EE 150 a Genomic Signal and Information Processing
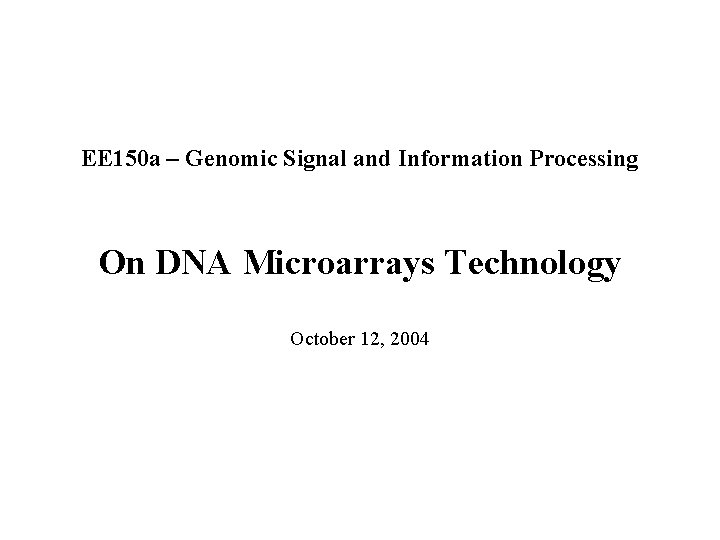
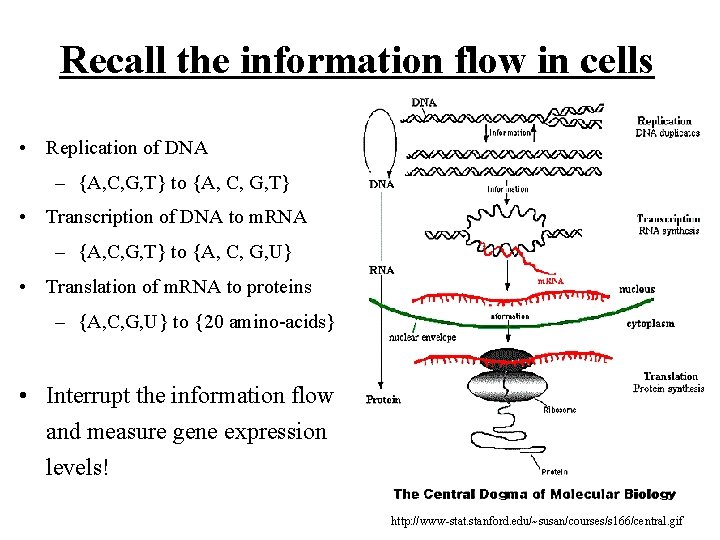
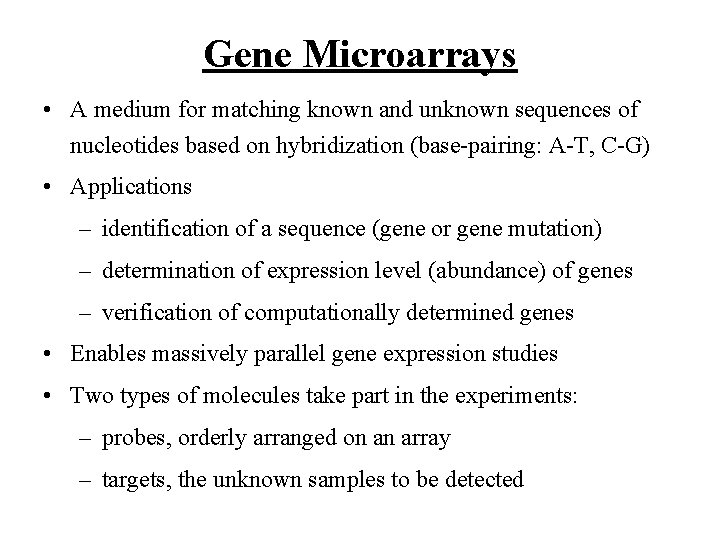
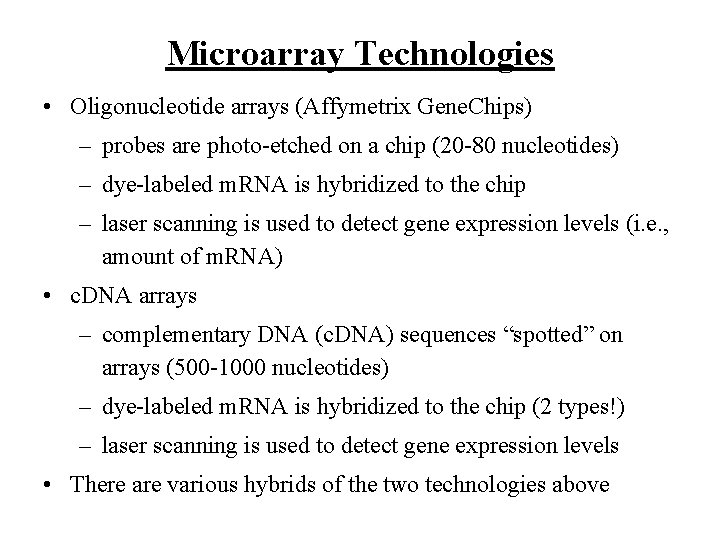
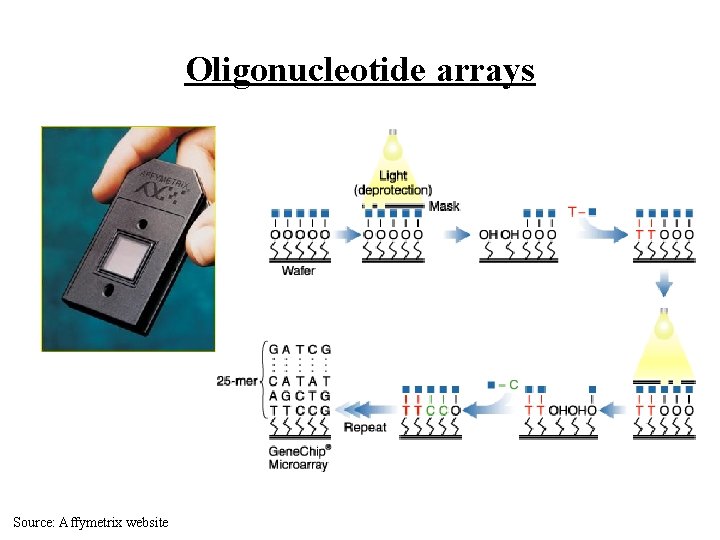
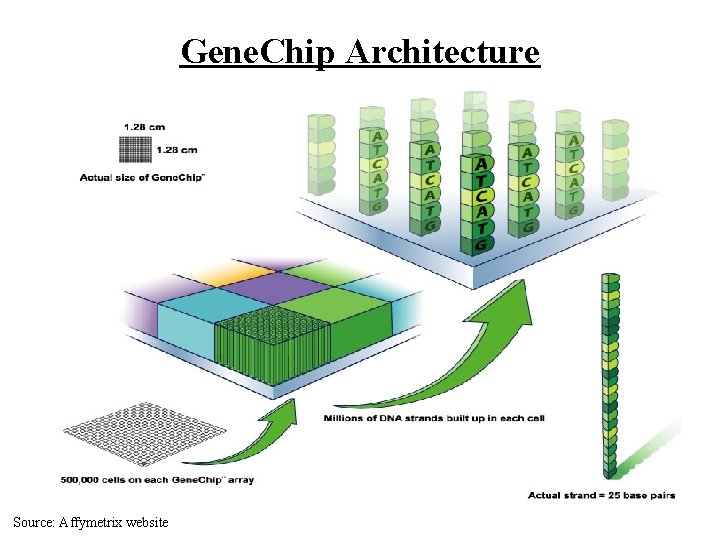
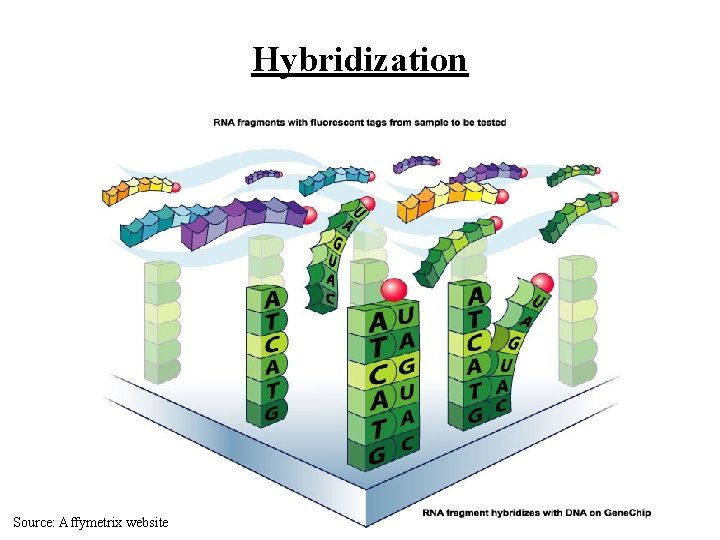
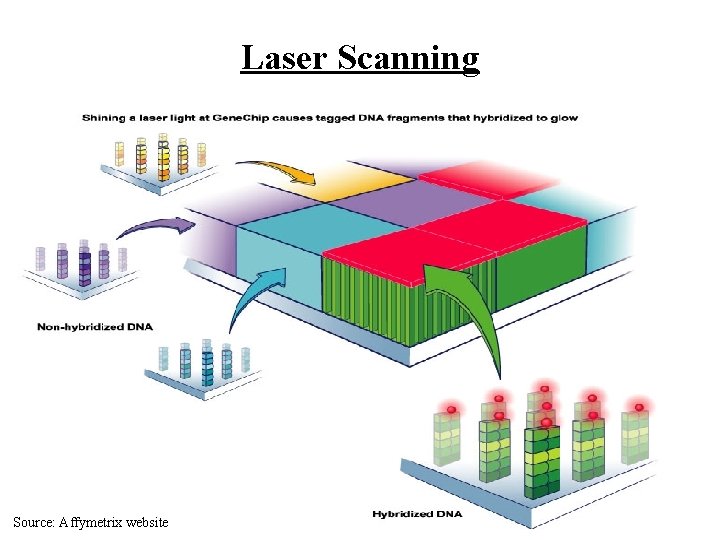
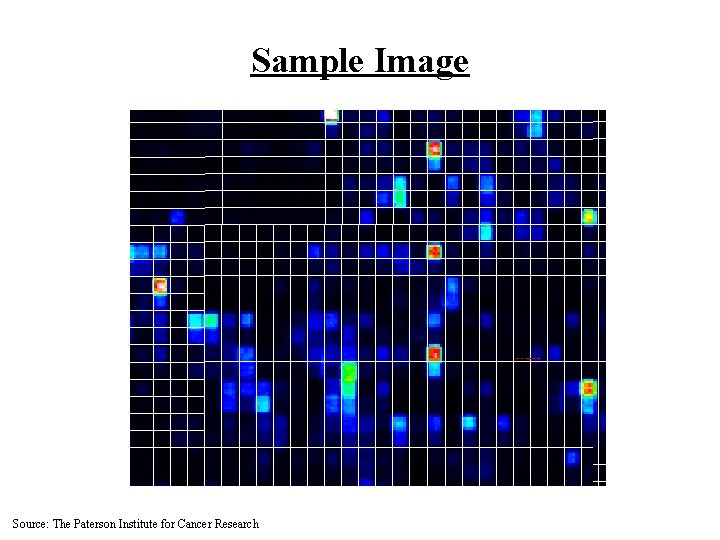
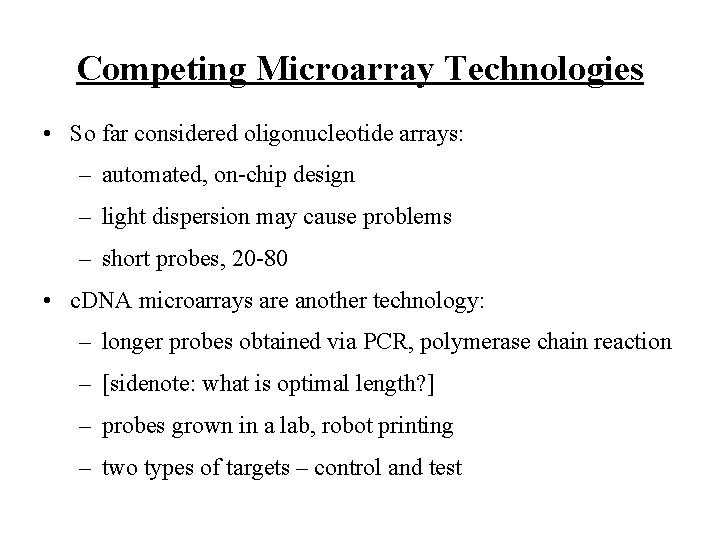
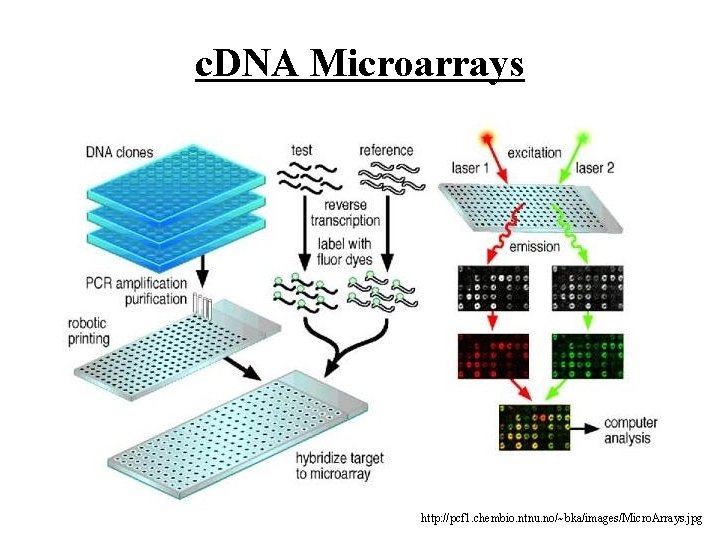
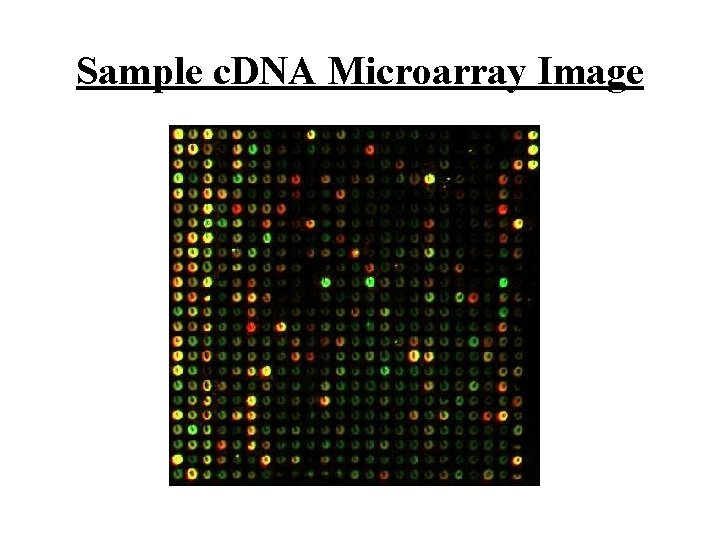
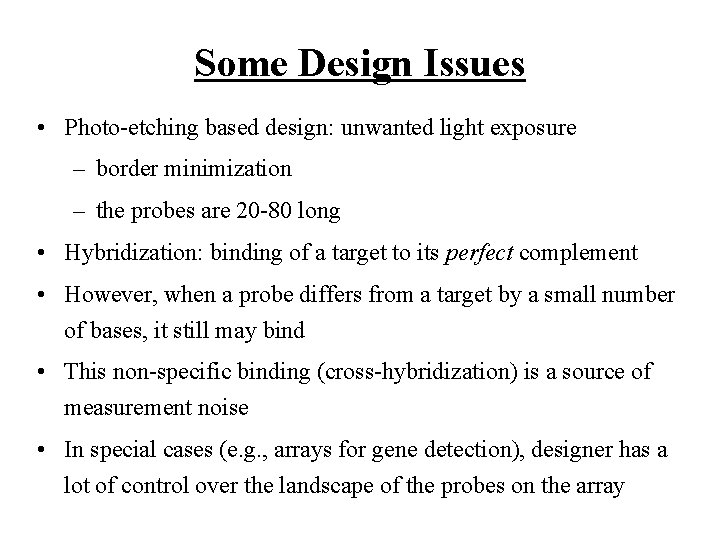
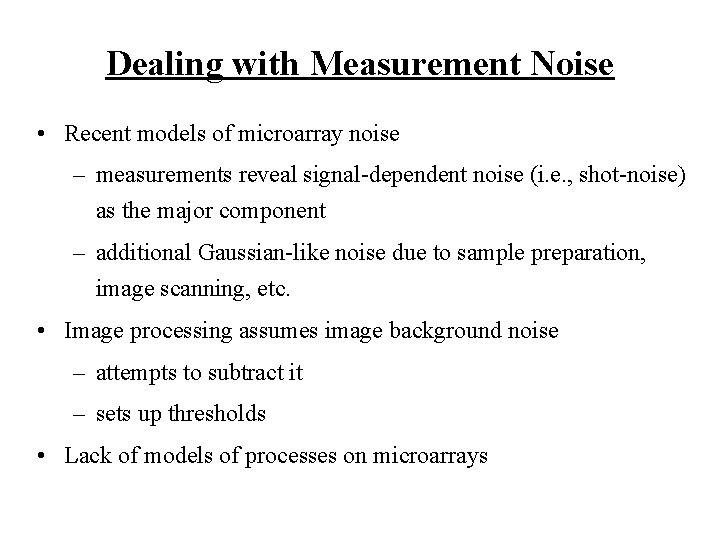
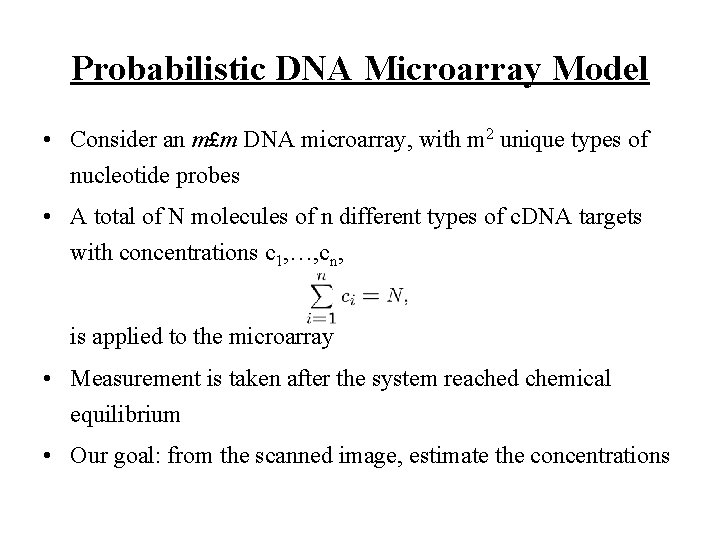
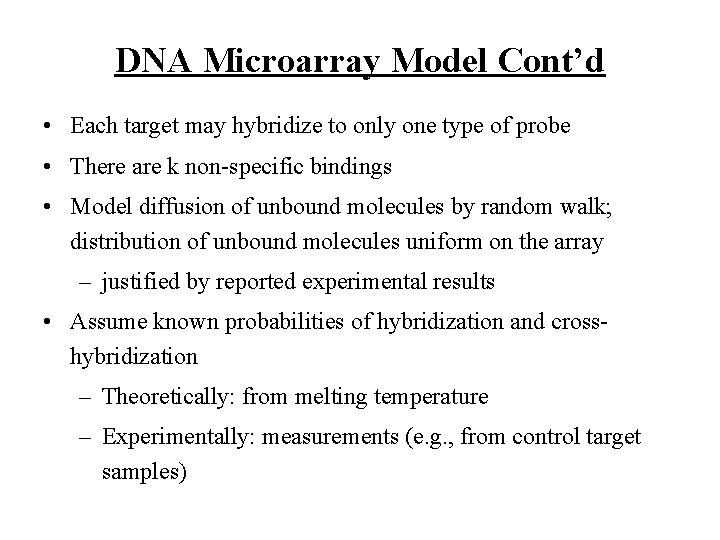
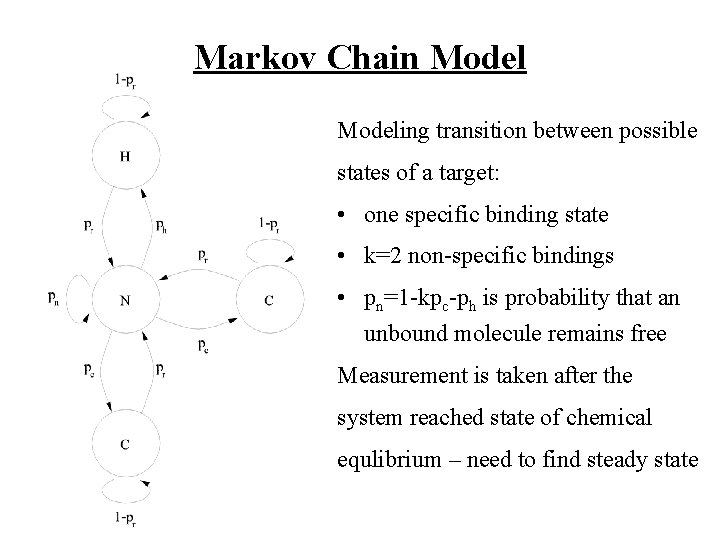
![Markov Chain Model Cont’d Let i=[ i, 1 i, 2 … i, k+2]T be Markov Chain Model Cont’d Let i=[ i, 1 i, 2 … i, k+2]T be](https://slidetodoc.com/presentation_image_h2/cfe2e81a85c82c4596dc9543ab723ac7/image-18.jpg)
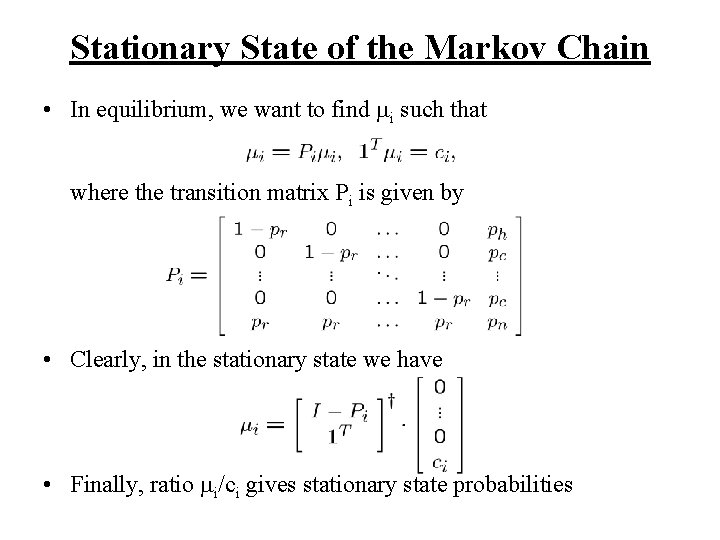
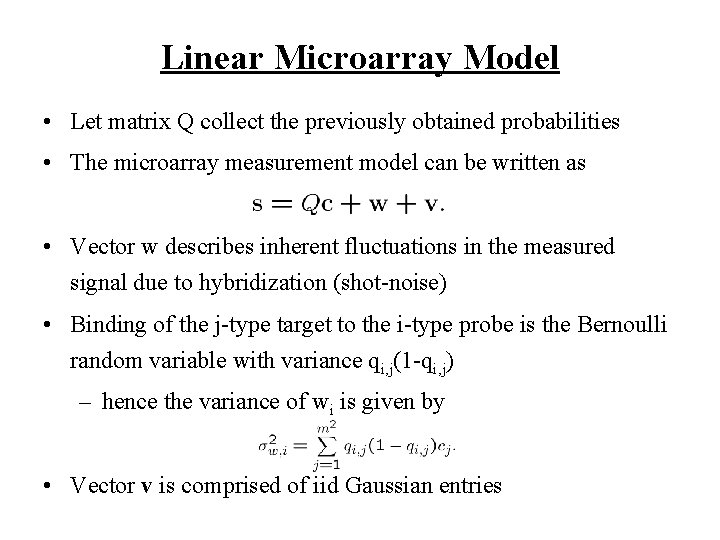
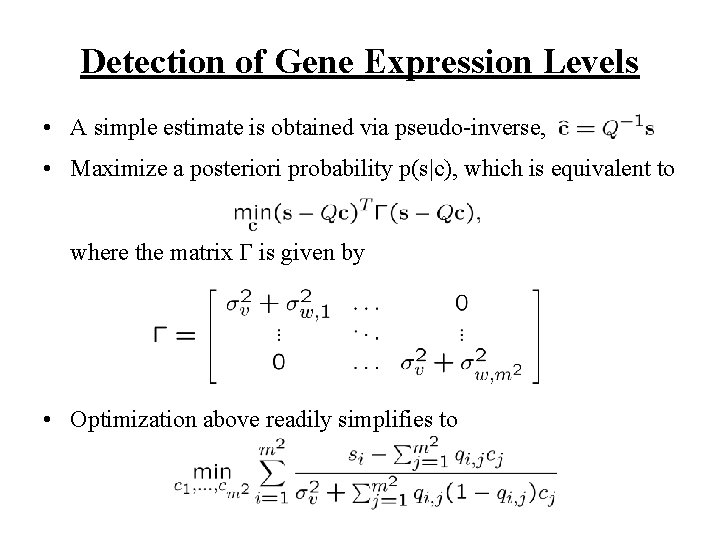
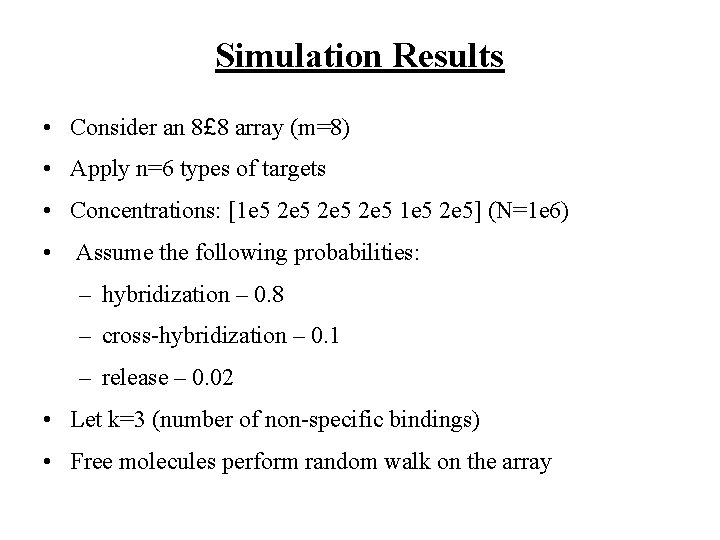
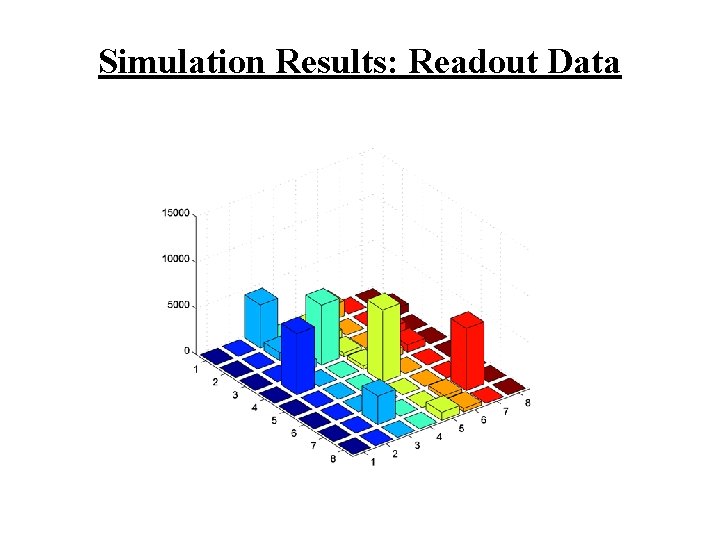
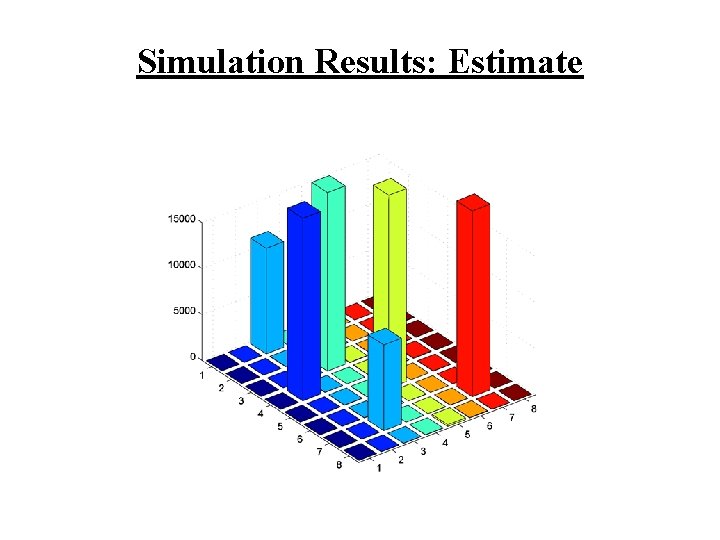
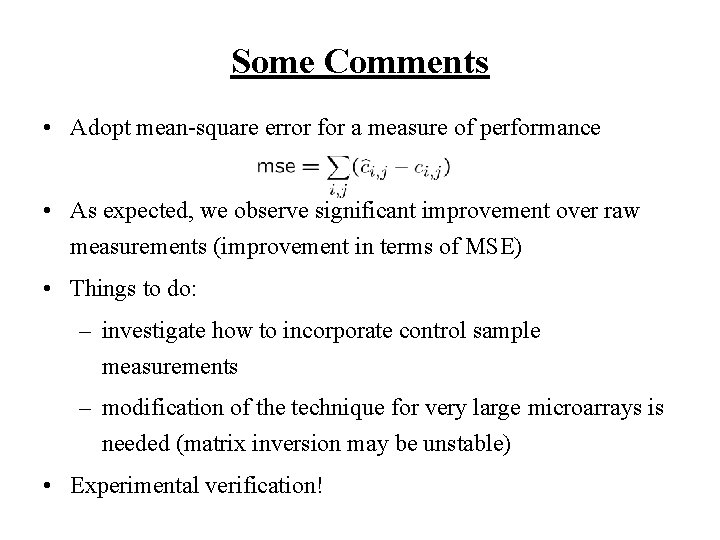
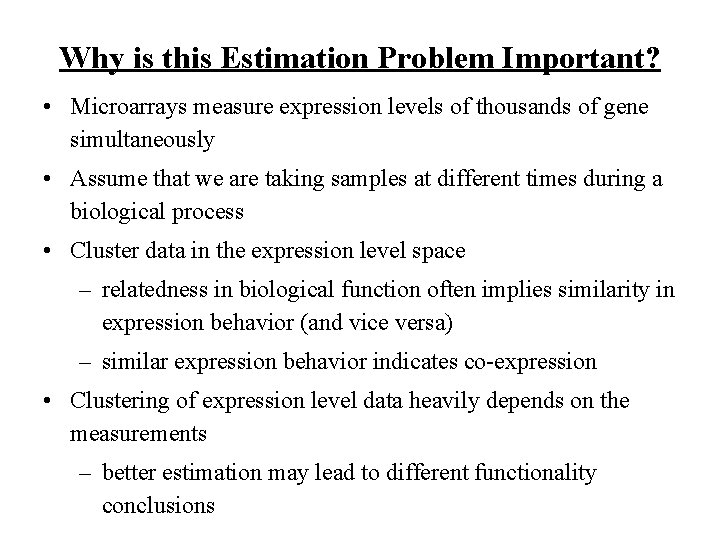
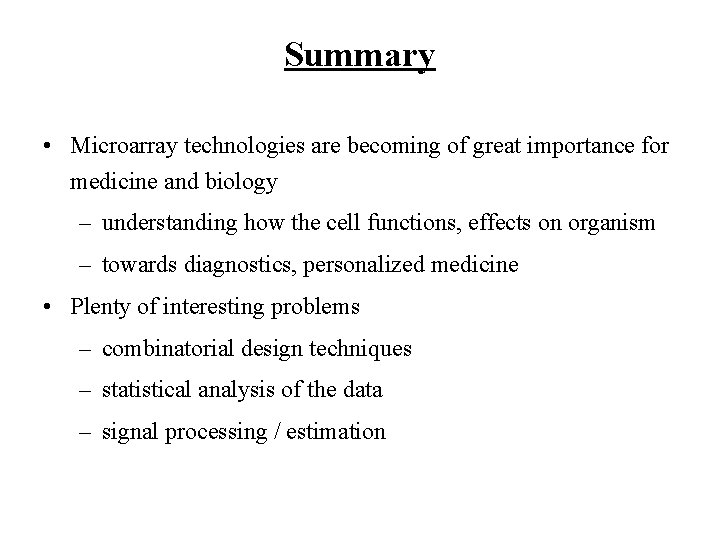
- Slides: 27
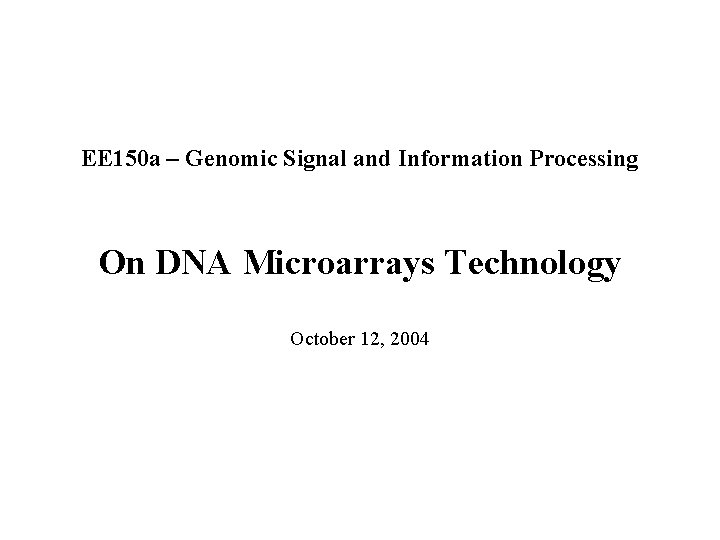
EE 150 a – Genomic Signal and Information Processing On DNA Microarrays Technology October 12, 2004
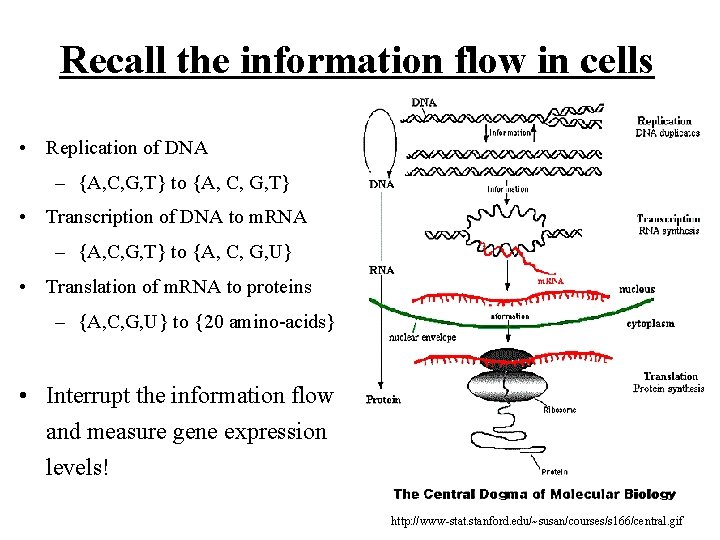
Recall the information flow in cells • Replication of DNA – {A, C, G, T} to {A, C, G, T} • Transcription of DNA to m. RNA – {A, C, G, T} to {A, C, G, U} • Translation of m. RNA to proteins – {A, C, G, U} to {20 amino-acids} • Interrupt the information flow and measure gene expression levels! http: //www-stat. stanford. edu/~susan/courses/s 166/central. gif
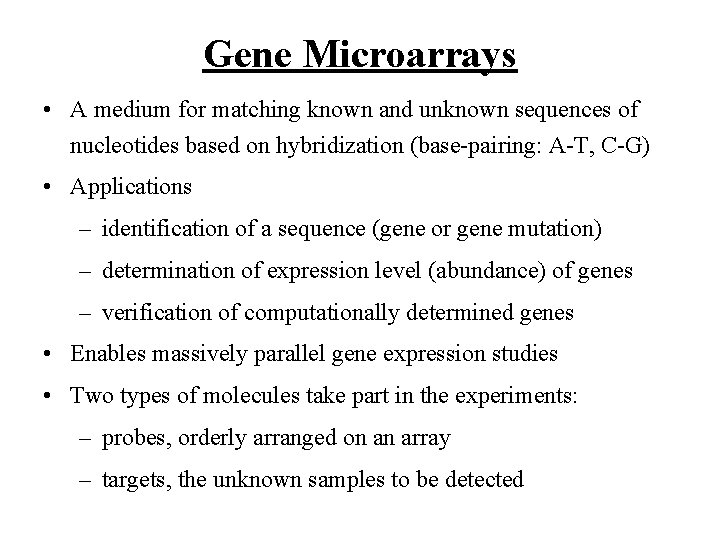
Gene Microarrays • A medium for matching known and unknown sequences of nucleotides based on hybridization (base-pairing: A-T, C-G) • Applications – identification of a sequence (gene or gene mutation) – determination of expression level (abundance) of genes – verification of computationally determined genes • Enables massively parallel gene expression studies • Two types of molecules take part in the experiments: – probes, orderly arranged on an array – targets, the unknown samples to be detected
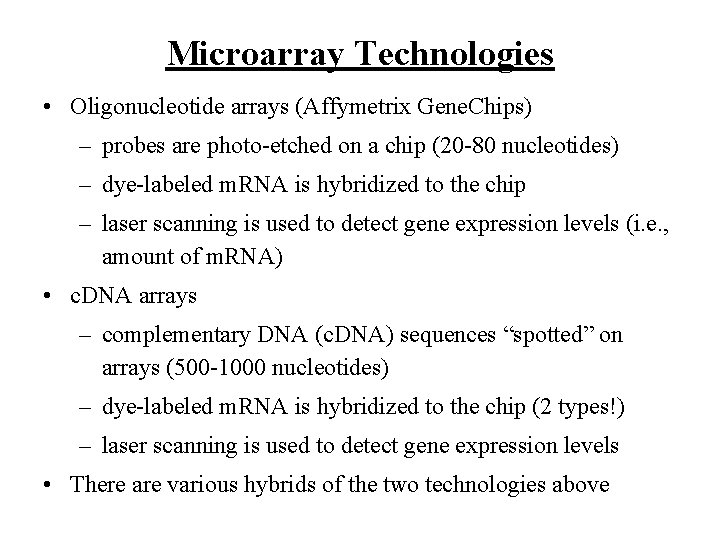
Microarray Technologies • Oligonucleotide arrays (Affymetrix Gene. Chips) – probes are photo-etched on a chip (20 -80 nucleotides) – dye-labeled m. RNA is hybridized to the chip – laser scanning is used to detect gene expression levels (i. e. , amount of m. RNA) • c. DNA arrays – complementary DNA (c. DNA) sequences “spotted” on arrays (500 -1000 nucleotides) – dye-labeled m. RNA is hybridized to the chip (2 types!) – laser scanning is used to detect gene expression levels • There are various hybrids of the two technologies above
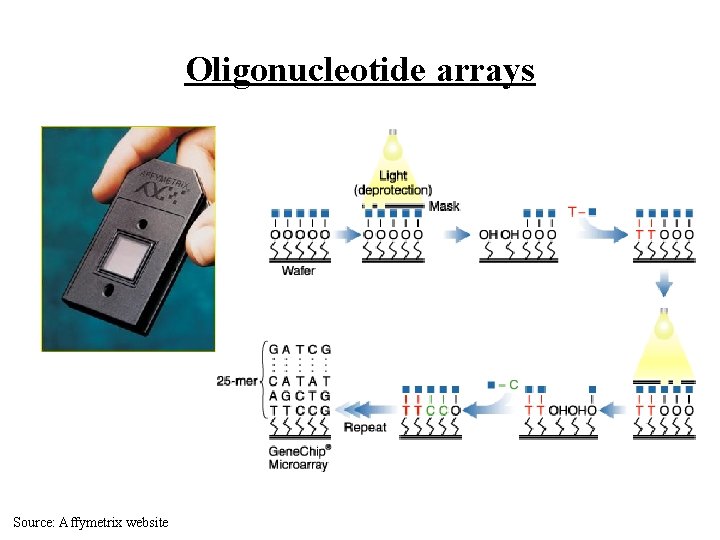
Oligonucleotide arrays Source: Affymetrix website
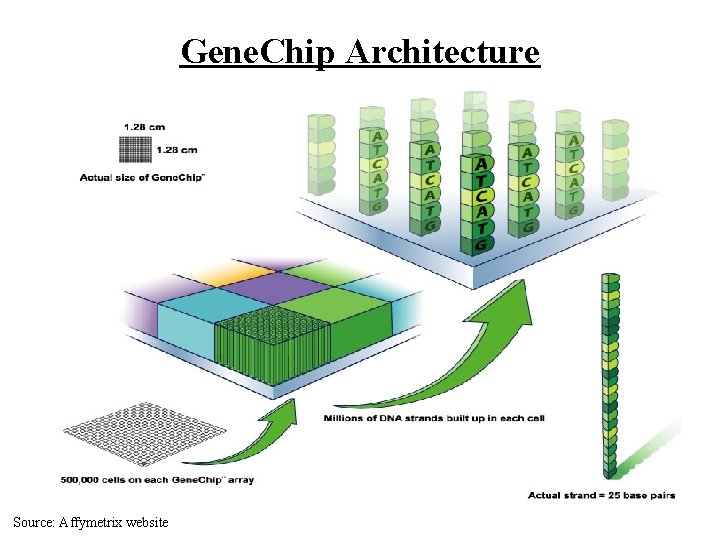
Gene. Chip Architecture Source: Affymetrix website
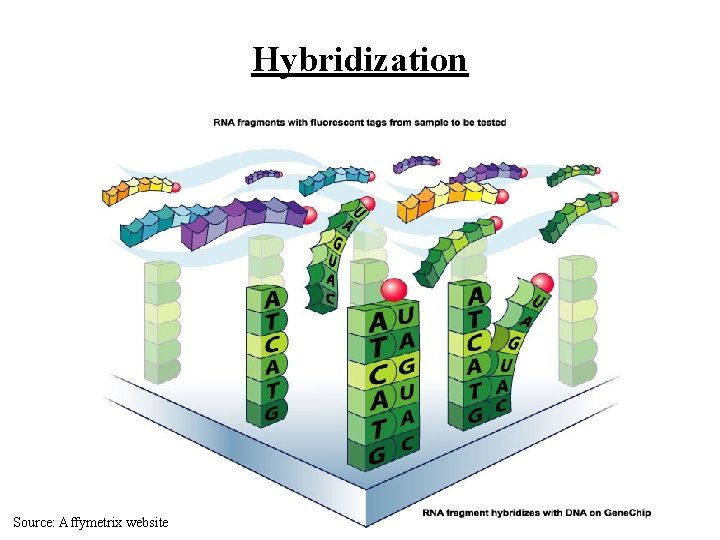
Hybridization Source: Affymetrix website
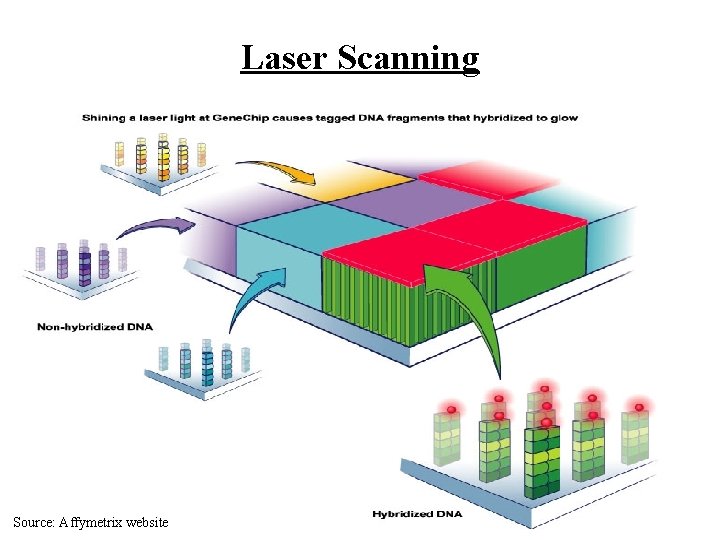
Laser Scanning Source: Affymetrix website
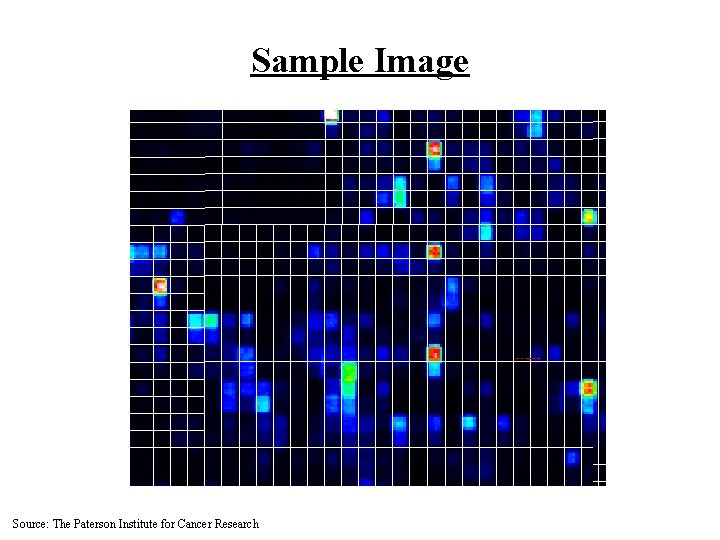
Sample Image Source: The Paterson Institute for Cancer Research
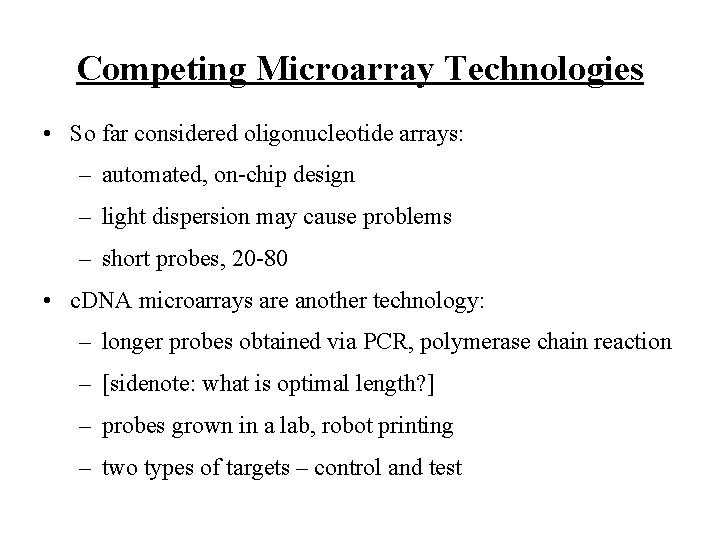
Competing Microarray Technologies • So far considered oligonucleotide arrays: – automated, on-chip design – light dispersion may cause problems – short probes, 20 -80 • c. DNA microarrays are another technology: – longer probes obtained via PCR, polymerase chain reaction – [sidenote: what is optimal length? ] – probes grown in a lab, robot printing – two types of targets – control and test
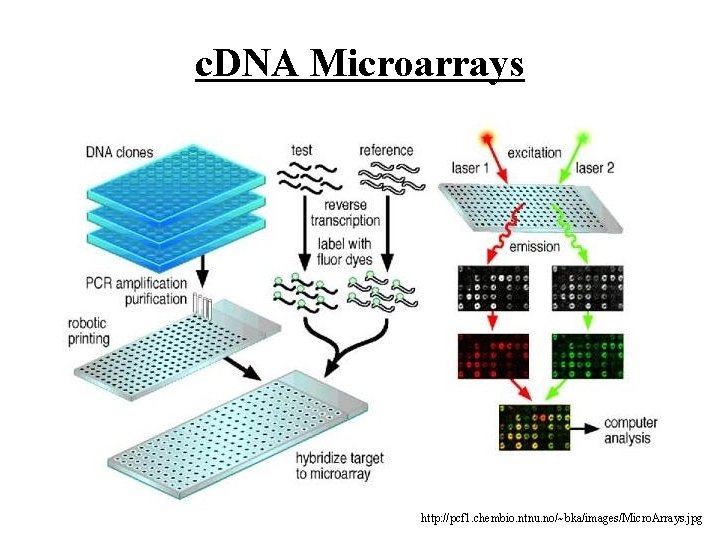
c. DNA Microarrays http: //pcf 1. chembio. ntnu. no/~bka/images/Micro. Arrays. jpg
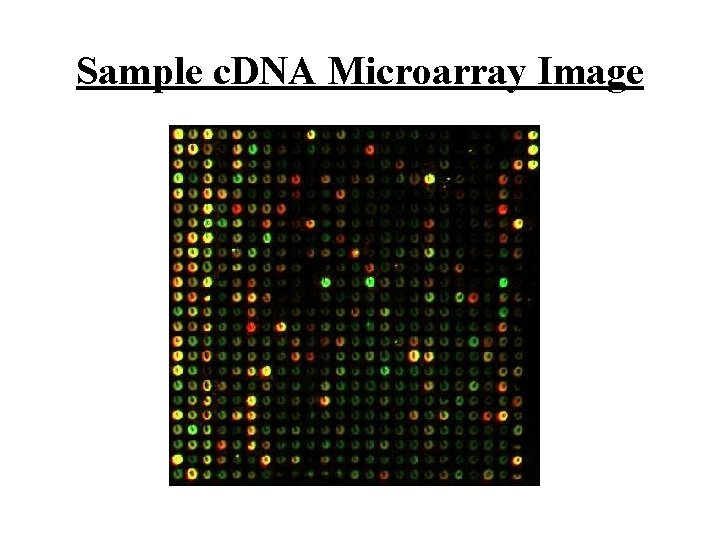
Sample c. DNA Microarray Image
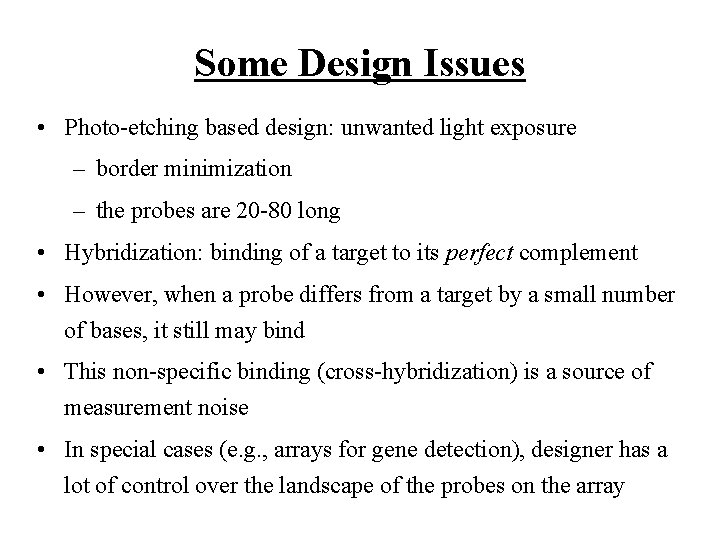
Some Design Issues • Photo-etching based design: unwanted light exposure – border minimization – the probes are 20 -80 long • Hybridization: binding of a target to its perfect complement • However, when a probe differs from a target by a small number of bases, it still may bind • This non-specific binding (cross-hybridization) is a source of measurement noise • In special cases (e. g. , arrays for gene detection), designer has a lot of control over the landscape of the probes on the array
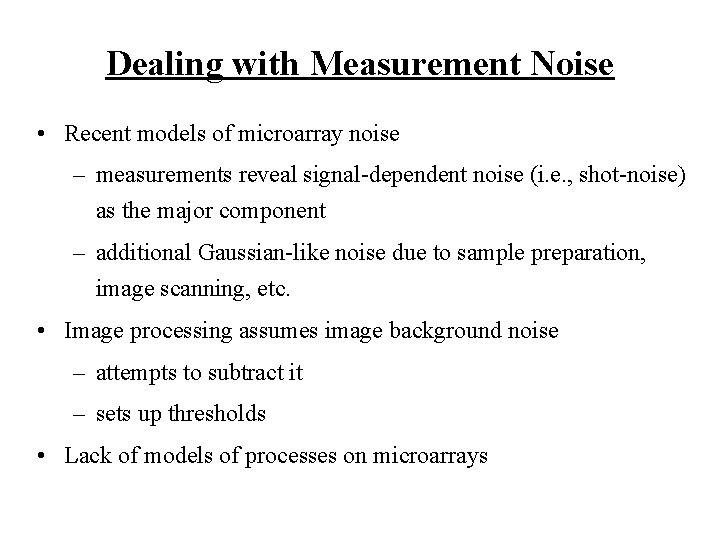
Dealing with Measurement Noise • Recent models of microarray noise – measurements reveal signal-dependent noise (i. e. , shot-noise) as the major component – additional Gaussian-like noise due to sample preparation, image scanning, etc. • Image processing assumes image background noise – attempts to subtract it – sets up thresholds • Lack of models of processes on microarrays
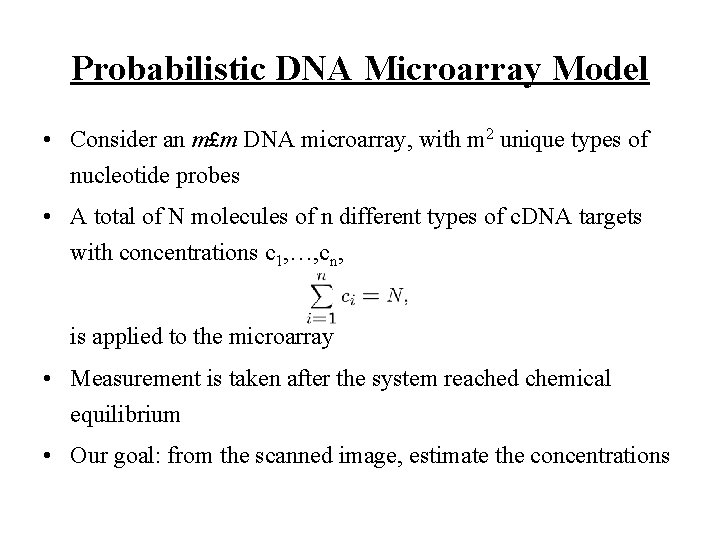
Probabilistic DNA Microarray Model • Consider an m£m DNA microarray, with m 2 unique types of nucleotide probes • A total of N molecules of n different types of c. DNA targets with concentrations c 1, …, cn, is applied to the microarray • Measurement is taken after the system reached chemical equilibrium • Our goal: from the scanned image, estimate the concentrations
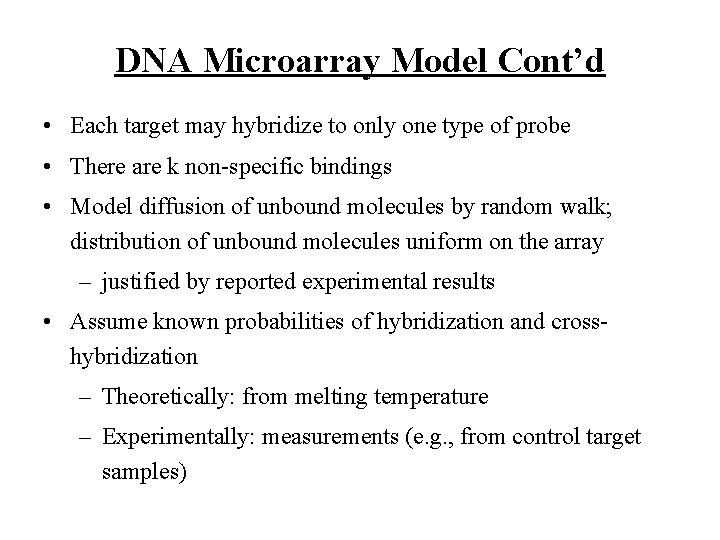
DNA Microarray Model Cont’d • Each target may hybridize to only one type of probe • There are k non-specific bindings • Model diffusion of unbound molecules by random walk; distribution of unbound molecules uniform on the array – justified by reported experimental results • Assume known probabilities of hybridization and crosshybridization – Theoretically: from melting temperature – Experimentally: measurements (e. g. , from control target samples)
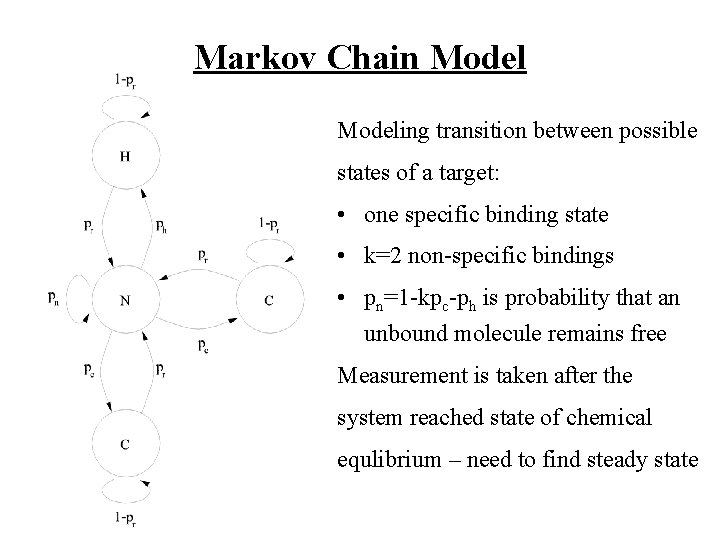
Markov Chain Modeling transition between possible states of a target: • one specific binding state • k=2 non-specific bindings • pn=1 -kpc-ph is probability that an unbound molecule remains free Measurement is taken after the system reached state of chemical equlibrium – need to find steady state
![Markov Chain Model Contd Let i i 1 i 2 i k2T be Markov Chain Model Cont’d Let i=[ i, 1 i, 2 … i, k+2]T be](https://slidetodoc.com/presentation_image_h2/cfe2e81a85c82c4596dc9543ab723ac7/image-18.jpg)
Markov Chain Model Cont’d Let i=[ i, 1 i, 2 … i, k+2]T be a vector whose components are numbers of the type i targets that are in one of the k+2 states of the Markov chain • i, 1 is the # of hybridized molecules • i, j, 2 < j · k+2 is # of cross-hybrid. Note that k=1 k+2 i, k=ci for every i.
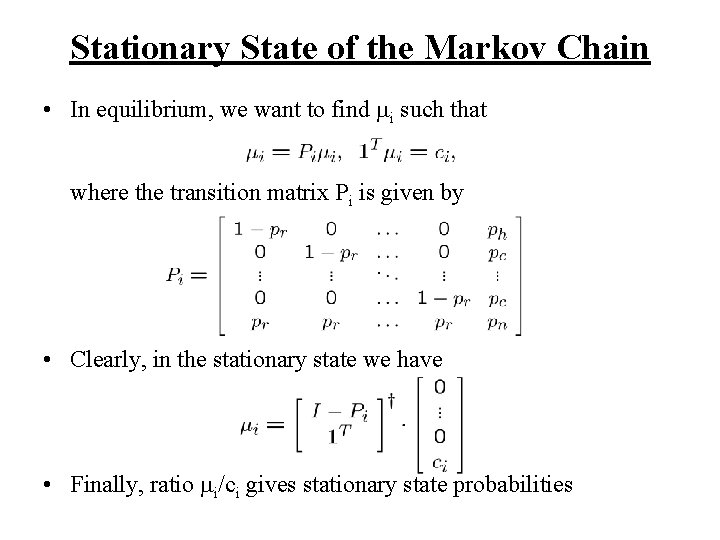
Stationary State of the Markov Chain • In equilibrium, we want to find i such that where the transition matrix Pi is given by • Clearly, in the stationary state we have • Finally, ratio i/ci gives stationary state probabilities
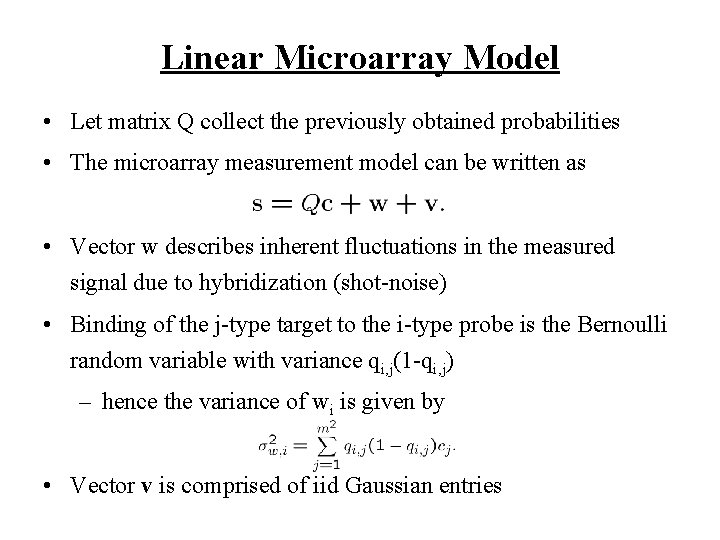
Linear Microarray Model • Let matrix Q collect the previously obtained probabilities • The microarray measurement model can be written as • Vector w describes inherent fluctuations in the measured signal due to hybridization (shot-noise) • Binding of the j-type target to the i-type probe is the Bernoulli random variable with variance qi, j(1 -qi, j) – hence the variance of wi is given by • Vector v is comprised of iid Gaussian entries
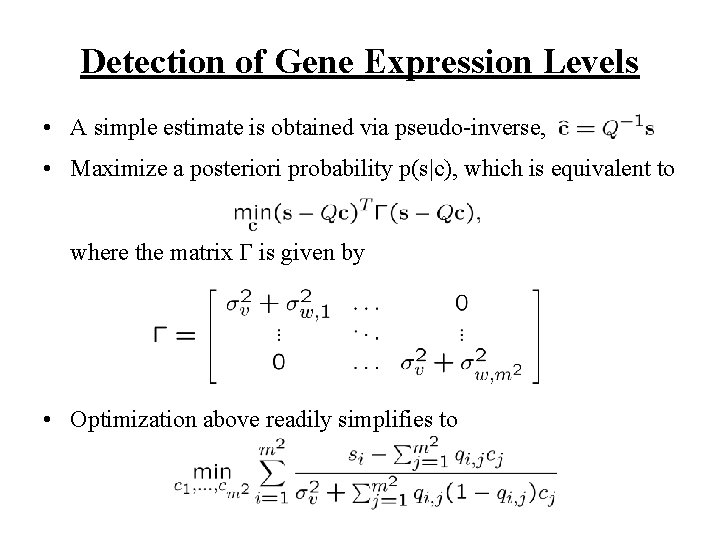
Detection of Gene Expression Levels • A simple estimate is obtained via pseudo-inverse, • Maximize a posteriori probability p(s|c), which is equivalent to where the matrix is given by • Optimization above readily simplifies to
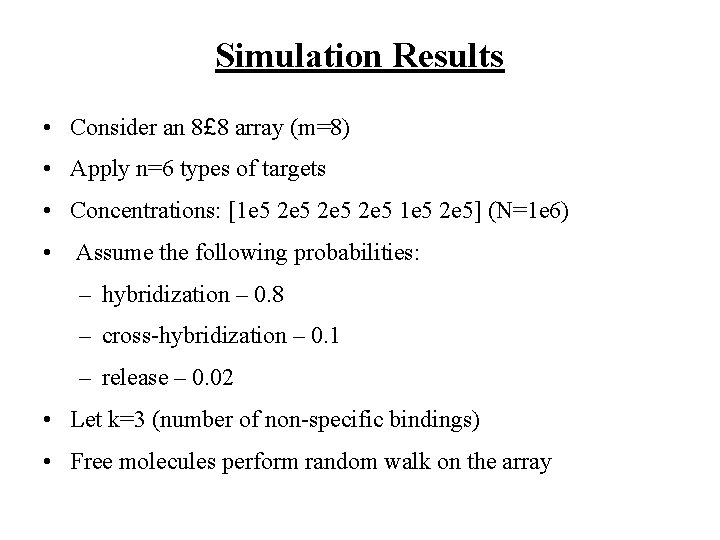
Simulation Results • Consider an 8£ 8 array (m=8) • Apply n=6 types of targets • Concentrations: [1 e 5 2 e 5 1 e 5 2 e 5] (N=1 e 6) • Assume the following probabilities: – hybridization – 0. 8 – cross-hybridization – 0. 1 – release – 0. 02 • Let k=3 (number of non-specific bindings) • Free molecules perform random walk on the array
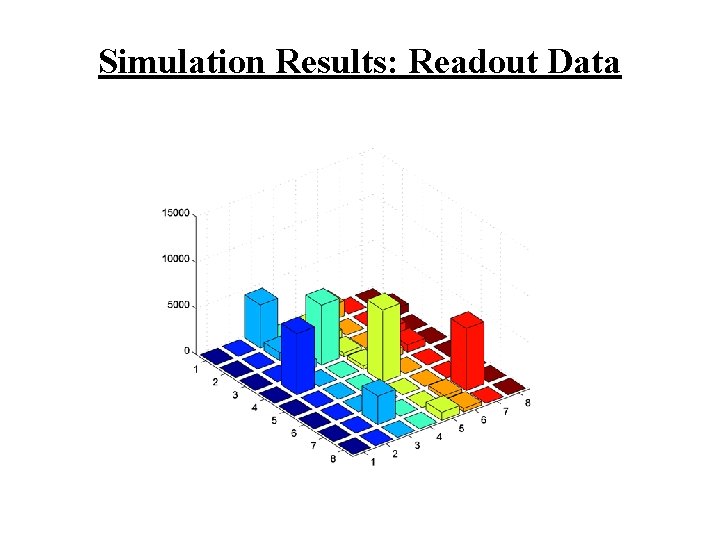
Simulation Results: Readout Data
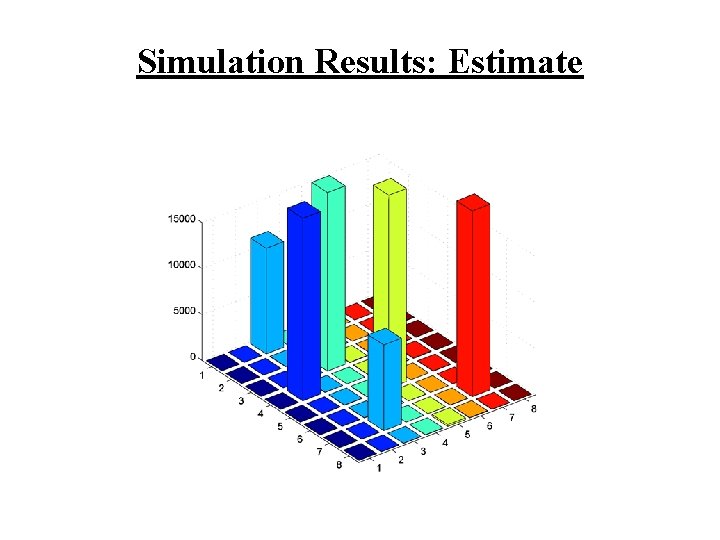
Simulation Results: Estimate
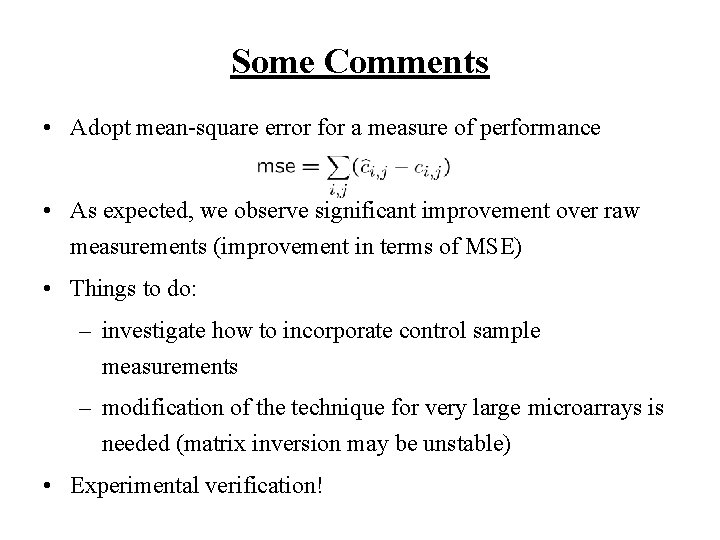
Some Comments • Adopt mean-square error for a measure of performance • As expected, we observe significant improvement over raw measurements (improvement in terms of MSE) • Things to do: – investigate how to incorporate control sample measurements – modification of the technique for very large microarrays is needed (matrix inversion may be unstable) • Experimental verification!
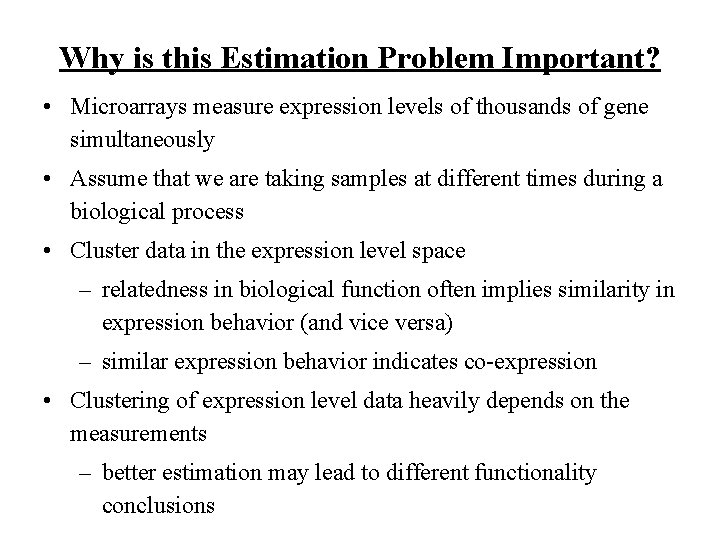
Why is this Estimation Problem Important? • Microarrays measure expression levels of thousands of gene simultaneously • Assume that we are taking samples at different times during a biological process • Cluster data in the expression level space – relatedness in biological function often implies similarity in expression behavior (and vice versa) – similar expression behavior indicates co-expression • Clustering of expression level data heavily depends on the measurements – better estimation may lead to different functionality conclusions
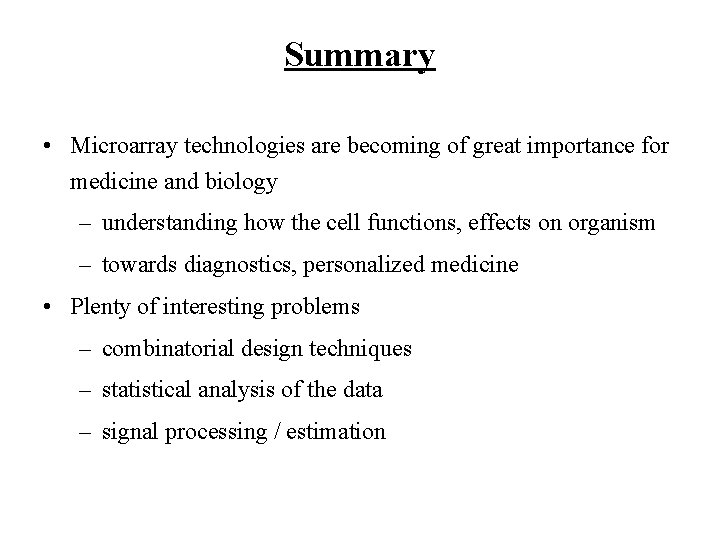
Summary • Microarray technologies are becoming of great importance for medicine and biology – understanding how the cell functions, effects on organism – towards diagnostics, personalized medicine • Plenty of interesting problems – combinatorial design techniques – statistical analysis of the data – signal processing / estimation
Genomic signal processing
Genomic equivalence definition
Genomic england
Genomic england
Anneke seller
Genomic instability
Genomic
Genomic imprinting definition
Comparative genomic hybridization animation
Genomic equivalence definition
Baseband signal and bandpass signal
Baseband signal and bandpass signal
Classification of signal
Digital signal as a composite analog signal
Signal processing first
Parallel system tsample ---- tclock
Unfolding in vlsi signal processing
Direct form 2 structure
Types of signal
Jatiya kabi kazi nazrul islam university logo
Digital signal processing
Signal processing toolbox matlab
Complex wave
Ica signal processing
Signal processing for big data
Financial signal processing
Super audio cd
Digital signal processing