Descriptive vs Analytic Epidemiology Descriptive Epidemiology deals with
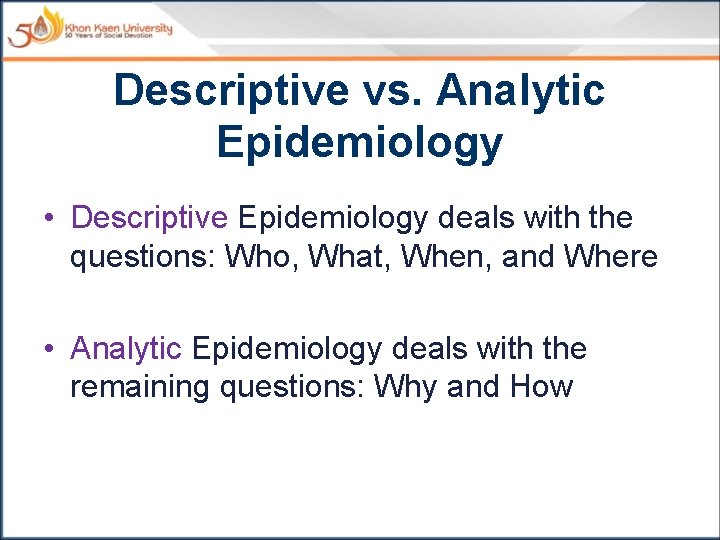
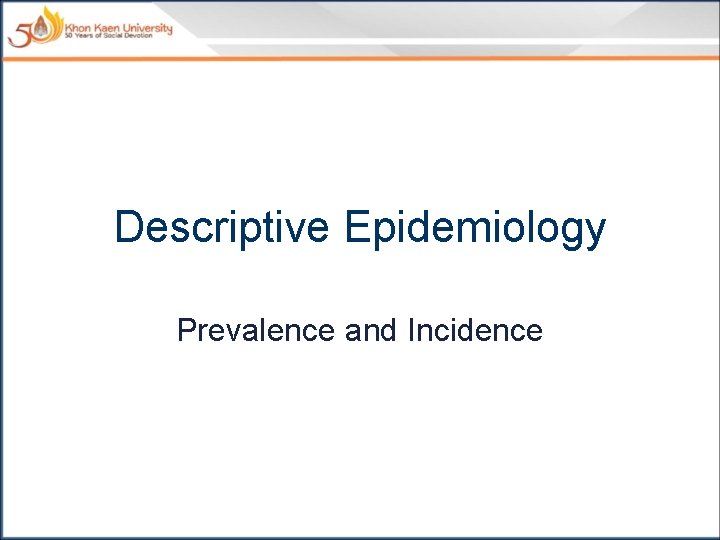
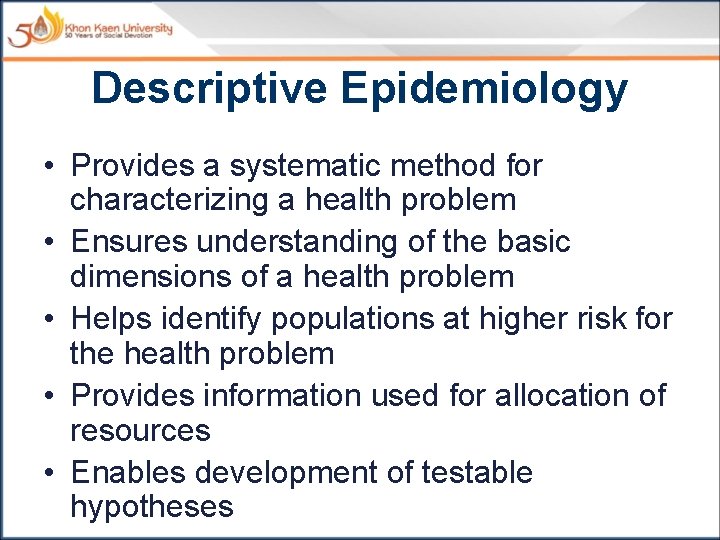
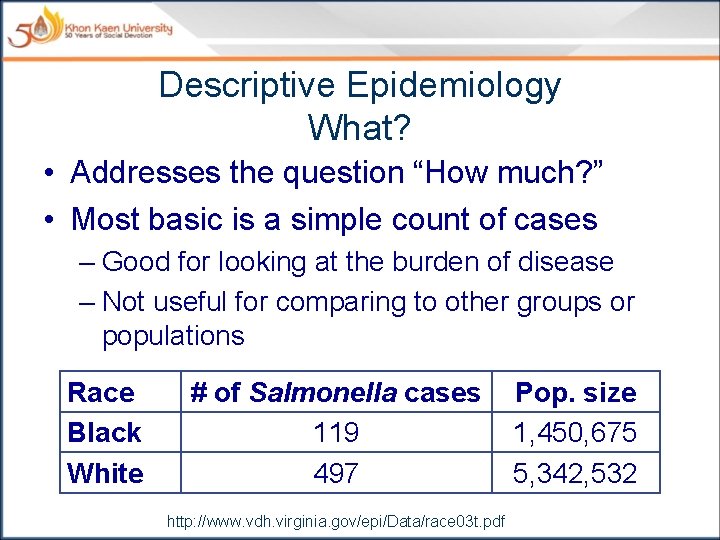
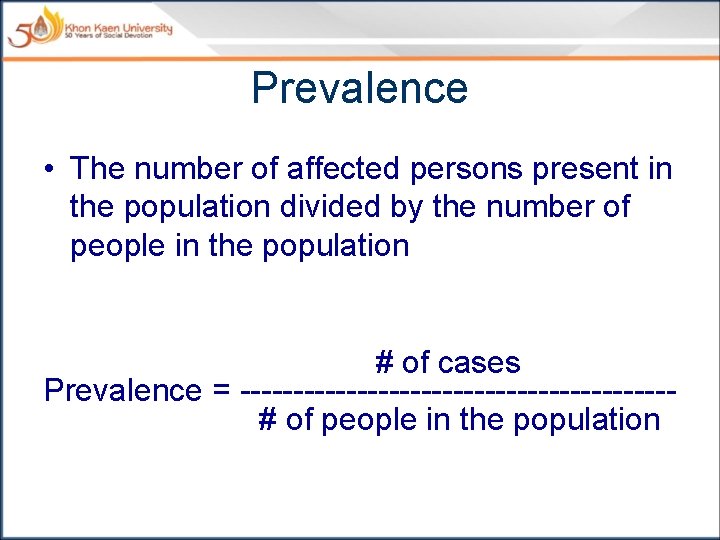
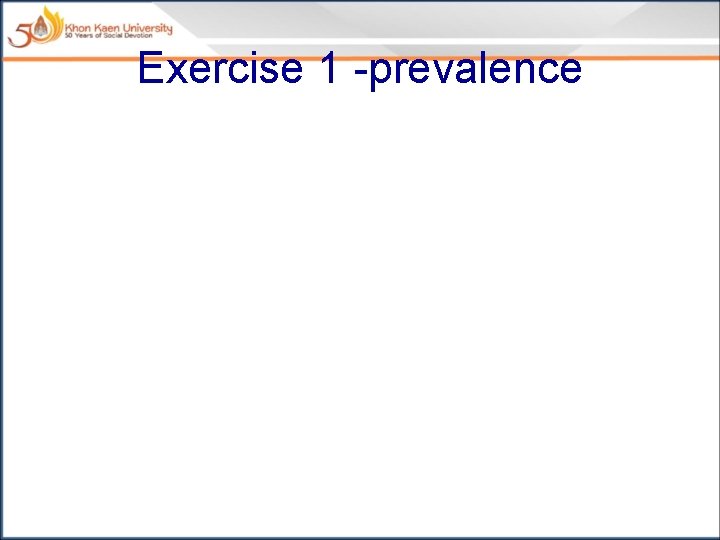
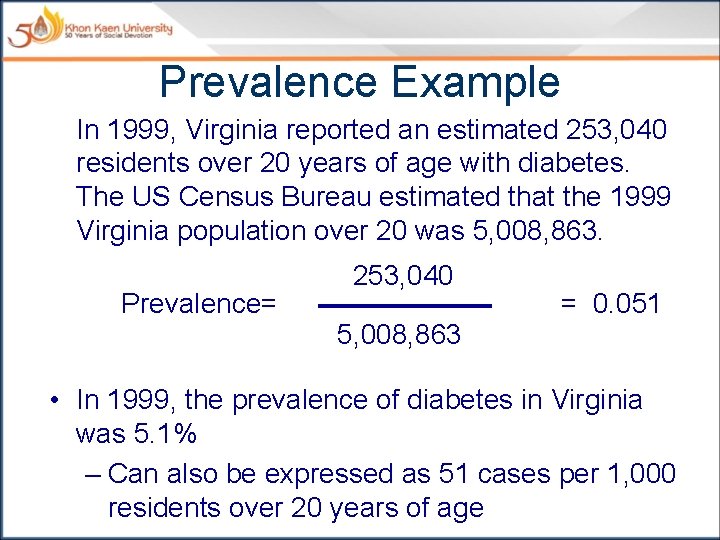
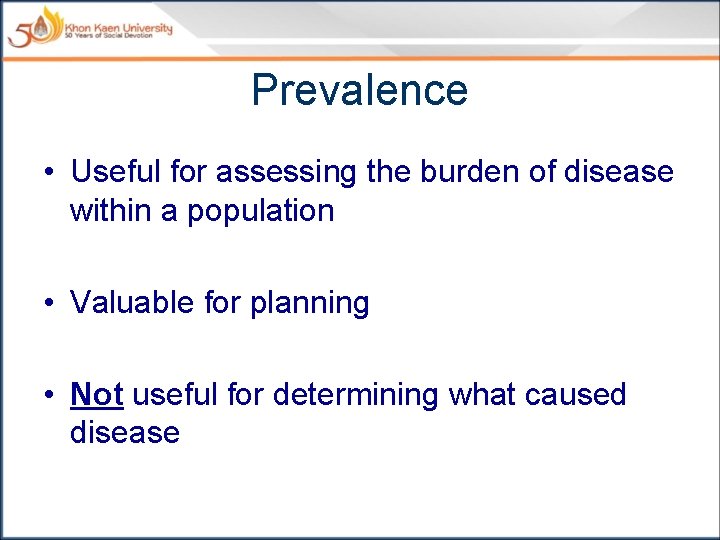
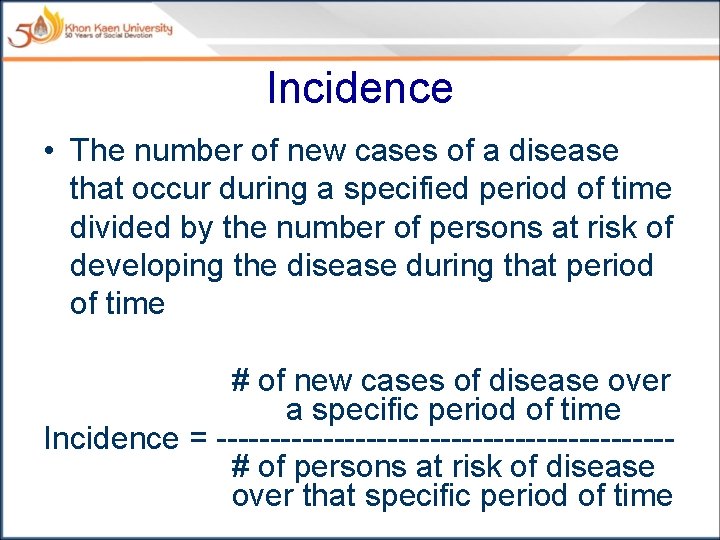
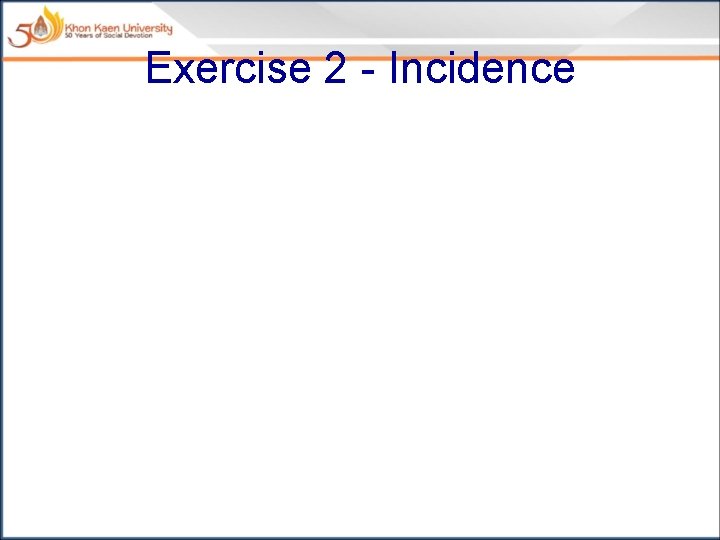
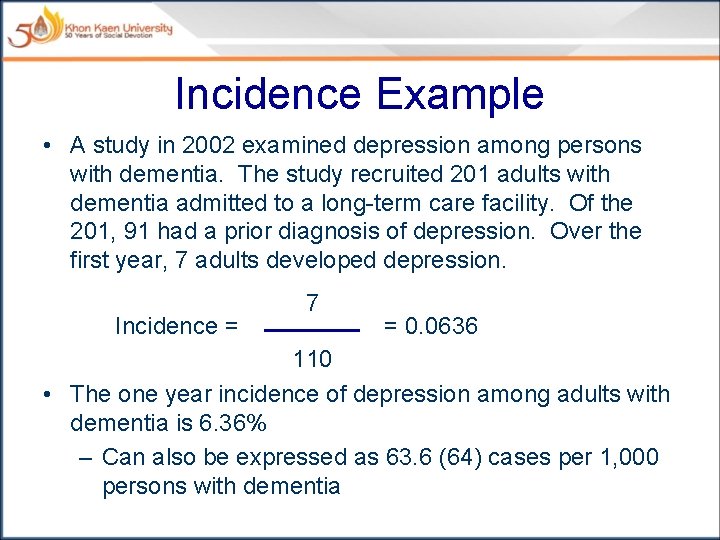
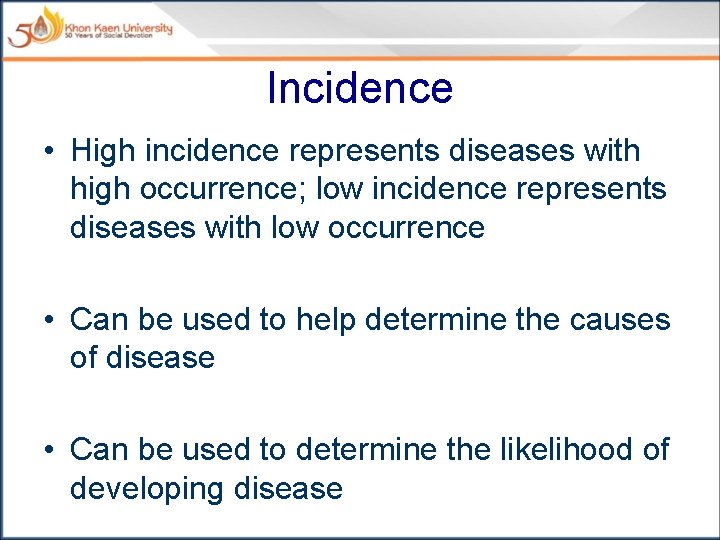
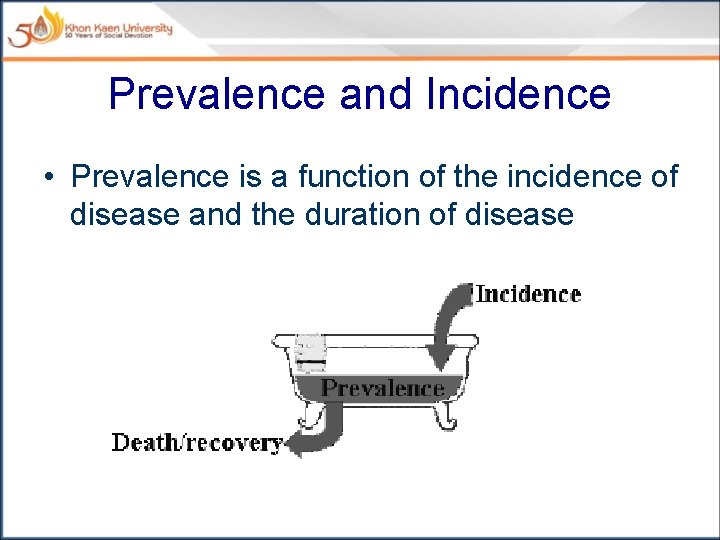
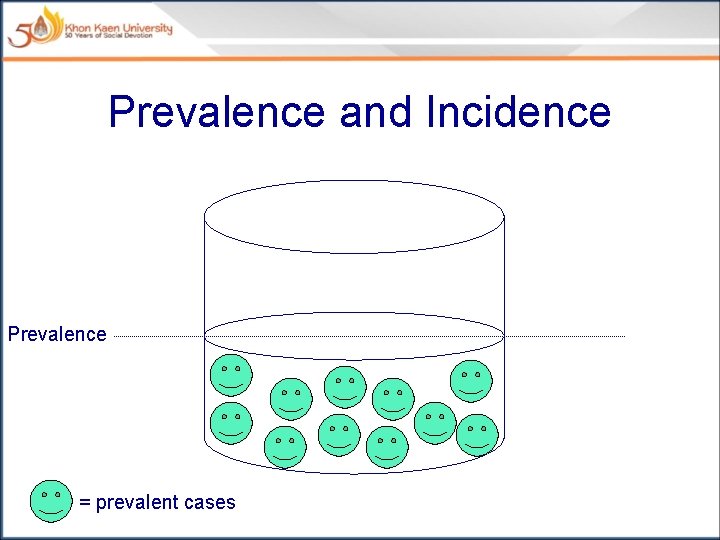
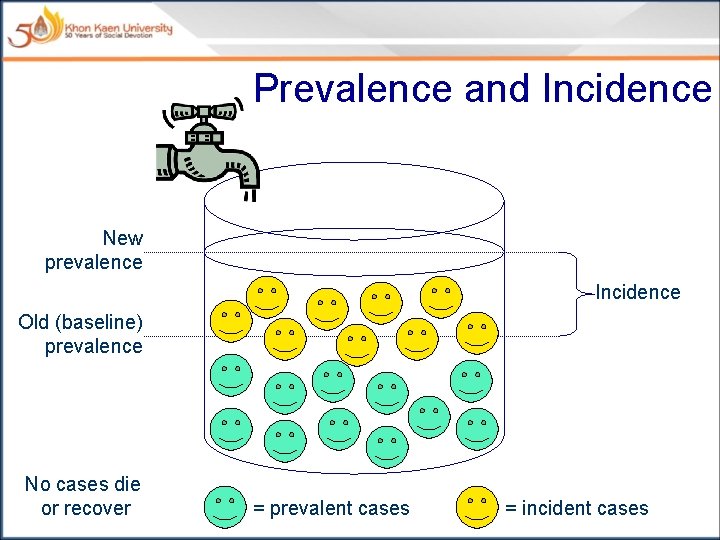
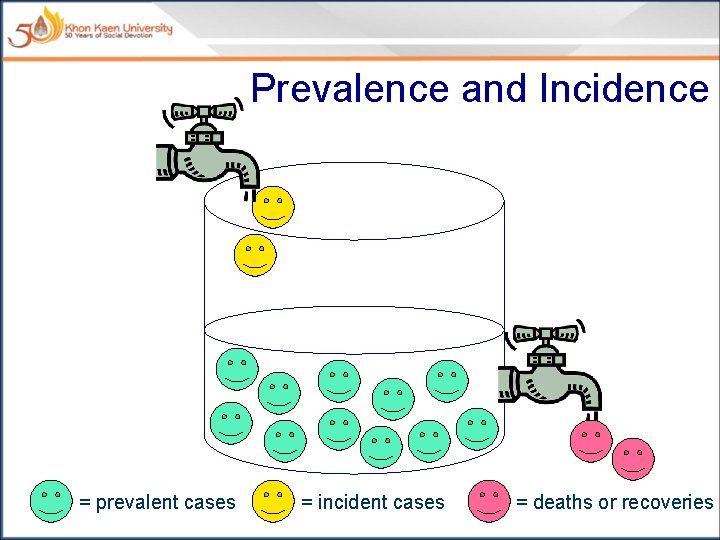
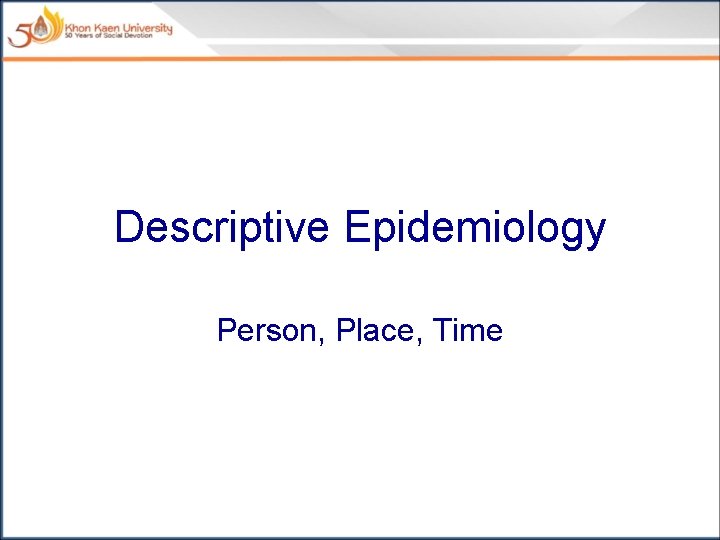
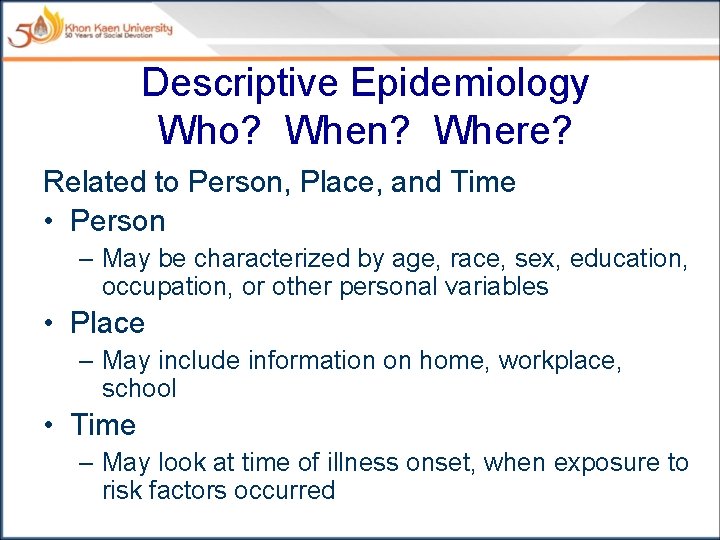
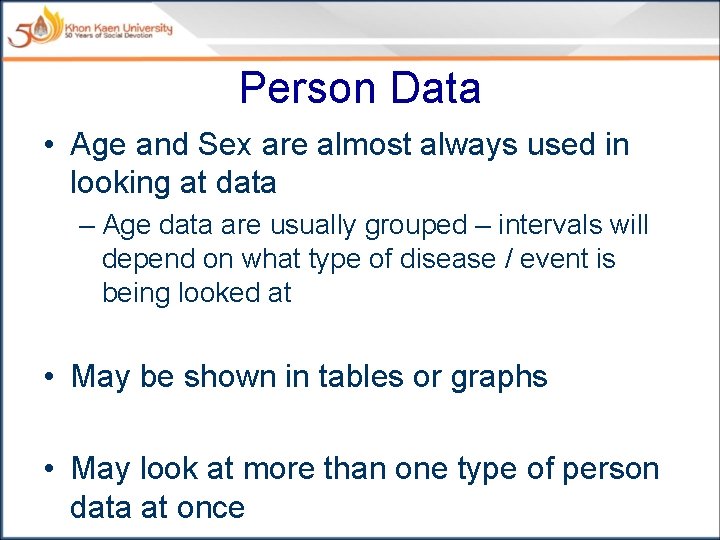
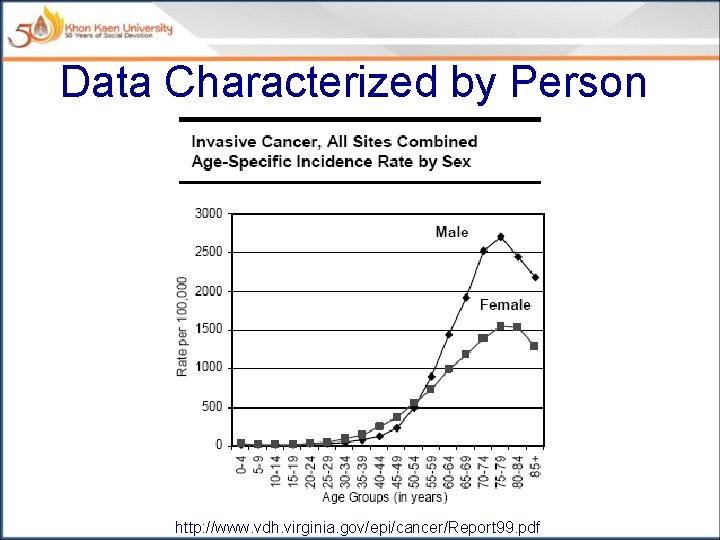
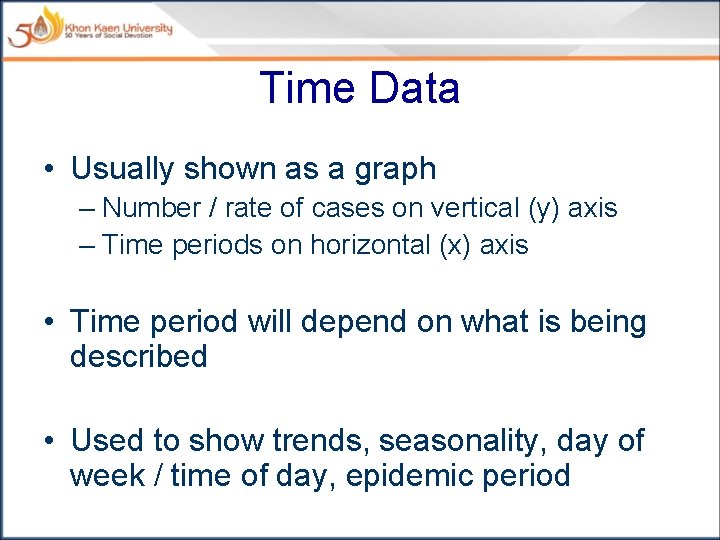
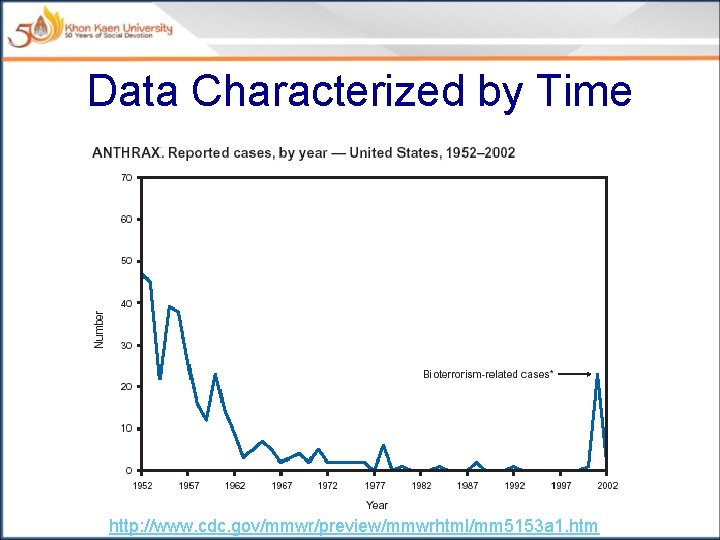
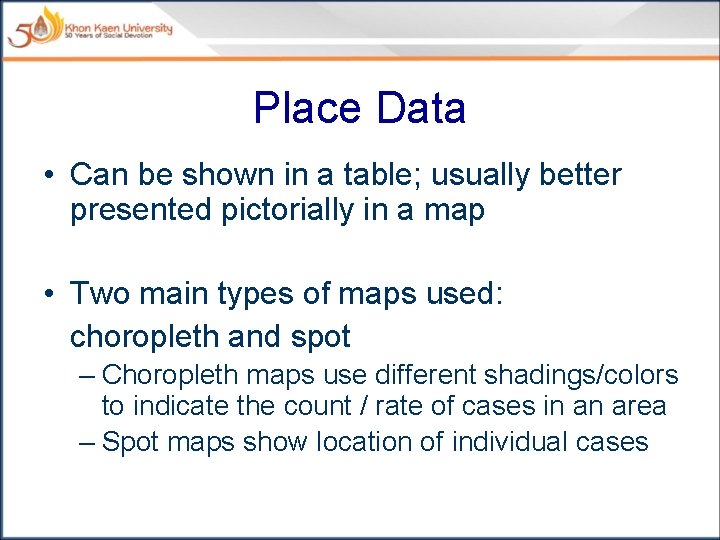
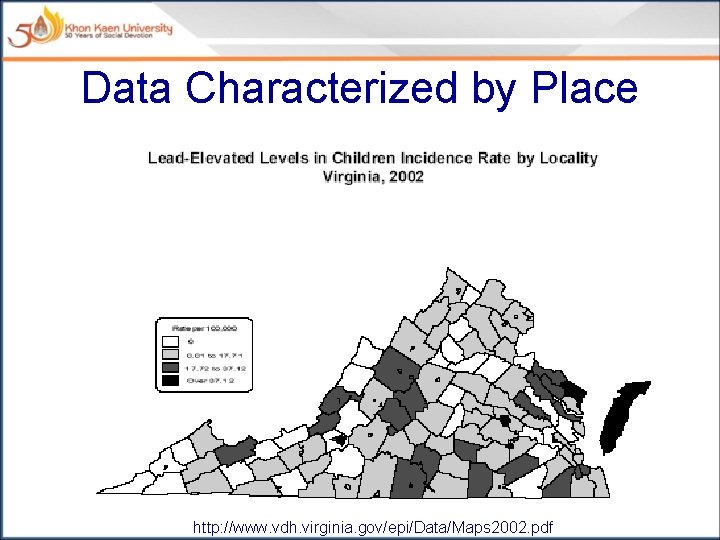
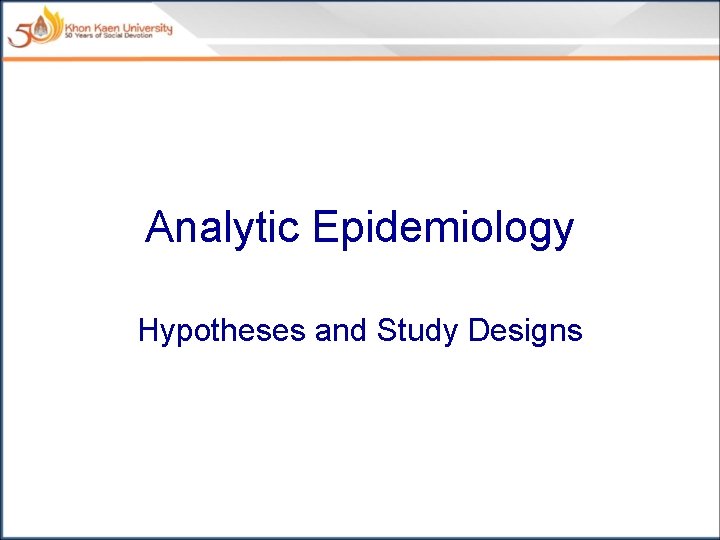
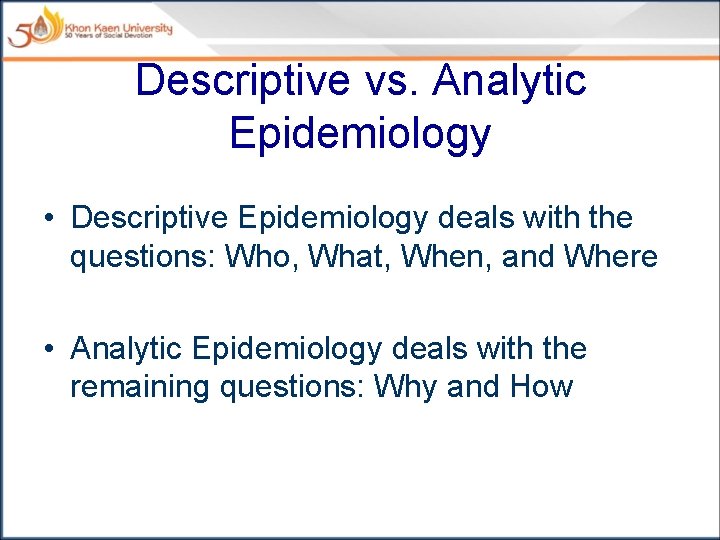
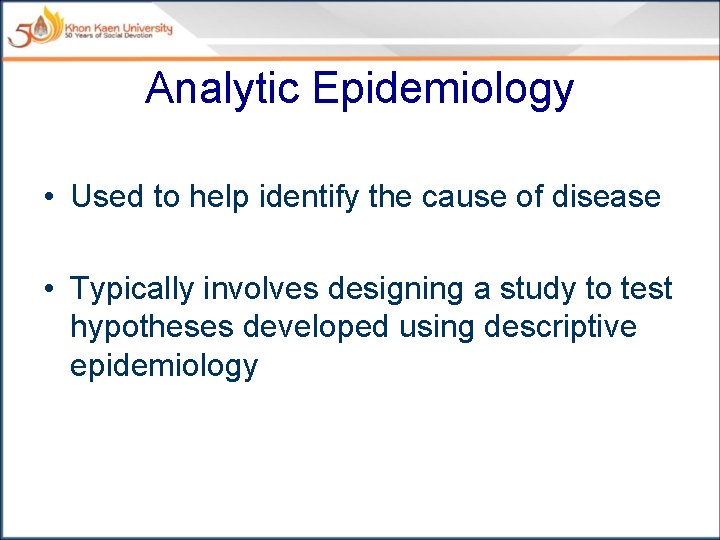
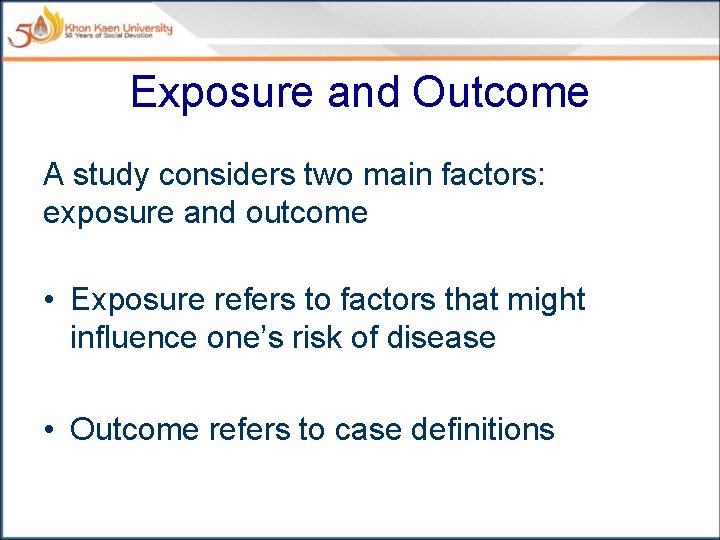
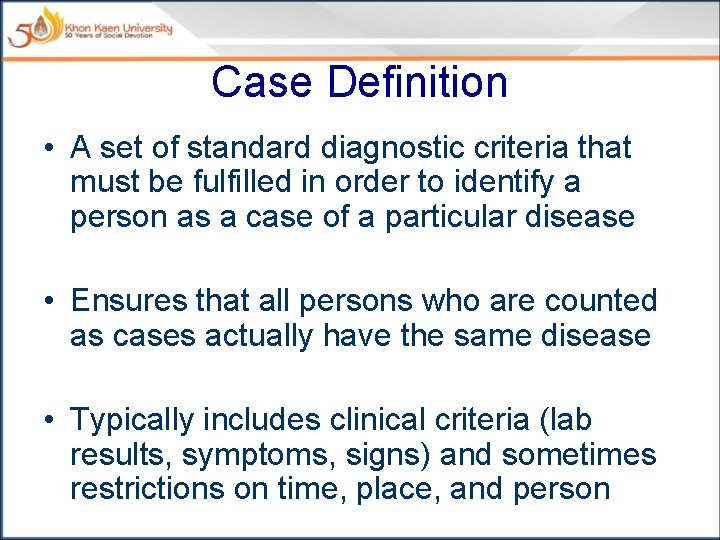
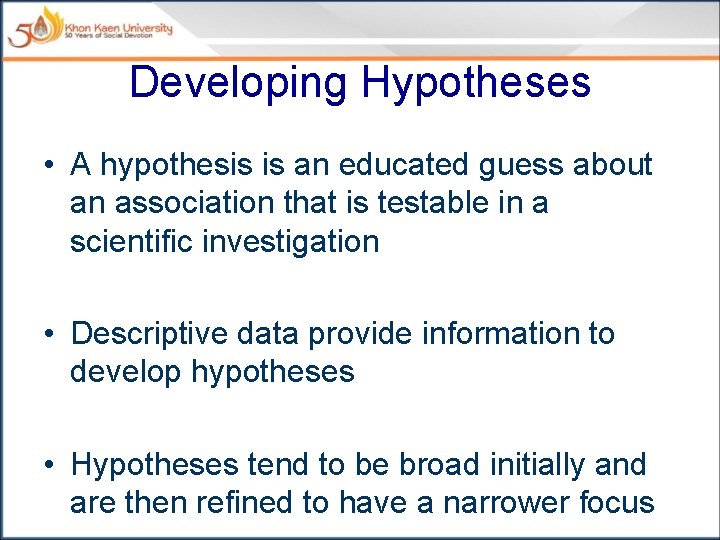
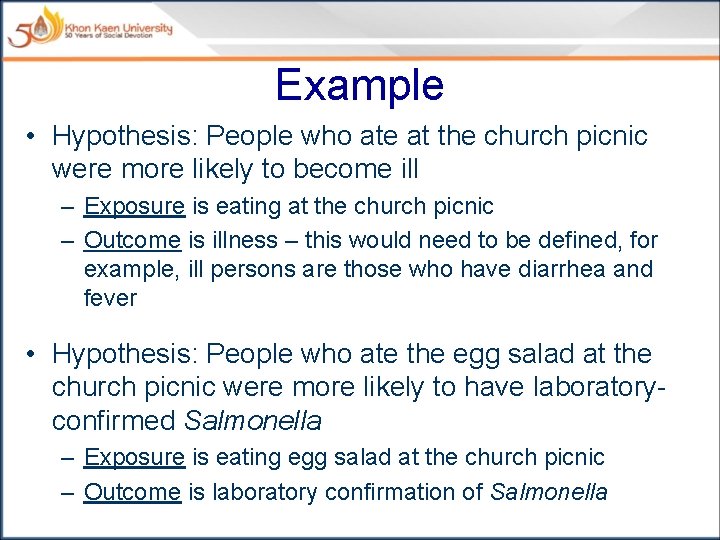
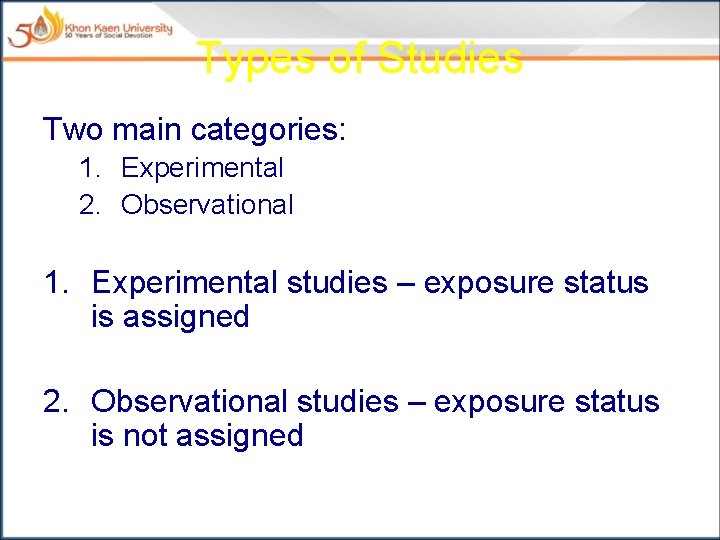
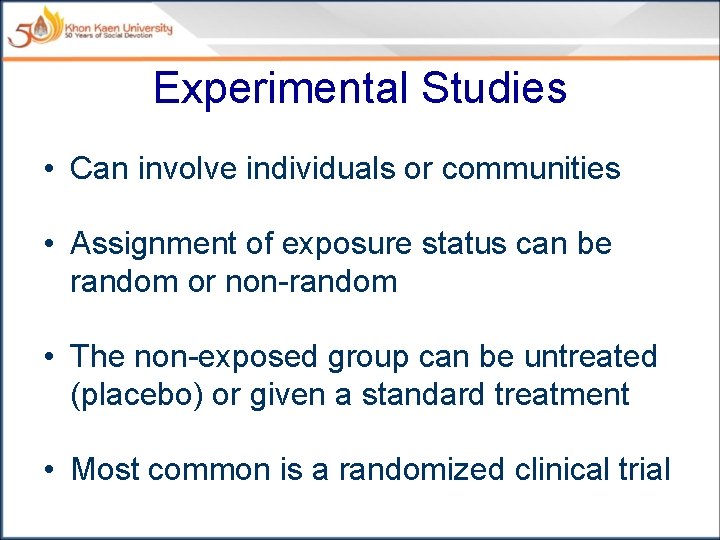
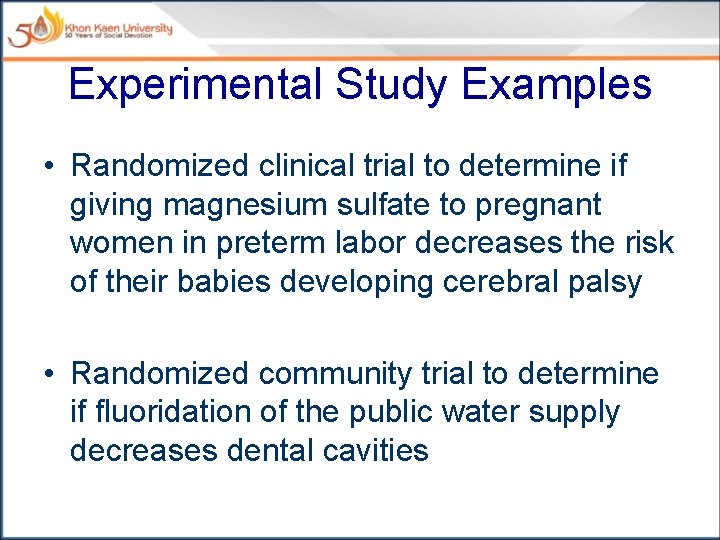
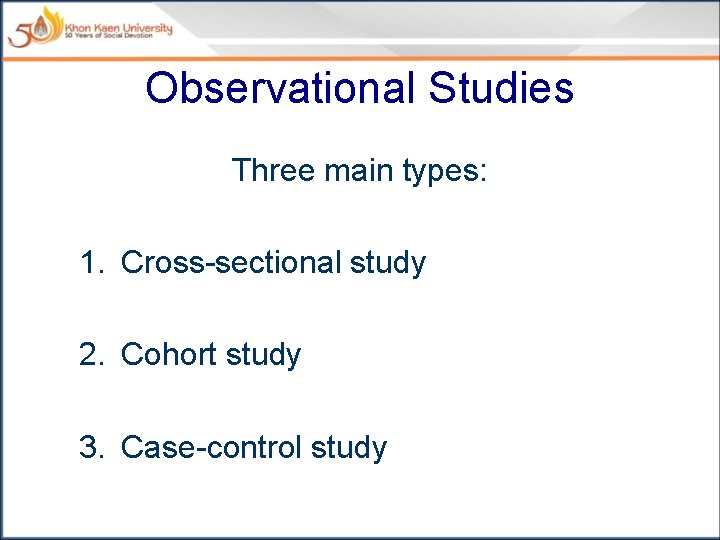
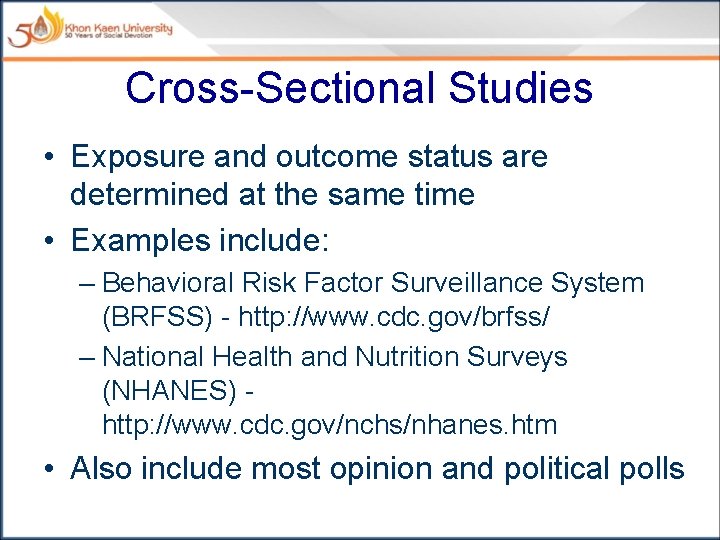
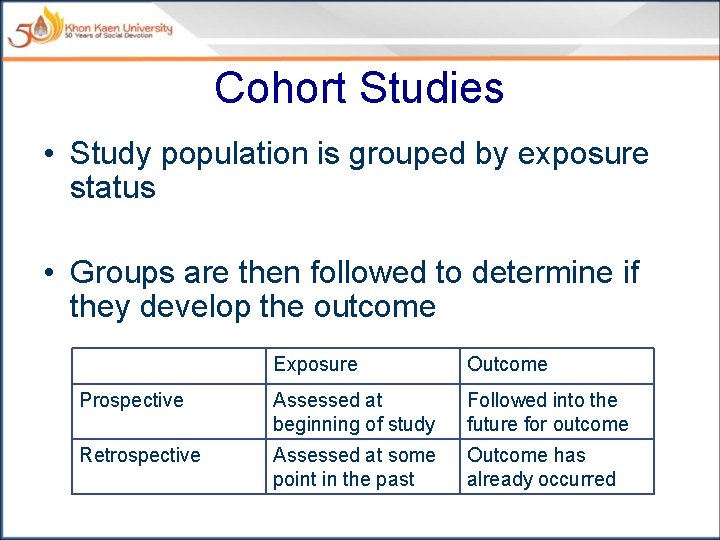
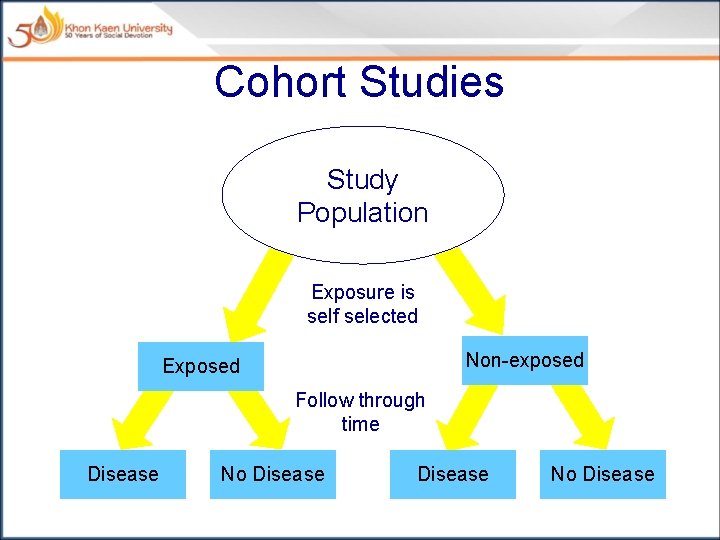
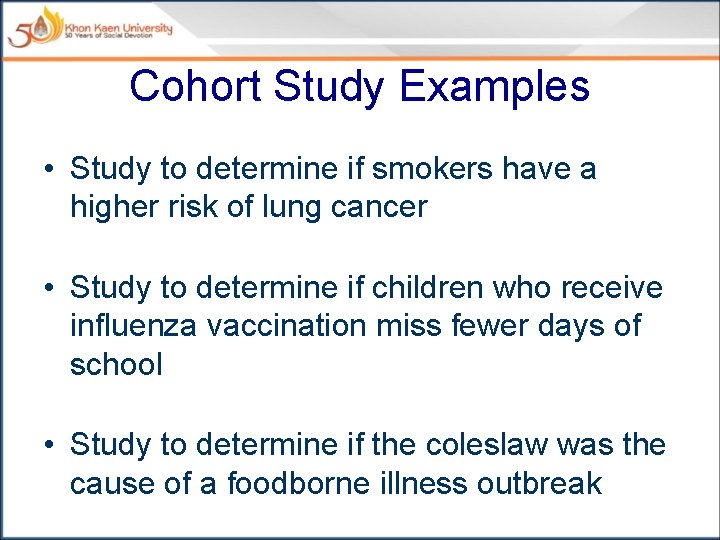
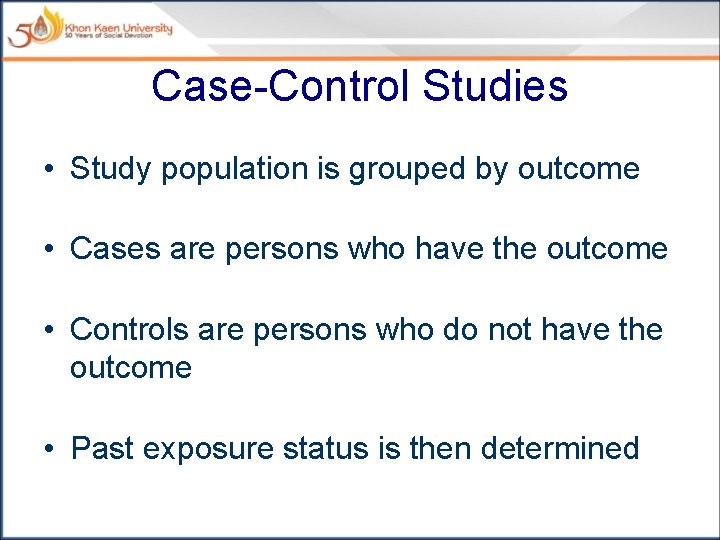
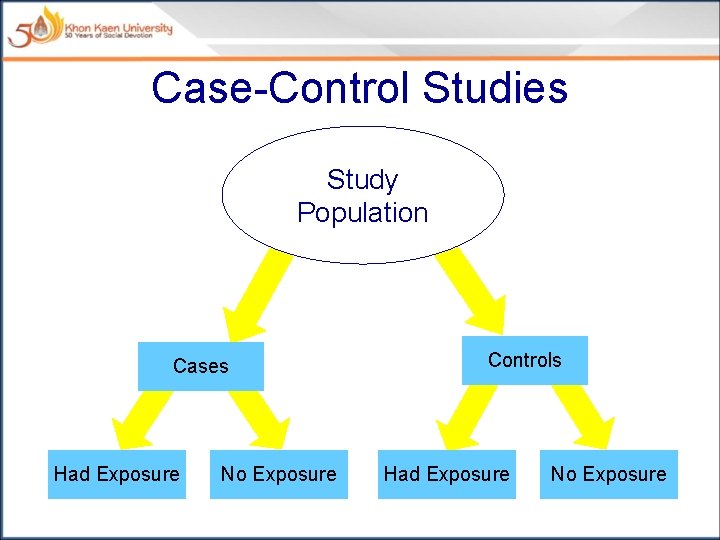
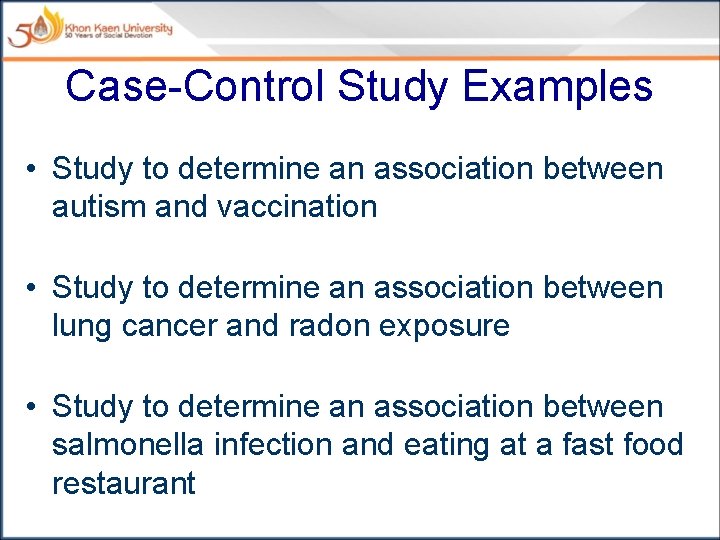
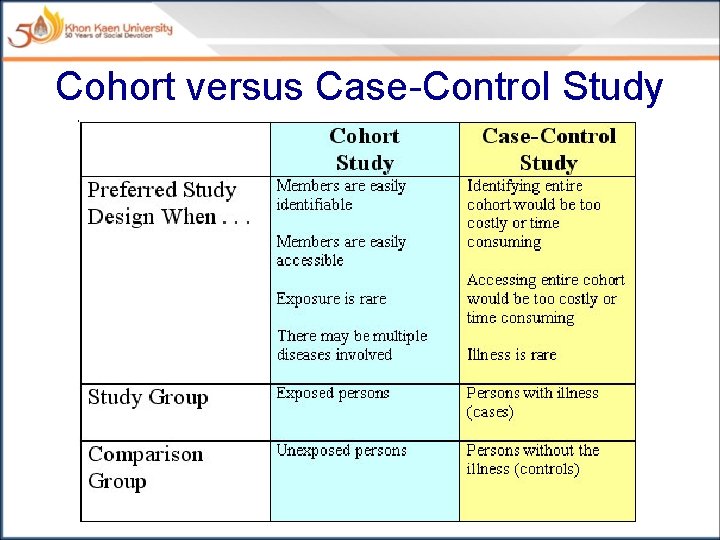
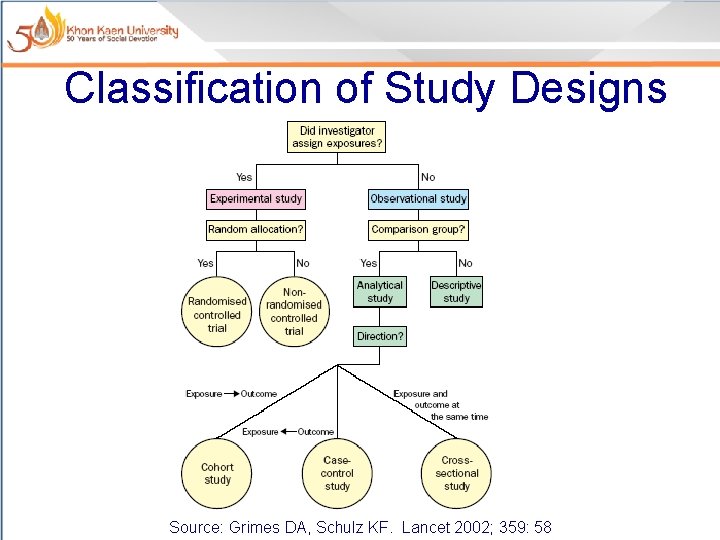
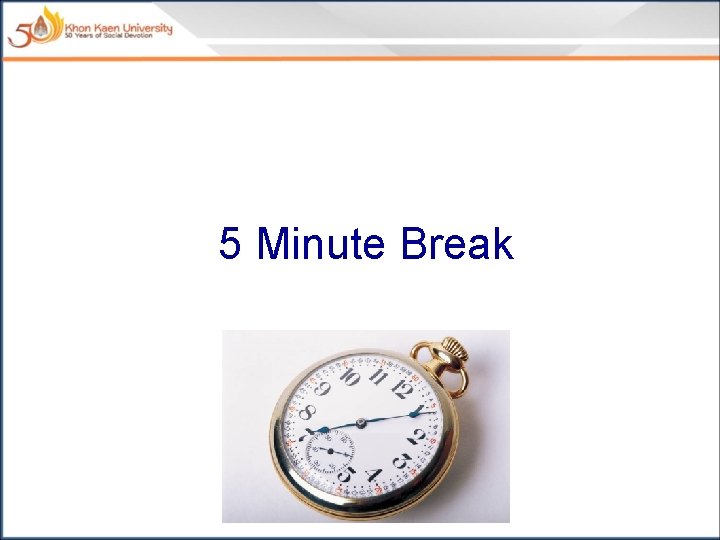
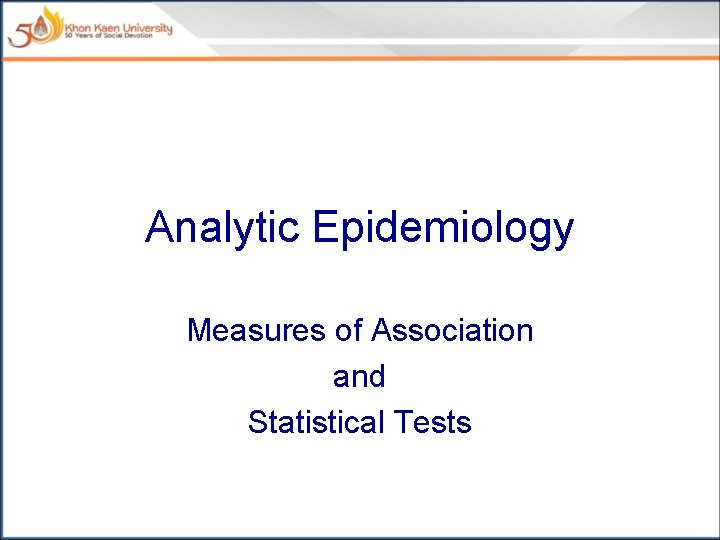
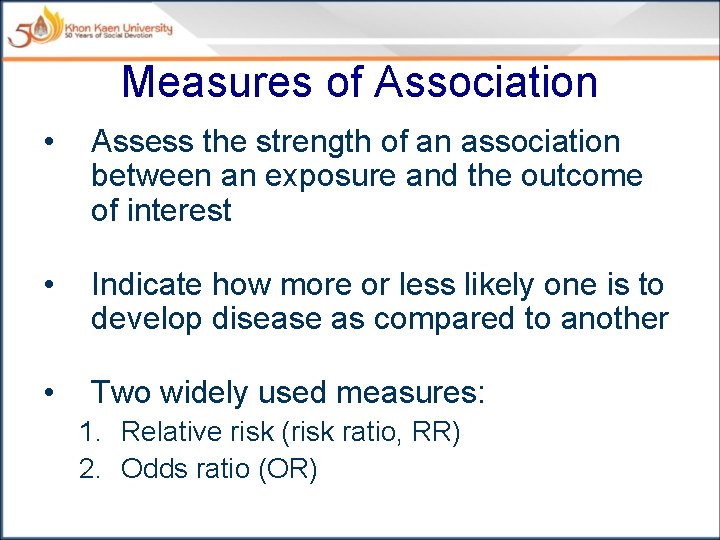
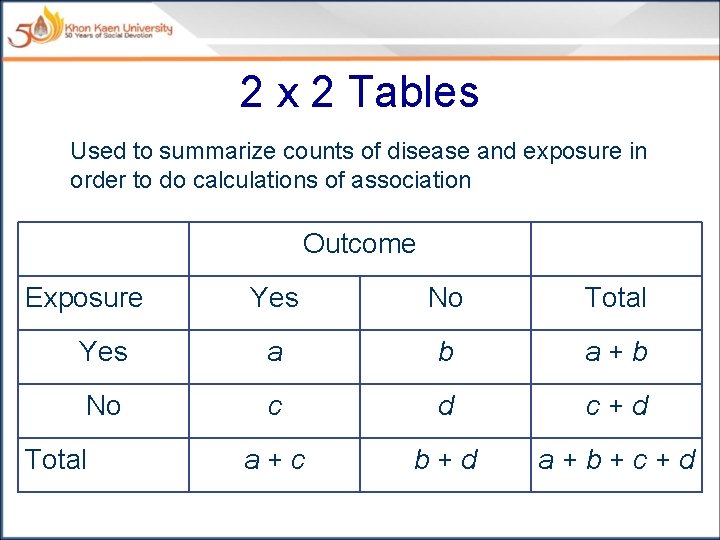
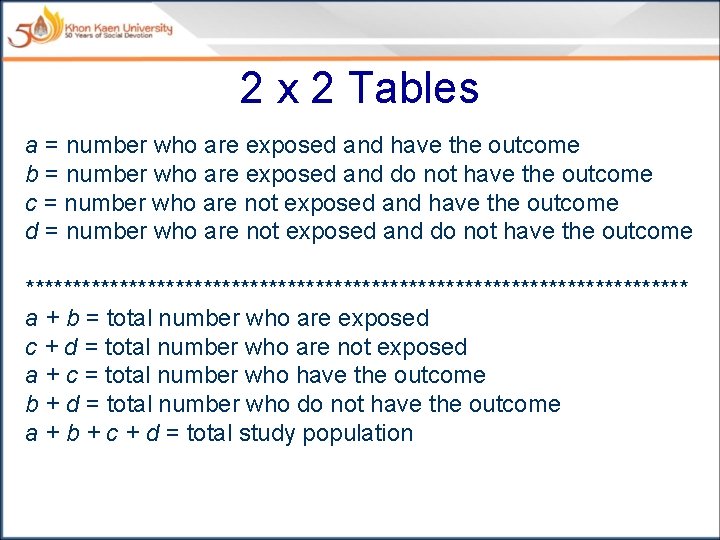
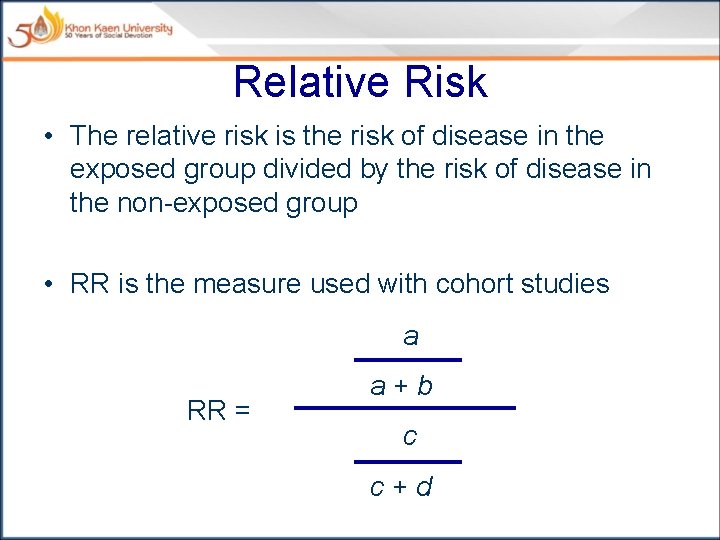
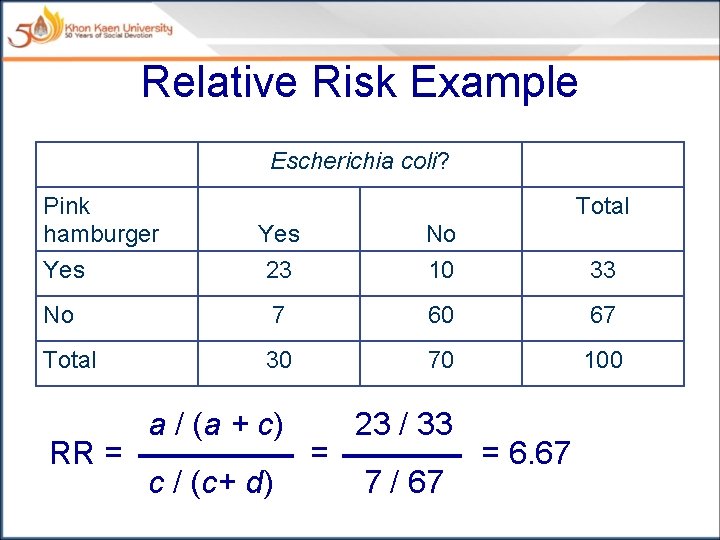
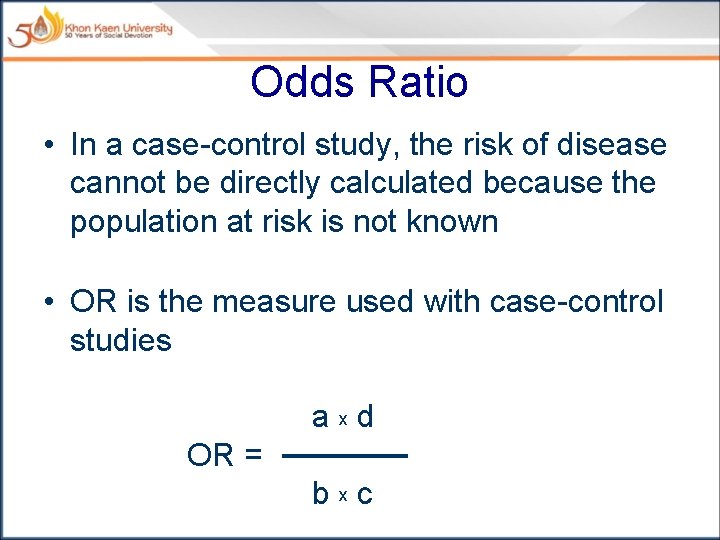
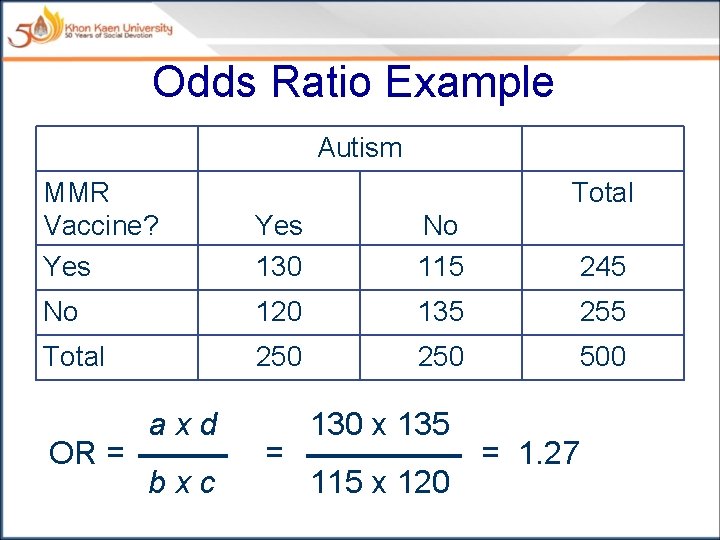
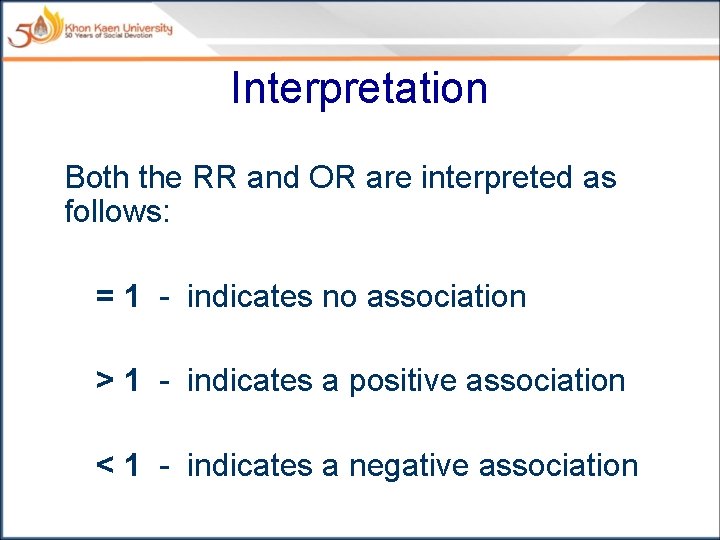
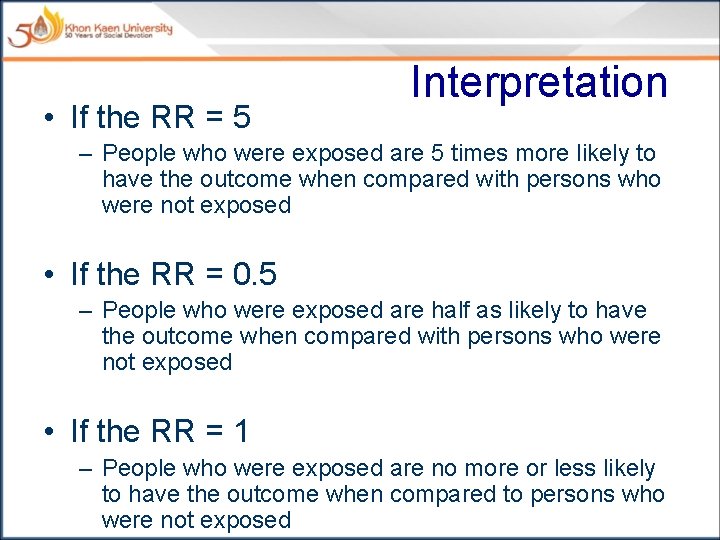
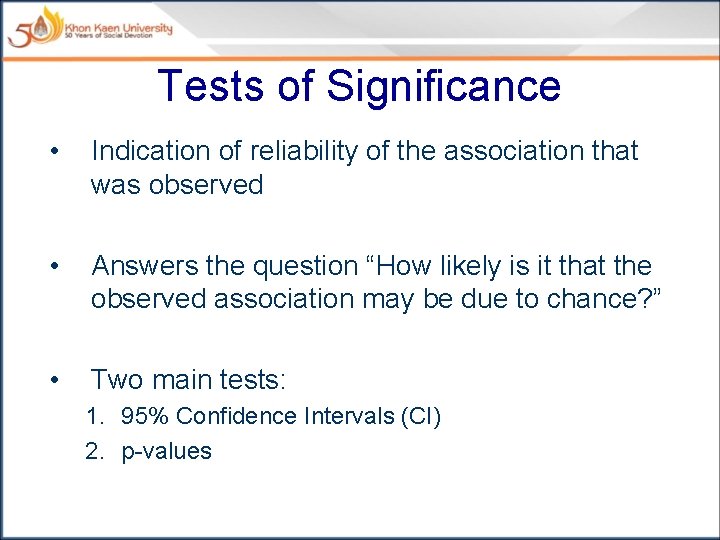
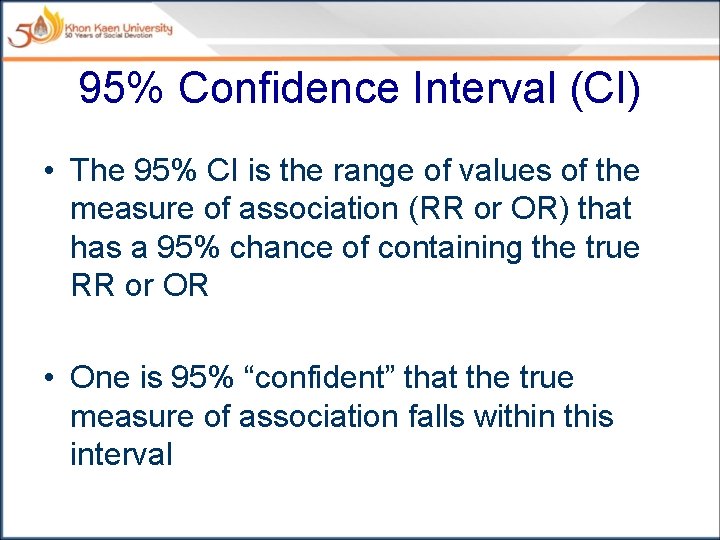
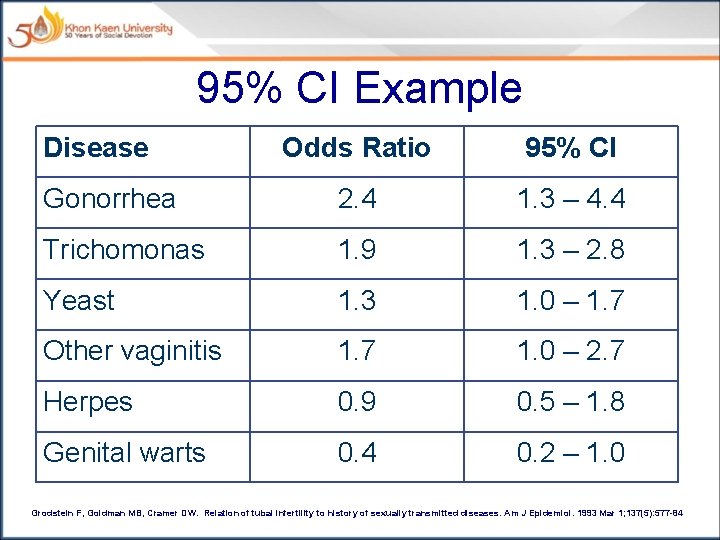
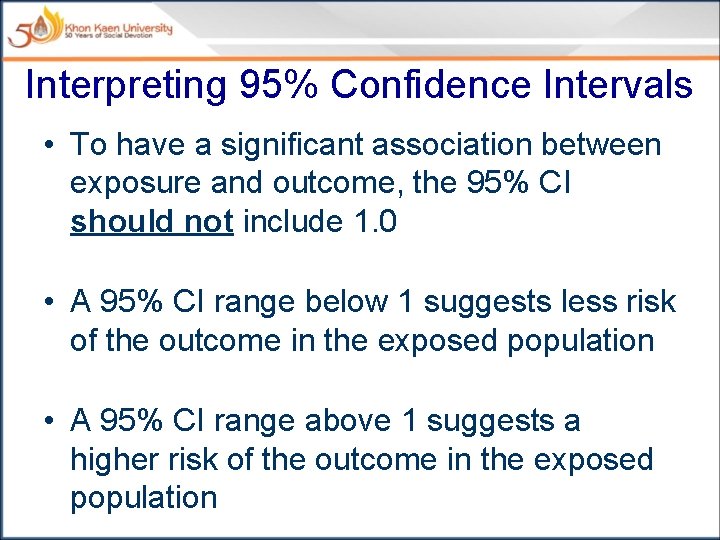
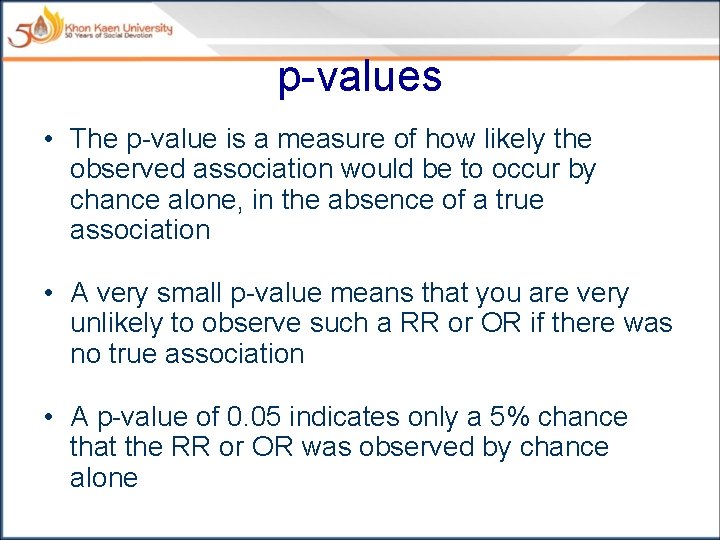
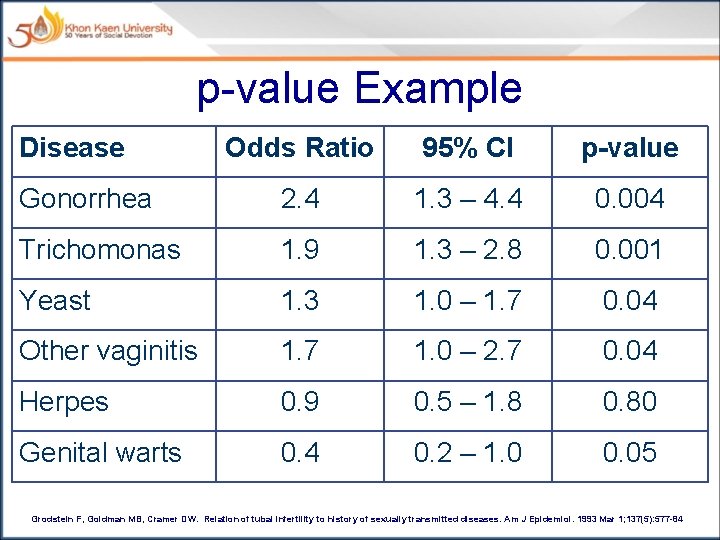
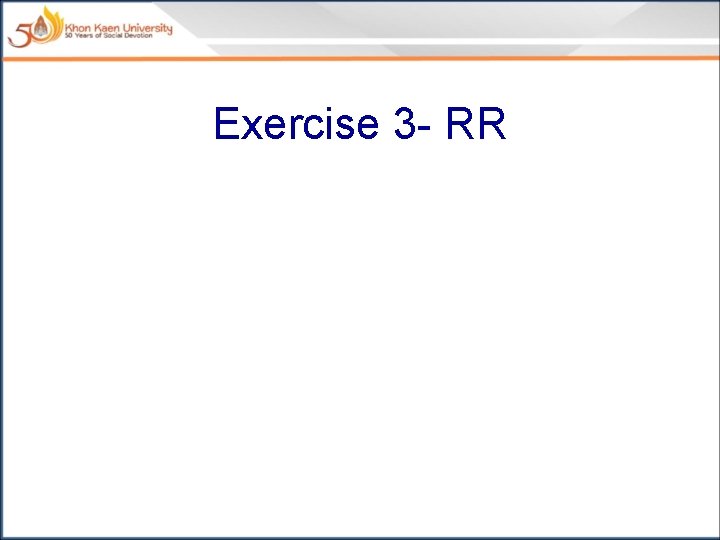
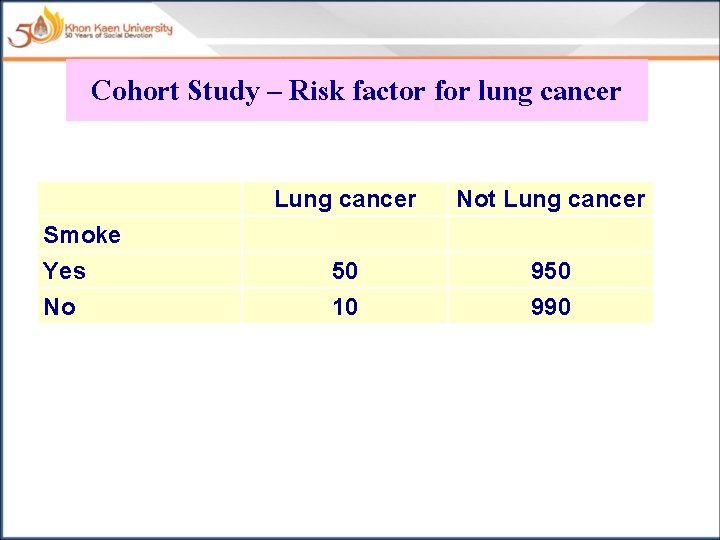
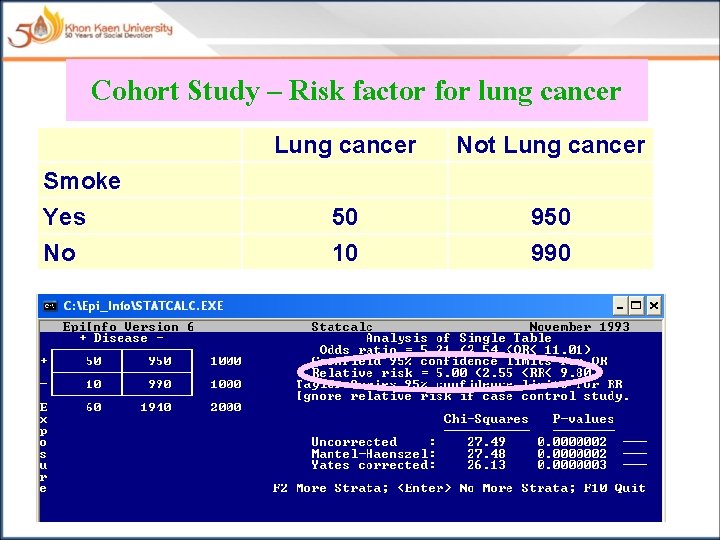
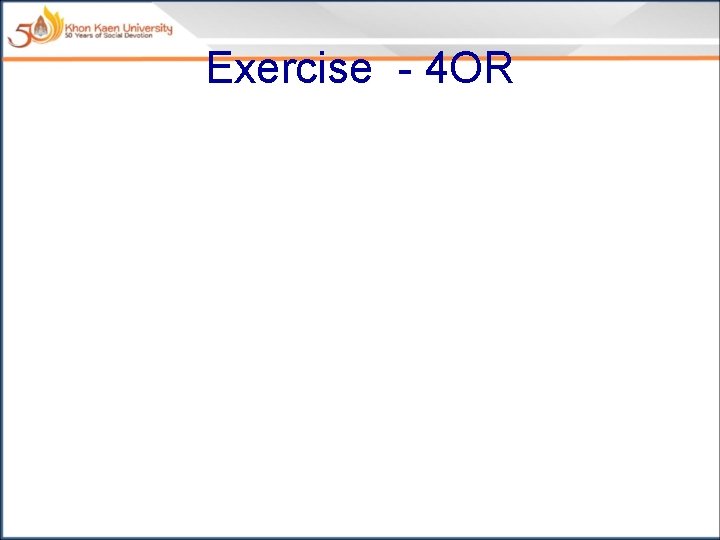
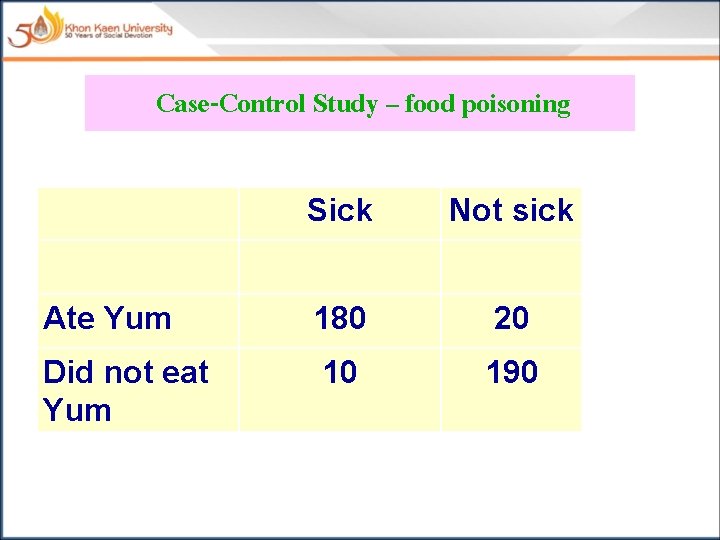
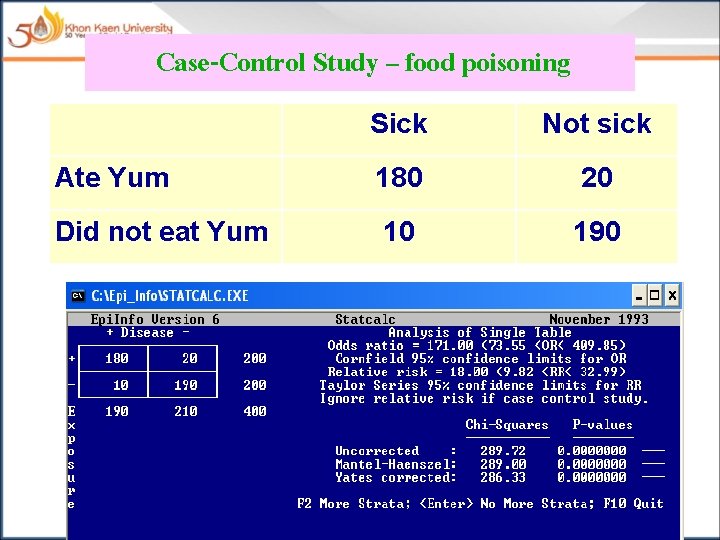
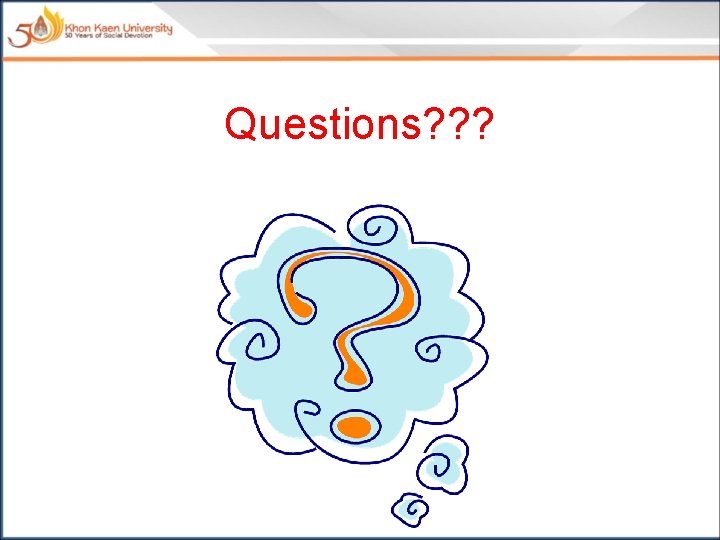
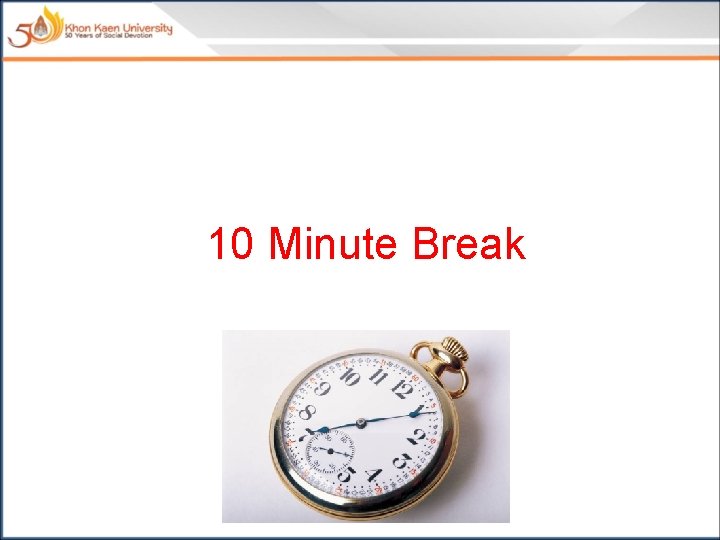
- Slides: 69
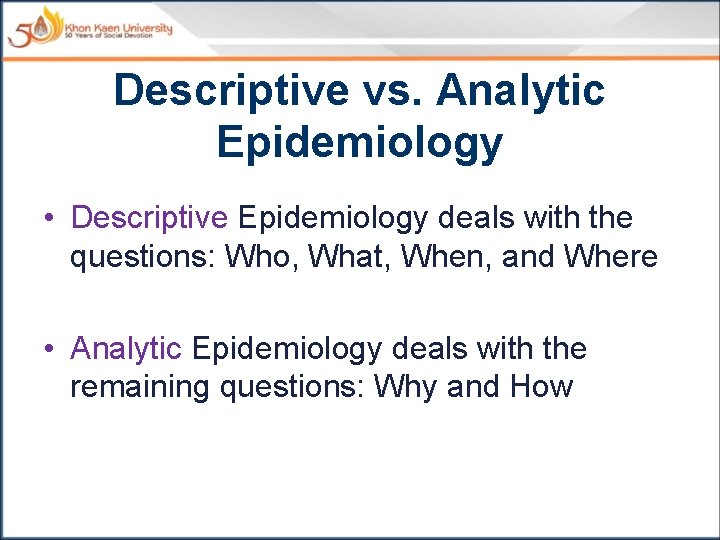
Descriptive vs. Analytic Epidemiology • Descriptive Epidemiology deals with the questions: Who, What, When, and Where • Analytic Epidemiology deals with the remaining questions: Why and How
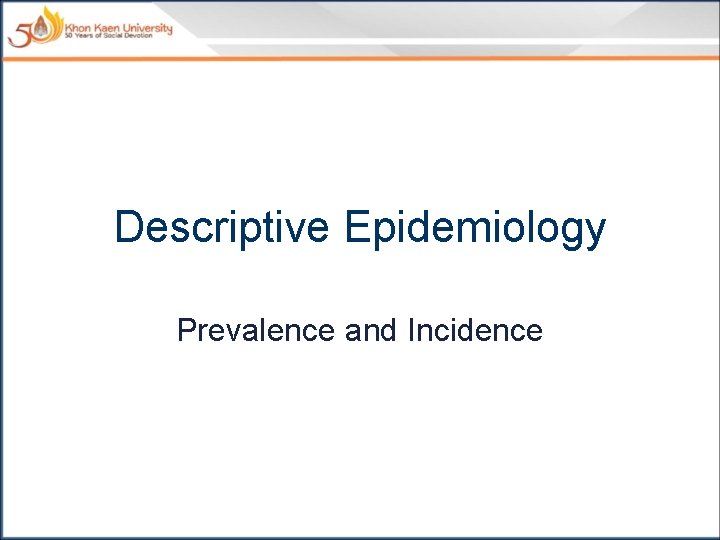
Descriptive Epidemiology Prevalence and Incidence
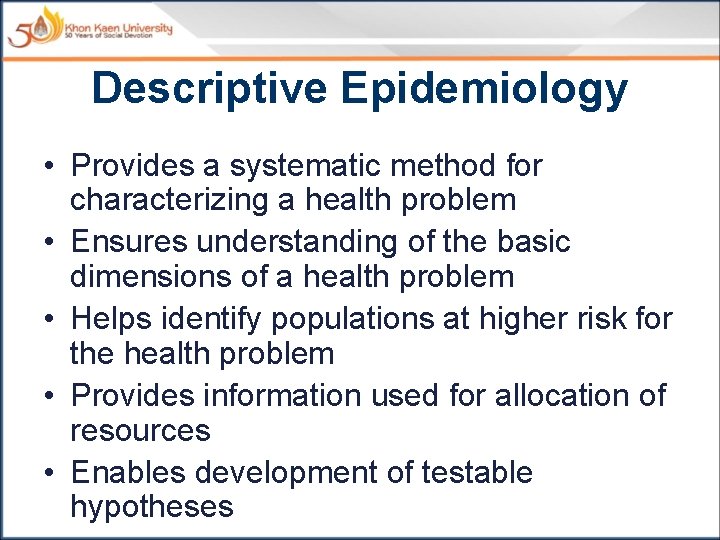
Descriptive Epidemiology • Provides a systematic method for characterizing a health problem • Ensures understanding of the basic dimensions of a health problem • Helps identify populations at higher risk for the health problem • Provides information used for allocation of resources • Enables development of testable hypotheses
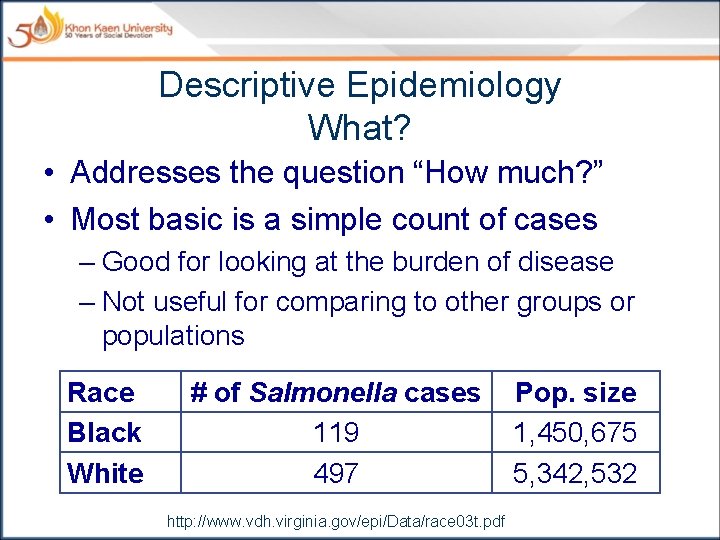
Descriptive Epidemiology What? • Addresses the question “How much? ” • Most basic is a simple count of cases – Good for looking at the burden of disease – Not useful for comparing to other groups or populations Race Black White # of Salmonella cases 119 497 http: //www. vdh. virginia. gov/epi/Data/race 03 t. pdf Pop. size 1, 450, 675 5, 342, 532
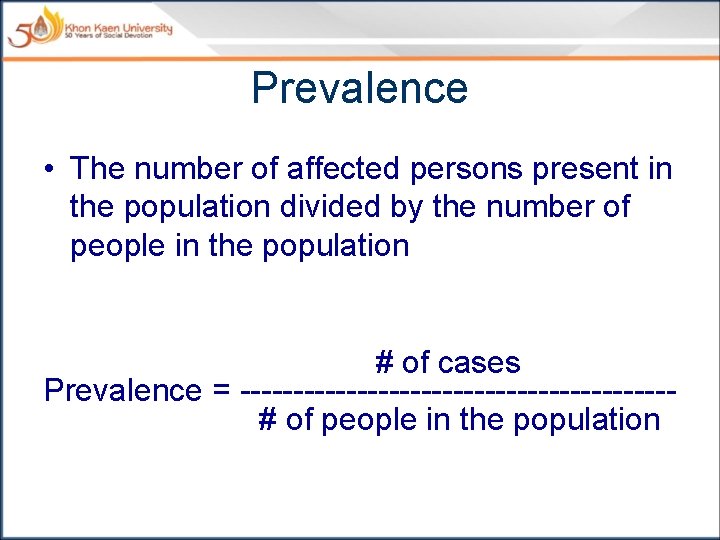
Prevalence • The number of affected persons present in the population divided by the number of people in the population # of cases Prevalence = --------------------# of people in the population
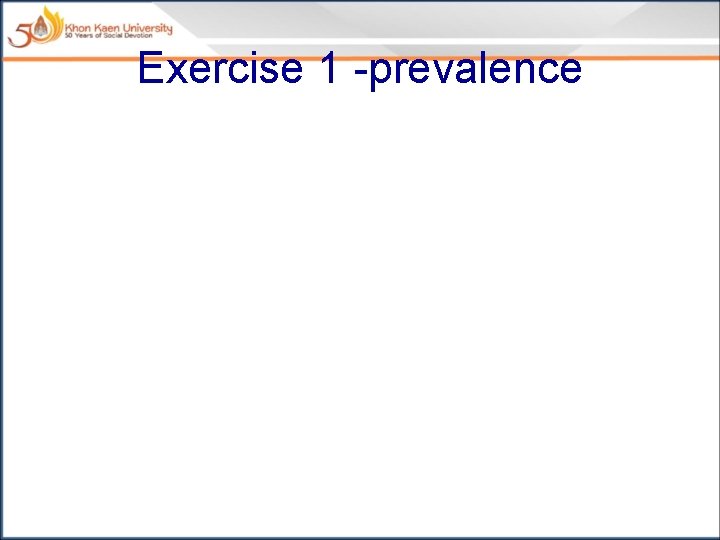
Exercise 1 -prevalence
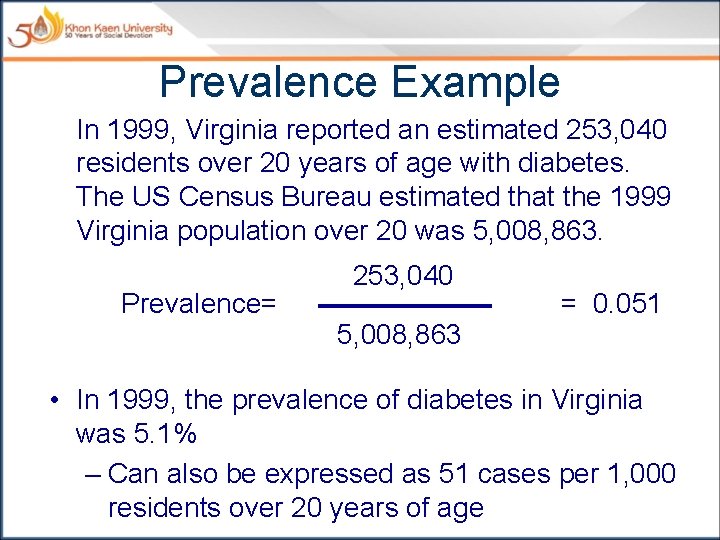
Prevalence Example In 1999, Virginia reported an estimated 253, 040 residents over 20 years of age with diabetes. The US Census Bureau estimated that the 1999 Virginia population over 20 was 5, 008, 863. Prevalence= 253, 040 = 0. 051 5, 008, 863 • In 1999, the prevalence of diabetes in Virginia was 5. 1% – Can also be expressed as 51 cases per 1, 000 residents over 20 years of age
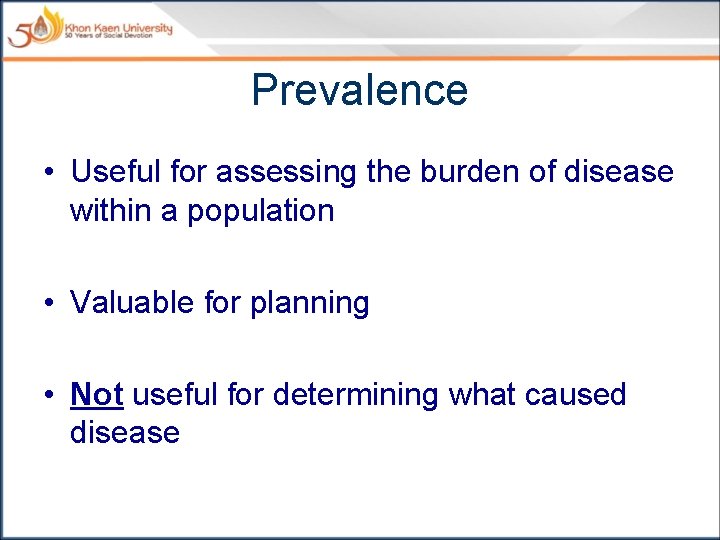
Prevalence • Useful for assessing the burden of disease within a population • Valuable for planning • Not useful for determining what caused disease
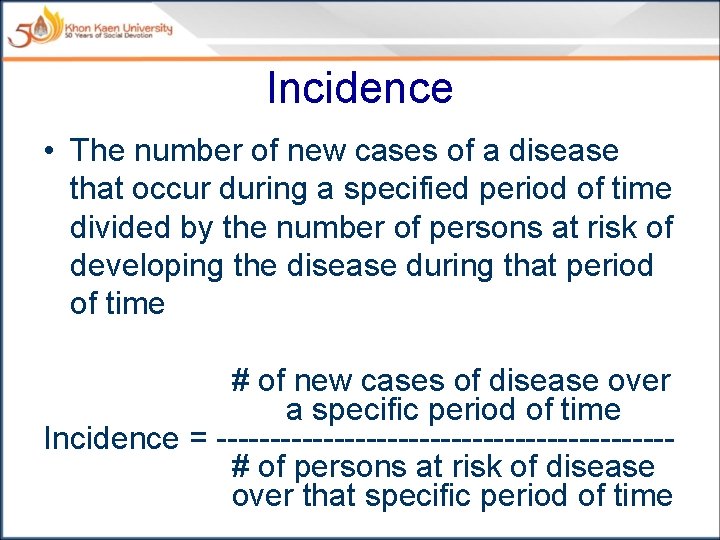
Incidence • The number of new cases of a disease that occur during a specified period of time divided by the number of persons at risk of developing the disease during that period of time # of new cases of disease over a specific period of time Incidence = ---------------------# of persons at risk of disease over that specific period of time
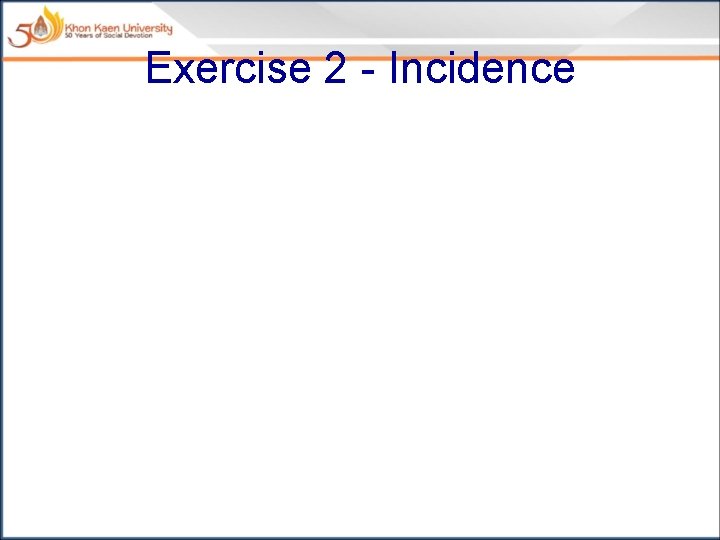
Exercise 2 - Incidence
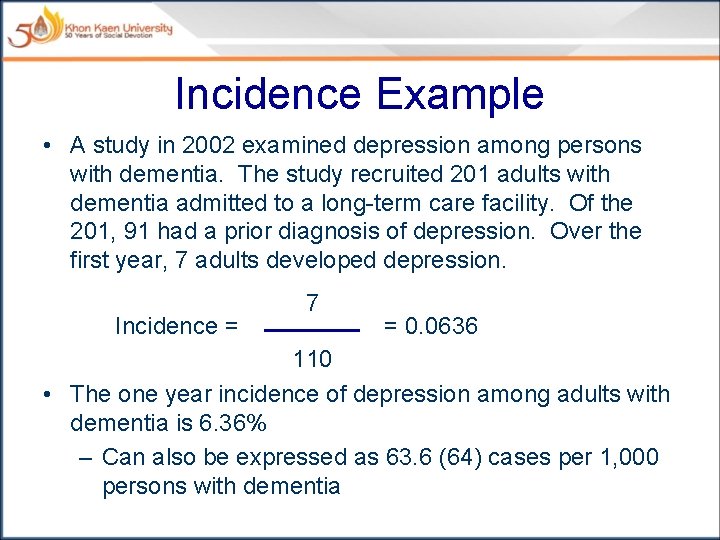
Incidence Example • A study in 2002 examined depression among persons with dementia. The study recruited 201 adults with dementia admitted to a long-term care facility. Of the 201, 91 had a prior diagnosis of depression. Over the first year, 7 adults developed depression. Incidence = 7 = 0. 0636 110 • The one year incidence of depression among adults with dementia is 6. 36% – Can also be expressed as 63. 6 (64) cases per 1, 000 persons with dementia
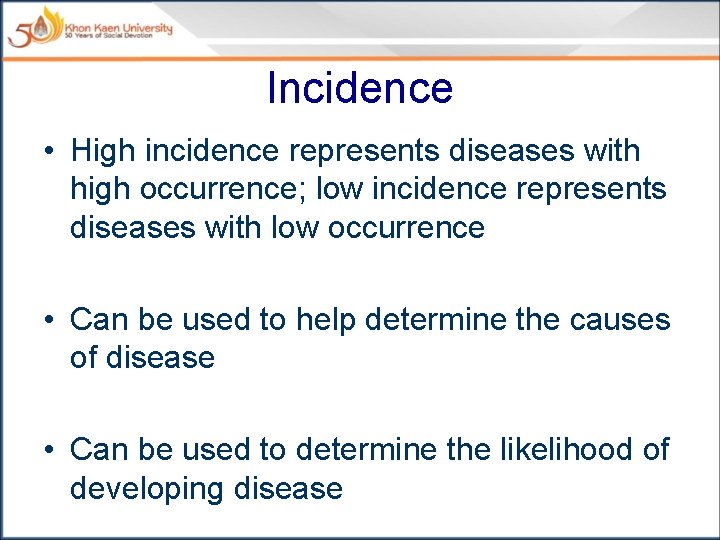
Incidence • High incidence represents diseases with high occurrence; low incidence represents diseases with low occurrence • Can be used to help determine the causes of disease • Can be used to determine the likelihood of developing disease
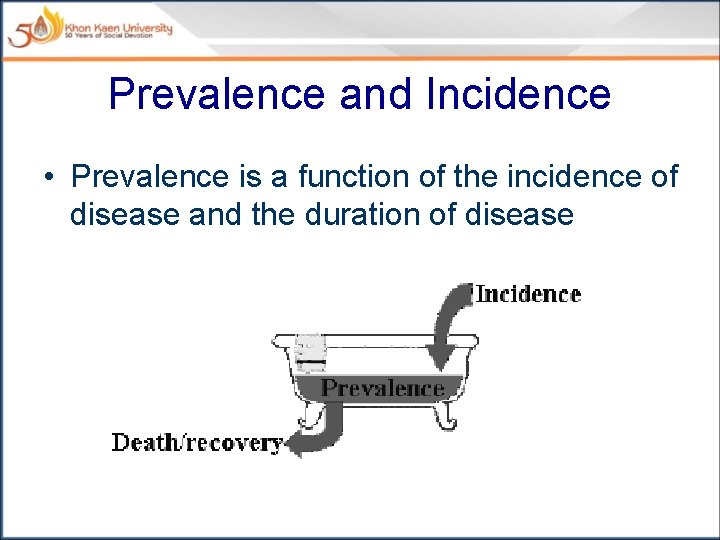
Prevalence and Incidence • Prevalence is a function of the incidence of disease and the duration of disease
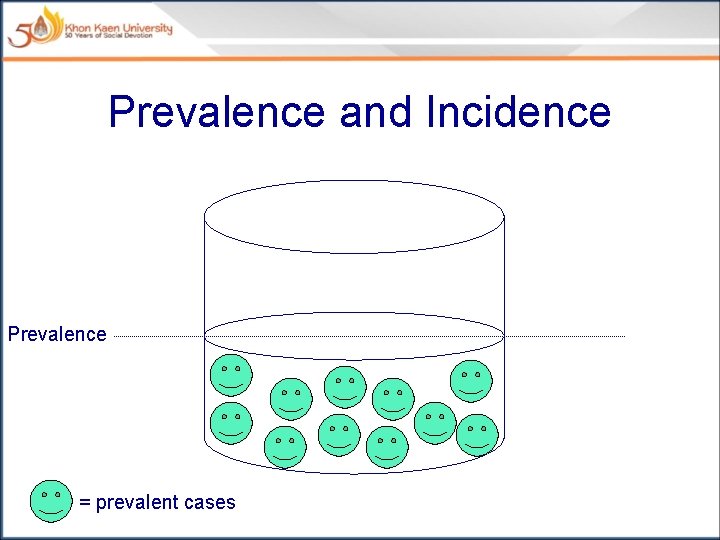
Prevalence and Incidence Prevalence = prevalent cases
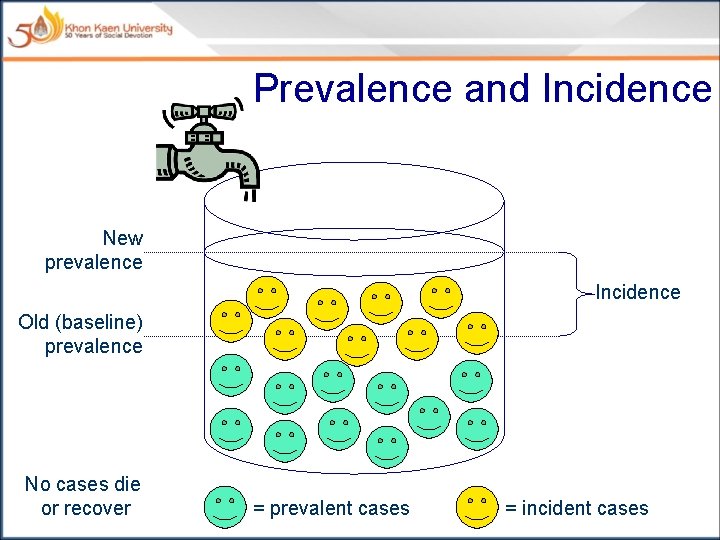
Prevalence and Incidence New prevalence Incidence Old (baseline) prevalence No cases die or recover = prevalent cases = incident cases
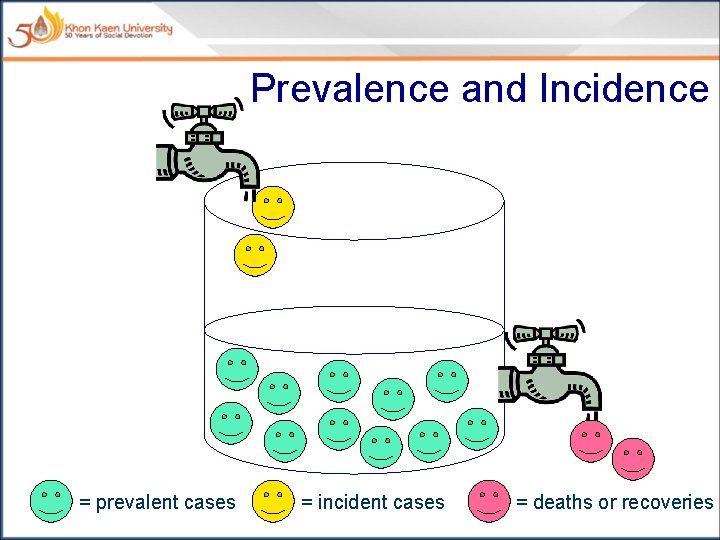
Prevalence and Incidence = prevalent cases = incident cases = deaths or recoveries
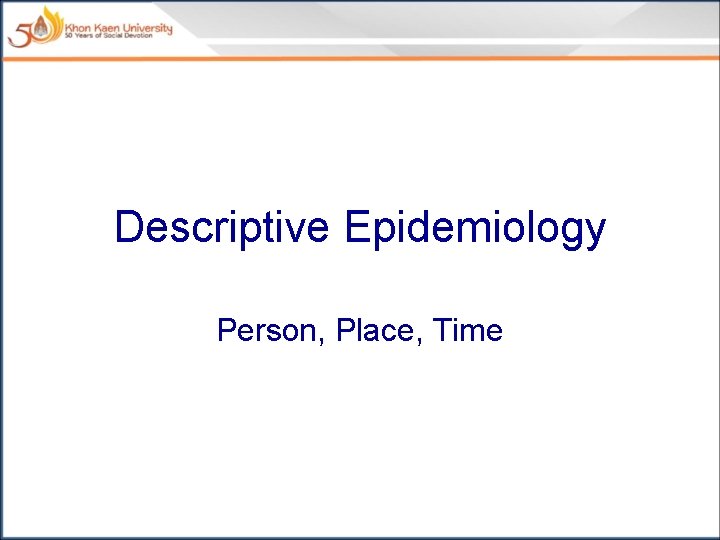
Descriptive Epidemiology Person, Place, Time
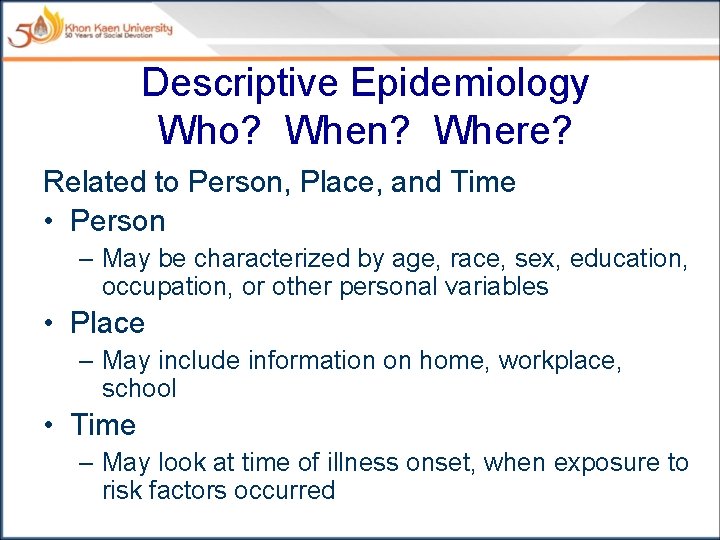
Descriptive Epidemiology Who? When? Where? Related to Person, Place, and Time • Person – May be characterized by age, race, sex, education, occupation, or other personal variables • Place – May include information on home, workplace, school • Time – May look at time of illness onset, when exposure to risk factors occurred
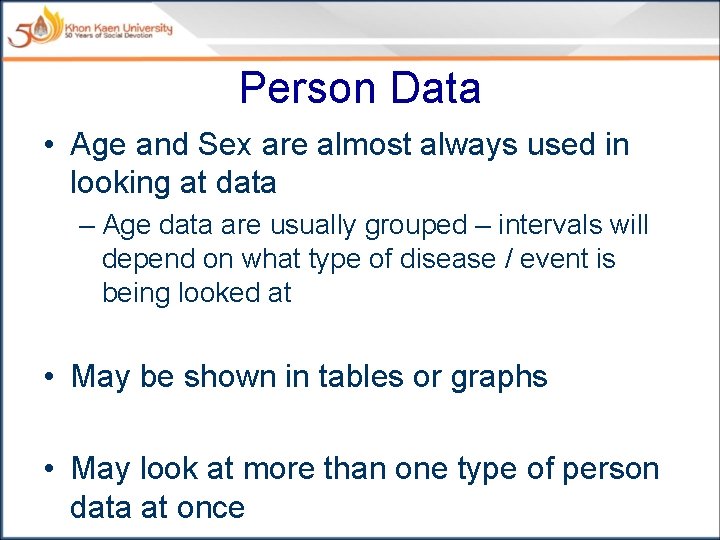
Person Data • Age and Sex are almost always used in looking at data – Age data are usually grouped – intervals will depend on what type of disease / event is being looked at • May be shown in tables or graphs • May look at more than one type of person data at once
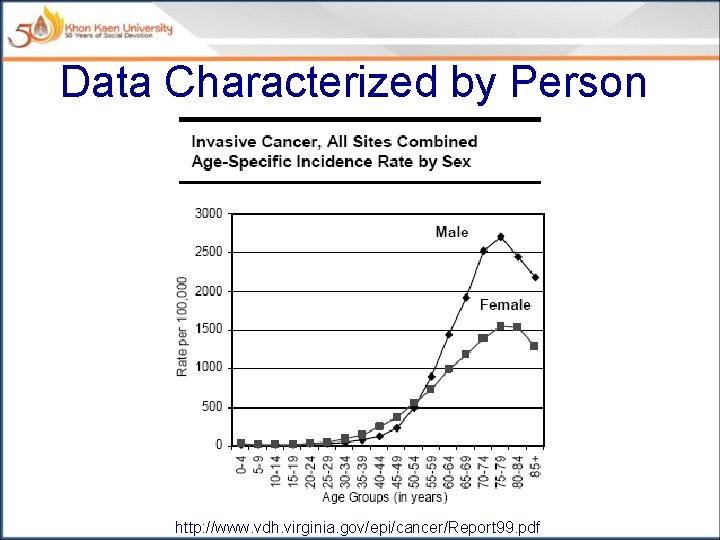
Data Characterized by Person http: //www. vdh. virginia. gov/epi/cancer/Report 99. pdf
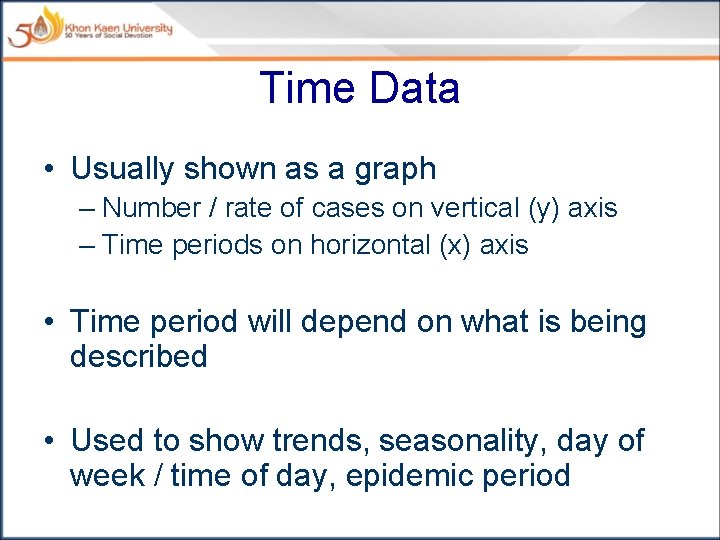
Time Data • Usually shown as a graph – Number / rate of cases on vertical (y) axis – Time periods on horizontal (x) axis • Time period will depend on what is being described • Used to show trends, seasonality, day of week / time of day, epidemic period
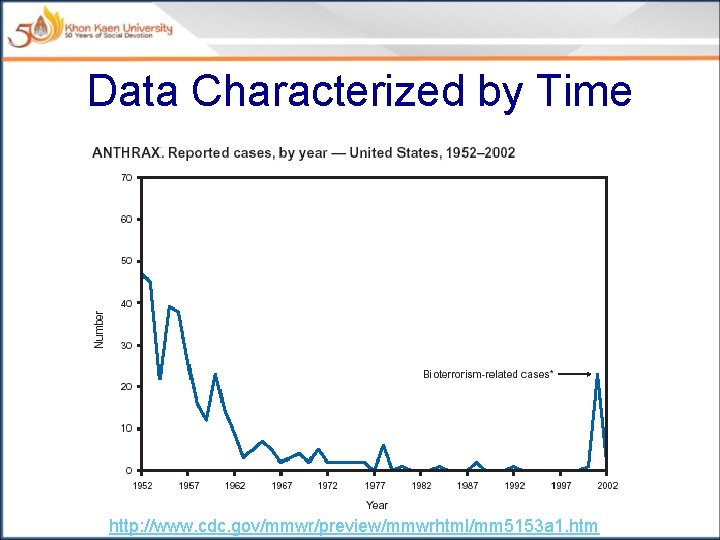
Data Characterized by Time http: //www. cdc. gov/mmwr/preview/mmwrhtml/mm 5153 a 1. htm
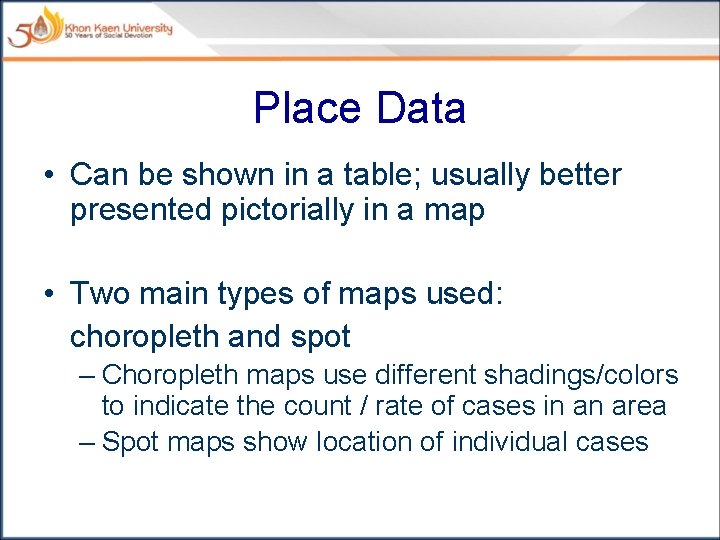
Place Data • Can be shown in a table; usually better presented pictorially in a map • Two main types of maps used: choropleth and spot – Choropleth maps use different shadings/colors to indicate the count / rate of cases in an area – Spot maps show location of individual cases
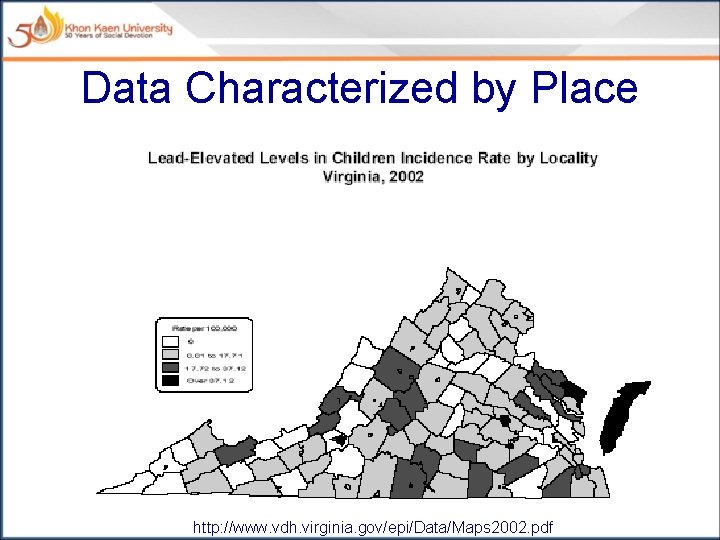
Data Characterized by Place http: //www. vdh. virginia. gov/epi/Data/Maps 2002. pdf
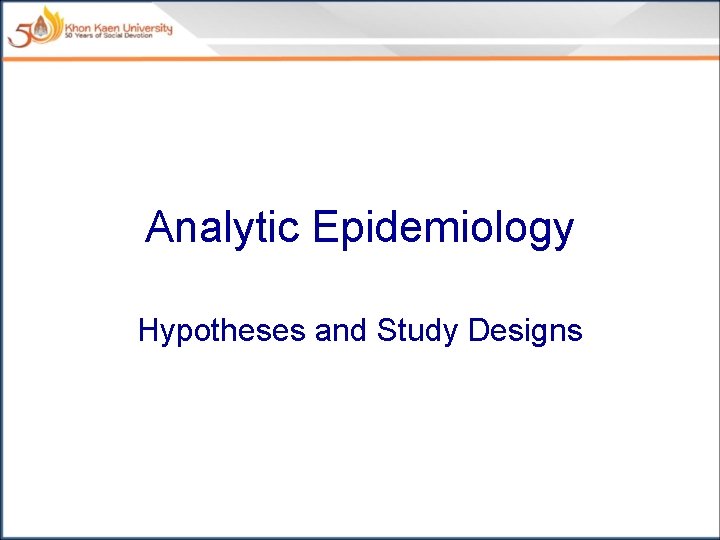
Analytic Epidemiology Hypotheses and Study Designs
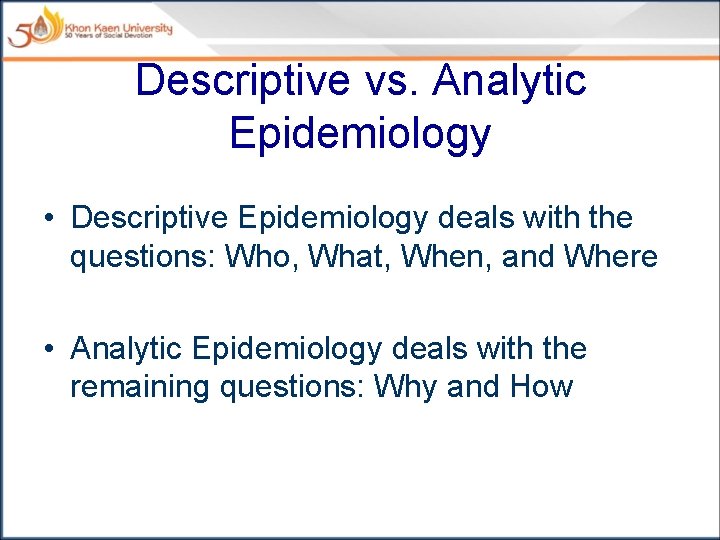
Descriptive vs. Analytic Epidemiology • Descriptive Epidemiology deals with the questions: Who, What, When, and Where • Analytic Epidemiology deals with the remaining questions: Why and How
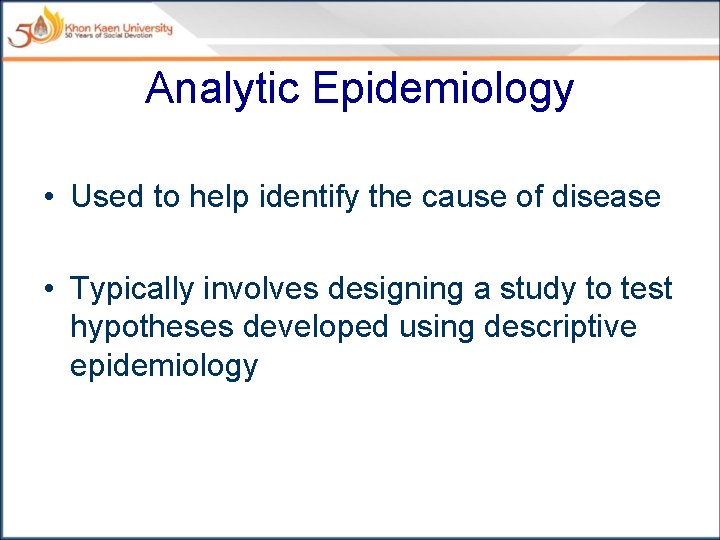
Analytic Epidemiology • Used to help identify the cause of disease • Typically involves designing a study to test hypotheses developed using descriptive epidemiology
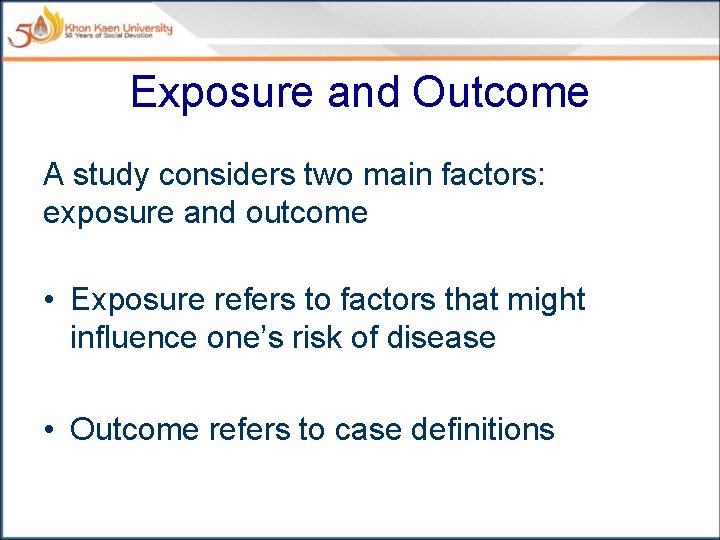
Exposure and Outcome A study considers two main factors: exposure and outcome • Exposure refers to factors that might influence one’s risk of disease • Outcome refers to case definitions
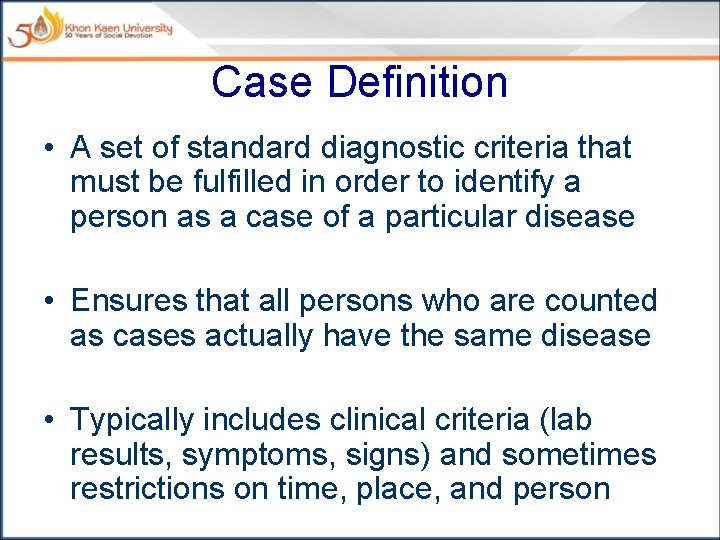
Case Definition • A set of standard diagnostic criteria that must be fulfilled in order to identify a person as a case of a particular disease • Ensures that all persons who are counted as cases actually have the same disease • Typically includes clinical criteria (lab results, symptoms, signs) and sometimes restrictions on time, place, and person
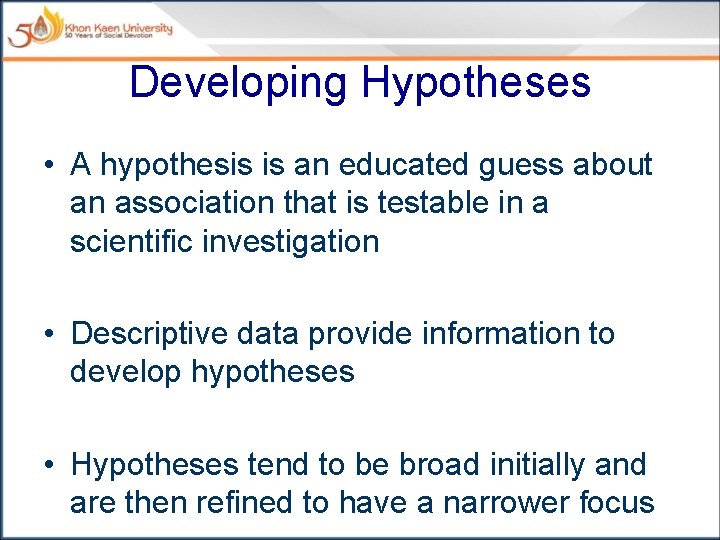
Developing Hypotheses • A hypothesis is an educated guess about an association that is testable in a scientific investigation • Descriptive data provide information to develop hypotheses • Hypotheses tend to be broad initially and are then refined to have a narrower focus
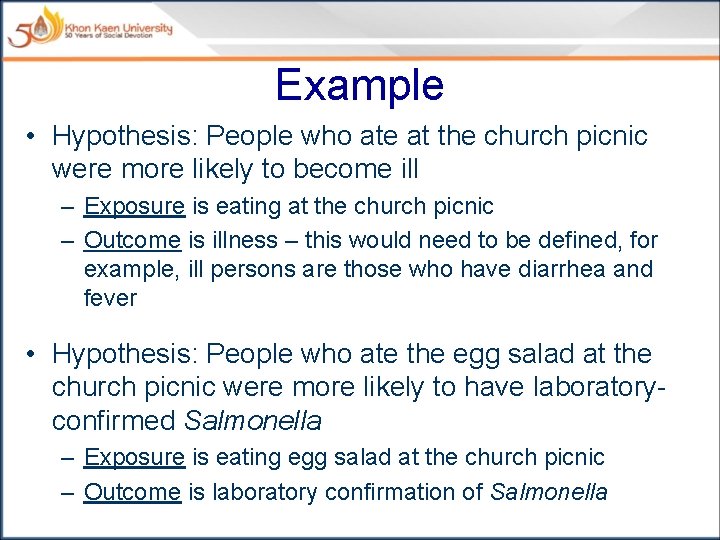
Example • Hypothesis: People who ate at the church picnic were more likely to become ill – Exposure is eating at the church picnic – Outcome is illness – this would need to be defined, for example, ill persons are those who have diarrhea and fever • Hypothesis: People who ate the egg salad at the church picnic were more likely to have laboratoryconfirmed Salmonella – Exposure is eating egg salad at the church picnic – Outcome is laboratory confirmation of Salmonella
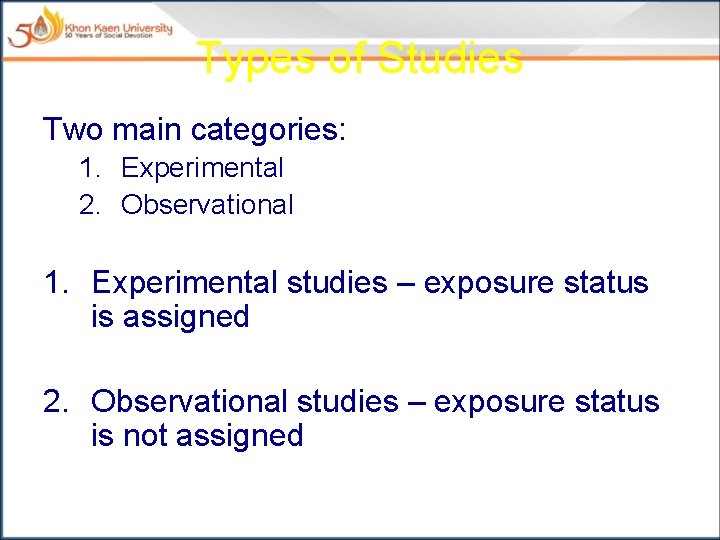
Types of Studies Two main categories: 1. Experimental 2. Observational 1. Experimental studies – exposure status is assigned 2. Observational studies – exposure status is not assigned
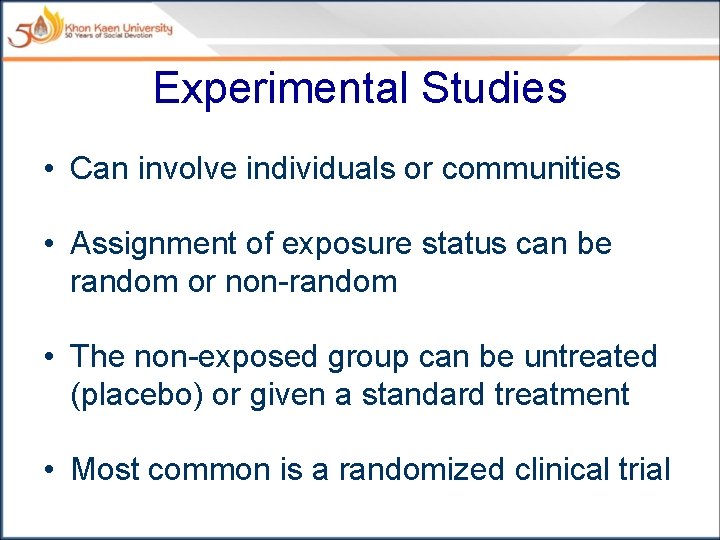
Experimental Studies • Can involve individuals or communities • Assignment of exposure status can be random or non-random • The non-exposed group can be untreated (placebo) or given a standard treatment • Most common is a randomized clinical trial
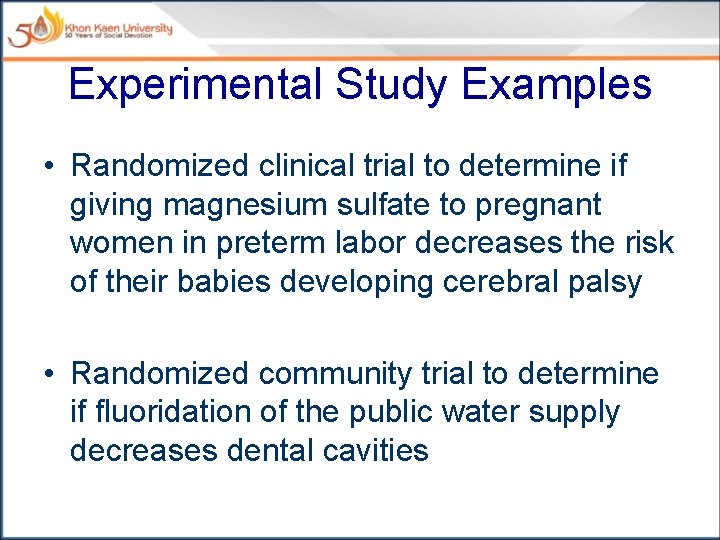
Experimental Study Examples • Randomized clinical trial to determine if giving magnesium sulfate to pregnant women in preterm labor decreases the risk of their babies developing cerebral palsy • Randomized community trial to determine if fluoridation of the public water supply decreases dental cavities
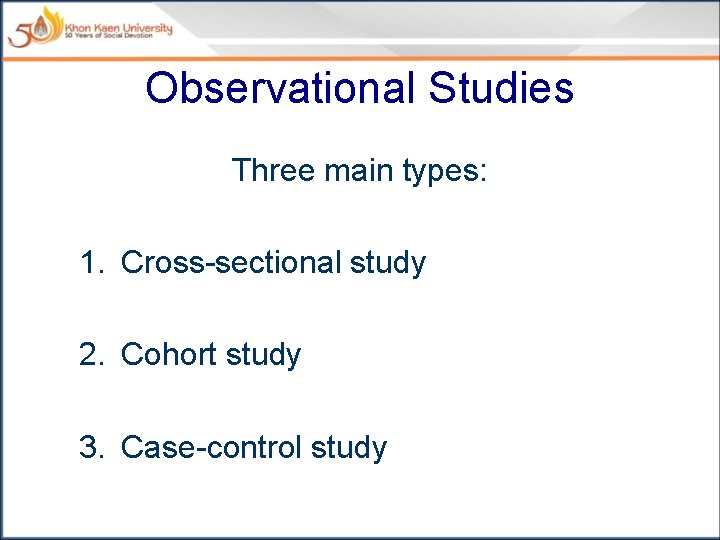
Observational Studies Three main types: 1. Cross-sectional study 2. Cohort study 3. Case-control study
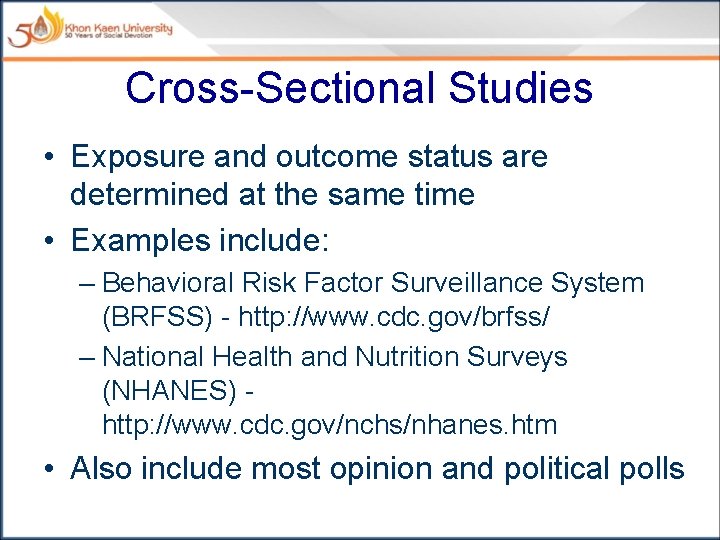
Cross-Sectional Studies • Exposure and outcome status are determined at the same time • Examples include: – Behavioral Risk Factor Surveillance System (BRFSS) - http: //www. cdc. gov/brfss/ – National Health and Nutrition Surveys (NHANES) http: //www. cdc. gov/nchs/nhanes. htm • Also include most opinion and political polls
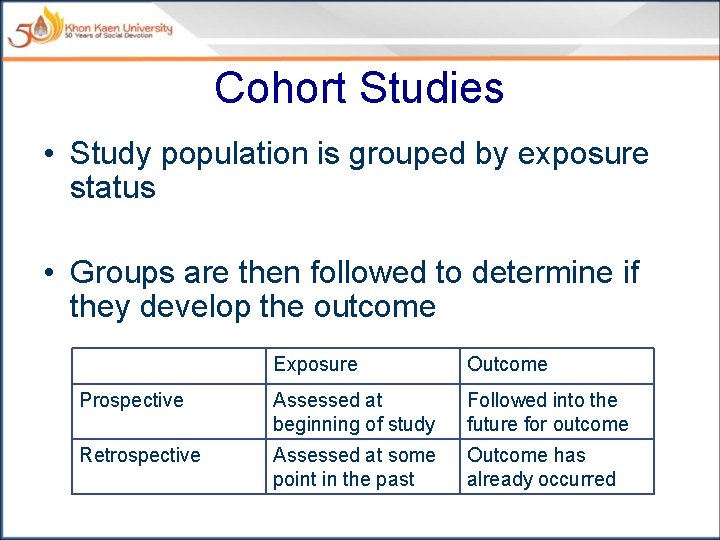
Cohort Studies • Study population is grouped by exposure status • Groups are then followed to determine if they develop the outcome Exposure Outcome Prospective Assessed at beginning of study Followed into the future for outcome Retrospective Assessed at some point in the past Outcome has already occurred
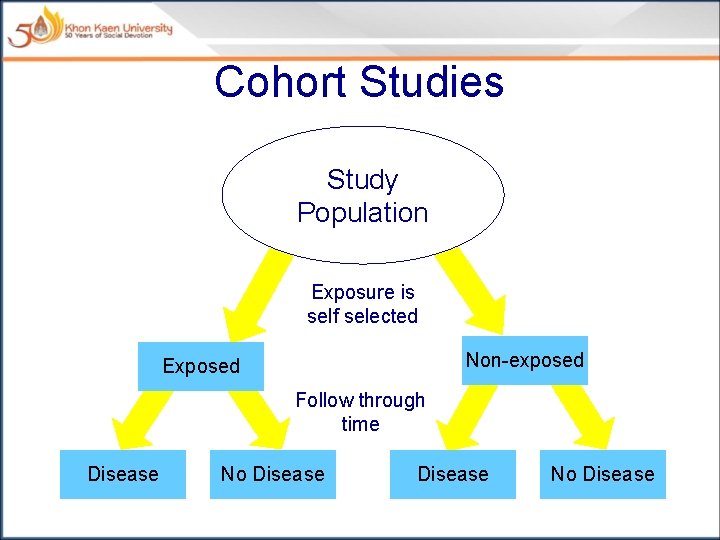
Cohort Studies Study Population Exposure is self selected Non-exposed Exposed Follow through time Disease No Disease
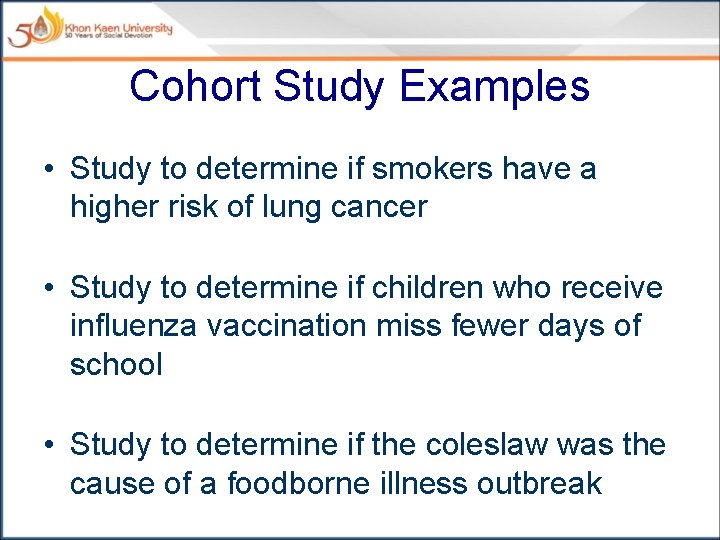
Cohort Study Examples • Study to determine if smokers have a higher risk of lung cancer • Study to determine if children who receive influenza vaccination miss fewer days of school • Study to determine if the coleslaw was the cause of a foodborne illness outbreak
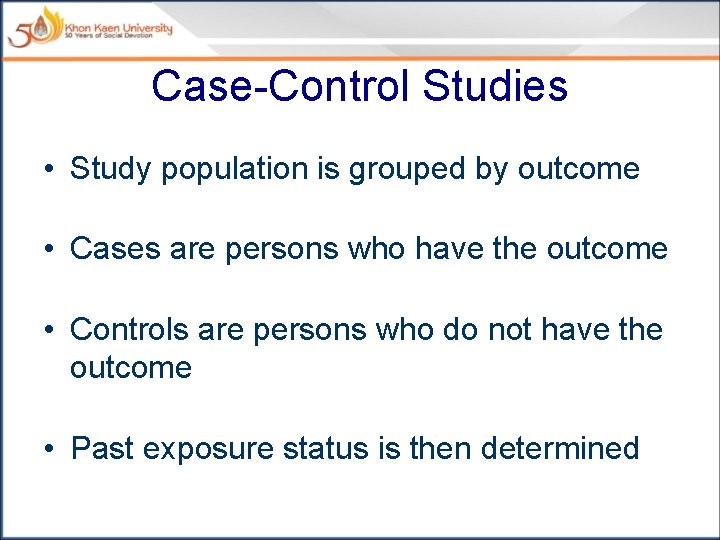
Case-Control Studies • Study population is grouped by outcome • Cases are persons who have the outcome • Controls are persons who do not have the outcome • Past exposure status is then determined
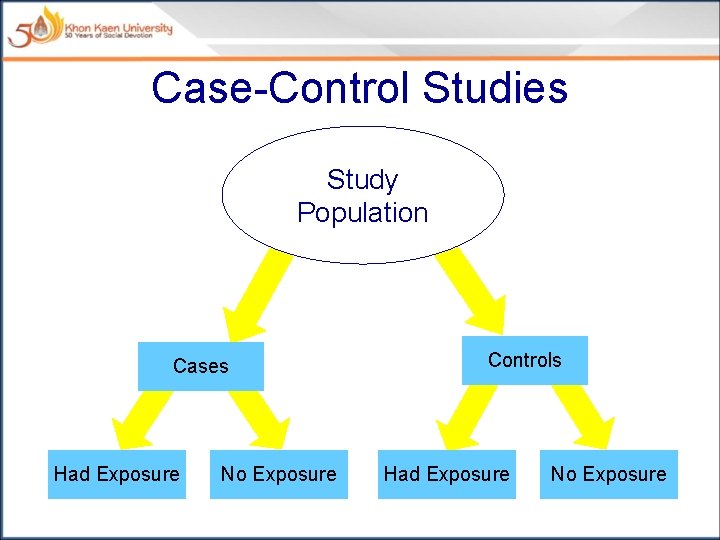
Case-Control Studies Study Population Cases Had Exposure No Exposure Controls Had Exposure No Exposure
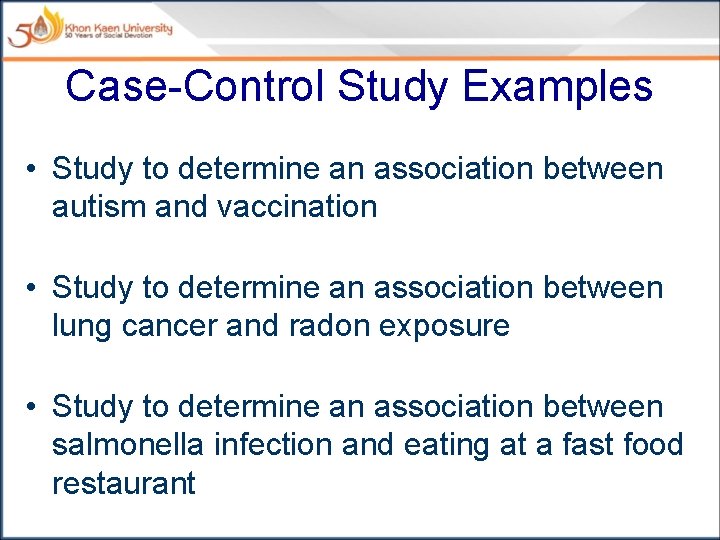
Case-Control Study Examples • Study to determine an association between autism and vaccination • Study to determine an association between lung cancer and radon exposure • Study to determine an association between salmonella infection and eating at a fast food restaurant
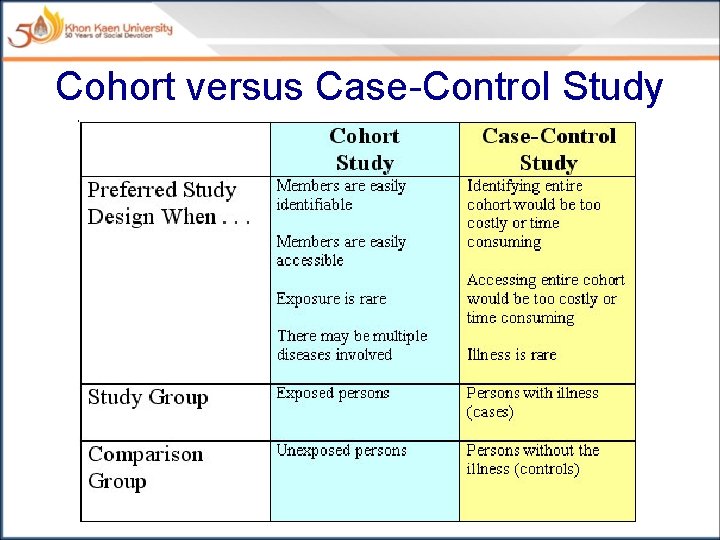
Cohort versus Case-Control Study
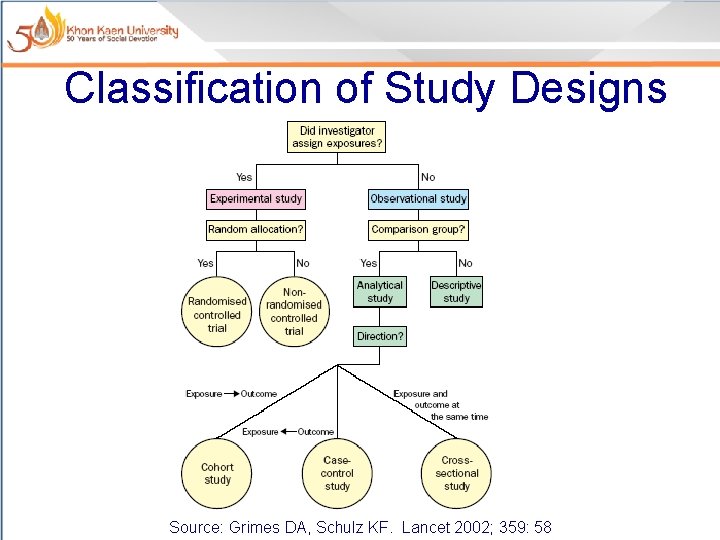
Classification of Study Designs Source: Grimes DA, Schulz KF. Lancet 2002; 359: 58
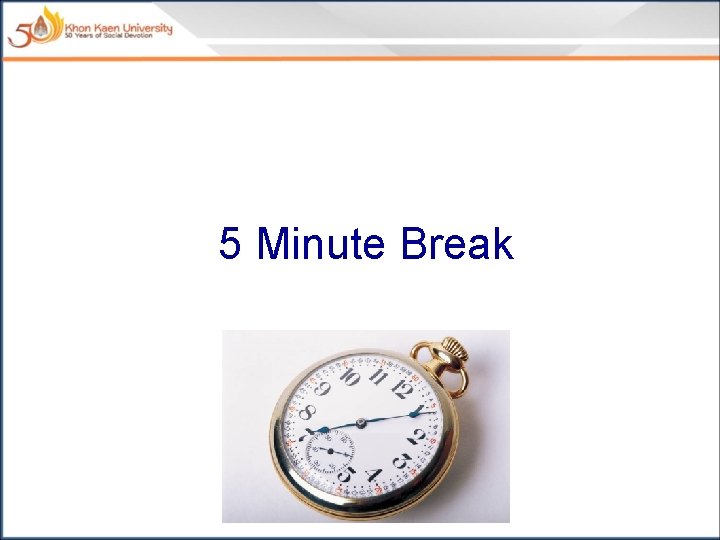
5 Minute Break
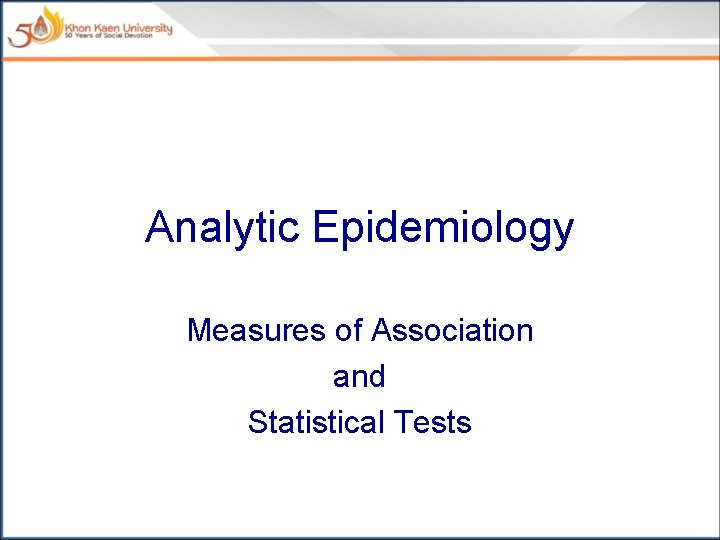
Analytic Epidemiology Measures of Association and Statistical Tests
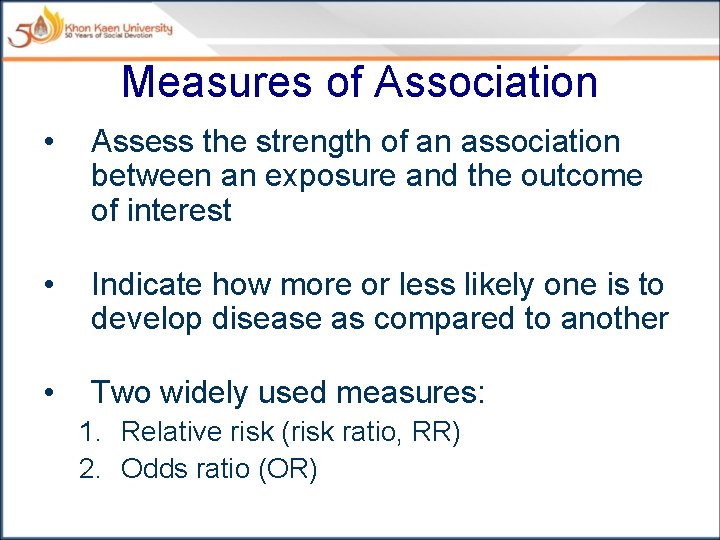
Measures of Association • Assess the strength of an association between an exposure and the outcome of interest • Indicate how more or less likely one is to develop disease as compared to another • Two widely used measures: 1. Relative risk (risk ratio, RR) 2. Odds ratio (OR)
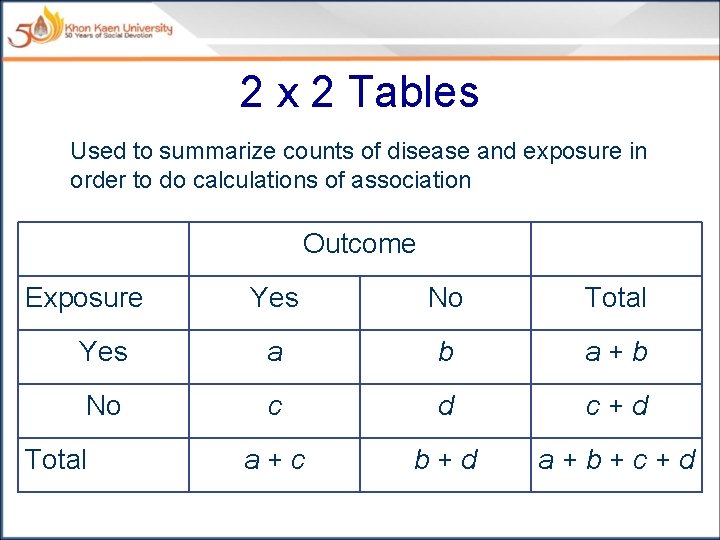
2 x 2 Tables Used to summarize counts of disease and exposure in order to do calculations of association Outcome Exposure Yes No Total Yes a b a+b No c d c+d a+c b+d a+b+c+d Total
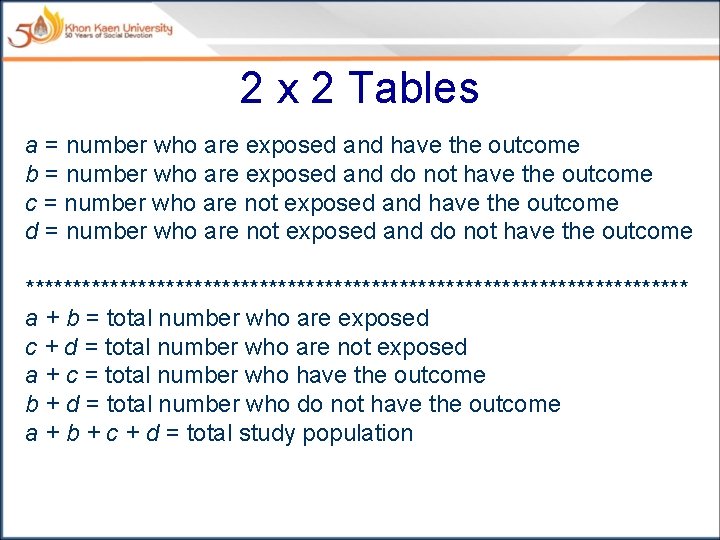
2 x 2 Tables a = number who are exposed and have the outcome b = number who are exposed and do not have the outcome c = number who are not exposed and have the outcome d = number who are not exposed and do not have the outcome ************************************ a + b = total number who are exposed c + d = total number who are not exposed a + c = total number who have the outcome b + d = total number who do not have the outcome a + b + c + d = total study population
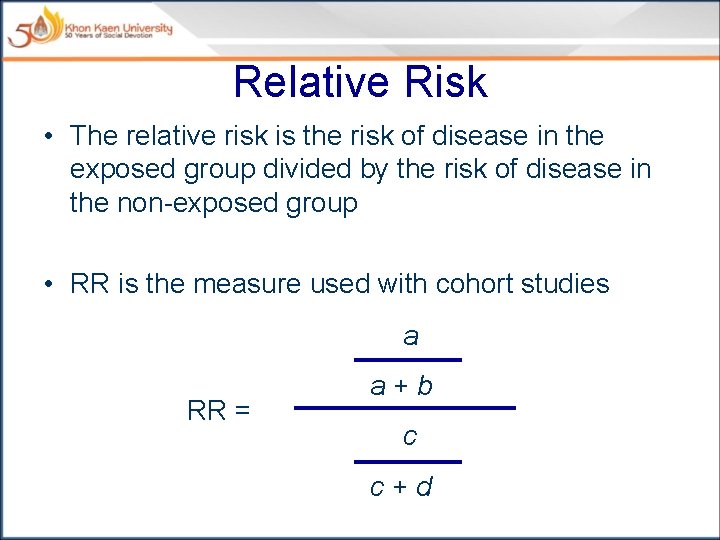
Relative Risk • The relative risk is the risk of disease in the exposed group divided by the risk of disease in the non-exposed group • RR is the measure used with cohort studies a RR = a+b c c+d
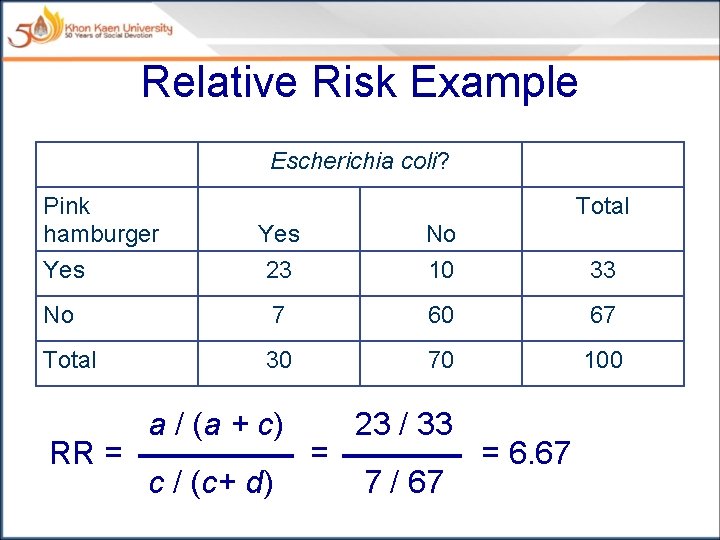
Relative Risk Example Escherichia coli? Pink hamburger Total Yes No Yes 23 10 33 No 7 60 67 Total 30 70 100 RR = a / (a + c) c / (c+ d) = 23 / 33 7 / 67 = 6. 67
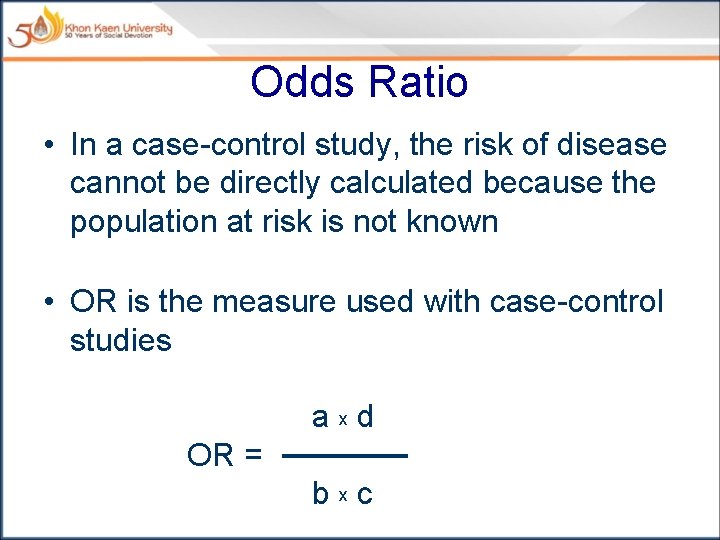
Odds Ratio • In a case-control study, the risk of disease cannot be directly calculated because the population at risk is not known • OR is the measure used with case-control studies axd OR = bxc
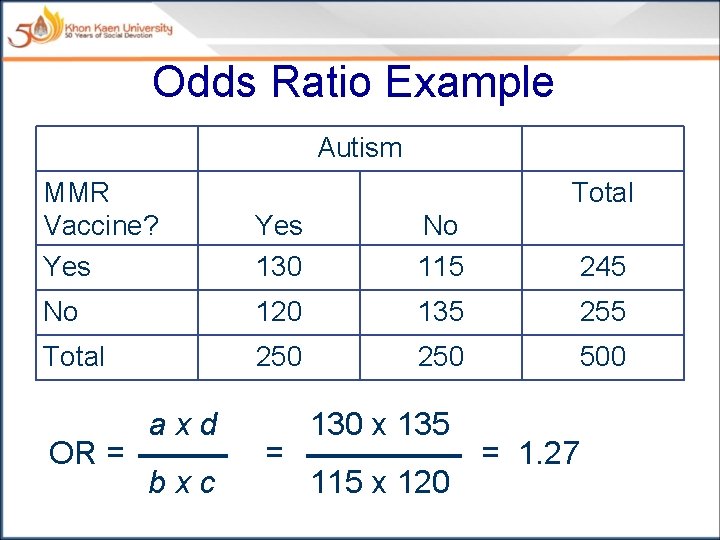
Odds Ratio Example Autism MMR Vaccine? Yes 130 No 115 245 No 120 135 255 Total 250 500 OR = axd bxc Total = 130 x 135 115 x 120 = 1. 27
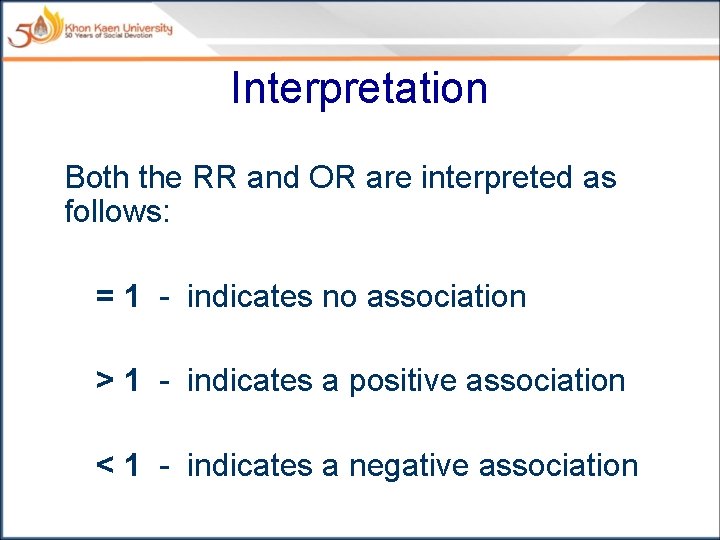
Interpretation Both the RR and OR are interpreted as follows: = 1 - indicates no association > 1 - indicates a positive association < 1 - indicates a negative association
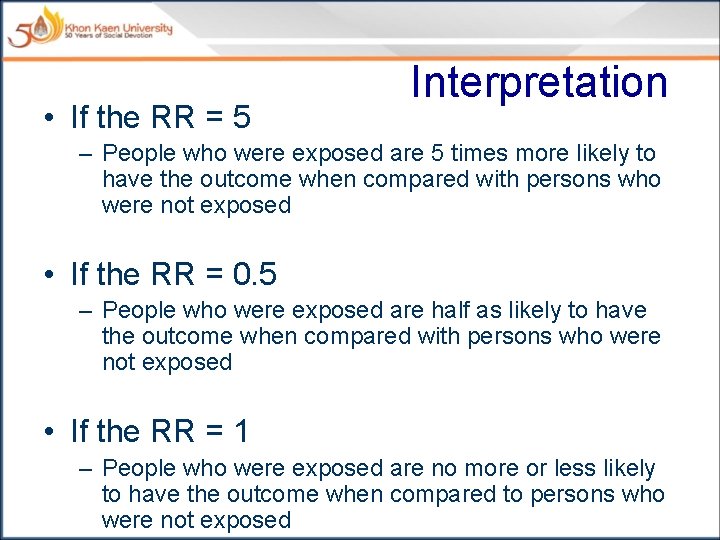
• If the RR = 5 Interpretation – People who were exposed are 5 times more likely to have the outcome when compared with persons who were not exposed • If the RR = 0. 5 – People who were exposed are half as likely to have the outcome when compared with persons who were not exposed • If the RR = 1 – People who were exposed are no more or less likely to have the outcome when compared to persons who were not exposed
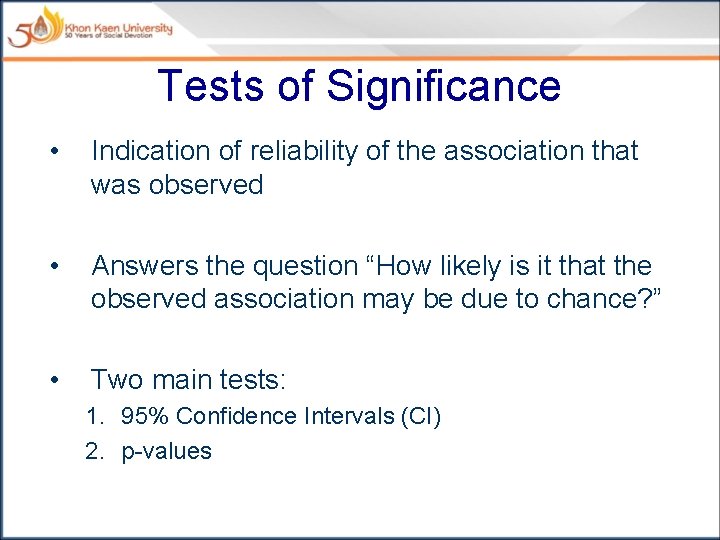
Tests of Significance • Indication of reliability of the association that was observed • Answers the question “How likely is it that the observed association may be due to chance? ” • Two main tests: 1. 95% Confidence Intervals (CI) 2. p-values
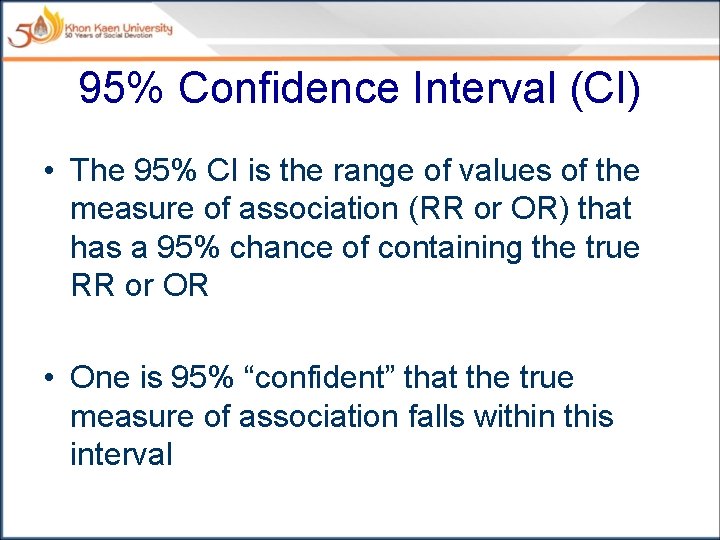
95% Confidence Interval (CI) • The 95% CI is the range of values of the measure of association (RR or OR) that has a 95% chance of containing the true RR or OR • One is 95% “confident” that the true measure of association falls within this interval
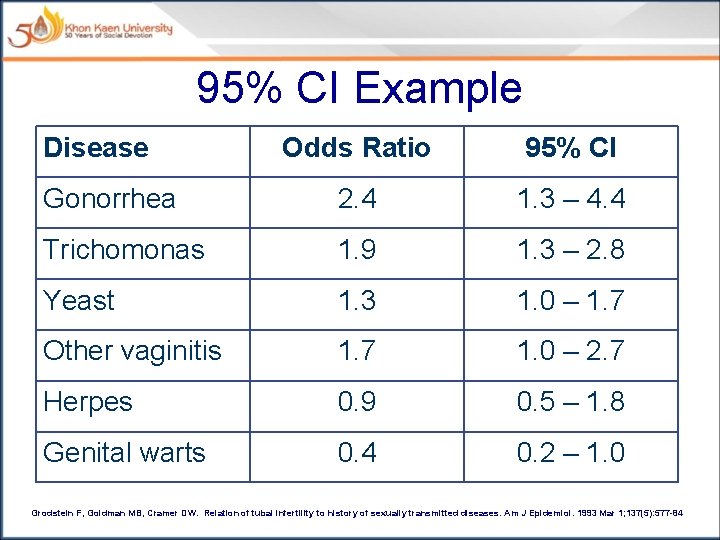
95% CI Example Disease Odds Ratio 95% CI Gonorrhea 2. 4 1. 3 – 4. 4 Trichomonas 1. 9 1. 3 – 2. 8 Yeast 1. 3 1. 0 – 1. 7 Other vaginitis 1. 7 1. 0 – 2. 7 Herpes 0. 9 0. 5 – 1. 8 Genital warts 0. 4 0. 2 – 1. 0 Grodstein F, Goldman MB, Cramer DW. Relation of tubal infertility to history of sexually transmitted diseases. Am J Epidemiol. 1993 Mar 1; 137(5): 577 -84
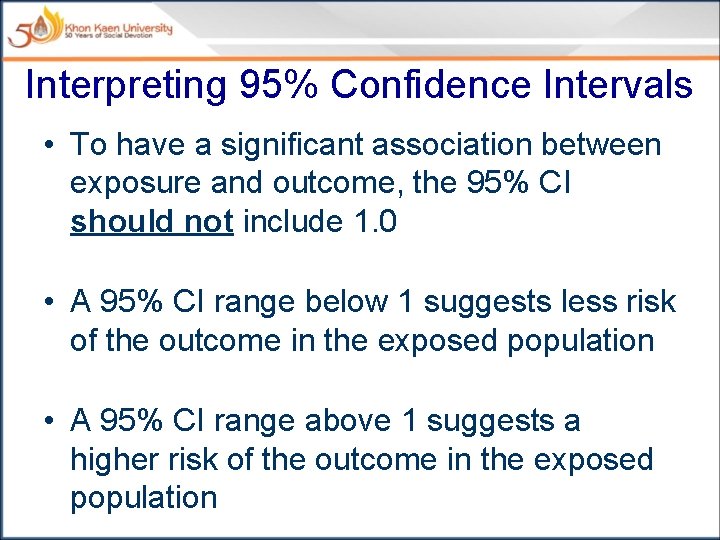
Interpreting 95% Confidence Intervals • To have a significant association between exposure and outcome, the 95% CI should not include 1. 0 • A 95% CI range below 1 suggests less risk of the outcome in the exposed population • A 95% CI range above 1 suggests a higher risk of the outcome in the exposed population
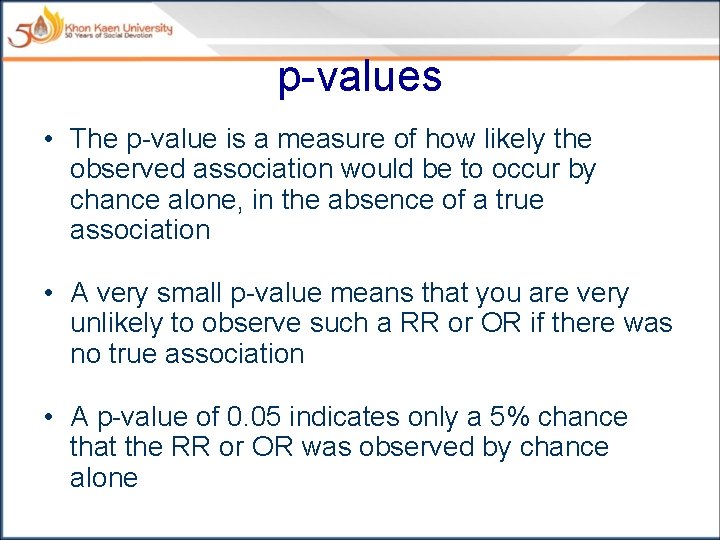
p-values • The p-value is a measure of how likely the observed association would be to occur by chance alone, in the absence of a true association • A very small p-value means that you are very unlikely to observe such a RR or OR if there was no true association • A p-value of 0. 05 indicates only a 5% chance that the RR or OR was observed by chance alone
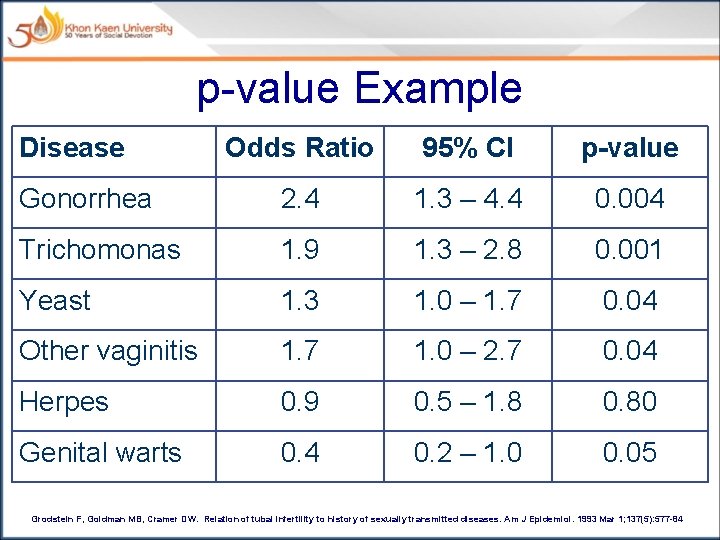
p-value Example Disease Odds Ratio 95% CI p-value Gonorrhea 2. 4 1. 3 – 4. 4 0. 004 Trichomonas 1. 9 1. 3 – 2. 8 0. 001 Yeast 1. 3 1. 0 – 1. 7 0. 04 Other vaginitis 1. 7 1. 0 – 2. 7 0. 04 Herpes 0. 9 0. 5 – 1. 8 0. 80 Genital warts 0. 4 0. 2 – 1. 0 0. 05 Grodstein F, Goldman MB, Cramer DW. Relation of tubal infertility to history of sexually transmitted diseases. Am J Epidemiol. 1993 Mar 1; 137(5): 577 -84
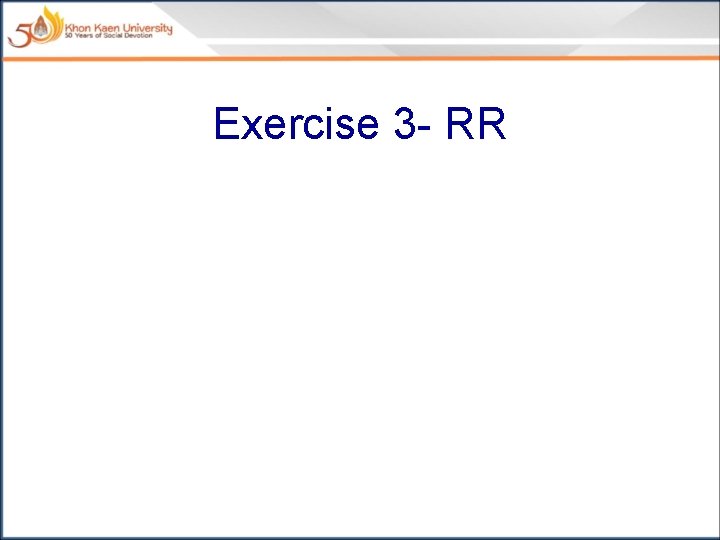
Exercise 3 - RR
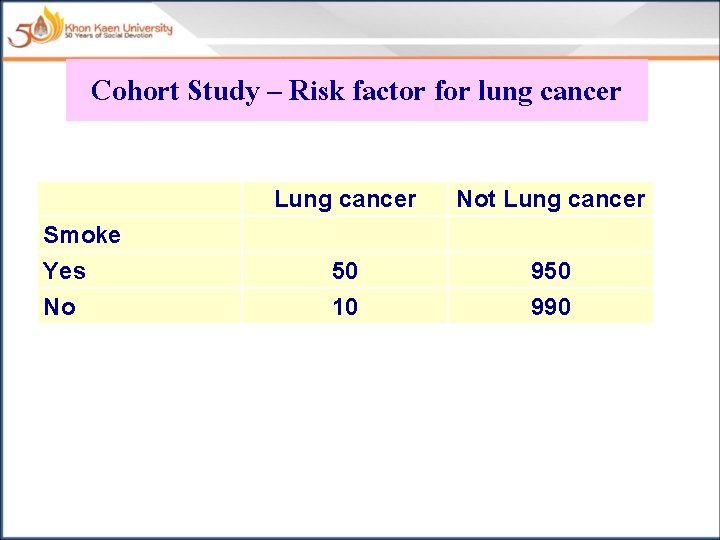
Cohort Study – Risk factor for lung cancer Smoke Yes No Lung cancer Not Lung cancer 50 10 950 990
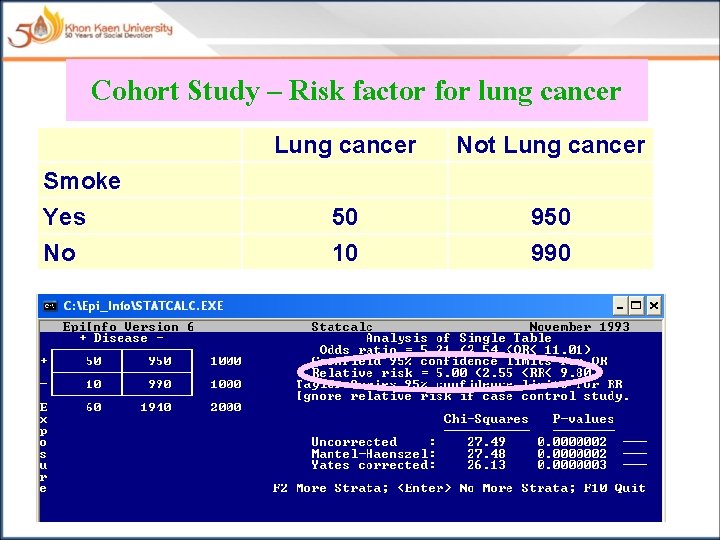
Cohort Study – Risk factor for lung cancer Smoke Yes No Lung cancer Not Lung cancer 50 10 950 990
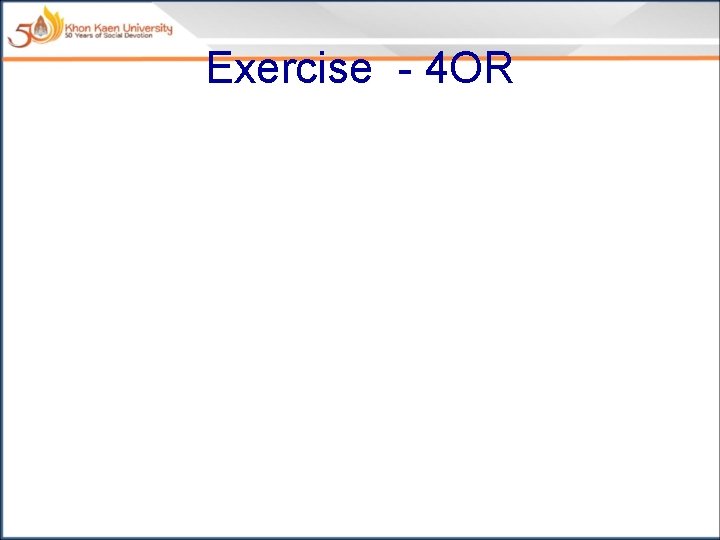
Exercise - 4 OR
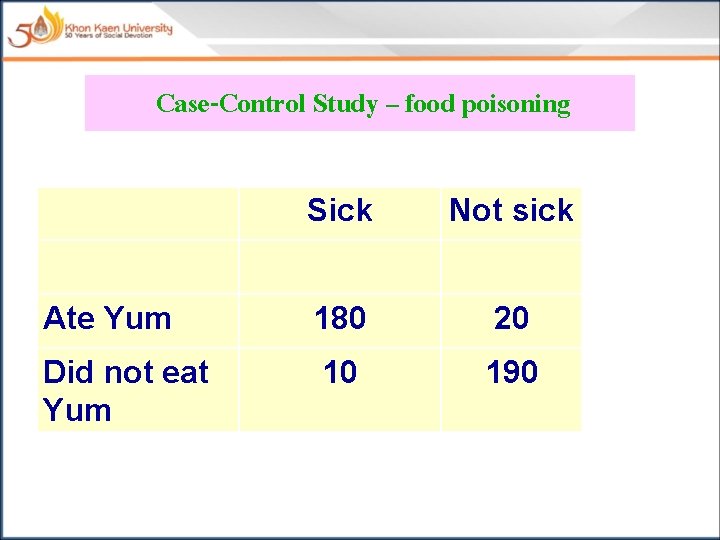
Case-Control Study – food poisoning Sick Not sick Ate Yum 180 20 Did not eat Yum 10 190
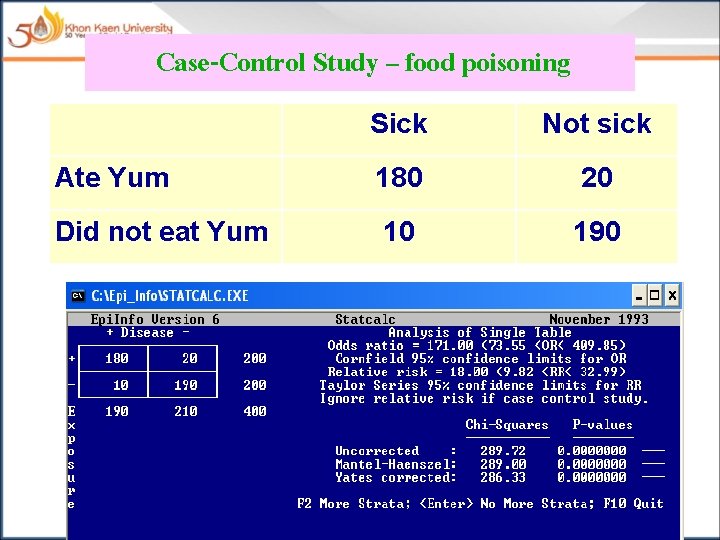
Case-Control Study – food poisoning Sick Not sick Ate Yum 180 20 Did not eat Yum 10 190
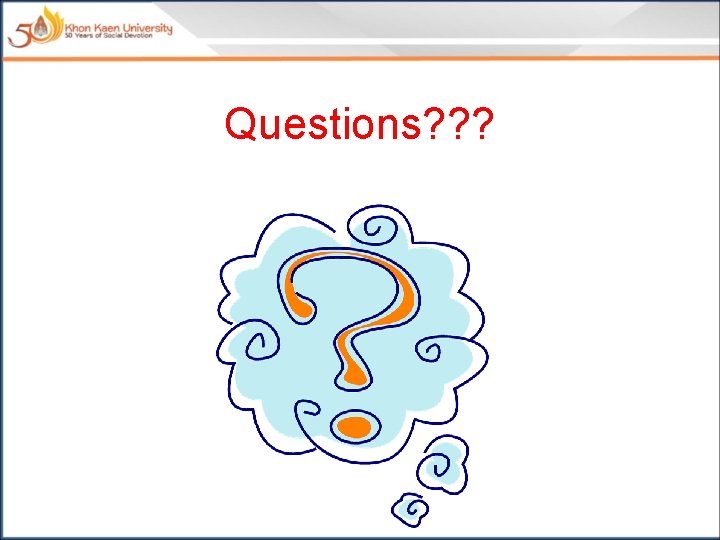
Questions? ? ?
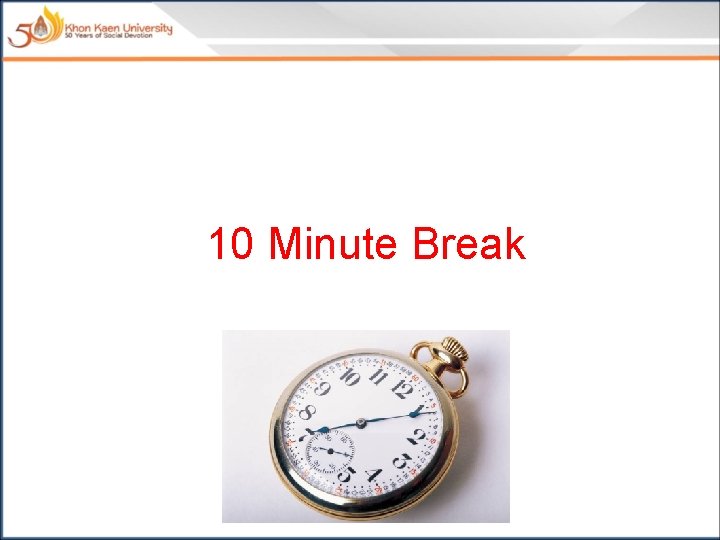
10 Minute Break
Difference between descriptive and analytic epidemiology
Classification of epidemiological studies
Incidence vs prevalence
What is descriptive study in epidemiology
Descriptive epidemiology
Descriptive vs analytical epidemiology
Spurious association in epidemiology
Celiac beri beri
Pros and cons of cross sectional study
Nutritional epidemiology
Gordon epidemiology
Period prevalence formula
Epidemiology definition
Effect modification vs confounding
Logistic regression epidemiology
Meaning of epidermiology
Field epidemiology ppt
Epidemiology made easy
Temporal relationship epidemiology example
What is ookinite
Epidemiology kept simple
Defination of epidemiology
Seven uses of epidemiology
Distribution in epidemiology
Prevalence calculation example
Prevalensi adalah
Define epidemiology
Formula for attack rate
Formula for attack rate
Measures of association in epidemiology
Diabetic ketoacidosis epidemiology
Epidemiology concept
Epidemiological triad
Ramboman
Formula for attack rate
How dr. wafaa elsadr epidemiology professor
Cbic recertification
Wheel model of causation
Ramboman
How dr. wafaa elsadr epidemiology professor
Advantages and disadvantages of nutritional epidemiology
Distribution in epidemiology
Chapter 9 conic sections and analytic geometry
Holistic and analytic rubrics
Difference between analytic and synthetic phonics
Analytic tools
Usf final exam matrix
Scoring rubrics about design a museum exhibit
Cookie judging rubric
Ellipse analytic geometry
Dichotomistic
What are the characteristics of good rubrics
Holistic and analytic rubrics
Analytic cubism synthetic cubism
Chapter 5 analytic trigonometry
Chapter 9 topics in analytic geometry
Analytic philosophy of education
Organizing qualitative data
Global analytic continuum
Max qda
Who is the father of analytic geometry
Ccgps analytic geometry answer key
Synthetic vs analytic phonics
Role playing rubrics for elementary
Analytic strategy example
Analytic memo qualitative research example
Scoring key example
Analyticowl
Analytic philosophy in education
Analytic reasoning