Chapter 10 Network Optimization Models 2015 Mc GrawHill
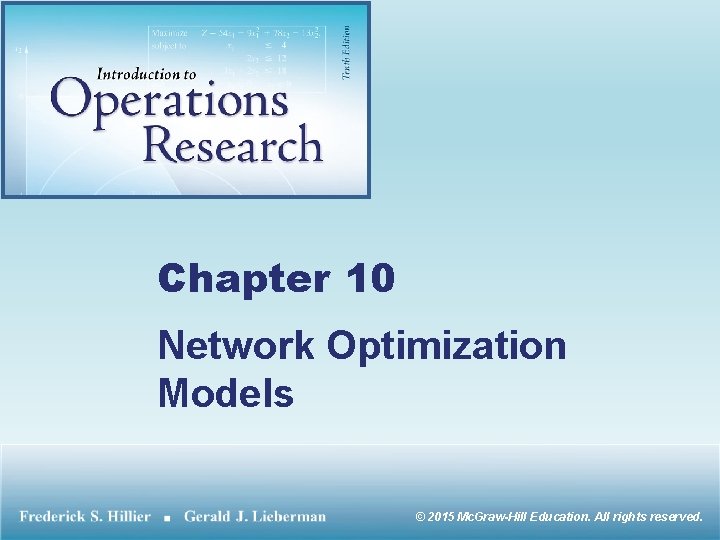
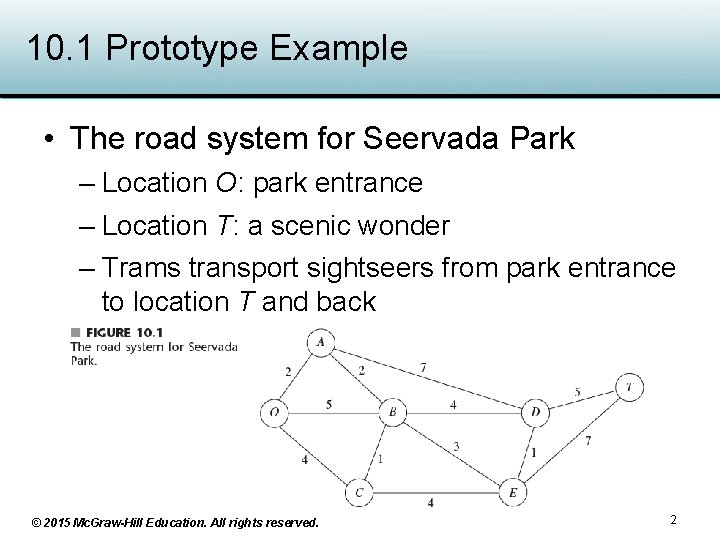
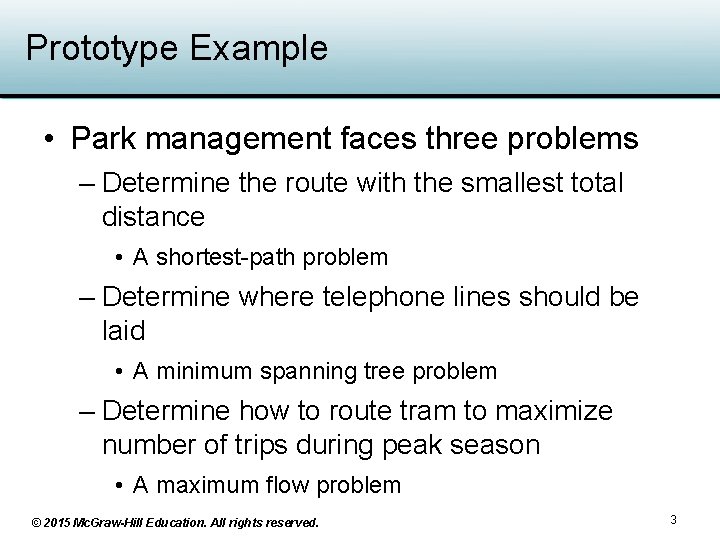
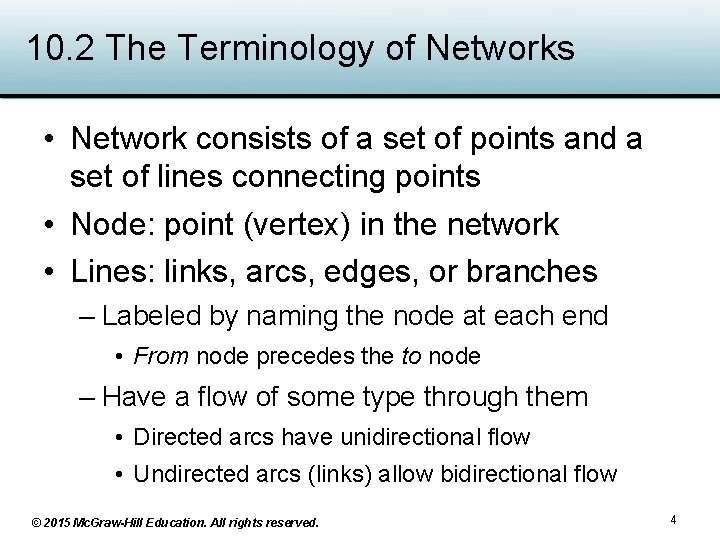
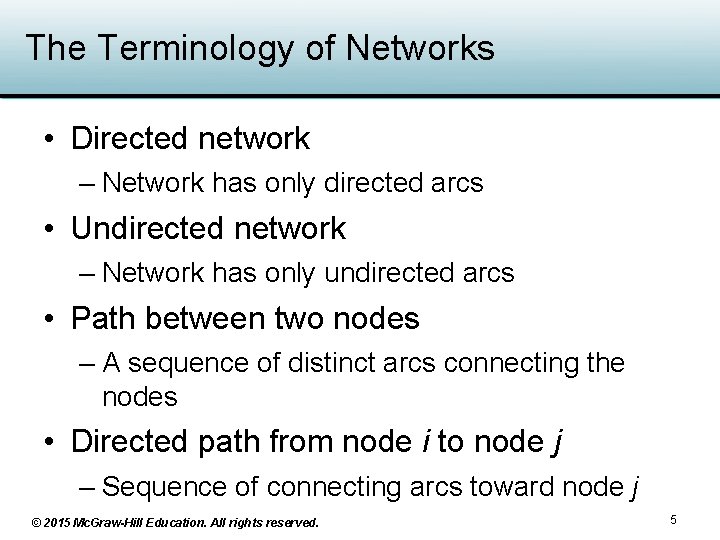
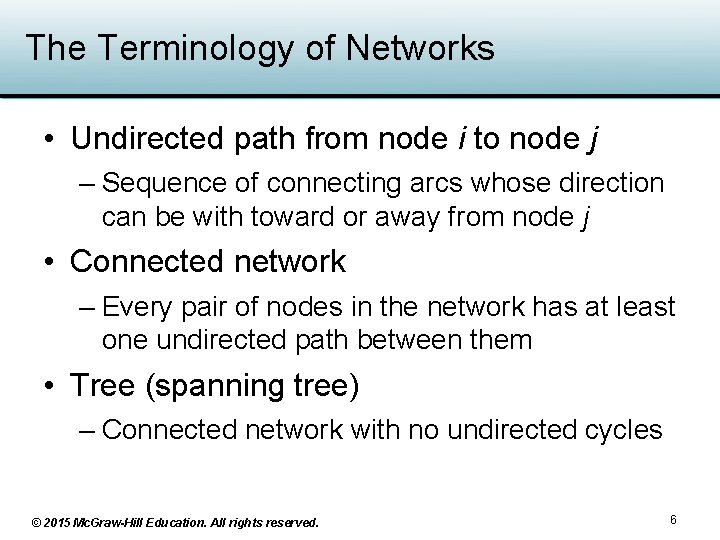
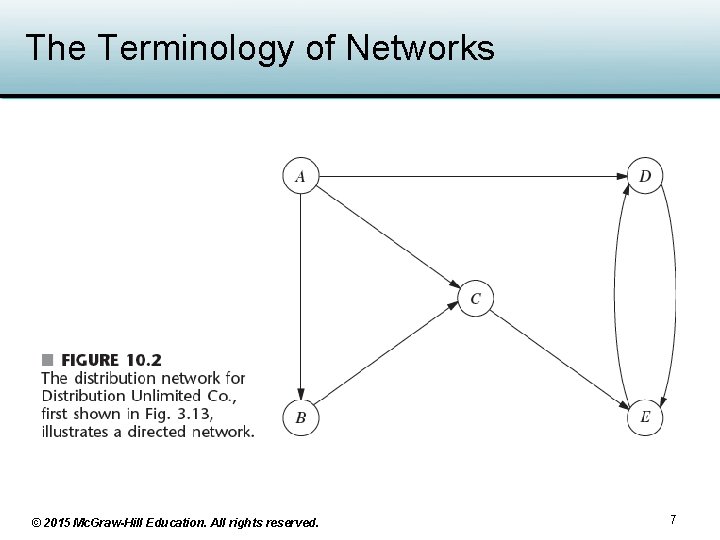
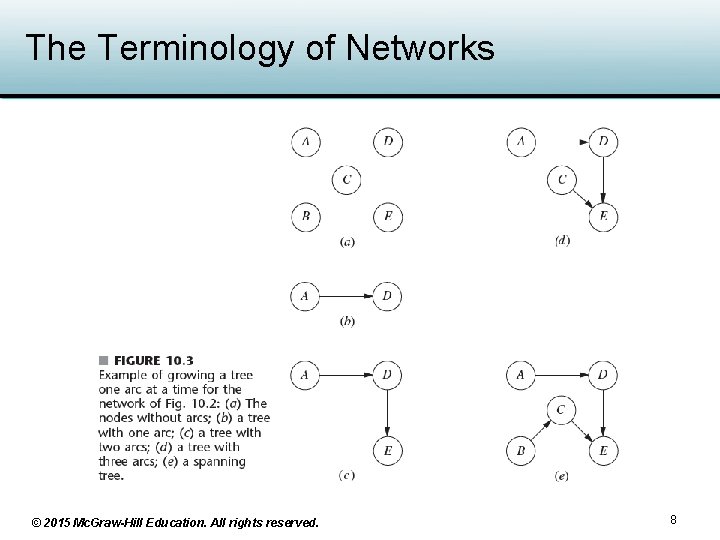
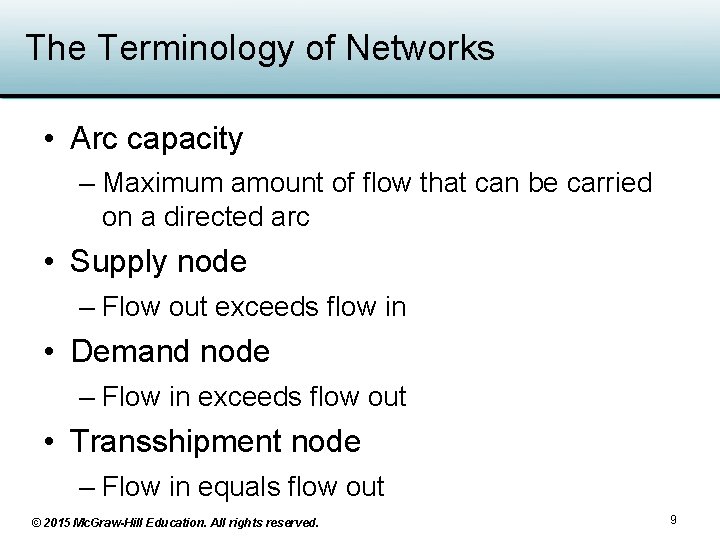
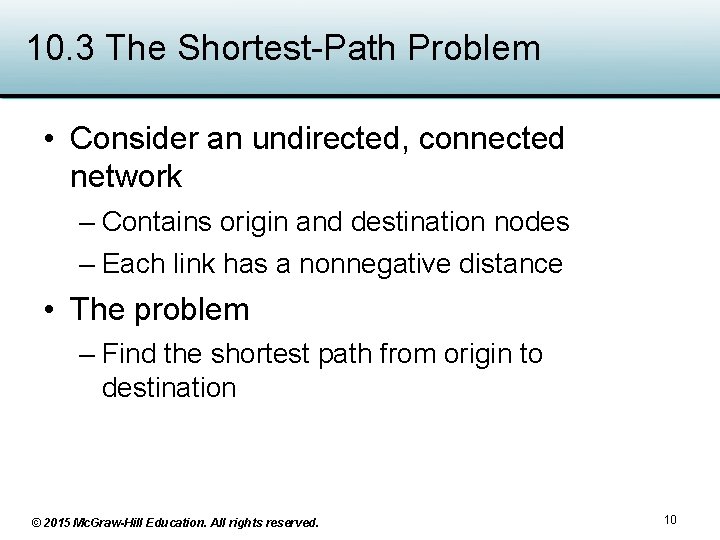
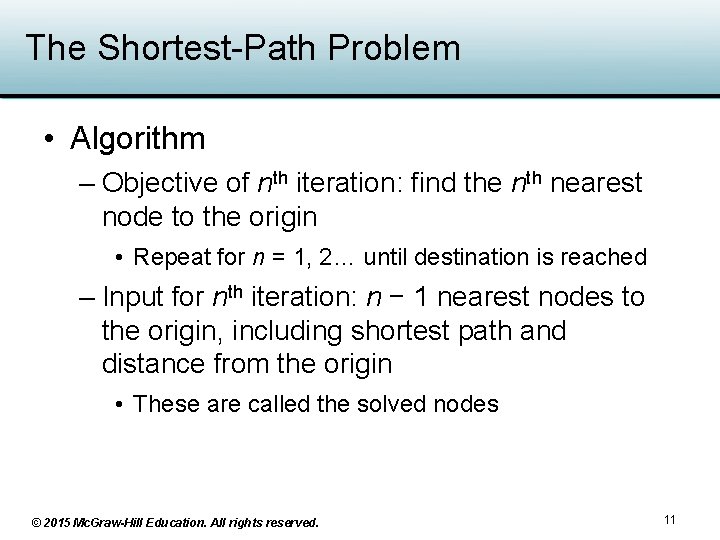
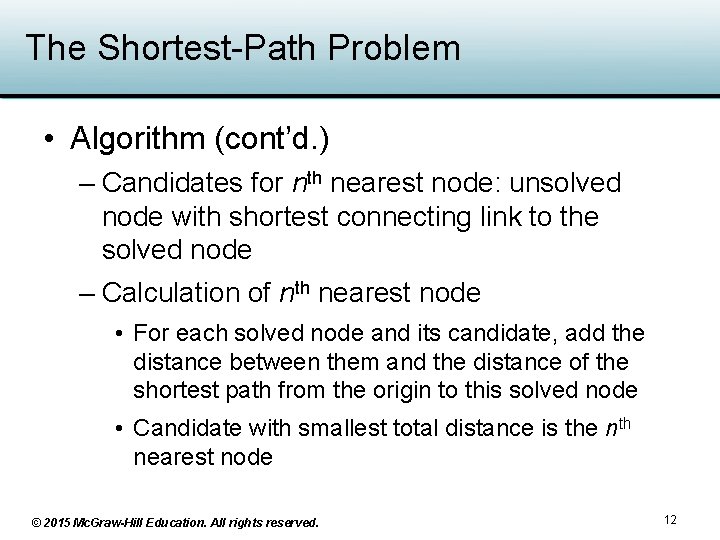
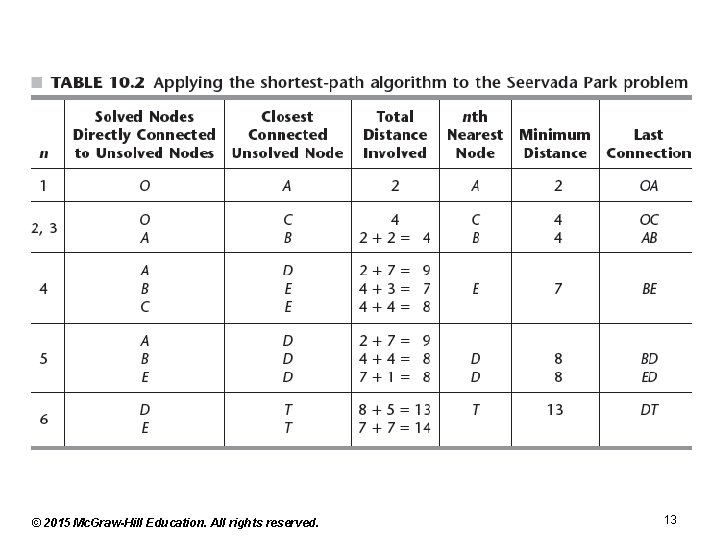
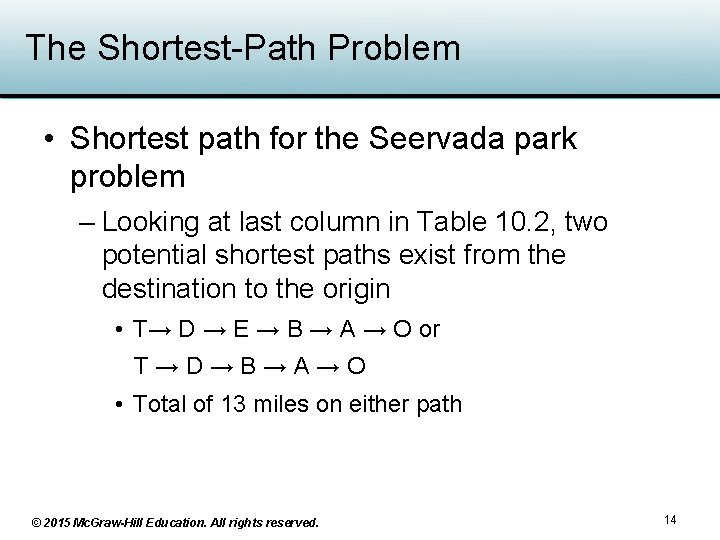
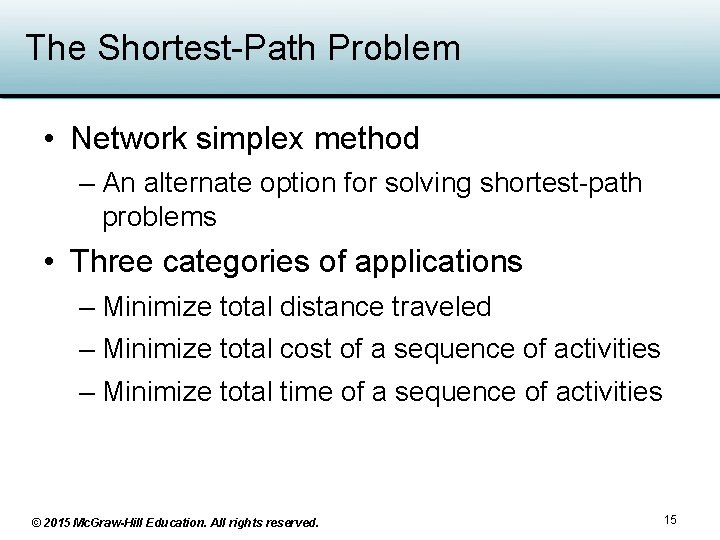
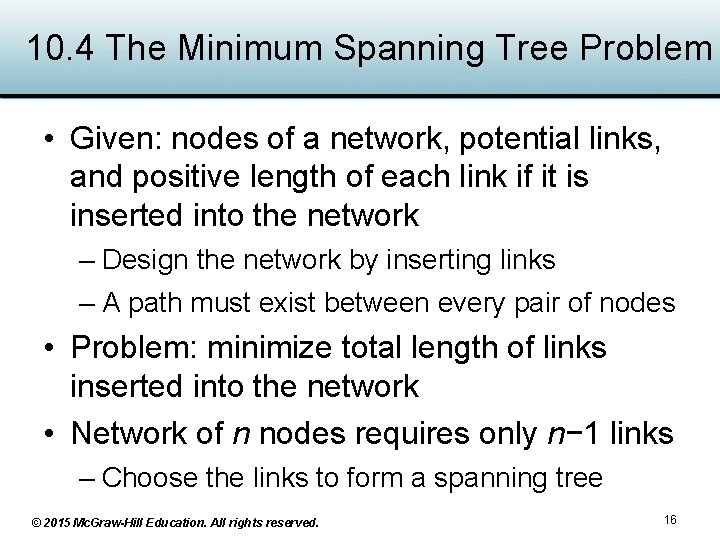
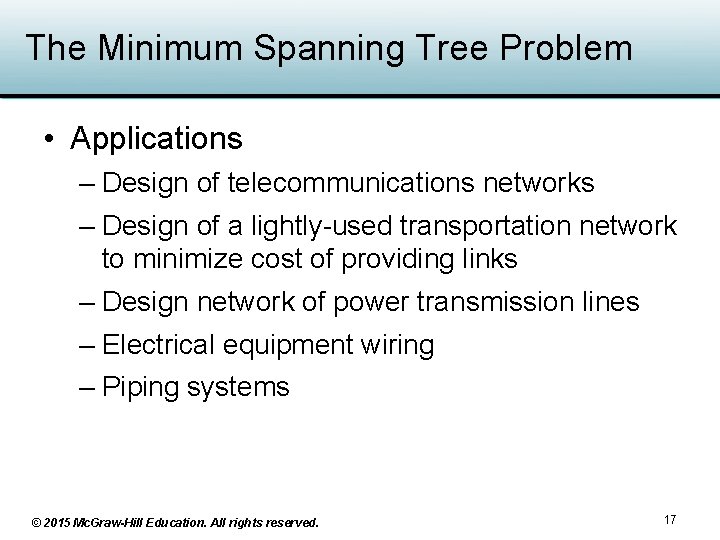
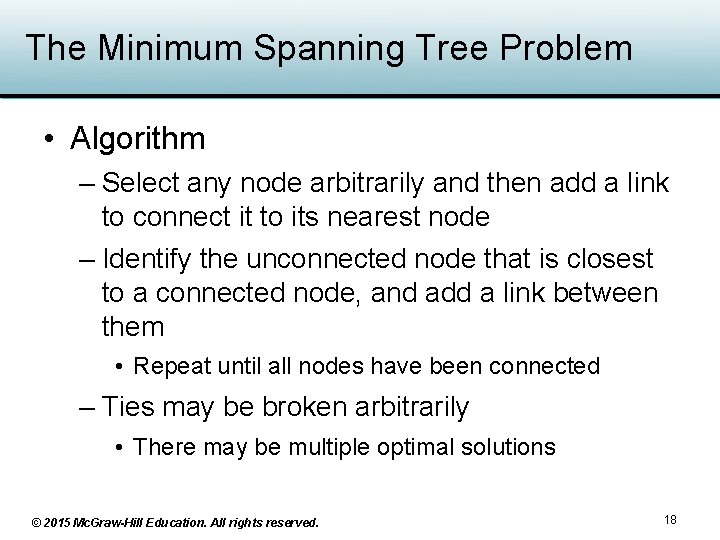
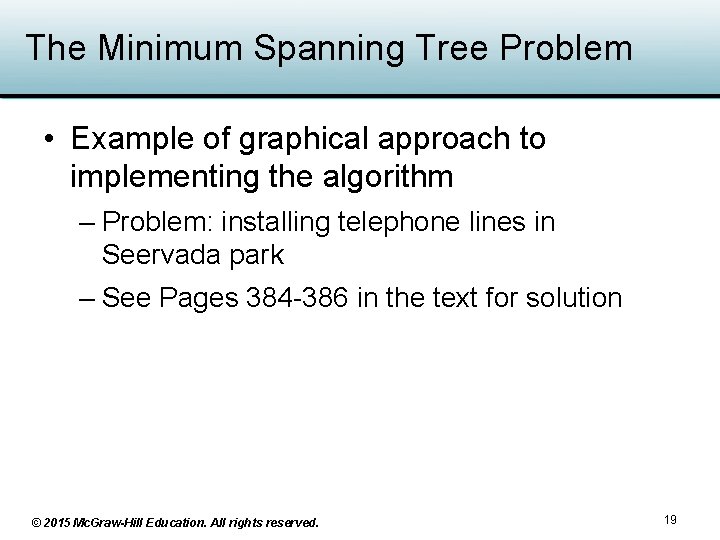
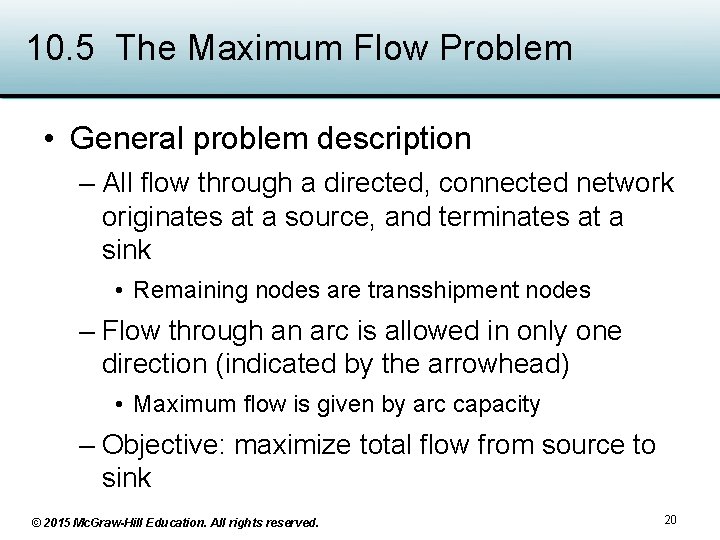
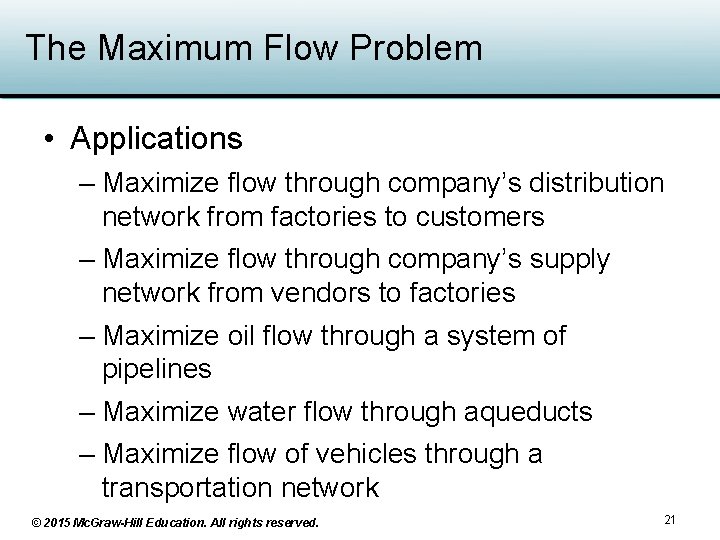
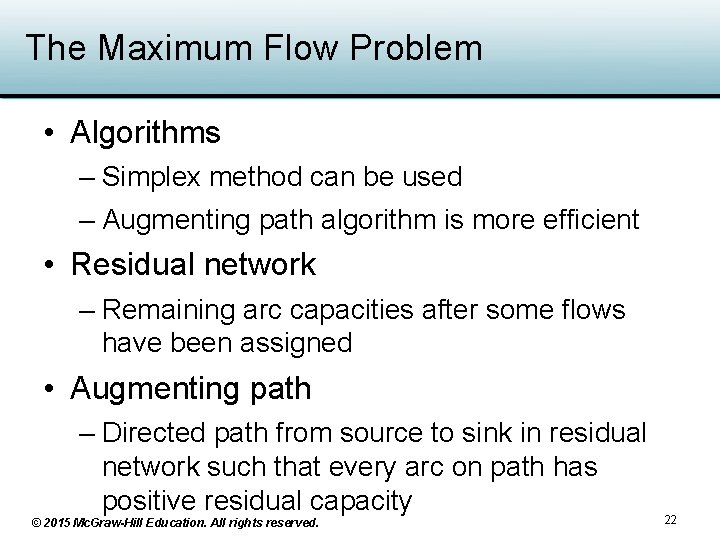
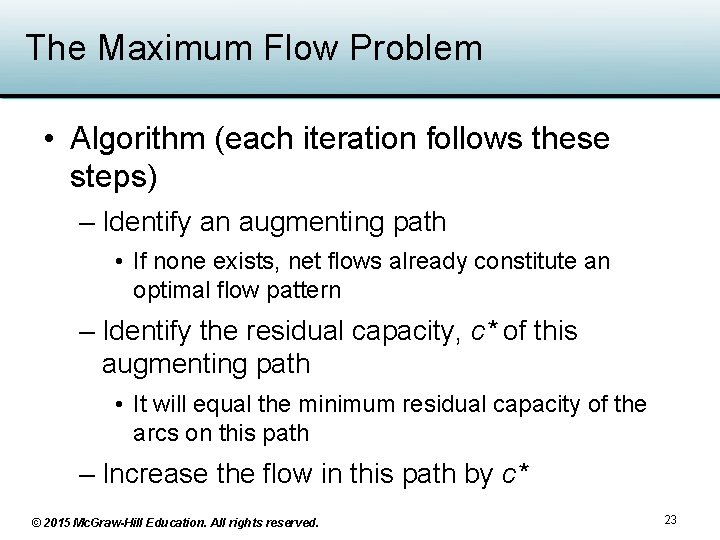
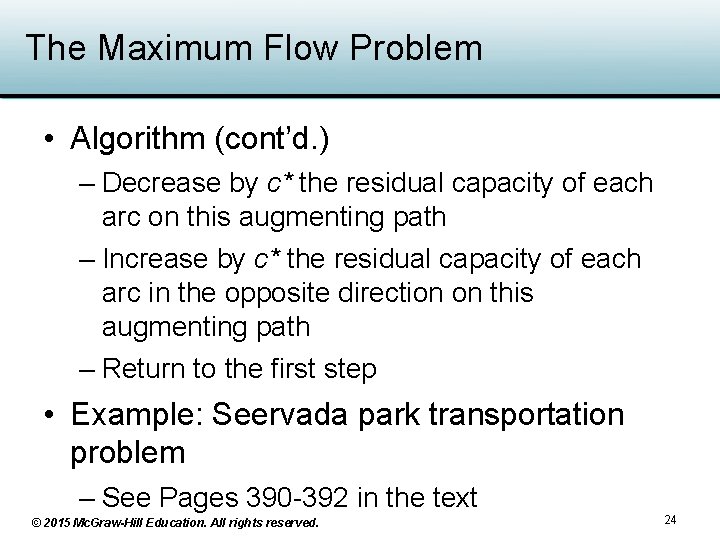
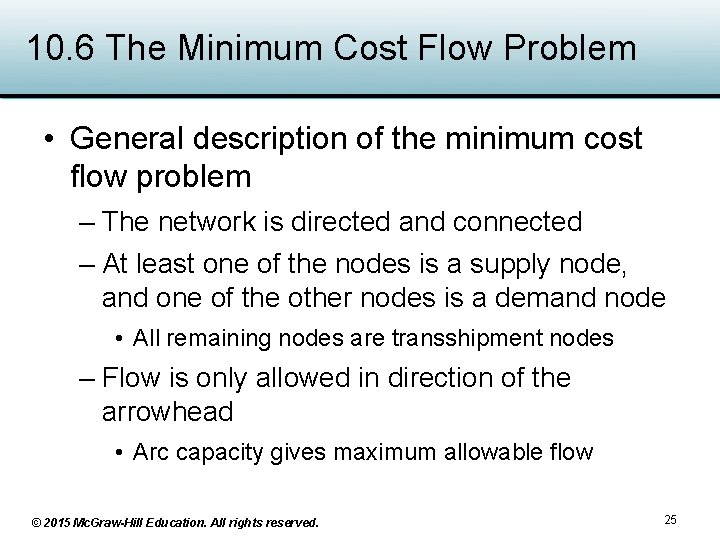
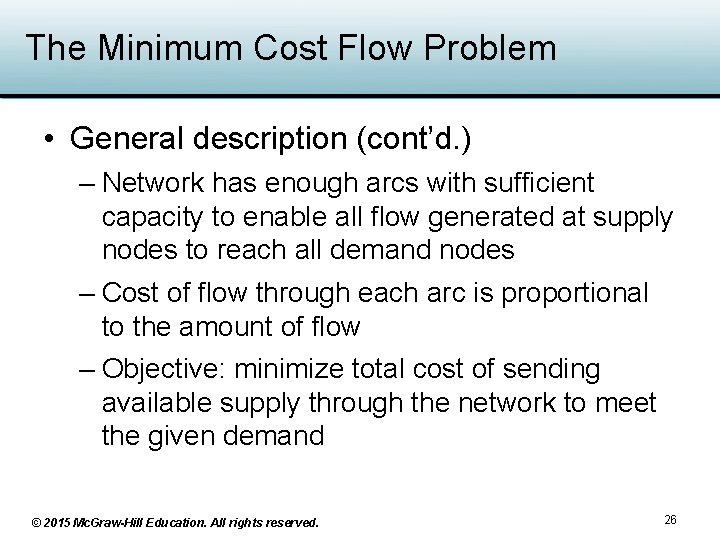
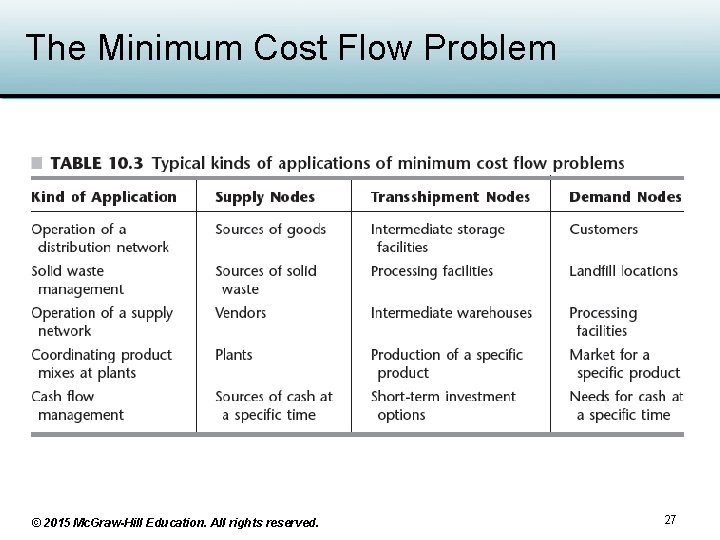
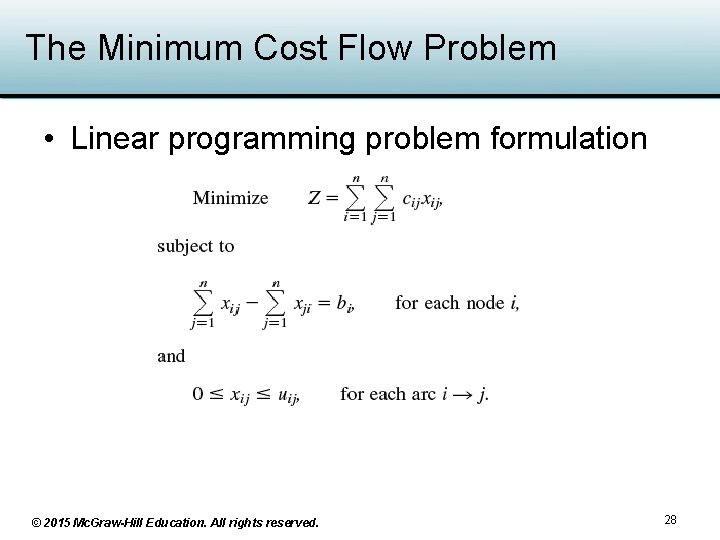
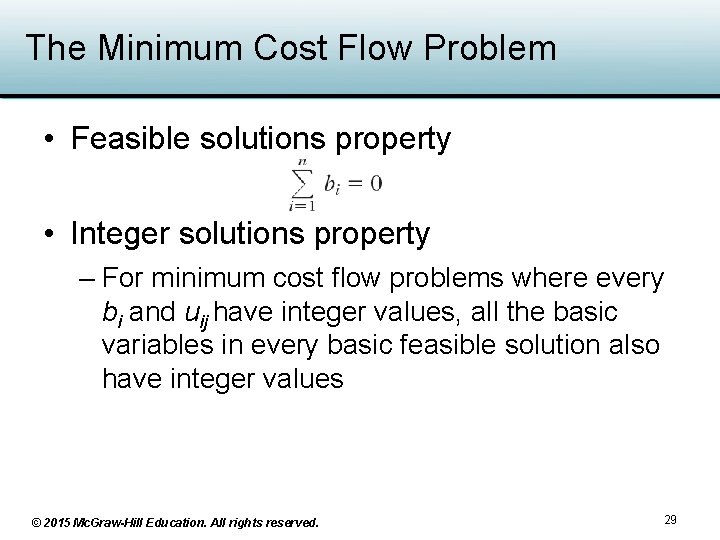
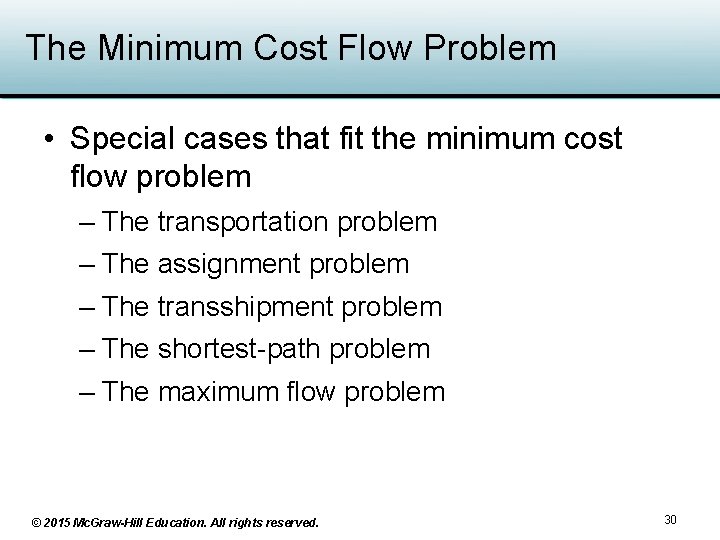
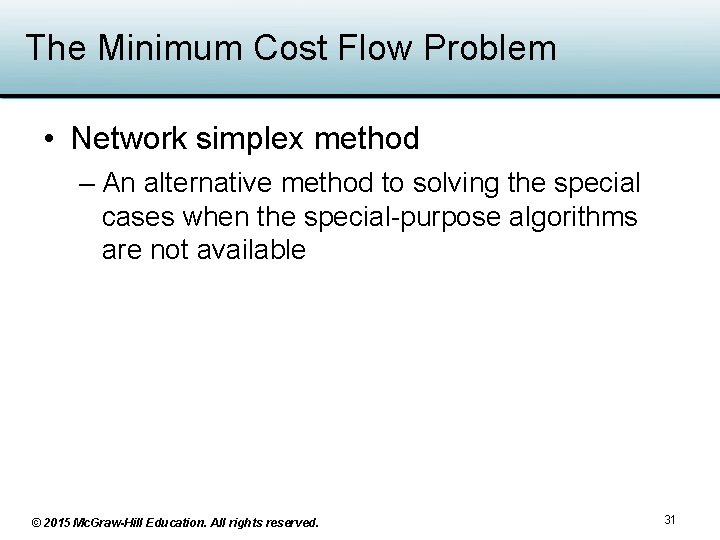
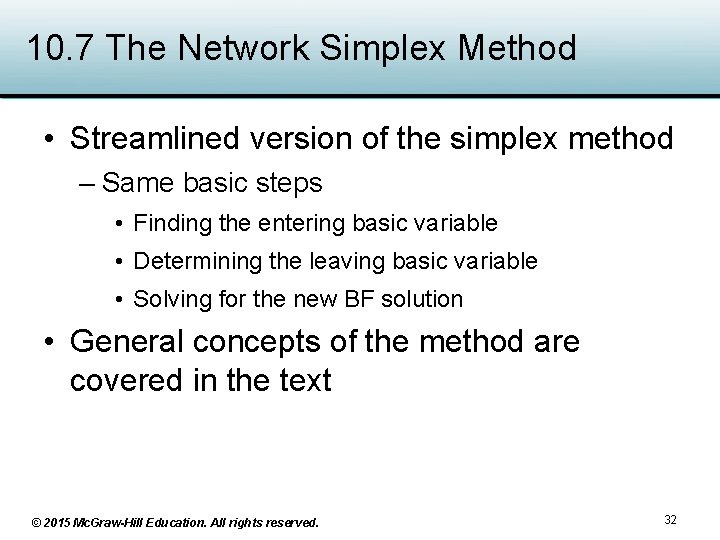
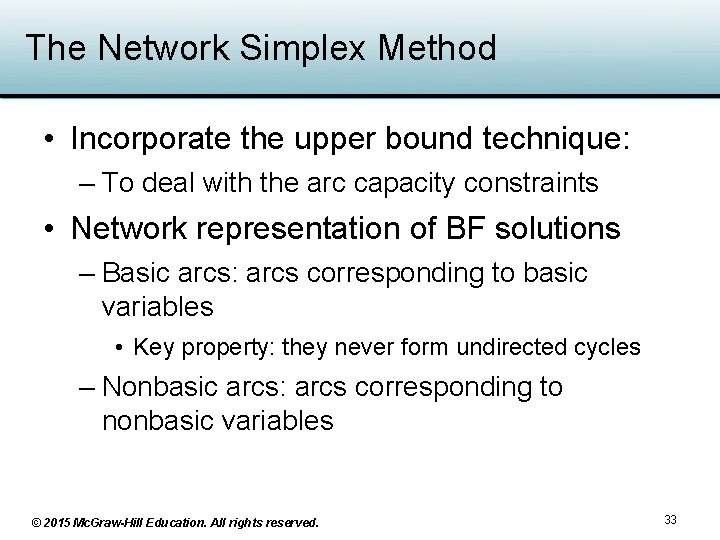
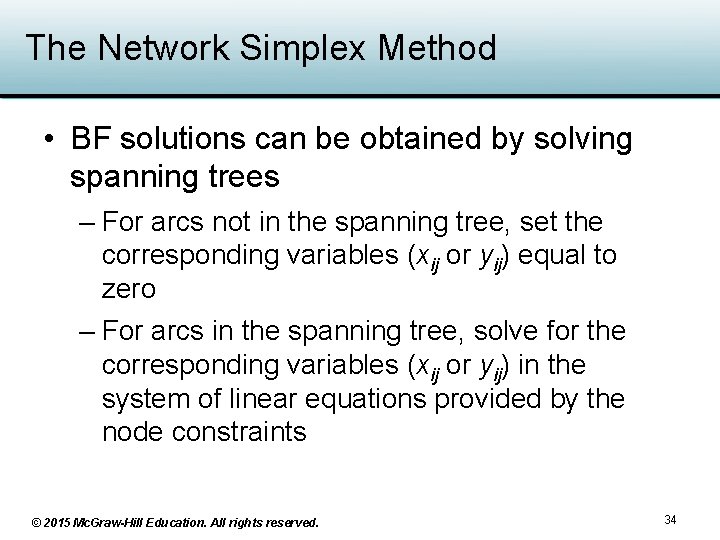
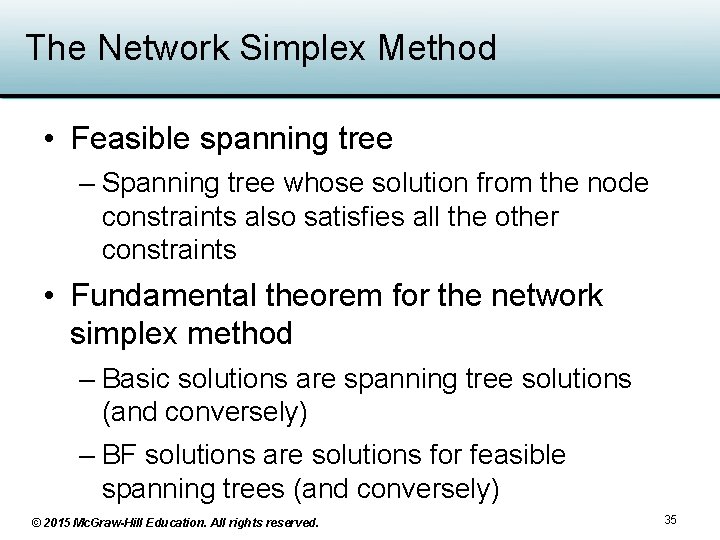
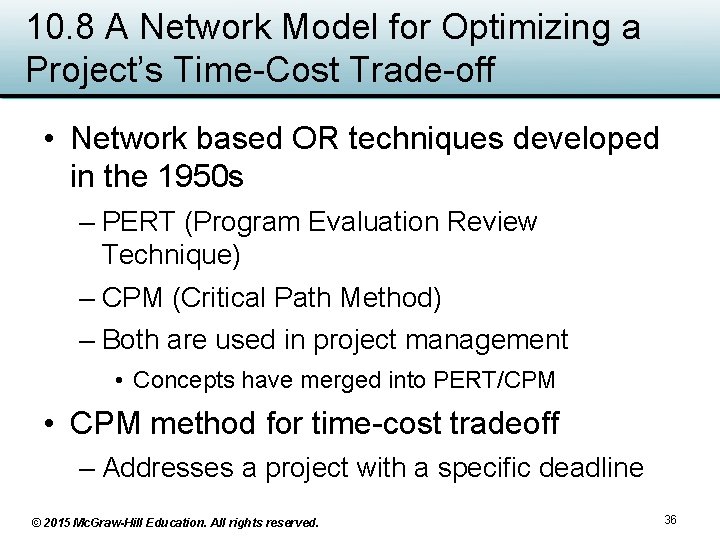
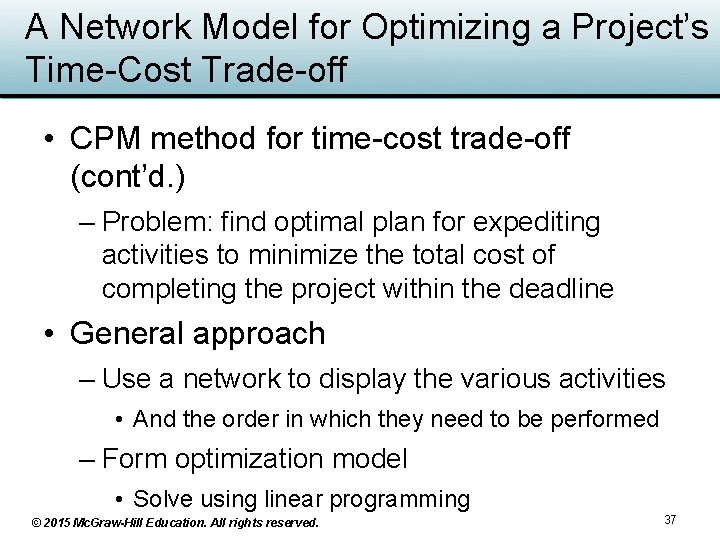
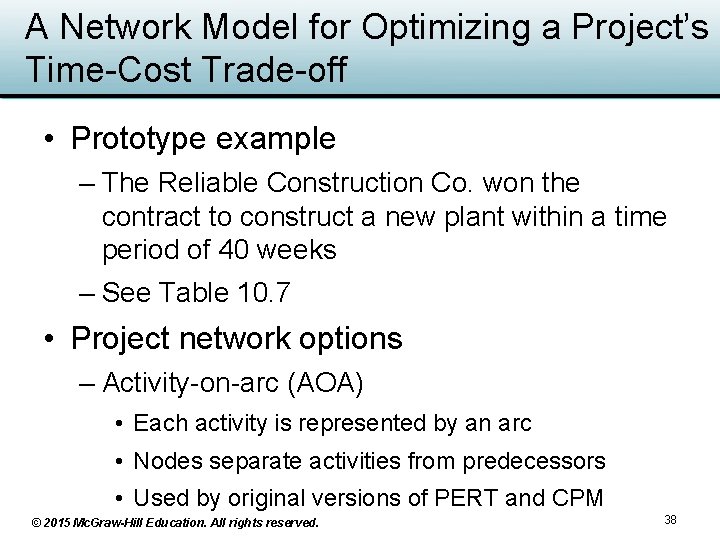
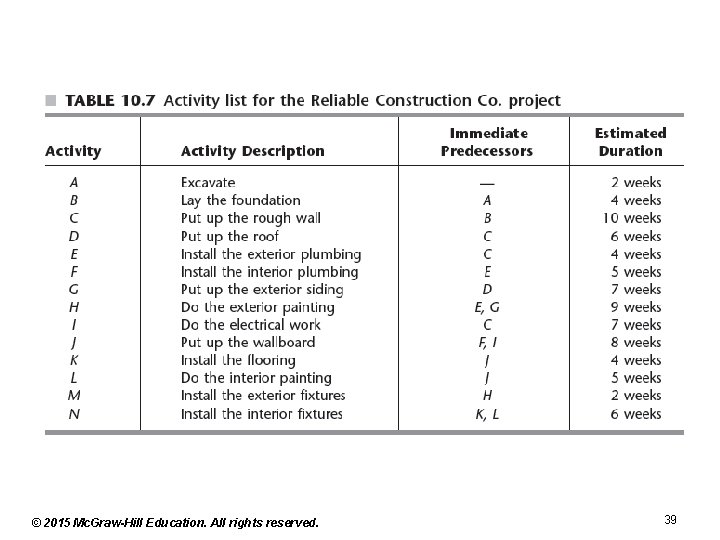
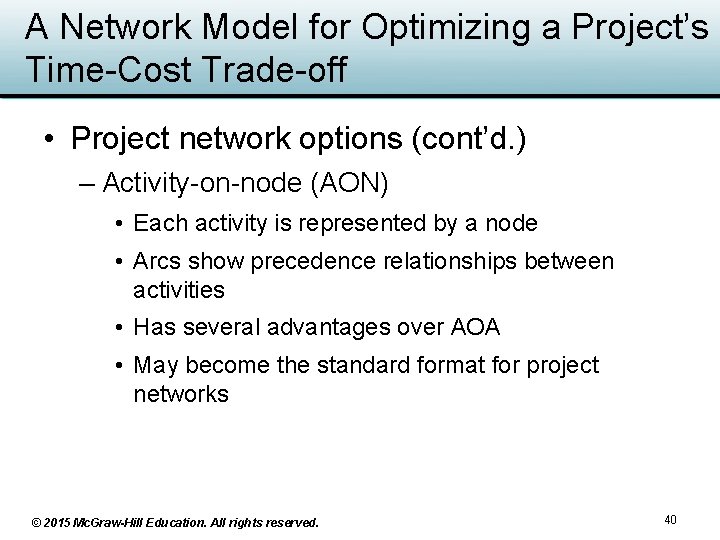
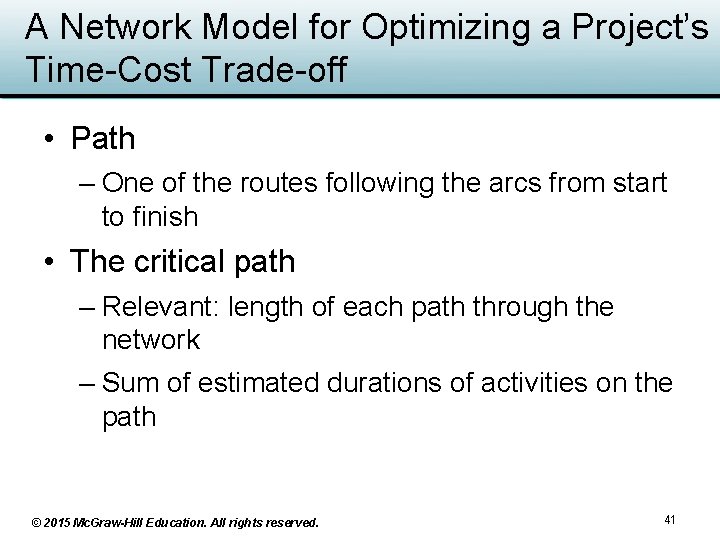
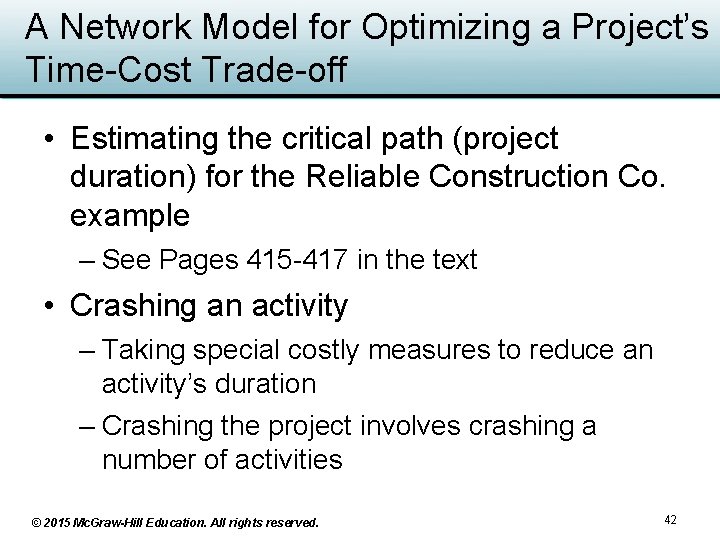
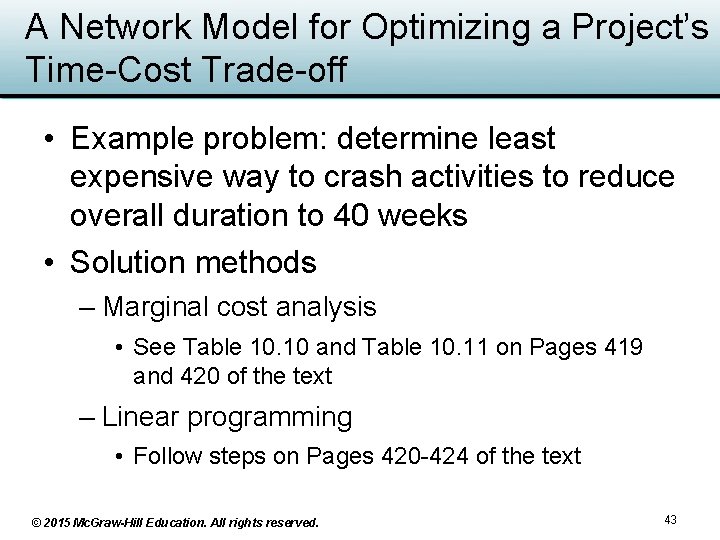
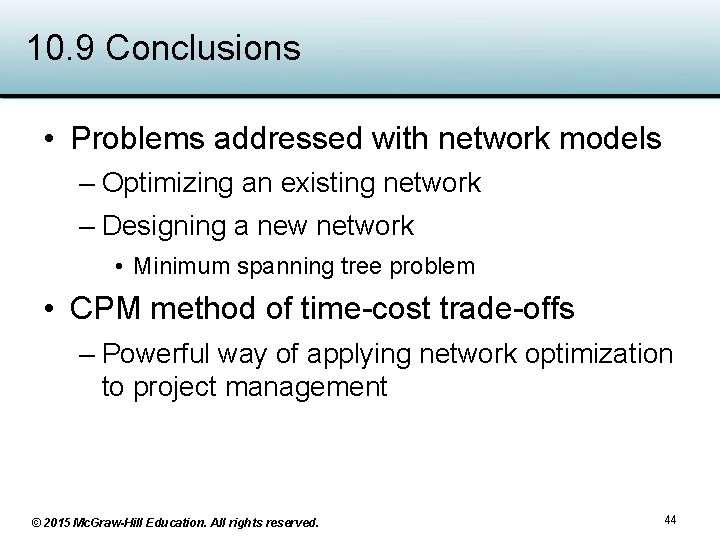
- Slides: 44
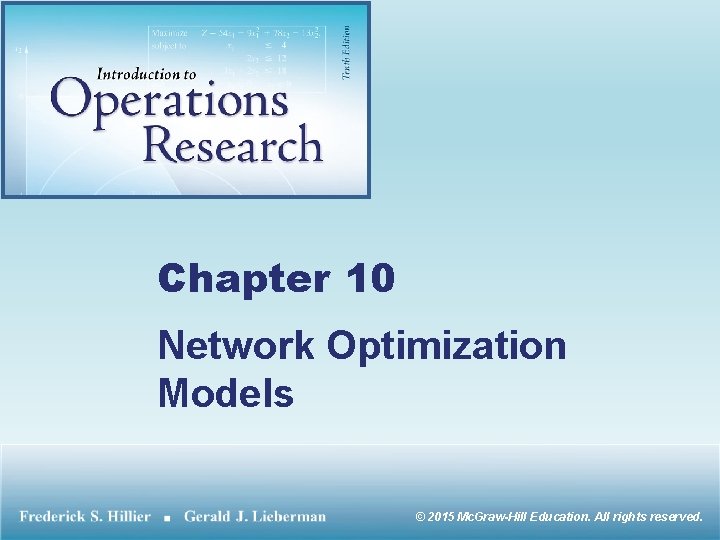
Chapter 10 Network Optimization Models © 2015 Mc. Graw-Hill Education. All rights reserved.
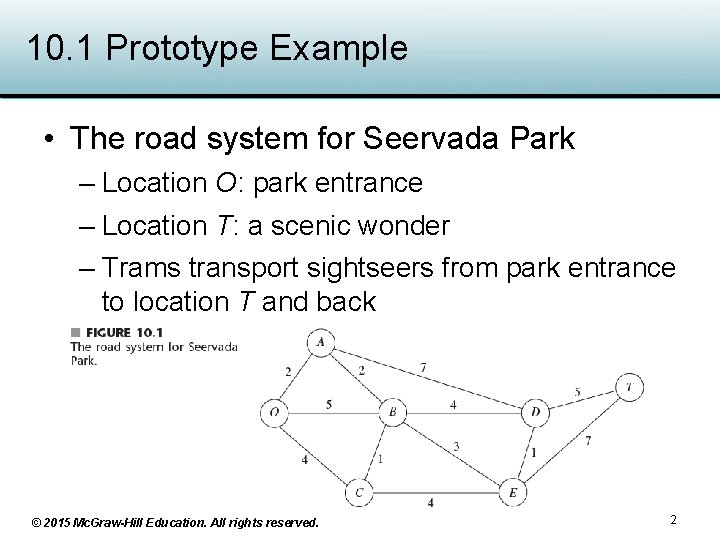
10. 1 Prototype Example • The road system for Seervada Park – Location O: park entrance – Location T: a scenic wonder – Trams transport sightseers from park entrance to location T and back © 2015 Mc. Graw-Hill Education. All rights reserved. 2
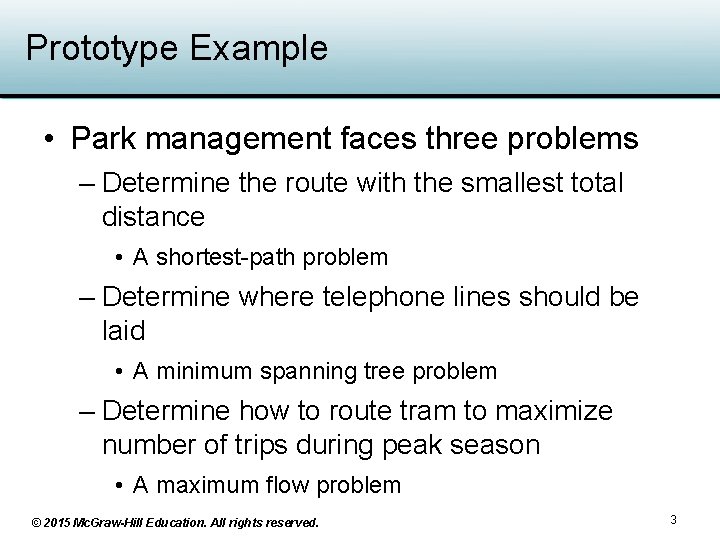
Prototype Example • Park management faces three problems – Determine the route with the smallest total distance • A shortest-path problem – Determine where telephone lines should be laid • A minimum spanning tree problem – Determine how to route tram to maximize number of trips during peak season • A maximum flow problem © 2015 Mc. Graw-Hill Education. All rights reserved. 3
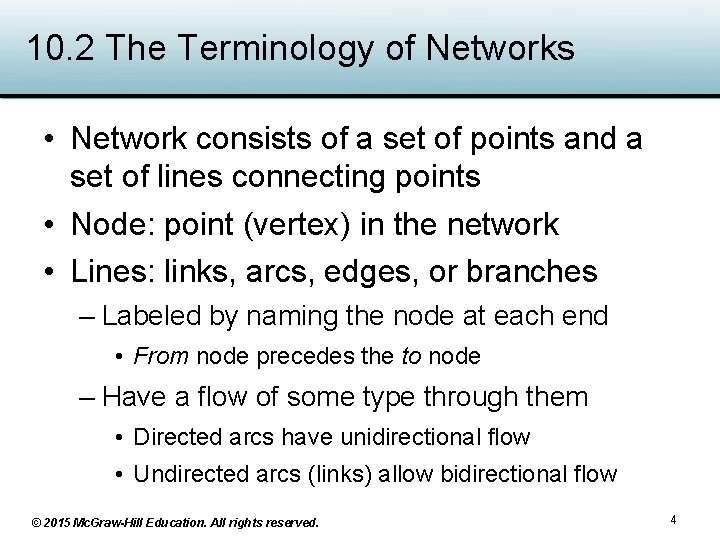
10. 2 The Terminology of Networks • Network consists of a set of points and a set of lines connecting points • Node: point (vertex) in the network • Lines: links, arcs, edges, or branches – Labeled by naming the node at each end • From node precedes the to node – Have a flow of some type through them • Directed arcs have unidirectional flow • Undirected arcs (links) allow bidirectional flow © 2015 Mc. Graw-Hill Education. All rights reserved. 4
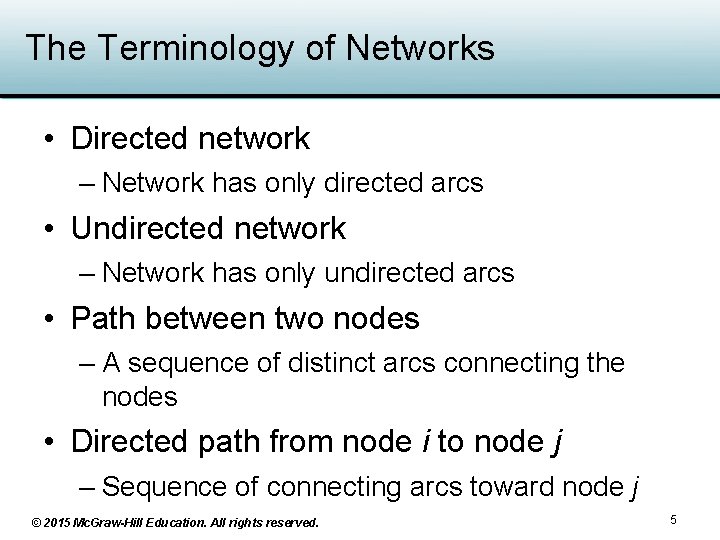
The Terminology of Networks • Directed network – Network has only directed arcs • Undirected network – Network has only undirected arcs • Path between two nodes – A sequence of distinct arcs connecting the nodes • Directed path from node i to node j – Sequence of connecting arcs toward node j © 2015 Mc. Graw-Hill Education. All rights reserved. 5
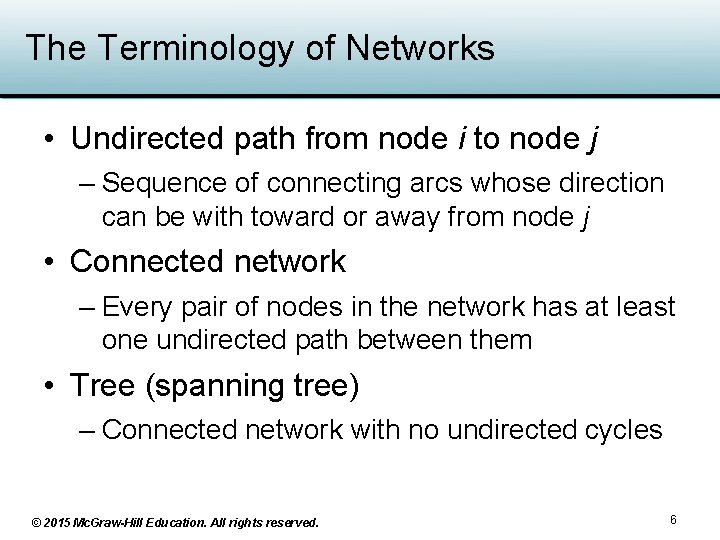
The Terminology of Networks • Undirected path from node i to node j – Sequence of connecting arcs whose direction can be with toward or away from node j • Connected network – Every pair of nodes in the network has at least one undirected path between them • Tree (spanning tree) – Connected network with no undirected cycles © 2015 Mc. Graw-Hill Education. All rights reserved. 6
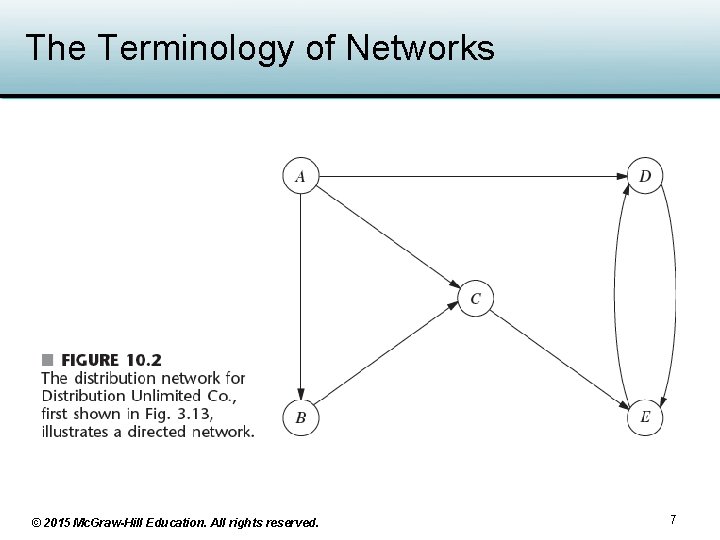
The Terminology of Networks © 2015 Mc. Graw-Hill Education. All rights reserved. 7
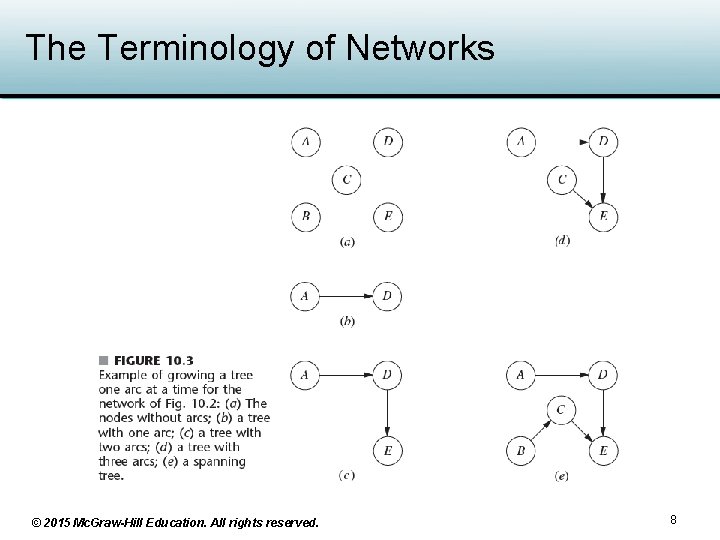
The Terminology of Networks © 2015 Mc. Graw-Hill Education. All rights reserved. 8
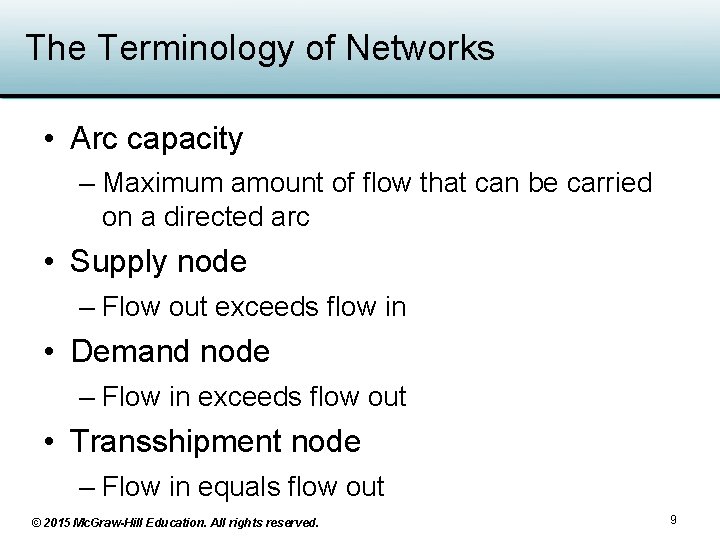
The Terminology of Networks • Arc capacity – Maximum amount of flow that can be carried on a directed arc • Supply node – Flow out exceeds flow in • Demand node – Flow in exceeds flow out • Transshipment node – Flow in equals flow out © 2015 Mc. Graw-Hill Education. All rights reserved. 9
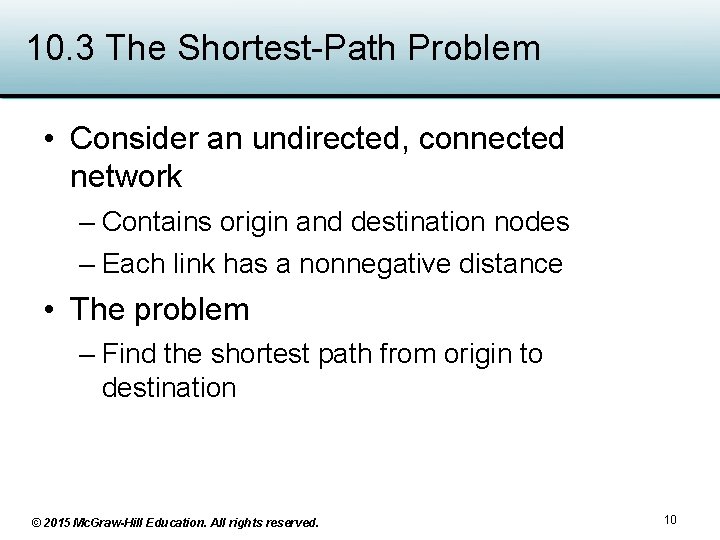
10. 3 The Shortest-Path Problem • Consider an undirected, connected network – Contains origin and destination nodes – Each link has a nonnegative distance • The problem – Find the shortest path from origin to destination © 2015 Mc. Graw-Hill Education. All rights reserved. 10
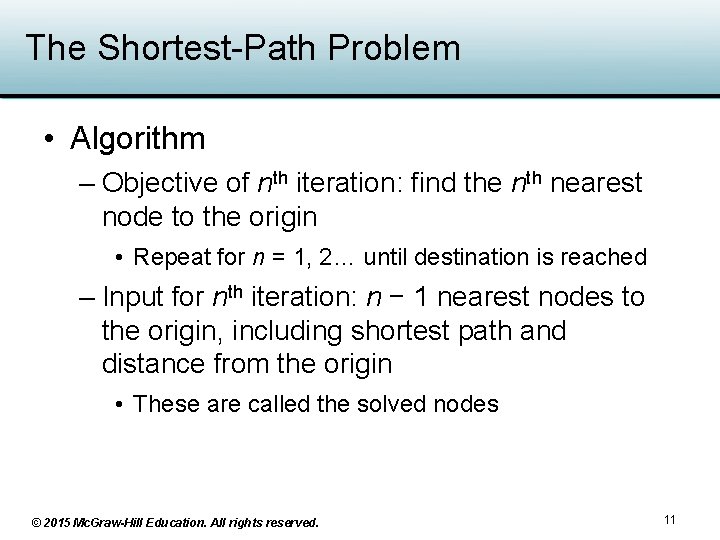
The Shortest-Path Problem • Algorithm – Objective of nth iteration: find the nth nearest node to the origin • Repeat for n = 1, 2… until destination is reached – Input for nth iteration: n − 1 nearest nodes to the origin, including shortest path and distance from the origin • These are called the solved nodes © 2015 Mc. Graw-Hill Education. All rights reserved. 11
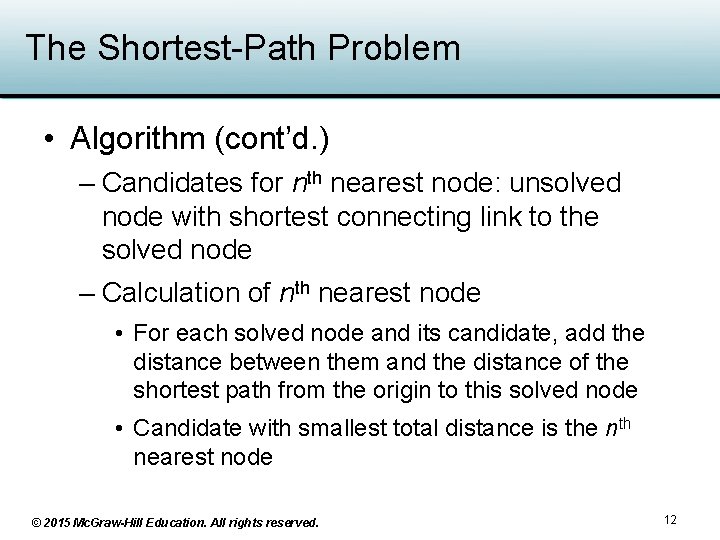
The Shortest-Path Problem • Algorithm (cont’d. ) – Candidates for nth nearest node: unsolved node with shortest connecting link to the solved node – Calculation of nth nearest node • For each solved node and its candidate, add the distance between them and the distance of the shortest path from the origin to this solved node • Candidate with smallest total distance is the nth nearest node © 2015 Mc. Graw-Hill Education. All rights reserved. 12
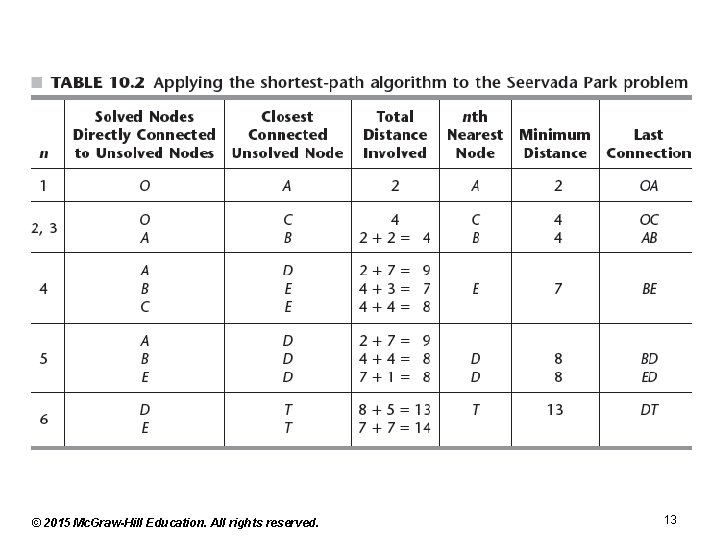
© 2015 Mc. Graw-Hill Education. All rights reserved. 13
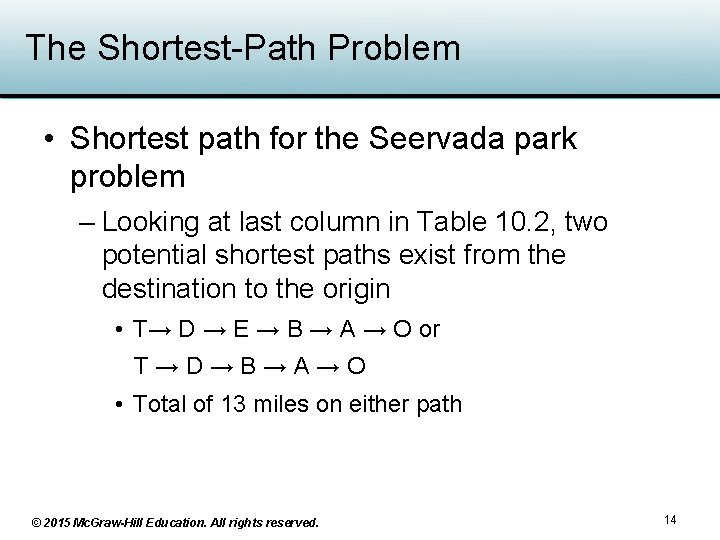
The Shortest-Path Problem • Shortest path for the Seervada park problem – Looking at last column in Table 10. 2, two potential shortest paths exist from the destination to the origin • T→ D → E → B → A → O or T→D→B→A→O • Total of 13 miles on either path © 2015 Mc. Graw-Hill Education. All rights reserved. 14
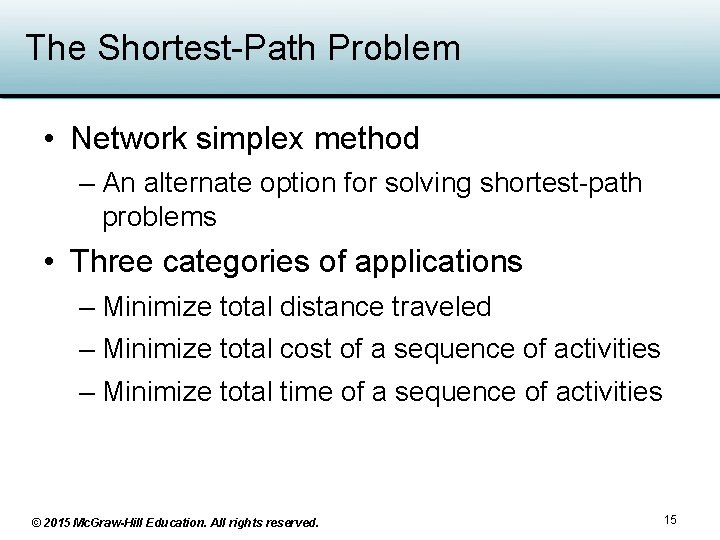
The Shortest-Path Problem • Network simplex method – An alternate option for solving shortest-path problems • Three categories of applications – Minimize total distance traveled – Minimize total cost of a sequence of activities – Minimize total time of a sequence of activities © 2015 Mc. Graw-Hill Education. All rights reserved. 15
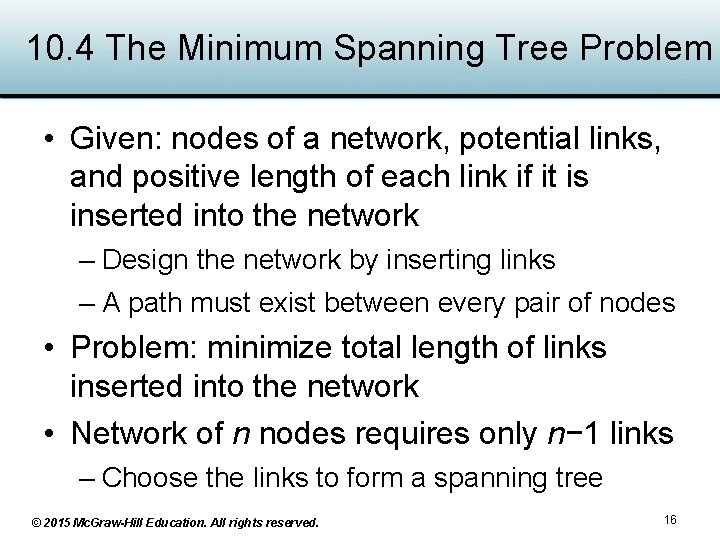
10. 4 The Minimum Spanning Tree Problem • Given: nodes of a network, potential links, and positive length of each link if it is inserted into the network – Design the network by inserting links – A path must exist between every pair of nodes • Problem: minimize total length of links inserted into the network • Network of n nodes requires only n− 1 links – Choose the links to form a spanning tree © 2015 Mc. Graw-Hill Education. All rights reserved. 16
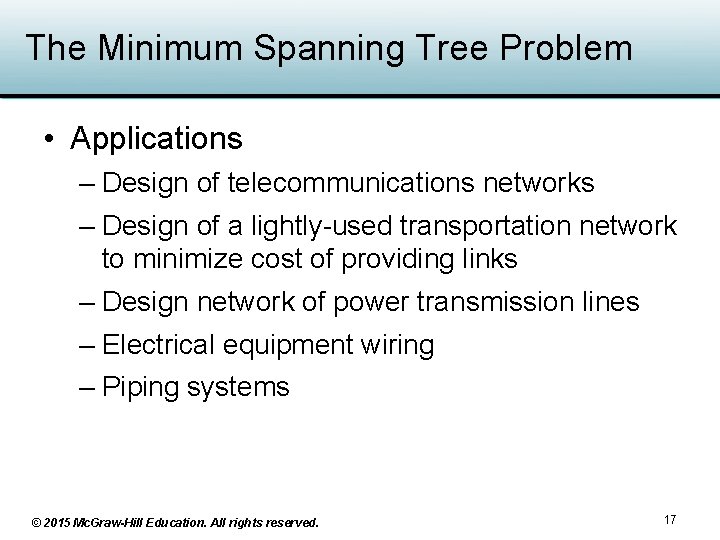
The Minimum Spanning Tree Problem • Applications – Design of telecommunications networks – Design of a lightly-used transportation network to minimize cost of providing links – Design network of power transmission lines – Electrical equipment wiring – Piping systems © 2015 Mc. Graw-Hill Education. All rights reserved. 17
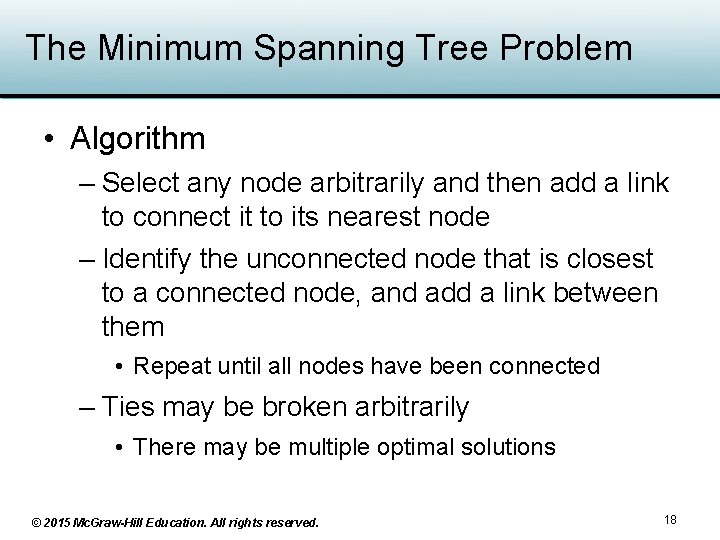
The Minimum Spanning Tree Problem • Algorithm – Select any node arbitrarily and then add a link to connect it to its nearest node – Identify the unconnected node that is closest to a connected node, and add a link between them • Repeat until all nodes have been connected – Ties may be broken arbitrarily • There may be multiple optimal solutions © 2015 Mc. Graw-Hill Education. All rights reserved. 18
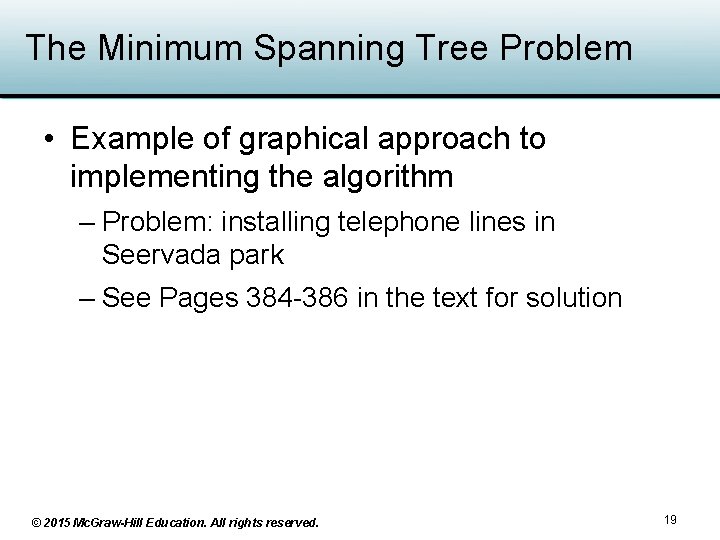
The Minimum Spanning Tree Problem • Example of graphical approach to implementing the algorithm – Problem: installing telephone lines in Seervada park – See Pages 384 -386 in the text for solution © 2015 Mc. Graw-Hill Education. All rights reserved. 19
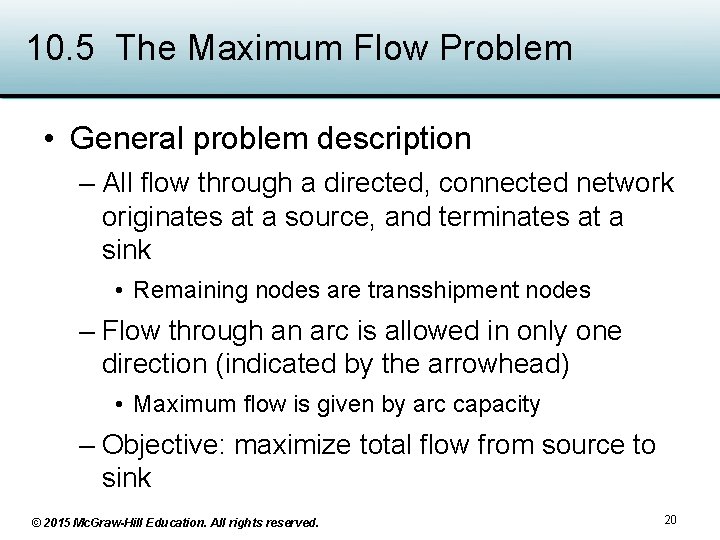
10. 5 The Maximum Flow Problem • General problem description – All flow through a directed, connected network originates at a source, and terminates at a sink • Remaining nodes are transshipment nodes – Flow through an arc is allowed in only one direction (indicated by the arrowhead) • Maximum flow is given by arc capacity – Objective: maximize total flow from source to sink © 2015 Mc. Graw-Hill Education. All rights reserved. 20
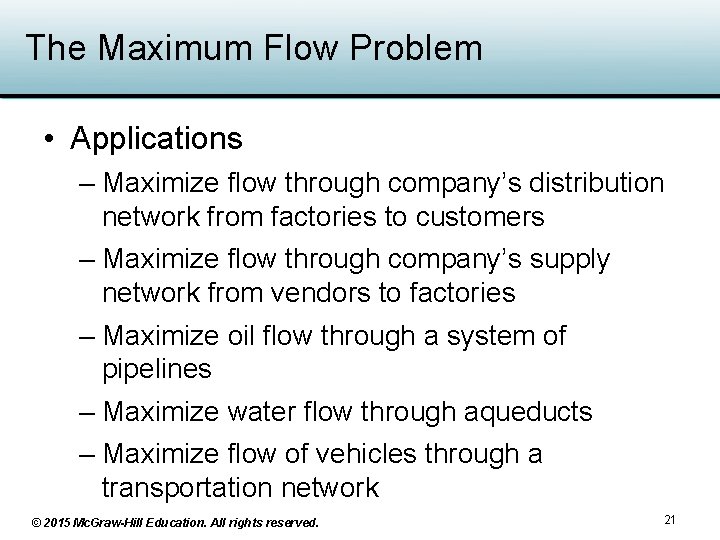
The Maximum Flow Problem • Applications – Maximize flow through company’s distribution network from factories to customers – Maximize flow through company’s supply network from vendors to factories – Maximize oil flow through a system of pipelines – Maximize water flow through aqueducts – Maximize flow of vehicles through a transportation network © 2015 Mc. Graw-Hill Education. All rights reserved. 21
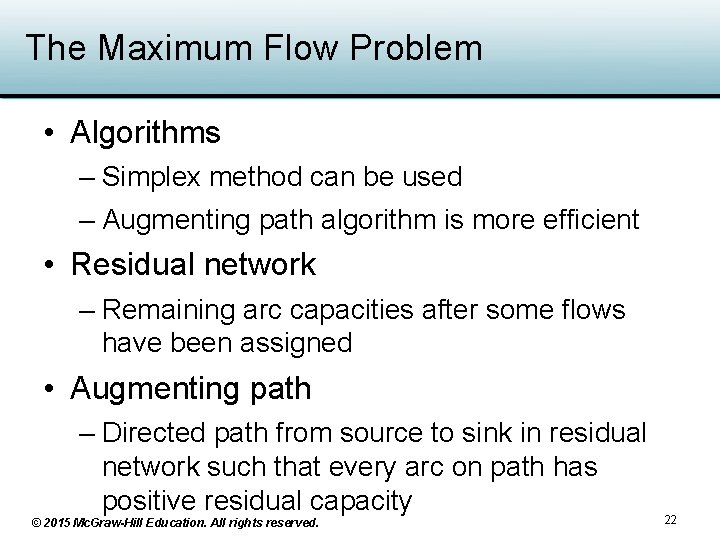
The Maximum Flow Problem • Algorithms – Simplex method can be used – Augmenting path algorithm is more efficient • Residual network – Remaining arc capacities after some flows have been assigned • Augmenting path – Directed path from source to sink in residual network such that every arc on path has positive residual capacity © 2015 Mc. Graw-Hill Education. All rights reserved. 22
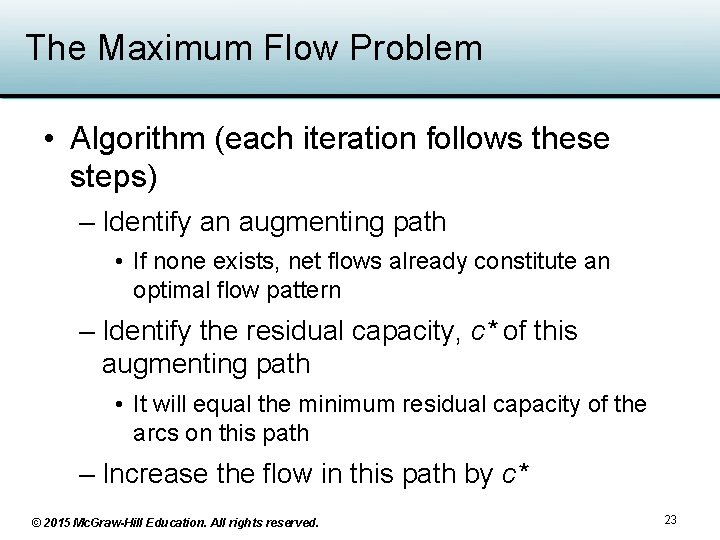
The Maximum Flow Problem • Algorithm (each iteration follows these steps) – Identify an augmenting path • If none exists, net flows already constitute an optimal flow pattern – Identify the residual capacity, c* of this augmenting path • It will equal the minimum residual capacity of the arcs on this path – Increase the flow in this path by c* © 2015 Mc. Graw-Hill Education. All rights reserved. 23
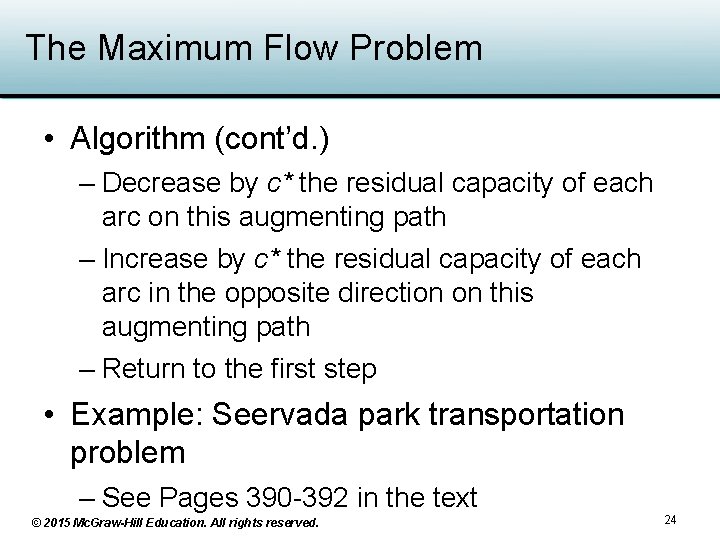
The Maximum Flow Problem • Algorithm (cont’d. ) – Decrease by c* the residual capacity of each arc on this augmenting path – Increase by c* the residual capacity of each arc in the opposite direction on this augmenting path – Return to the first step • Example: Seervada park transportation problem – See Pages 390 -392 in the text © 2015 Mc. Graw-Hill Education. All rights reserved. 24
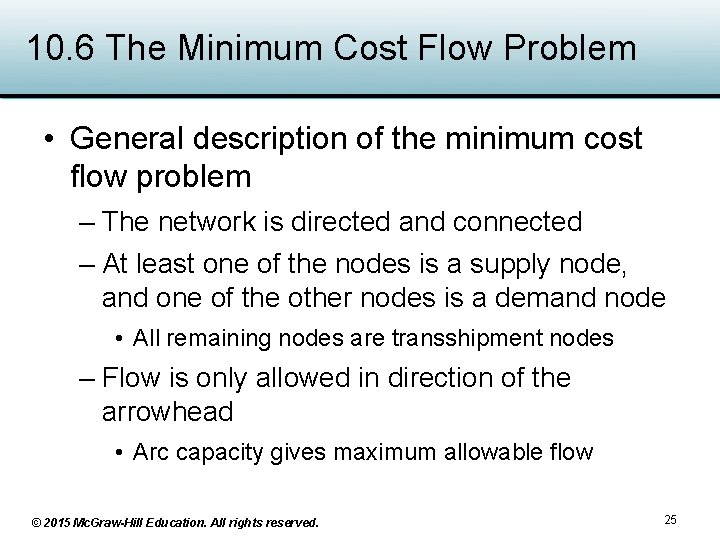
10. 6 The Minimum Cost Flow Problem • General description of the minimum cost flow problem – The network is directed and connected – At least one of the nodes is a supply node, and one of the other nodes is a demand node • All remaining nodes are transshipment nodes – Flow is only allowed in direction of the arrowhead • Arc capacity gives maximum allowable flow © 2015 Mc. Graw-Hill Education. All rights reserved. 25
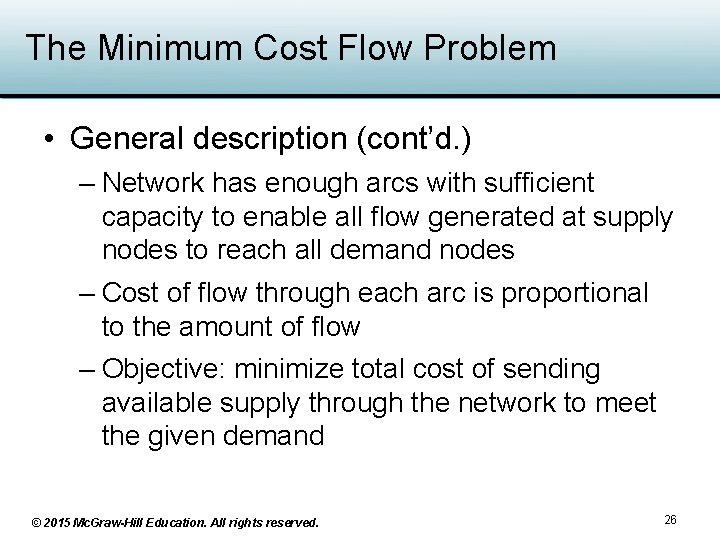
The Minimum Cost Flow Problem • General description (cont’d. ) – Network has enough arcs with sufficient capacity to enable all flow generated at supply nodes to reach all demand nodes – Cost of flow through each arc is proportional to the amount of flow – Objective: minimize total cost of sending available supply through the network to meet the given demand © 2015 Mc. Graw-Hill Education. All rights reserved. 26
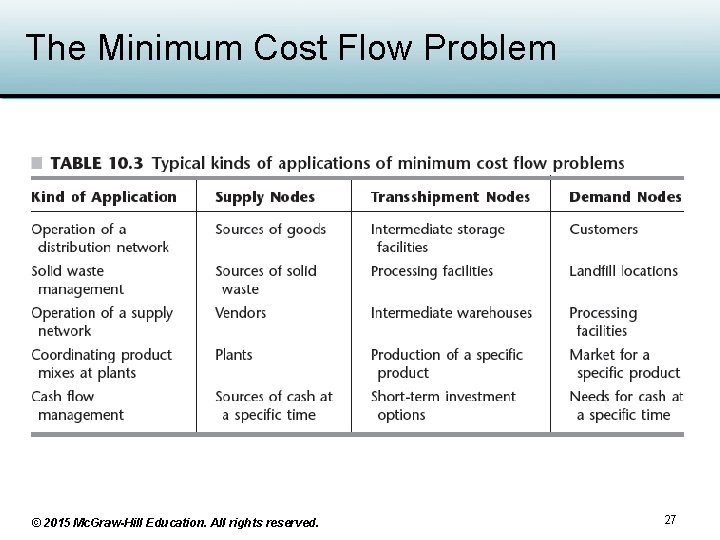
The Minimum Cost Flow Problem © 2015 Mc. Graw-Hill Education. All rights reserved. 27
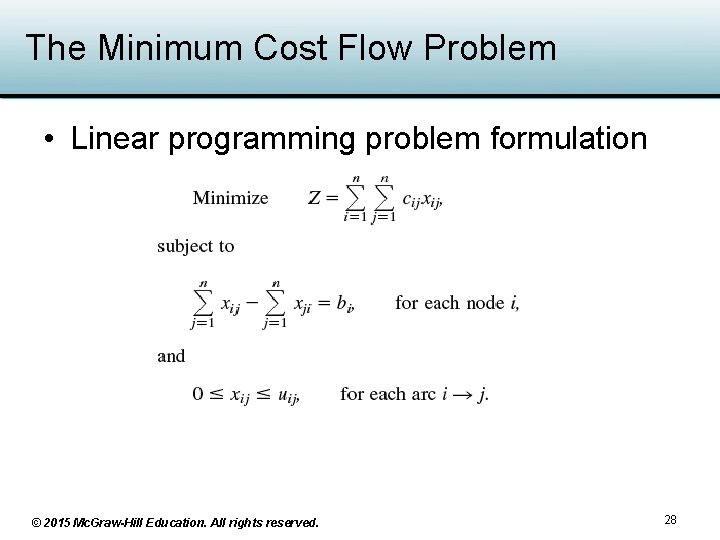
The Minimum Cost Flow Problem • Linear programming problem formulation © 2015 Mc. Graw-Hill Education. All rights reserved. 28
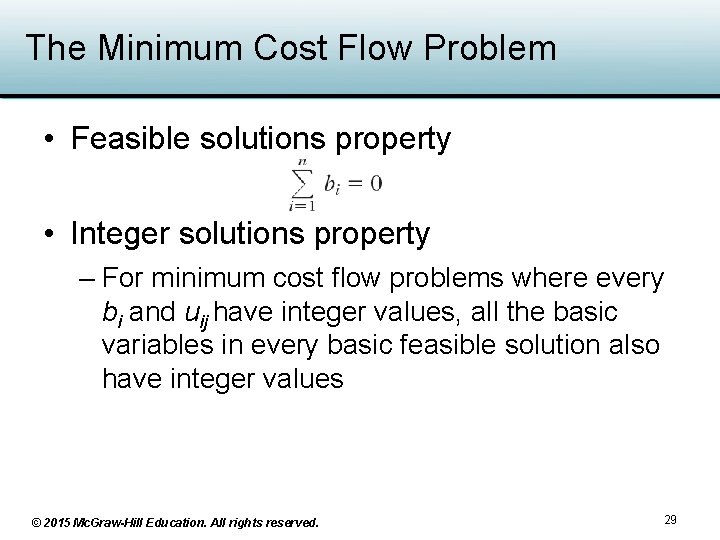
The Minimum Cost Flow Problem • Feasible solutions property • Integer solutions property – For minimum cost flow problems where every bi and uij have integer values, all the basic variables in every basic feasible solution also have integer values © 2015 Mc. Graw-Hill Education. All rights reserved. 29
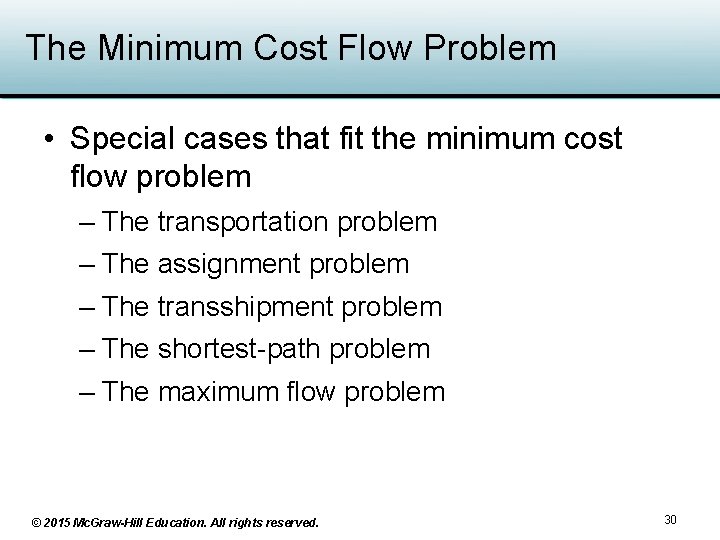
The Minimum Cost Flow Problem • Special cases that fit the minimum cost flow problem – The transportation problem – The assignment problem – The transshipment problem – The shortest-path problem – The maximum flow problem © 2015 Mc. Graw-Hill Education. All rights reserved. 30
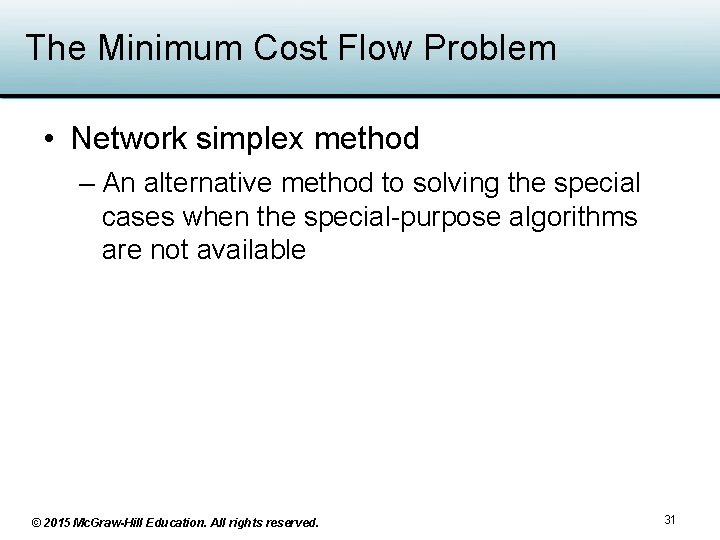
The Minimum Cost Flow Problem • Network simplex method – An alternative method to solving the special cases when the special-purpose algorithms are not available © 2015 Mc. Graw-Hill Education. All rights reserved. 31
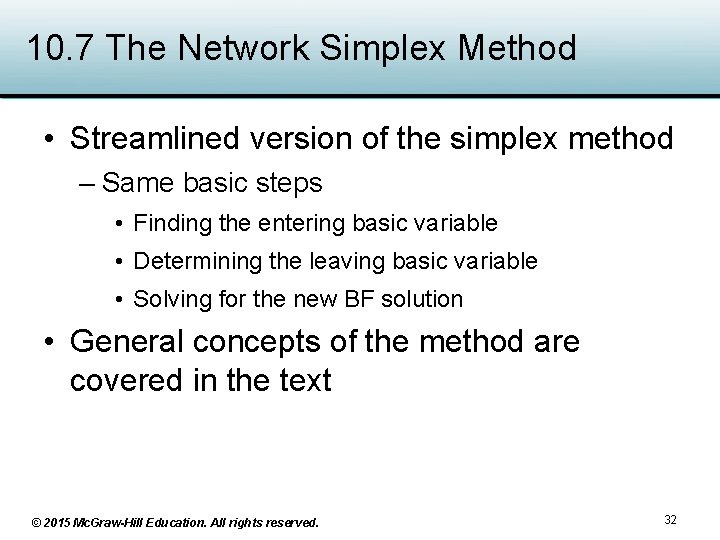
10. 7 The Network Simplex Method • Streamlined version of the simplex method – Same basic steps • Finding the entering basic variable • Determining the leaving basic variable • Solving for the new BF solution • General concepts of the method are covered in the text © 2015 Mc. Graw-Hill Education. All rights reserved. 32
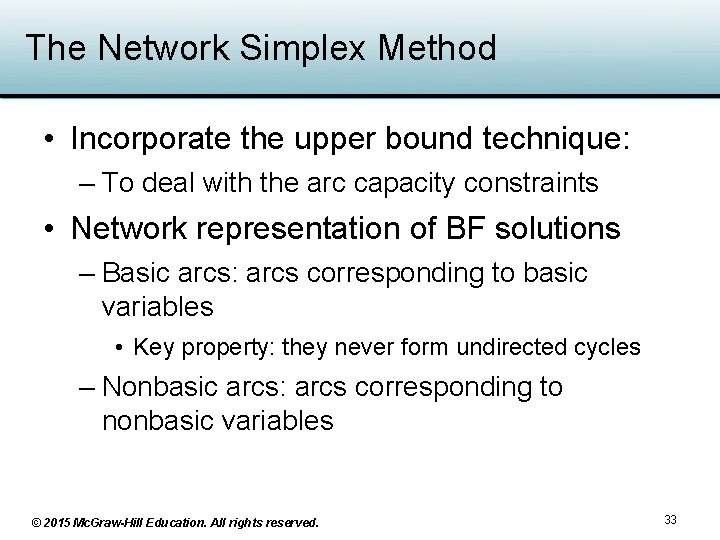
The Network Simplex Method • Incorporate the upper bound technique: – To deal with the arc capacity constraints • Network representation of BF solutions – Basic arcs: arcs corresponding to basic variables • Key property: they never form undirected cycles – Nonbasic arcs: arcs corresponding to nonbasic variables © 2015 Mc. Graw-Hill Education. All rights reserved. 33
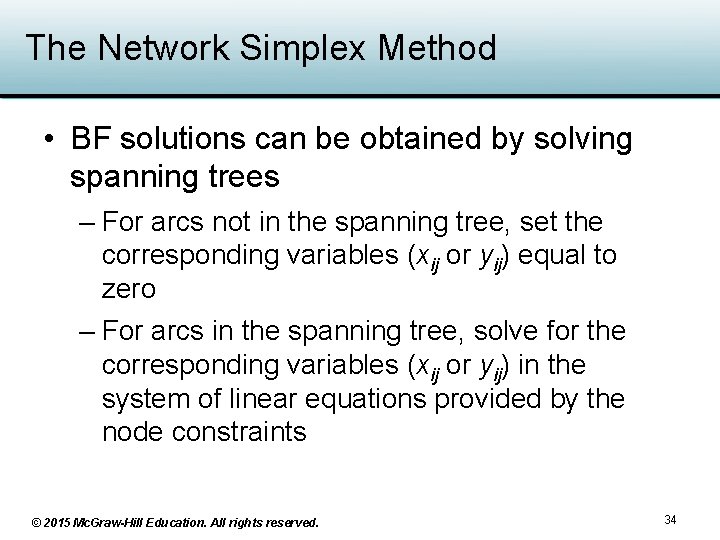
The Network Simplex Method • BF solutions can be obtained by solving spanning trees – For arcs not in the spanning tree, set the corresponding variables (xij or yij) equal to zero – For arcs in the spanning tree, solve for the corresponding variables (xij or yij) in the system of linear equations provided by the node constraints © 2015 Mc. Graw-Hill Education. All rights reserved. 34
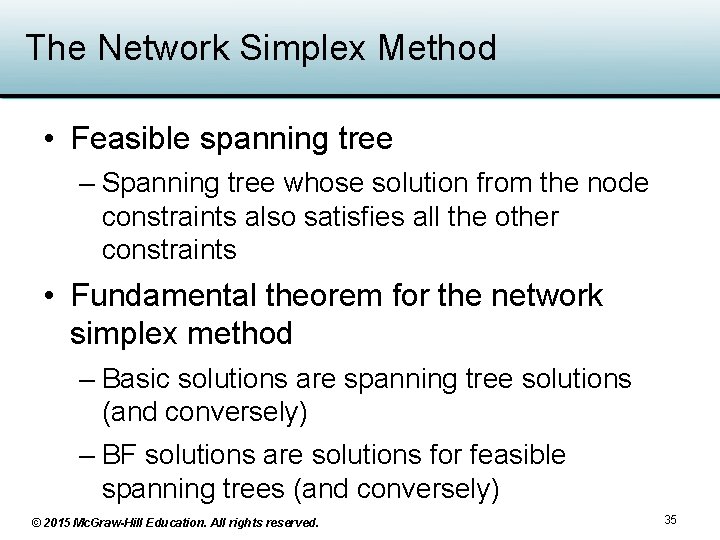
The Network Simplex Method • Feasible spanning tree – Spanning tree whose solution from the node constraints also satisfies all the other constraints • Fundamental theorem for the network simplex method – Basic solutions are spanning tree solutions (and conversely) – BF solutions are solutions for feasible spanning trees (and conversely) © 2015 Mc. Graw-Hill Education. All rights reserved. 35
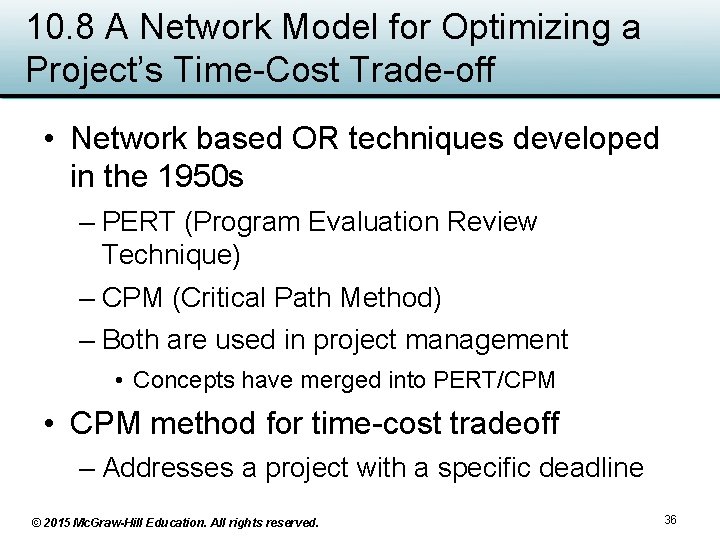
10. 8 A Network Model for Optimizing a Project’s Time-Cost Trade-off • Network based OR techniques developed in the 1950 s – PERT (Program Evaluation Review Technique) – CPM (Critical Path Method) – Both are used in project management • Concepts have merged into PERT/CPM • CPM method for time-cost tradeoff – Addresses a project with a specific deadline © 2015 Mc. Graw-Hill Education. All rights reserved. 36
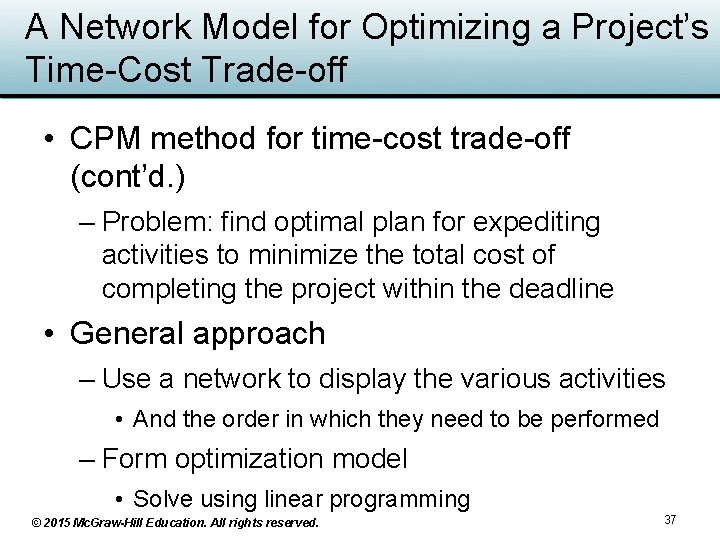
A Network Model for Optimizing a Project’s Time-Cost Trade-off • CPM method for time-cost trade-off (cont’d. ) – Problem: find optimal plan for expediting activities to minimize the total cost of completing the project within the deadline • General approach – Use a network to display the various activities • And the order in which they need to be performed – Form optimization model • Solve using linear programming © 2015 Mc. Graw-Hill Education. All rights reserved. 37
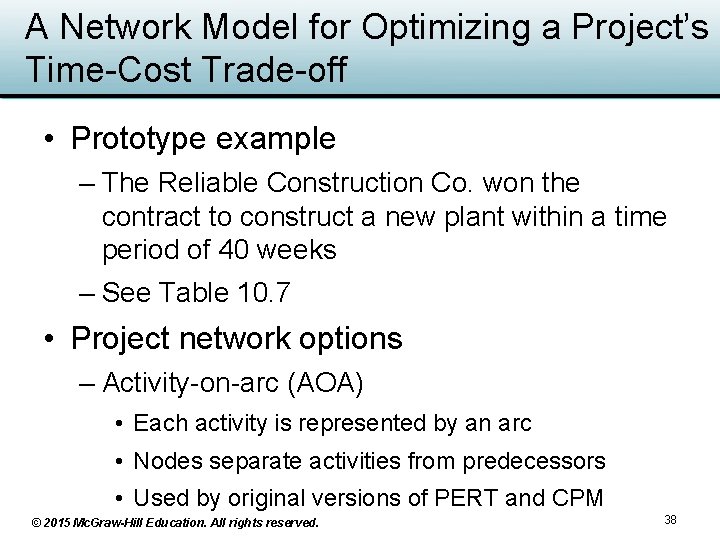
A Network Model for Optimizing a Project’s Time-Cost Trade-off • Prototype example – The Reliable Construction Co. won the contract to construct a new plant within a time period of 40 weeks – See Table 10. 7 • Project network options – Activity-on-arc (AOA) • Each activity is represented by an arc • Nodes separate activities from predecessors • Used by original versions of PERT and CPM © 2015 Mc. Graw-Hill Education. All rights reserved. 38
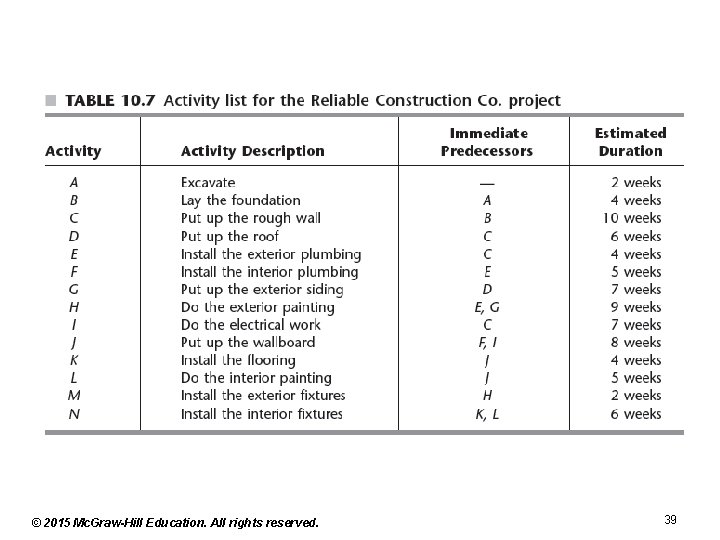
© 2015 Mc. Graw-Hill Education. All rights reserved. 39
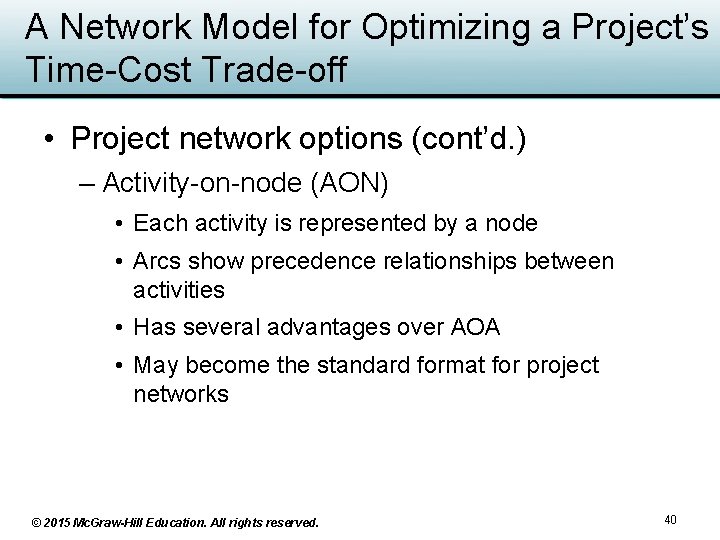
A Network Model for Optimizing a Project’s Time-Cost Trade-off • Project network options (cont’d. ) – Activity-on-node (AON) • Each activity is represented by a node • Arcs show precedence relationships between activities • Has several advantages over AOA • May become the standard format for project networks © 2015 Mc. Graw-Hill Education. All rights reserved. 40
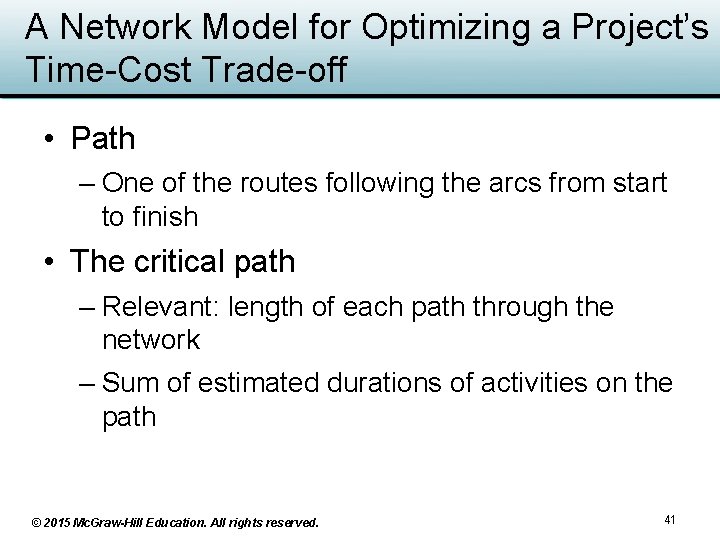
A Network Model for Optimizing a Project’s Time-Cost Trade-off • Path – One of the routes following the arcs from start to finish • The critical path – Relevant: length of each path through the network – Sum of estimated durations of activities on the path © 2015 Mc. Graw-Hill Education. All rights reserved. 41
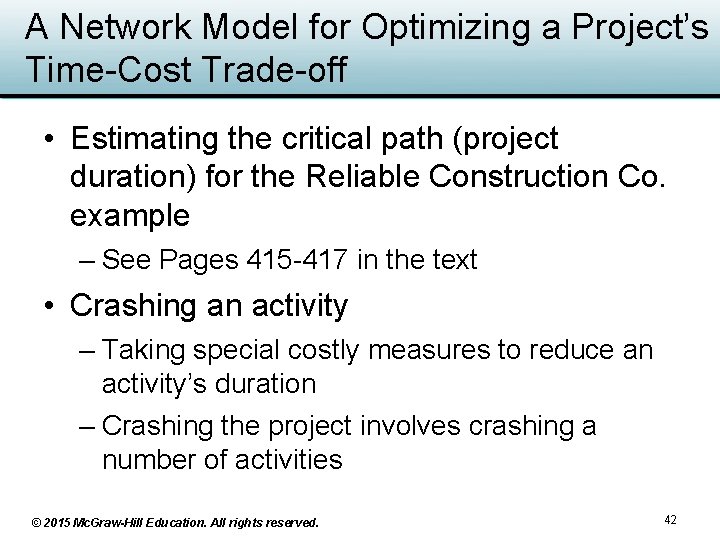
A Network Model for Optimizing a Project’s Time-Cost Trade-off • Estimating the critical path (project duration) for the Reliable Construction Co. example – See Pages 415 -417 in the text • Crashing an activity – Taking special costly measures to reduce an activity’s duration – Crashing the project involves crashing a number of activities © 2015 Mc. Graw-Hill Education. All rights reserved. 42
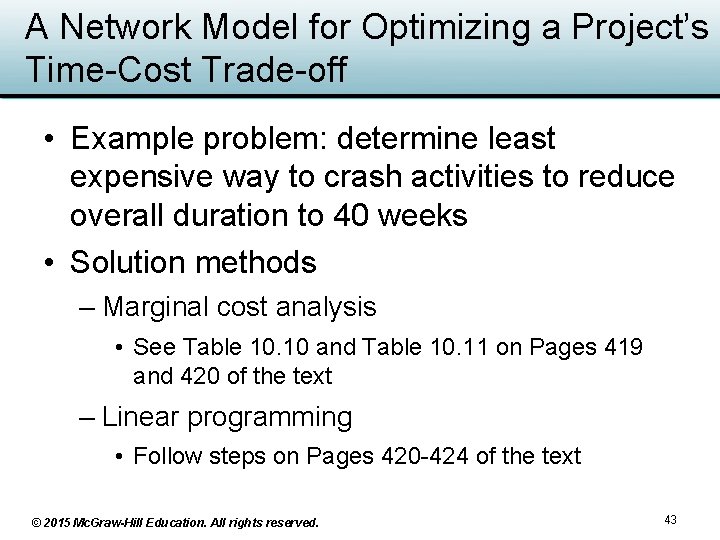
A Network Model for Optimizing a Project’s Time-Cost Trade-off • Example problem: determine least expensive way to crash activities to reduce overall duration to 40 weeks • Solution methods – Marginal cost analysis • See Table 10. 10 and Table 10. 11 on Pages 419 and 420 of the text – Linear programming • Follow steps on Pages 420 -424 of the text © 2015 Mc. Graw-Hill Education. All rights reserved. 43
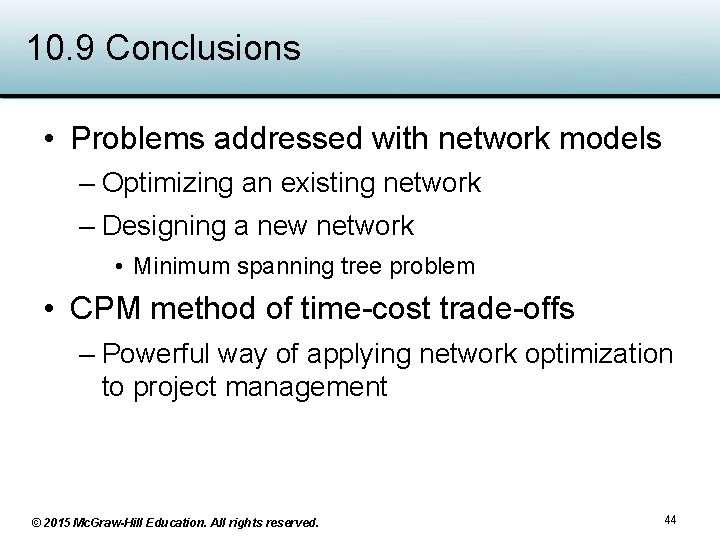
10. 9 Conclusions • Problems addressed with network models – Optimizing an existing network – Designing a new network • Minimum spanning tree problem • CPM method of time-cost trade-offs – Powerful way of applying network optimization to project management © 2015 Mc. Graw-Hill Education. All rights reserved. 44
Grawhill
Grawhill
Grawhill
Grawhill
Mc graw hill
Single user multitasking os
Grawhill
Mc grawhill
Grawhill
Network design and optimization
Network configuration optimization analysis
Mail network optimization project
What is the difference between models & semi modals?
Distribution and network models
Iso networking standards
Explain the concept of layered task
Differences between virtual circuits and datagram networks
What is topology in computer
Features of peer to peer network and client server network
Ece 526
Network centric computing
Advantages and disadvantages of circuit switching
Chapter 9 transportation and assignment models solutions
Chapter 17 sampling distribution models
Ap stats chapter 17 sampling distribution models
Ap stats chapter 17 sampling distribution models
Chapter 1 graphs functions and models answers
Chapter 17 probability models
Shape optimization ansys
Instrument processing optimization
Constrained and unconstrained optimization in economics
Bin collection optimization
Global optimization matlab
Divbar
Sequential model based optimization
Seo off page optimization tutorial
Trellian keyword discovery tool
Language
Sas marketing optimization
Wan optimization gartner 2014
Optimal value
Qp formula
Mainframe performance optimization
Relative vs absolute max and min
Optimization problems