Forecasting Chapter 5 OPS 370 Forecasting What is
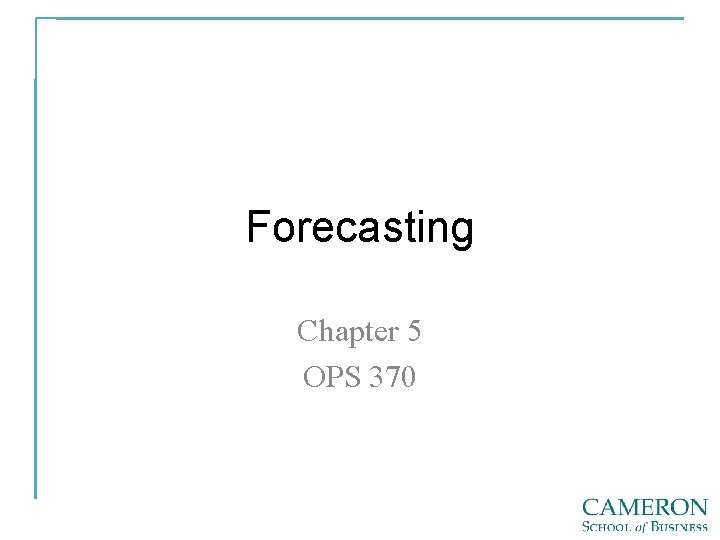
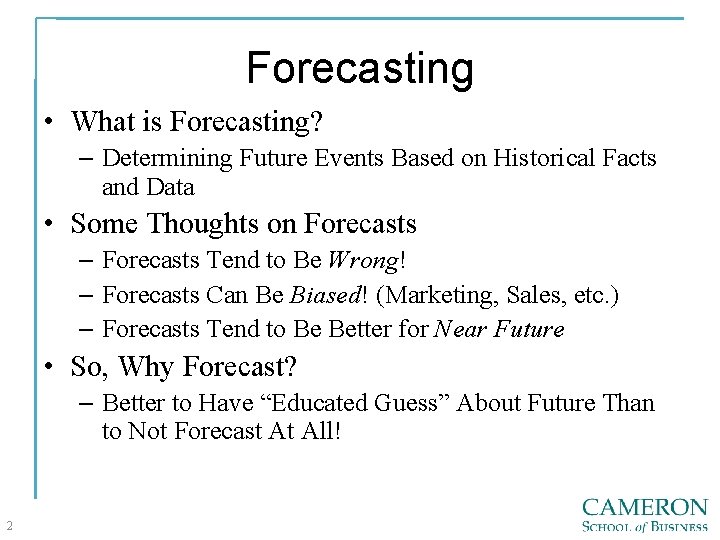
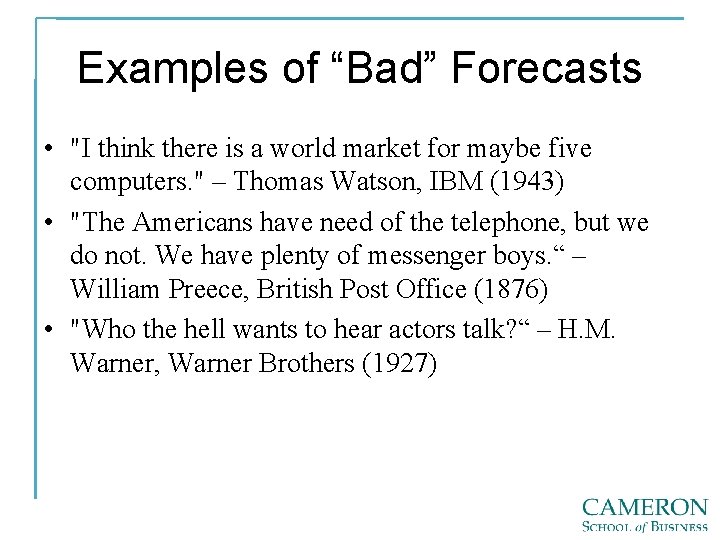
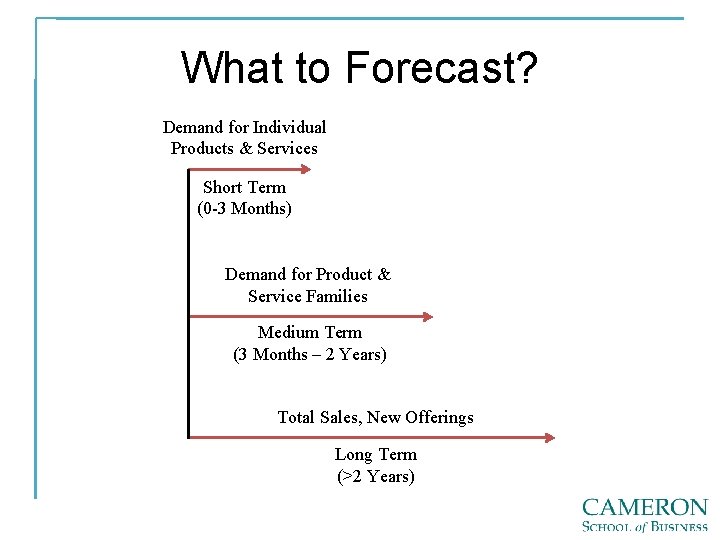
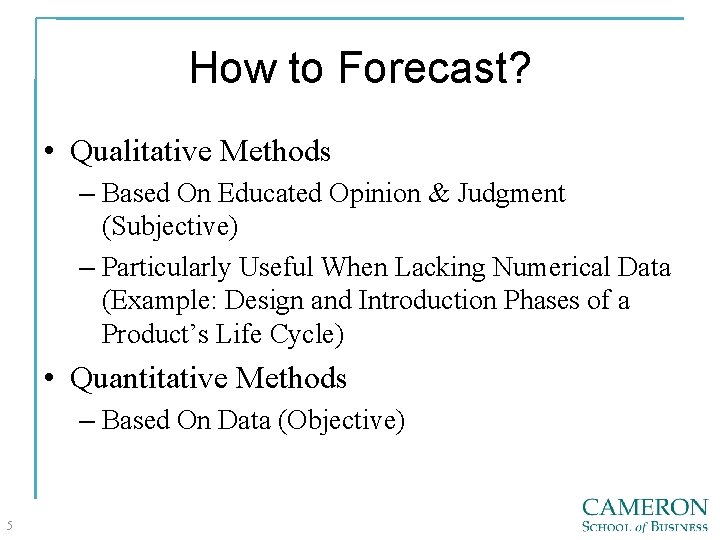
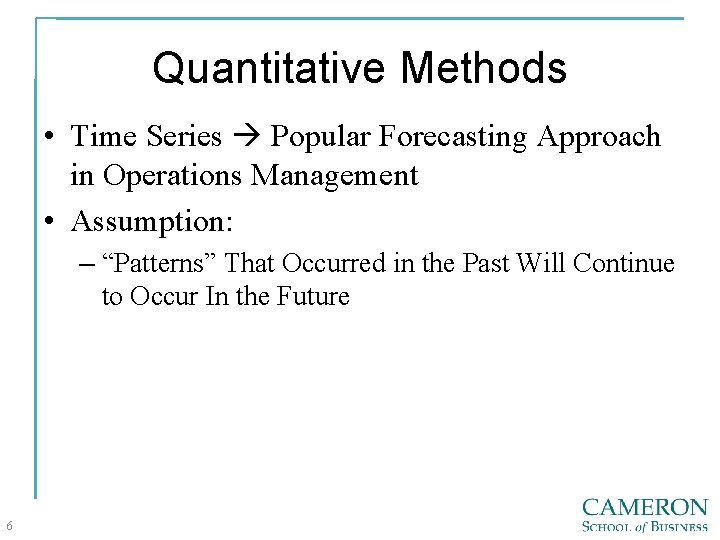
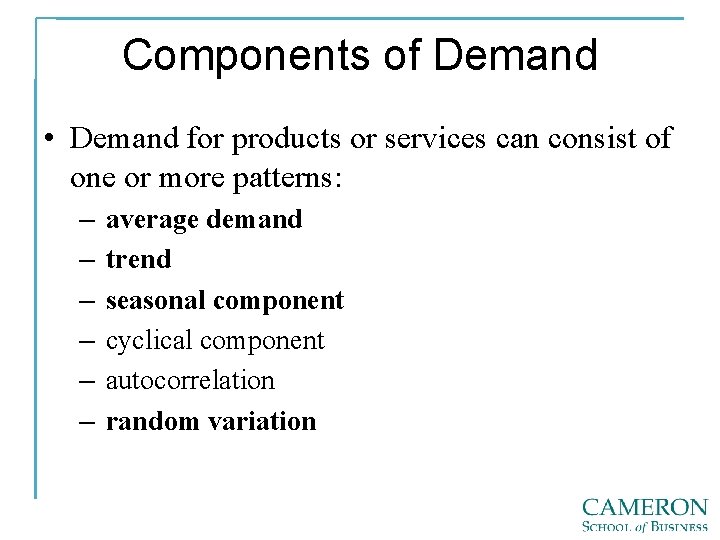
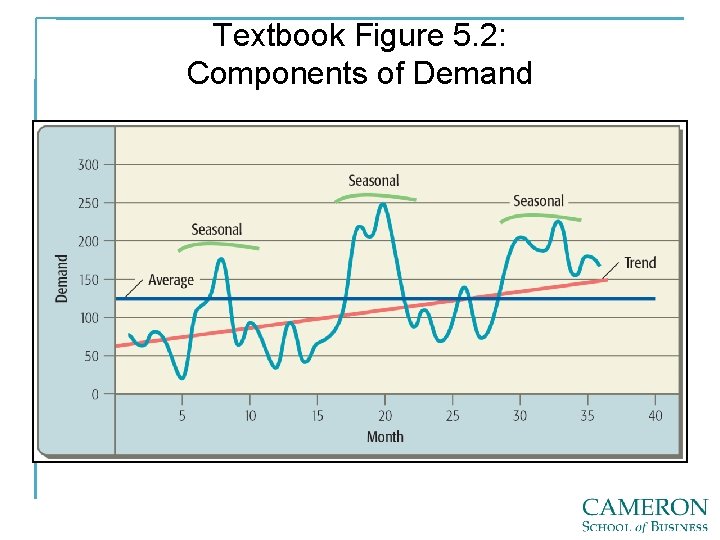
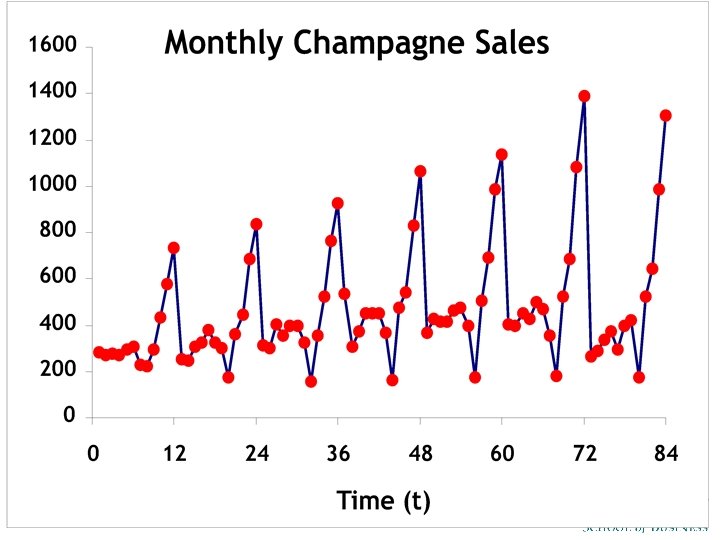
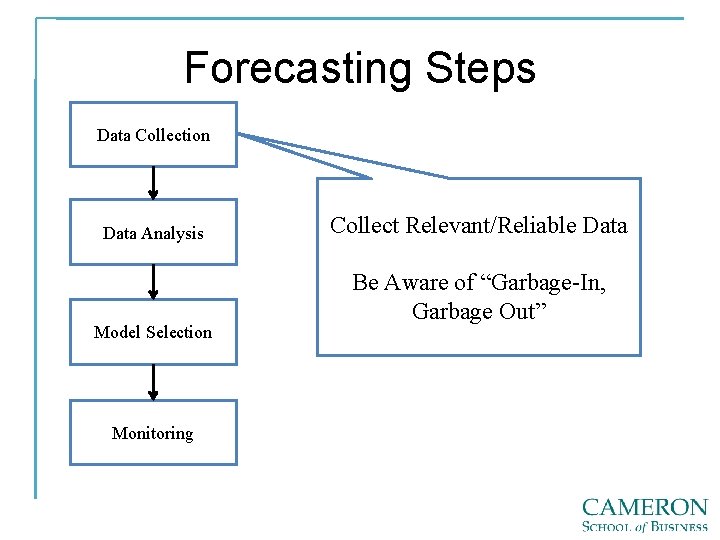
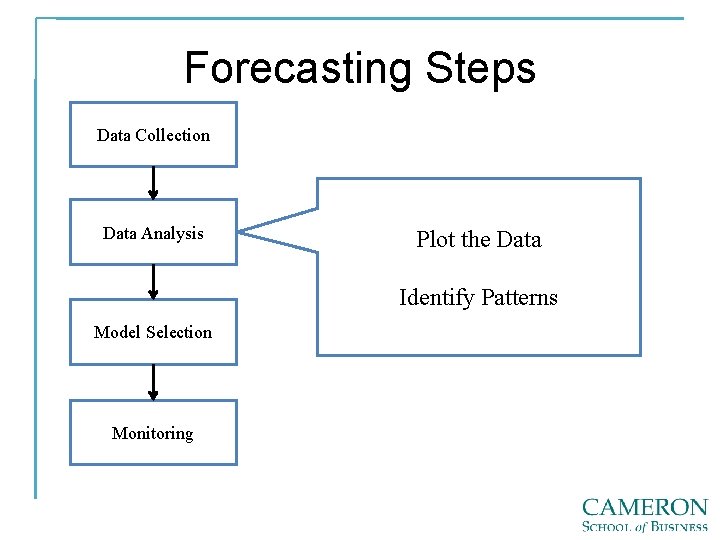
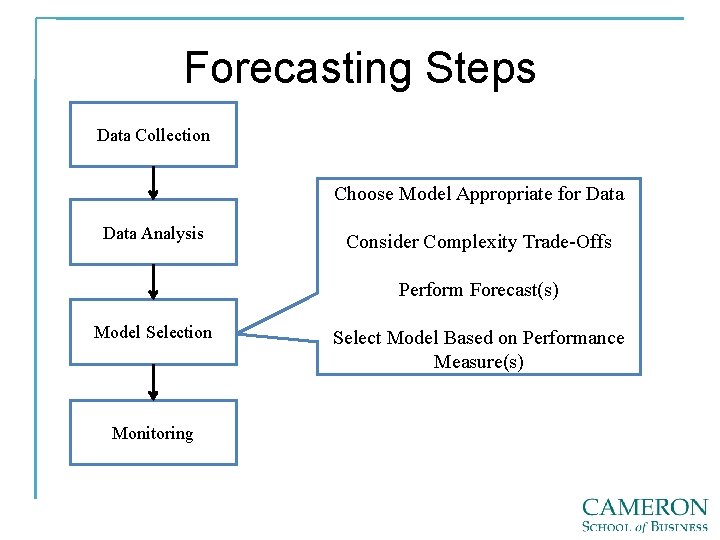
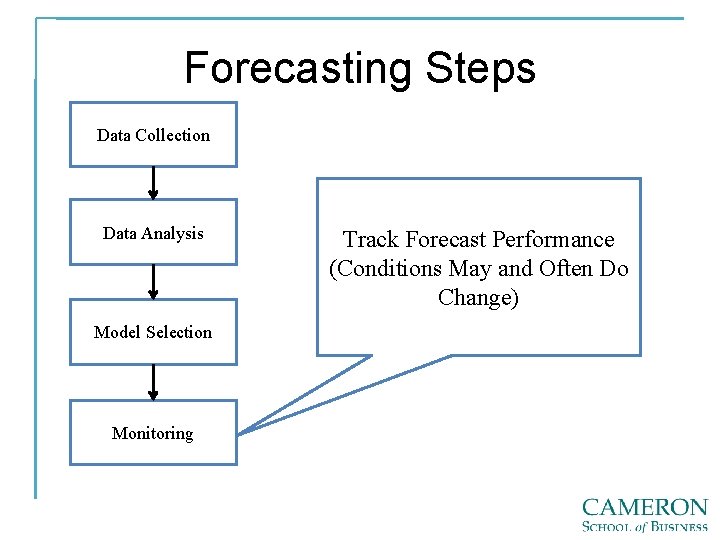
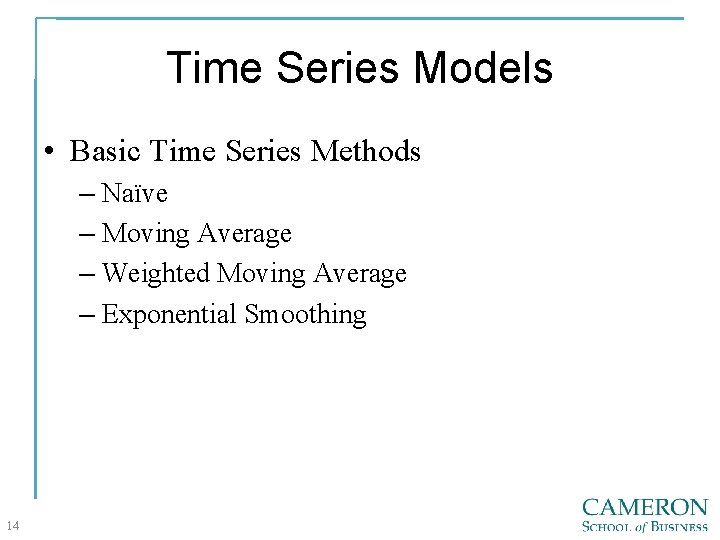
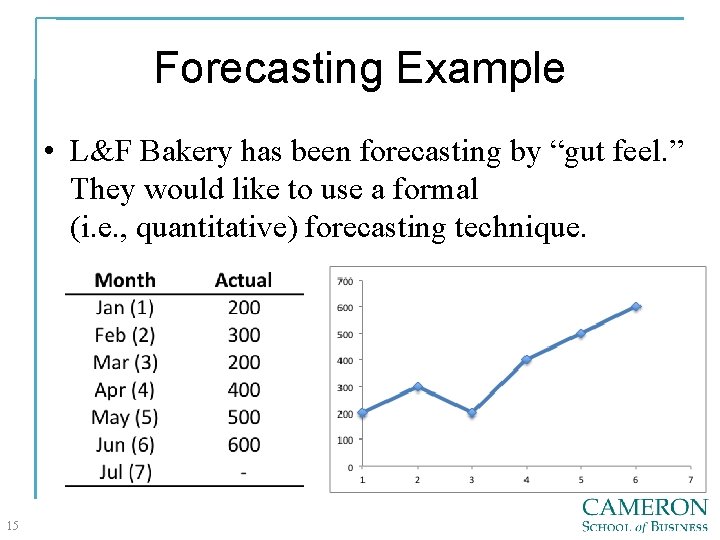
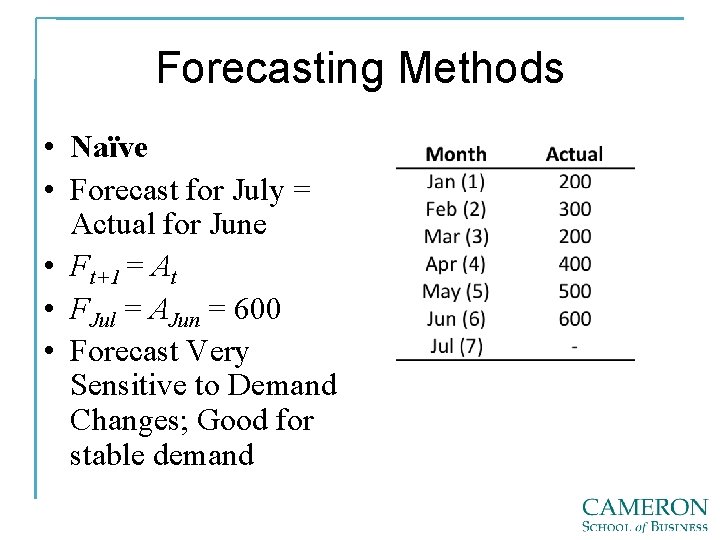
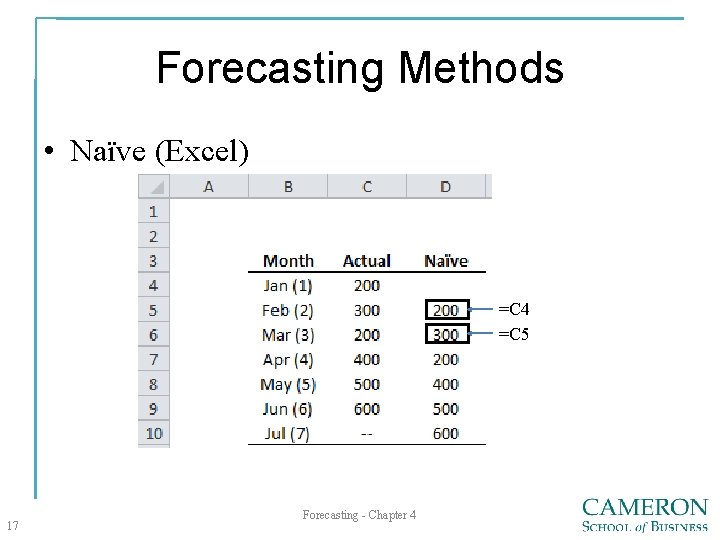
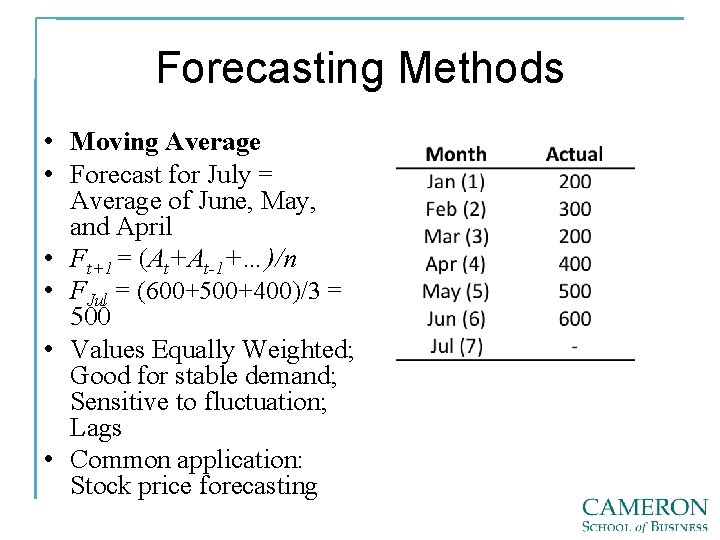
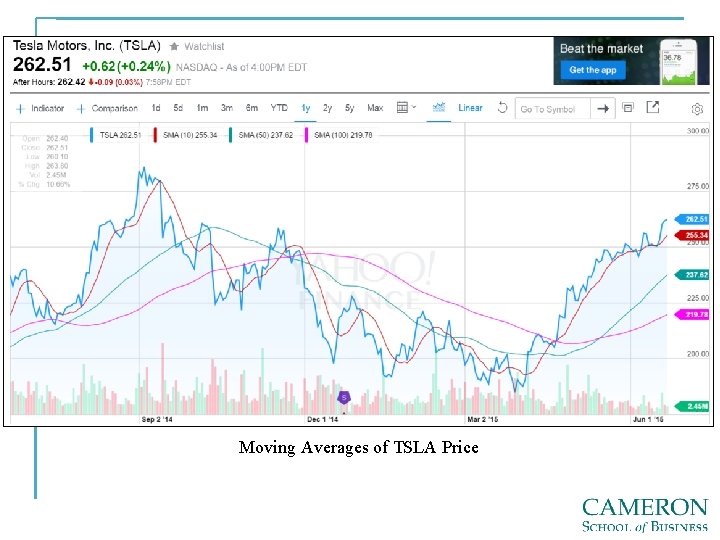
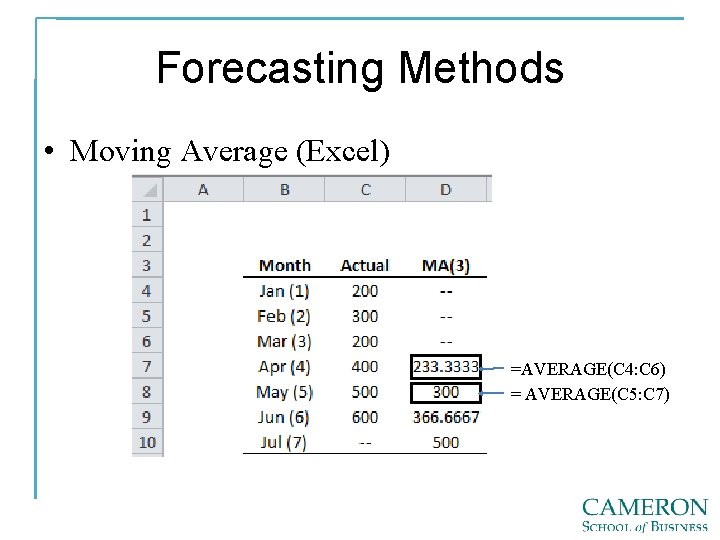
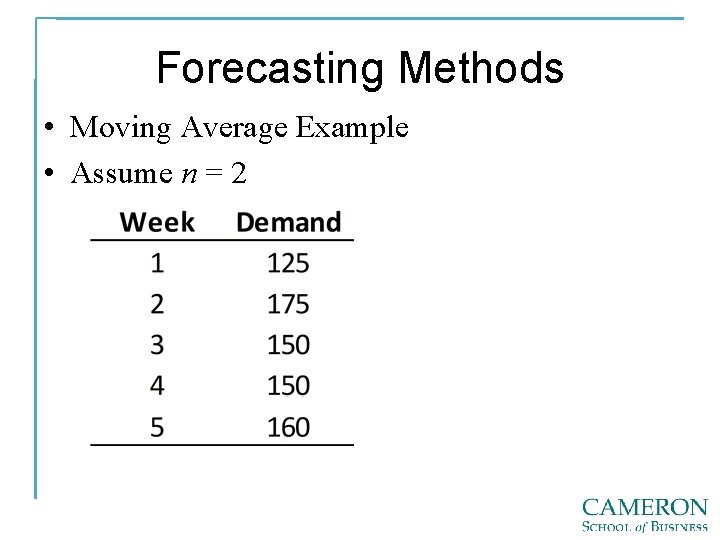
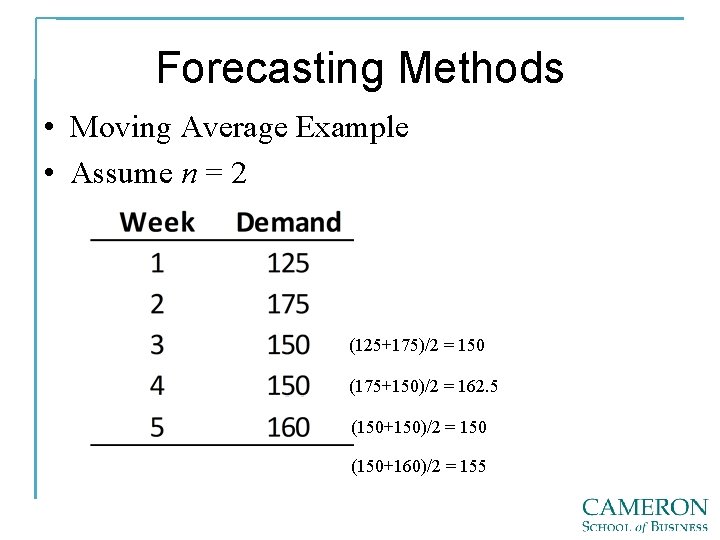
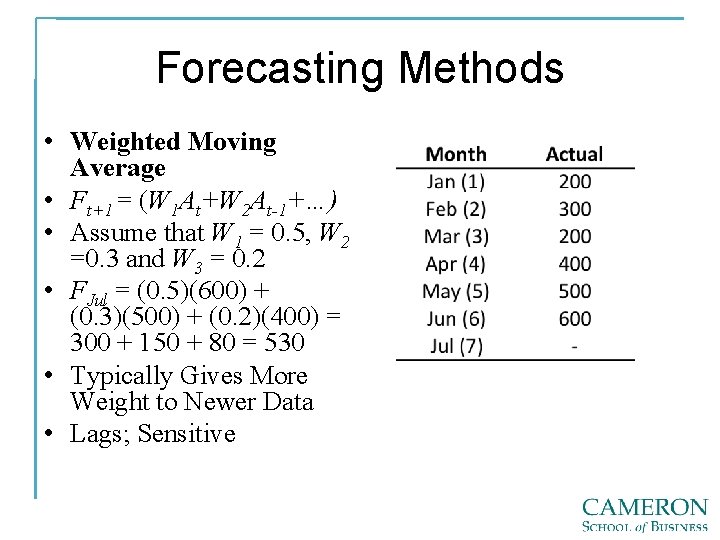
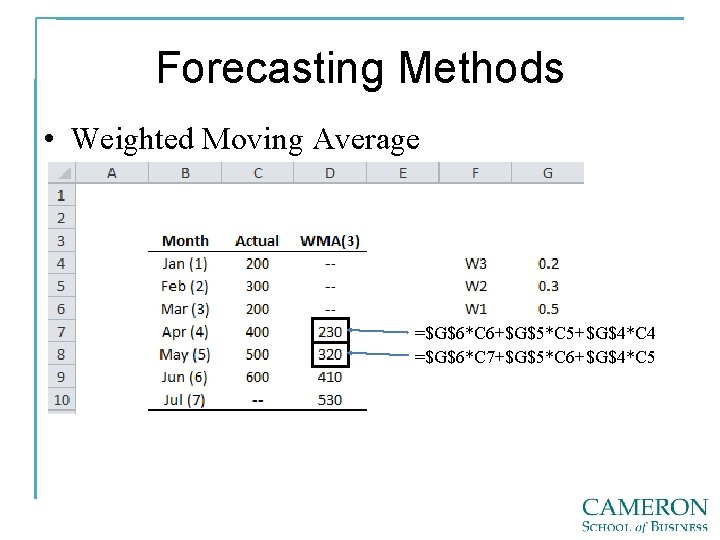
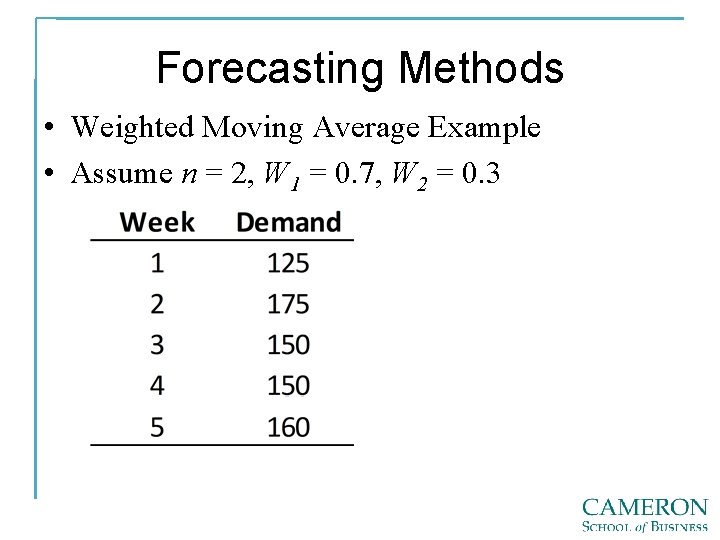
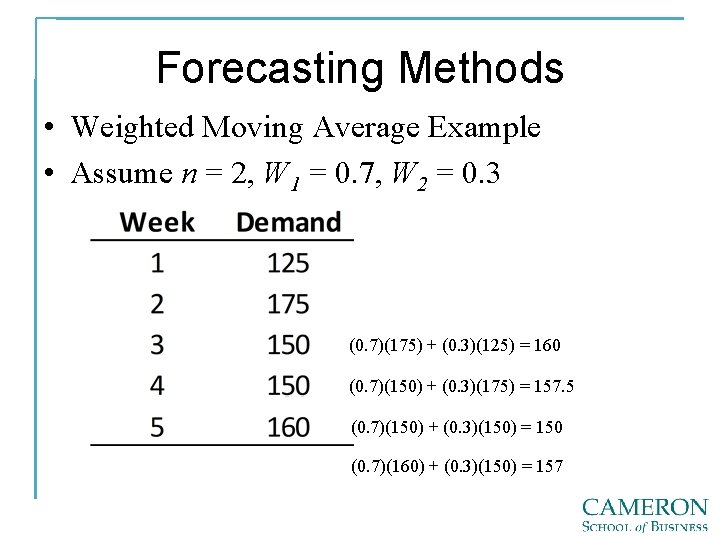
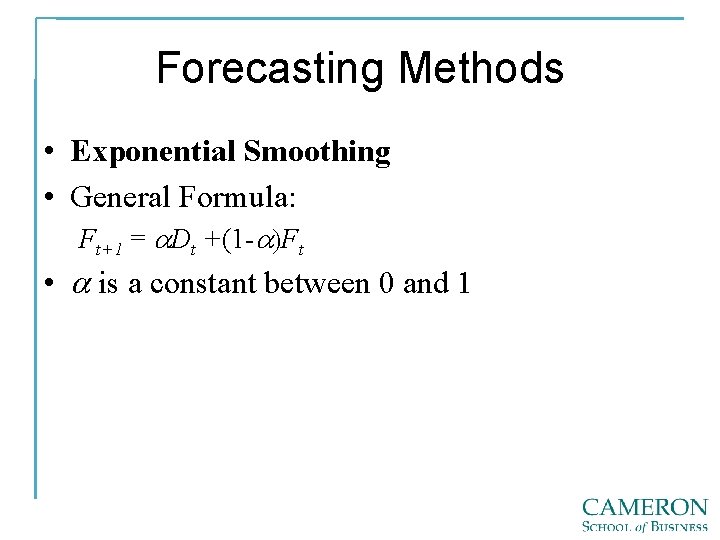
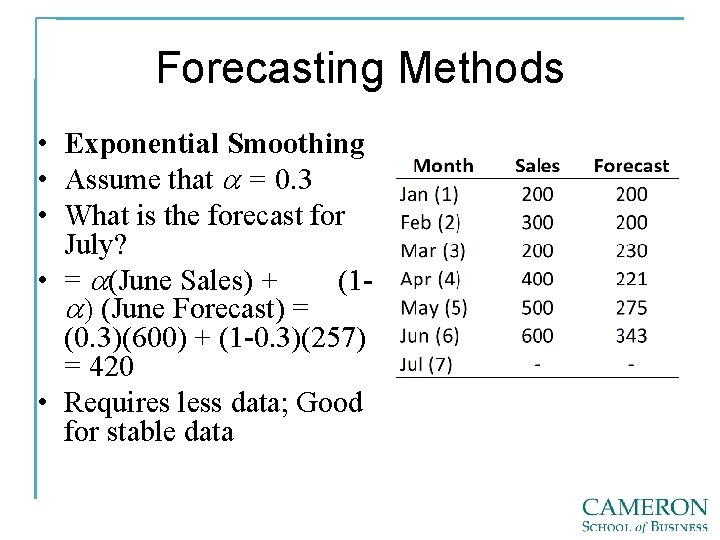
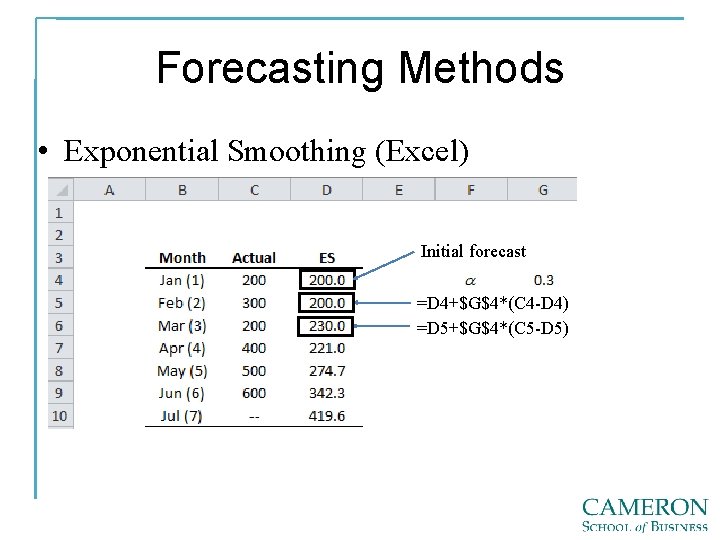
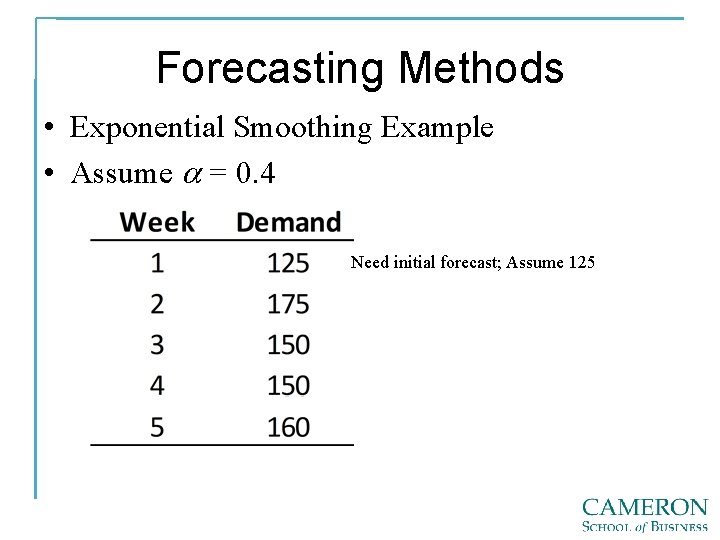
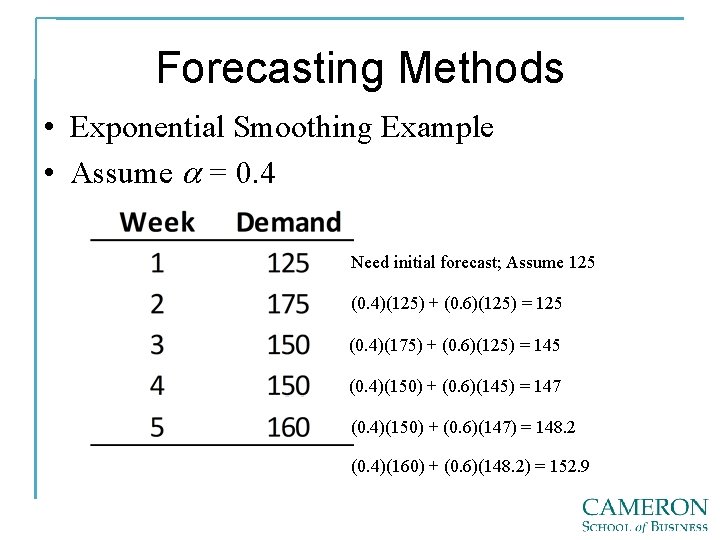
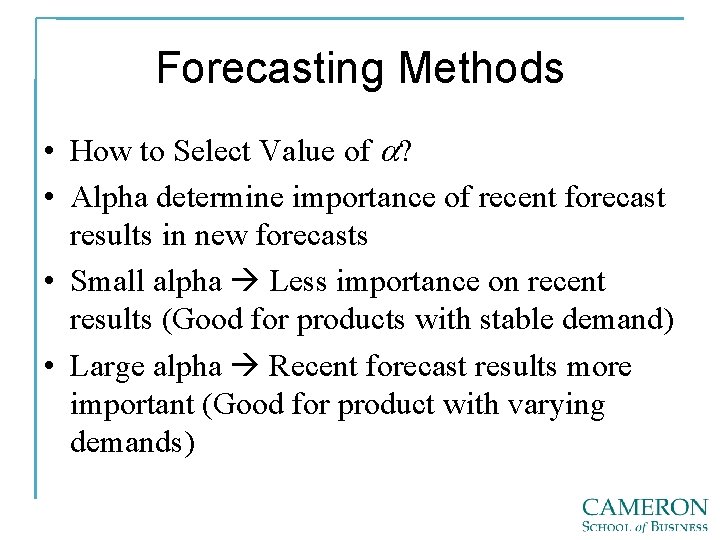
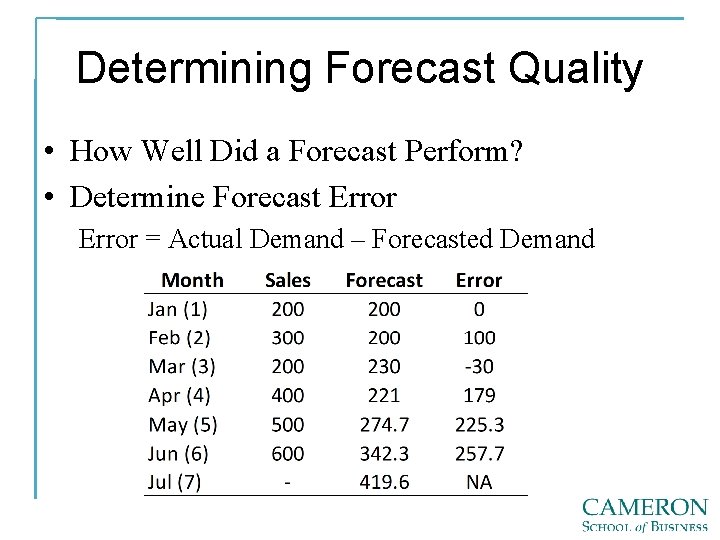
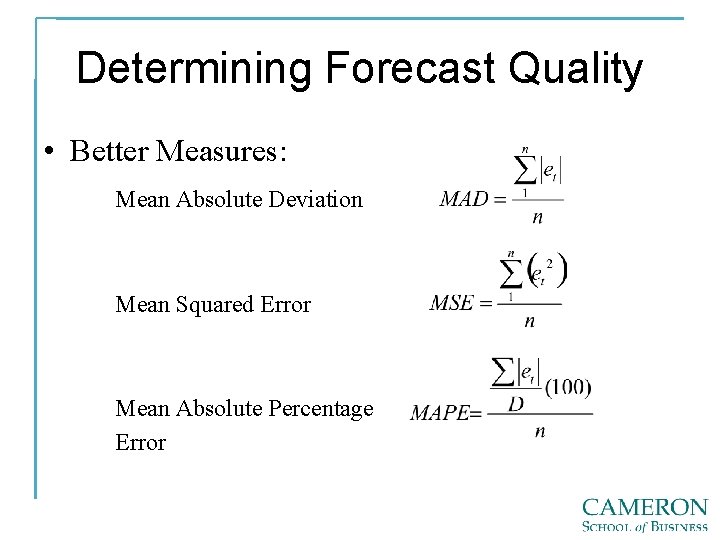
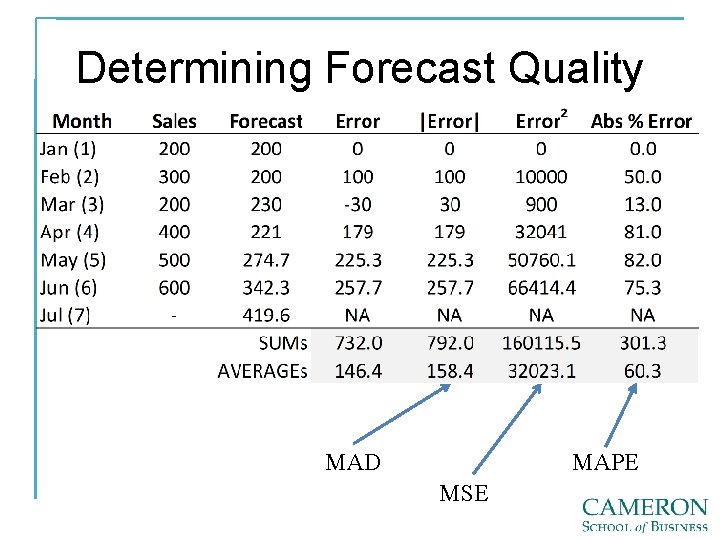
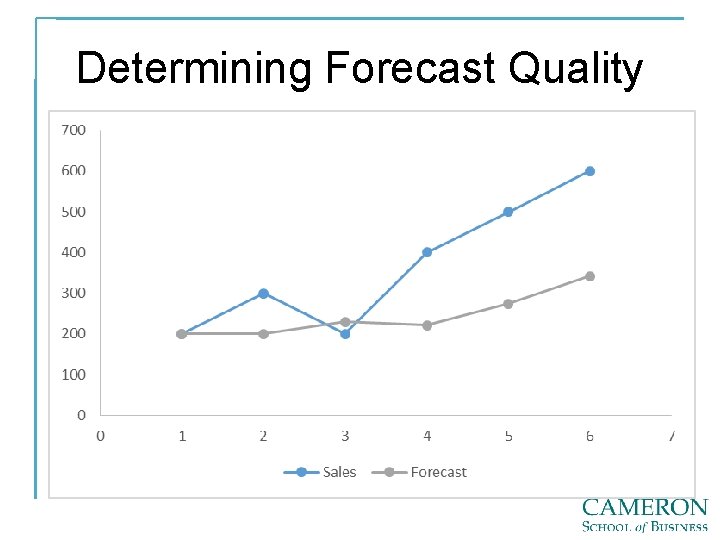
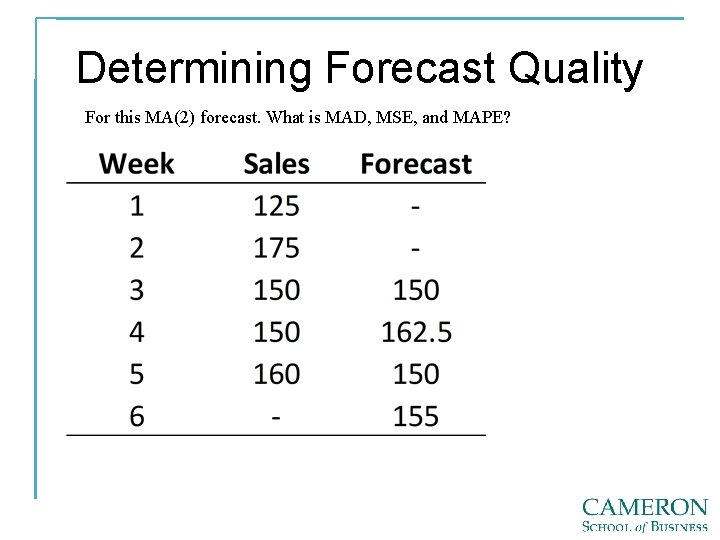
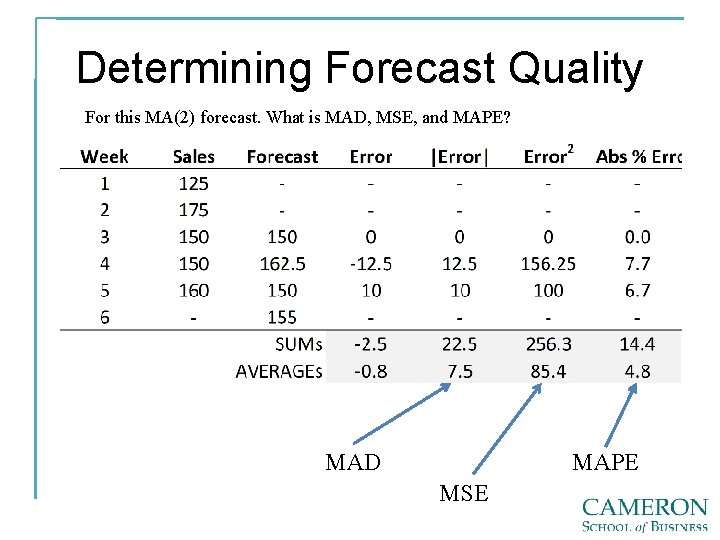
- Slides: 38
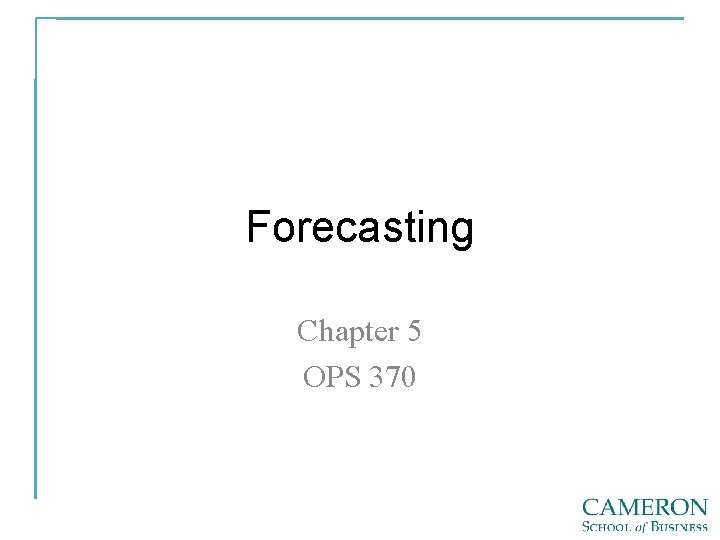
Forecasting Chapter 5 OPS 370
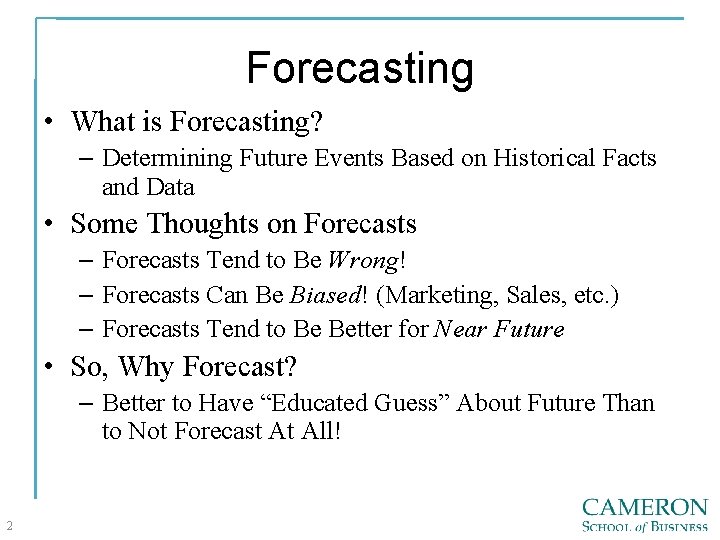
Forecasting • What is Forecasting? – Determining Future Events Based on Historical Facts and Data • Some Thoughts on Forecasts – Forecasts Tend to Be Wrong! – Forecasts Can Be Biased! (Marketing, Sales, etc. ) – Forecasts Tend to Be Better for Near Future • So, Why Forecast? – Better to Have “Educated Guess” About Future Than to Not Forecast At All! 2
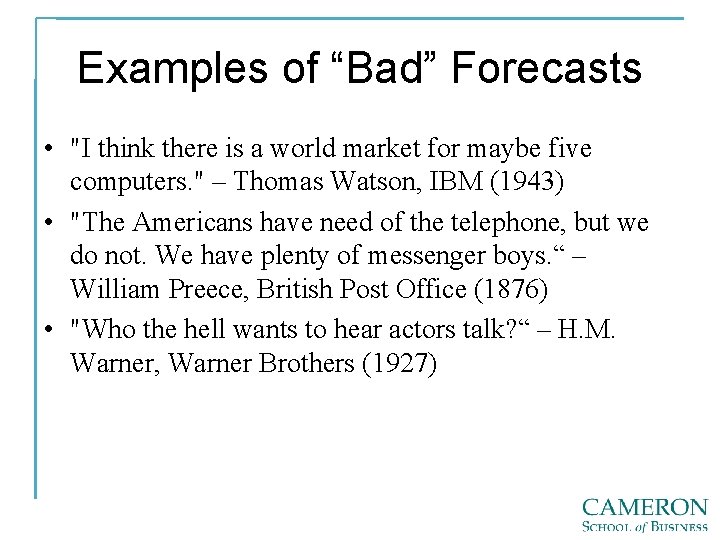
Examples of “Bad” Forecasts • "I think there is a world market for maybe five computers. " – Thomas Watson, IBM (1943) • "The Americans have need of the telephone, but we do not. We have plenty of messenger boys. “ – William Preece, British Post Office (1876) • "Who the hell wants to hear actors talk? “ – H. M. Warner, Warner Brothers (1927)
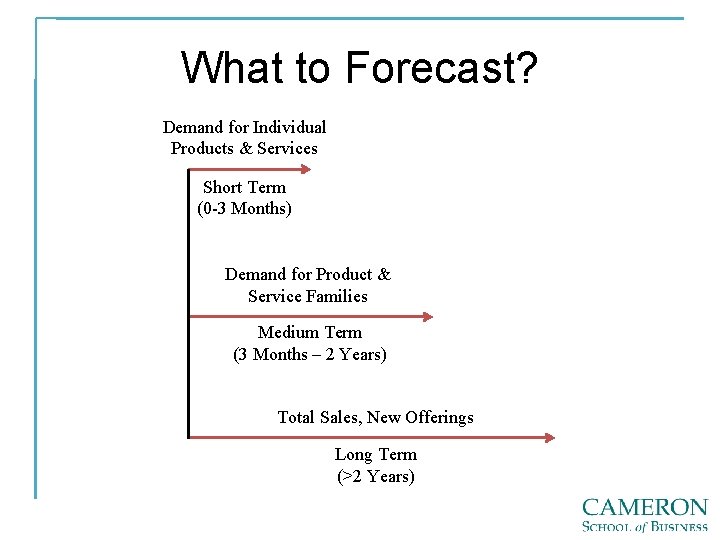
What to Forecast? Demand for Individual Products & Services Short Term (0 -3 Months) Demand for Product & Service Families Medium Term (3 Months – 2 Years) Total Sales, New Offerings Long Term (>2 Years)
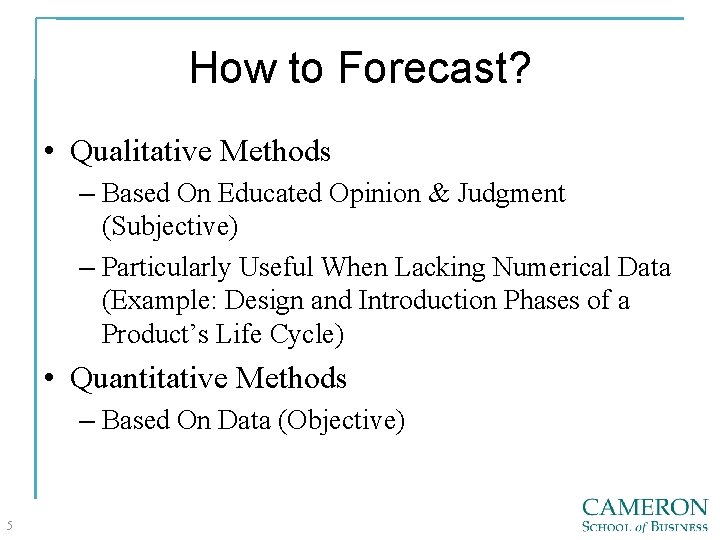
How to Forecast? • Qualitative Methods – Based On Educated Opinion & Judgment (Subjective) – Particularly Useful When Lacking Numerical Data (Example: Design and Introduction Phases of a Product’s Life Cycle) • Quantitative Methods – Based On Data (Objective) 5
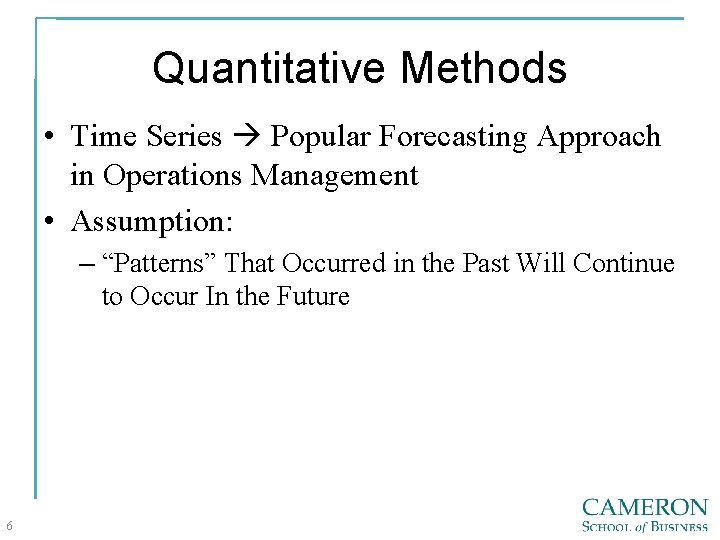
Quantitative Methods • Time Series Popular Forecasting Approach in Operations Management • Assumption: – “Patterns” That Occurred in the Past Will Continue to Occur In the Future 6
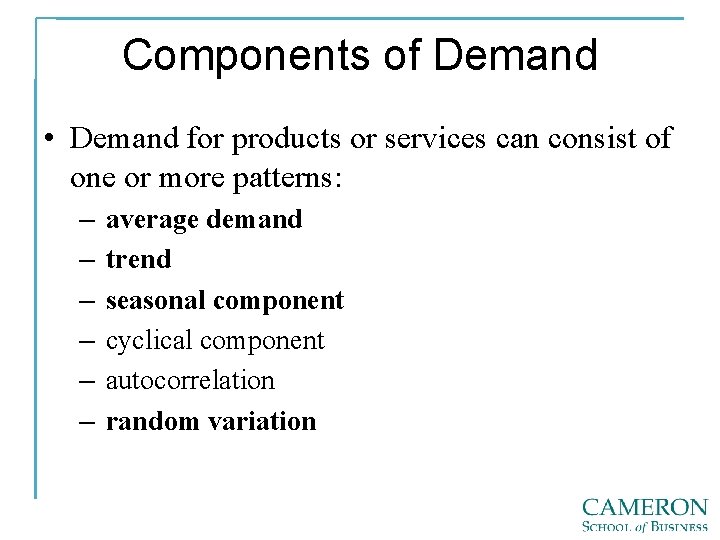
Components of Demand • Demand for products or services can consist of one or more patterns: – – – average demand trend seasonal component cyclical component autocorrelation random variation
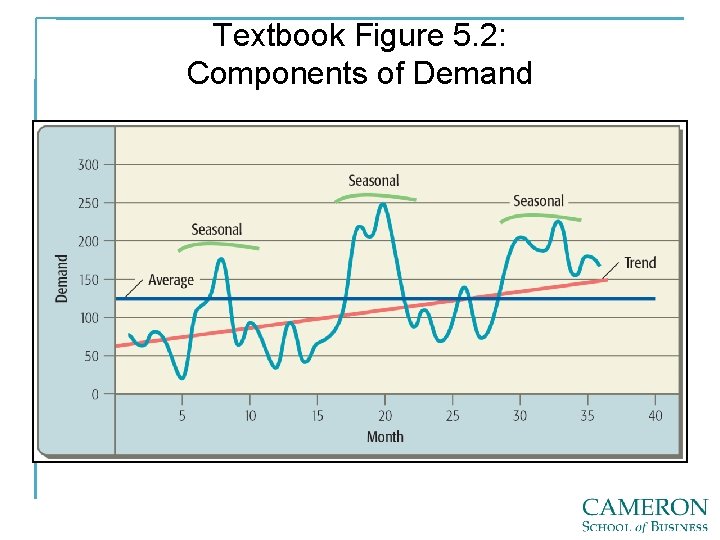
Textbook Figure 5. 2: Components of Demand
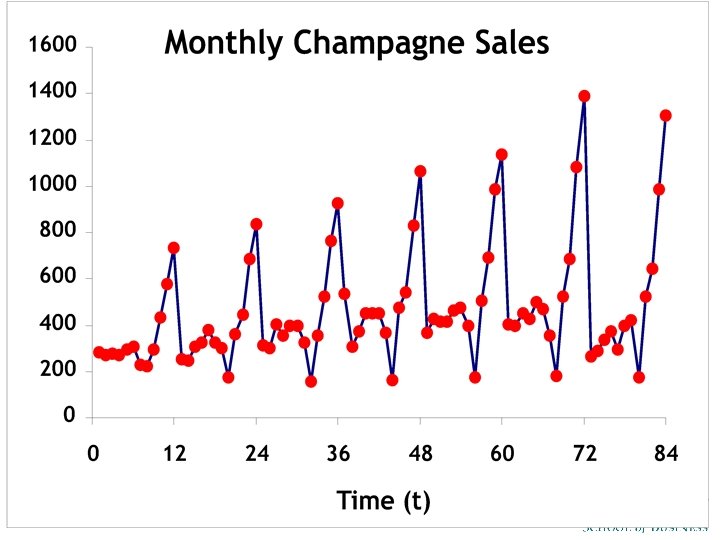
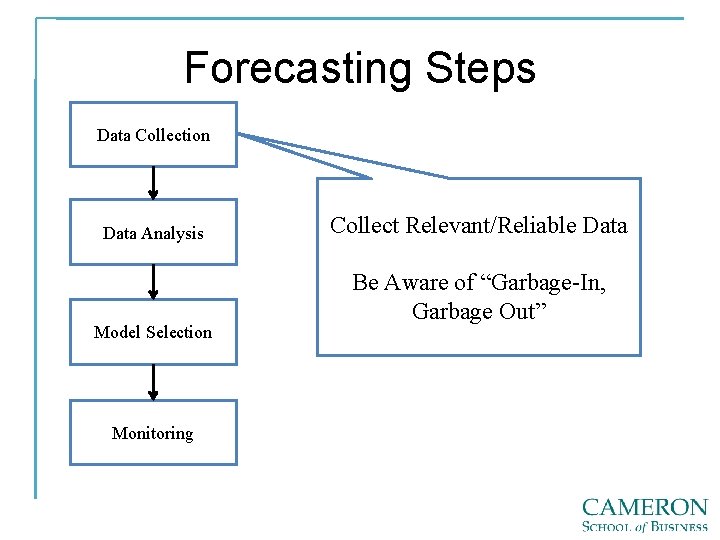
Forecasting Steps Data Collection Data Analysis Model Selection Monitoring Collect Relevant/Reliable Data Be Aware of “Garbage-In, Garbage Out”
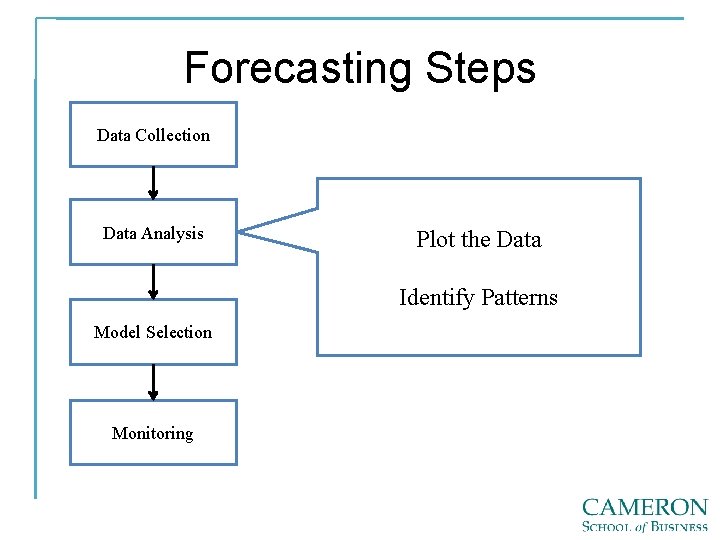
Forecasting Steps Data Collection Data Analysis Plot the Data Identify Patterns Model Selection Monitoring
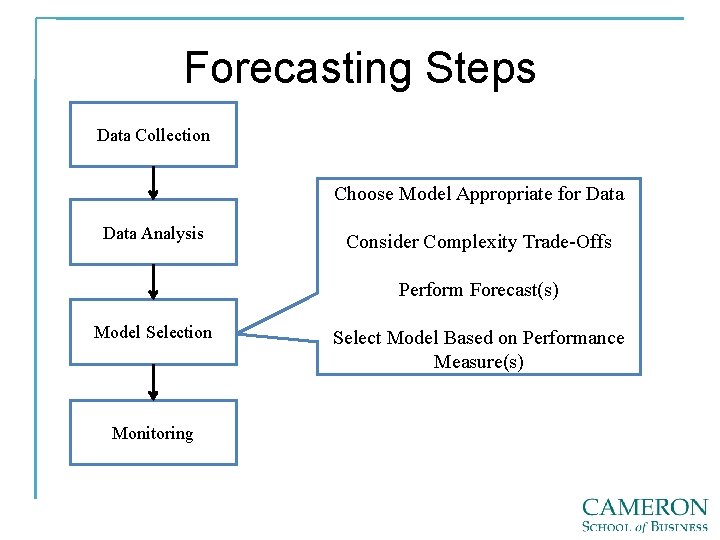
Forecasting Steps Data Collection Choose Model Appropriate for Data Analysis Consider Complexity Trade-Offs Perform Forecast(s) Model Selection Monitoring Select Model Based on Performance Measure(s)
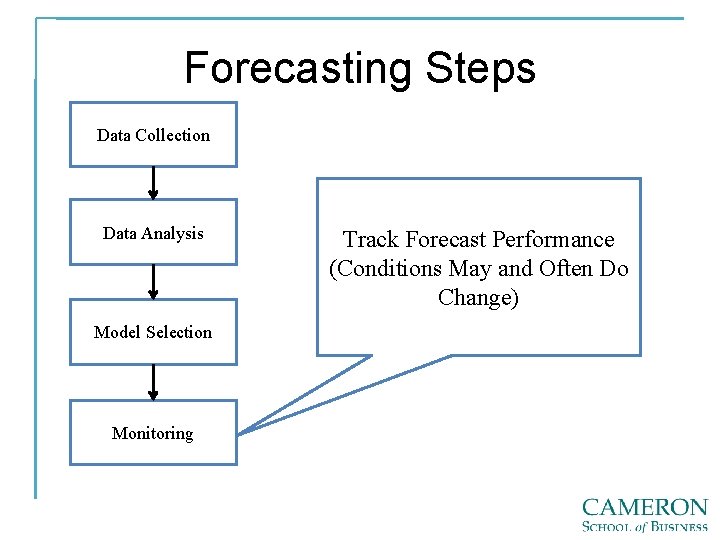
Forecasting Steps Data Collection Data Analysis Model Selection Monitoring Track Forecast Performance (Conditions May and Often Do Change)
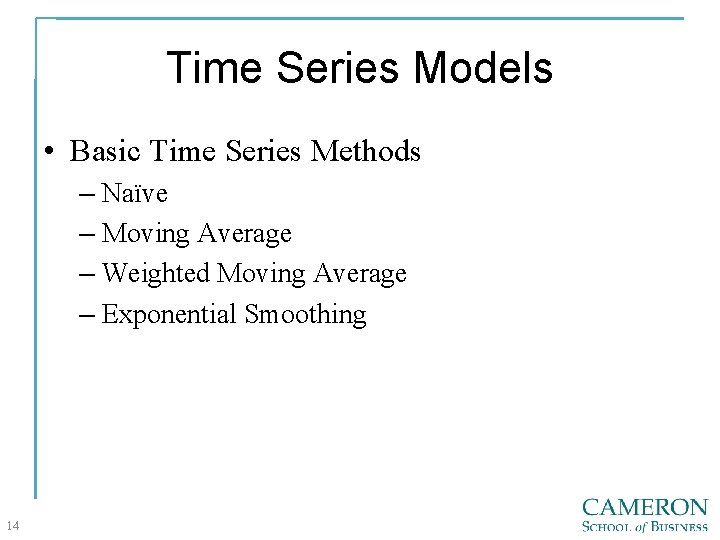
Time Series Models • Basic Time Series Methods – Naïve – Moving Average – Weighted Moving Average – Exponential Smoothing 14
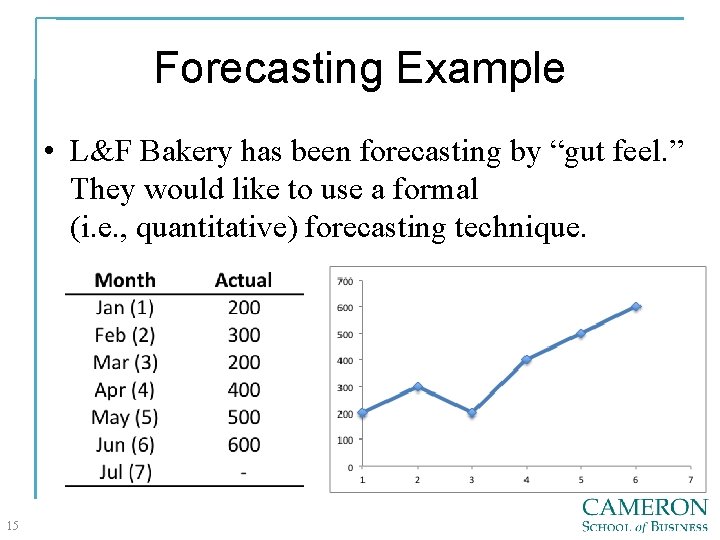
Forecasting Example • L&F Bakery has been forecasting by “gut feel. ” They would like to use a formal (i. e. , quantitative) forecasting technique. 15
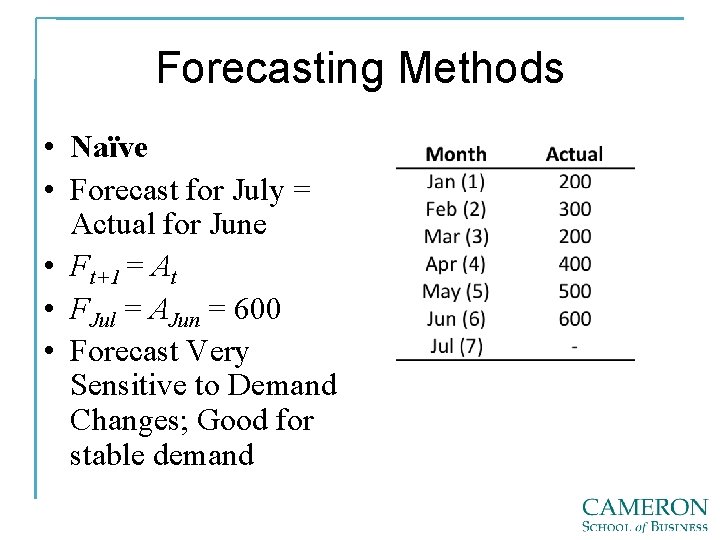
Forecasting Methods • Naïve • Forecast for July = Actual for June • Ft+1 = At • FJul = AJun = 600 • Forecast Very Sensitive to Demand Changes; Good for stable demand
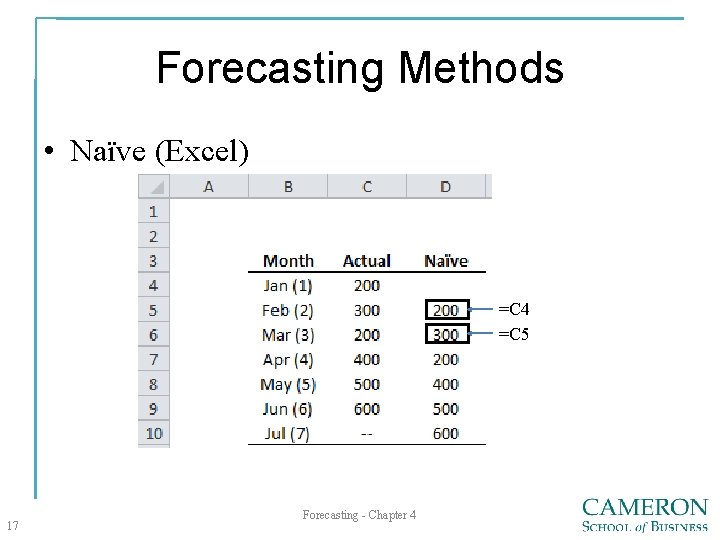
Forecasting Methods • Naïve (Excel) =C 4 =C 5 17 Forecasting - Chapter 4
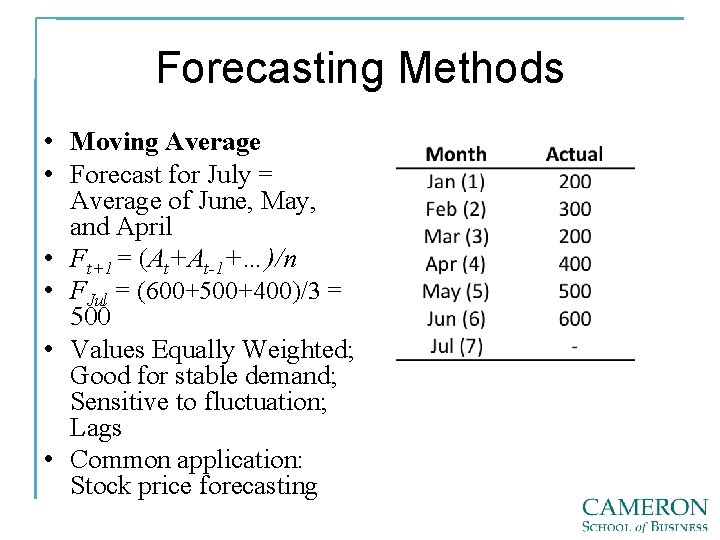
Forecasting Methods • Moving Average • Forecast for July = Average of June, May, and April • Ft+1 = (At+At-1+…)/n • FJul = (600+500+400)/3 = 500 • Values Equally Weighted; Good for stable demand; Sensitive to fluctuation; Lags • Common application: Stock price forecasting
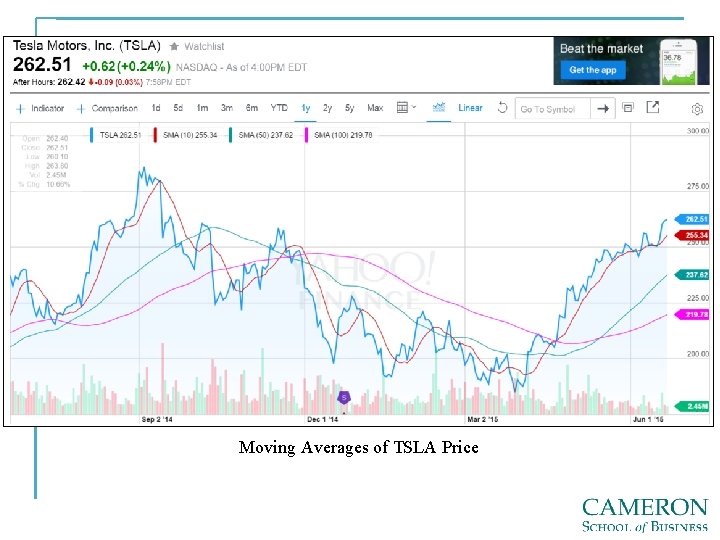
Moving Averages of TSLA Price
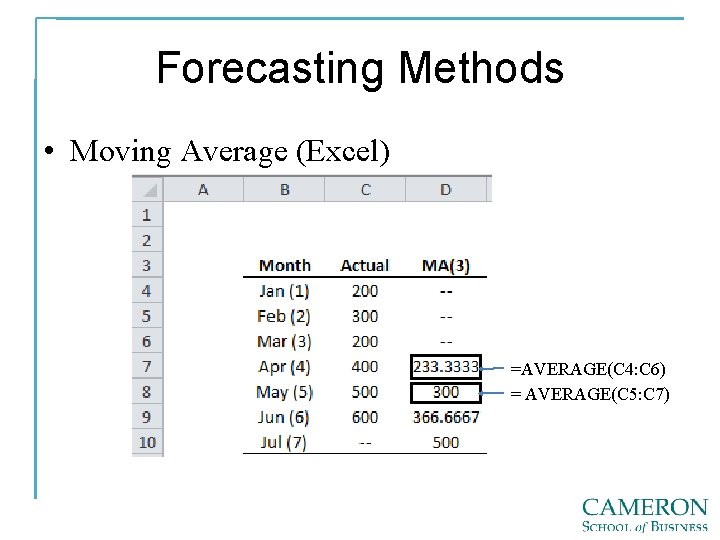
Forecasting Methods • Moving Average (Excel) =AVERAGE(C 4: C 6) = AVERAGE(C 5: C 7)
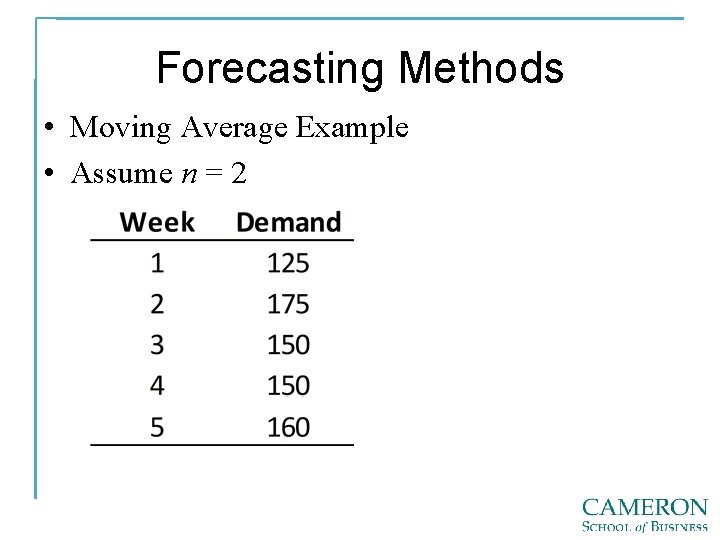
Forecasting Methods • Moving Average Example • Assume n = 2
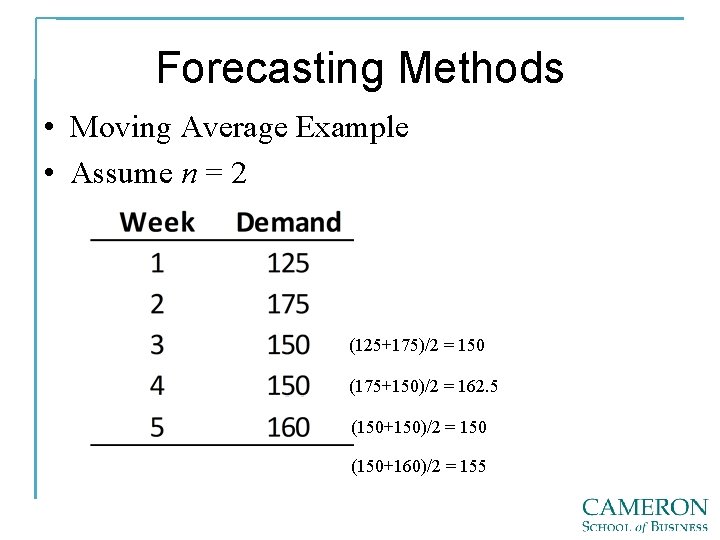
Forecasting Methods • Moving Average Example • Assume n = 2 (125+175)/2 = 150 (175+150)/2 = 162. 5 (150+150)/2 = 150 (150+160)/2 = 155
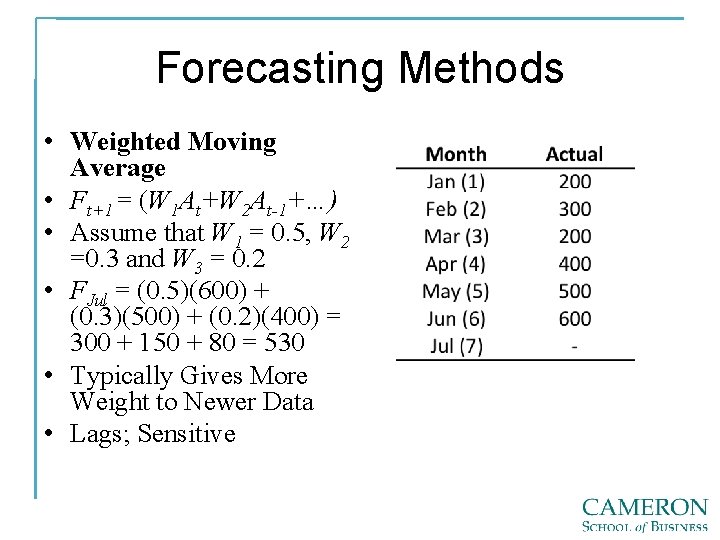
Forecasting Methods • Weighted Moving Average • Ft+1 = (W 1 At+W 2 At-1+…) • Assume that W 1 = 0. 5, W 2 =0. 3 and W 3 = 0. 2 • FJul = (0. 5)(600) + (0. 3)(500) + (0. 2)(400) = 300 + 150 + 80 = 530 • Typically Gives More Weight to Newer Data • Lags; Sensitive
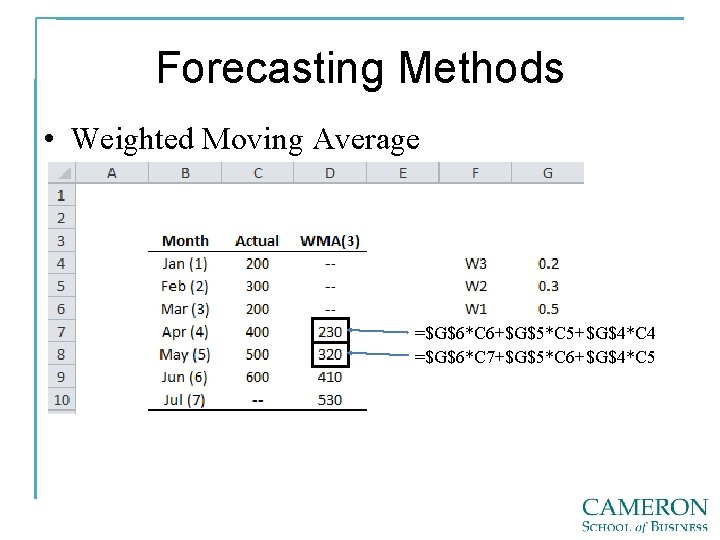
Forecasting Methods • Weighted Moving Average =$G$6*C 6+$G$5*C 5+$G$4*C 4 =$G$6*C 7+$G$5*C 6+$G$4*C 5
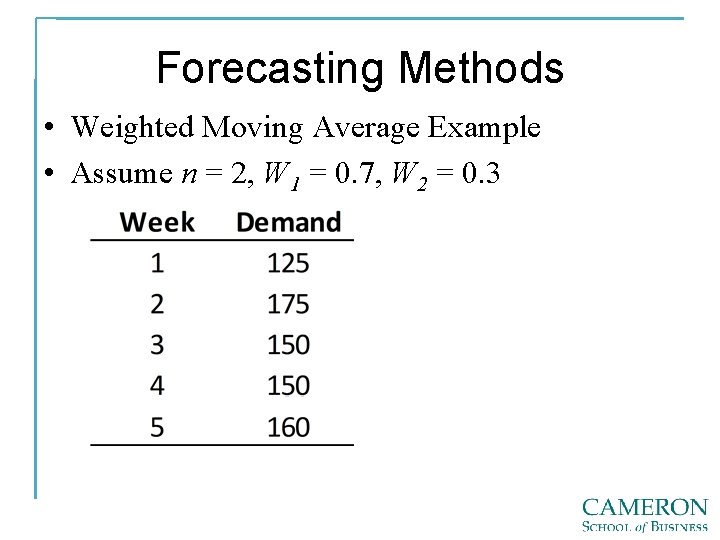
Forecasting Methods • Weighted Moving Average Example • Assume n = 2, W 1 = 0. 7, W 2 = 0. 3
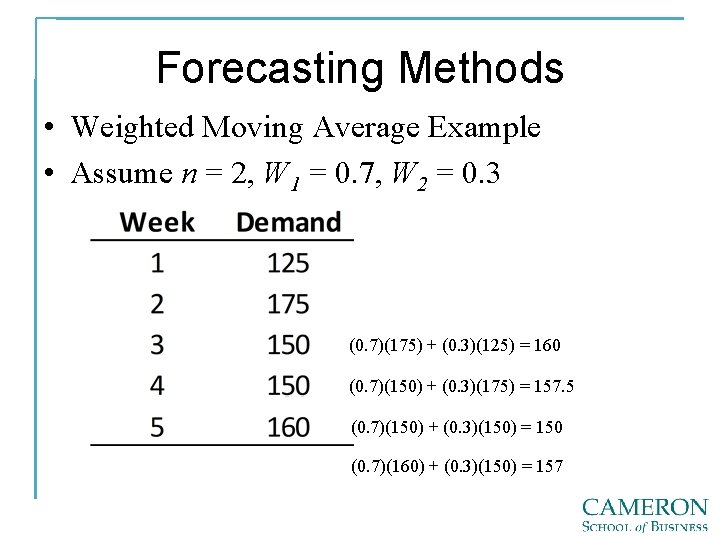
Forecasting Methods • Weighted Moving Average Example • Assume n = 2, W 1 = 0. 7, W 2 = 0. 3 (0. 7)(175) + (0. 3)(125) = 160 (0. 7)(150) + (0. 3)(175) = 157. 5 (0. 7)(150) + (0. 3)(150) = 150 (0. 7)(160) + (0. 3)(150) = 157
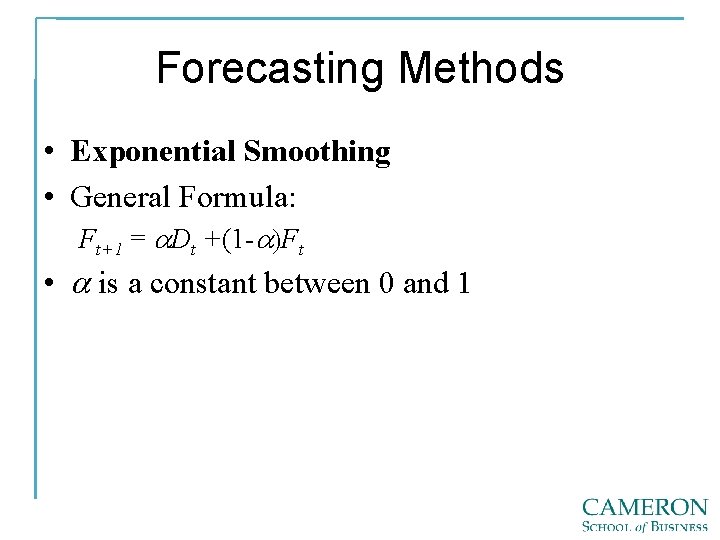
Forecasting Methods • Exponential Smoothing • General Formula: Ft+1 = a. Dt +(1 -a)Ft • a is a constant between 0 and 1
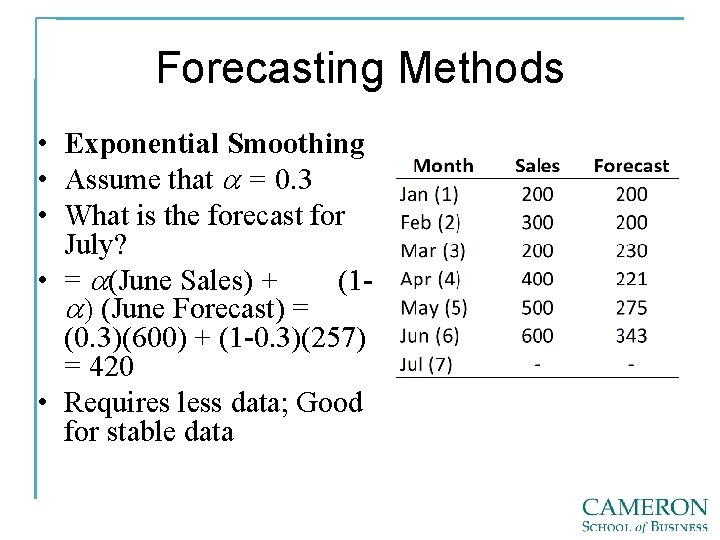
Forecasting Methods • Exponential Smoothing • Assume that a = 0. 3 • What is the forecast for July? • = a(June Sales) + (1 a) (June Forecast) = (0. 3)(600) + (1 -0. 3)(257) = 420 • Requires less data; Good for stable data
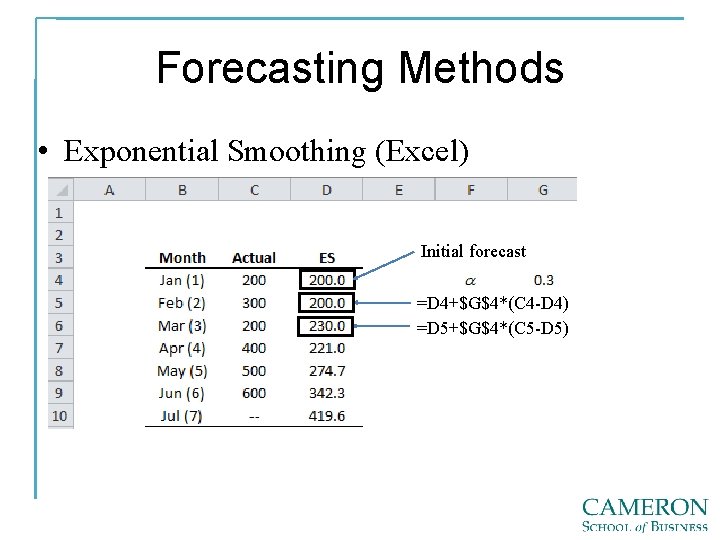
Forecasting Methods • Exponential Smoothing (Excel) Initial forecast =D 4+$G$4*(C 4 -D 4) =D 5+$G$4*(C 5 -D 5)
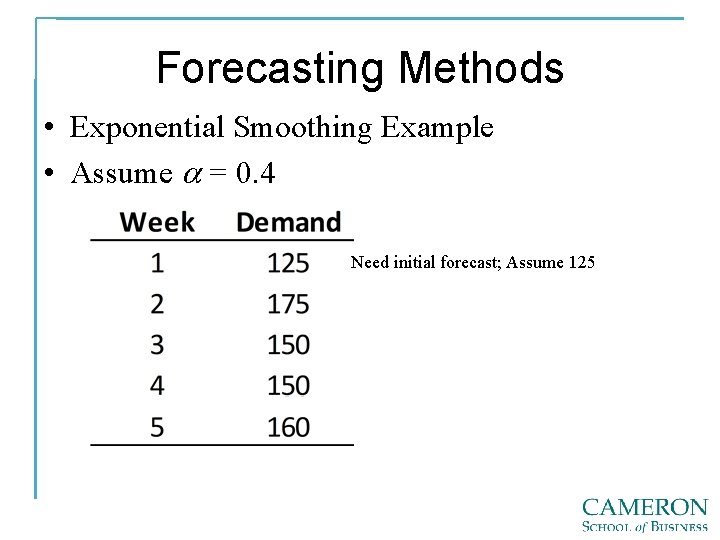
Forecasting Methods • Exponential Smoothing Example • Assume a = 0. 4 Need initial forecast; Assume 125
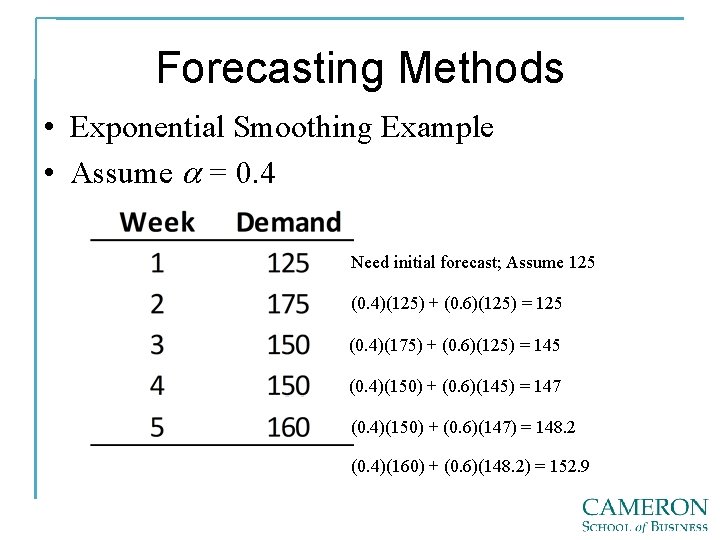
Forecasting Methods • Exponential Smoothing Example • Assume a = 0. 4 Need initial forecast; Assume 125 (0. 4)(125) + (0. 6)(125) = 125 (0. 4)(175) + (0. 6)(125) = 145 (0. 4)(150) + (0. 6)(145) = 147 (0. 4)(150) + (0. 6)(147) = 148. 2 (0. 4)(160) + (0. 6)(148. 2) = 152. 9
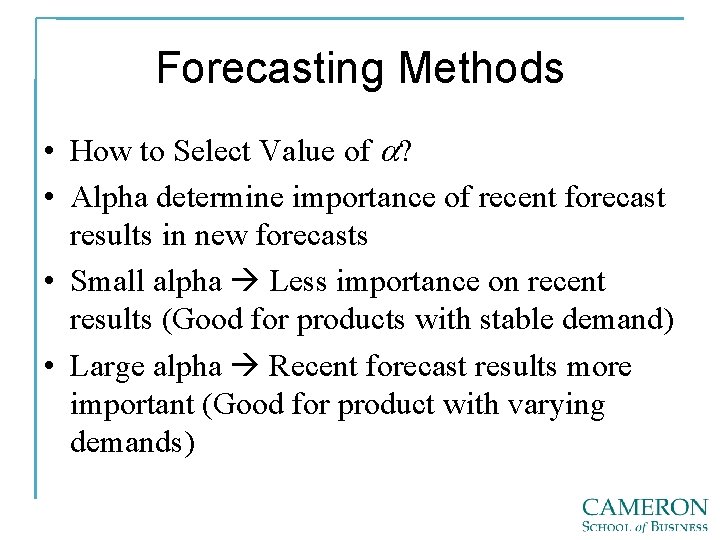
Forecasting Methods • How to Select Value of a? • Alpha determine importance of recent forecast results in new forecasts • Small alpha Less importance on recent results (Good for products with stable demand) • Large alpha Recent forecast results more important (Good for product with varying demands)
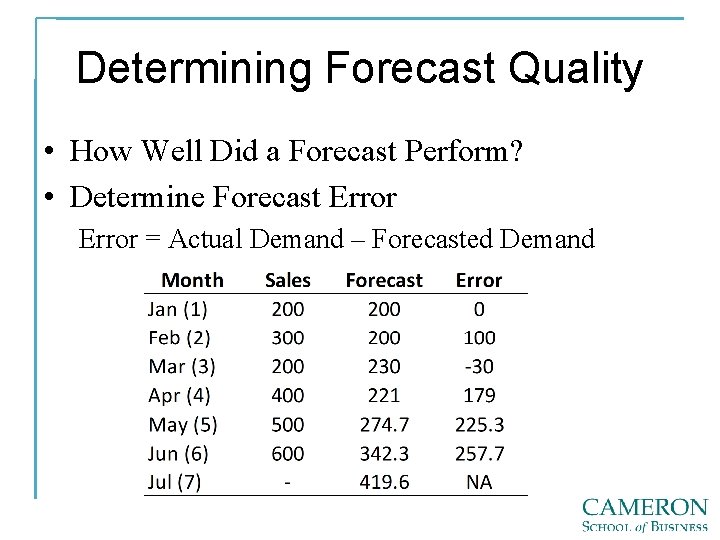
Determining Forecast Quality • How Well Did a Forecast Perform? • Determine Forecast Error = Actual Demand – Forecasted Demand
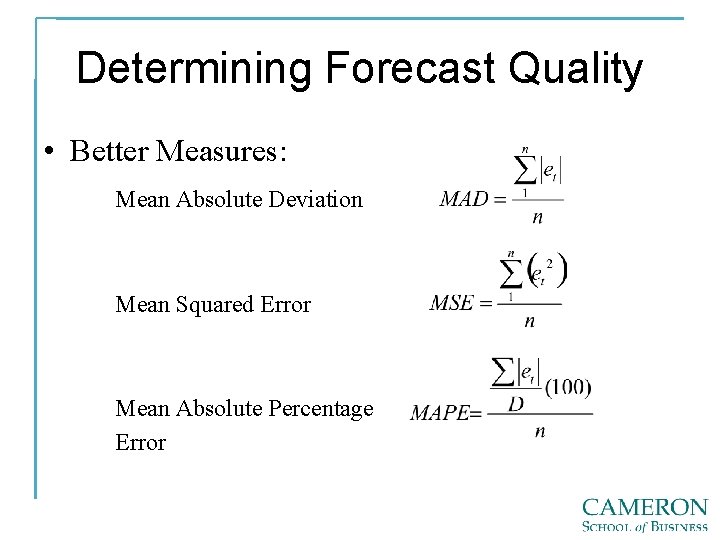
Determining Forecast Quality • Better Measures: Mean Absolute Deviation Mean Squared Error Mean Absolute Percentage Error
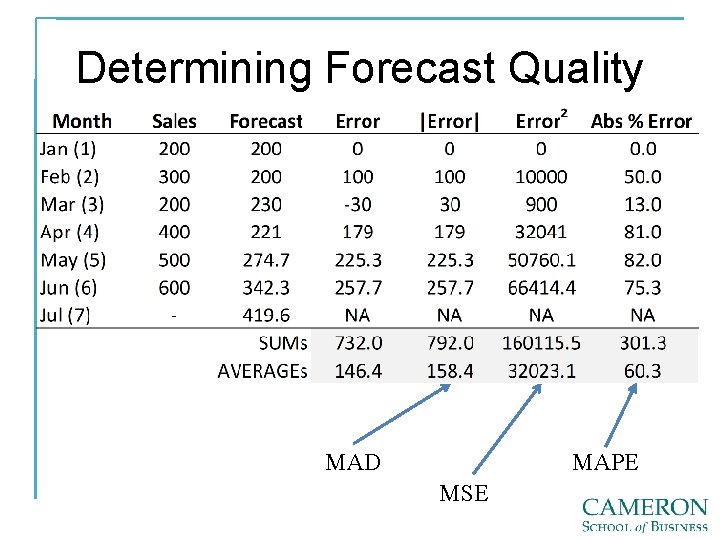
Determining Forecast Quality MAD MAPE MSE
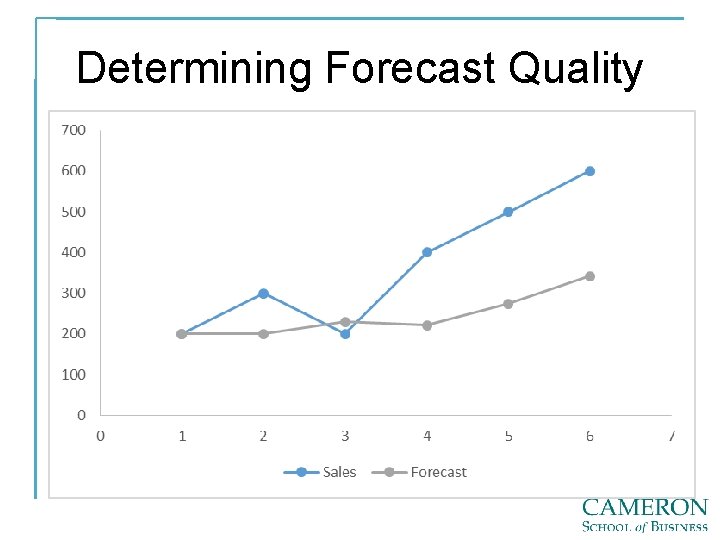
Determining Forecast Quality
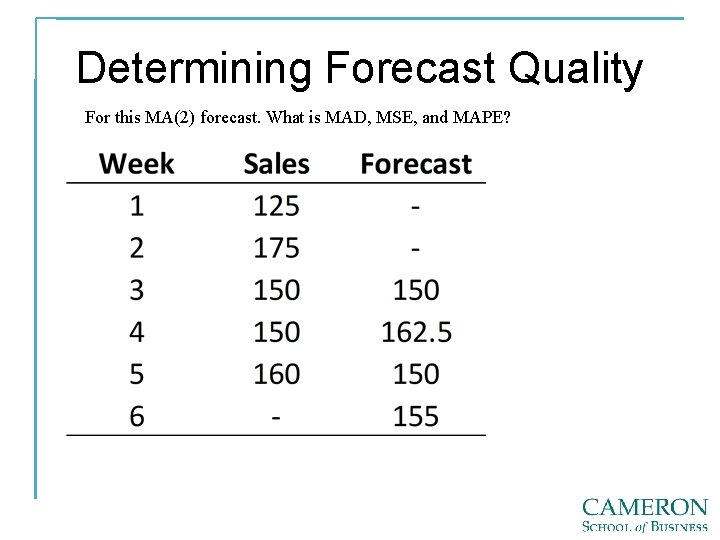
Determining Forecast Quality For this MA(2) forecast. What is MAD, MSE, and MAPE?
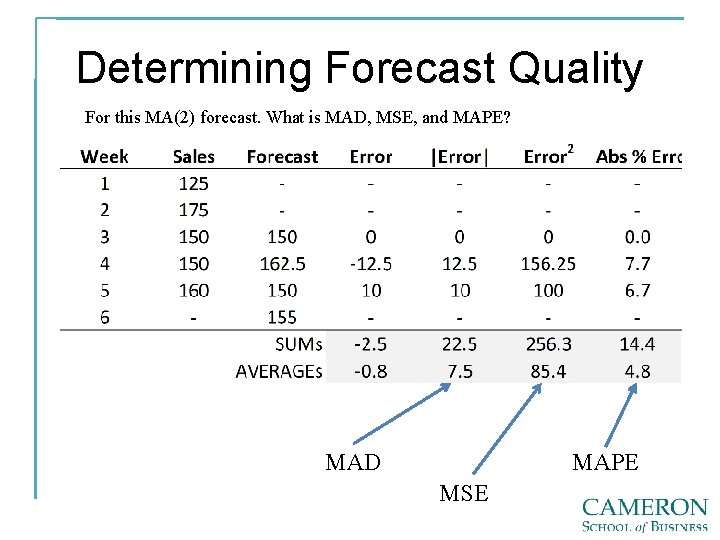
Determining Forecast Quality For this MA(2) forecast. What is MAD, MSE, and MAPE? MAD MAPE MSE
Eecs 496 umich
Ibm 360 instruction format
Ese 370
Ese 370
Ese 370
Ese 370
Xkcd
Eecs 370
Eecs 370 project 4
Acq 370
Ese 370
Ese 370
Www.xkcd.com
Eecs 370 curve
Cmpt 370
Csc 370
Csc 370
Ese 370
Ese 370
Eecs 370 project 2
649 en yakın yüzlük
Eecs 370 curve
866-370-3040
Democritus theory of the universe
Eecs370
Isy 370
Amd opteron
Ibm 360 series
Csc 370
Acq 370
Perwabkeu
Ops technology sign up
How to occupy a patrol base
Susunan organisasi polda
Omaha public school transportation
Pansops
Terps vs pans ops
Struktur biro sdm polda
Ops operating system