SingleFactor Studies KNNL Chapter 16 SingleFactor Models Independent
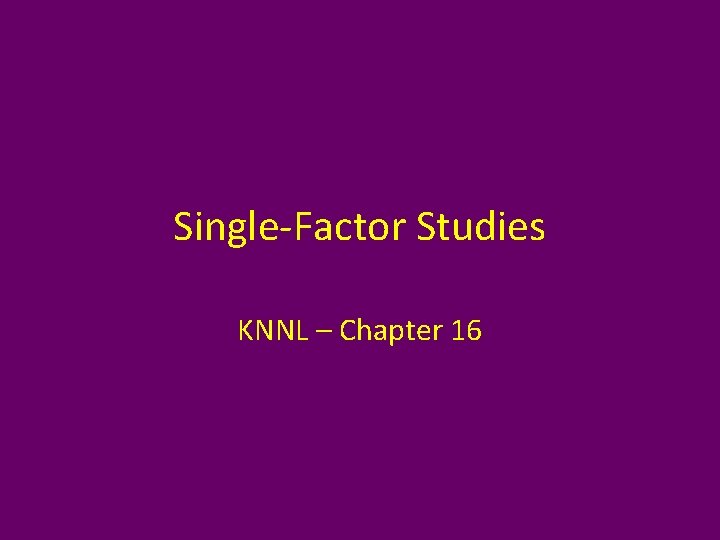
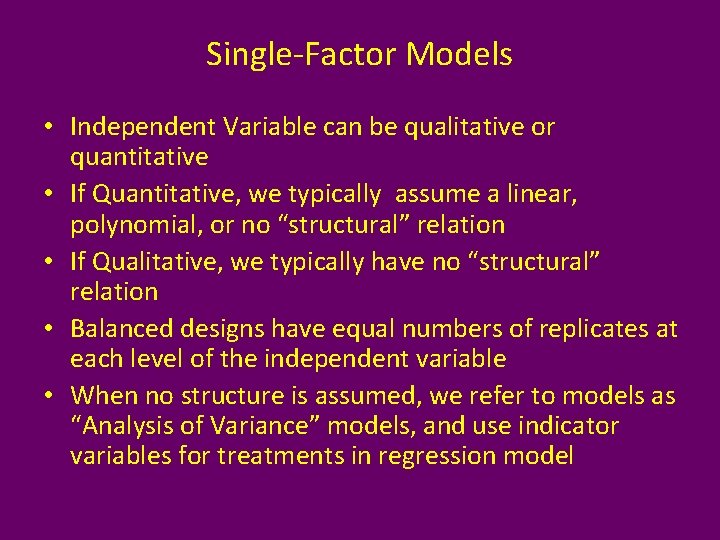
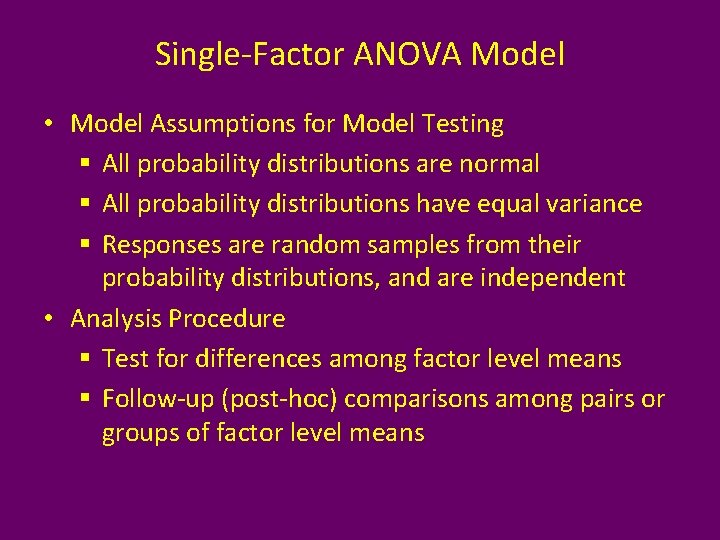
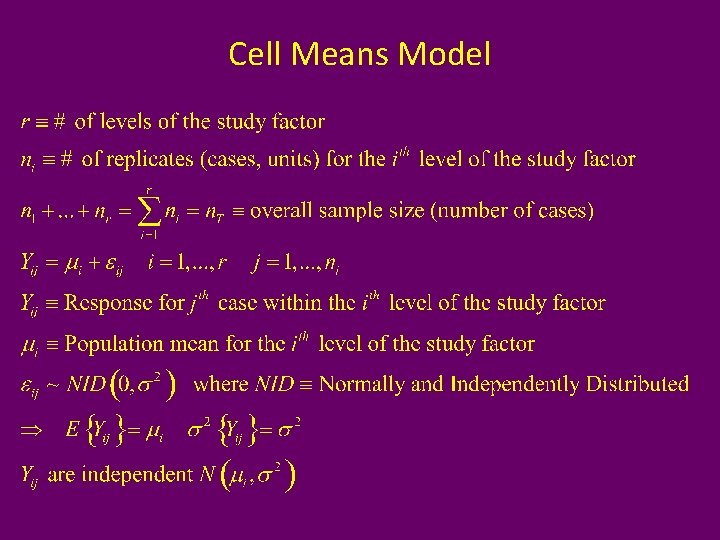
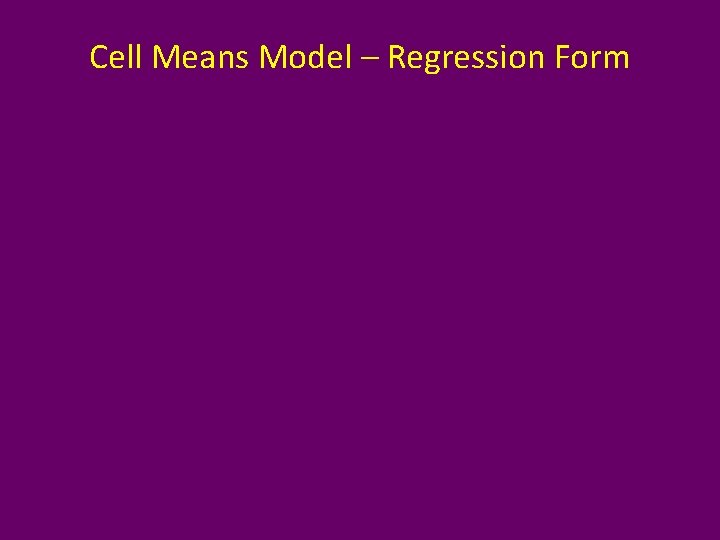
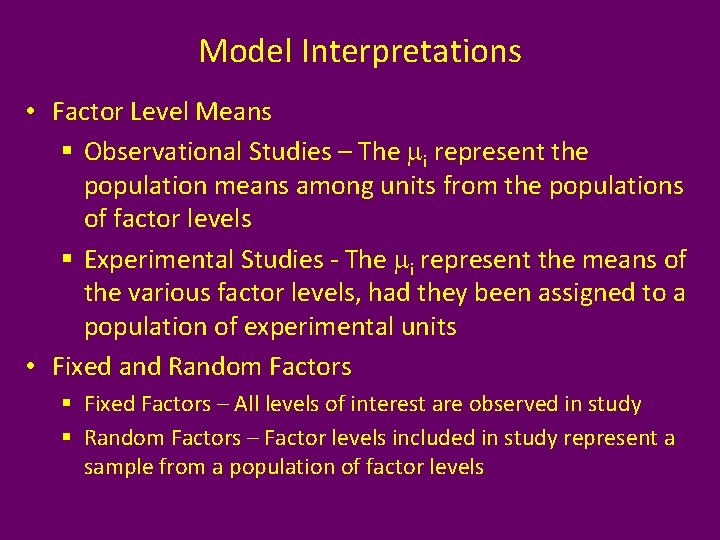
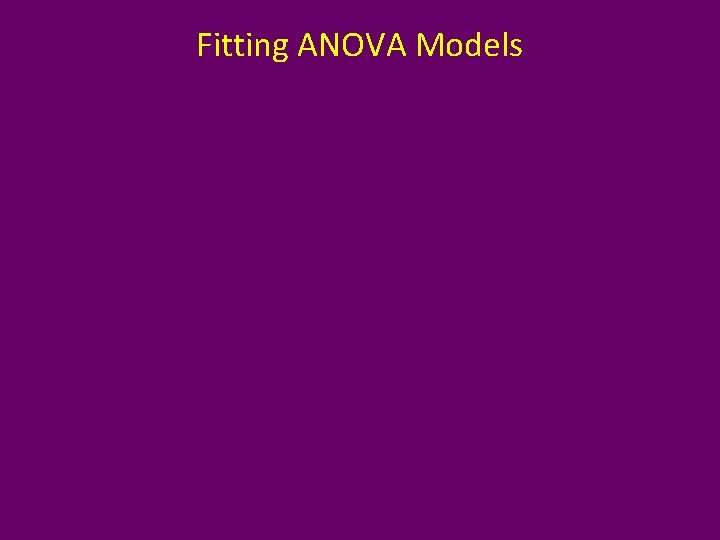
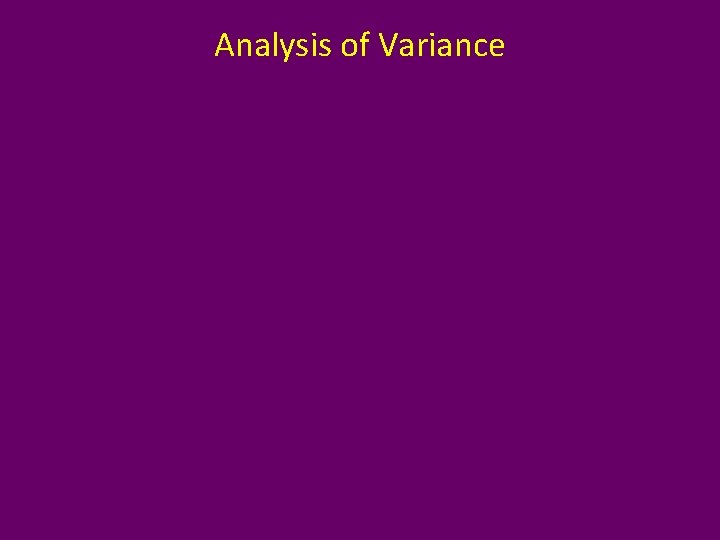
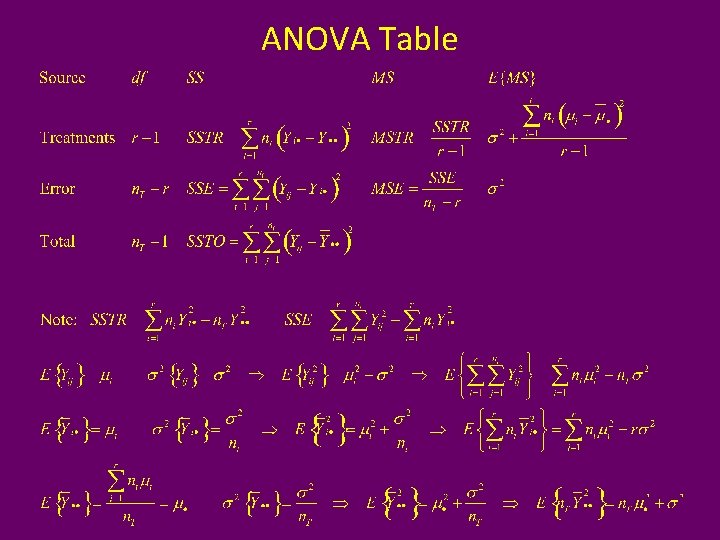
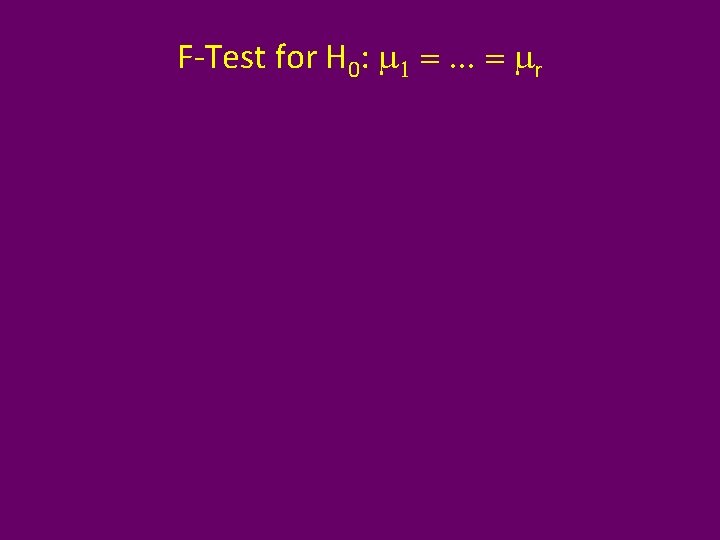
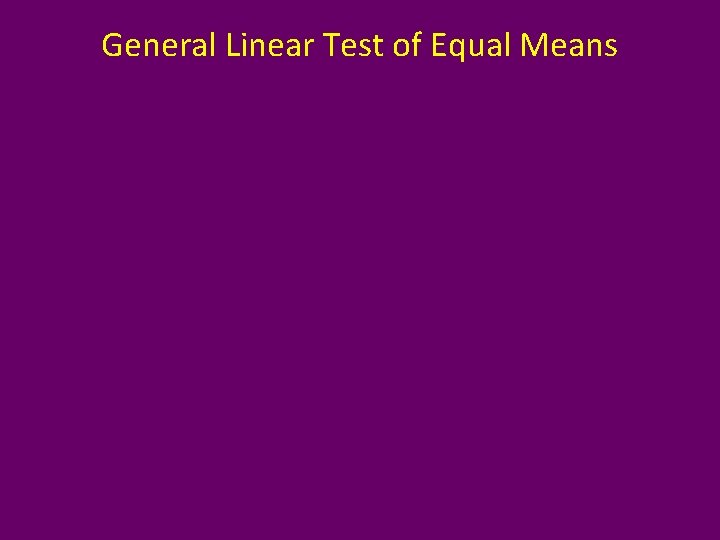
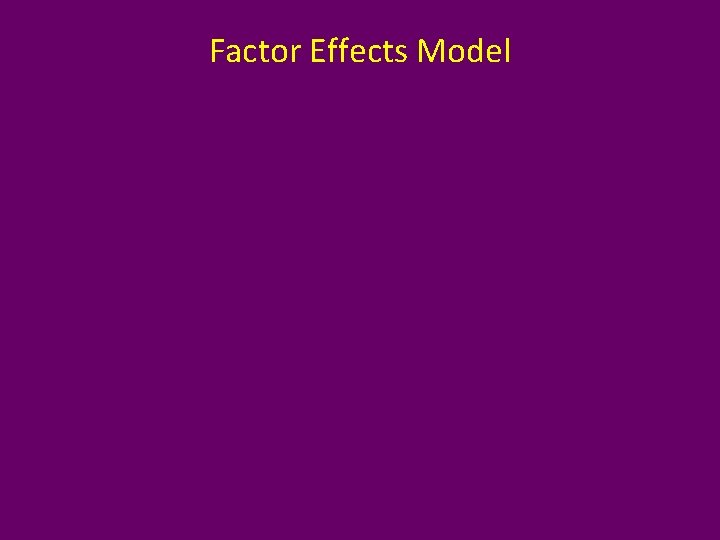
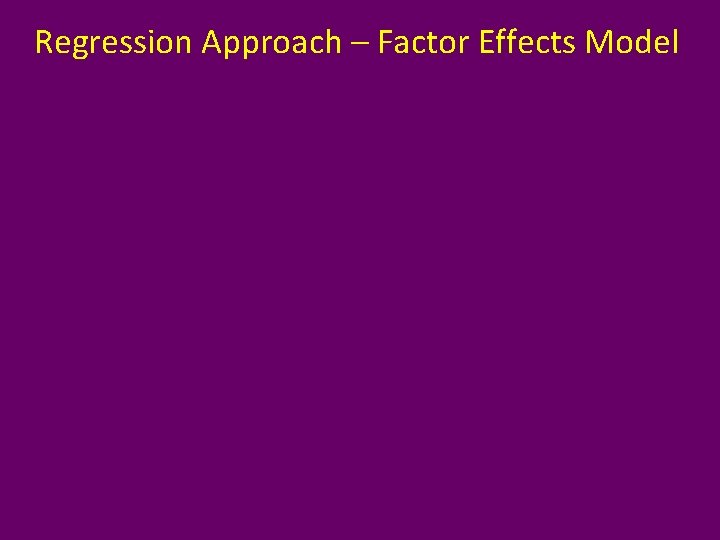
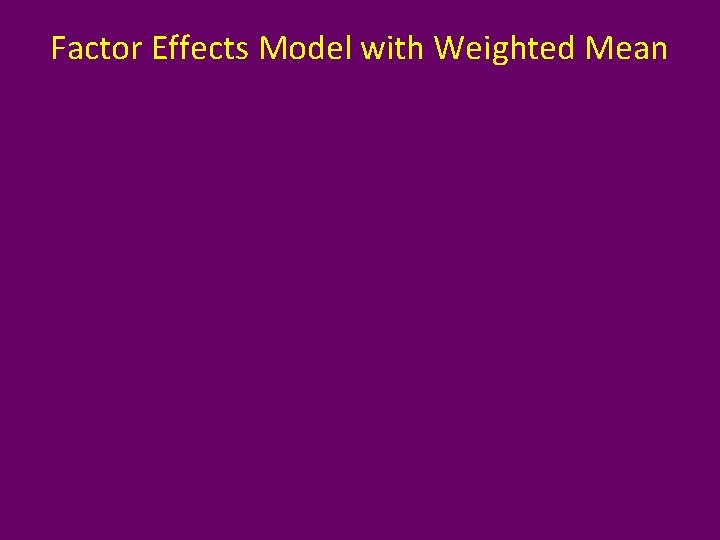
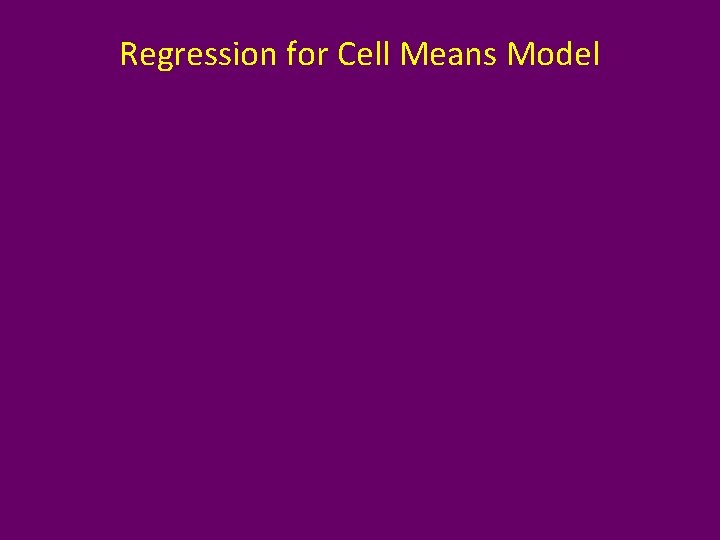
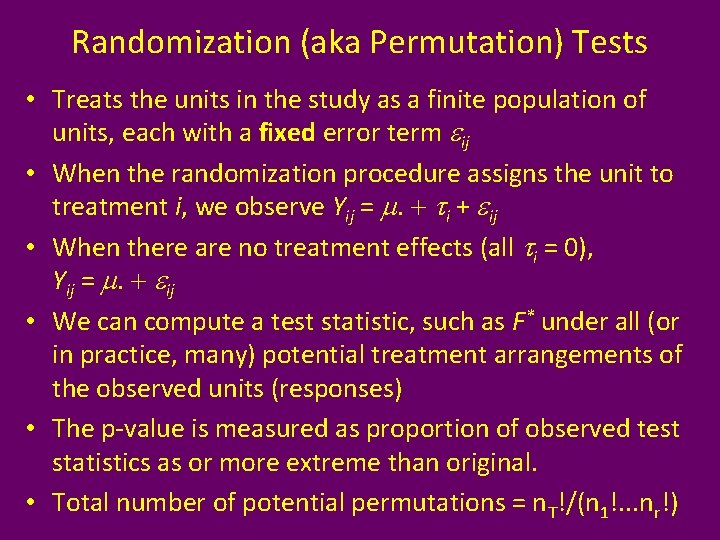
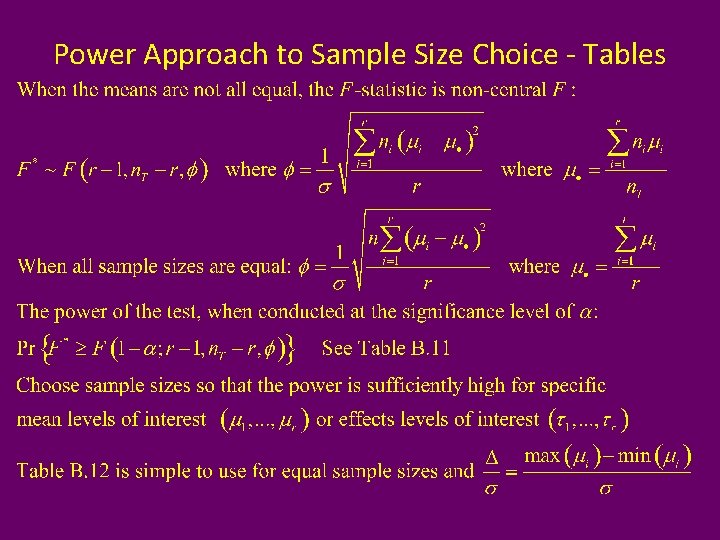
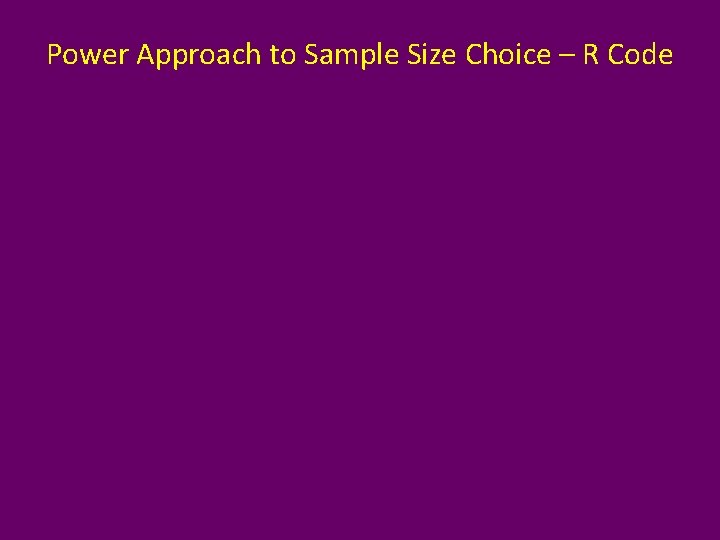
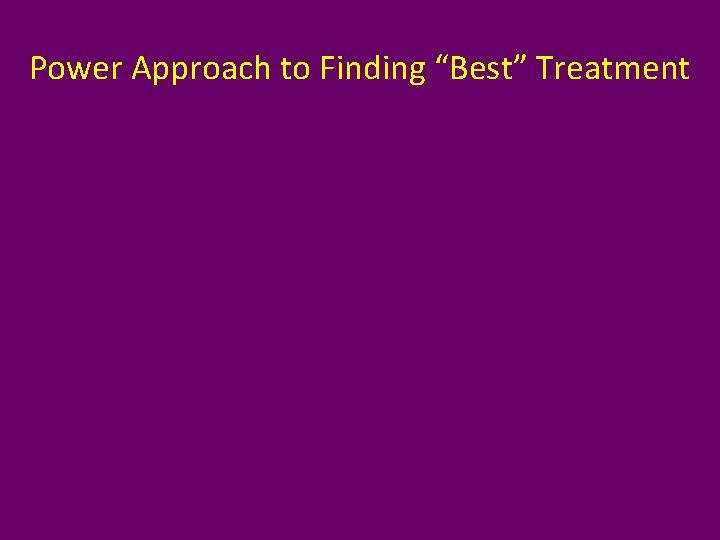
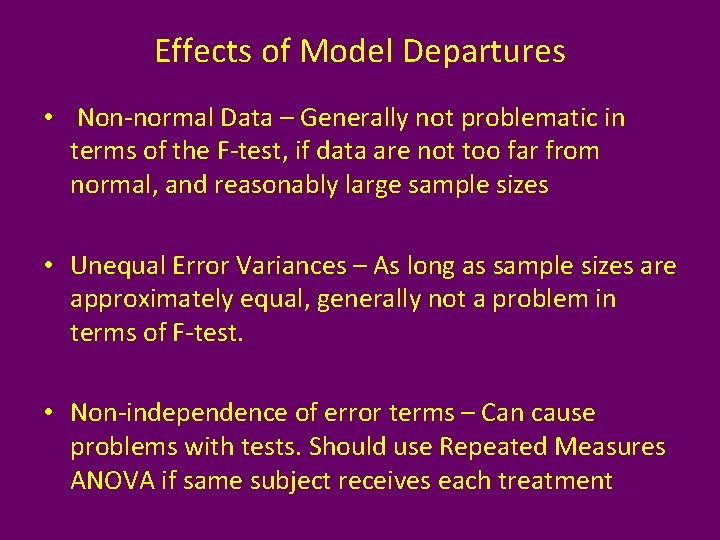
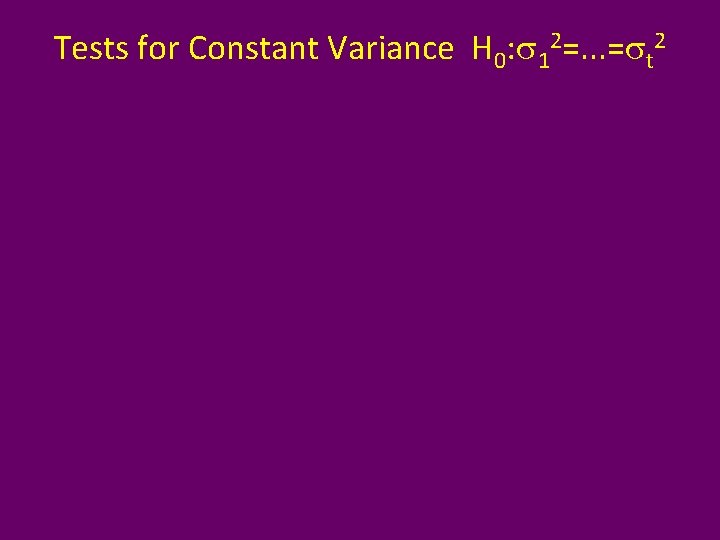
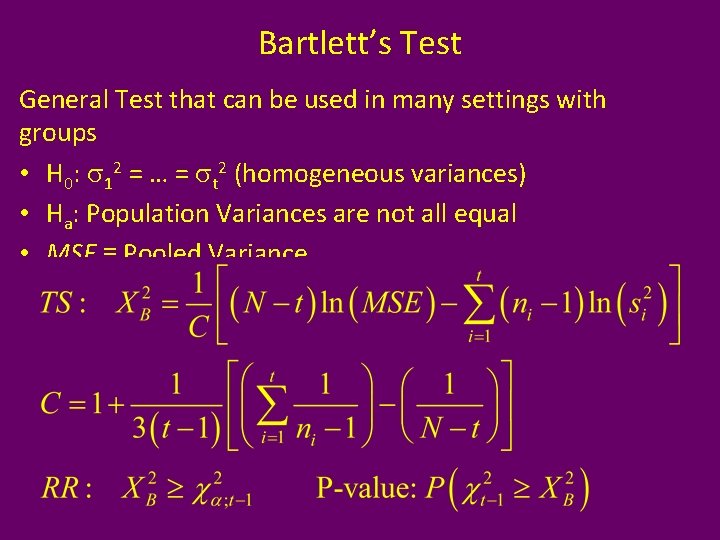
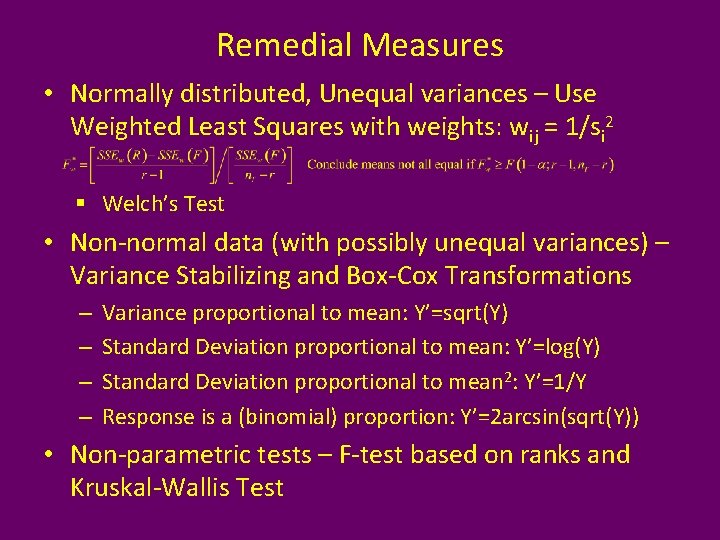
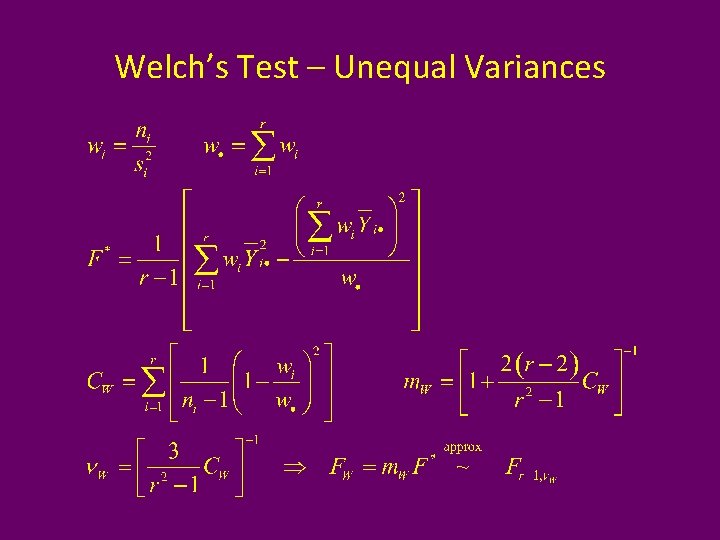
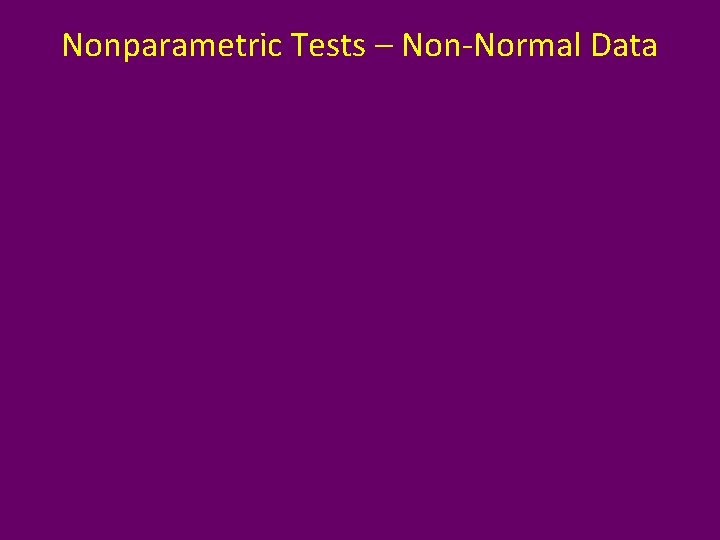
- Slides: 25
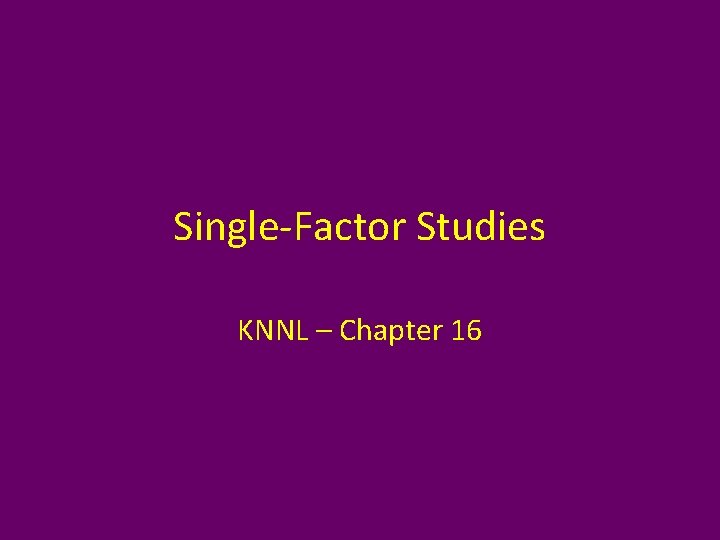
Single-Factor Studies KNNL – Chapter 16
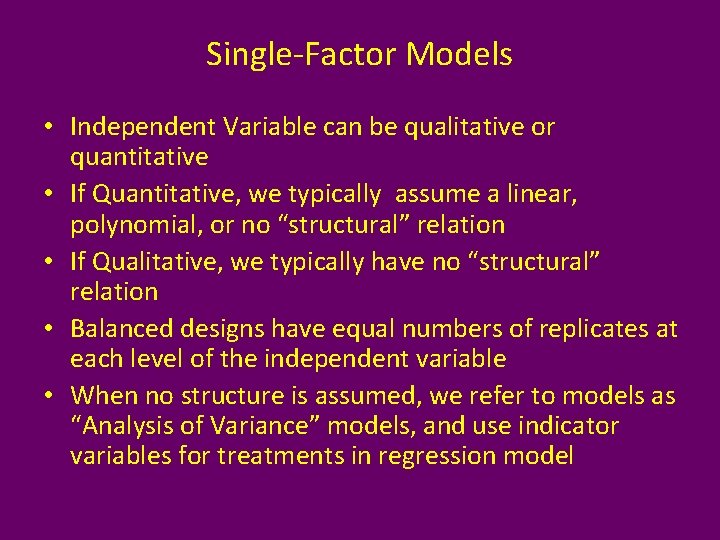
Single-Factor Models • Independent Variable can be qualitative or quantitative • If Quantitative, we typically assume a linear, polynomial, or no “structural” relation • If Qualitative, we typically have no “structural” relation • Balanced designs have equal numbers of replicates at each level of the independent variable • When no structure is assumed, we refer to models as “Analysis of Variance” models, and use indicator variables for treatments in regression model
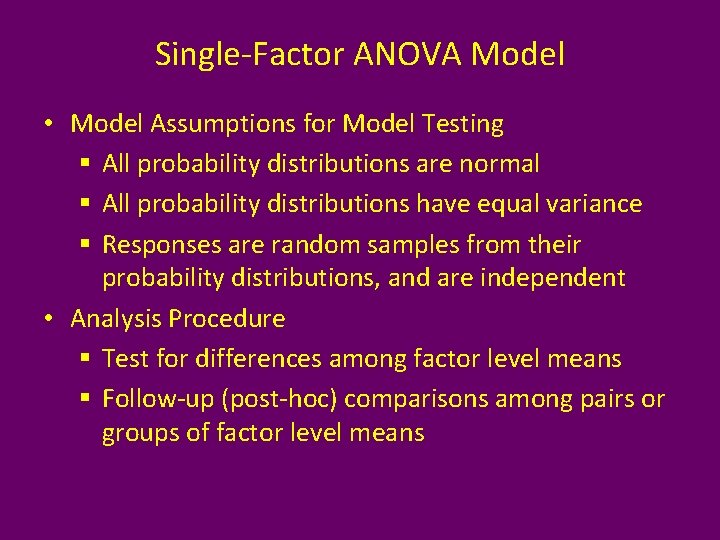
Single-Factor ANOVA Model • Model Assumptions for Model Testing § All probability distributions are normal § All probability distributions have equal variance § Responses are random samples from their probability distributions, and are independent • Analysis Procedure § Test for differences among factor level means § Follow-up (post-hoc) comparisons among pairs or groups of factor level means
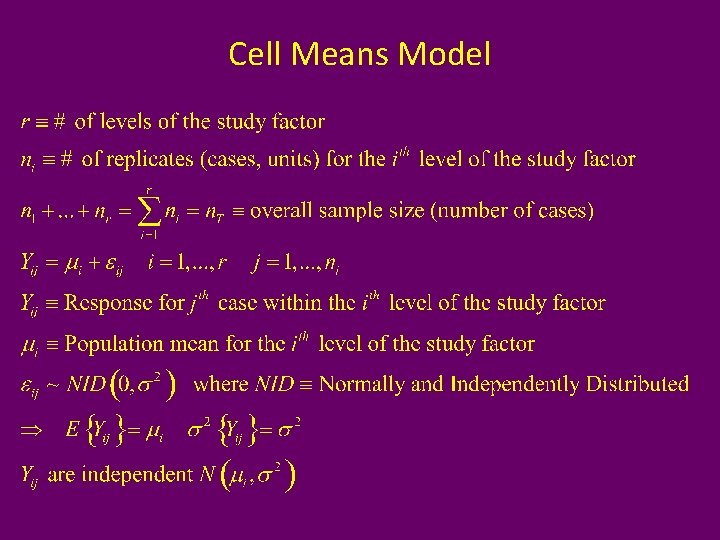
Cell Means Model
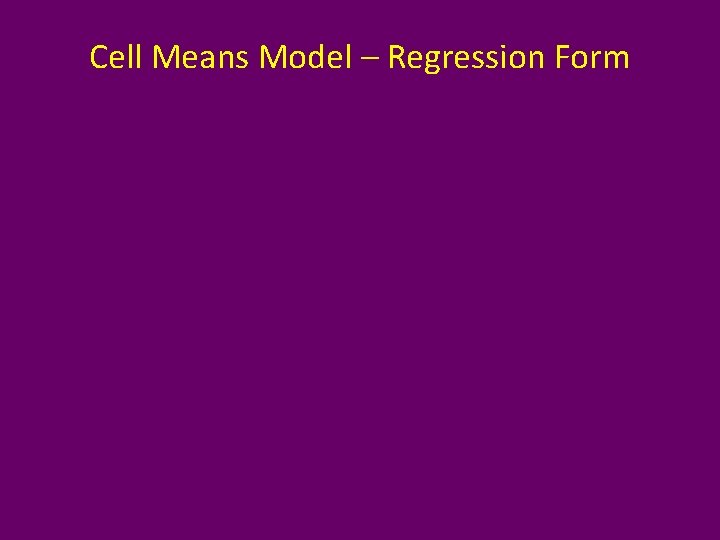
Cell Means Model – Regression Form
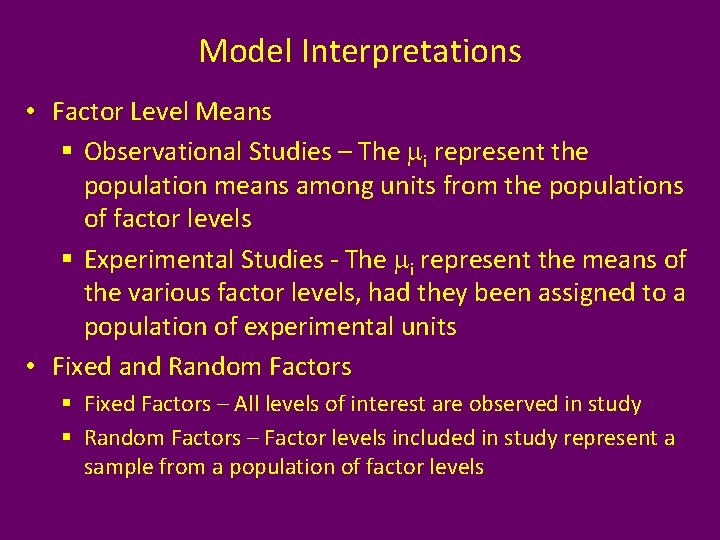
Model Interpretations • Factor Level Means § Observational Studies – The mi represent the population means among units from the populations of factor levels § Experimental Studies - The mi represent the means of the various factor levels, had they been assigned to a population of experimental units • Fixed and Random Factors § Fixed Factors – All levels of interest are observed in study § Random Factors – Factor levels included in study represent a sample from a population of factor levels
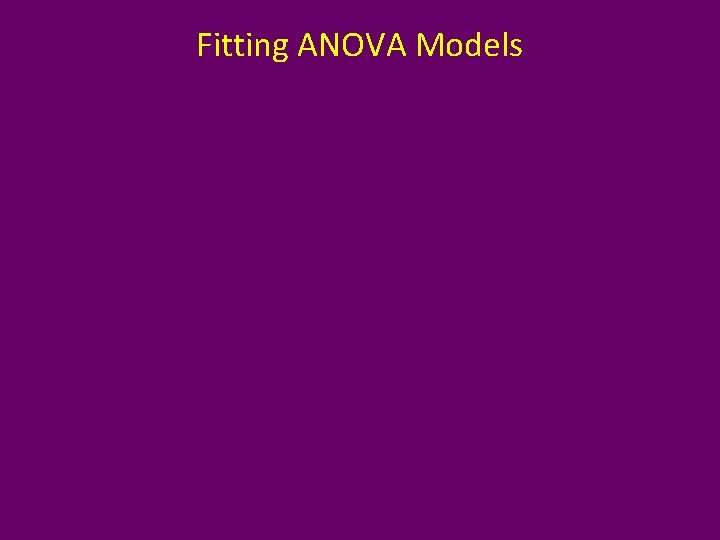
Fitting ANOVA Models
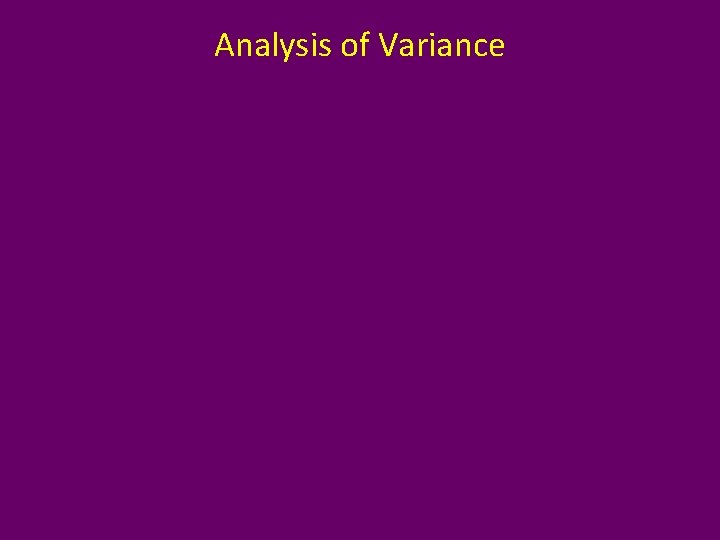
Analysis of Variance
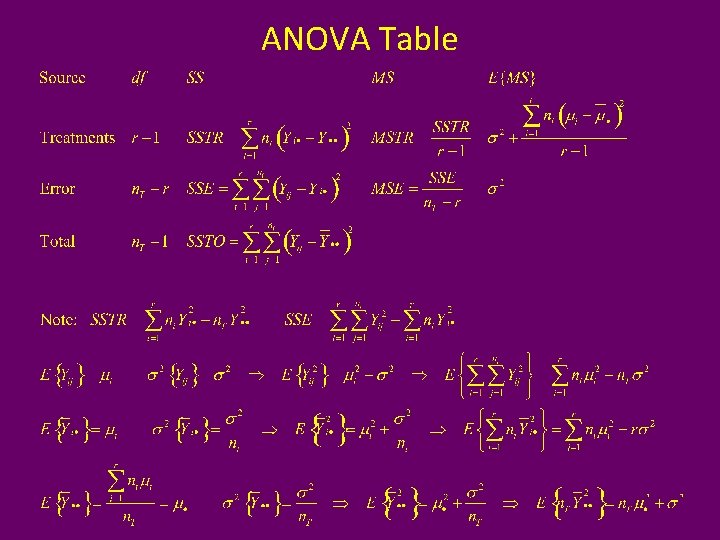
ANOVA Table
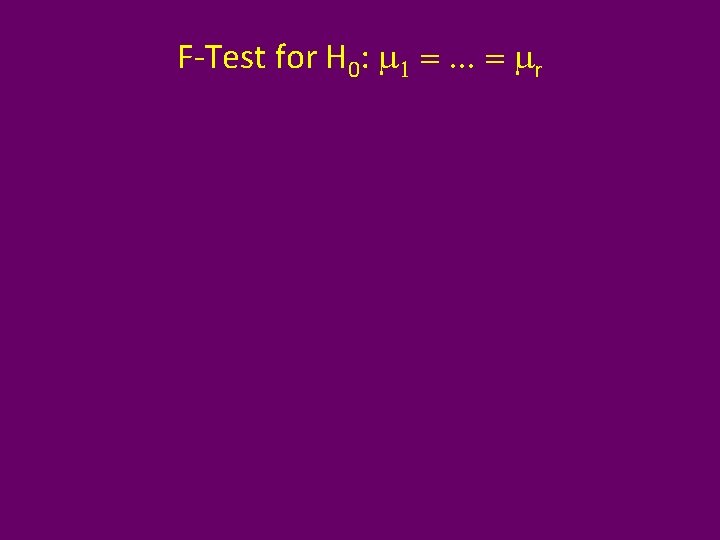
F-Test for H 0: m 1 =. . . = mr
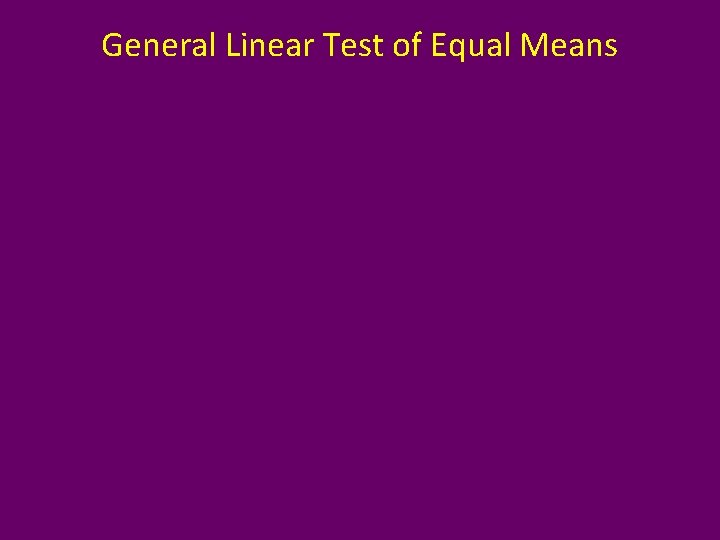
General Linear Test of Equal Means
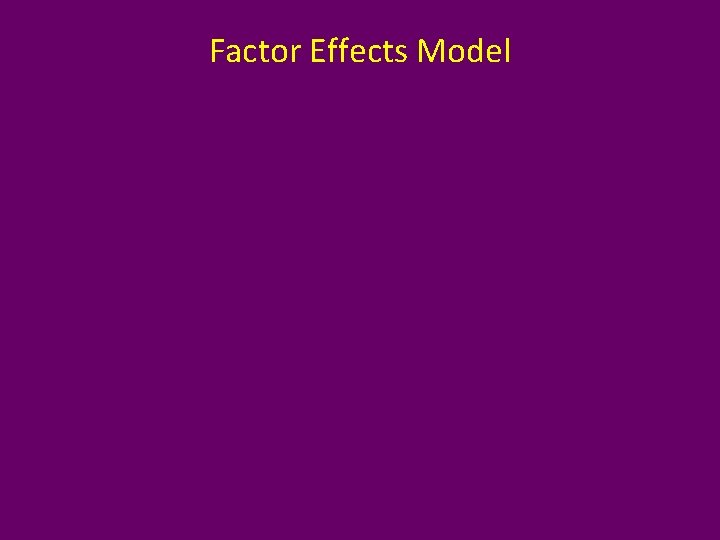
Factor Effects Model
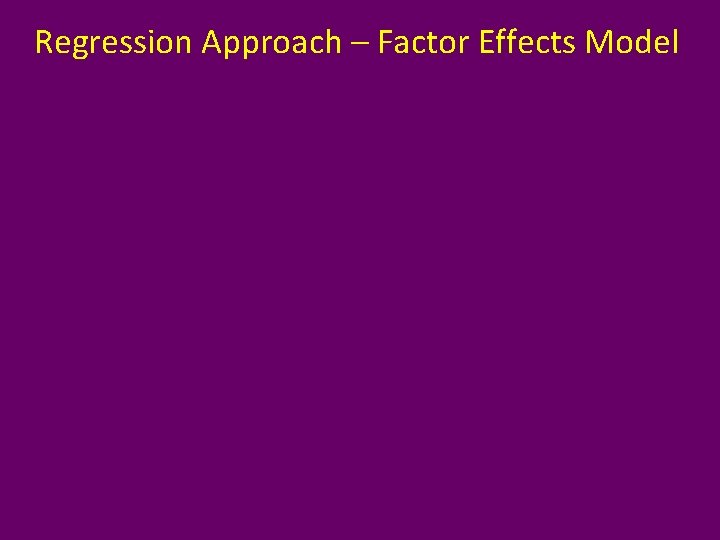
Regression Approach – Factor Effects Model
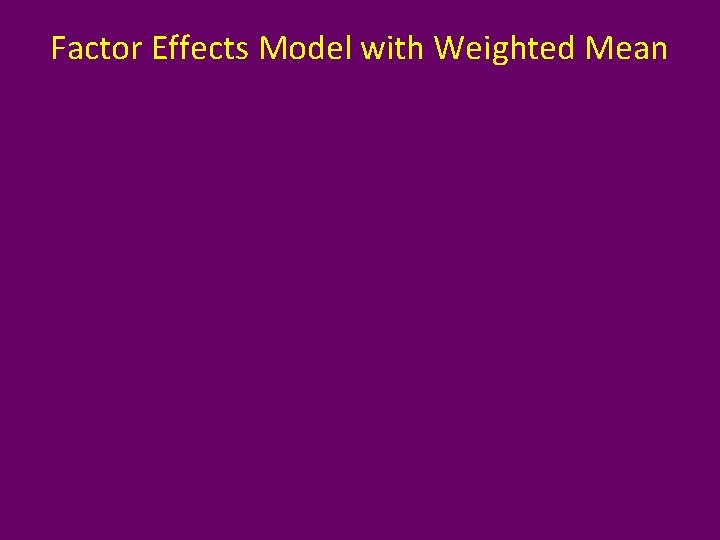
Factor Effects Model with Weighted Mean
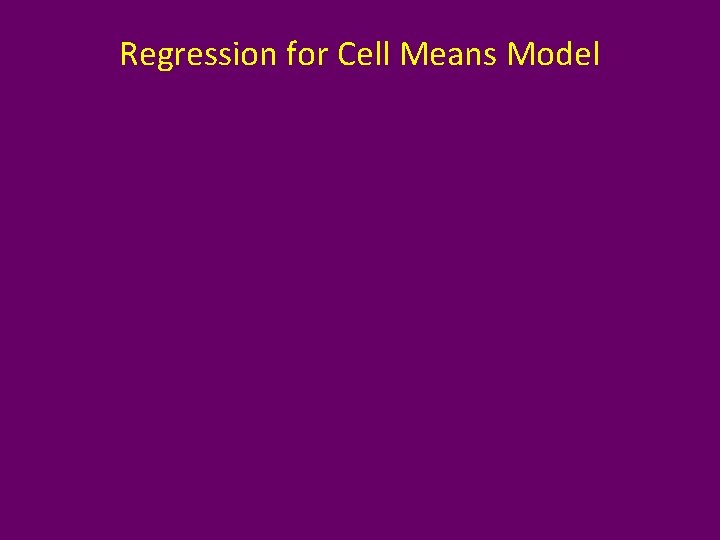
Regression for Cell Means Model
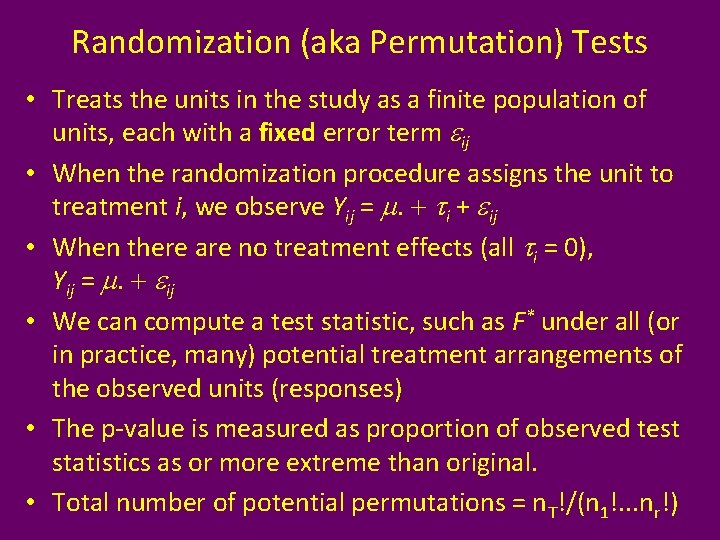
Randomization (aka Permutation) Tests • Treats the units in the study as a finite population of units, each with a fixed error term eij • When the randomization procedure assigns the unit to treatment i, we observe Yij = m. + ti + eij • When there are no treatment effects (all ti = 0), Yij = m. + eij • We can compute a test statistic, such as F* under all (or in practice, many) potential treatment arrangements of the observed units (responses) • The p-value is measured as proportion of observed test statistics as or more extreme than original. • Total number of potential permutations = n. T!/(n 1!. . . nr!)
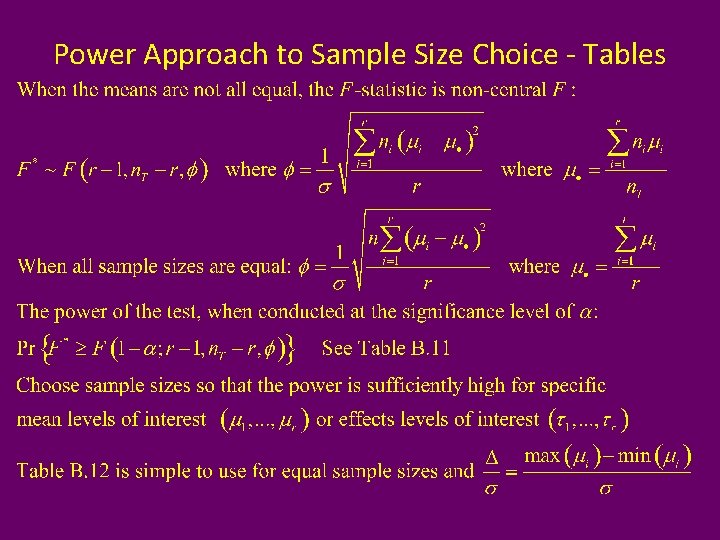
Power Approach to Sample Size Choice - Tables
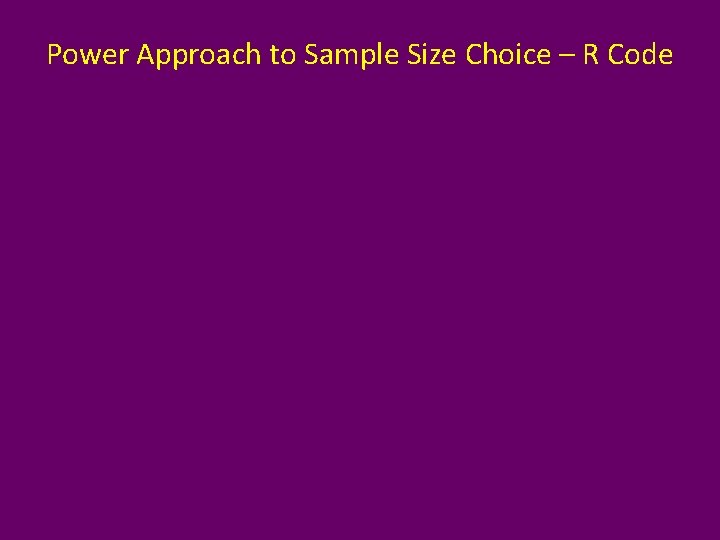
Power Approach to Sample Size Choice – R Code
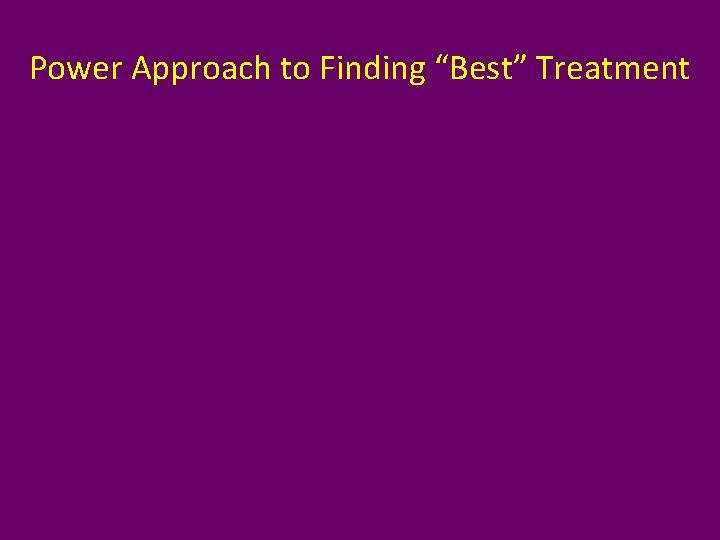
Power Approach to Finding “Best” Treatment
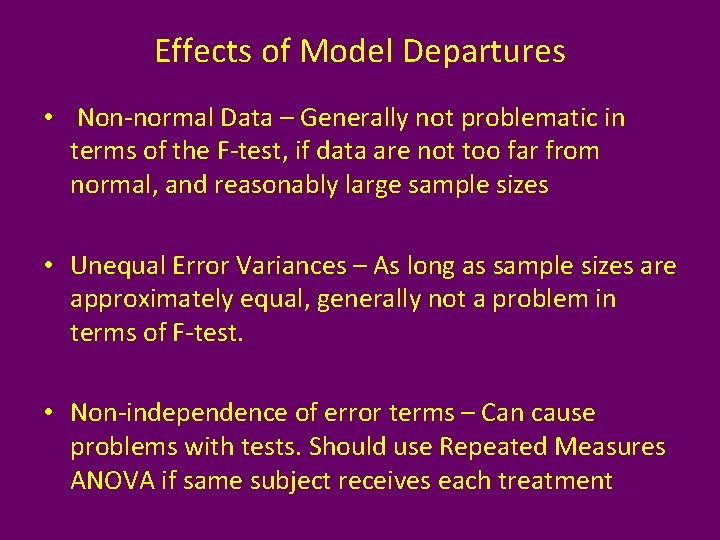
Effects of Model Departures • Non-normal Data – Generally not problematic in terms of the F-test, if data are not too far from normal, and reasonably large sample sizes • Unequal Error Variances – As long as sample sizes are approximately equal, generally not a problem in terms of F-test. • Non-independence of error terms – Can cause problems with tests. Should use Repeated Measures ANOVA if same subject receives each treatment
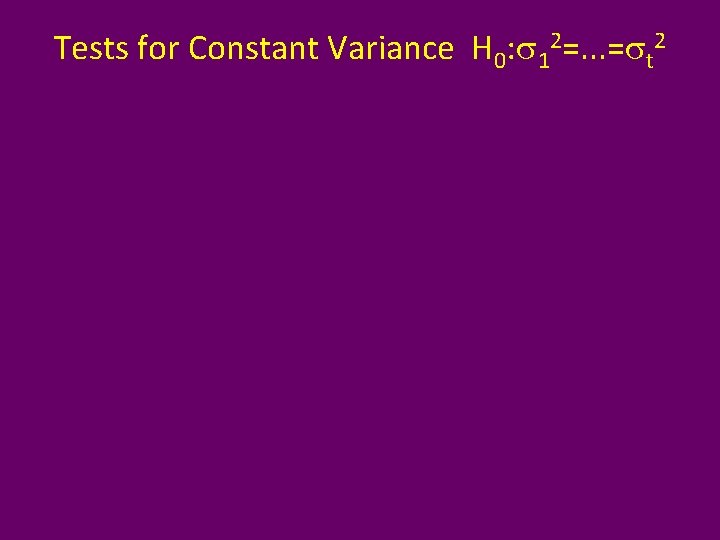
Tests for Constant Variance H 0: s 12=. . . =st 2
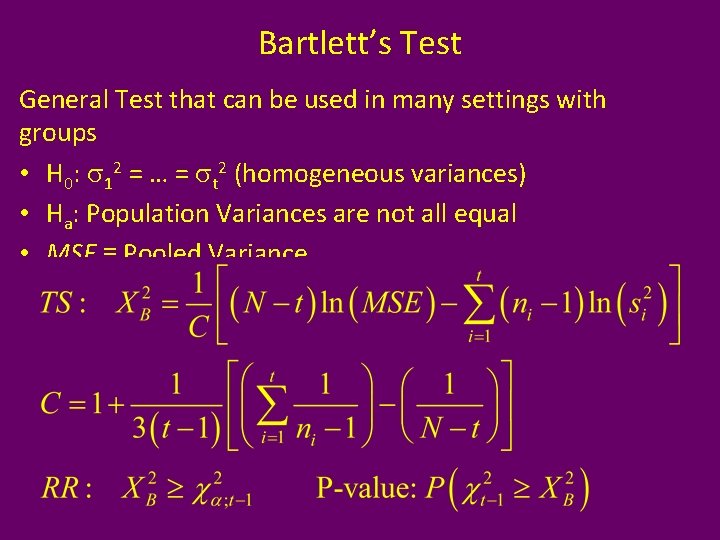
Bartlett’s Test General Test that can be used in many settings with groups • H 0: s 12 = … = st 2 (homogeneous variances) • Ha: Population Variances are not all equal • MSE ≡ Pooled Variance
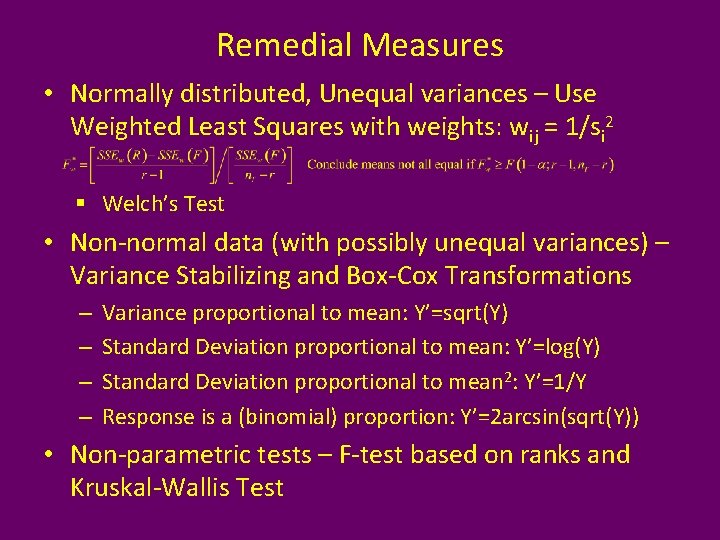
Remedial Measures • Normally distributed, Unequal variances – Use Weighted Least Squares with weights: wij = 1/si 2 § Welch’s Test • Non-normal data (with possibly unequal variances) – Variance Stabilizing and Box-Cox Transformations – – Variance proportional to mean: Y’=sqrt(Y) Standard Deviation proportional to mean: Y’=log(Y) Standard Deviation proportional to mean 2: Y’=1/Y Response is a (binomial) proportion: Y’=2 arcsin(sqrt(Y)) • Non-parametric tests – F-test based on ranks and Kruskal-Wallis Test
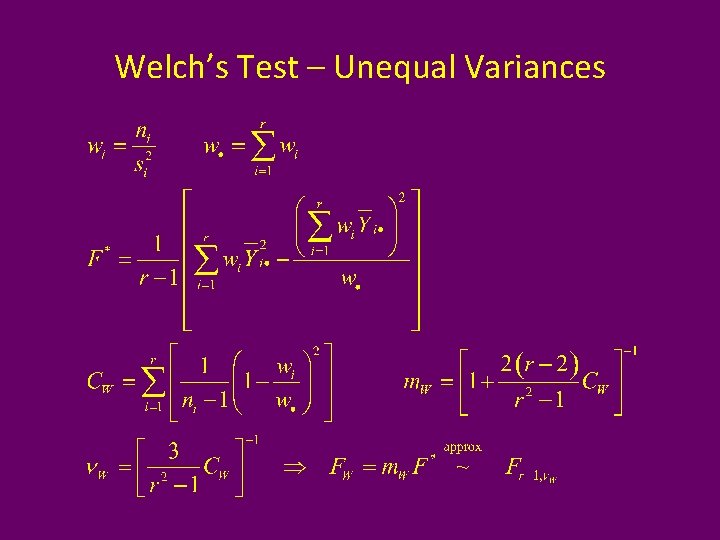
Welch’s Test – Unequal Variances
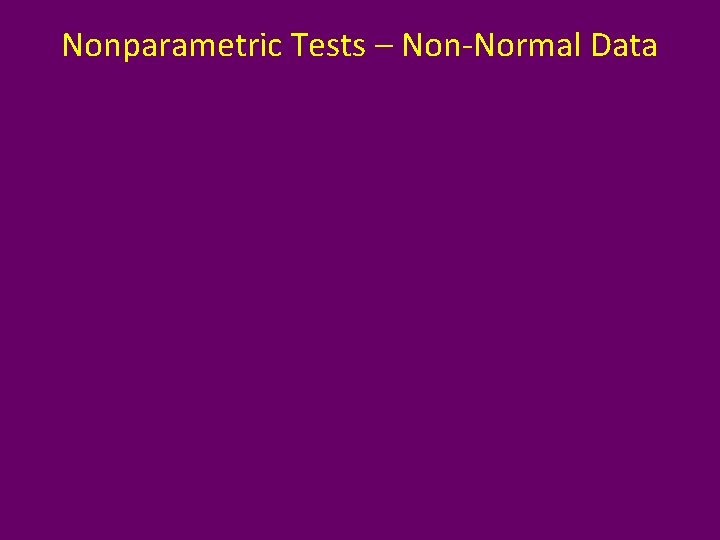
Nonparametric Tests – Non-Normal Data
Paradigm shift from women studies to gender studies
Two main clauses
Independent demand inventory management
Independent demand inventory management
Inventory models for independent demand
Independant demand
Independent school tuition models
What is independent demand
Difference between model and semi modals
Malaysian studies chapter 1
Social 9 chapter 3 test
Chapter 2 social studies grade 7
Chapter 13 experiments and observational studies
Mississippi studies chapter 1 assignments
Mississippi studies
Mississippi studies chapter 1
Chapter 7 lesson 2 social studies
Matched pairs design statistics
Ms studies chapter 1
Chapter 4 designing studies
Social studies chapter 3
Social studies chapter 3
Hungarian algorithm worksheet
Sampling distribution models chapter 17
Ap stats chapter 17 sampling distribution models
Ap stats chapter 17 sampling distribution models