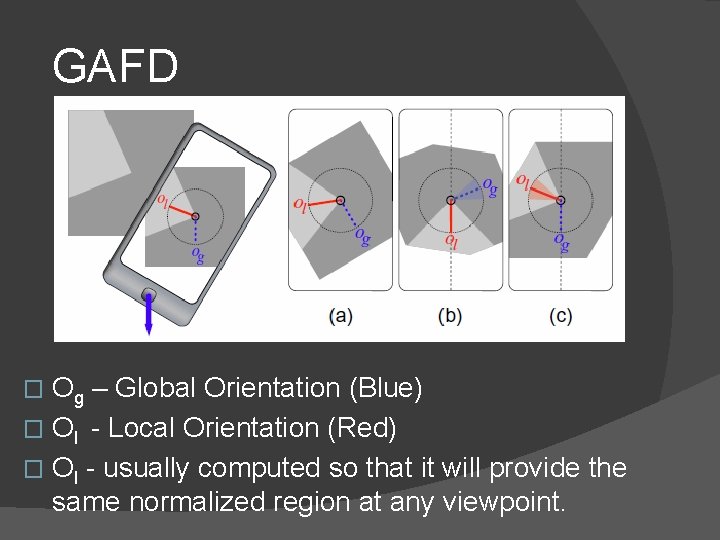
GAFD Og – Global Orientation (Blue) � Ol - Local Orientation (Red) � Ol - usually computed so that it will provide the same normalized region at any viewpoint. �
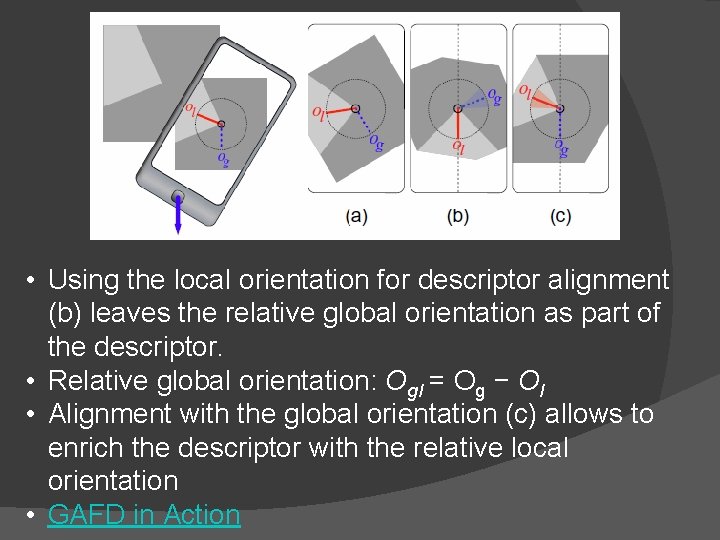
• Using the local orientation for descriptor alignment (b) leaves the relative global orientation as part of the descriptor. • Relative global orientation: Ogl = Og − Ol • Alignment with the global orientation (c) allows to enrich the descriptor with the relative local orientation • GAFD in Action
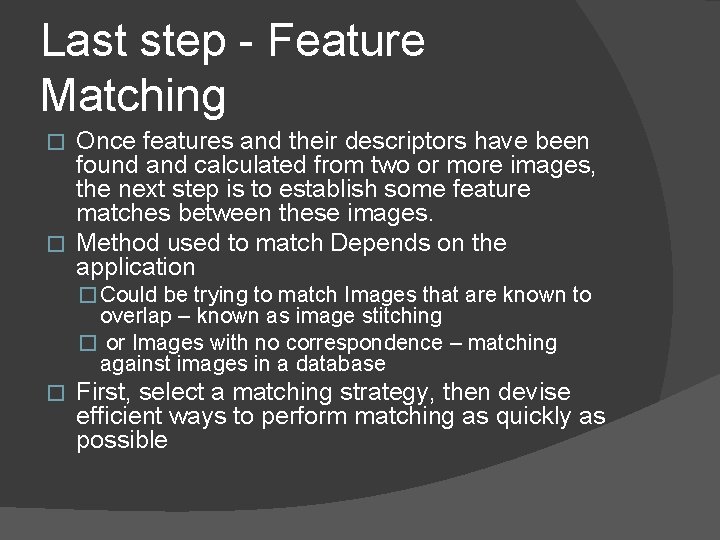
Last step - Feature Matching Once features and their descriptors have been found and calculated from two or more images, the next step is to establish some feature matches between these images. � Method used to match Depends on the application � � Could be trying to match Images that are known to overlap – known as image stitching � or Images with no correspondence – matching against images in a database � First, select a matching strategy, then devise efficient ways to perform matching as quickly as possible
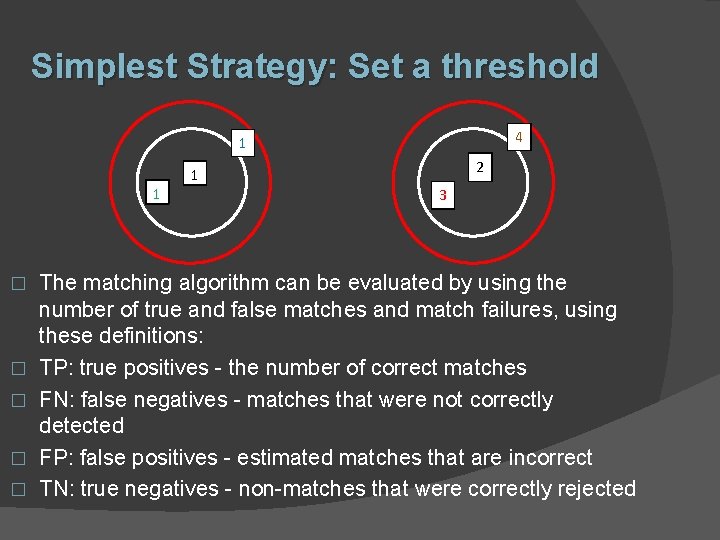
Simplest Strategy: Set a threshold 4 1 1 � � � 2 1 3 The matching algorithm can be evaluated by using the number of true and false matches and match failures, using these definitions: TP: true positives - the number of correct matches FN: false negatives - matches that were not correctly detected FP: false positives - estimated matches that are incorrect TN: true negatives - non-matches that were correctly rejected
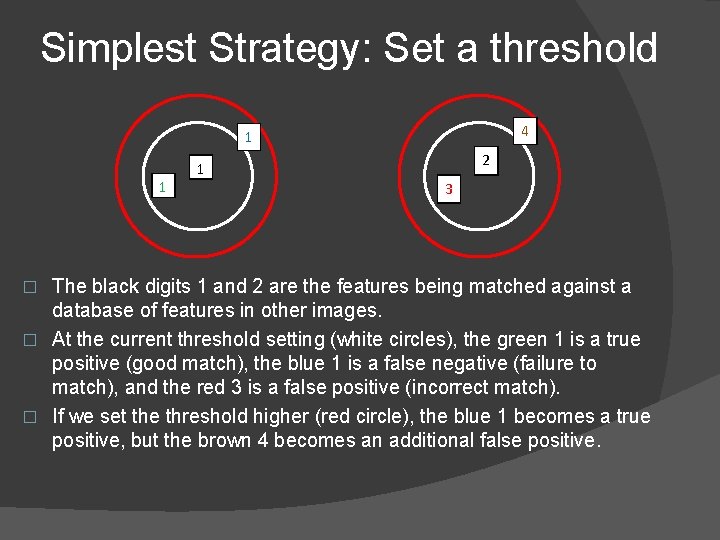
Simplest Strategy: Set a threshold 4 1 1 2 1 3 The black digits 1 and 2 are the features being matched against a database of features in other images. � At the current threshold setting (white circles), the green 1 is a true positive (good match), the blue 1 is a false negative (failure to match), and the red 3 is a false positive (incorrect match). � If we set the threshold higher (red circle), the blue 1 becomes a true positive, but the brown 4 becomes an additional false positive. �
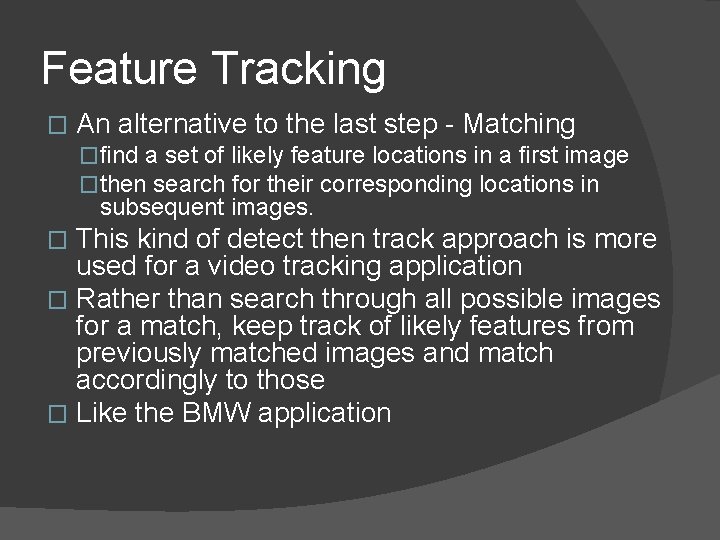
Feature Tracking � An alternative to the last step - Matching �find a set of likely feature locations in a first image �then search for their corresponding locations in subsequent images. This kind of detect then track approach is more used for a video tracking application � Rather than search through all possible images for a match, keep track of likely features from previously matched images and match accordingly to those � Like the BMW application �
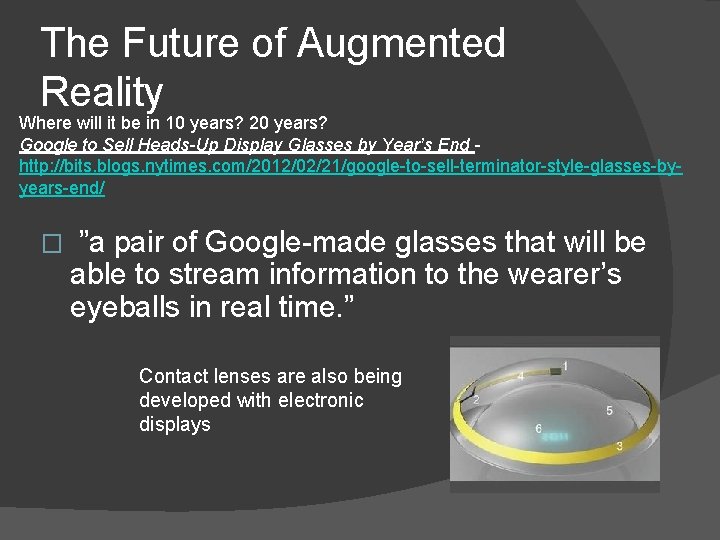
The Future of Augmented Reality Where will it be in 10 years? 20 years? Google to Sell Heads-Up Display Glasses by Year’s End http: //bits. blogs. nytimes. com/2012/02/21/google-to-sell-terminator-style-glasses-byyears-end/ � ”a pair of Google-made glasses that will be able to stream information to the wearer’s eyeballs in real time. ” Contact lenses are also being developed with electronic displays
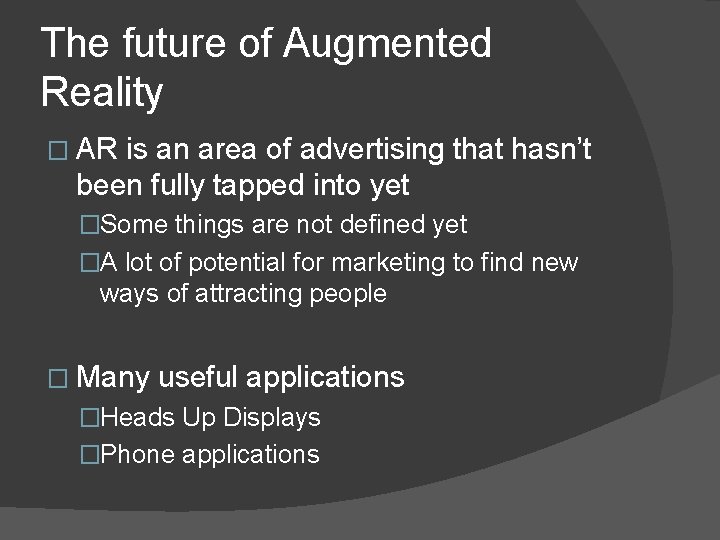
The future of Augmented Reality � AR is an area of advertising that hasn’t been fully tapped into yet �Some things are not defined yet �A lot of potential for marketing to find new ways of attracting people � Many useful applications �Heads Up Displays �Phone applications
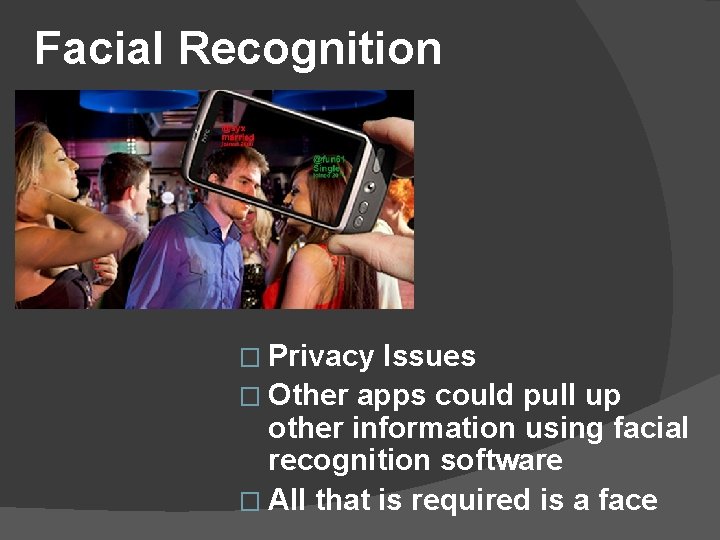
Facial Recognition � Privacy Issues � Other apps could pull up other information using facial recognition software � All that is required is a face
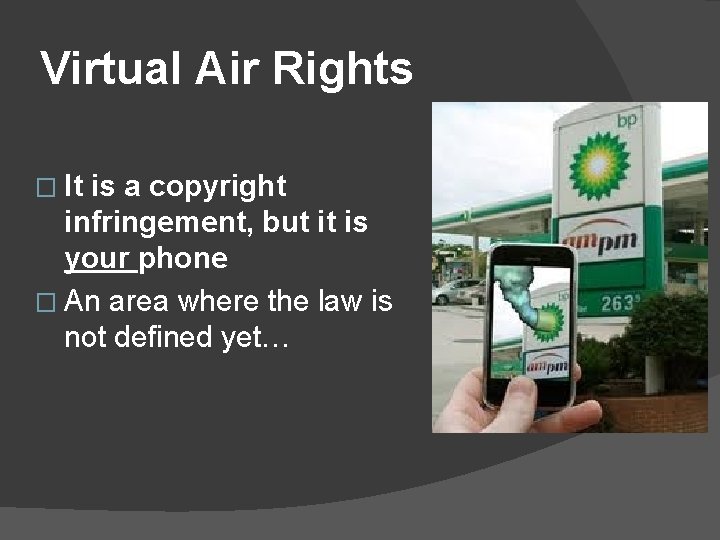
Virtual Air Rights � It is a copyright infringement, but it is your phone � An area where the law is not defined yet…
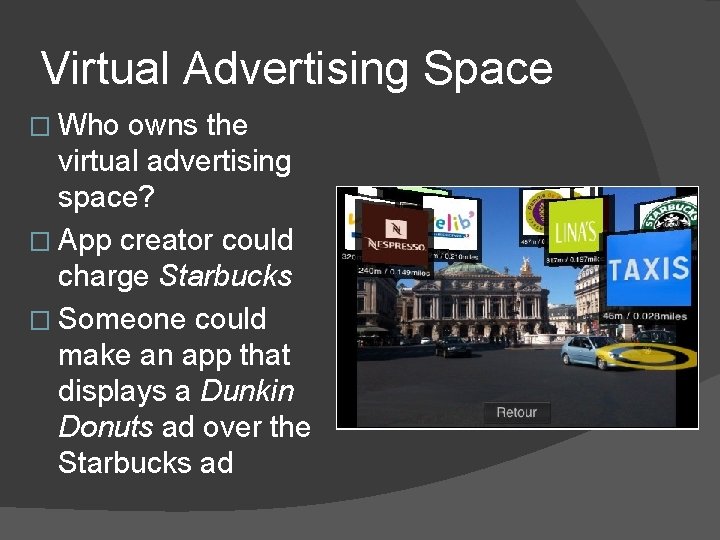
Virtual Advertising Space � Who owns the virtual advertising space? � App creator could charge Starbucks � Someone could make an app that displays a Dunkin Donuts ad over the Starbucks ad
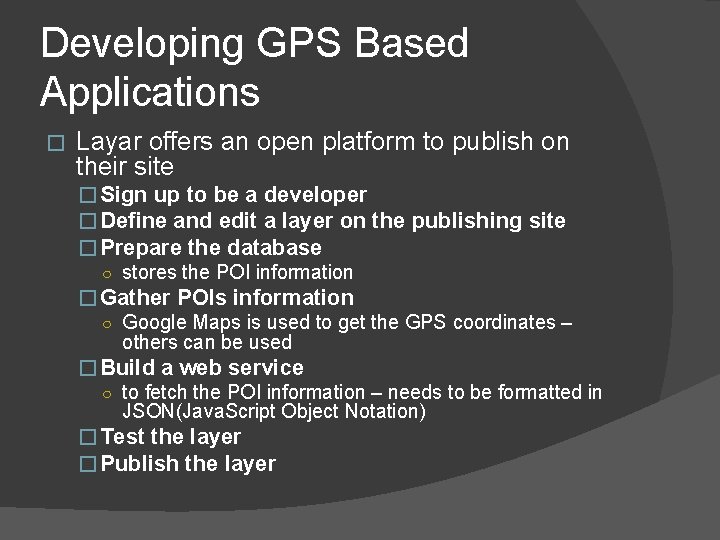
Developing GPS Based Applications � Layar offers an open platform to publish on their site � Sign up to be a developer � Define and edit a layer on the publishing site � Prepare the database ○ stores the POI information � Gather POIs information ○ Google Maps is used to get the GPS coordinates – others can be used � Build a web service ○ to fetch the POI information – needs to be formatted in JSON(Java. Script Object Notation) � Test the layer � Publish the layer
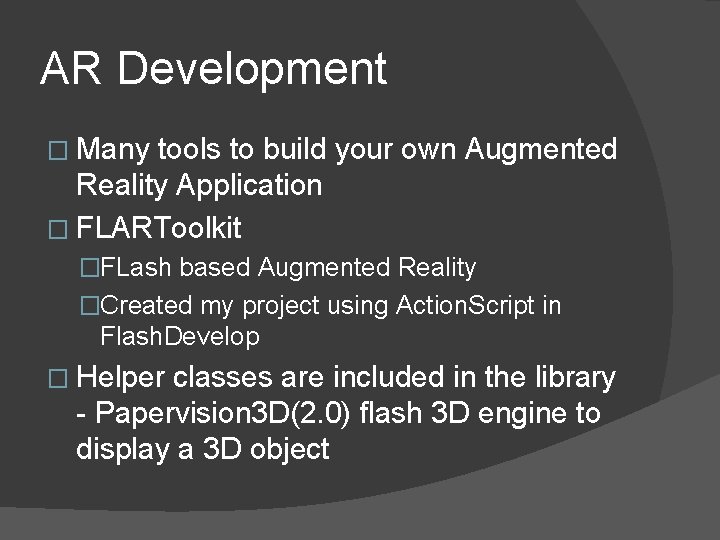
AR Development � Many tools to build your own Augmented Reality Application � FLARToolkit �FLash based Augmented Reality �Created my project using Action. Script in Flash. Develop � Helper classes are included in the library - Papervision 3 D(2. 0) flash 3 D engine to display a 3 D object
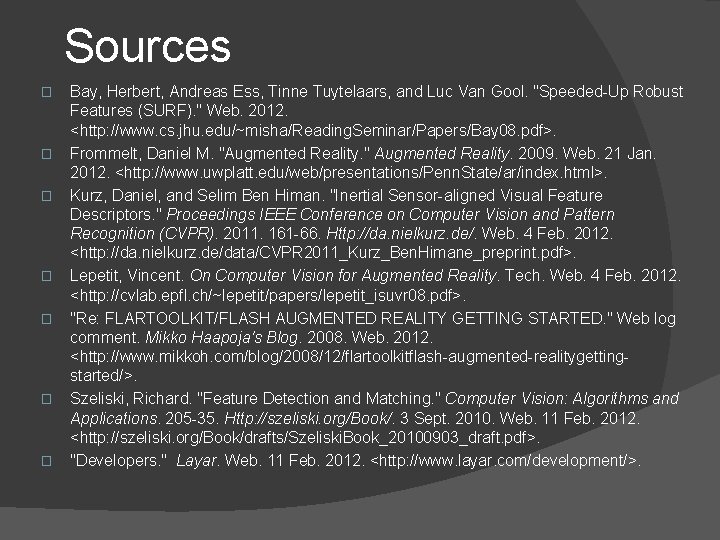
Sources � � � � Bay, Herbert, Andreas Ess, Tinne Tuytelaars, and Luc Van Gool. "Speeded-Up Robust Features (SURF). " Web. 2012. <http: //www. cs. jhu. edu/~misha/Reading. Seminar/Papers/Bay 08. pdf>. Frommelt, Daniel M. "Augmented Reality. " Augmented Reality. 2009. Web. 21 Jan. 2012. <http: //www. uwplatt. edu/web/presentations/Penn. State/ar/index. html>. Kurz, Daniel, and Selim Ben Himan. "Inertial Sensor-aligned Visual Feature Descriptors. " Proceedings IEEE Conference on Computer Vision and Pattern Recognition (CVPR). 2011. 161 -66. Http: //da. nielkurz. de/. Web. 4 Feb. 2012. <http: //da. nielkurz. de/data/CVPR 2011_Kurz_Ben. Himane_preprint. pdf>. Lepetit, Vincent. On Computer Vision for Augmented Reality. Tech. Web. 4 Feb. 2012. <http: //cvlab. epfl. ch/~lepetit/papers/lepetit_isuvr 08. pdf>. "Re: FLARTOOLKIT/FLASH AUGMENTED REALITY GETTING STARTED. " Web log comment. Mikko Haapoja's Blog. 2008. Web. 2012. <http: //www. mikkoh. com/blog/2008/12/flartoolkitflash-augmented-realitygettingstarted/>. Szeliski, Richard. "Feature Detection and Matching. " Computer Vision: Algorithms and Applications. 205 -35. Http: //szeliski. org/Book/. 3 Sept. 2010. Web. 11 Feb. 2012. <http: //szeliski. org/Book/drafts/Szeliski. Book_20100903_draft. pdf>. "Developers. " Layar. Web. 11 Feb. 2012. <http: //www. layar. com/development/>.
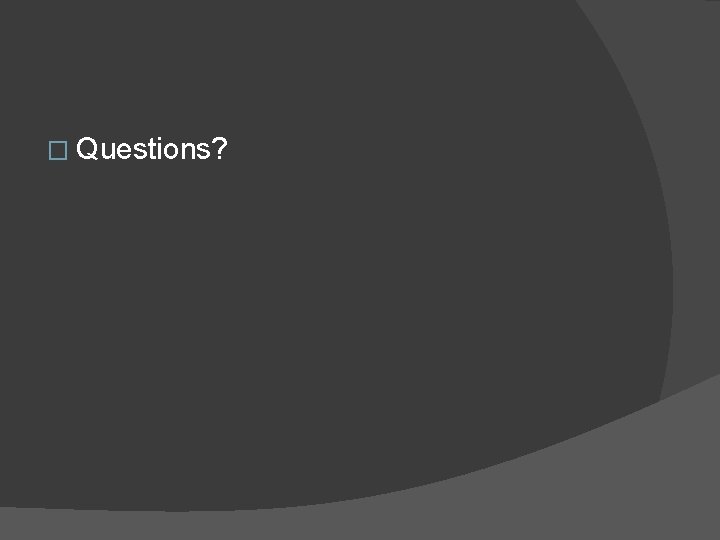
� Questions?
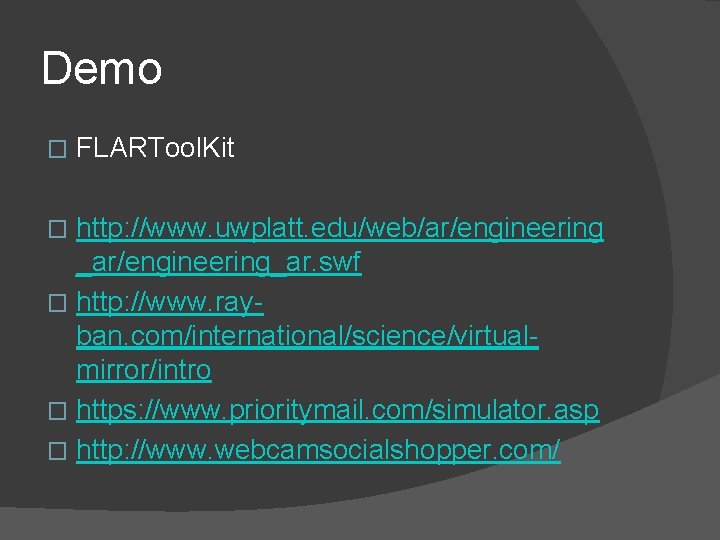
Demo � FLARTool. Kit http: //www. uwplatt. edu/web/ar/engineering _ar/engineering_ar. swf � http: //www. rayban. com/international/science/virtualmirror/intro � https: //www. prioritymail. com/simulator. asp � http: //www. webcamsocialshopper. com/ �
Augmented reality user experience
Augmented reality: principles and practice
Augmented reality big data
Augmented reality final year projects
Bayer augmented reality
Augmented reality
Pengertian augmented reality
Multi user augmented reality
Www.aurasma.com
What is augmented reality
Make augmented reality business card
Ricky tsui
Augmented reality table
Augmented reality landscaping app
Virtual reality remote maintenance
What is augmented reality
Create augmented reality poster
Microsoft augmented reality for students
Ear-ar: indoor acoustic augmented reality on earphones
Use case diagram for augmented reality
Ionic augmented reality
Gerald kador folkvord
Gerald coley
Gerald levey and mark newman
Gerald biedermann
Dr gerald saldanha
Gerald marchewka
They say i say by gerald graff and cathy birkenstein
Gerald kemmer
Gerald nunn
Marie-theres himmler
Gerald biedermann
Gerald levey and mark newman
Gerald ehgartner
An inspector calls characters
Gerald levey and mark newman
Gerald drucker
Gerald deimel
Data fabrication definition
Gerald f kein
Gerald süchting
Gerald weisfogel
Gerald durrell madagascar
Gerald ford
Hmm gerald
Donald + gerald = robert
Magnesium ammonium phosphate stones
Rancangan kerajaan melalui perang saraf
Sir william golding
Donald + gerald = robert solution
Gerald shahu
Netscape composer download