Using Structural Equation Modeling to Analyze Monitoring Data
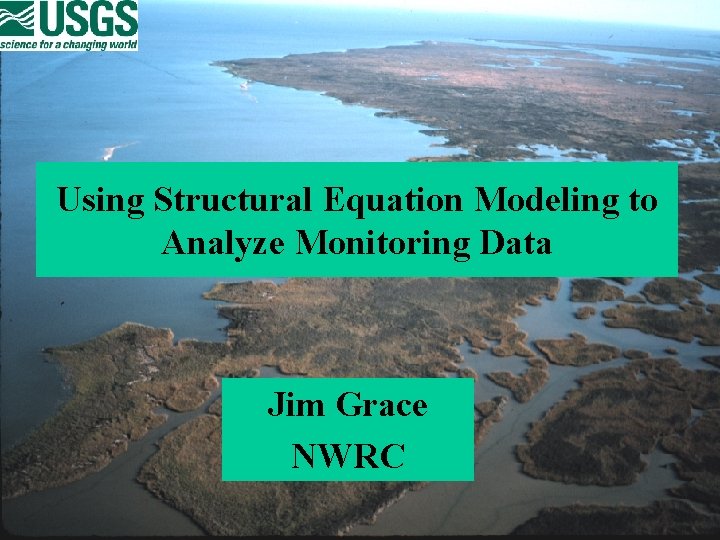
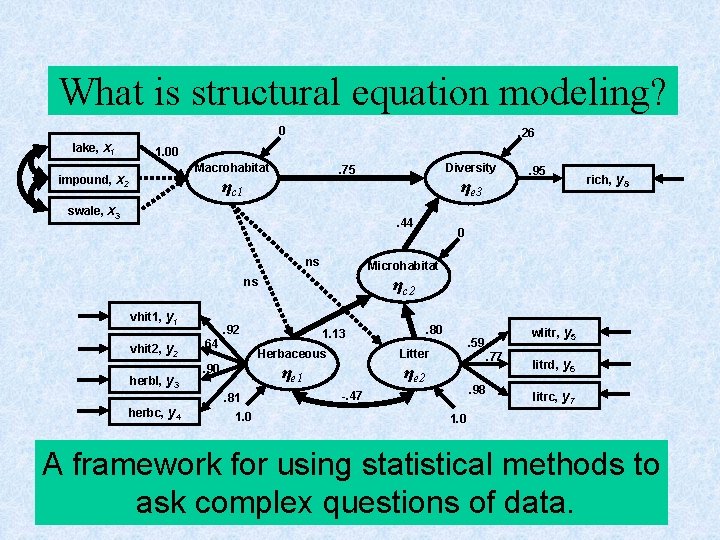
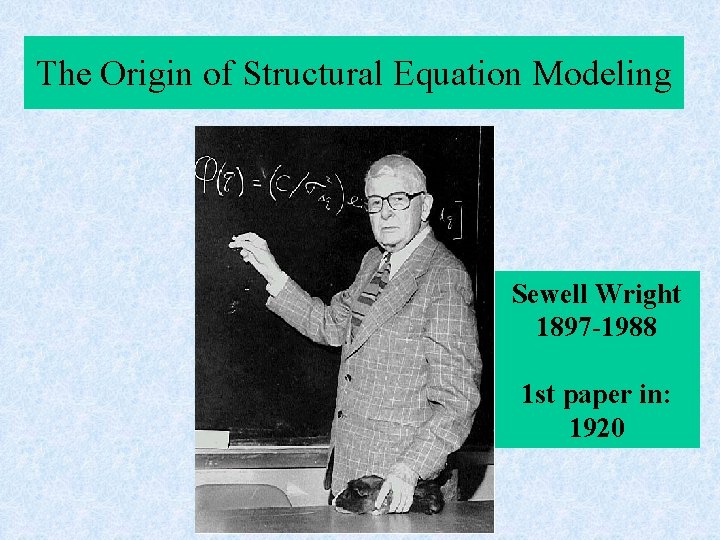
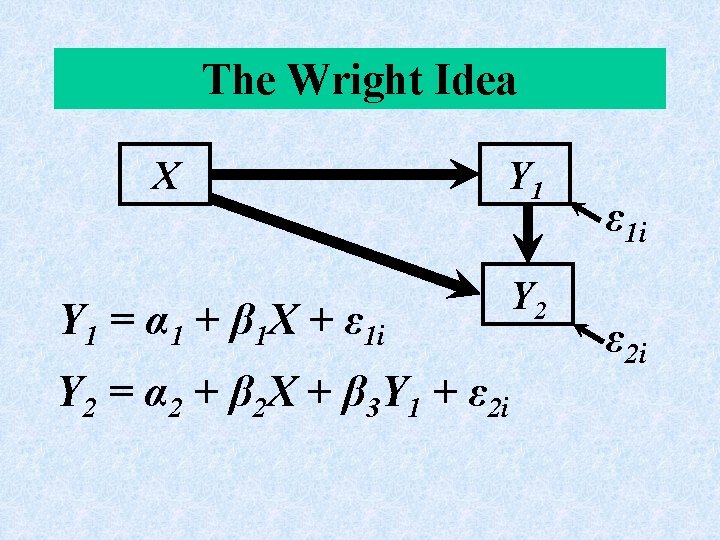
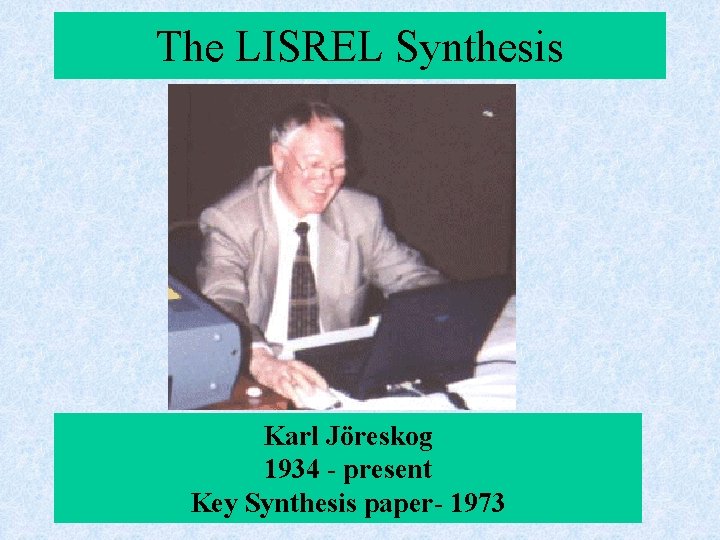
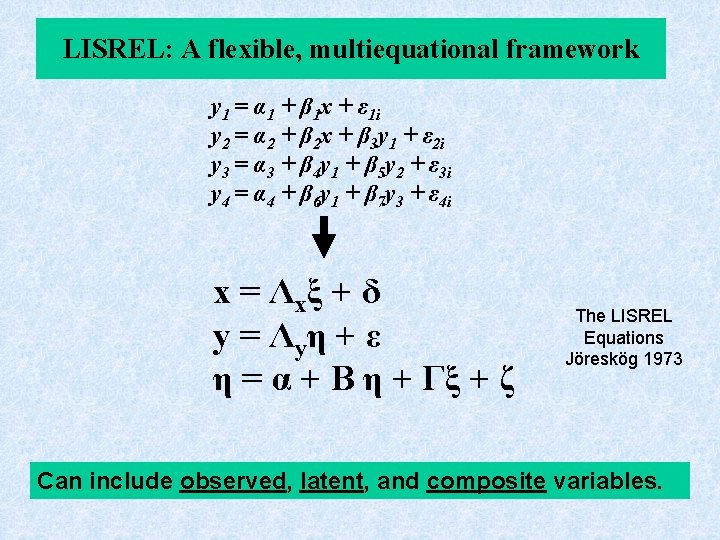
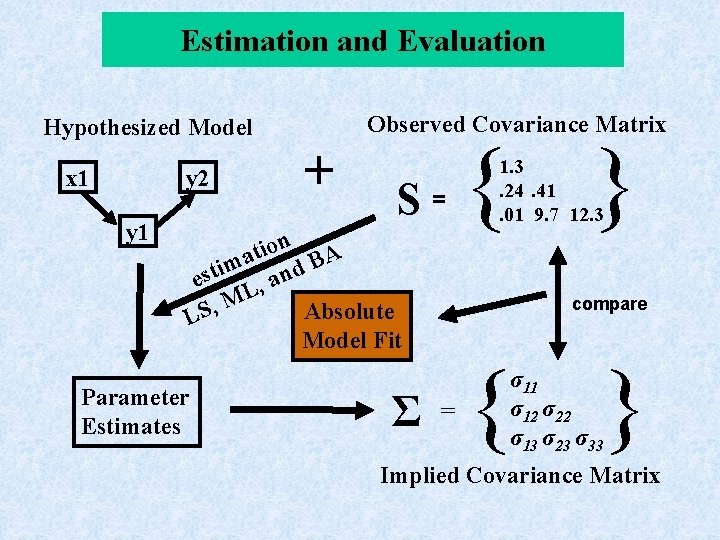
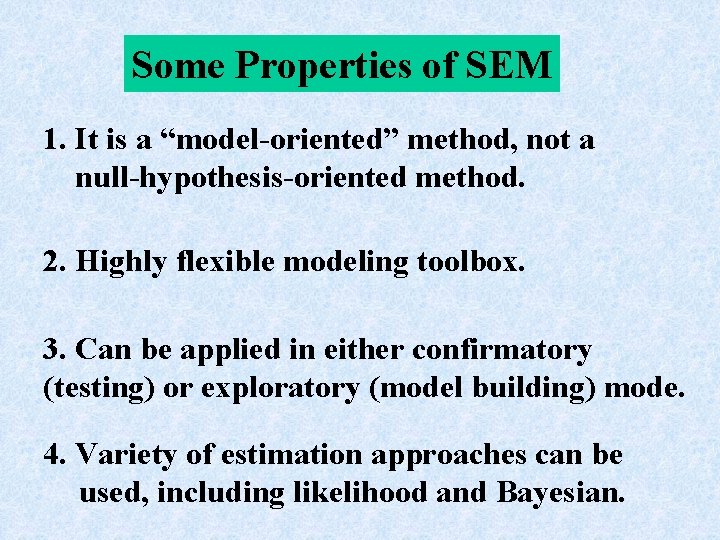
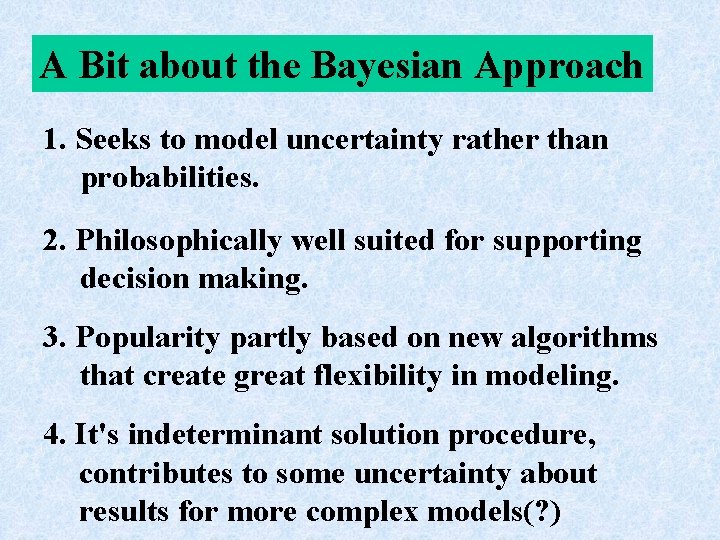
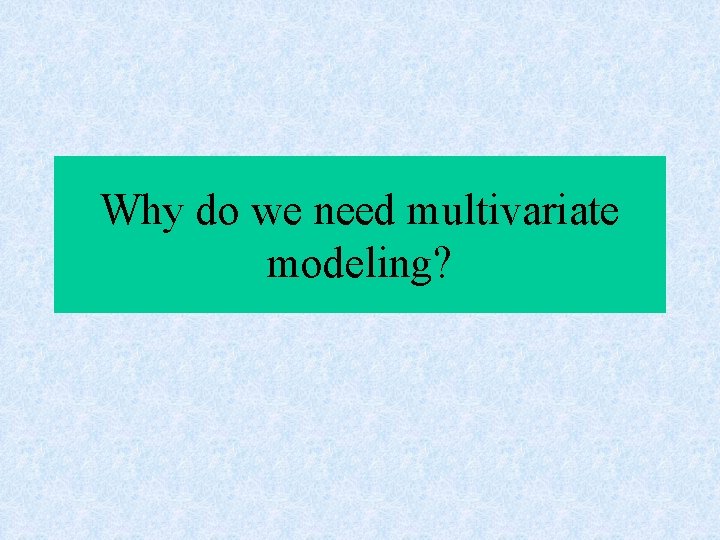
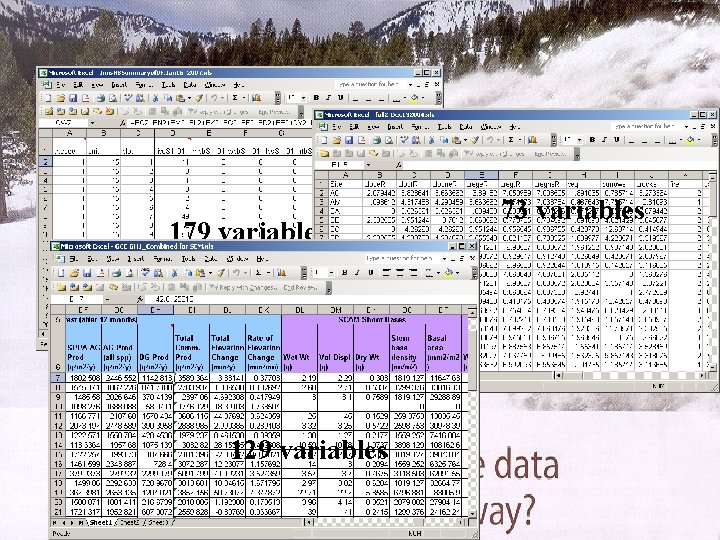
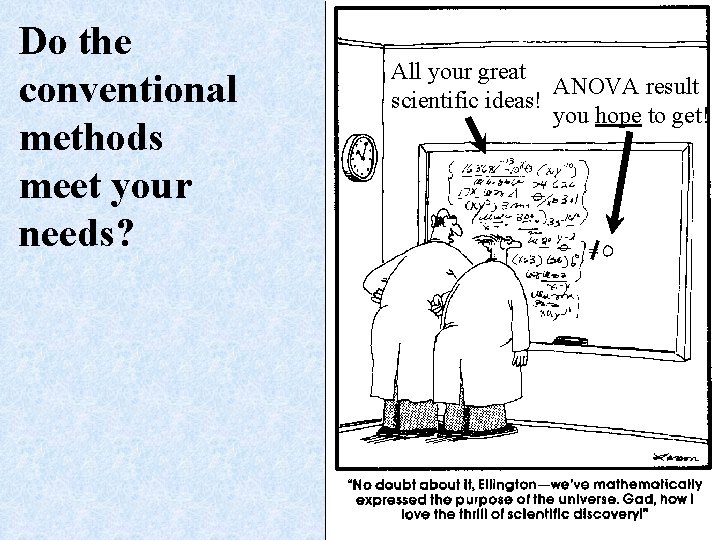
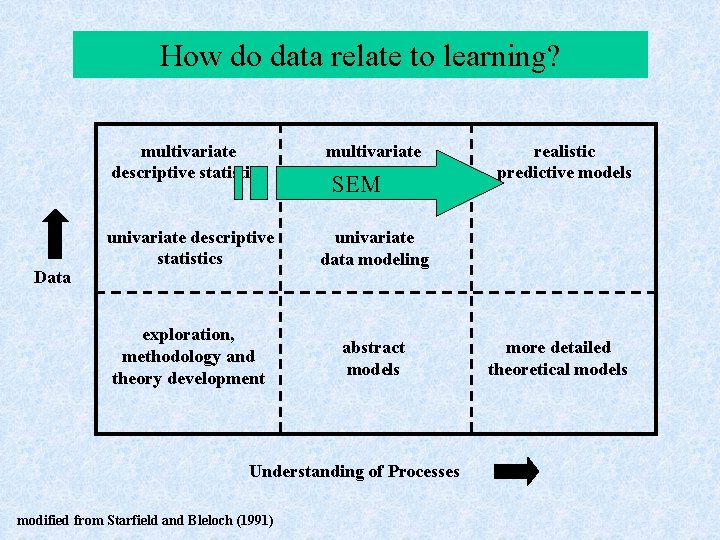
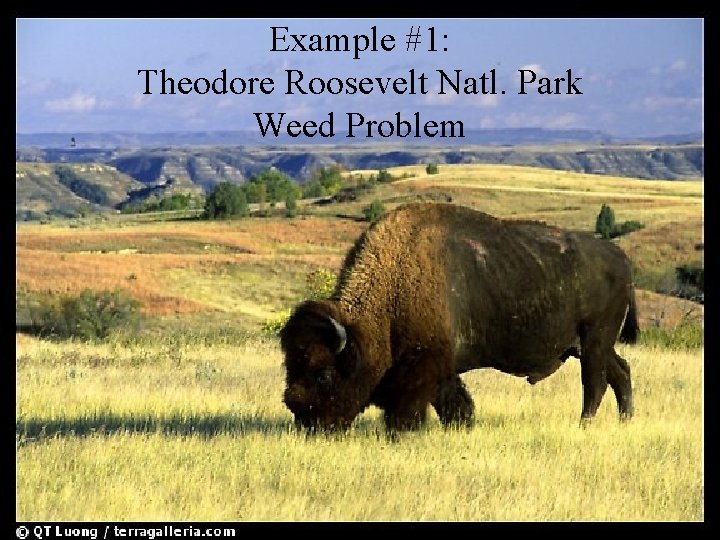
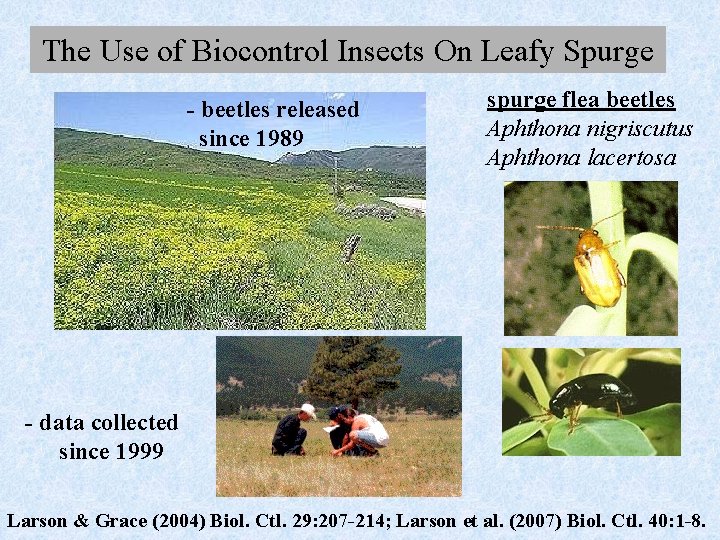
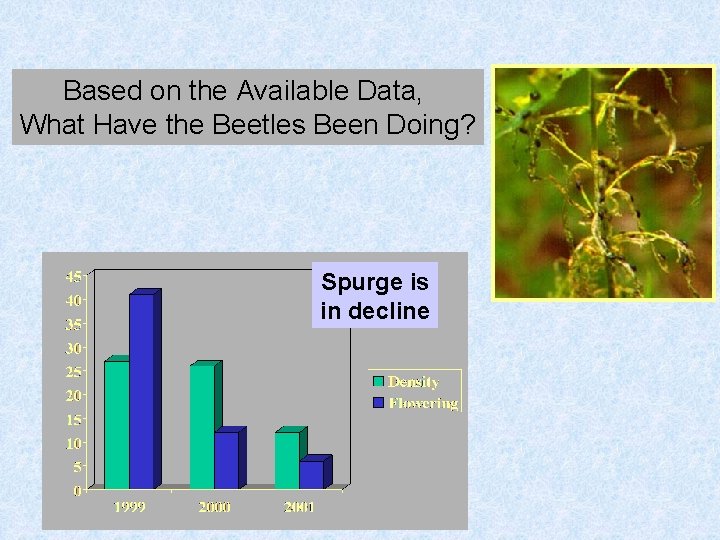
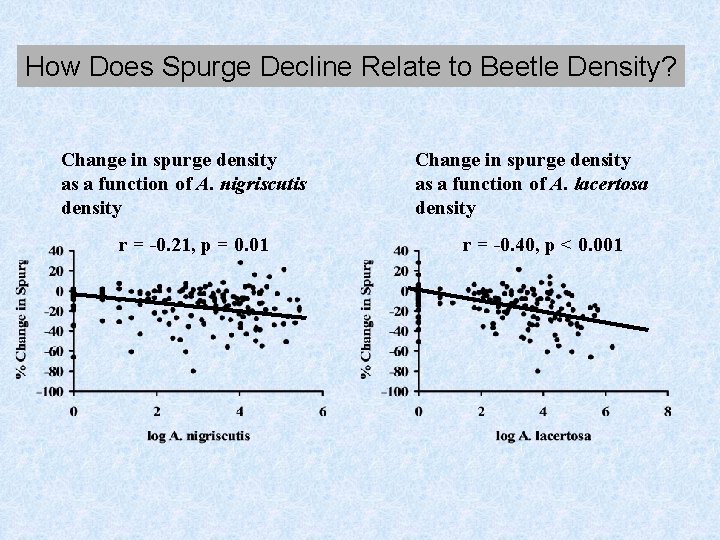
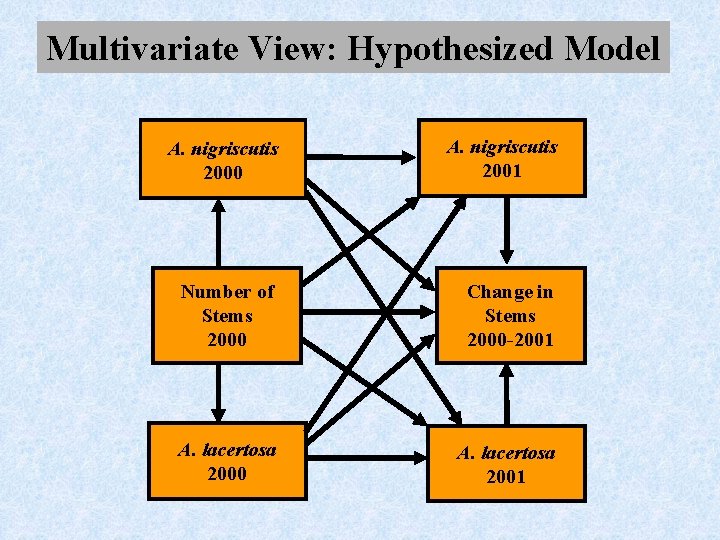
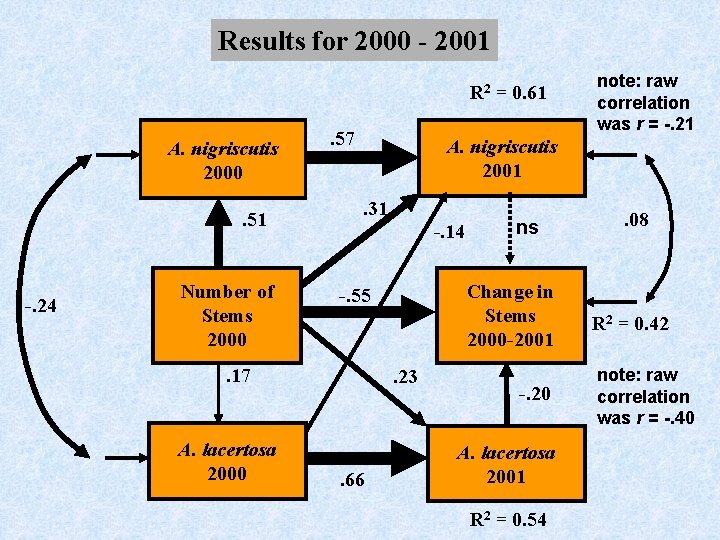
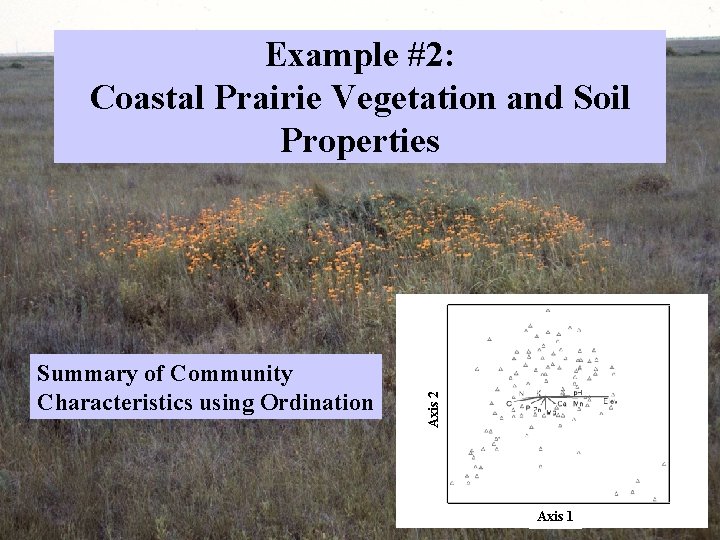
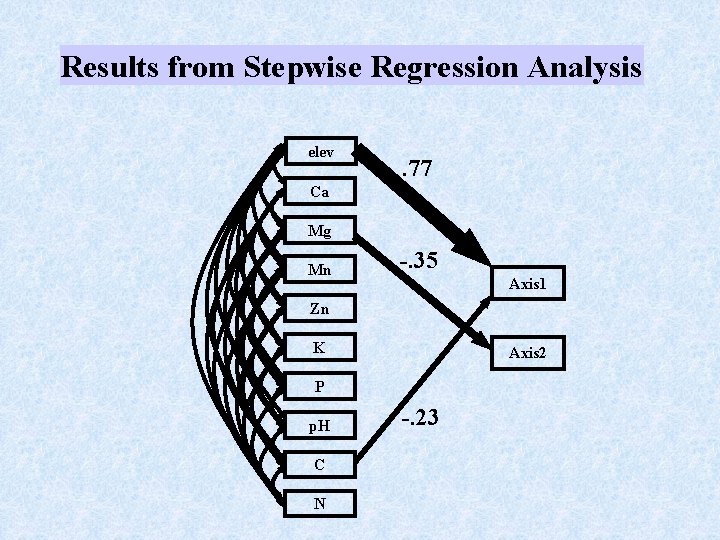
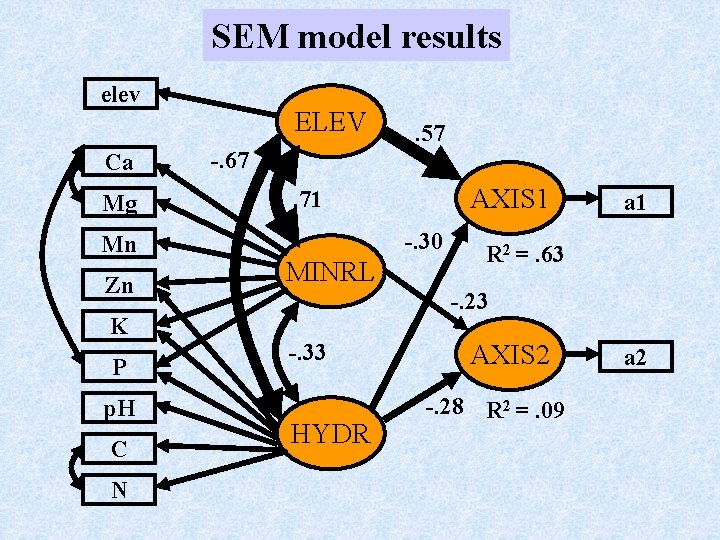
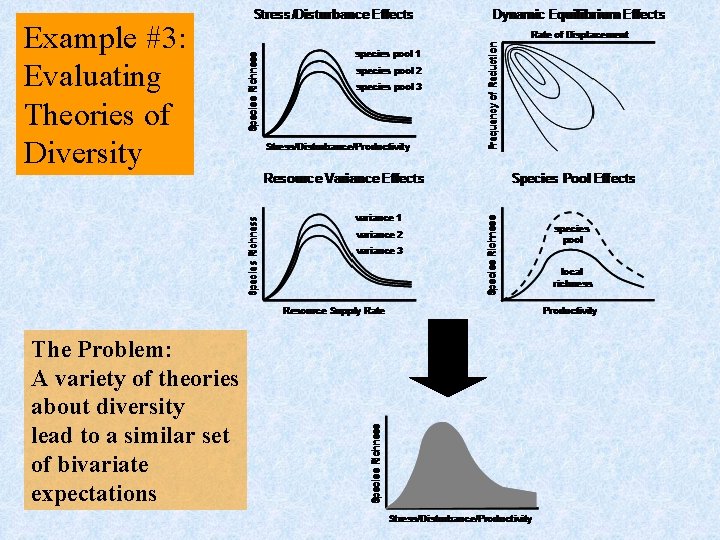
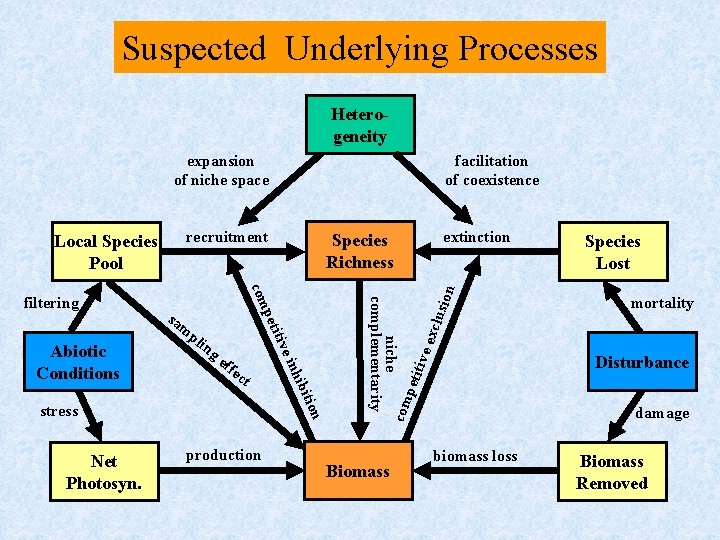
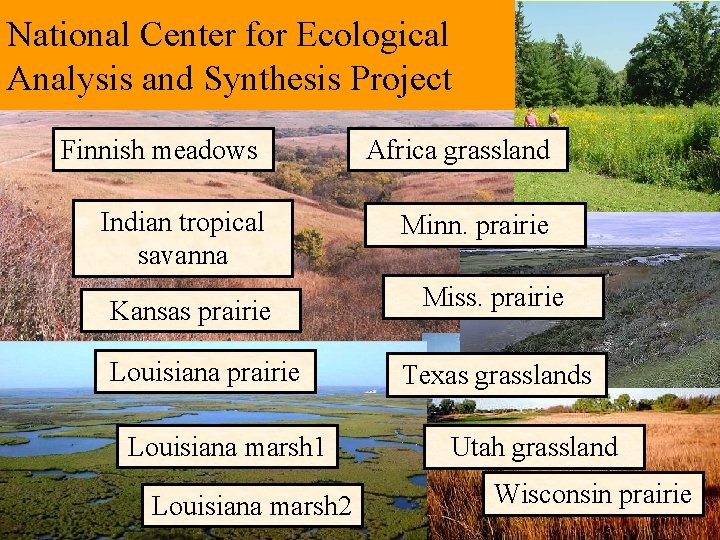
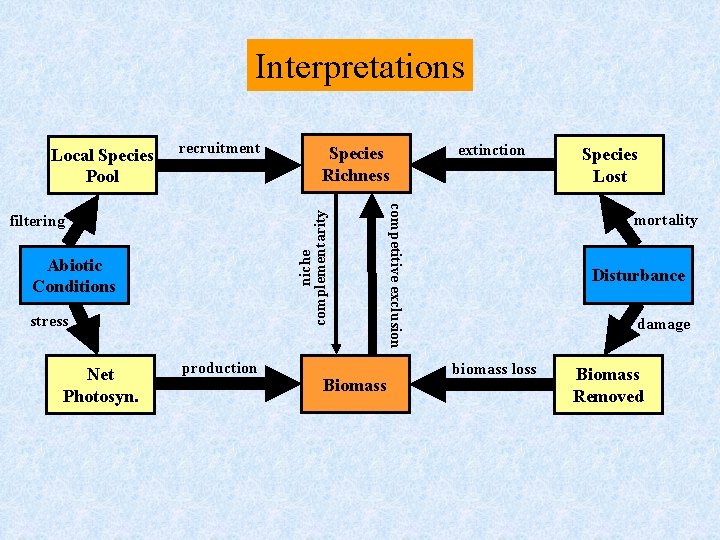
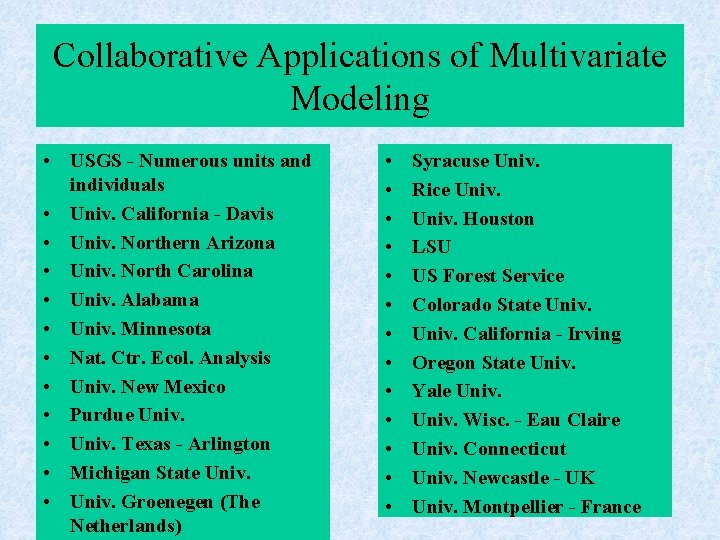
- Slides: 27
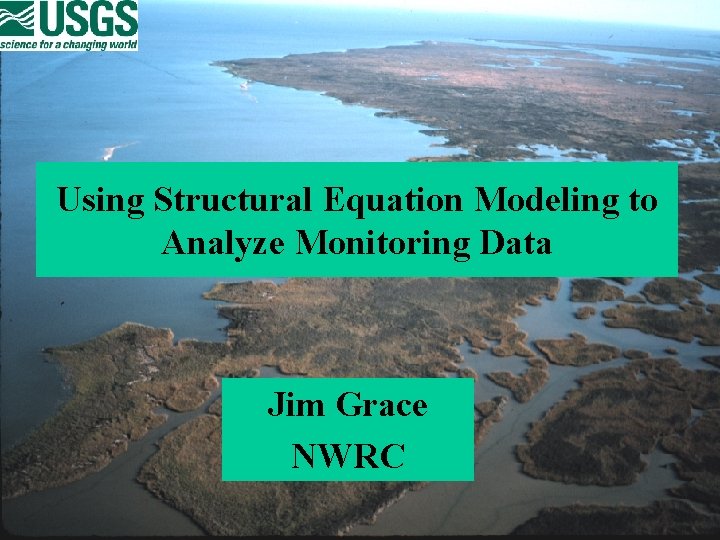
Using Structural Equation Modeling to Analyze Monitoring Data Jim Grace NWRC
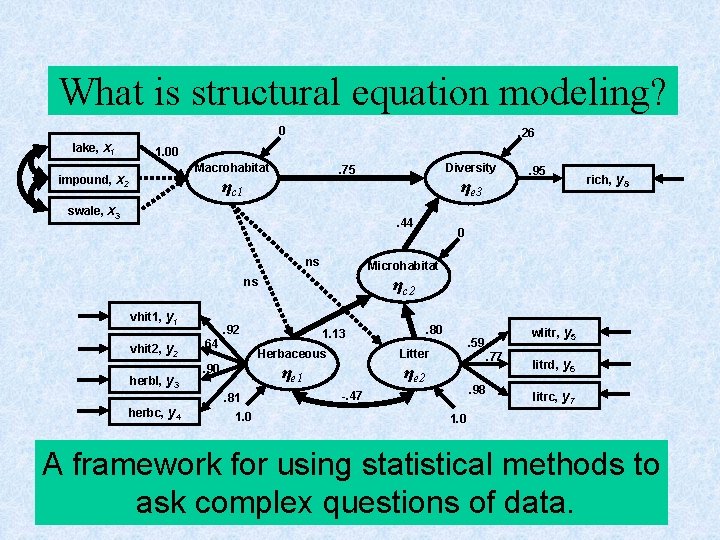
What is structural equation modeling? 0 lake, x 1 . 26 1. 00 Macrohabitat impound, x 2 Diversity . 75 ηc 1 swale, x 3 ηe 3. 44 ns vhit 2, y 2 herbl, y 3 herbc, y 4 . 90. 81 1. 0 0 ηc 2 . 92. 64 rich, y 8 Microhabitat ns vhit 1, y 1 . 95 . 80 1. 13 Herbaceous Litter ηe 1 ηe 2 wlitr, y 5 . 59. 77. 98 -. 47 litrd, y 6 litrc, y 7 1. 0 A framework for using statistical methods to ask complex questions of data.
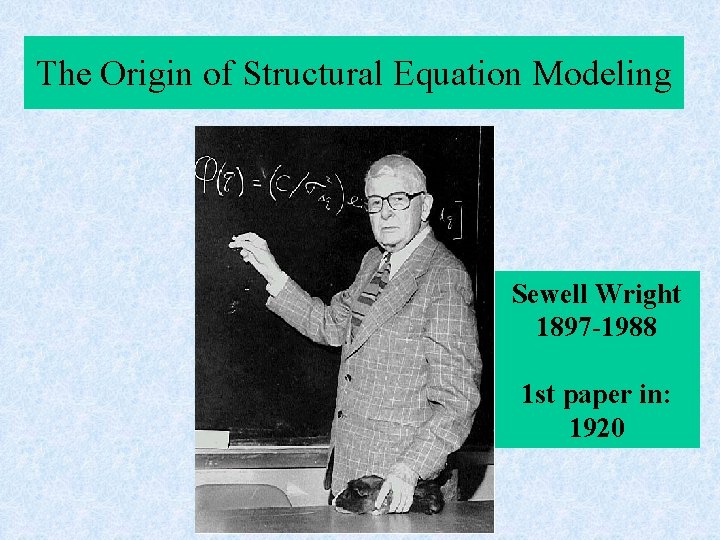
The Origin of Structural Equation Modeling Sewell Wright 1897 -1988 1 st paper in: 1920
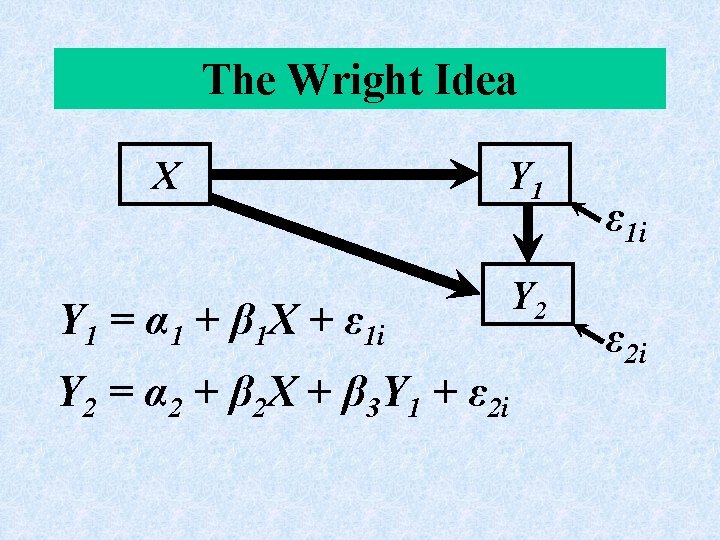
The Wright Idea X Y 1 = α 1 + β 1 X + ε 1 i Y 2 = α 2 + β 2 X + β 3 Y 1 + ε 2 i Y 2 ε 1 i ε 2 i
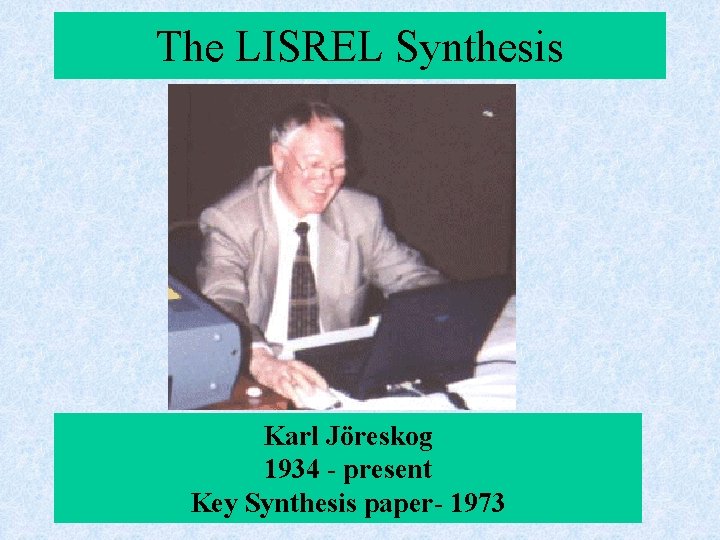
The LISREL Synthesis Karl Jöreskog 1934 - present Key Synthesis paper- 1973
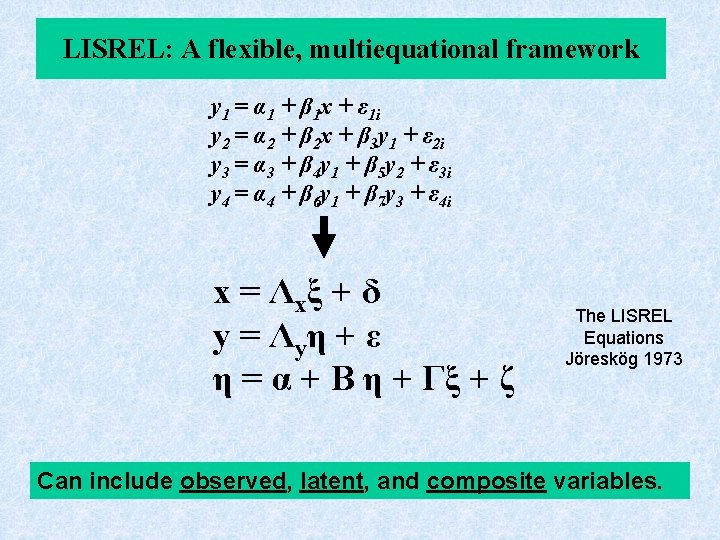
LISREL: A flexible, multiequational framework y 1 = α 1 + β 1 x + ε 1 i y 2 = α 2 + β 2 x + β 3 y 1 + ε 2 i y 3 = α 3 + β 4 y 1 + β 5 y 2 + ε 3 i y 4 = α 4 + β 6 y 1 + β 7 y 3 + ε 4 i x = Λxξ + δ y = Λyη + ε η = α + Β η + Γξ + ζ The LISREL Equations Jöreskög 1973 Can include observed, latent, and composite variables.
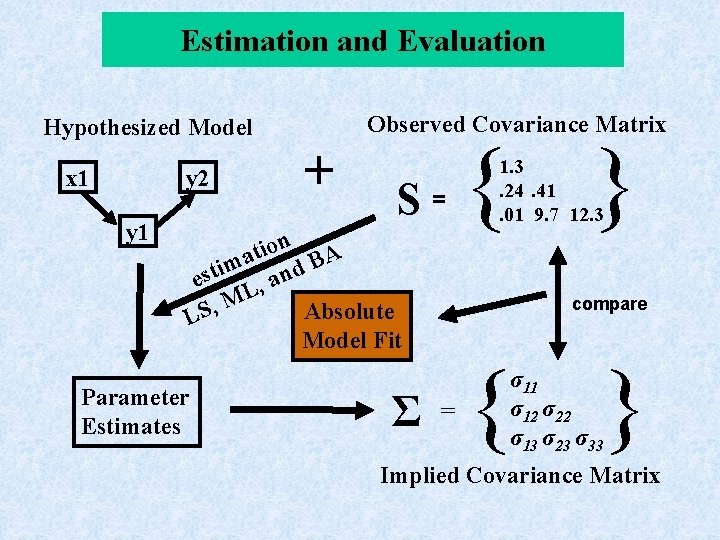
Estimation and Evaluation Hypothesized Model x 1 y 2 y 1 Observed Covariance Matrix + S= n o i t A a B m esti , and L M Absolute LS, Model Fit Parameter Estimates Σ { } 1. 3. 24. 41. 01 9. 7 12. 3 compare = { } σ11 σ12 σ22 σ13 σ23 σ33 Implied Covariance Matrix
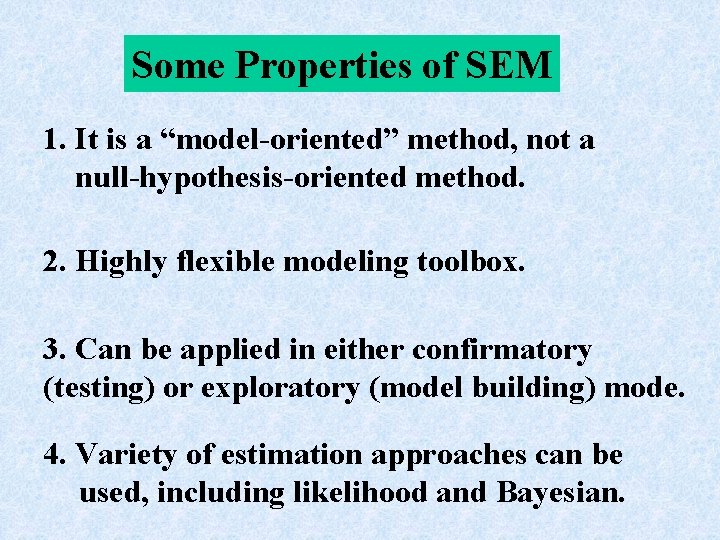
Some Properties of SEM 1. It is a “model-oriented” method, not a null-hypothesis-oriented method. 2. Highly flexible modeling toolbox. 3. Can be applied in either confirmatory (testing) or exploratory (model building) mode. 4. Variety of estimation approaches can be used, including likelihood and Bayesian.
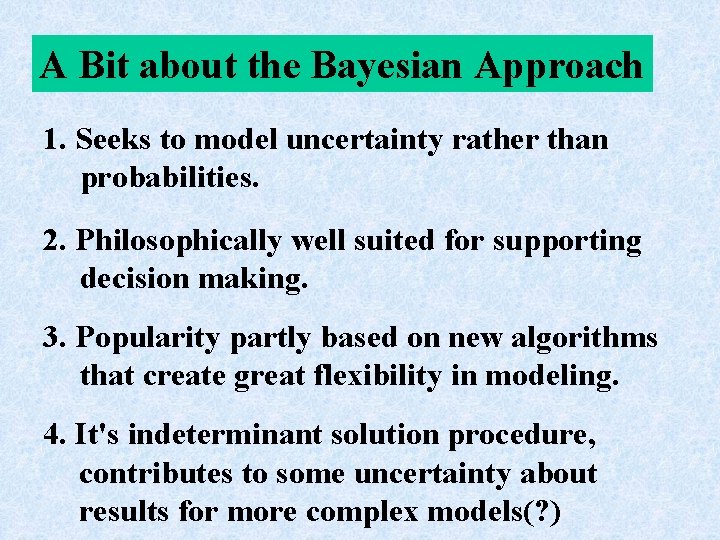
A Bit about the Bayesian Approach 1. Seeks to model uncertainty rather than probabilities. 2. Philosophically well suited for supporting decision making. 3. Popularity partly based on new algorithms that create great flexibility in modeling. 4. It's indeterminant solution procedure, contributes to some uncertainty about results for more complex models(? )
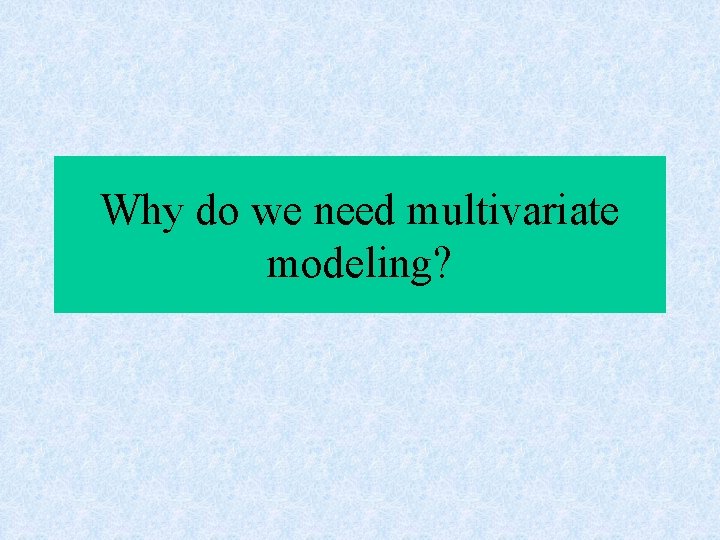
Why do we need multivariate modeling?
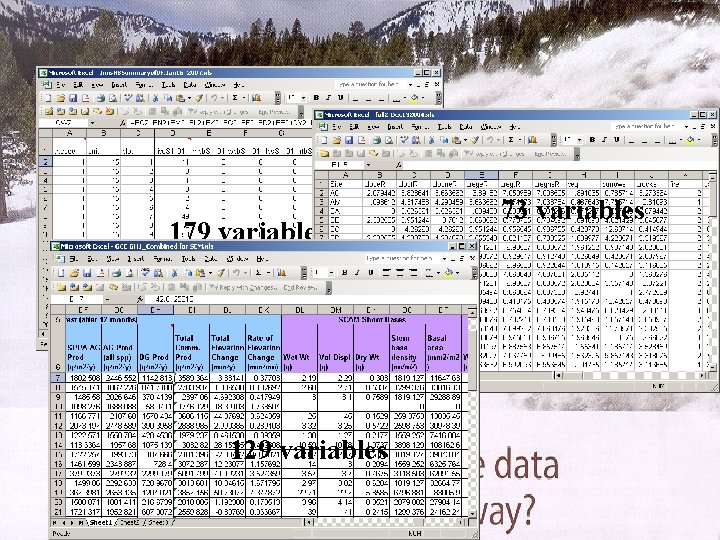
179 variables 129 variables 73 variables
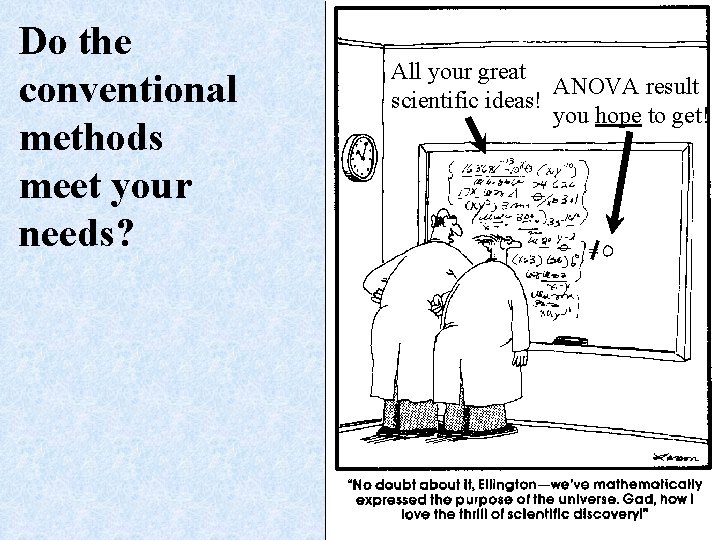
Do the conventional methods meet your needs? All your great ANOVA result scientific ideas! you hope to get!
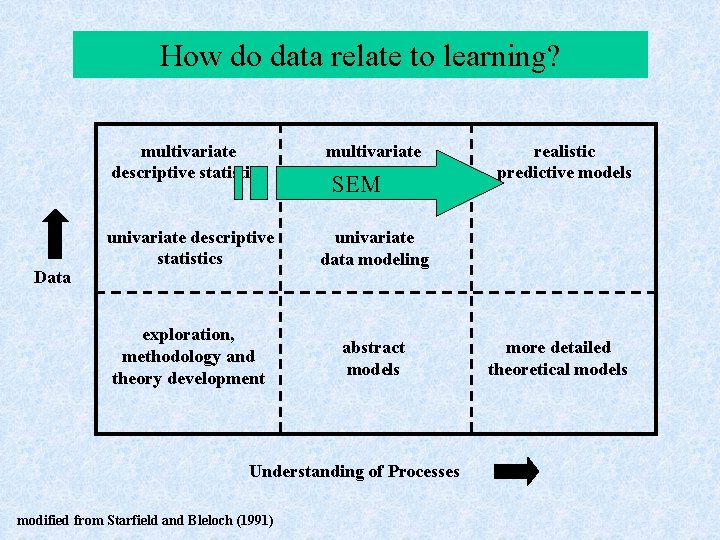
How do data relate to learning? Data multivariate descriptive statistics multivariate data modeling univariate descriptive statistics univariate data modeling exploration, methodology and theory development abstract models SEM Understanding of Processes modified from Starfield and Bleloch (1991) realistic predictive models more detailed theoretical models
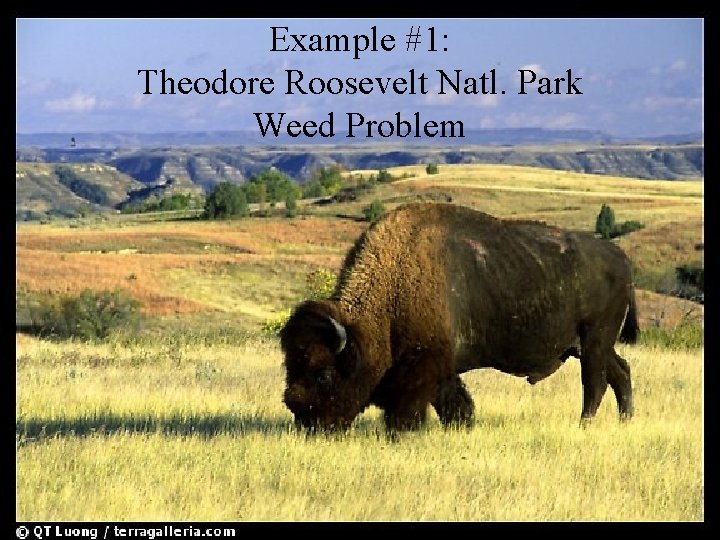
Example #1: Theodore Roosevelt Natl. Park Weed Problem
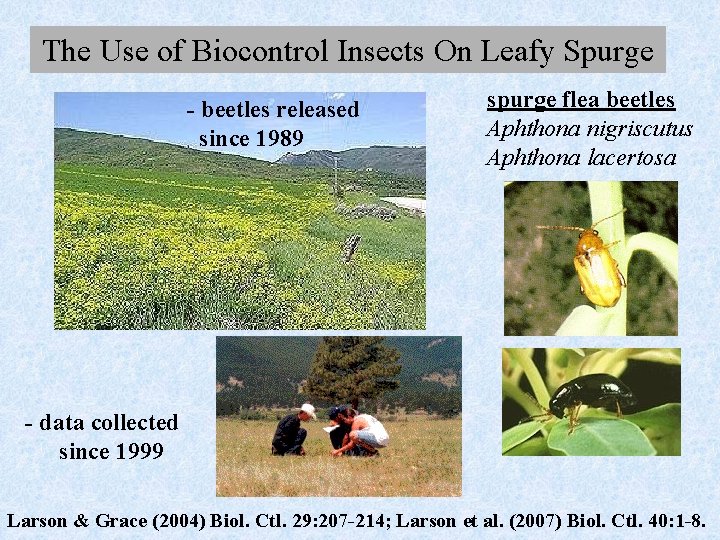
The Use of Biocontrol Insects On Leafy Spurge - beetles released since 1989 spurge flea beetles Aphthona nigriscutus Aphthona lacertosa - data collected since 1999 Larson & Grace (2004) Biol. Ctl. 29: 207 -214; Larson et al. (2007) Biol. Ctl. 40: 1 -8.
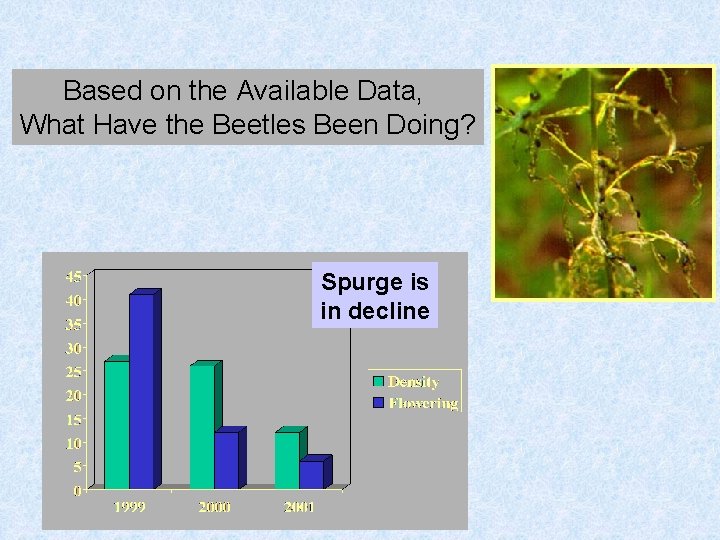
Based on the Available Data, What Have the Beetles Been Doing? Spurge is in decline
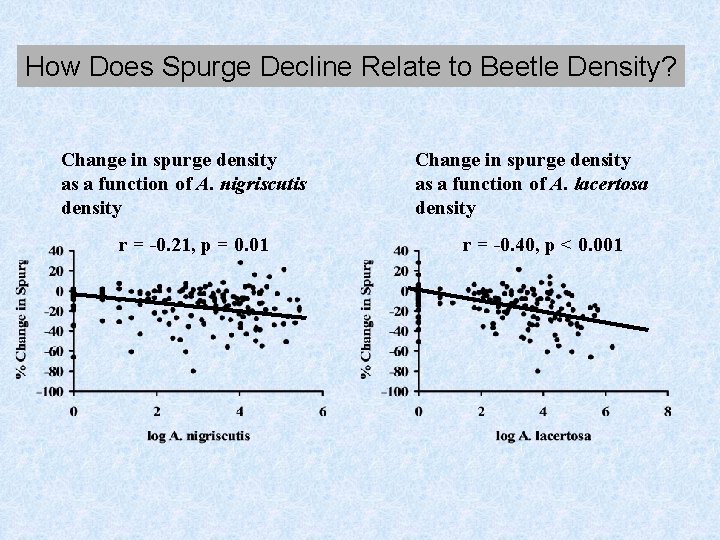
How Does Spurge Decline Relate to Beetle Density? Change in spurge density as a function of A. nigriscutis density r = -0. 21, p = 0. 01 Change in spurge density as a function of A. lacertosa density r = -0. 40, p < 0. 001
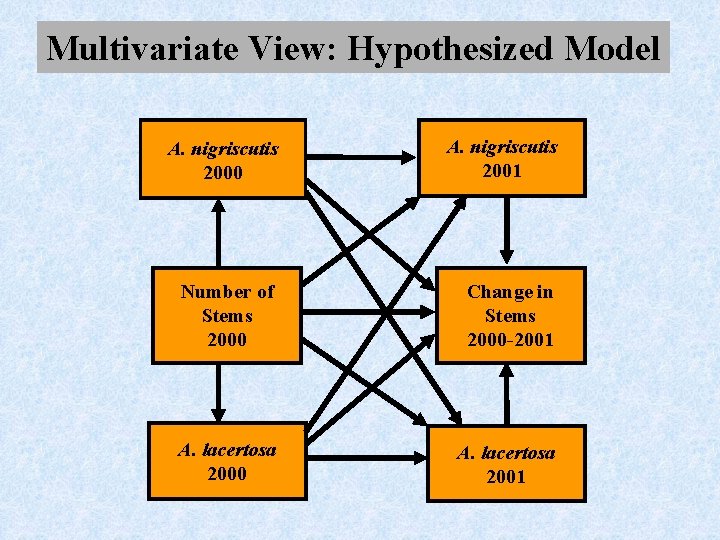
Multivariate View: Hypothesized Model A. nigriscutis 2000 A. nigriscutis 2001 Number of Stems 2000 Change in Stems 2000 -2001 A. lacertosa 2000 A. lacertosa 2001
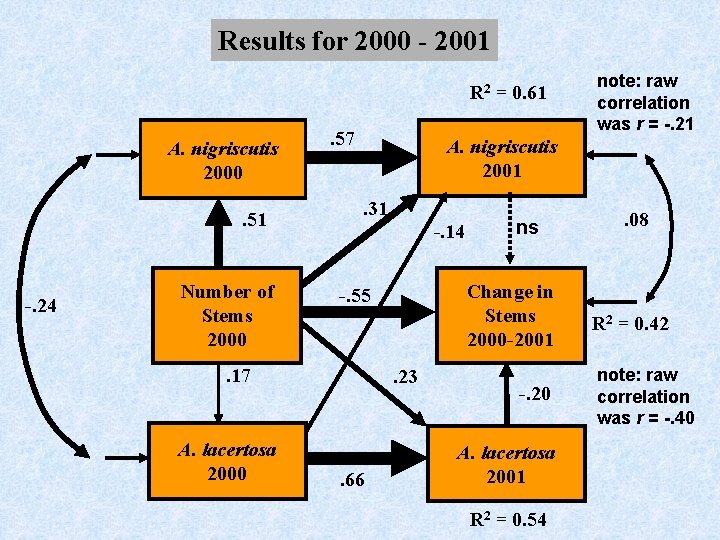
Results for 2000 - 2001 R 2 = 0. 61 A. nigriscutis 2000. 51 -. 24 Number of Stems 2000 . 57 A. nigriscutis 2001. 31 -. 14 . 23 . 66 ns Change in Stems 2000 -2001 -. 55 . 17 A. lacertosa 2000 note: raw correlation was r = -. 21 -. 20 A. lacertosa 2001 R 2 = 0. 54 . 08 R 2 = 0. 42 note: raw correlation was r = -. 40
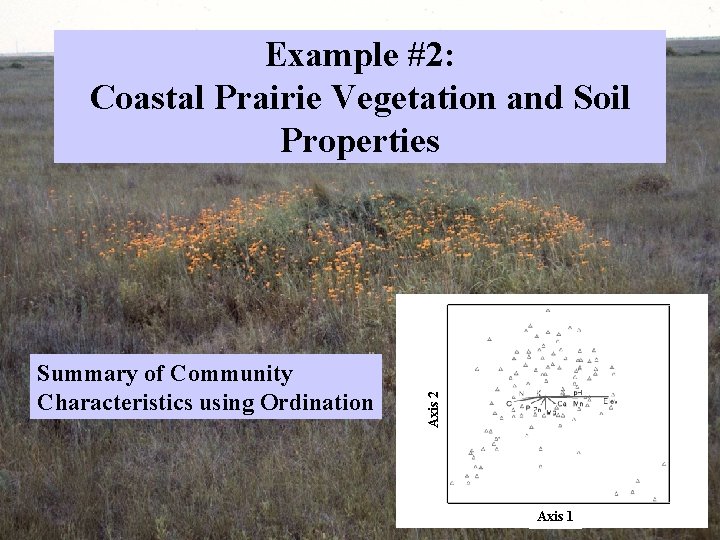
Summary of Community Characteristics using Ordination Axis 2 Example #2: Coastal Prairie Vegetation and Soil Properties Axis 1
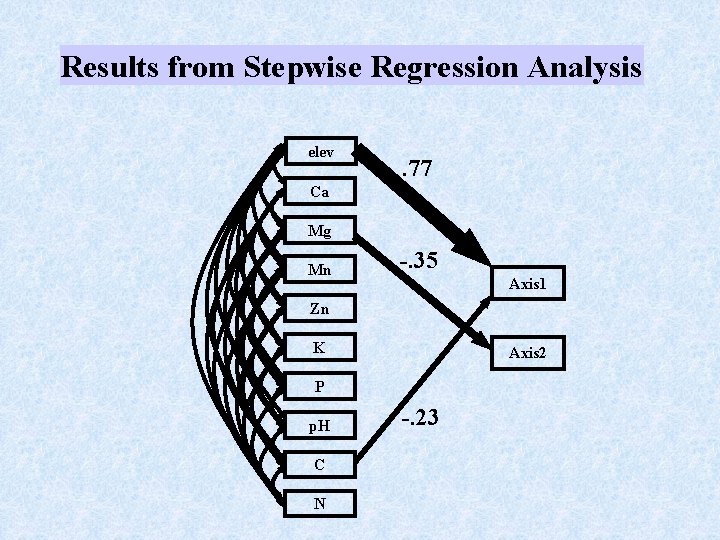
Results from Stepwise Regression Analysis elev . 77 Ca Mg Mn -. 35 Axis 1 Zn K Axis 2 P p. H C N -. 23
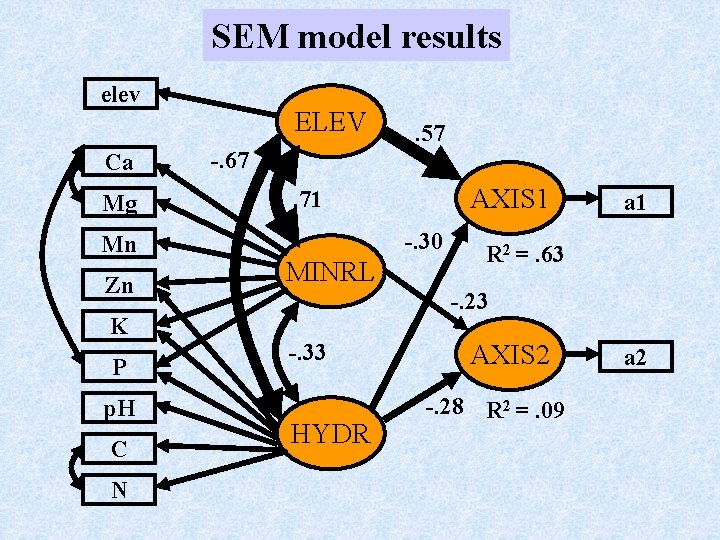
SEM model results elev Ca Mg Mn Zn K P p. H C N ELEV . 57 -. 67 AXIS 1 . 71 -. 30 MINRL -. 33 HYDR a 1 R 2 =. 63 -. 23 AXIS 2 -. 28 R 2 =. 09 a 2
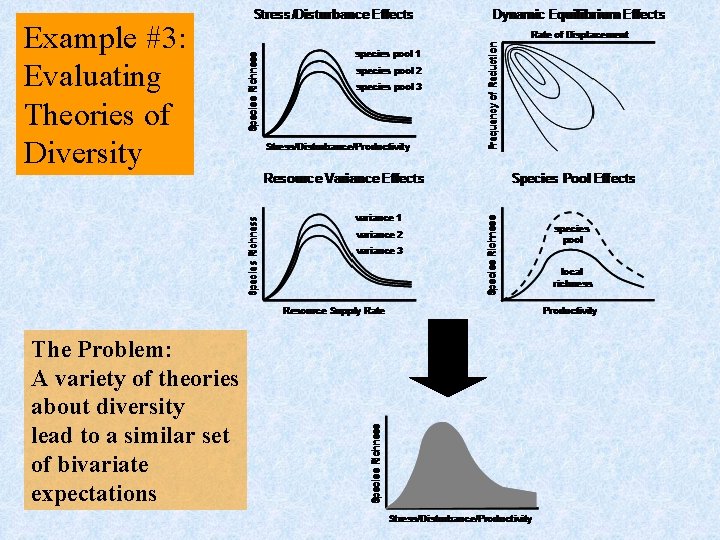
Example #3: Evaluating Theories of Diversity The Problem: A variety of theories about diversity lead to a similar set of bivariate expectations
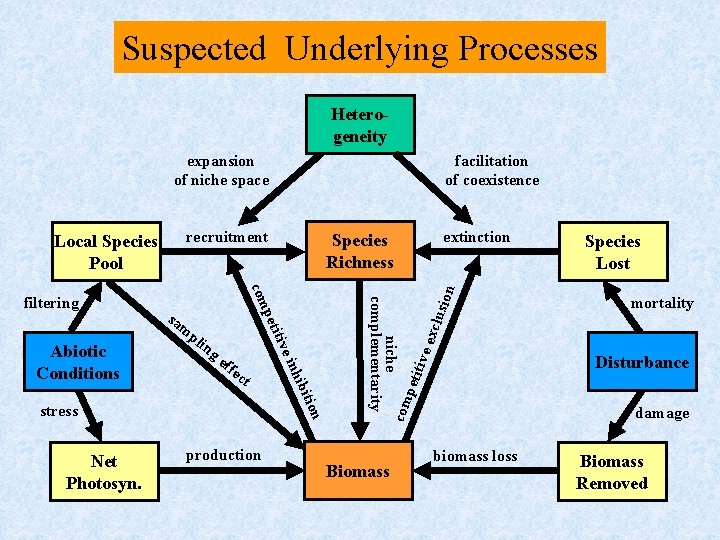
Suspected Underlying Processes Heterogeneity expansion of niche space recruitment itio Net Photosyn. n stress production usio mortality excl tive ct hib ffe Species Lost Disturbance peti ge niche complementarity in e in itiv pet m pl n com Abiotic Conditions sa extinction Species Richness com Local Species Pool filtering facilitation of coexistence Biomass damage biomass loss Biomass Removed
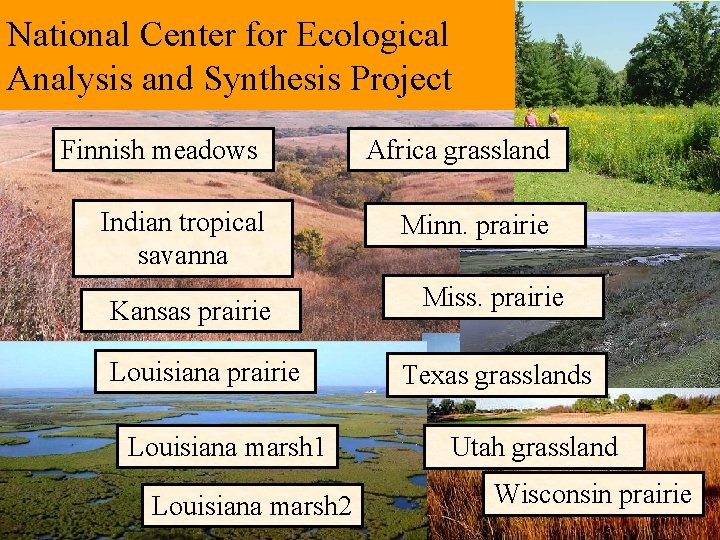
National Center for Ecological Analysis and Synthesis Project Finnish meadows Africa grassland Indian tropical savanna Minn. prairie Kansas prairie Louisiana marsh 1 Louisiana marsh 2 Miss. prairie Texas grasslands Utah grassland Wisconsin prairie
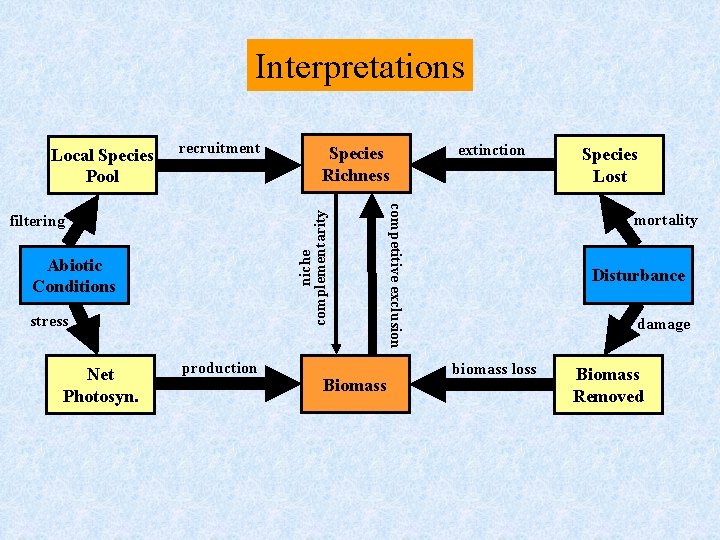
Interpretations recruitment Abiotic Conditions stress production Biomass extinction competitive exclusion filtering Net Photosyn. Species Richness niche complementarity Local Species Pool Species Lost mortality Disturbance damage biomass loss Biomass Removed
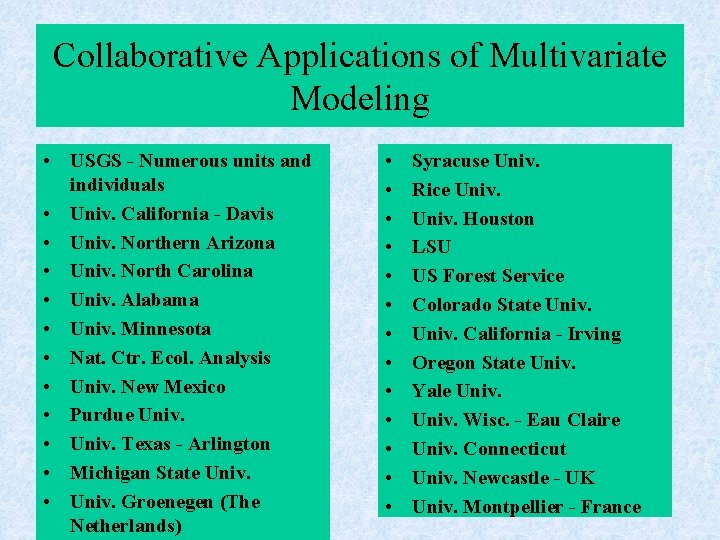
Collaborative Applications of Multivariate Modeling • USGS - Numerous units and individuals • Univ. California - Davis • Univ. Northern Arizona • Univ. North Carolina • Univ. Alabama • Univ. Minnesota • Nat. Ctr. Ecol. Analysis • Univ. New Mexico • Purdue Univ. • Univ. Texas - Arlington • Michigan State Univ. • Univ. Groenegen (The Netherlands) • • • • Syracuse Univ. Rice Univ. Houston LSU US Forest Service Colorado State Univ. California - Irving Oregon State Univ. Yale Univ. Wisc. - Eau Claire Univ. Connecticut Univ. Newcastle - UK Univ. Montpellier - France
Structural equation modeling for dummies
Structural equation modeling
Dynamic structural equation modeling
Data modeling using entity relationship model
Er model diagram
Role modeling theory
Relational modeling vs dimensional modeling
Structural modeling in uml
Common mechanisms in basic structural modeling
Structural modeling vhdl
Best practices for data warehousing
Structural health monitoring
Structural health monitoring aircraft
Comparative vacuum monitoring
4-4 using t accounts to analyze transactions
Data-data monitoring lingkungan merupakan gambaran dari
Modeling using variation calculator
Lesson 12 modeling using similarity
Modeling of digital communication systems using simulink
Modeling of digital communication systems using simulink
Programs that organize analyze and graph numerical data
The act of drawing conclusions from the analyzed data
Data "projectile analyze" position
Common features of software
Compatibility equation in structural analysis
Modeling relational data with graph convolutional networks
Data modeling techniques
Data warehouse modeling tutorial