Frailty Assessment in Hospitalized Older Adults using the
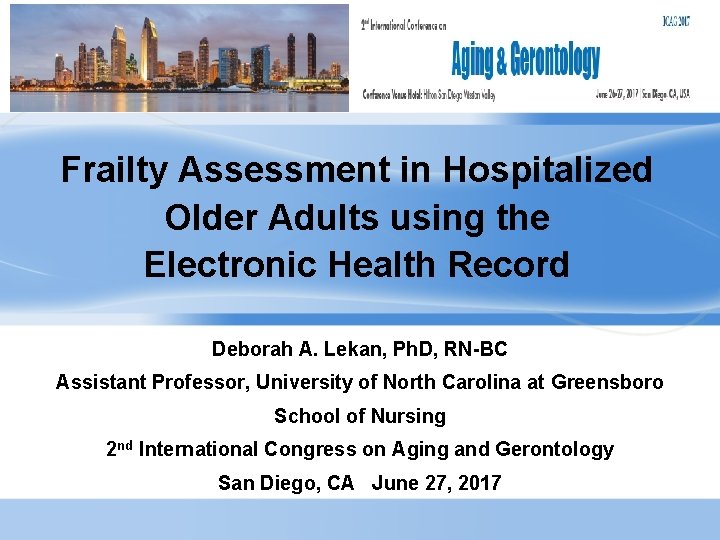
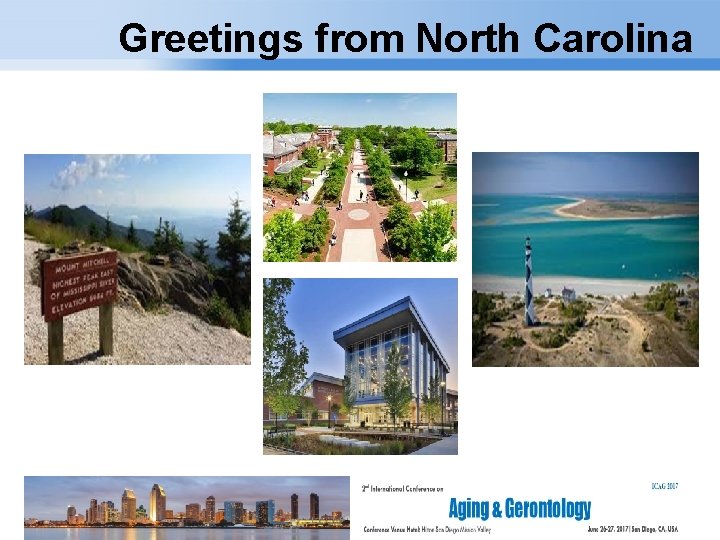
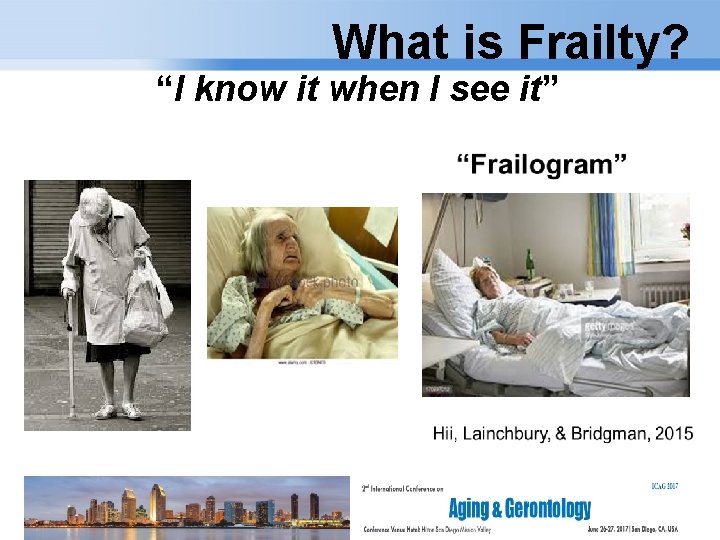
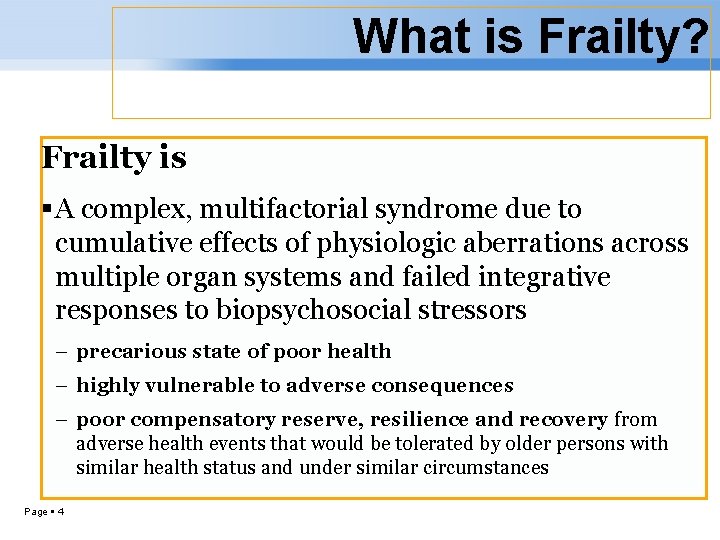
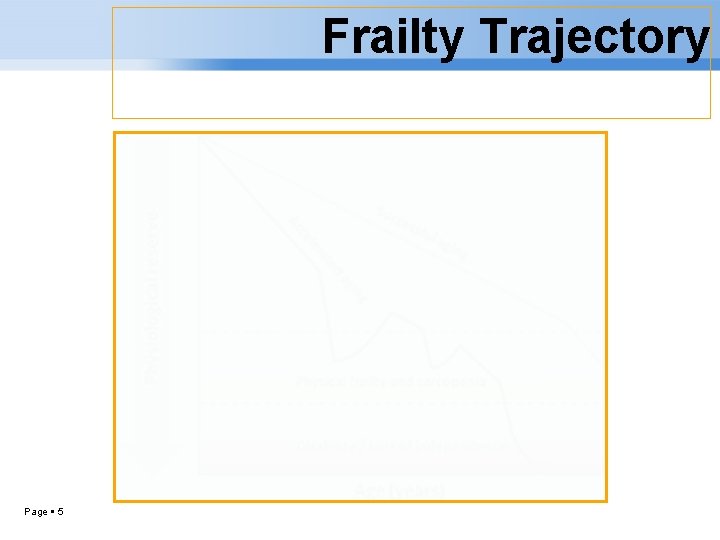
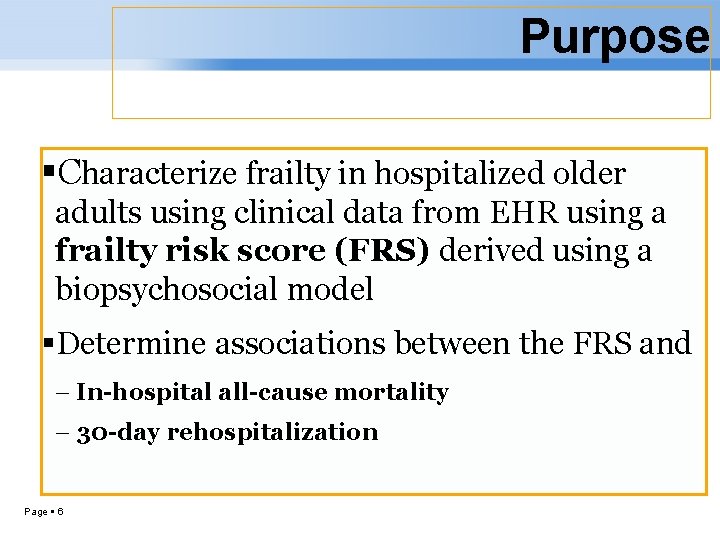
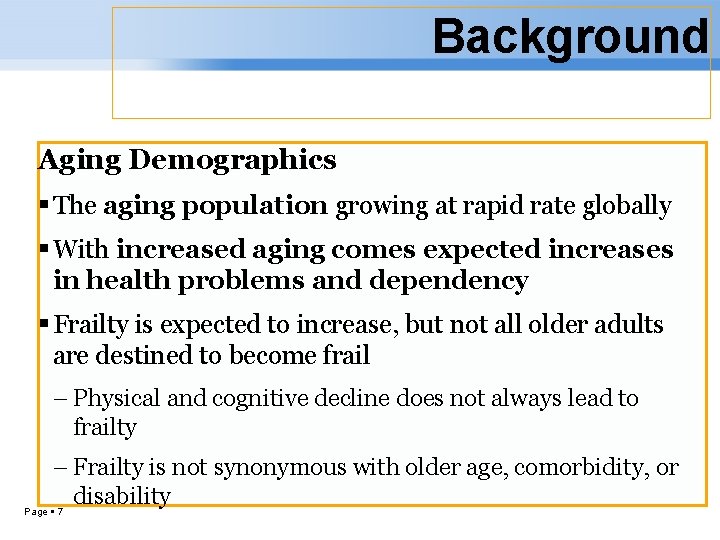
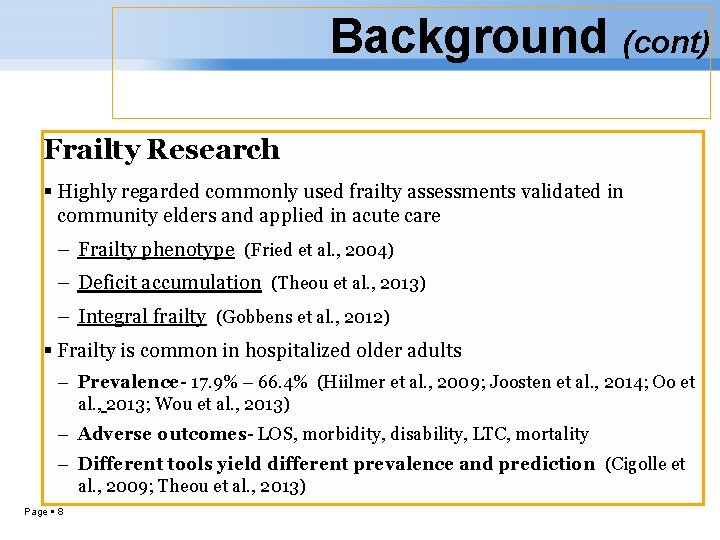
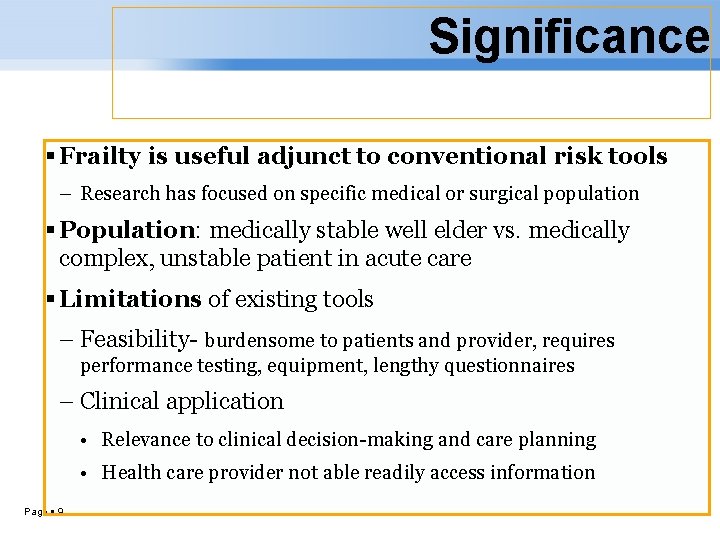
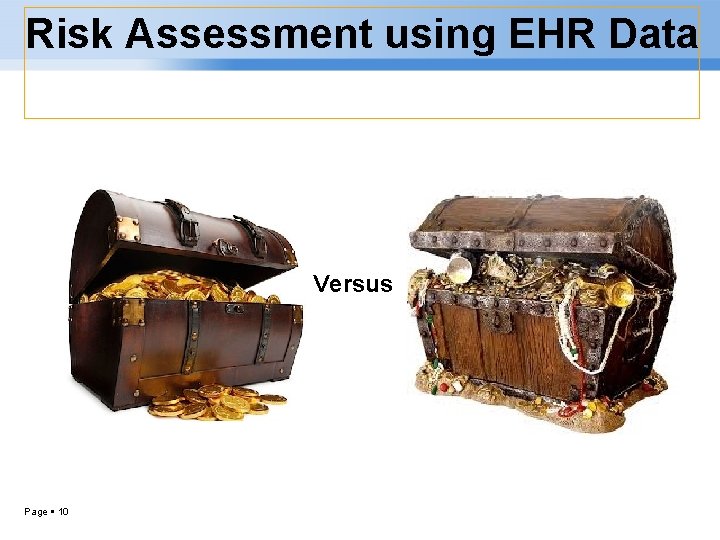
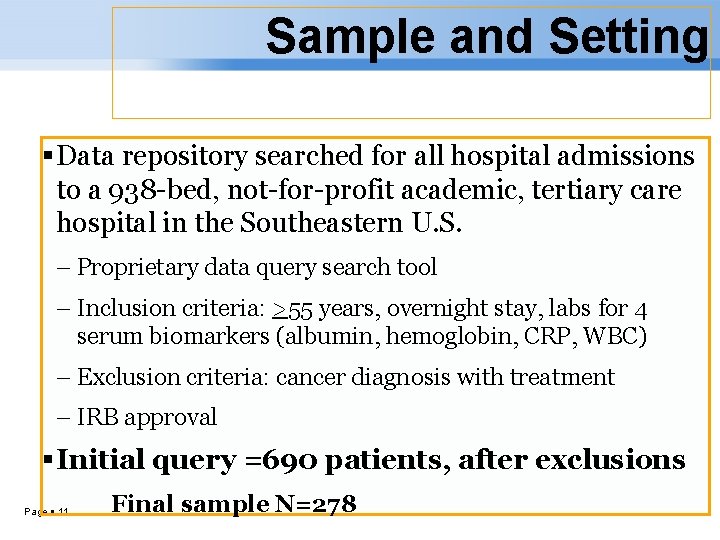
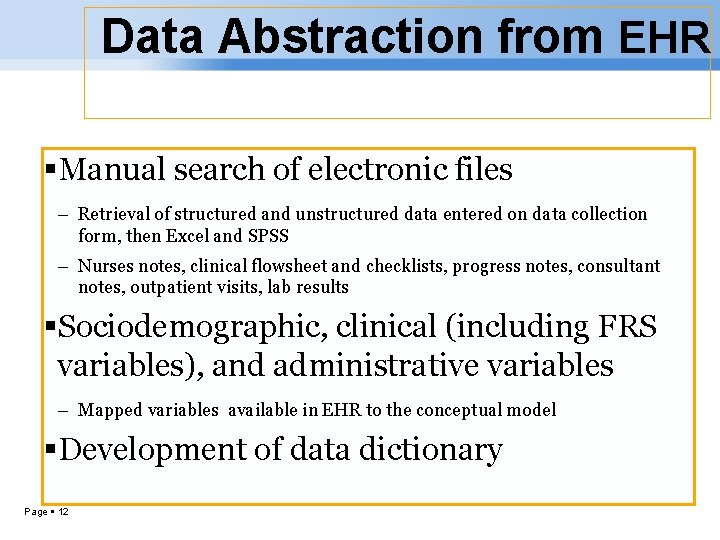
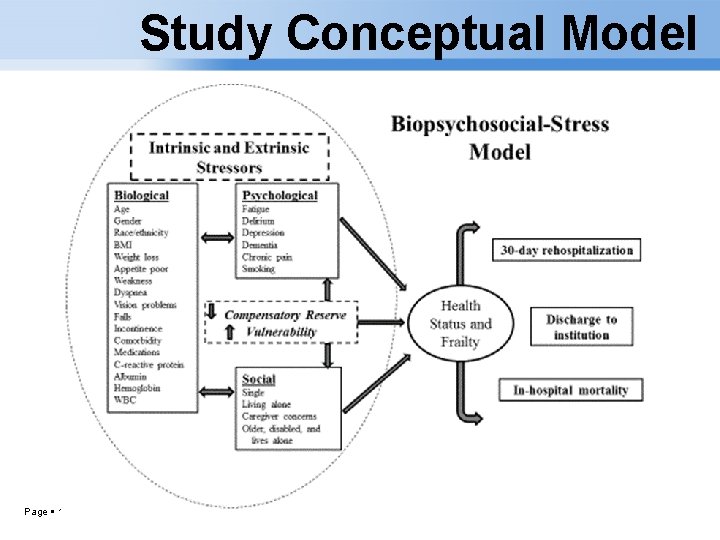
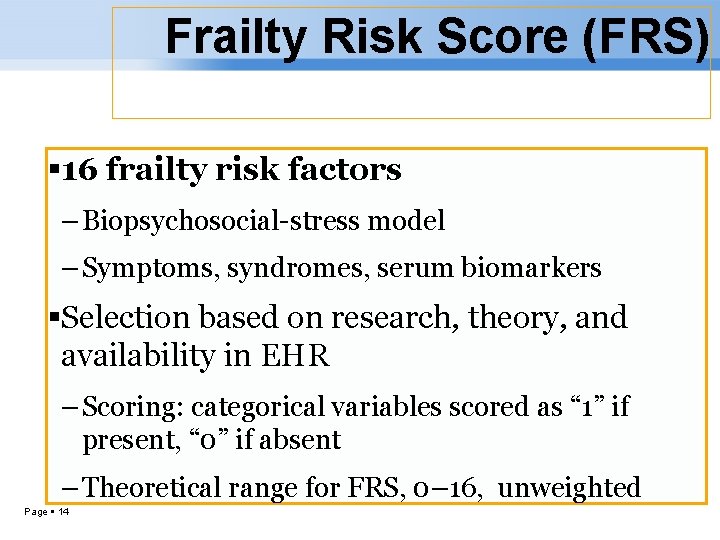
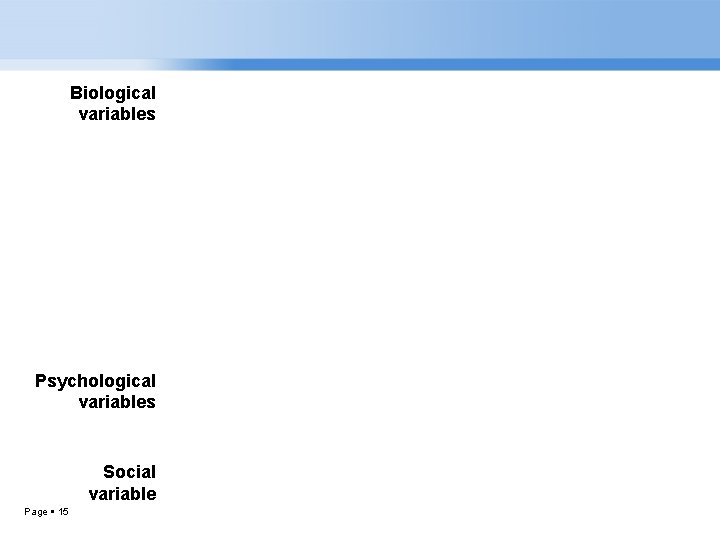
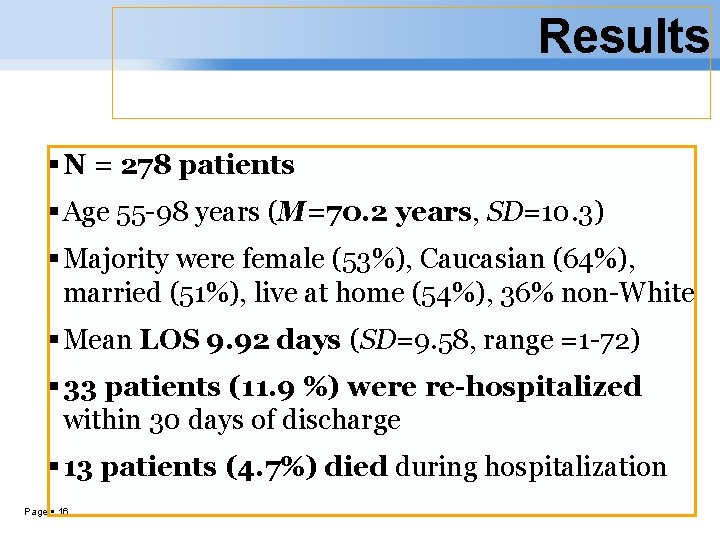
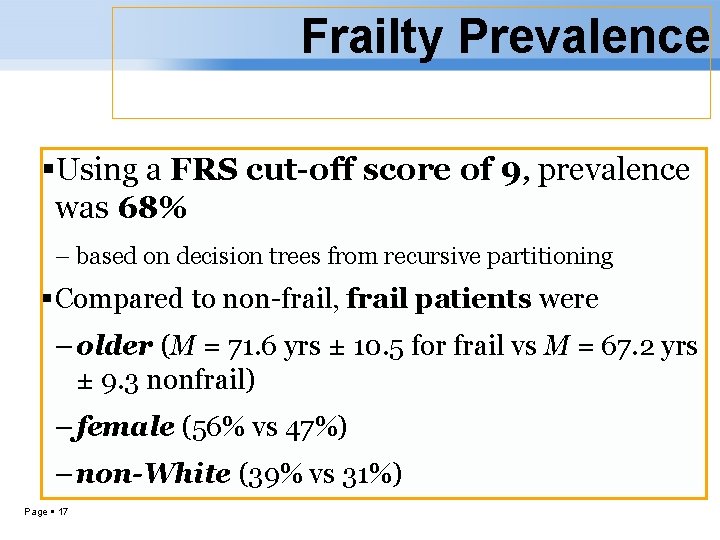
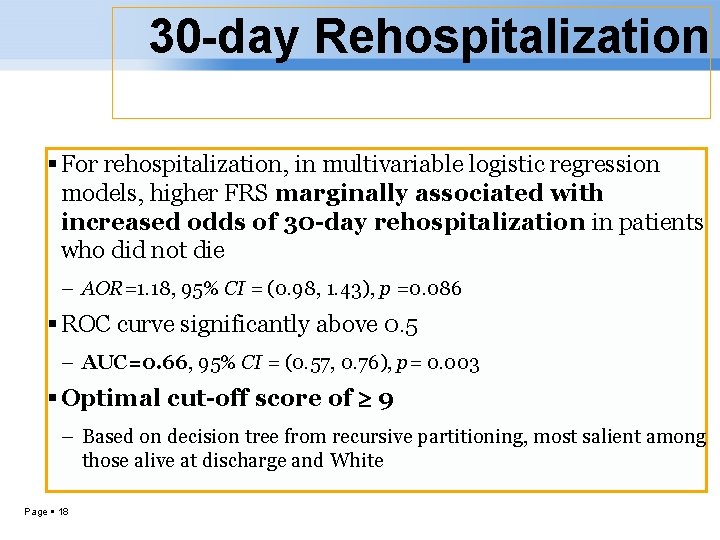
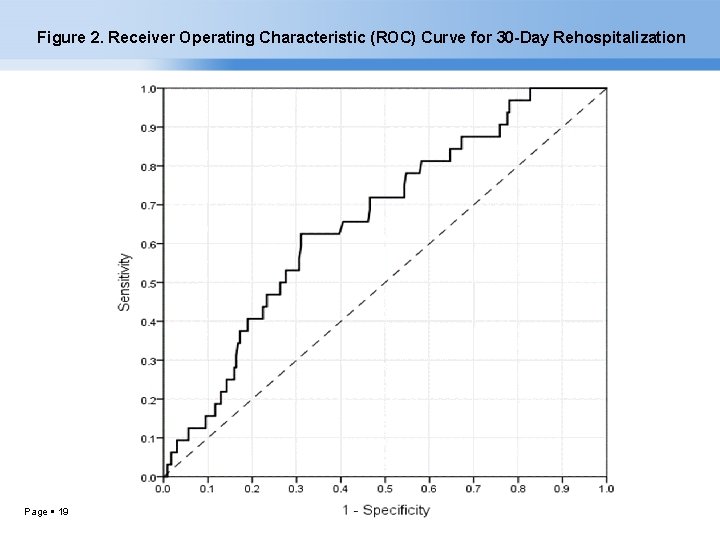
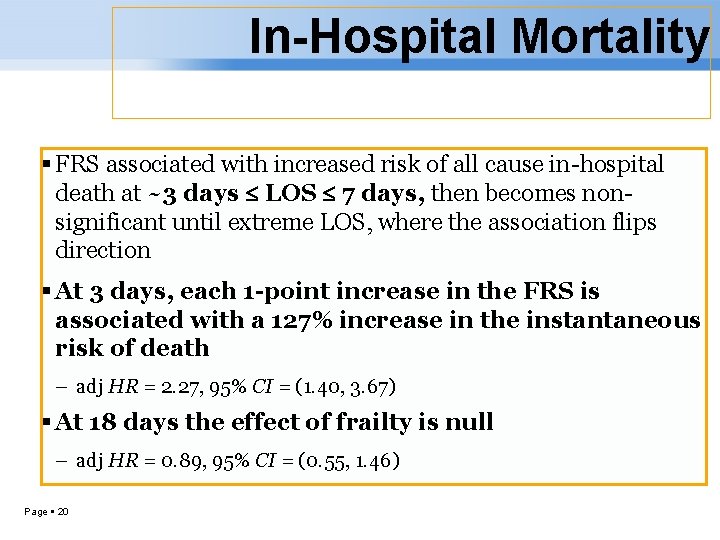
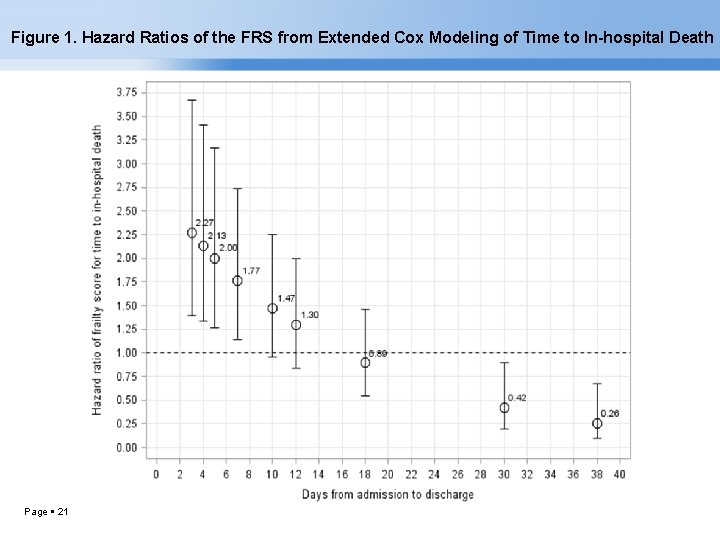
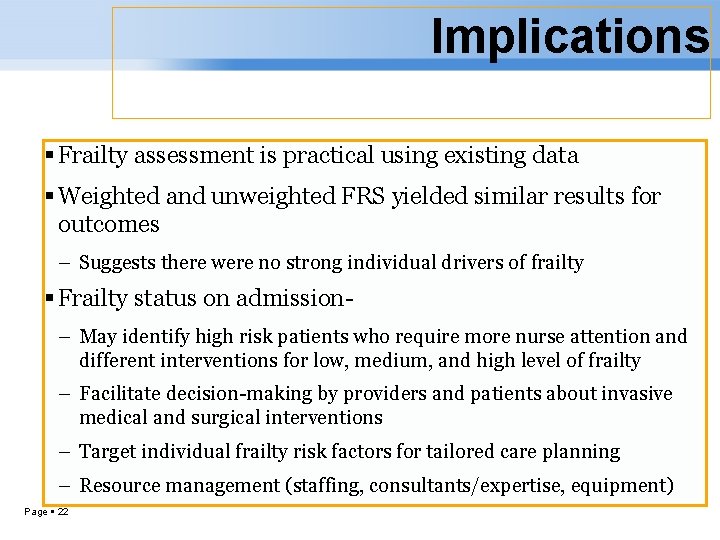
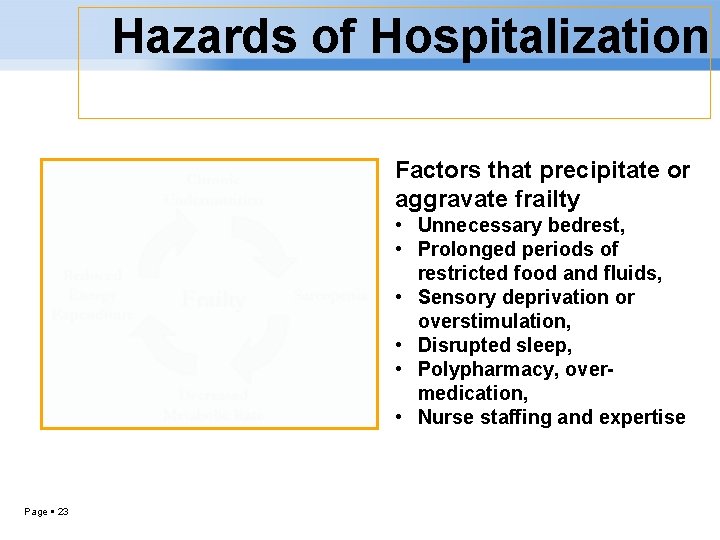
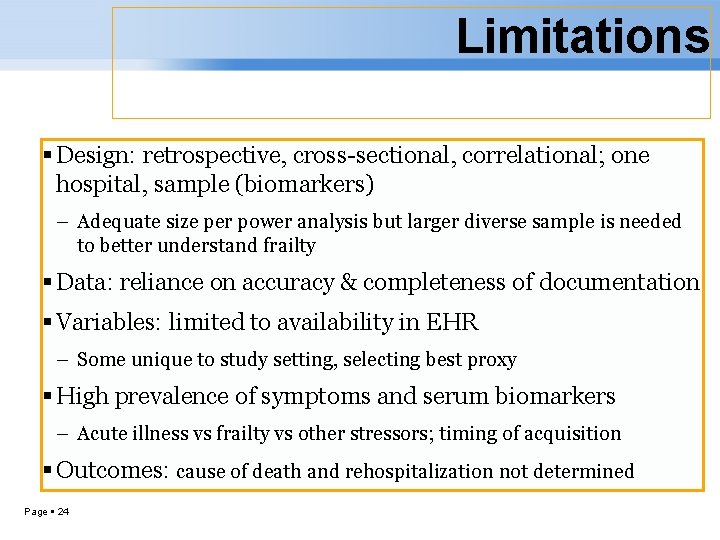
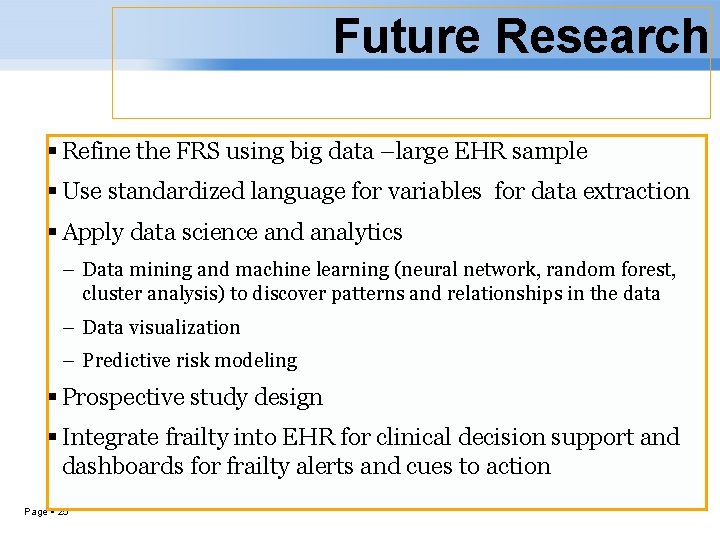
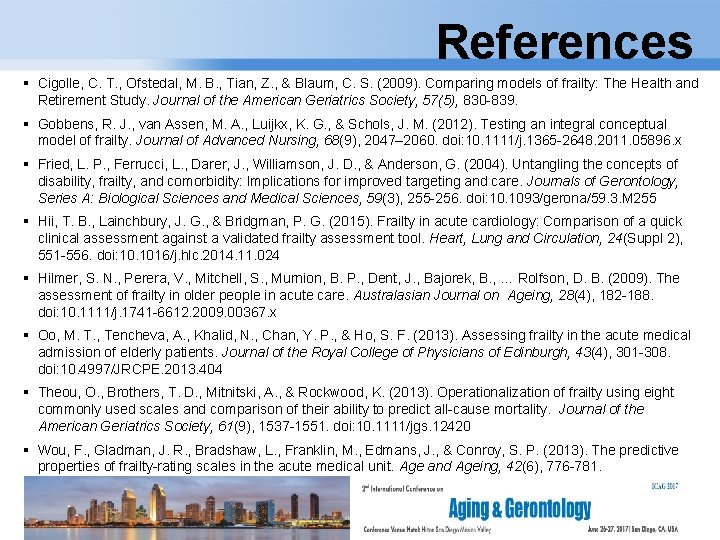
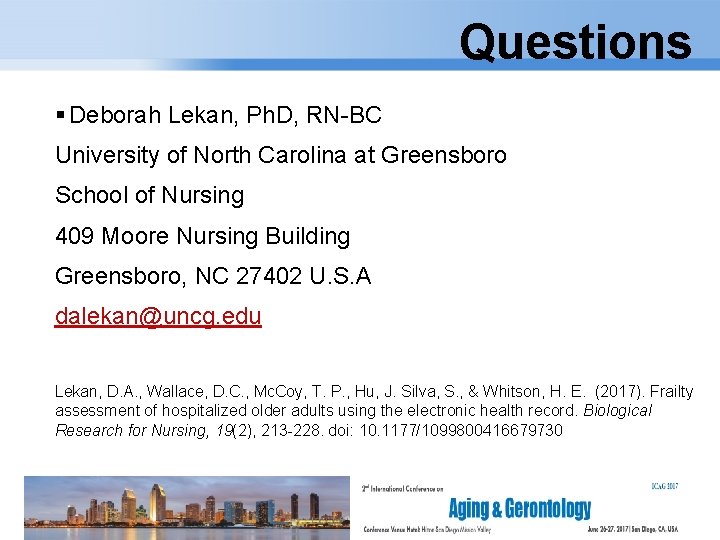
- Slides: 27
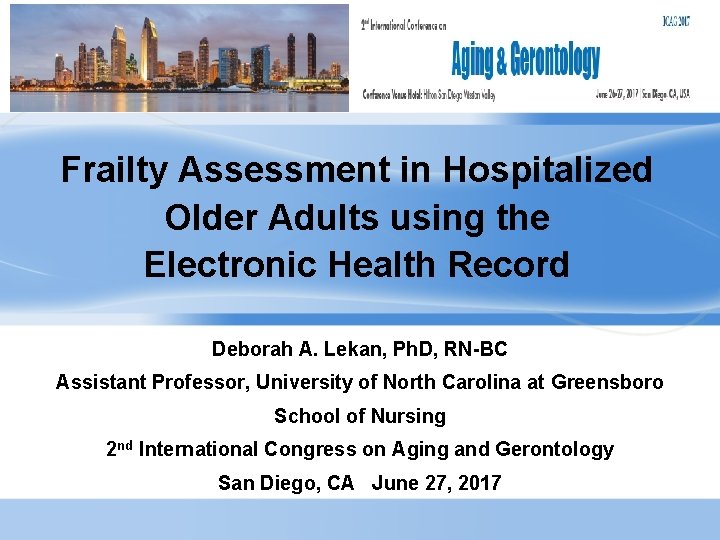
Frailty Assessment in Hospitalized Older Adults using the Electronic Health Record Deborah A. Lekan, Ph. D, RN-BC Assistant Professor, University of North Carolina at Greensboro School of Nursing 2 nd International Congress on Aging and Gerontology San Diego, CA June 27, 2017
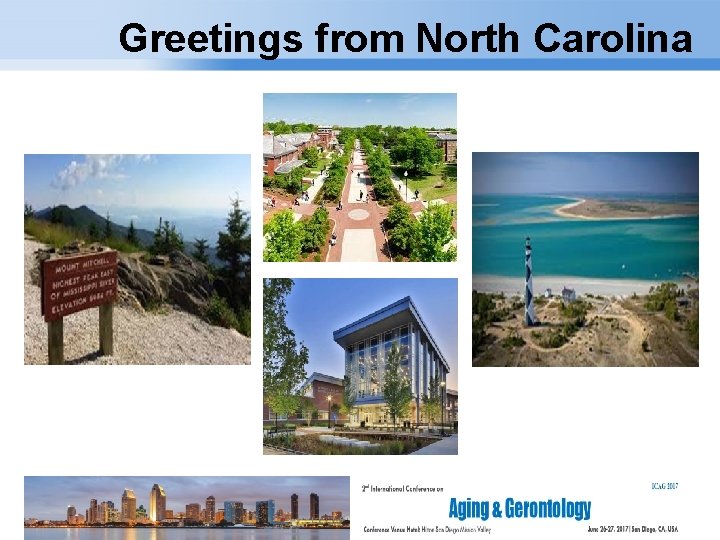
Greetings from North Carolina Page 2
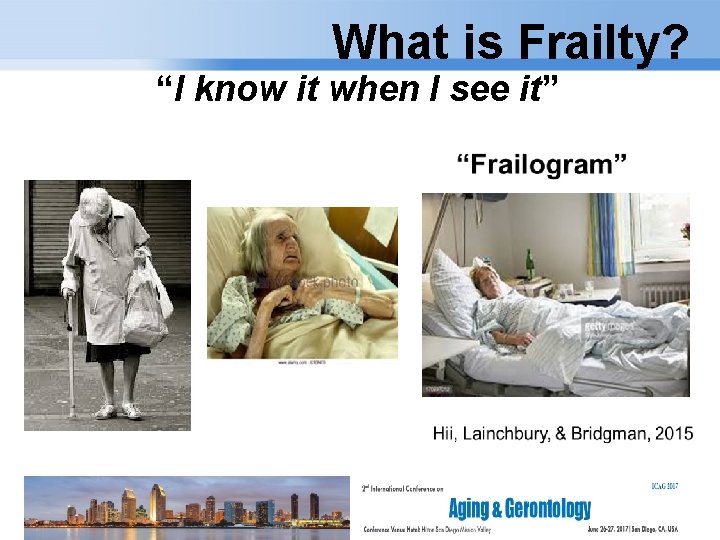
What is Frailty? “I know it when I see it” Page 3
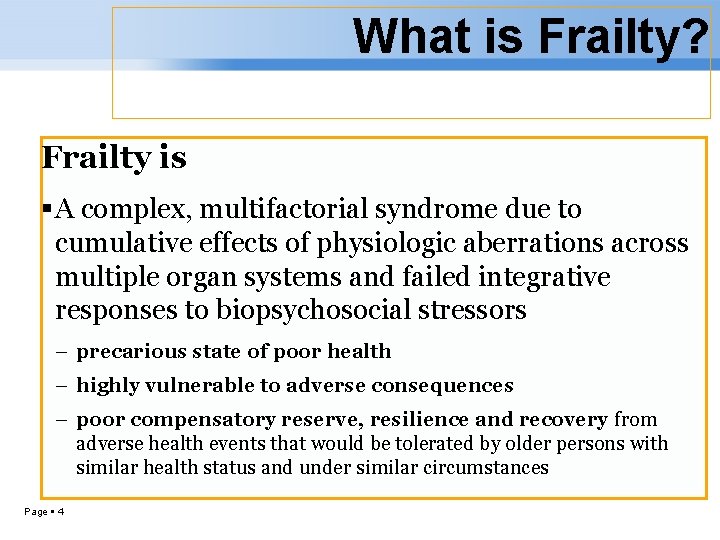
What is Frailty? Frailty is A complex, multifactorial syndrome due to cumulative effects of physiologic aberrations across multiple organ systems and failed integrative responses to biopsychosocial stressors – precarious state of poor health – highly vulnerable to adverse consequences – poor compensatory reserve, resilience and recovery from adverse health events that would be tolerated by older persons with similar health status and under similar circumstances Page 4
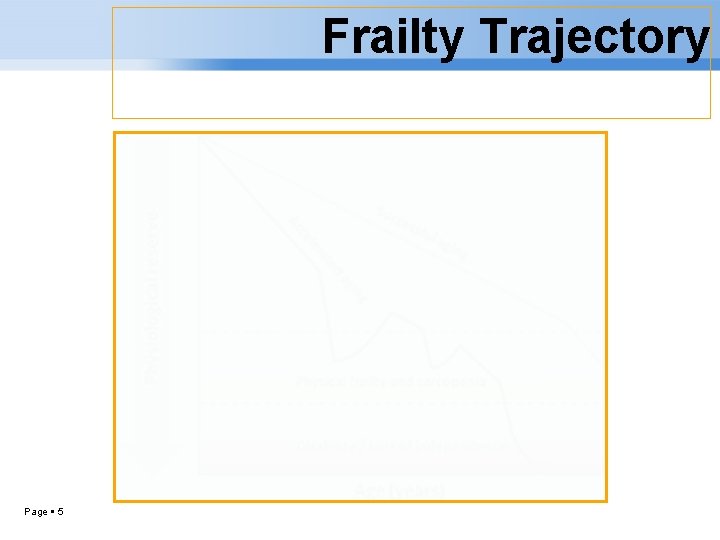
Frailty Trajectory Page 5
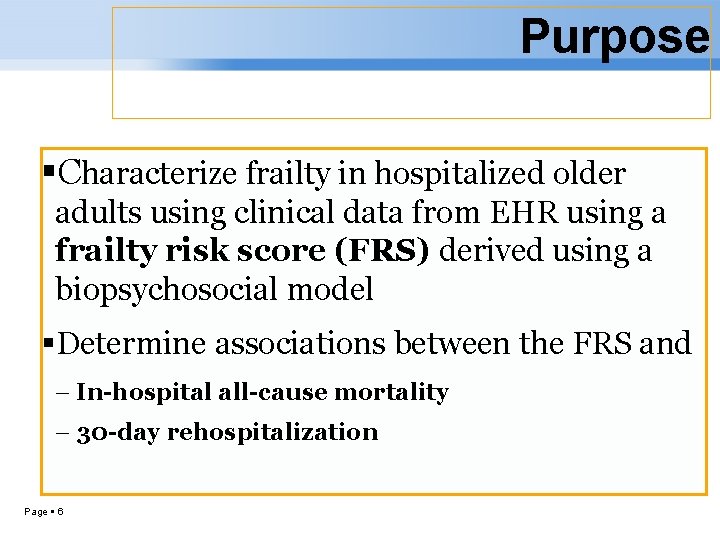
Purpose Characterize frailty in hospitalized older adults using clinical data from EHR using a frailty risk score (FRS) derived using a biopsychosocial model Determine associations between the FRS and – In-hospital all-cause mortality – 30 -day rehospitalization Page 6
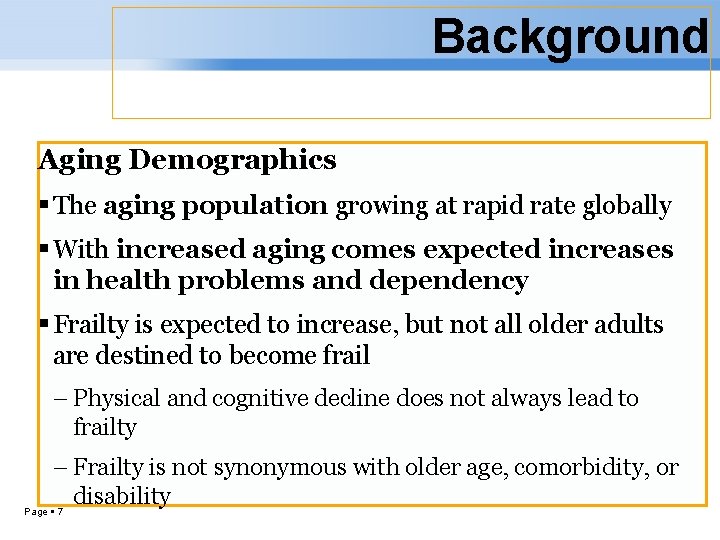
Background Aging Demographics The aging population growing at rapid rate globally With increased aging comes expected increases in health problems and dependency Frailty is expected to increase, but not all older adults are destined to become frail – Physical and cognitive decline does not always lead to frailty – Frailty is not synonymous with older age, comorbidity, or disability Page 7
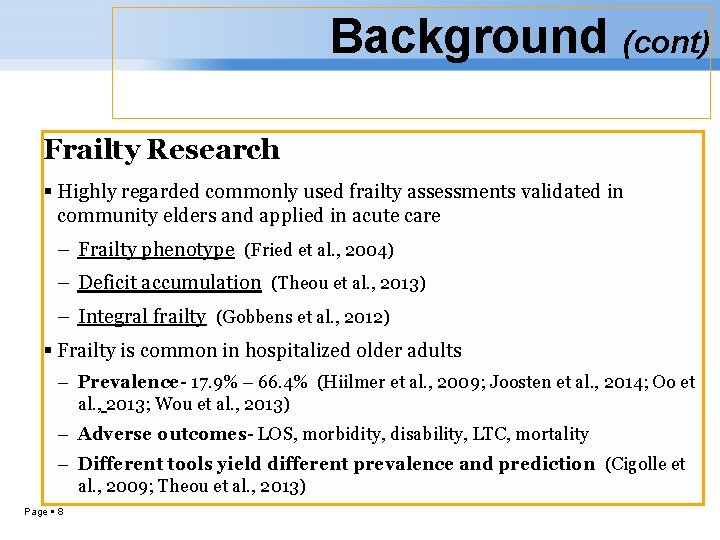
Background (cont) Frailty Research Highly regarded commonly used frailty assessments validated in community elders and applied in acute care – Frailty phenotype (Fried et al. , 2004) – Deficit accumulation (Theou et al. , 2013) – Integral frailty (Gobbens et al. , 2012) Frailty is common in hospitalized older adults – Prevalence- 17. 9% – 66. 4% (Hiilmer et al. , 2009; Joosten et al. , 2014; Oo et al. , 2013; Wou et al. , 2013) – Adverse outcomes- LOS, morbidity, disability, LTC, mortality – Different tools yield different prevalence and prediction (Cigolle et al. , 2009; Theou et al. , 2013) Page 8
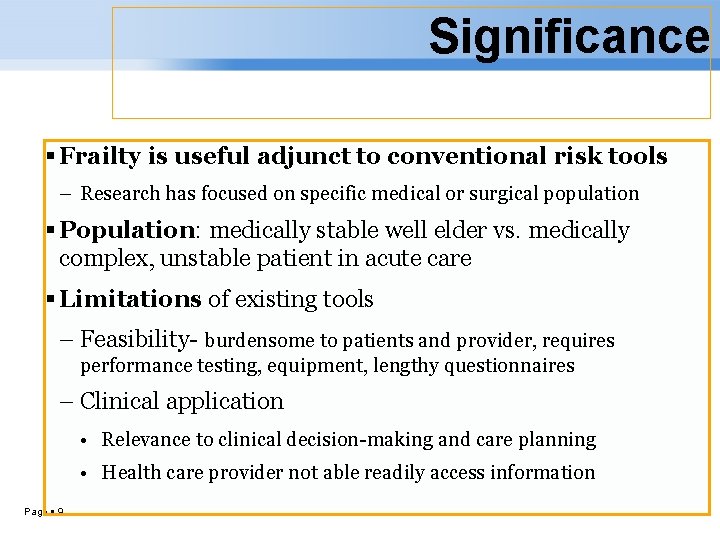
Significance Frailty is useful adjunct to conventional risk tools – Research has focused on specific medical or surgical population Population: medically stable well elder vs. medically complex, unstable patient in acute care Limitations of existing tools – Feasibility- burdensome to patients and provider, requires performance testing, equipment, lengthy questionnaires – Clinical application • Relevance to clinical decision-making and care planning • Health care provider not able readily access information Page 9
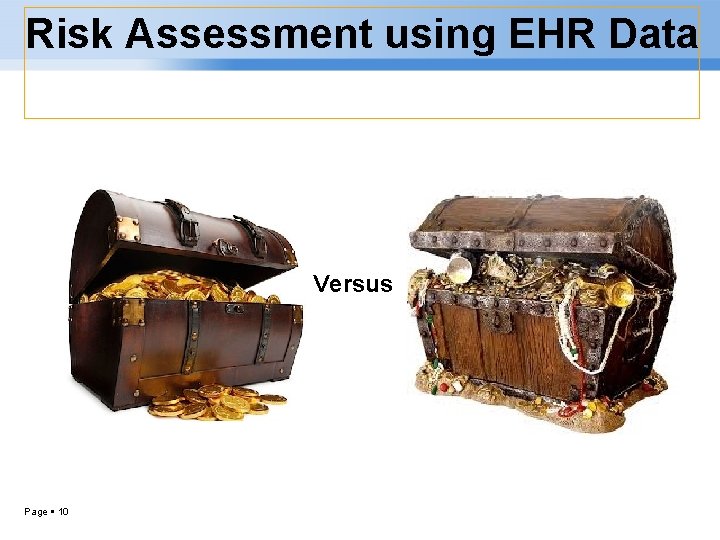
Risk Assessment using EHR Data Versus Page 10
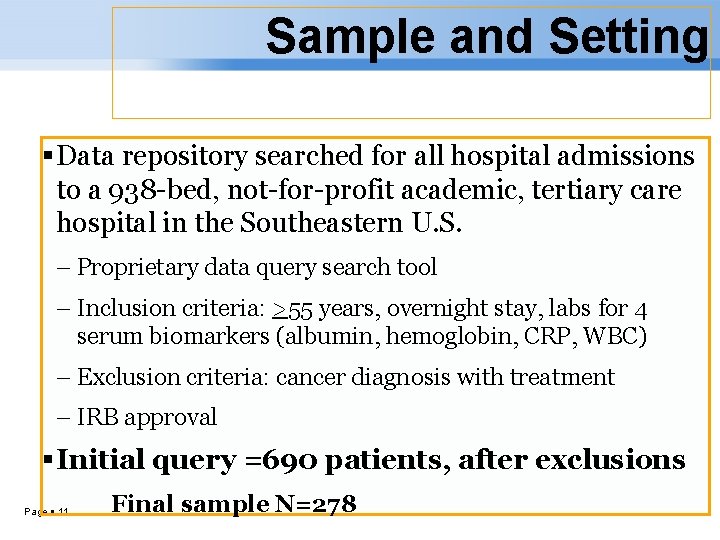
Sample and Setting Data repository searched for all hospital admissions to a 938 -bed, not-for-profit academic, tertiary care hospital in the Southeastern U. S. – Proprietary data query search tool – Inclusion criteria: >55 years, overnight stay, labs for 4 serum biomarkers (albumin, hemoglobin, CRP, WBC) – Exclusion criteria: cancer diagnosis with treatment – IRB approval Initial query =690 patients, after exclusions Page 11 Final sample N=278
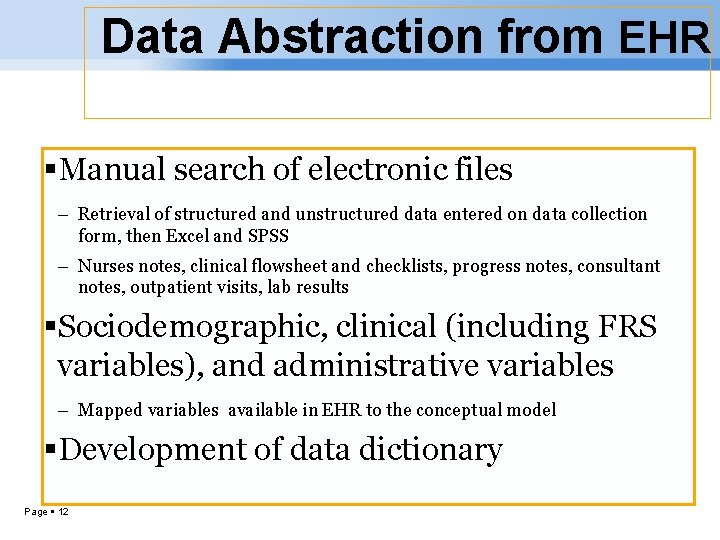
Data Abstraction from EHR Manual search of electronic files – Retrieval of structured and unstructured data entered on data collection form, then Excel and SPSS – Nurses notes, clinical flowsheet and checklists, progress notes, consultant notes, outpatient visits, lab results Sociodemographic, clinical (including FRS variables), and administrative variables – Mapped variables available in EHR to the conceptual model Development of data dictionary Page 12
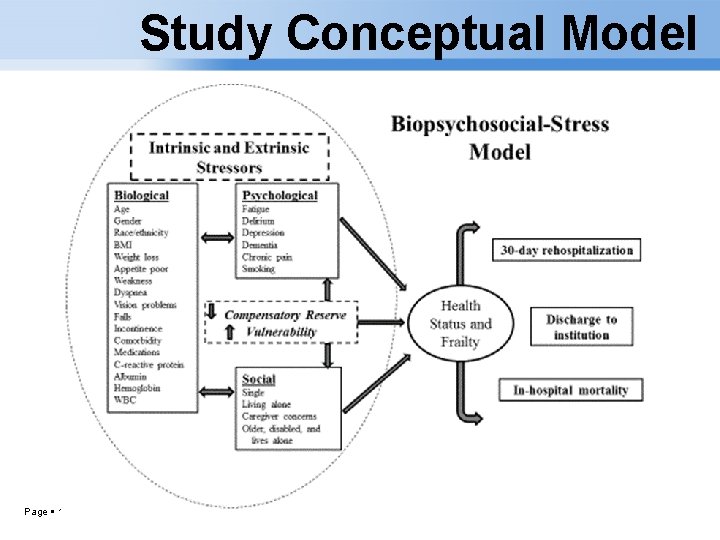
Study Conceptual Model Page 13
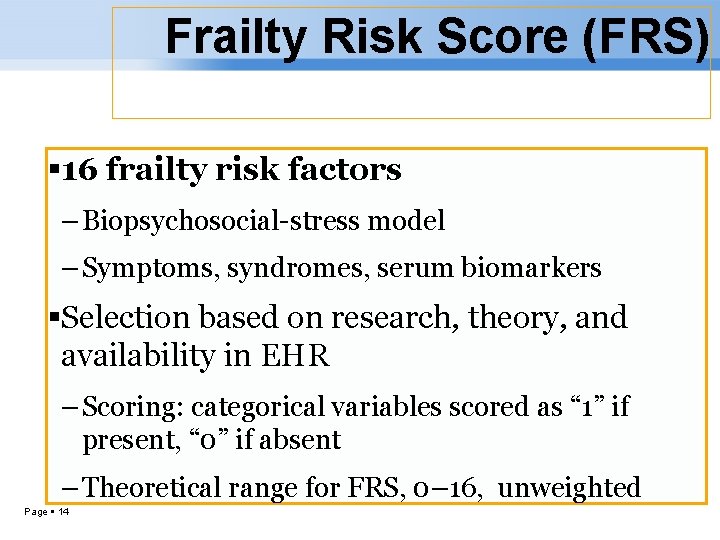
Frailty Risk Score (FRS) 16 frailty risk factors – Biopsychosocial-stress model – Symptoms, syndromes, serum biomarkers Selection based on research, theory, and availability in EHR – Scoring: categorical variables scored as “ 1” if present, “ 0” if absent – Theoretical range for FRS, 0– 16, unweighted Page 14
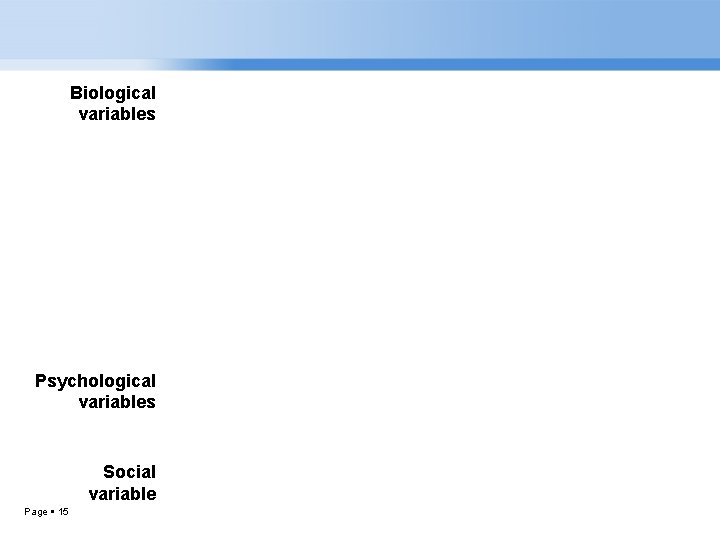
Biological variables Psychological variables Social variable Page 15
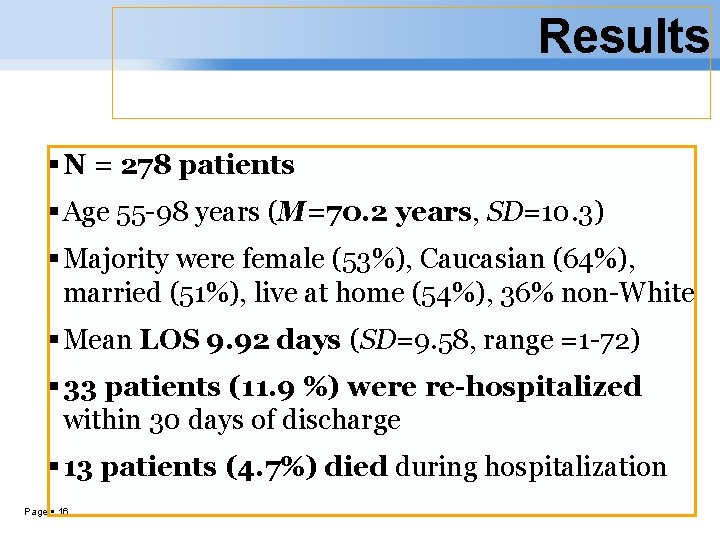
Results N = 278 patients Age 55 -98 years (M=70. 2 years, SD=10. 3) Majority were female (53%), Caucasian (64%), married (51%), live at home (54%), 36% non-White Mean LOS 9. 92 days (SD=9. 58, range =1 -72) 33 patients (11. 9 %) were re-hospitalized within 30 days of discharge 13 patients (4. 7%) died during hospitalization Page 16
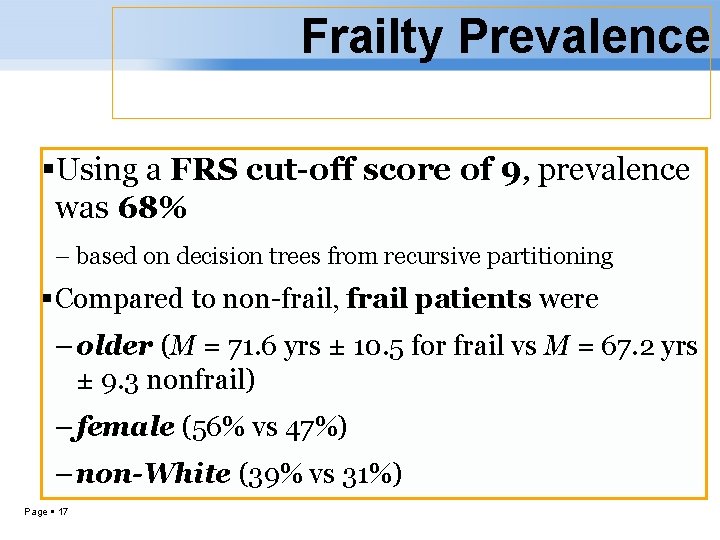
Frailty Prevalence Using a FRS cut-off score of 9, prevalence was 68% – based on decision trees from recursive partitioning Compared to non-frail, frail patients were – older (M = 71. 6 yrs ± 10. 5 for frail vs M = 67. 2 yrs ± 9. 3 nonfrail) – female (56% vs 47%) – non-White (39% vs 31%) Page 17
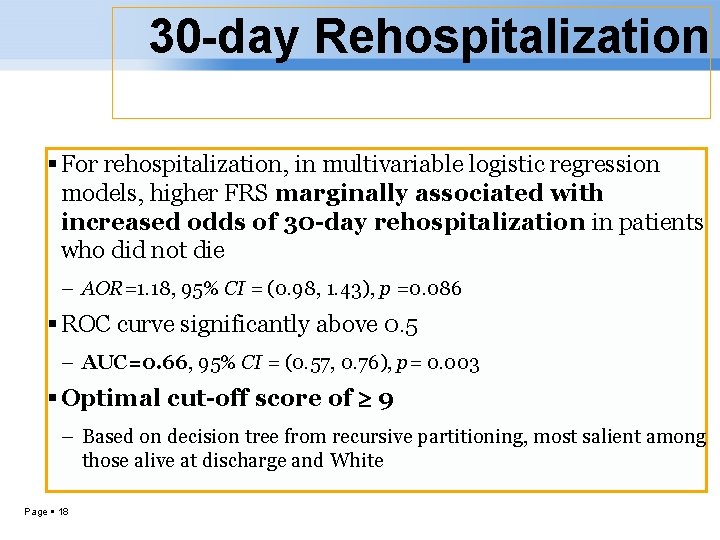
30 -day Rehospitalization For rehospitalization, in multivariable logistic regression models, higher FRS marginally associated with increased odds of 30 -day rehospitalization in patients who did not die – AOR=1. 18, 95% CI = (0. 98, 1. 43), p =0. 086 ROC curve significantly above 0. 5 – AUC=0. 66, 95% CI = (0. 57, 0. 76), p= 0. 003 Optimal cut-off score of 9 – Based on decision tree from recursive partitioning, most salient among those alive at discharge and White Page 18
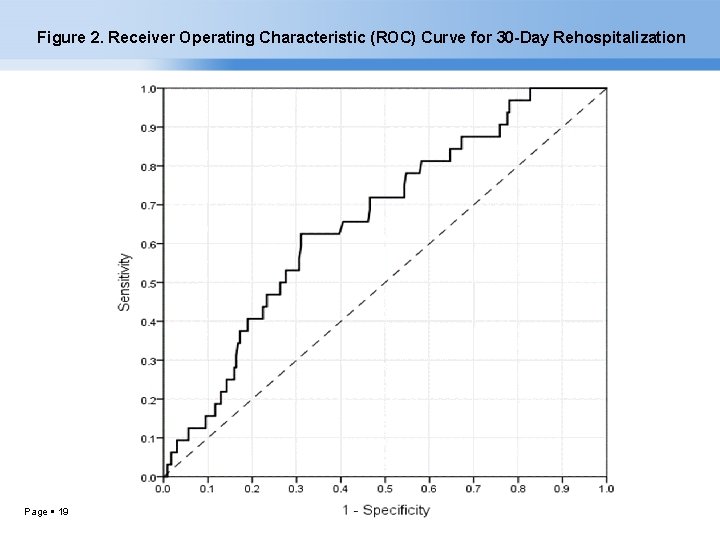
Figure 2. Receiver Operating Characteristic (ROC) Curve for 30 -Day Rehospitalization Page 19
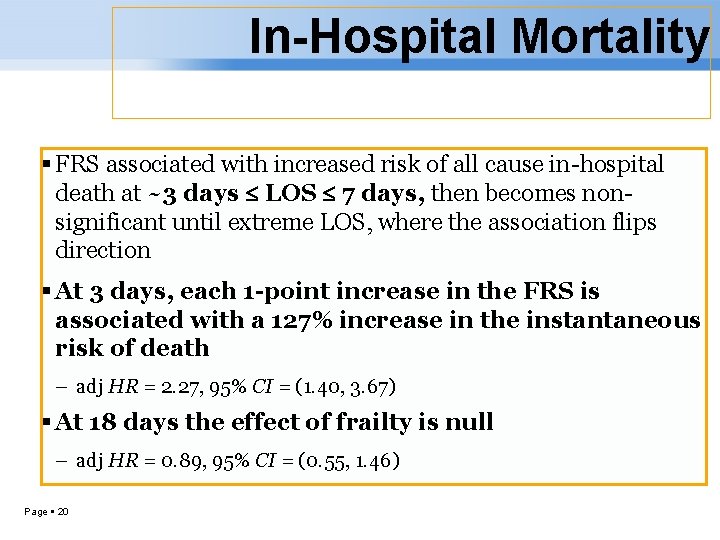
In-Hospital Mortality FRS associated with increased risk of all cause in-hospital death at ~3 days LOS 7 days, then becomes nonsignificant until extreme LOS, where the association flips direction At 3 days, each 1 -point increase in the FRS is associated with a 127% increase in the instantaneous risk of death – adj HR = 2. 27, 95% CI = (1. 40, 3. 67) At 18 days the effect of frailty is null – adj HR = 0. 89, 95% CI = (0. 55, 1. 46) Page 20
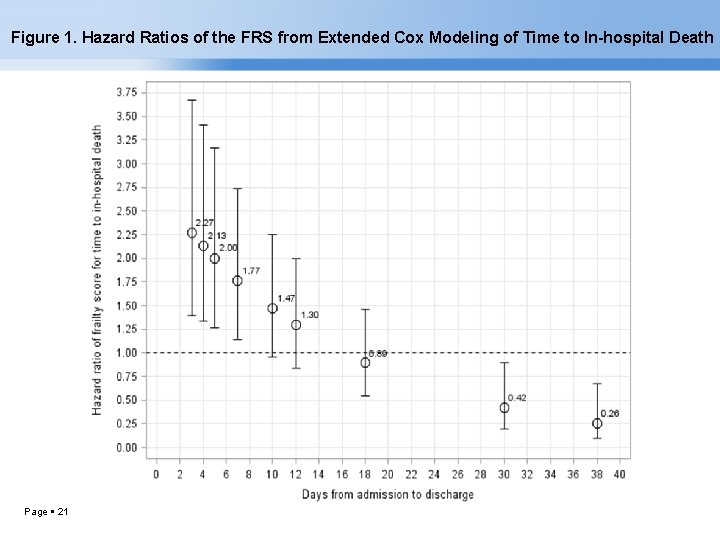
Figure 1. Hazard Ratios of the FRS from Extended Cox Modeling of Time to In-hospital Death Page 21
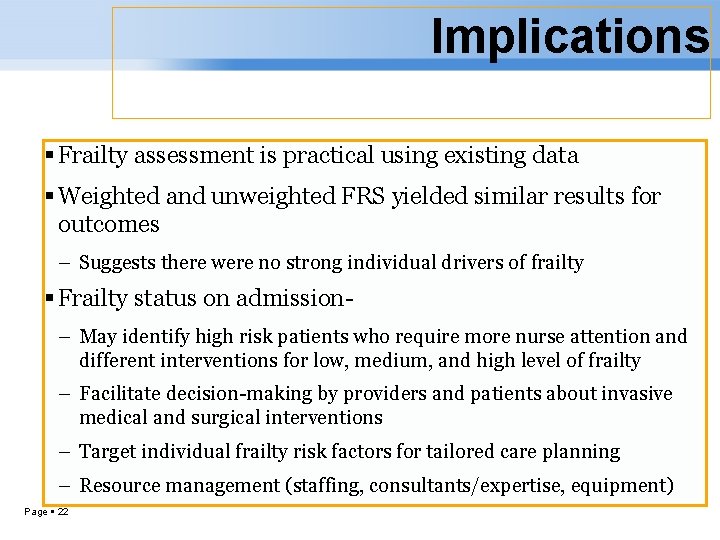
Implications Frailty assessment is practical using existing data Weighted and unweighted FRS yielded similar results for outcomes – Suggests there were no strong individual drivers of frailty Frailty status on admission– May identify high risk patients who require more nurse attention and different interventions for low, medium, and high level of frailty – Facilitate decision-making by providers and patients about invasive medical and surgical interventions – Target individual frailty risk factors for tailored care planning – Resource management (staffing, consultants/expertise, equipment) Page 22
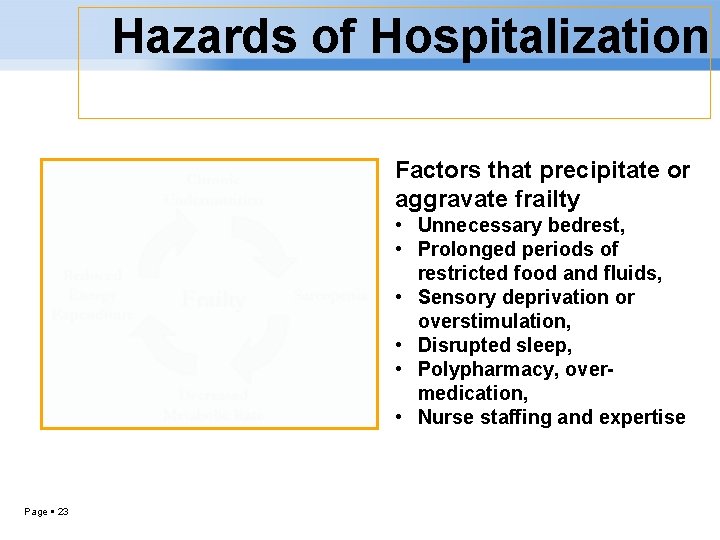
Hazards of Hospitalization Factors that precipitate or aggravate frailty • Unnecessary bedrest, • Prolonged periods of restricted food and fluids, • Sensory deprivation or overstimulation, • Disrupted sleep, • Polypharmacy, overmedication, • Nurse staffing and expertise Page 23
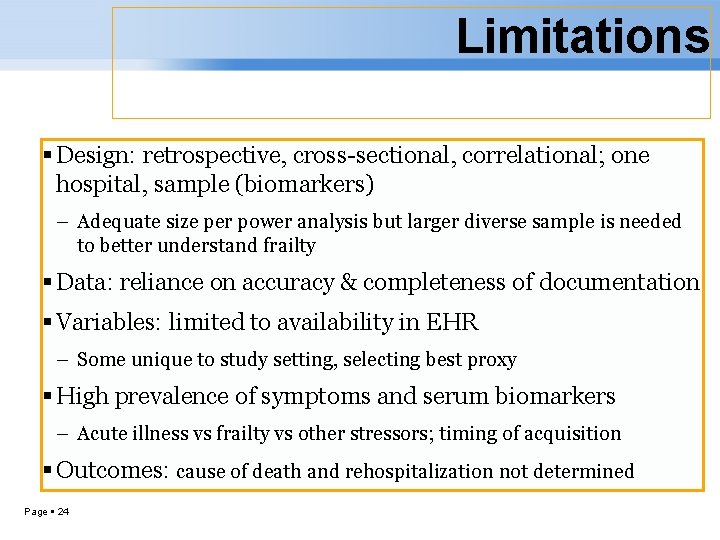
Limitations Design: retrospective, cross-sectional, correlational; one hospital, sample (biomarkers) – Adequate size per power analysis but larger diverse sample is needed to better understand frailty Data: reliance on accuracy & completeness of documentation Variables: limited to availability in EHR – Some unique to study setting, selecting best proxy High prevalence of symptoms and serum biomarkers – Acute illness vs frailty vs other stressors; timing of acquisition Outcomes: cause of death and rehospitalization not determined Page 24
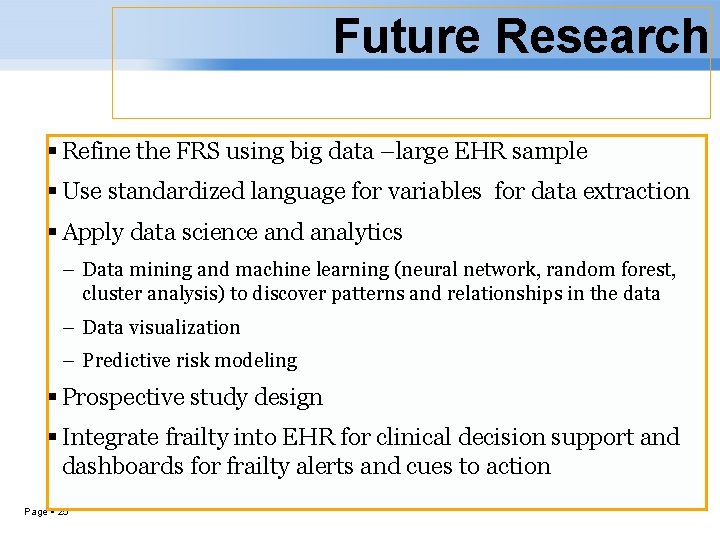
Future Research Refine the FRS using big data –large EHR sample Use standardized language for variables for data extraction Apply data science and analytics – Data mining and machine learning (neural network, random forest, cluster analysis) to discover patterns and relationships in the data – Data visualization – Predictive risk modeling Prospective study design Integrate frailty into EHR for clinical decision support and dashboards for frailty alerts and cues to action Page 25
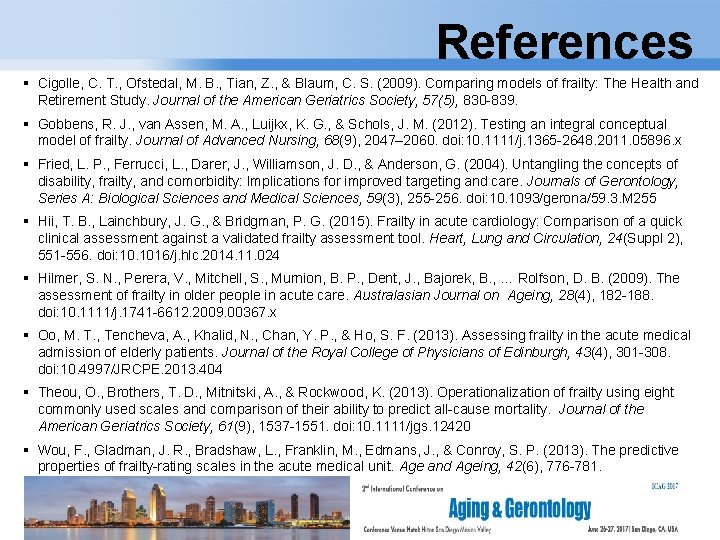
References Cigolle, C. T. , Ofstedal, M. B. , Tian, Z. , & Blaum, C. S. (2009). Comparing models of frailty: The Health and Retirement Study. Journal of the American Geriatrics Society, 57(5), 830 -839. Gobbens, R. J. , van Assen, M. A. , Luijkx, K. G. , & Schols, J. M. (2012). Testing an integral conceptual model of frailty. Journal of Advanced Nursing, 68(9), 2047– 2060. doi: 10. 1111/j. 1365 -2648. 2011. 05896. x Fried, L. P. , Ferrucci, L. , Darer, J. , Williamson, J. D. , & Anderson, G. (2004). Untangling the concepts of disability, frailty, and comorbidity: Implications for improved targeting and care. Journals of Gerontology, Series A: Biological Sciences and Medical Sciences, 59(3), 255 -256. doi: 10. 1093/gerona/59. 3. M 255 Hii, T. B. , Lainchbury, J. G. , & Bridgman, P. G. (2015). Frailty in acute cardiology: Comparison of a quick clinical assessment against a validated frailty assessment tool. Heart, Lung and Circulation, 24(Suppl 2), 551 -556. doi: 10. 1016/j. hlc. 2014. 11. 024 Hilmer, S. N. , Perera, V. , Mitchell, S. , Murnion, B. P. , Dent, J. , Bajorek, B. , … Rolfson, D. B. (2009). The assessment of frailty in older people in acute care. Australasian Journal on Ageing, 28(4), 182 -188. doi: 10. 1111/j. 1741 -6612. 2009. 00367. x Oo, M. T. , Tencheva, A. , Khalid, N. , Chan, Y. P. , & Ho, S. F. (2013). Assessing frailty in the acute medical admission of elderly patients. Journal of the Royal College of Physicians of Edinburgh, 43(4), 301 -308. doi: 10. 4997/JRCPE. 2013. 404 Theou, O. , Brothers, T. D. , Mitnitski, A. , & Rockwood, K. (2013). Operationalization of frailty using eight commonly used scales and comparison of their ability to predict all-cause mortality. Journal of the American Geriatrics Society, 61(9), 1537 -1551. doi: 10. 1111/jgs. 12420 Wou, F. , Gladman, J. R. , Bradshaw, L. , Franklin, M. , Edmans, J. , & Conroy, S. P. (2013). The predictive properties of frailty-rating scales in the acute medical unit. Age and Ageing, 42(6), 776 -781. doi: 10. 1093/ageing/aft 055 Page 26
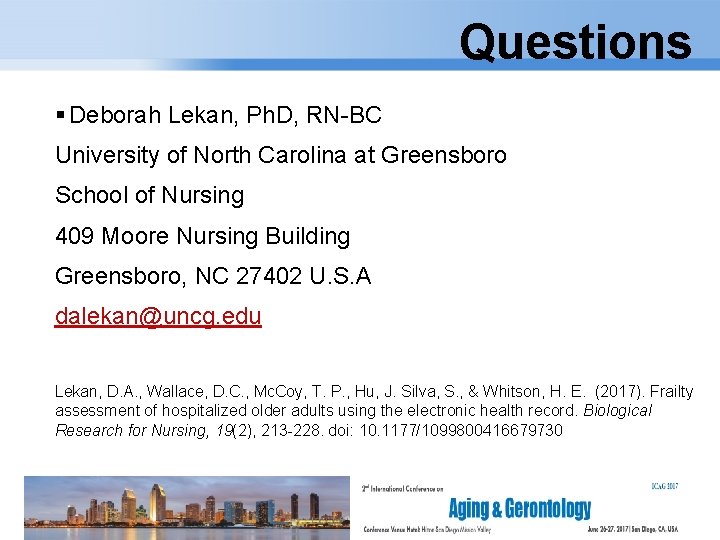
Questions Deborah Lekan, Ph. D, RN-BC University of North Carolina at Greensboro School of Nursing 409 Moore Nursing Building Greensboro, NC 27402 U. S. A dalekan@uncg. edu Lekan, D. A. , Wallace, D. C. , Mc. Coy, T. P. , Hu, J. Silva, S. , & Whitson, H. E. (2017). Frailty assessment of hospitalized older adults using the electronic health record. Biological Research for Nursing, 19(2), 213 -228. doi: 10. 1177/1099800416679730 Page 27
Life is older older than the trees
Mental health and older adults
Mental health and older adults
Altered cognition in older adults is commonly attributed to
Physical examination conclusion
Older adults
Covids older adults
Older adults mental health
Dynamic stretching for older adults
Nursing care of hospitalized child
Nursing care of hospitalized child
Frailty thy name is woman meaning
Hamlet baroque
Frailty syndrome
Fried score frailty
Rockwood frailty index
Frailty index
Sarcoporosis
Assessment strategies for adults
Web accessibility for older users
Younger shorter older old
Syncope in the older patient is
Synformal anticline
Dq98 assessment form
Late adulthood cognitive development
Older individuals who are blind program
As people grow older
Although it was raining maria went for a jog at the park