Driving force of calculations Driving force of algorithm
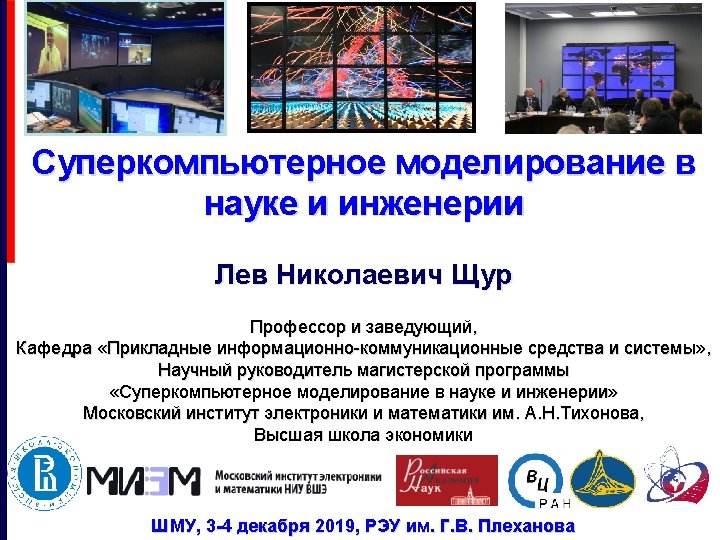
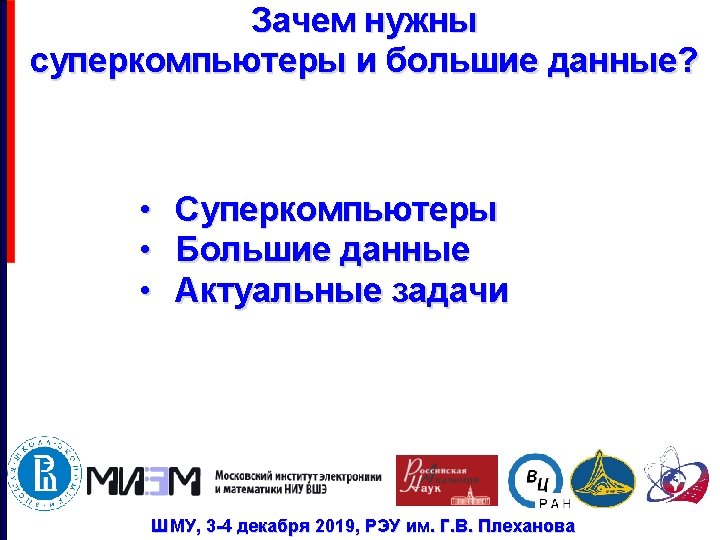
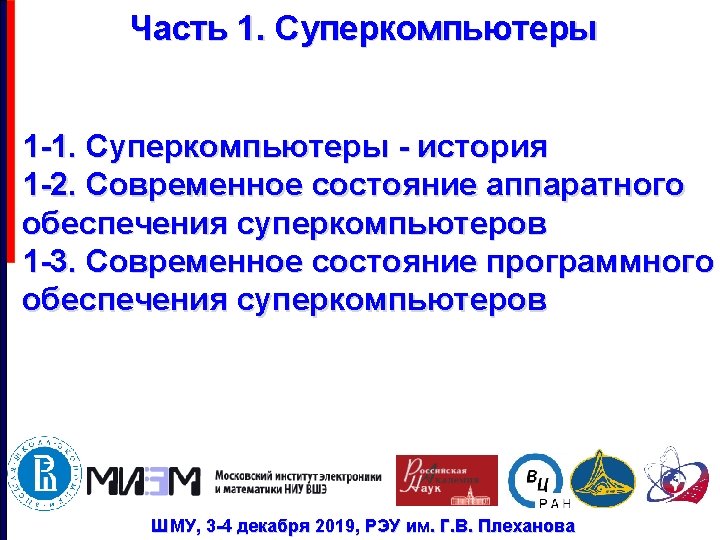
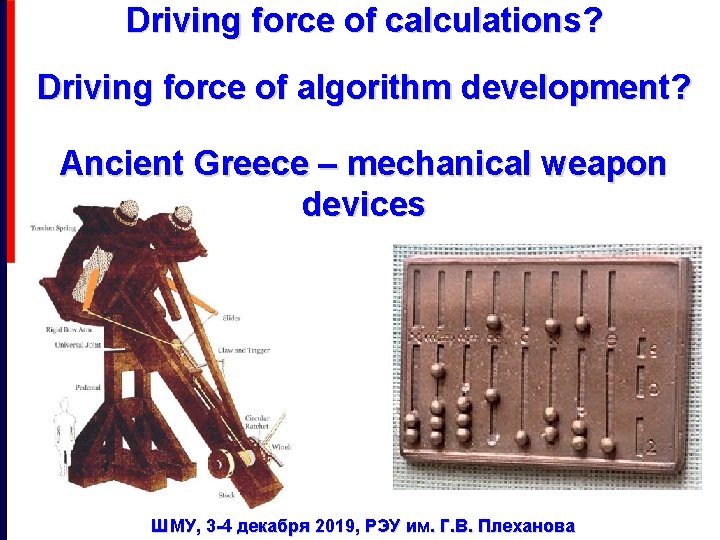
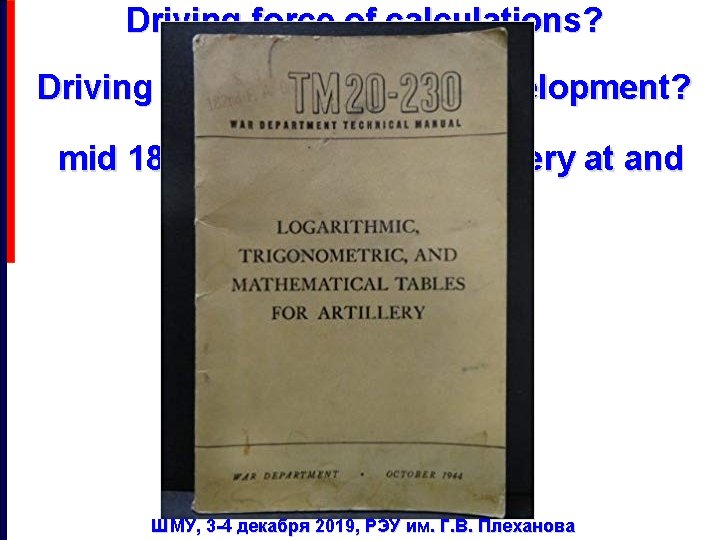
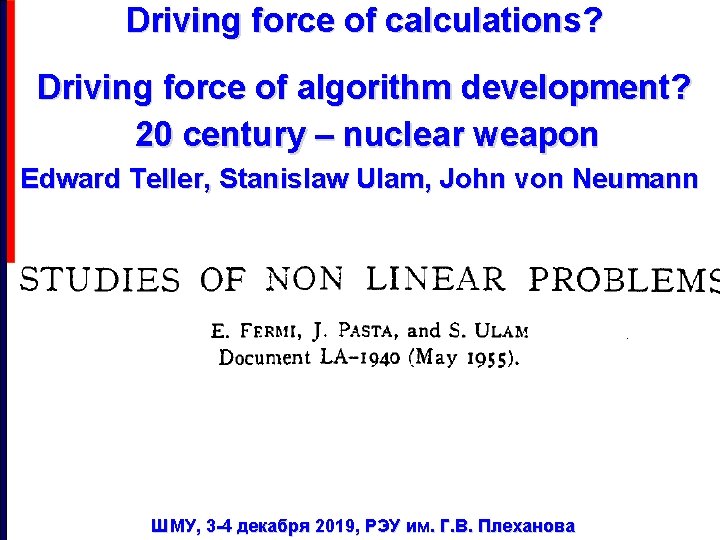
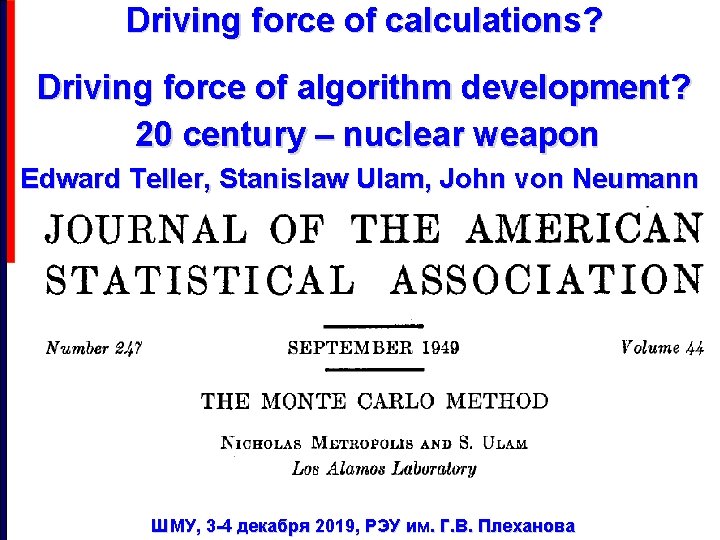
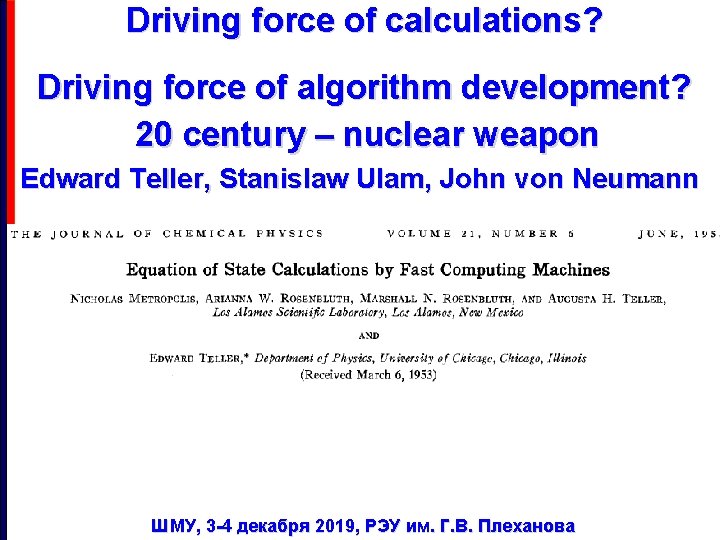
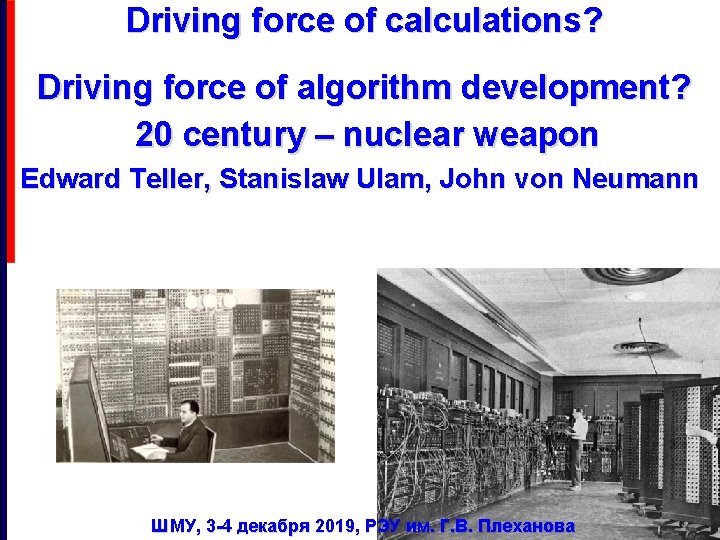
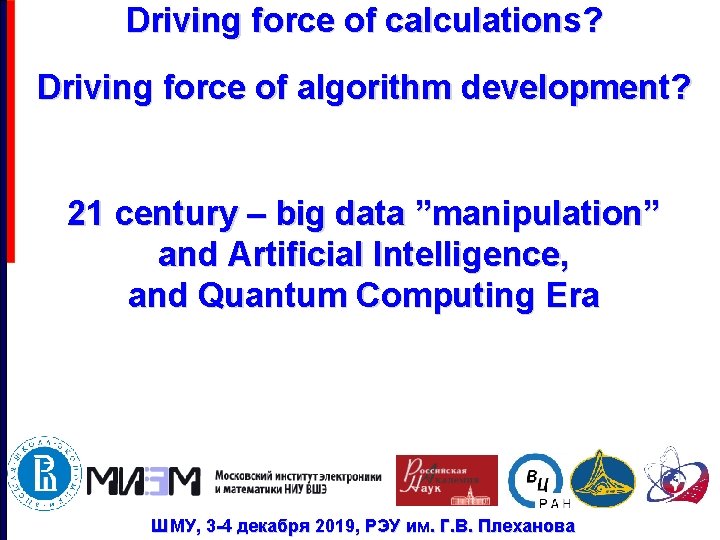
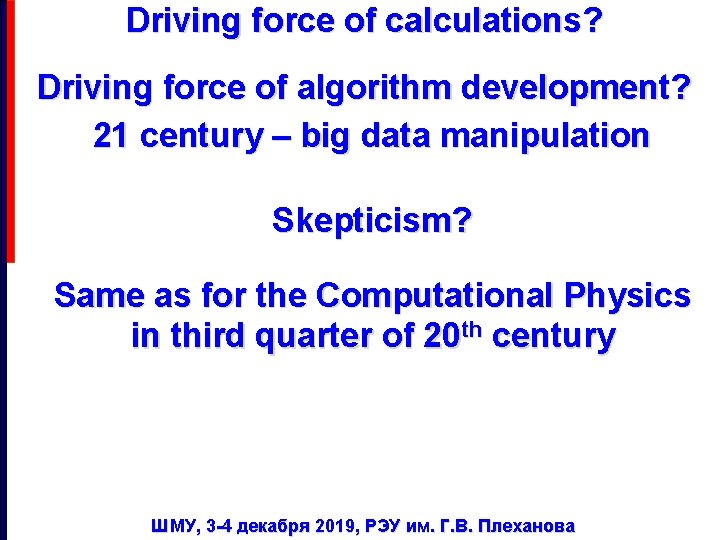
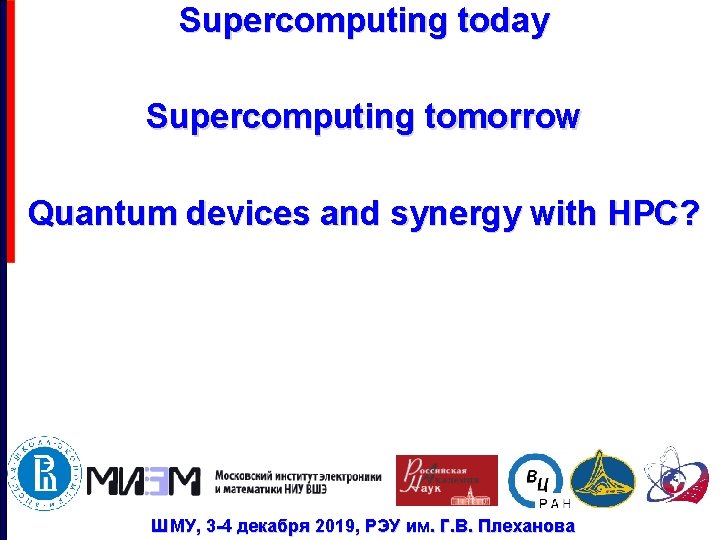
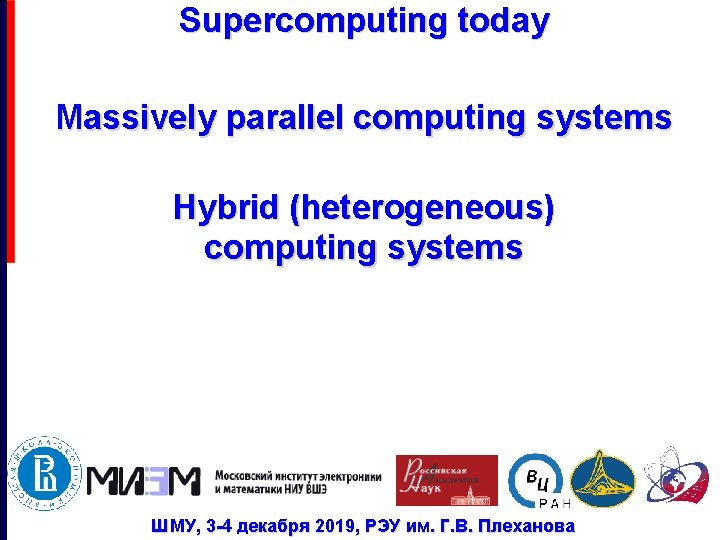
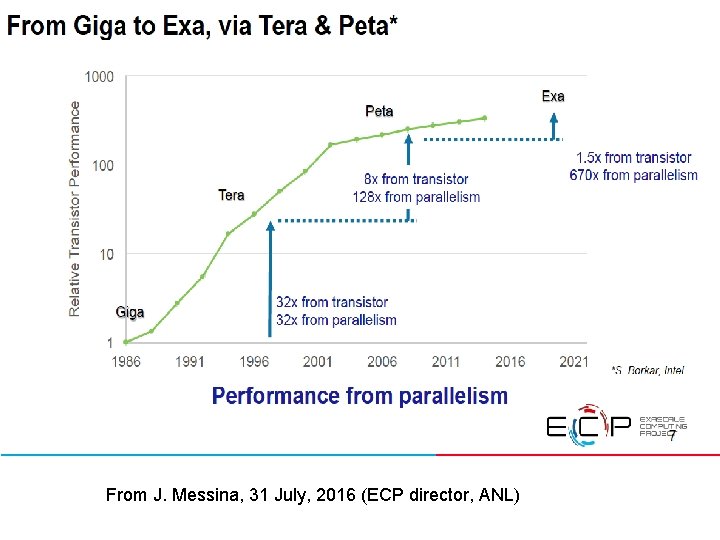
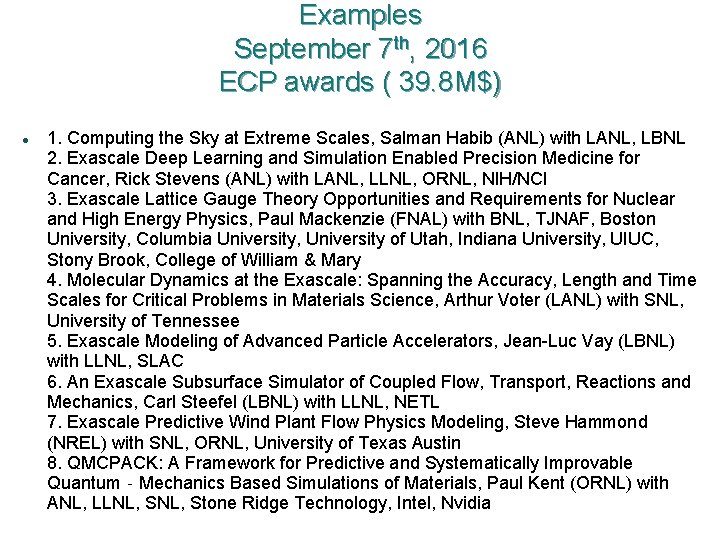
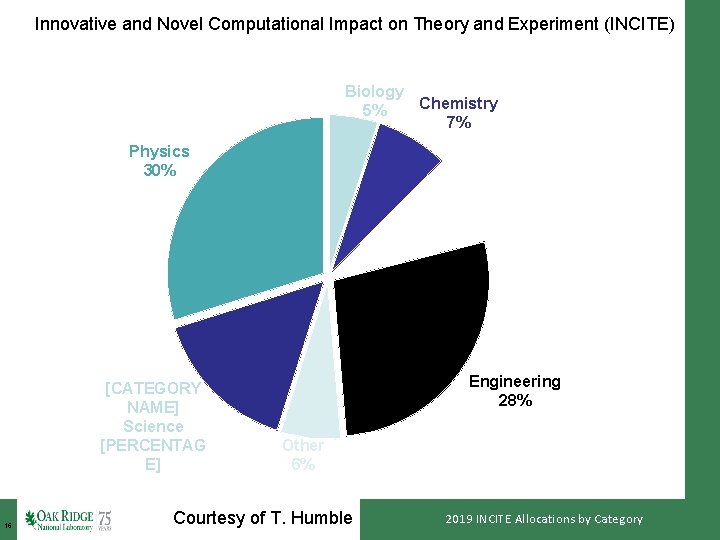
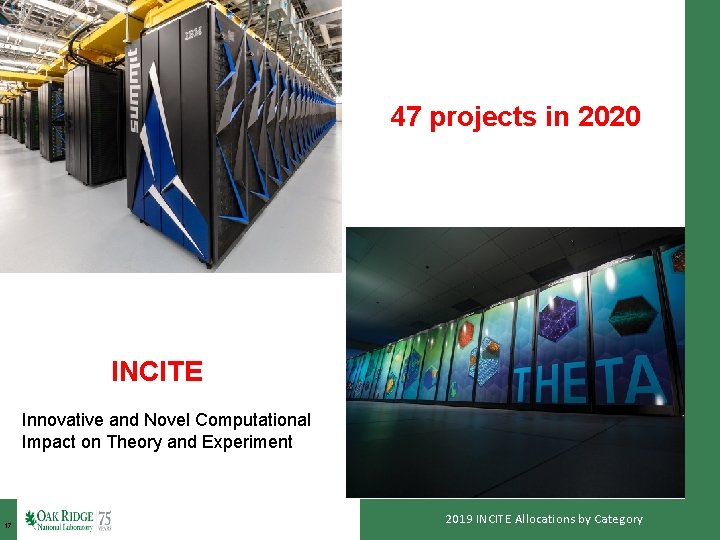
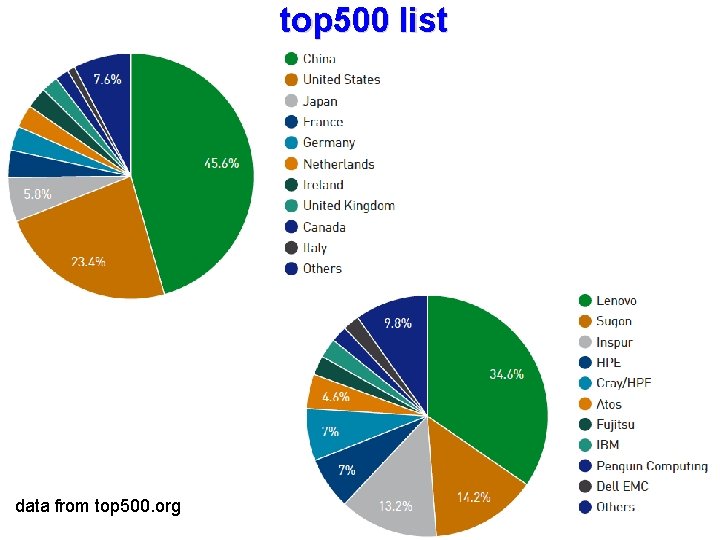
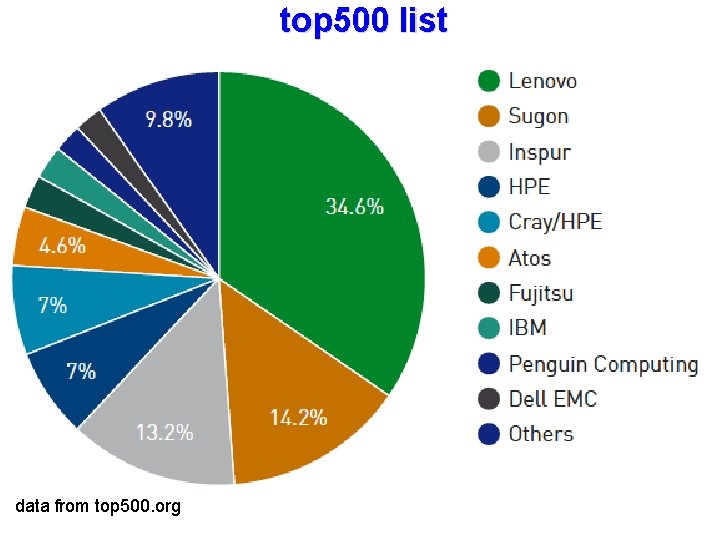
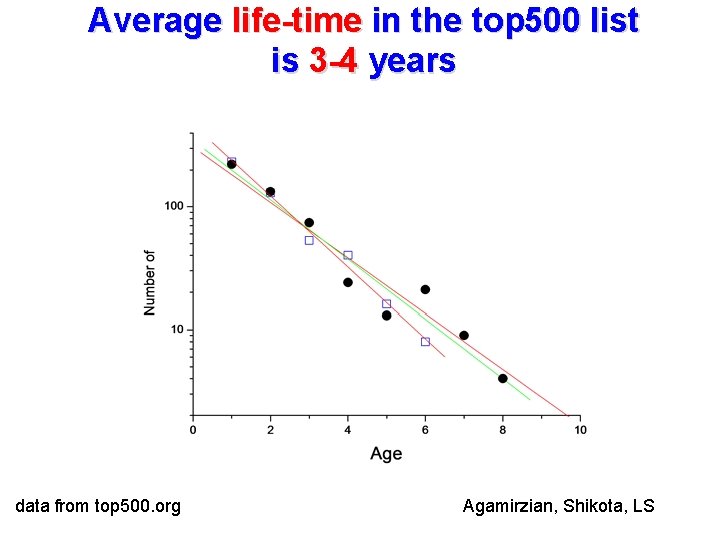
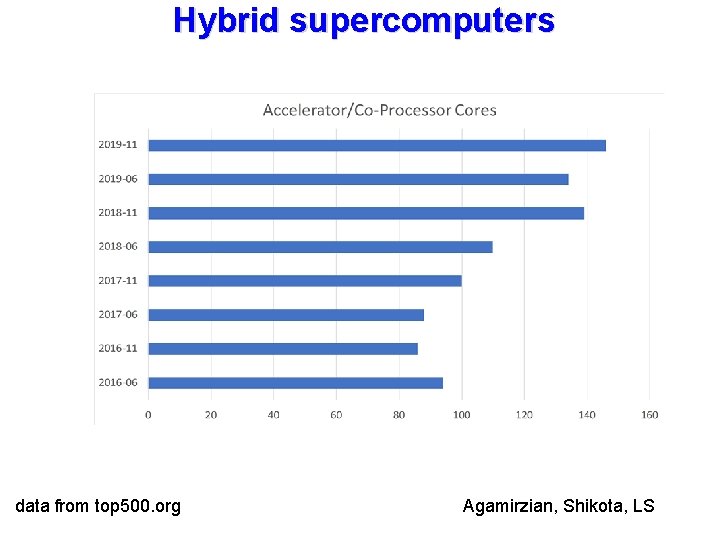
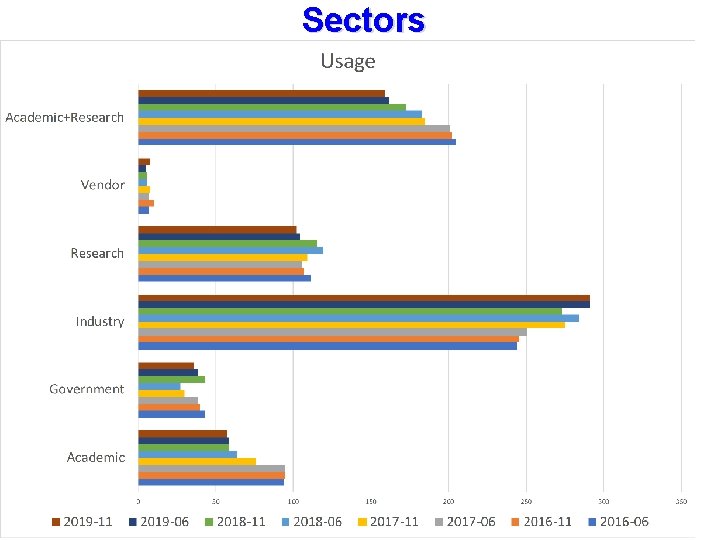
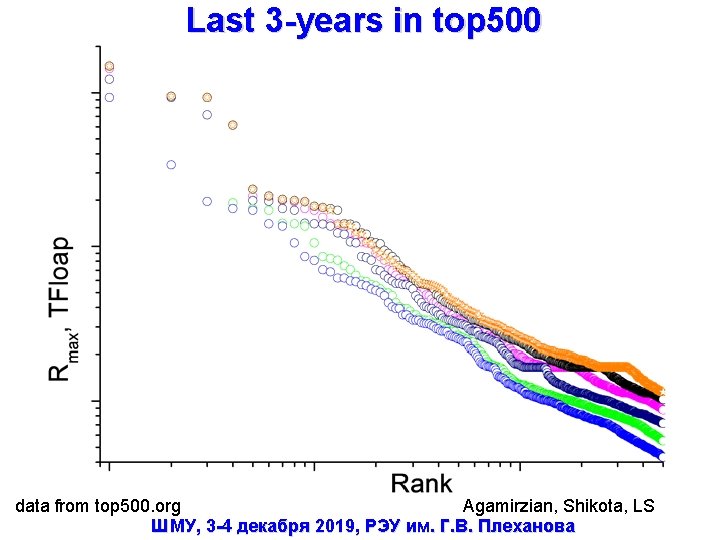
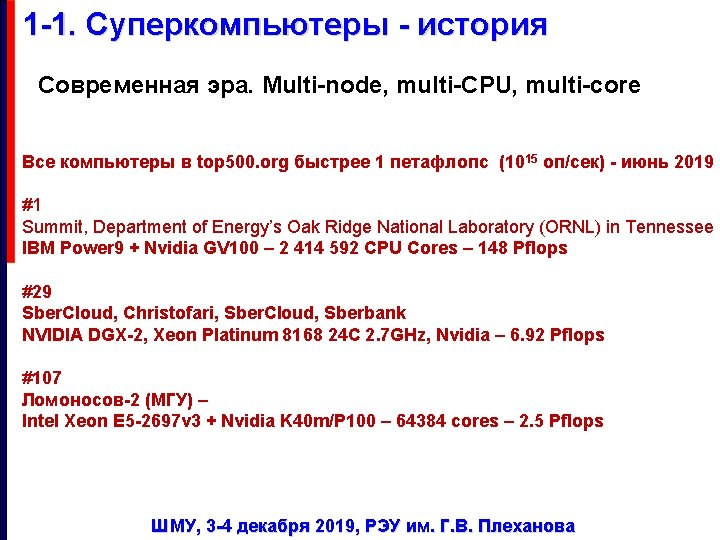
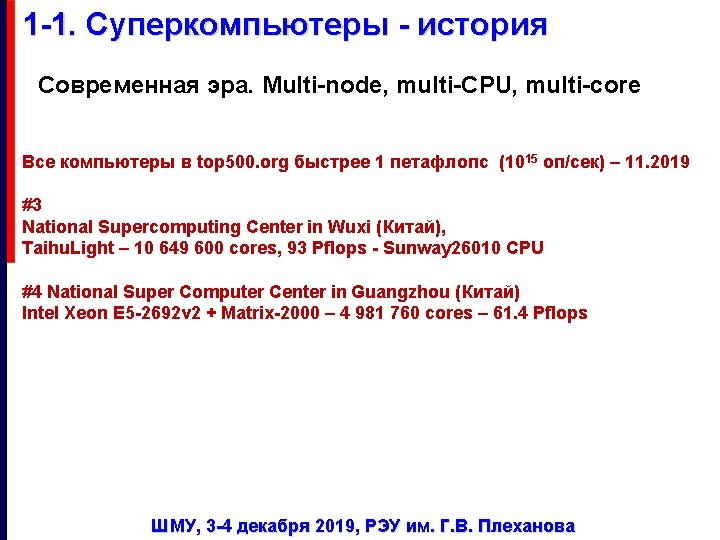
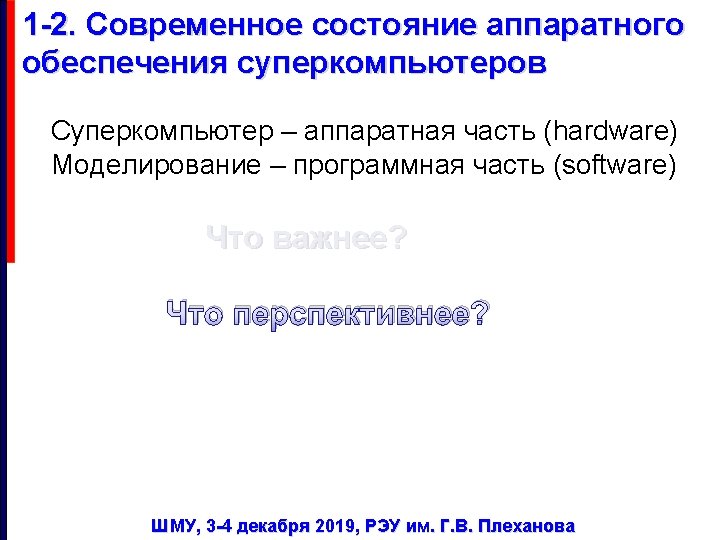
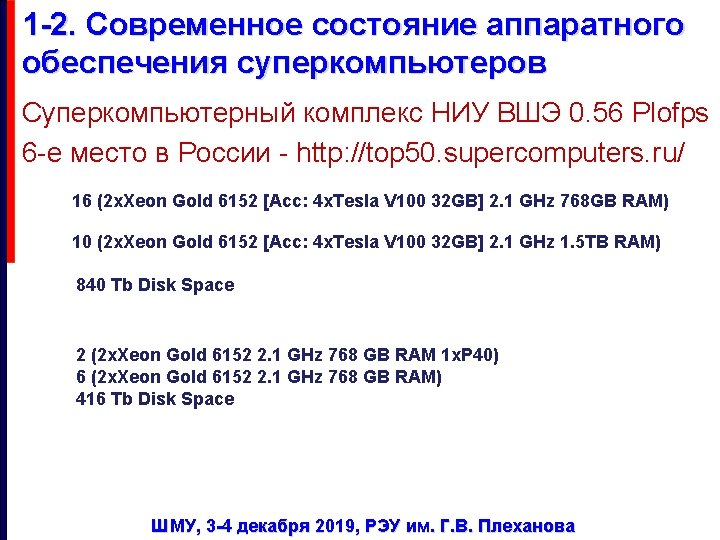
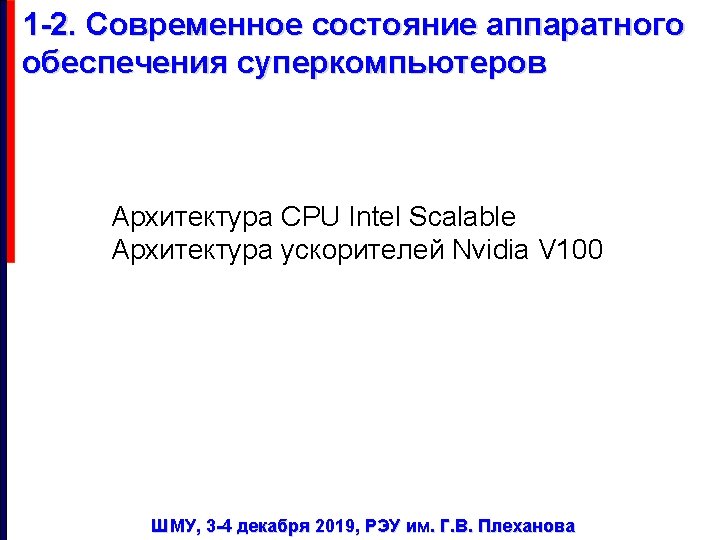
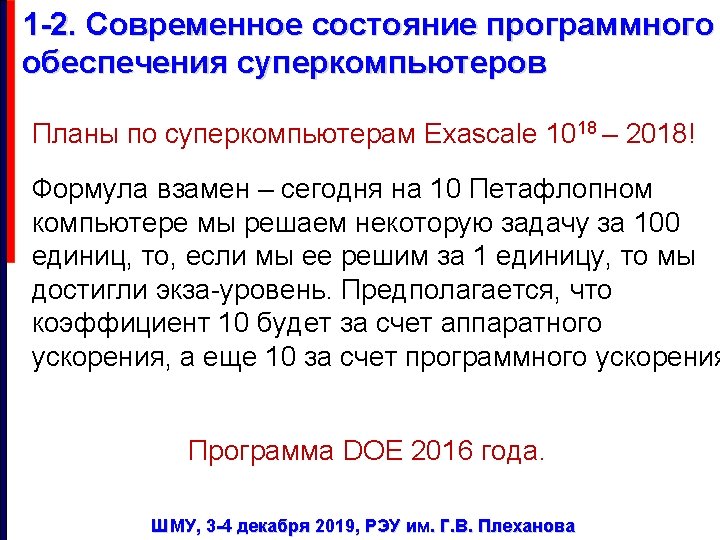
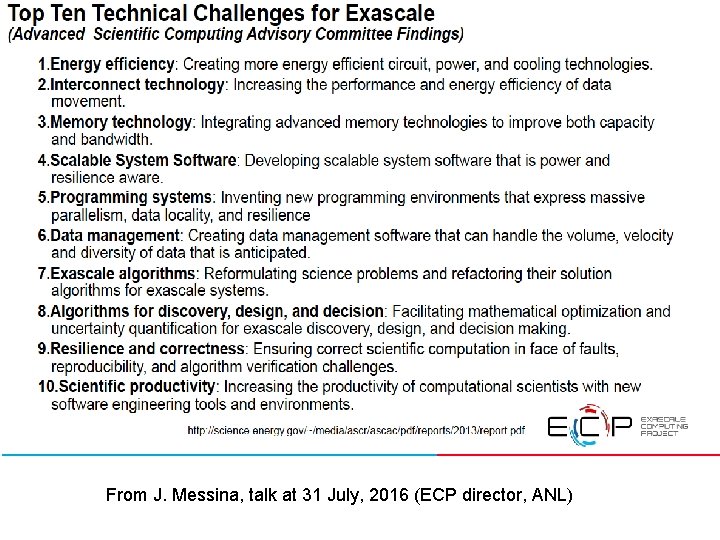
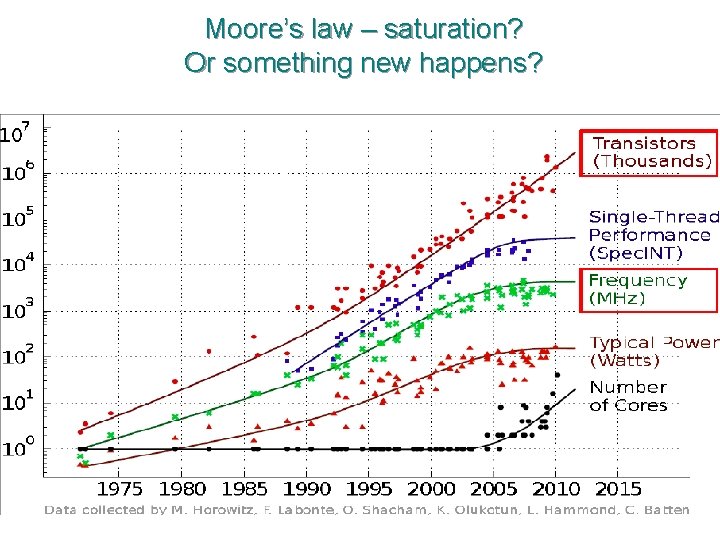
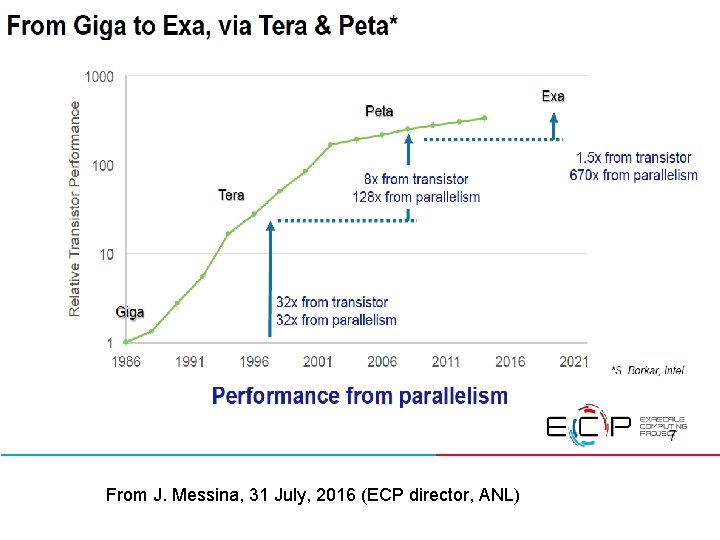
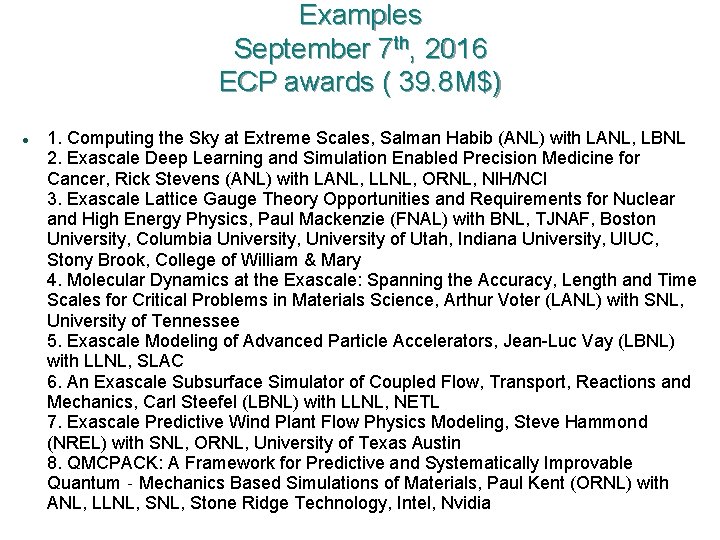
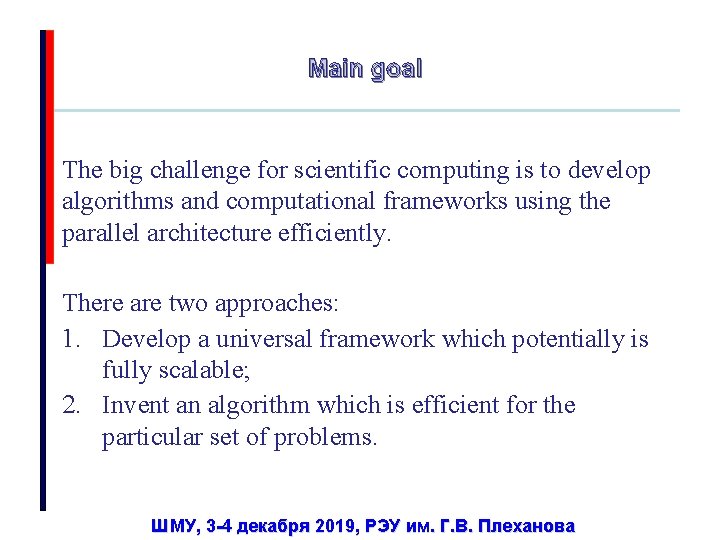
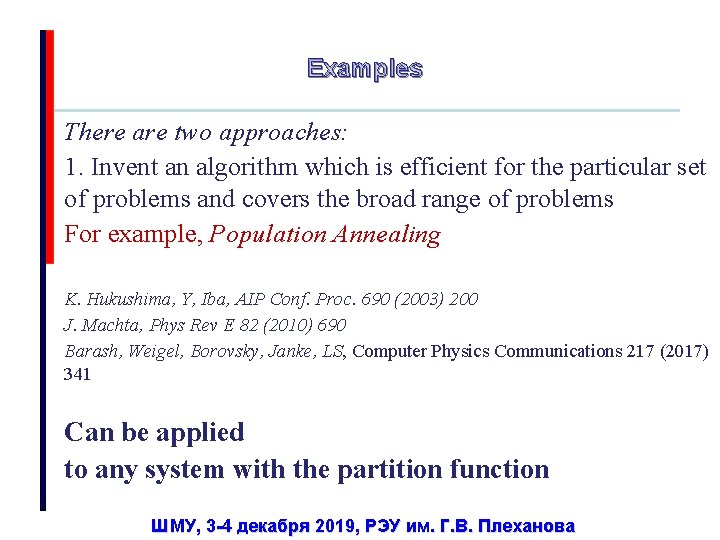
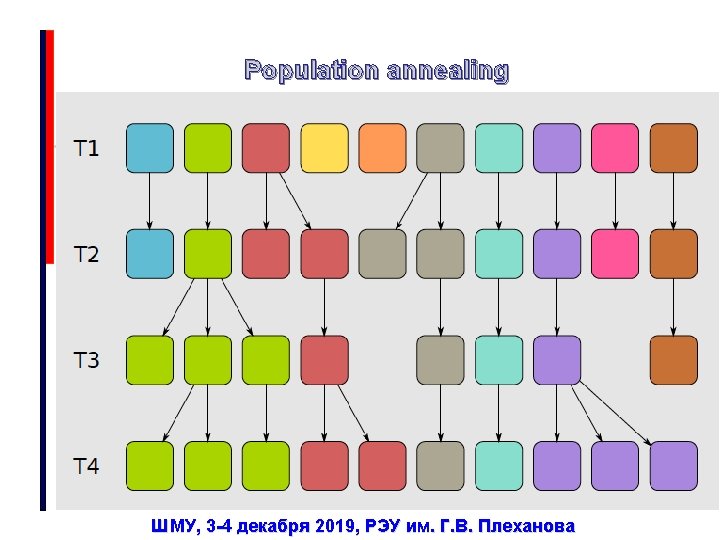
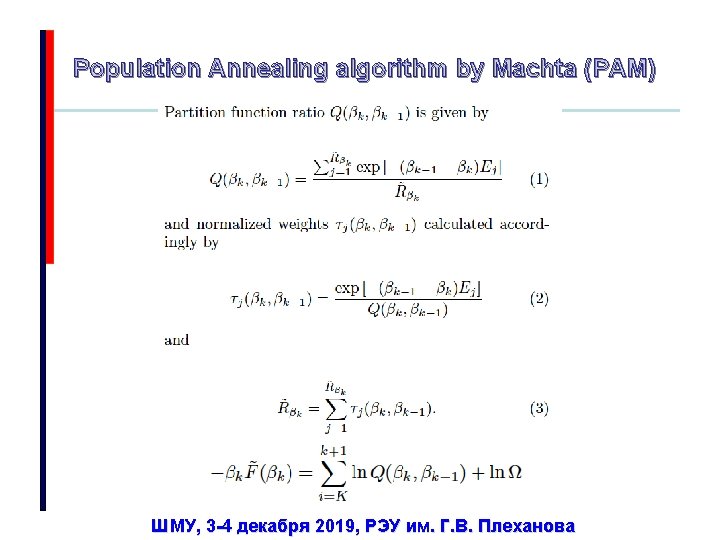
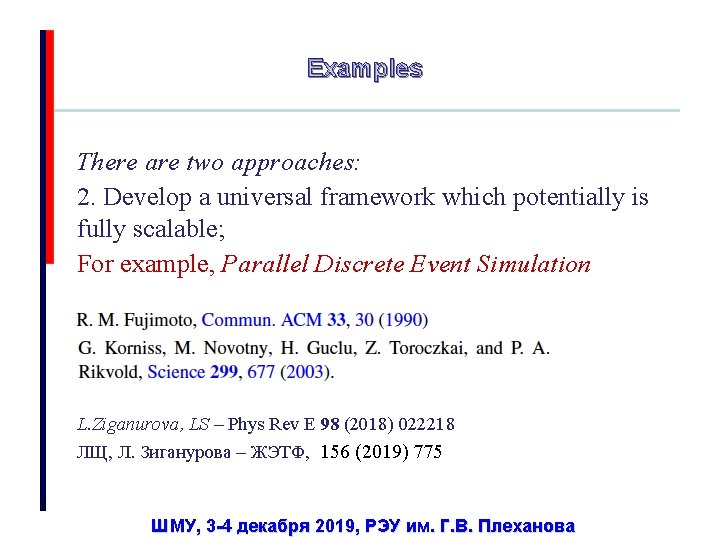
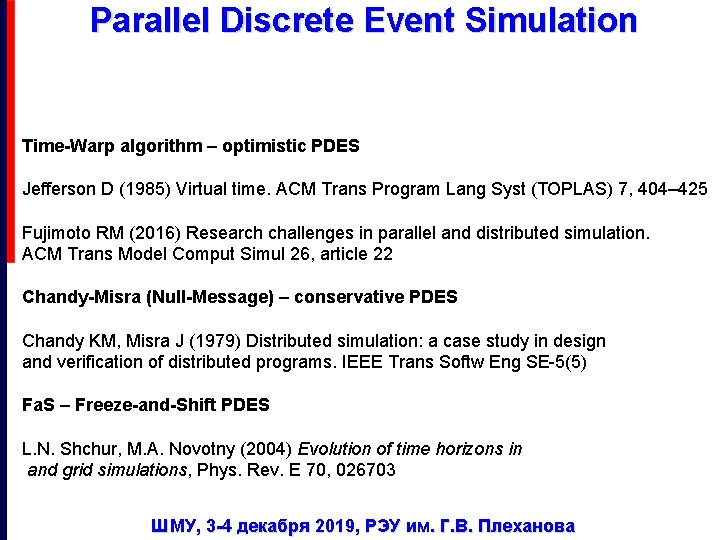
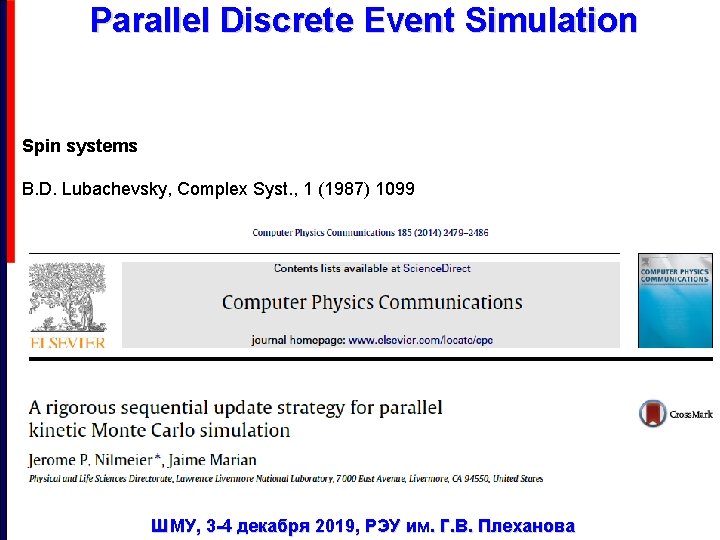
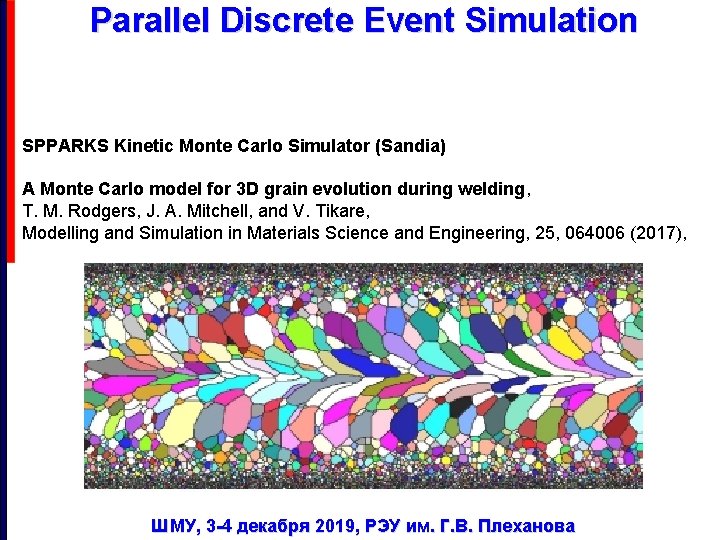
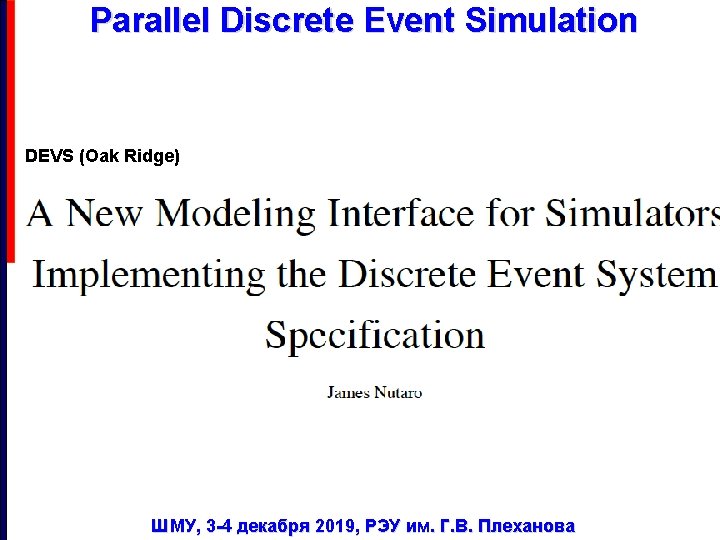
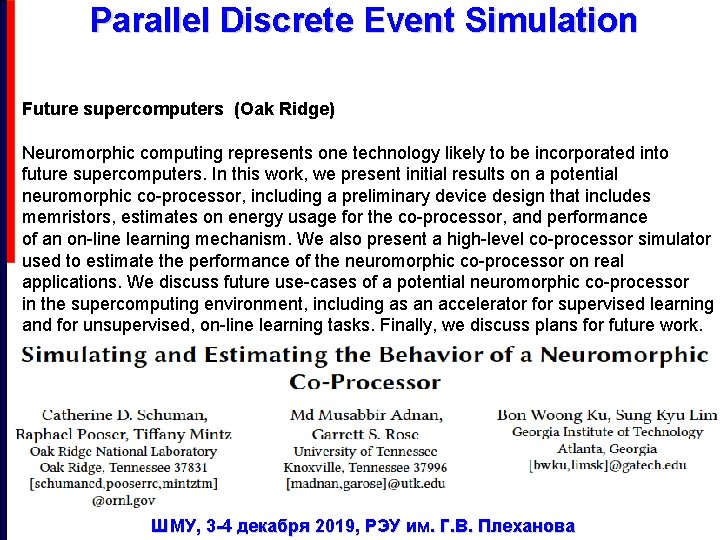
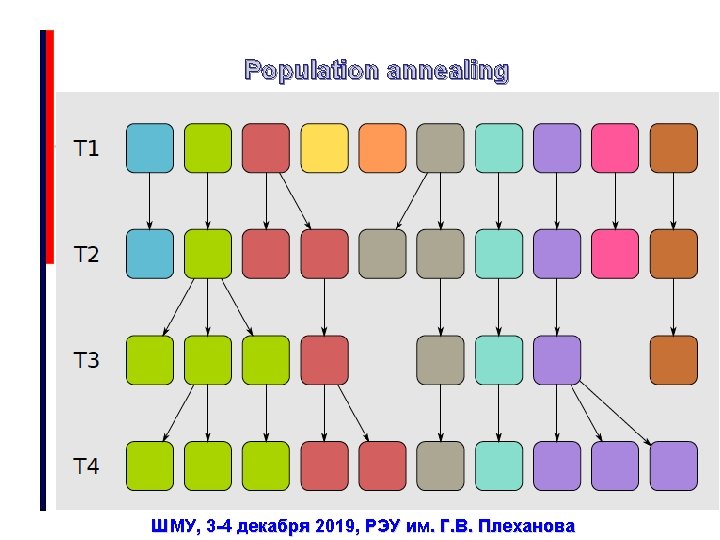
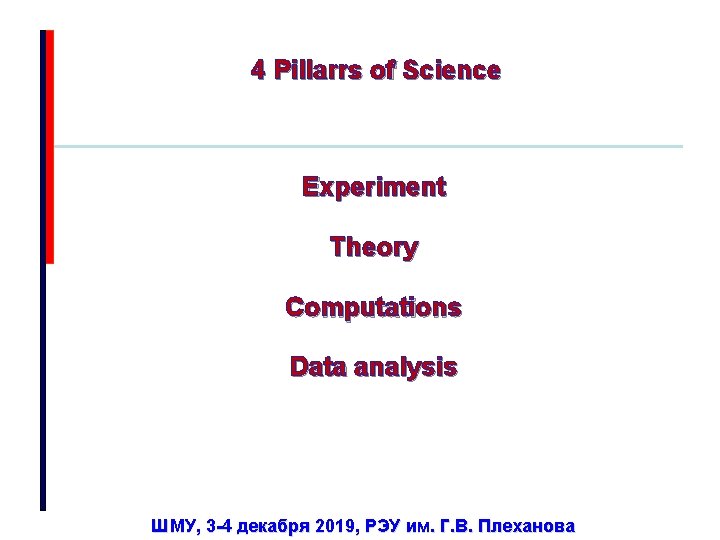
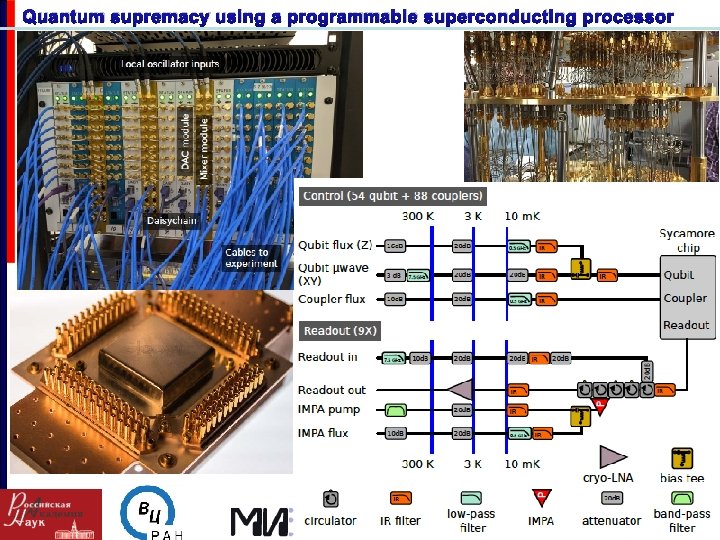
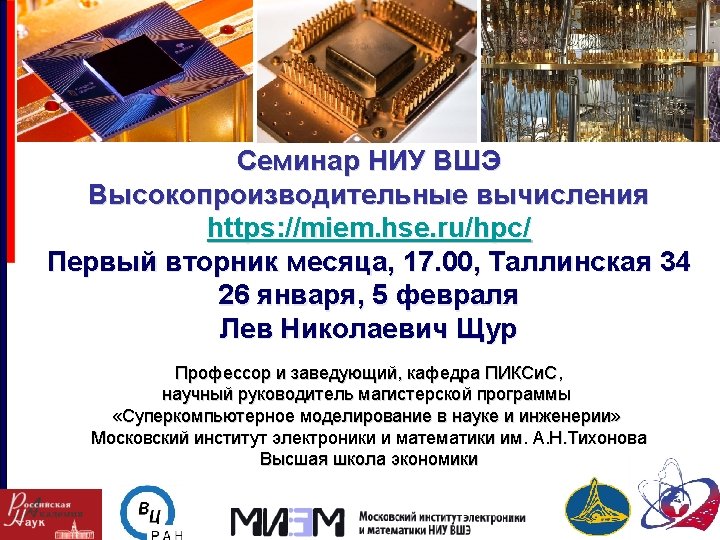
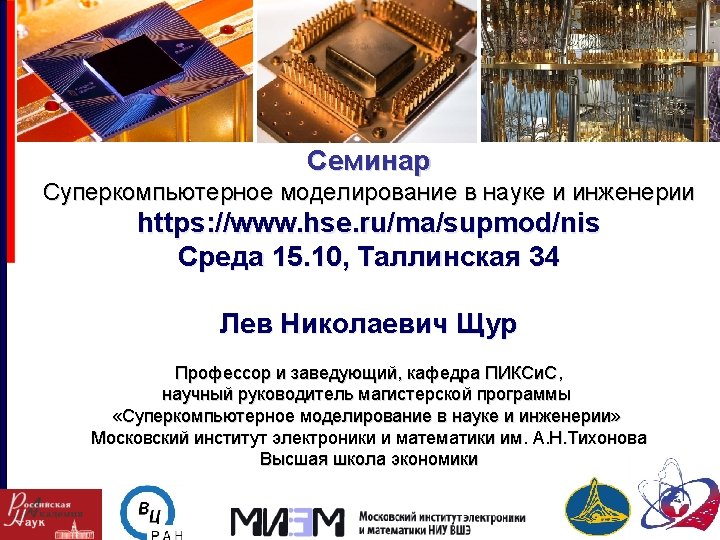
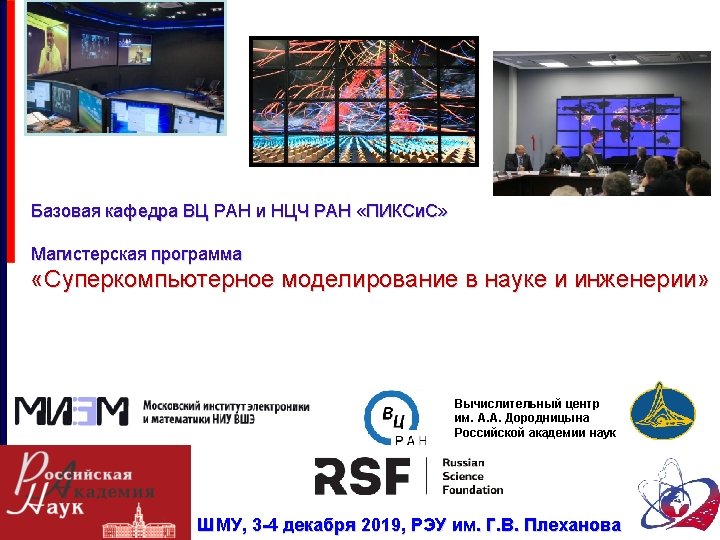
- Slides: 49
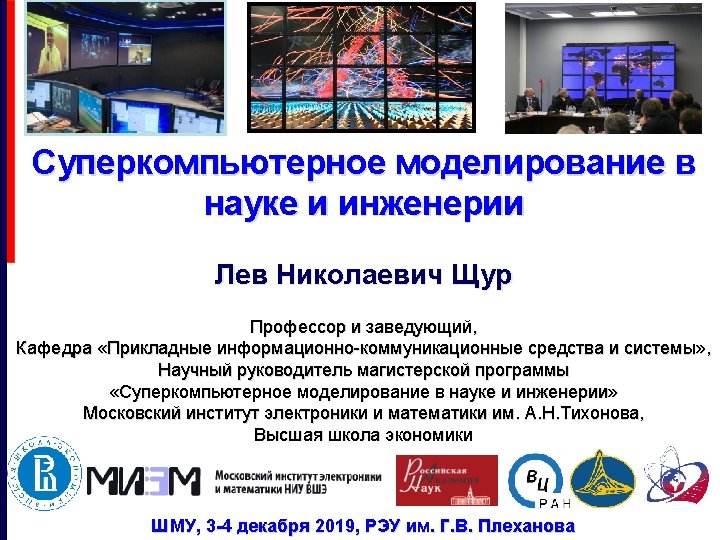
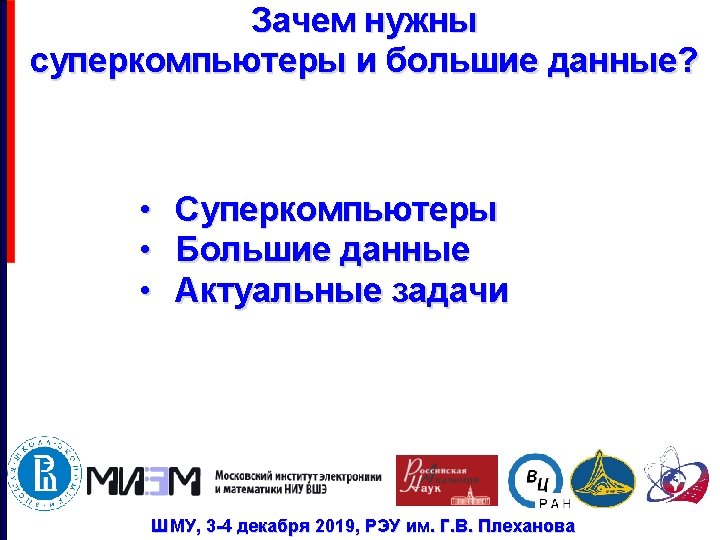
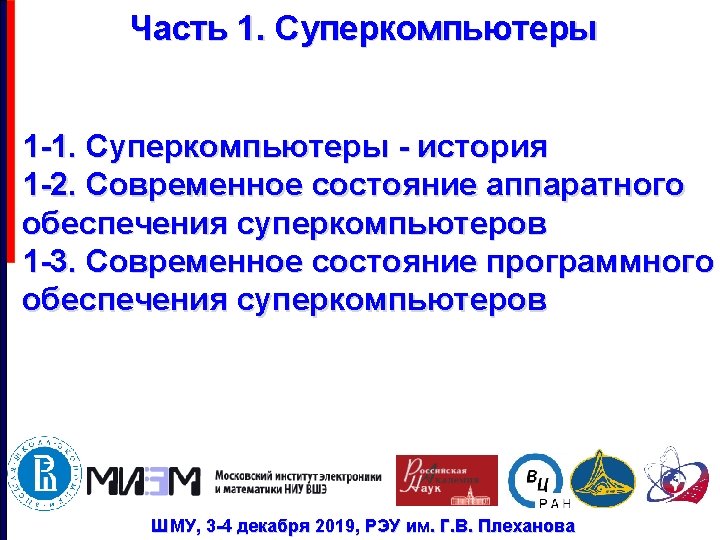
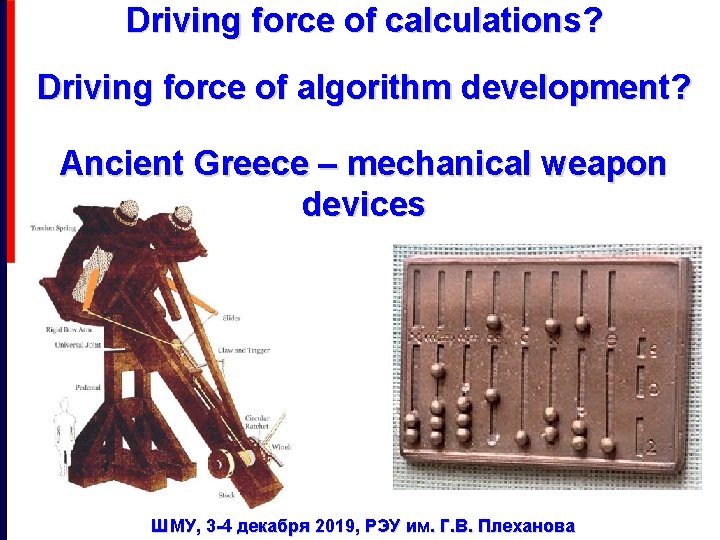
Driving force of calculations? Driving force of algorithm development? Ancient Greece – mechanical weapon devices ШМУ, 3 -4 декабря 2019, РЭУ им. Г. В. Плеханова
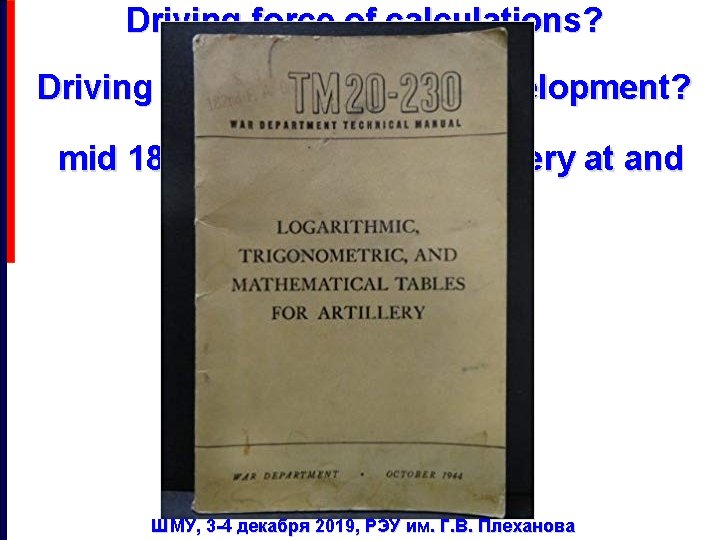
Driving force of calculations? Driving force of algorithm development? mid 18 century – British artillery at and after Crimean War ШМУ, 3 -4 декабря 2019, РЭУ им. Г. В. Плеханова
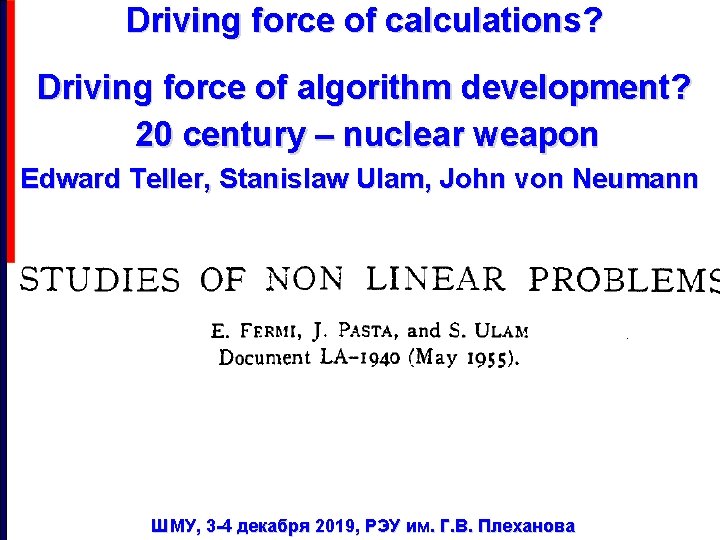
Driving force of calculations? Driving force of algorithm development? 20 century – nuclear weapon Edward Teller, Stanislaw Ulam, John von Neumann ШМУ, 3 -4 декабря 2019, РЭУ им. Г. В. Плеханова
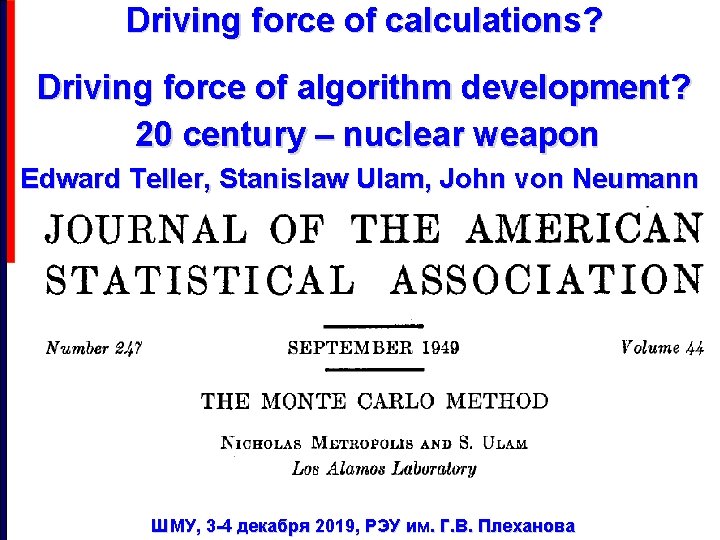
Driving force of calculations? Driving force of algorithm development? 20 century – nuclear weapon Edward Teller, Stanislaw Ulam, John von Neumann ШМУ, 3 -4 декабря 2019, РЭУ им. Г. В. Плеханова
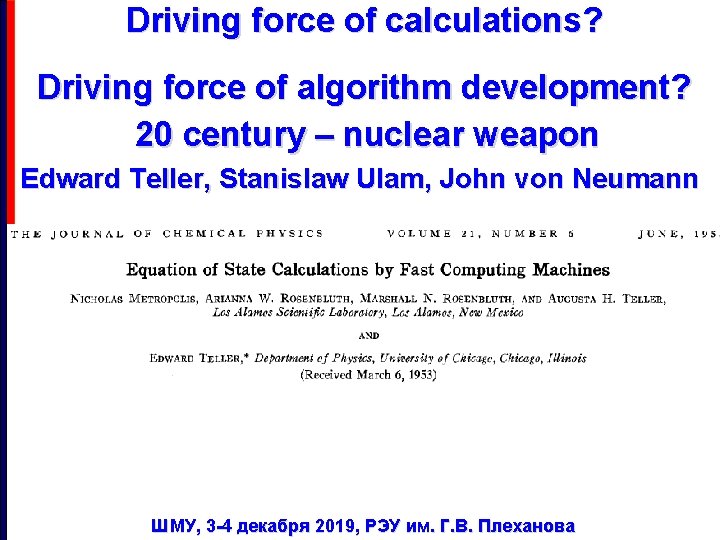
Driving force of calculations? Driving force of algorithm development? 20 century – nuclear weapon Edward Teller, Stanislaw Ulam, John von Neumann ШМУ, 3 -4 декабря 2019, РЭУ им. Г. В. Плеханова
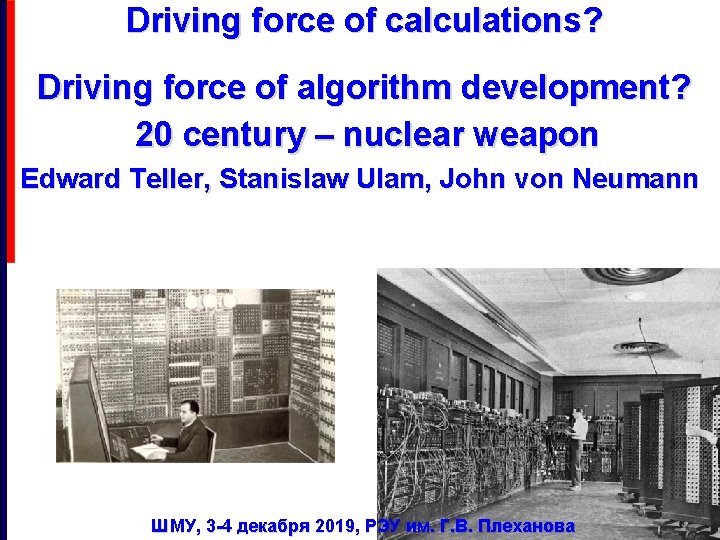
Driving force of calculations? Driving force of algorithm development? 20 century – nuclear weapon Edward Teller, Stanislaw Ulam, John von Neumann ШМУ, 3 -4 декабря 2019, РЭУ им. Г. В. Плеханова
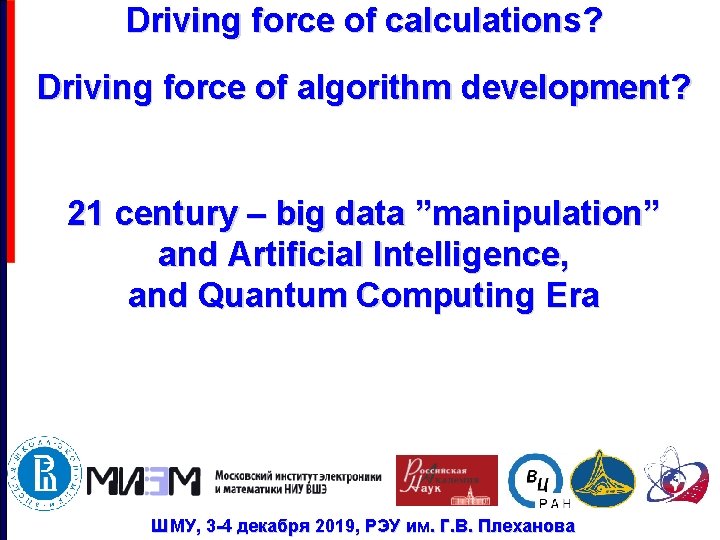
Driving force of calculations? Driving force of algorithm development? 21 century – big data ”manipulation” and Artificial Intelligence, and Quantum Computing Era ШМУ, 3 -4 декабря 2019, РЭУ им. Г. В. Плеханова
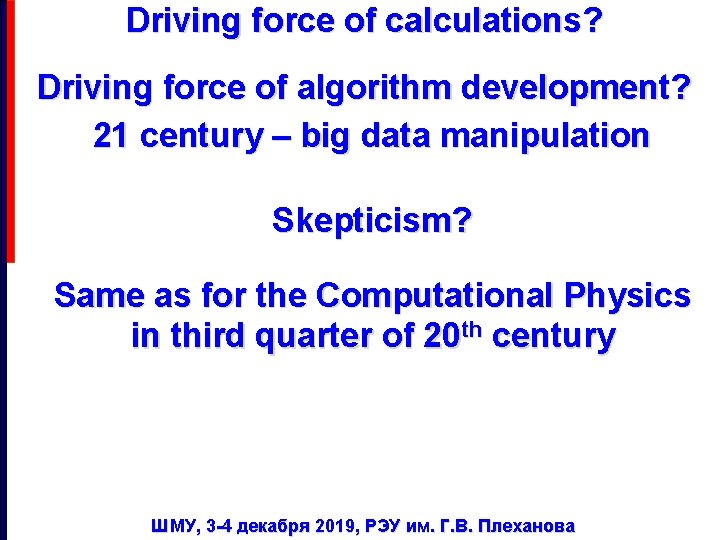
Driving force of calculations? Driving force of algorithm development? 21 century – big data manipulation Skepticism? Same as for the Computational Physics in third quarter of 20 th century ШМУ, 3 -4 декабря 2019, РЭУ им. Г. В. Плеханова
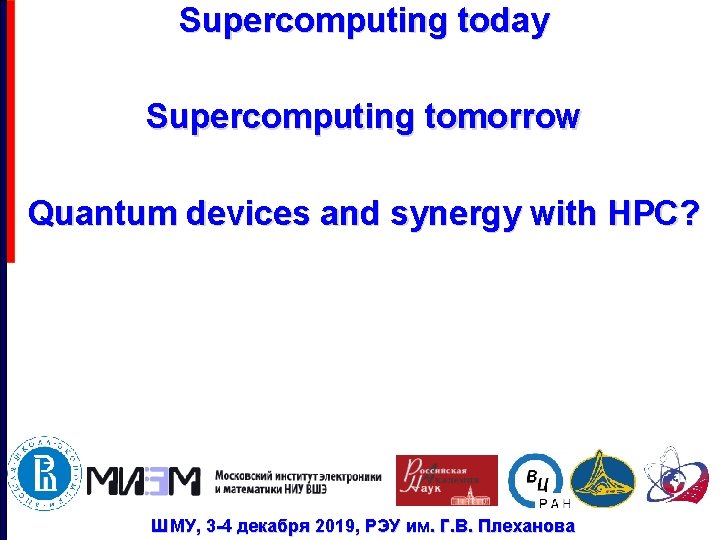
Supercomputing today Supercomputing tomorrow Quantum devices and synergy with HPC? ШМУ, 3 -4 декабря 2019, РЭУ им. Г. В. Плеханова
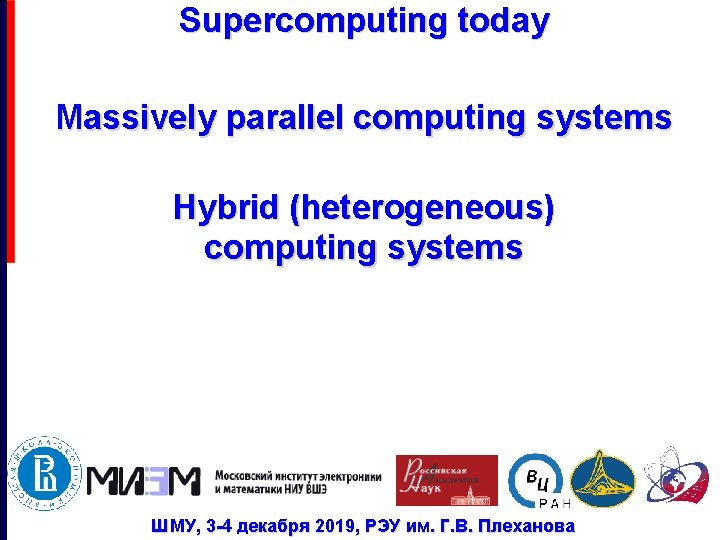
Supercomputing today Massively parallel computing systems Hybrid (heterogeneous) computing systems ШМУ, 3 -4 декабря 2019, РЭУ им. Г. В. Плеханова
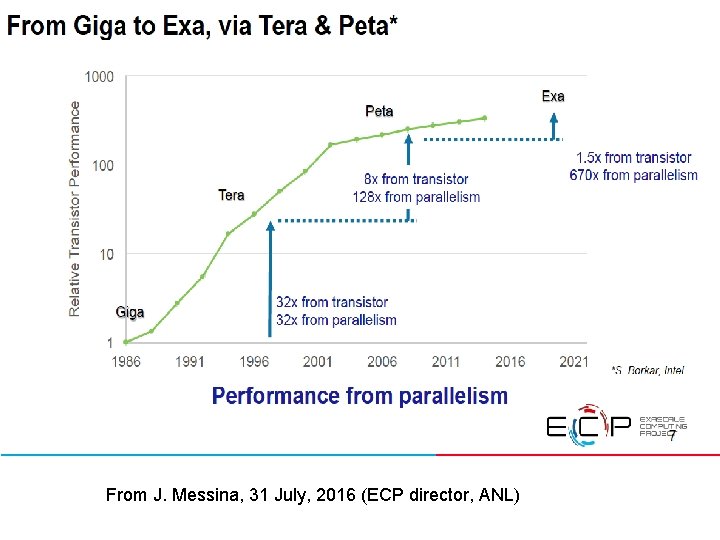
Moore’s law – saturaion? From J. Messina, 31 July, 2016 (ECP director, ANL) 2019 INCITE Allocations by
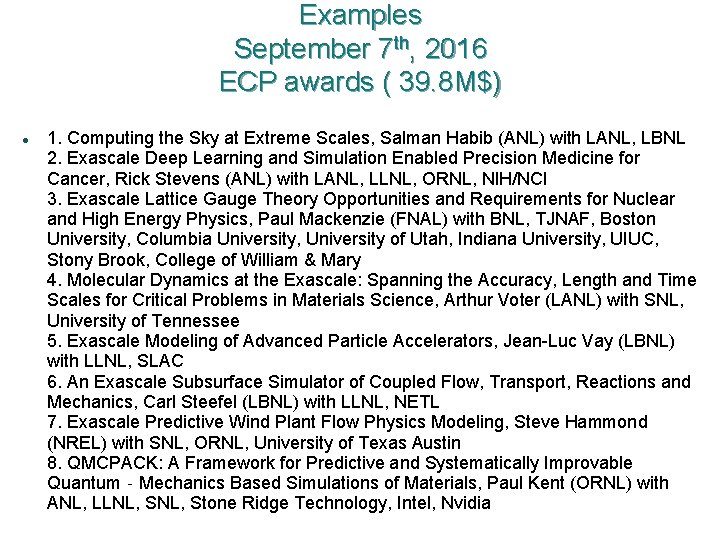
Examples September 7 th, 2016 ECP awards ( 39. 8 M$) 1. Computing the Sky at Extreme Scales, Salman Habib (ANL) with LANL, LBNL 2. Exascale Deep Learning and Simulation Enabled Precision Medicine for Cancer, Rick Stevens (ANL) with LANL, LLNL, ORNL, NIH/NCI 3. Exascale Lattice Gauge Theory Opportunities and Requirements for Nuclear and High Energy Physics, Paul Mackenzie (FNAL) with BNL, TJNAF, Boston University, Columbia University, University of Utah, Indiana University, UIUC, Stony Brook, College of William & Mary 4. Molecular Dynamics at the Exascale: Spanning the Accuracy, Length and Time Scales for Critical Problems in Materials Science, Arthur Voter (LANL) with SNL, University of Tennessee 5. Exascale Modeling of Advanced Particle Accelerators, Jean-Luc Vay (LBNL) with LLNL, SLAC 6. An Exascale Subsurface Simulator of Coupled Flow, Transport, Reactions and Mechanics, Carl Steefel (LBNL) with LLNL, NETL 7. Exascale Predictive Wind Plant Flow Physics Modeling, Steve Hammond (NREL) with SNL, ORNL, University of Texas Austin 8. QMCPACK: A Framework for Predictive and Systematically Improvable Quantum‐Mechanics Based Simulations of Materials, Paul Kent (ORNL) with ANL, LLNL, Stone Ridge Technology, Intel, Nvidia
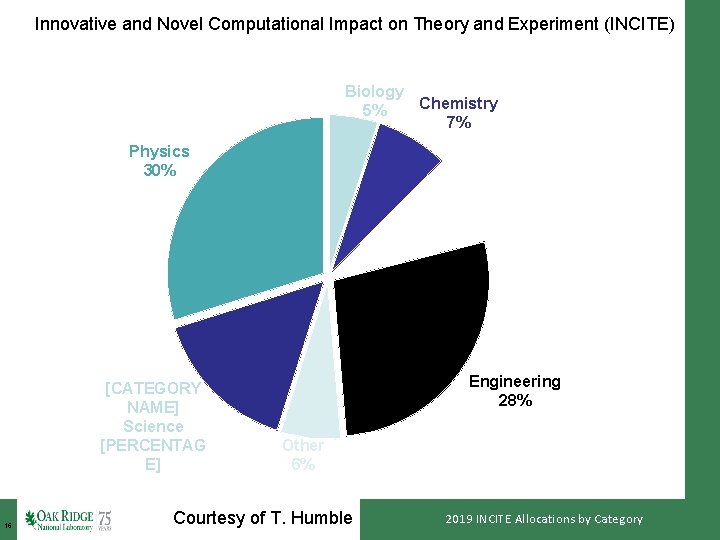
Innovative and Novel Computational Impact on Theory and Experiment (INCITE) Biology Chemistry 5% 7% Physics 30% [CATEGORY NAME] Science [PERCENTAG E] 16 Earth Science 9% Engineering 28% Other 6% Courtesy of T. Humble 2019 INCITE Allocations by Category
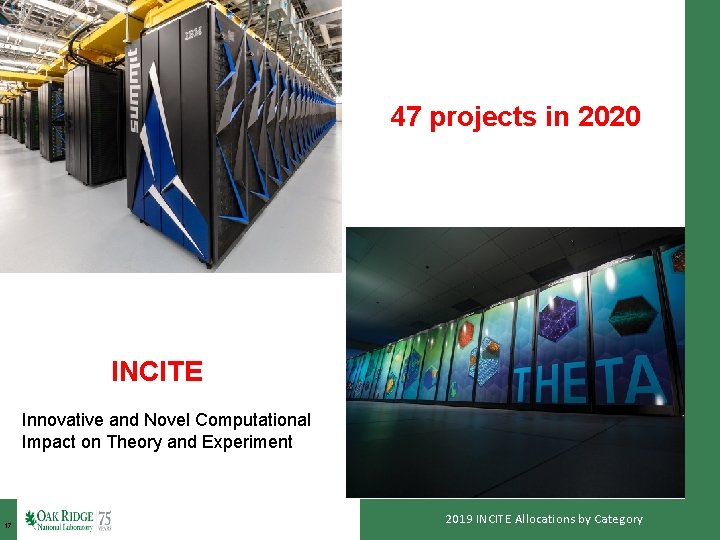
47 projects in 2020 INCITE Innovative and Novel Computational Impact on Theory and Experiment 17 2019 INCITE Allocations by Category
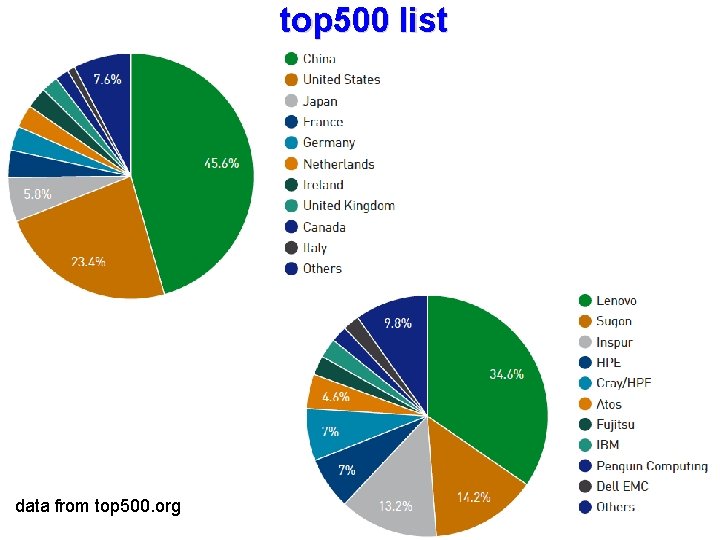
top 500 list data from top 500. org
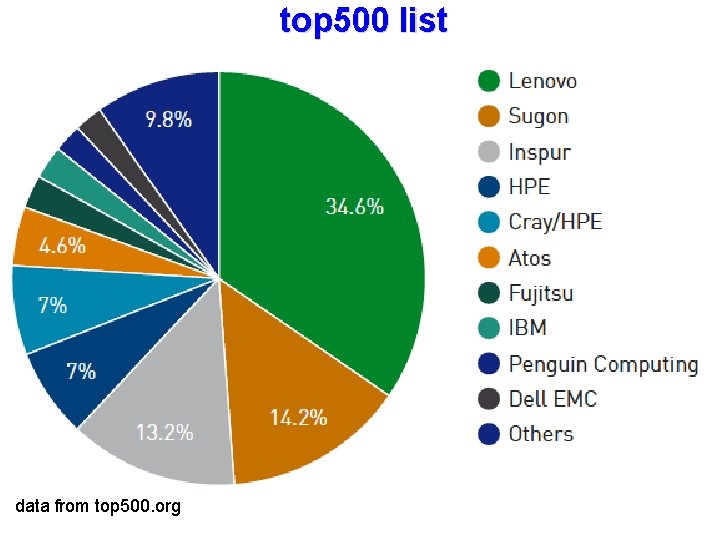
top 500 list data from top 500. org
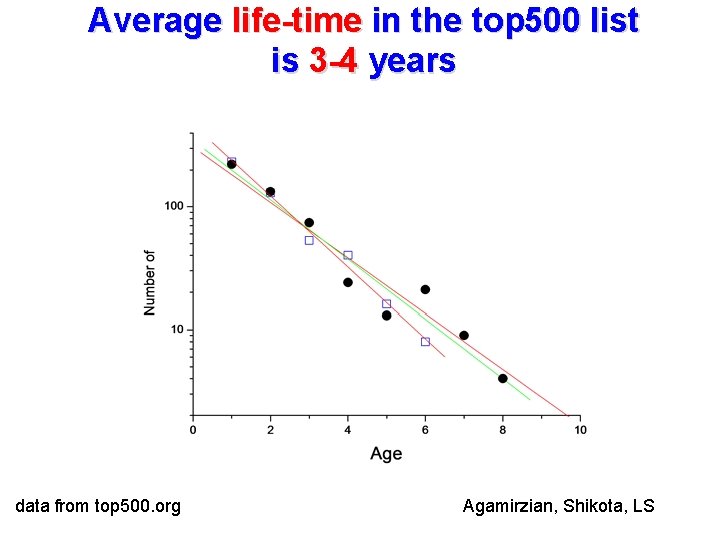
Average life-time in the top 500 list is 3 -4 years data from top 500. org Agamirzian, Shikota, LS
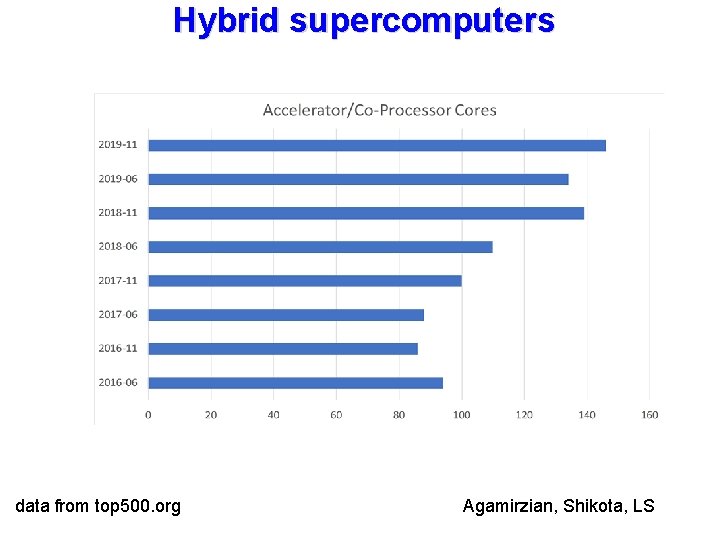
Hybrid supercomputers data from top 500. org Agamirzian, Shikota, LS
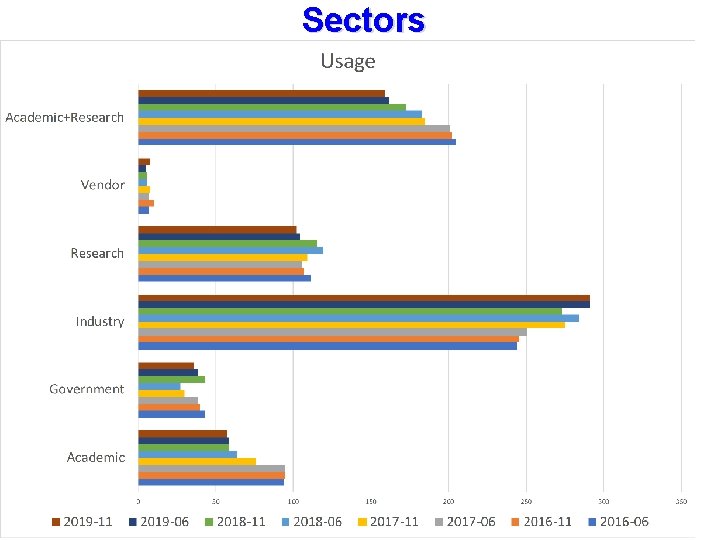
Sectors in the top 500 list is 3 -4 years data from top 500. org Agamirzian, Shikota, LS
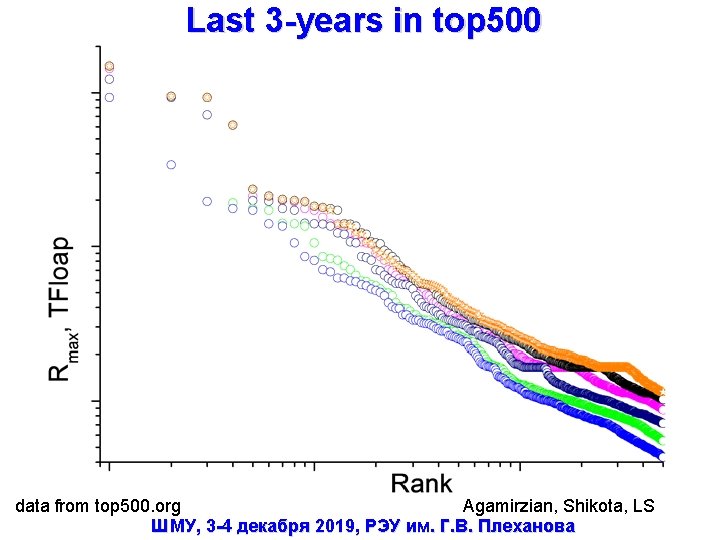
Last 3 -years in top 500 data from top 500. org Agamirzian, Shikota, LS ШМУ, 3 -4 декабря 2019, РЭУ им. Г. В. Плеханова
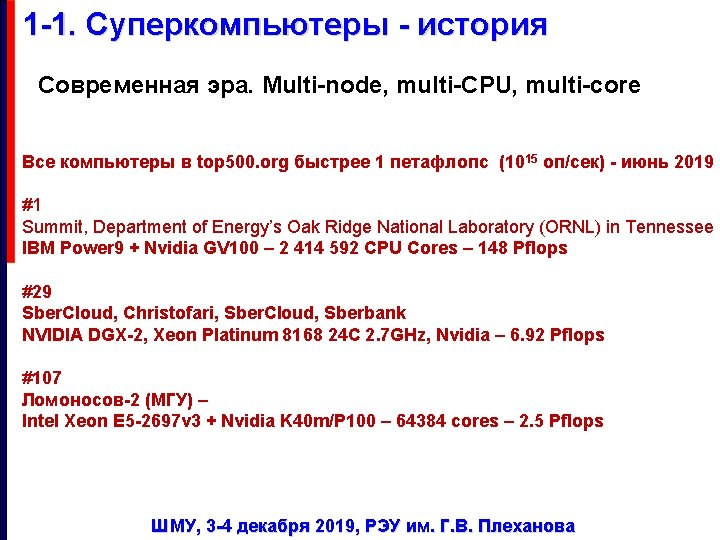
1 -1. Суперкомпьютеры - история Современная эра. Multi-node, multi-CPU, multi-core Все компьютеры в top 500. org быстрее 1 петафлопс (1015 оп/сек) - июнь 2019 #1 Summit, Department of Energy’s Oak Ridge National Laboratory (ORNL) in Tennessee IBM Power 9 + Nvidia GV 100 – 2 414 592 CPU Cores – 148 Pflops #29 Sber. Cloud, Christofari, Sber. Cloud, Sberbank NVIDIA DGX-2, Xeon Platinum 8168 24 C 2. 7 GHz, Nvidia – 6. 92 Pflops #107 Ломоносов-2 (МГУ) – Intel Xeon E 5 -2697 v 3 + Nvidia K 40 m/P 100 – 64384 cores – 2. 5 Pflops ШМУ, 3 -4 декабря 2019, РЭУ им. Г. В. Плеханова
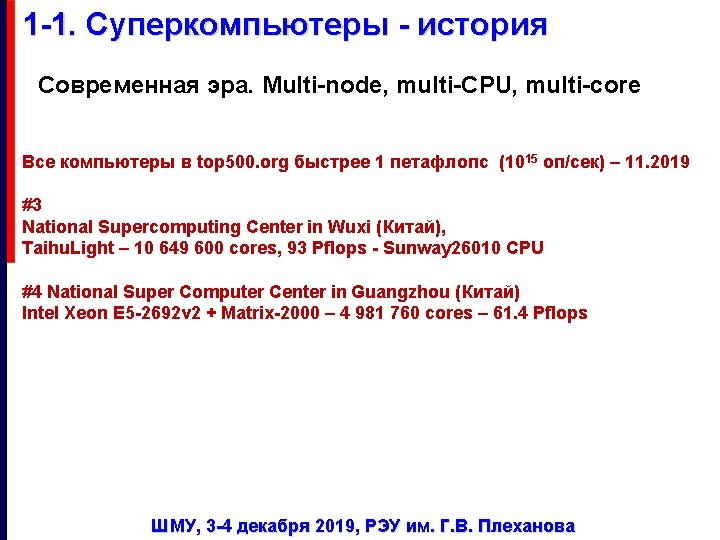
1 -1. Суперкомпьютеры - история Современная эра. Multi-node, multi-CPU, multi-core Все компьютеры в top 500. org быстрее 1 петафлопс (1015 оп/сек) – 11. 2019 #3 National Supercomputing Center in Wuxi (Китай), Taihu. Light – 10 649 600 cores, 93 Pflops - Sunway 26010 CPU #4 National Super Computer Center in Guangzhou (Китай) Intel Xeon E 5 -2692 v 2 + Matrix-2000 – 4 981 760 cores – 61. 4 Pflops ШМУ, 3 -4 декабря 2019, РЭУ им. Г. В. Плеханова
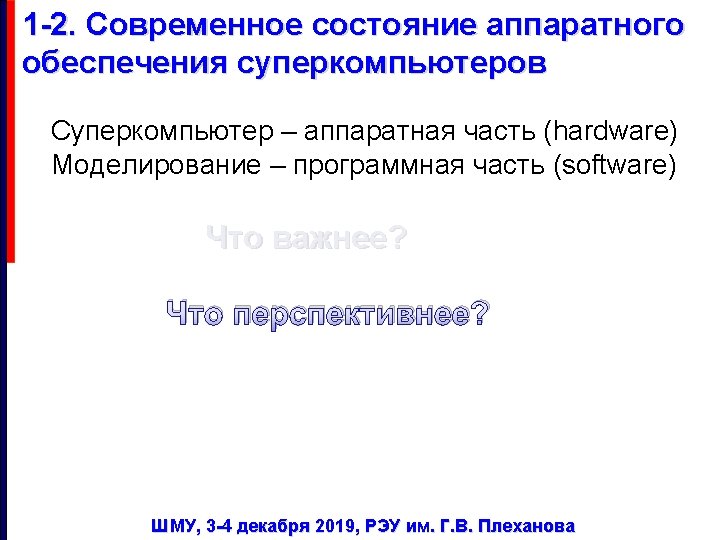
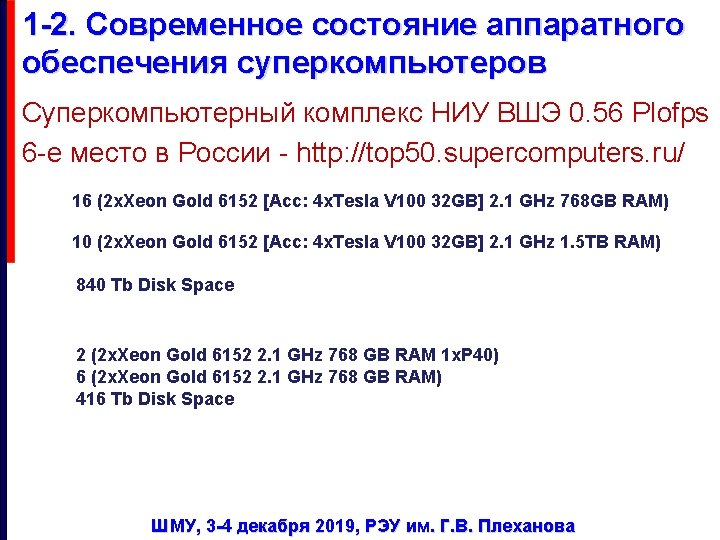
1 -2. Современное состояние аппаратного обеспечения суперкомпьютеров Суперкомпьютерный комплекс НИУ ВШЭ 0. 56 Plofps 6 -е место в России - http: //top 50. supercomputers. ru/ 16 (2 x. Xeon Gold 6152 [Acc: 4 x. Tesla V 100 32 GB] 2. 1 GHz 768 GB RAM) 10 (2 x. Xeon Gold 6152 [Acc: 4 x. Tesla V 100 32 GB] 2. 1 GHz 1. 5 TB RAM) 840 Tb Disk Space 2 (2 x. Xeon Gold 6152 2. 1 GHz 768 GB RAM 1 x. P 40) 6 (2 x. Xeon Gold 6152 2. 1 GHz 768 GB RAM) 416 Tb Disk Space ШМУ, 3 -4 декабря 2019, РЭУ им. Г. В. Плеханова
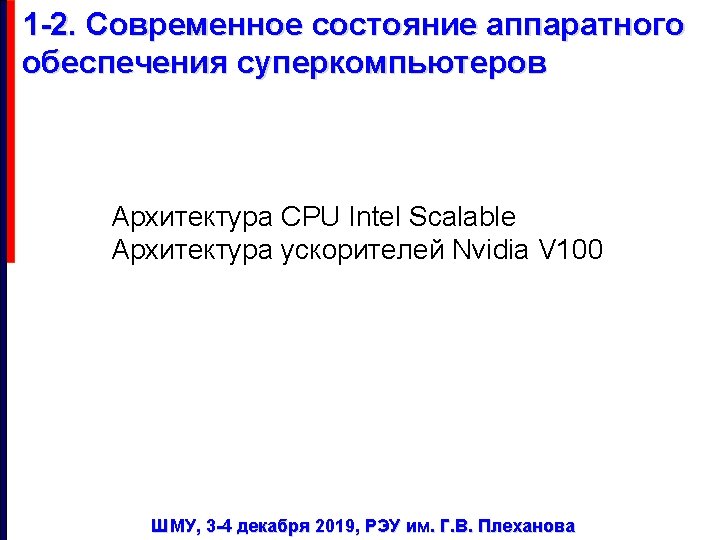
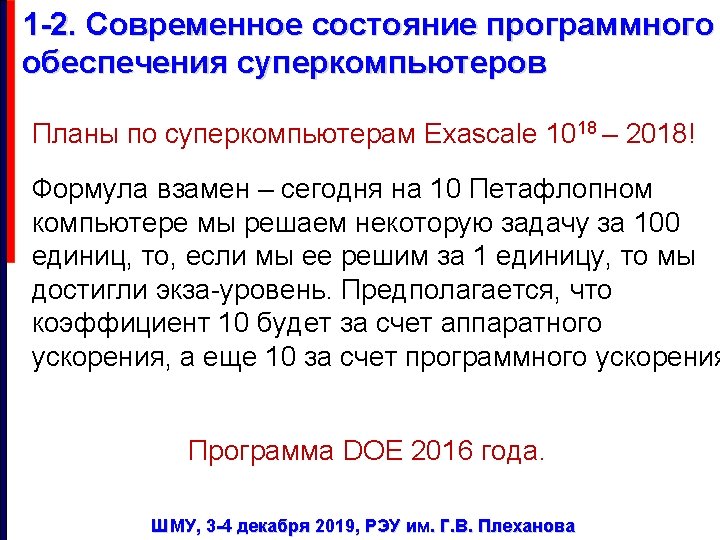
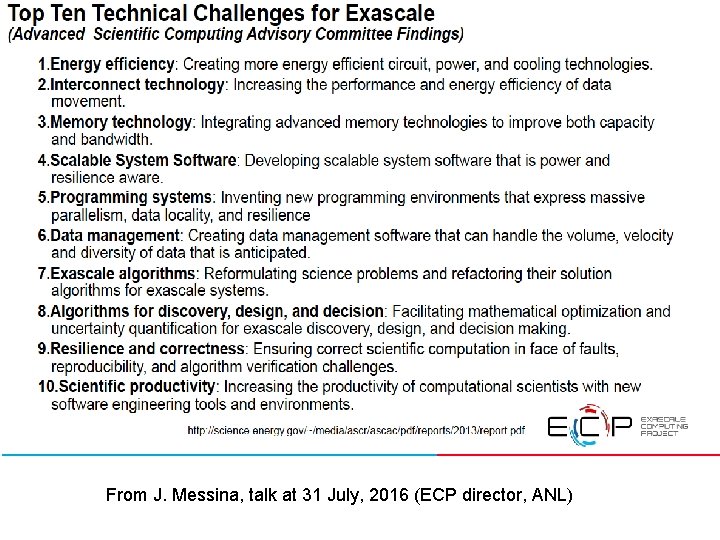
From J. Messina, talk at 31 July, 2016 (ECP director, ANL)
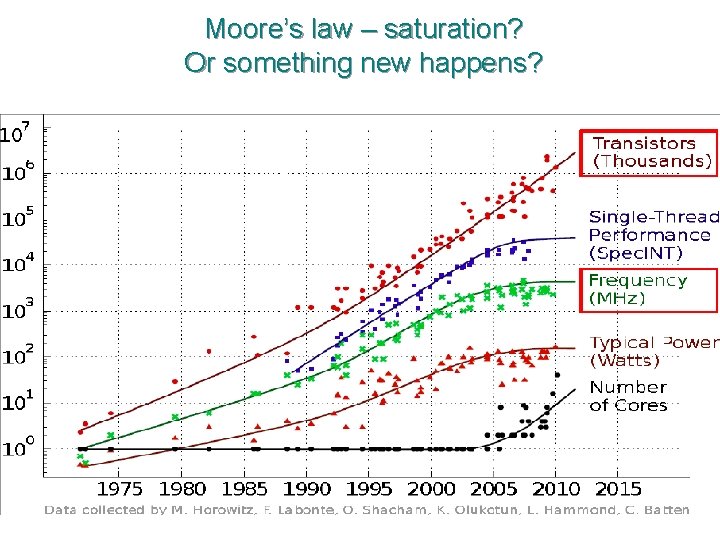
Moore’s law – saturation? Or something new happens?
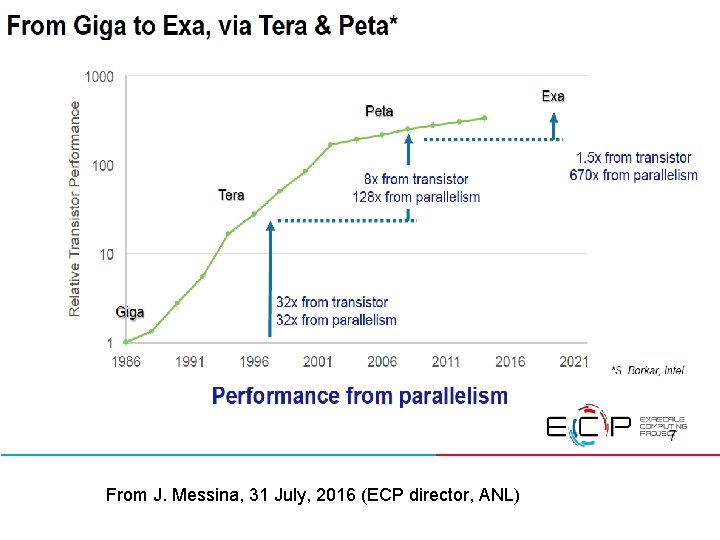
Moore’s law – saturaion? From J. Messina, 31 July, 2016 (ECP director, ANL)
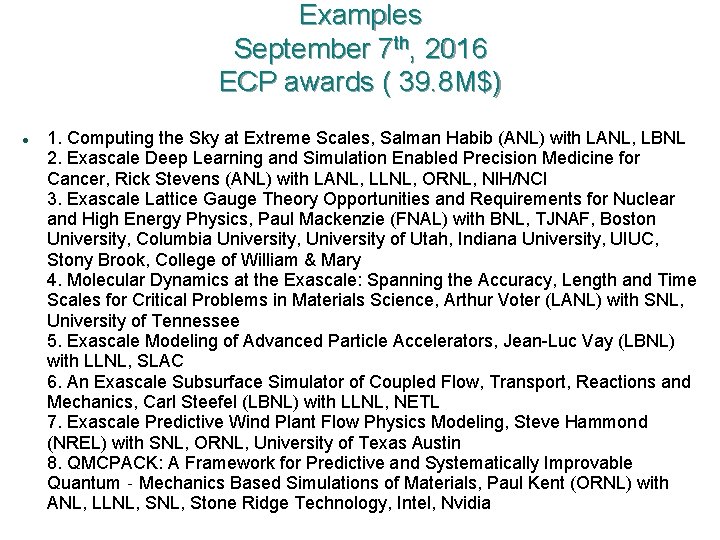
Examples September 7 th, 2016 ECP awards ( 39. 8 M$) 1. Computing the Sky at Extreme Scales, Salman Habib (ANL) with LANL, LBNL 2. Exascale Deep Learning and Simulation Enabled Precision Medicine for Cancer, Rick Stevens (ANL) with LANL, LLNL, ORNL, NIH/NCI 3. Exascale Lattice Gauge Theory Opportunities and Requirements for Nuclear and High Energy Physics, Paul Mackenzie (FNAL) with BNL, TJNAF, Boston University, Columbia University, University of Utah, Indiana University, UIUC, Stony Brook, College of William & Mary 4. Molecular Dynamics at the Exascale: Spanning the Accuracy, Length and Time Scales for Critical Problems in Materials Science, Arthur Voter (LANL) with SNL, University of Tennessee 5. Exascale Modeling of Advanced Particle Accelerators, Jean-Luc Vay (LBNL) with LLNL, SLAC 6. An Exascale Subsurface Simulator of Coupled Flow, Transport, Reactions and Mechanics, Carl Steefel (LBNL) with LLNL, NETL 7. Exascale Predictive Wind Plant Flow Physics Modeling, Steve Hammond (NREL) with SNL, ORNL, University of Texas Austin 8. QMCPACK: A Framework for Predictive and Systematically Improvable Quantum‐Mechanics Based Simulations of Materials, Paul Kent (ORNL) with ANL, LLNL, Stone Ridge Technology, Intel, Nvidia
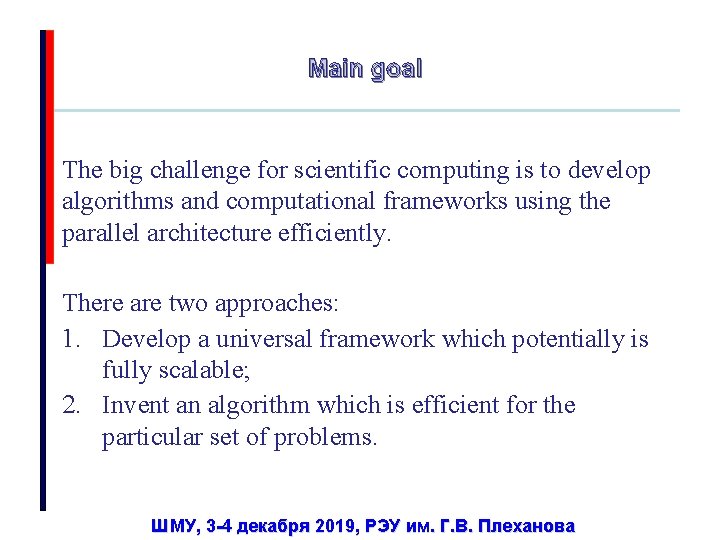
Main goal The big challenge for scientific computing is to develop algorithms and computational frameworks using the parallel architecture efficiently. There are two approaches: 1. Develop a universal framework which potentially is fully scalable; 2. Invent an algorithm which is efficient for the particular set of problems. ШМУ, 3 -4 декабря 2019, РЭУ им. Г. В. Плеханова
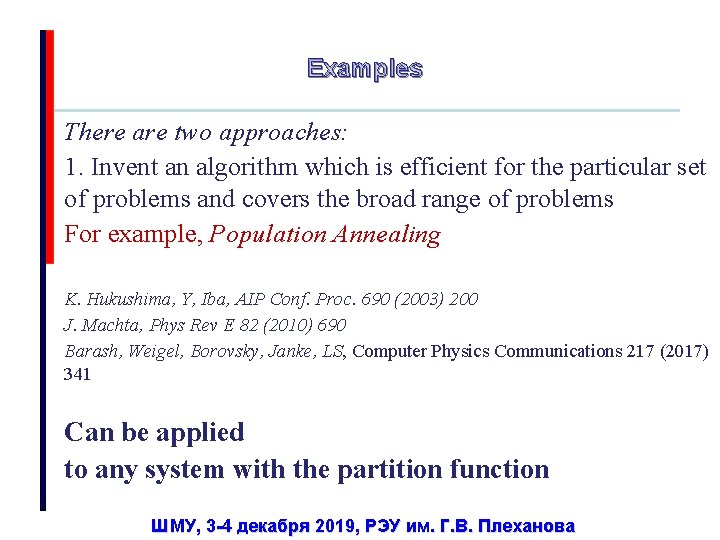
Examples There are two approaches: 1. Invent an algorithm which is efficient for the particular set of problems and covers the broad range of problems For example, Population Annealing K. Hukushima, Y, Iba, AIP Conf. Proc. 690 (2003) 200 J. Machta, Phys Rev E 82 (2010) 690 Barash, Weigel, Borovsky, Janke, LS, Computer Physics Communications 217 (2017) 341 Can be applied to any system with the partition function ШМУ, 3 -4 декабря 2019, РЭУ им. Г. В. Плеханова
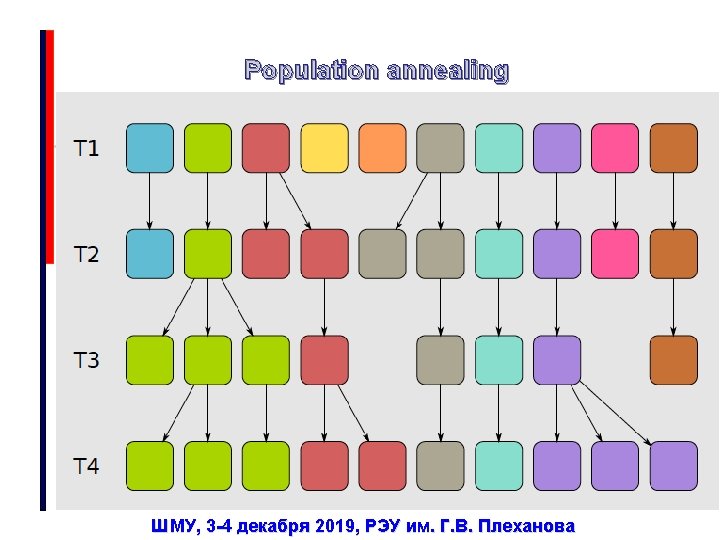
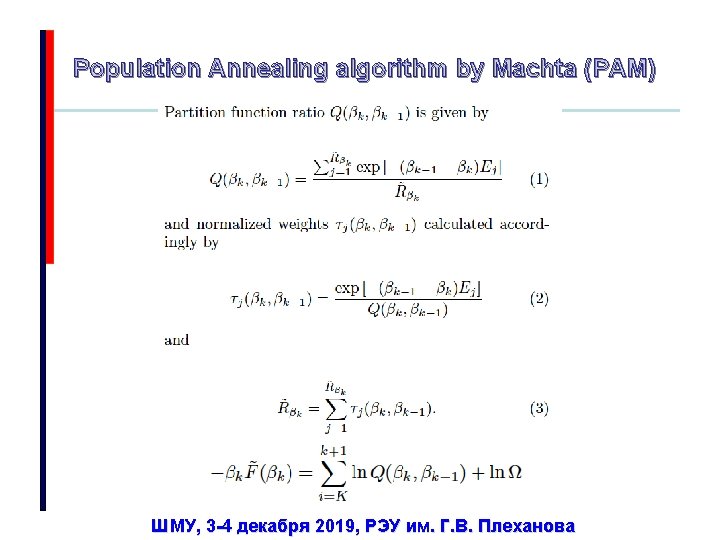
Population Annealing algorithm by Machta (PAM) ШМУ, 3 -4 декабря 2019, РЭУ им. Г. В. Плеханова
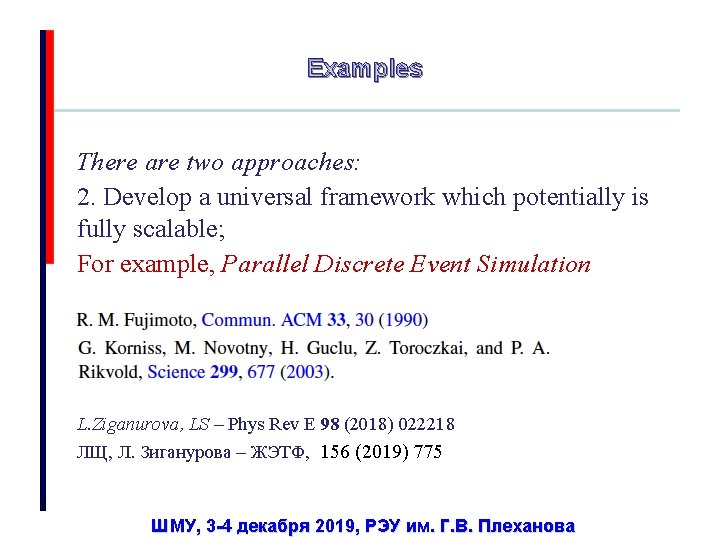
Examples There are two approaches: 2. Develop a universal framework which potentially is fully scalable; For example, Parallel Discrete Event Simulation L. Ziganurova, LS – Phys Rev E 98 (2018) 022218 ЛЩ, Л. Зиганурова – ЖЭТФ, 156 (2019) 775 ШМУ, 3 -4 декабря 2019, РЭУ им. Г. В. Плеханова
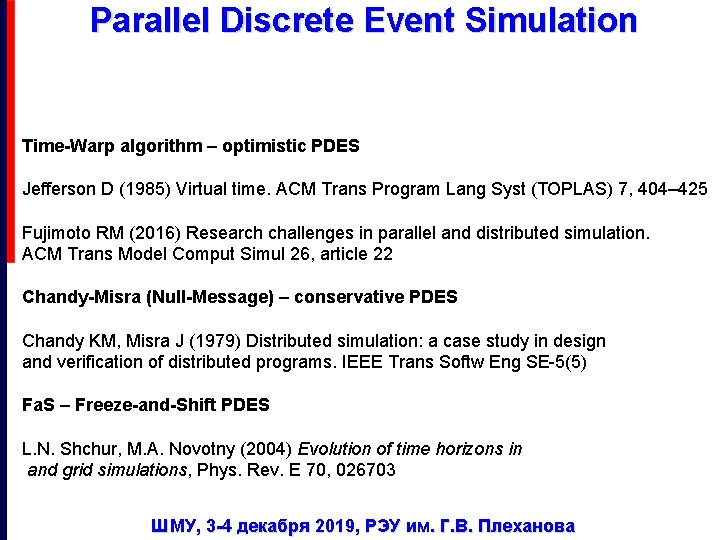
Parallel Discrete Event Simulation Time-Warp algorithm – optimistic PDES Jefferson D (1985) Virtual time. ACM Trans Program Lang Syst (TOPLAS) 7, 404– 425 Fujimoto RM (2016) Research challenges in parallel and distributed simulation. ACM Trans Model Comput Simul 26, article 22 Chandy-Misra (Null-Message) – conservative PDES Chandy KM, Misra J (1979) Distributed simulation: a case study in design and verification of distributed programs. IEEE Trans Softw Eng SE-5(5) Fa. S – Freeze-and-Shift PDES L. N. Shchur, M. A. Novotny (2004) Evolution of time horizons in and grid simulations, Phys. Rev. E 70, 026703 ШМУ, 3 -4 декабря 2019, РЭУ им. Г. В. Плеханова
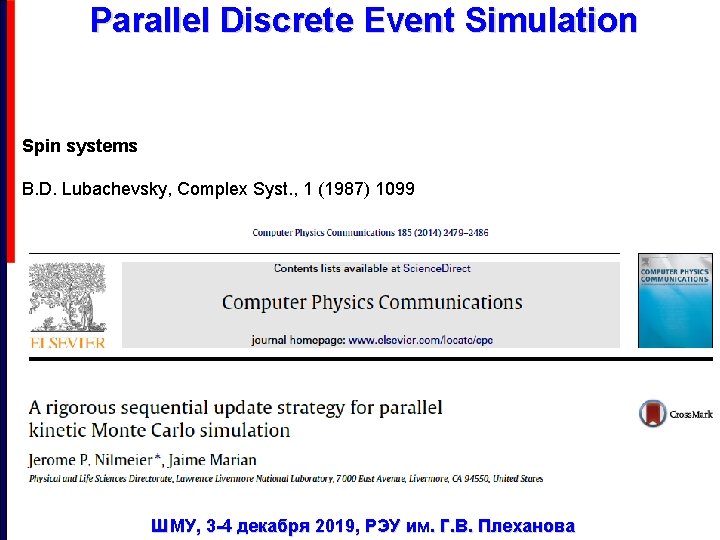
Parallel Discrete Event Simulation Spin systems B. D. Lubachevsky, Complex Syst. , 1 (1987) 1099 ШМУ, 3 -4 декабря 2019, РЭУ им. Г. В. Плеханова
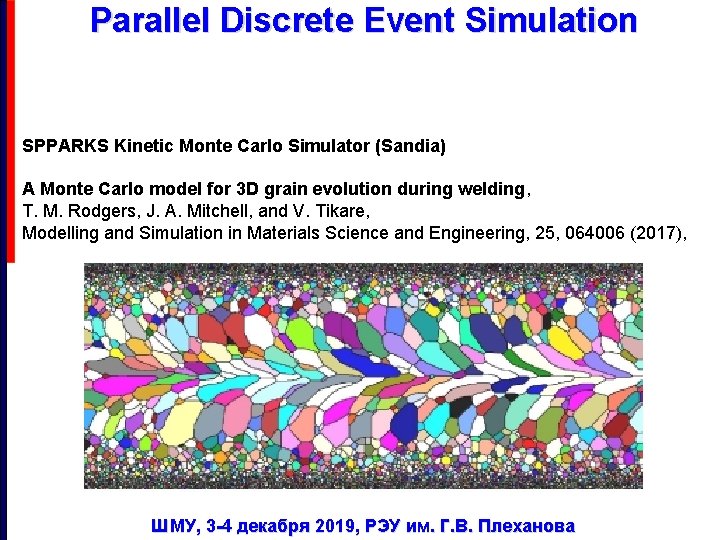
Parallel Discrete Event Simulation SPPARKS Kinetic Monte Carlo Simulator (Sandia) A Monte Carlo model for 3 D grain evolution during welding, T. M. Rodgers, J. A. Mitchell, and V. Tikare, Modelling and Simulation in Materials Science and Engineering, 25, 064006 (2017), ШМУ, 3 -4 декабря 2019, РЭУ им. Г. В. Плеханова
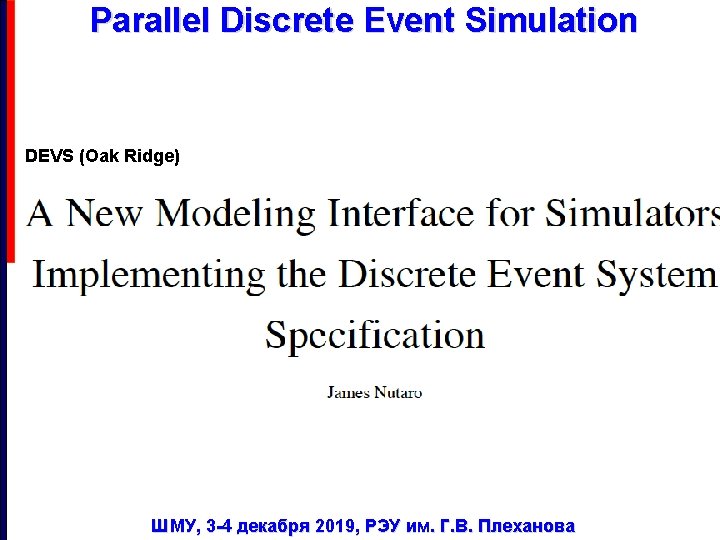
Parallel Discrete Event Simulation DEVS (Oak Ridge) ШМУ, 3 -4 декабря 2019, РЭУ им. Г. В. Плеханова
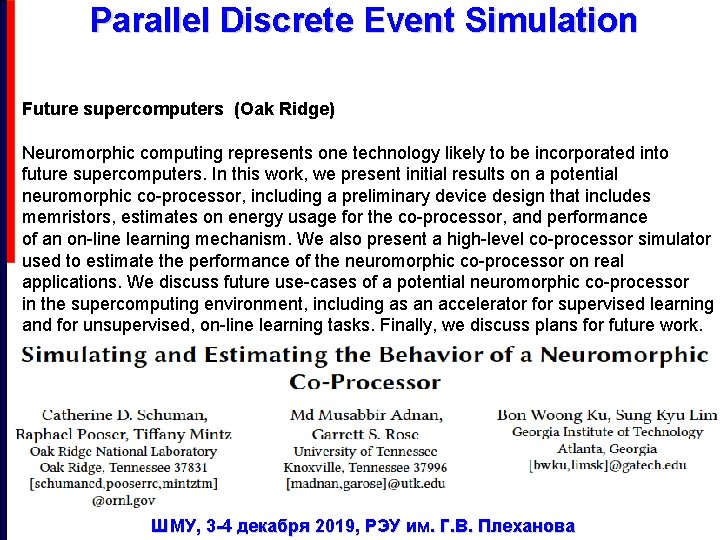
Parallel Discrete Event Simulation Future supercomputers (Oak Ridge) Neuromorphic computing represents one technology likely to be incorporated into future supercomputers. In this work, we present initial results on a potential neuromorphic co-processor, including a preliminary device design that includes memristors, estimates on energy usage for the co-processor, and performance of an on-line learning mechanism. We also present a high-level co-processor simulator used to estimate the performance of the neuromorphic co-processor on real applications. We discuss future use-cases of a potential neuromorphic co-processor in the supercomputing environment, including as an accelerator for supervised learning and for unsupervised, on-line learning tasks. Finally, we discuss plans for future work. ШМУ, 3 -4 декабря 2019, РЭУ им. Г. В. Плеханова
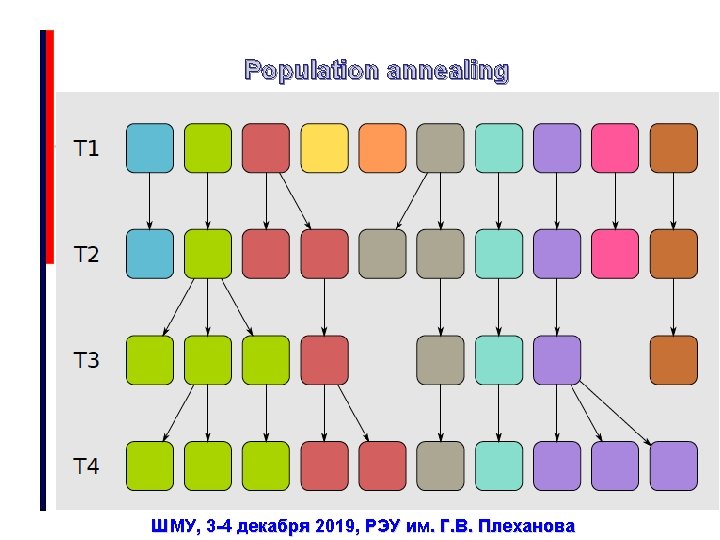
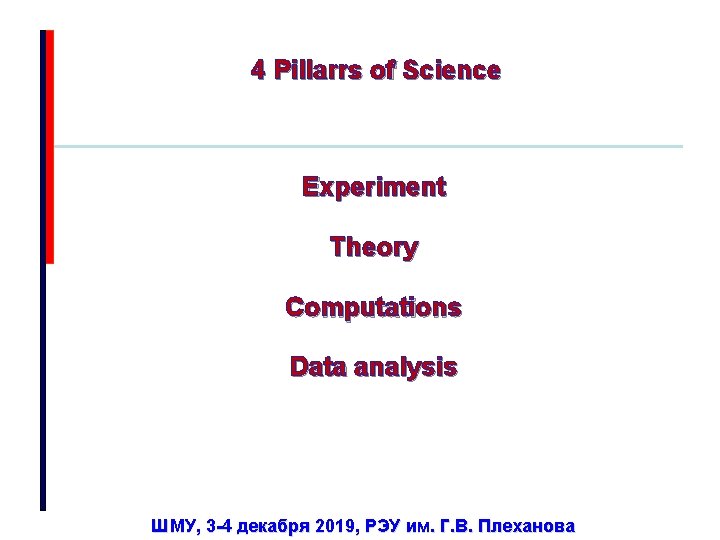
4 Pillarrs of Science Experiment Theory Computations Data analysis ШМУ, 3 -4 декабря 2019, РЭУ им. Г. В. Плеханова
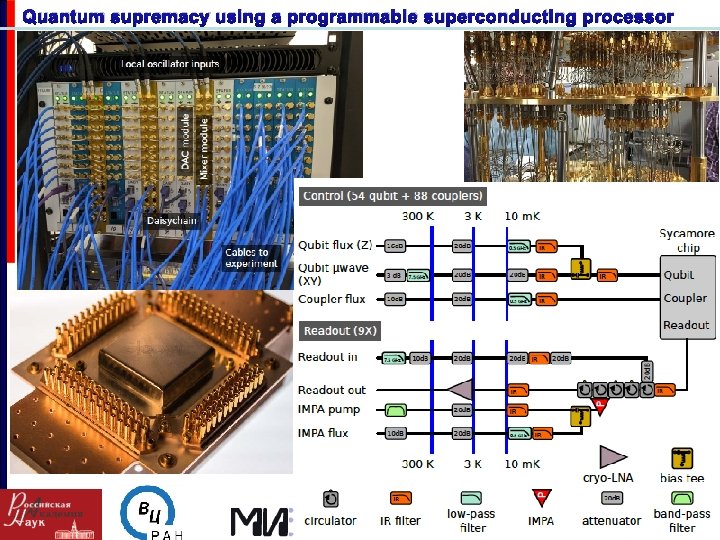
Quantum supremacy using a programmable superconducting processor
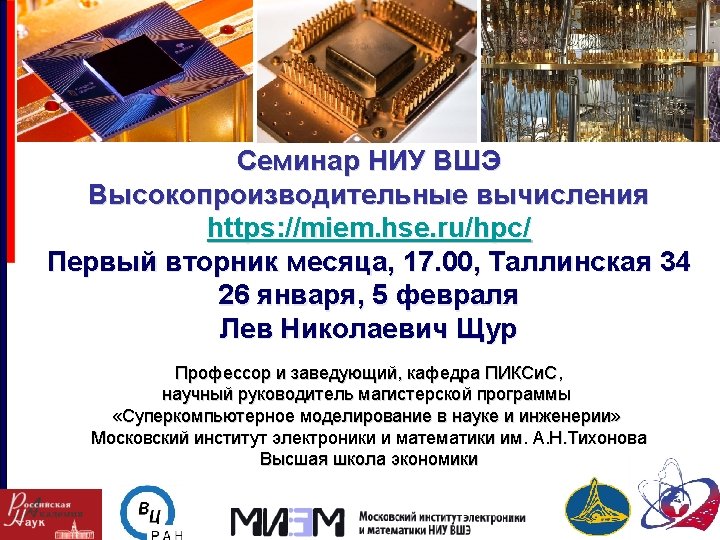
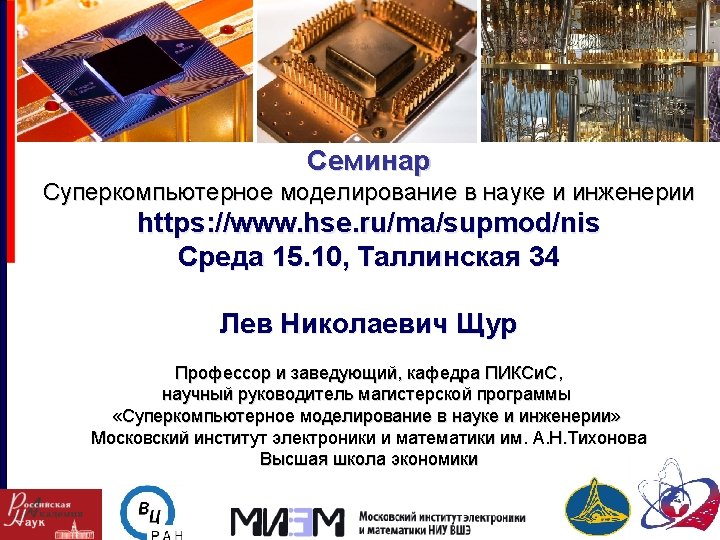
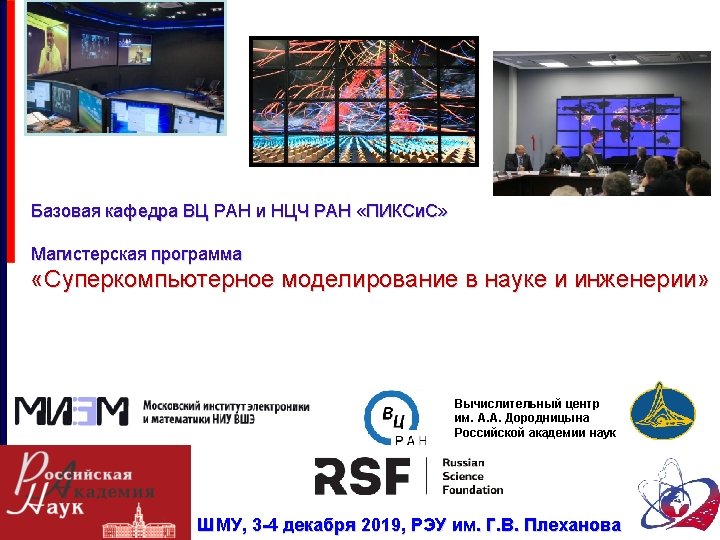
Structural steel connection calculations calculations
Bmd and sfd diagrams
Ao* search algorithm
Prof. slim codeforces
Driving force of plate tectonics
Driving force
The driving force behind european imperialism in africa
What is the primary driving force of surface-ocean currents
Driving force
Driving force
Driving force
Driving force
Driving force
Driving force
Linear search brute force
Brute force design
Brute force polynomial evaluation algorithm
Explain the features of bayesian learning methods
Knapsack problem
Force-directed algorithm
Brute force polynomial evaluation algorithm
Brute force polynomial evaluation algorithm
Pattern matching
Hamiltonian circuit examples
Dividing head calculation formula
Vitamin c titration calculations
Thinking algebraically stock market game answer key
Level circuit surveying
The stock market game activity sheet 2 answers
The calculation of quantities in chemical reactions
Standard molar enthalpy equation
How to do order of magnitude
Sap campus management
Condensed q method
Tray dryer calculations
Double pipe heat exchanger calculations
How to calculate percentage strength of a solution
Pharmaceutical calculations percentage strength
Tpn calculation example
Drip calculations
Mixer sizing calculations
Tariff meaning in mathematical literacy
How to do tpn calculations
Sap calculations lewisham
Fire sprinkler hydraulic calculation example
Chapter 2 measurements and calculations
Graham's law of effusion
Reverse calculations to check answers
Hess law for combustion
Electric machine design coaching