Autocorrelation in Time Series KNNL Chapter 12 Issues
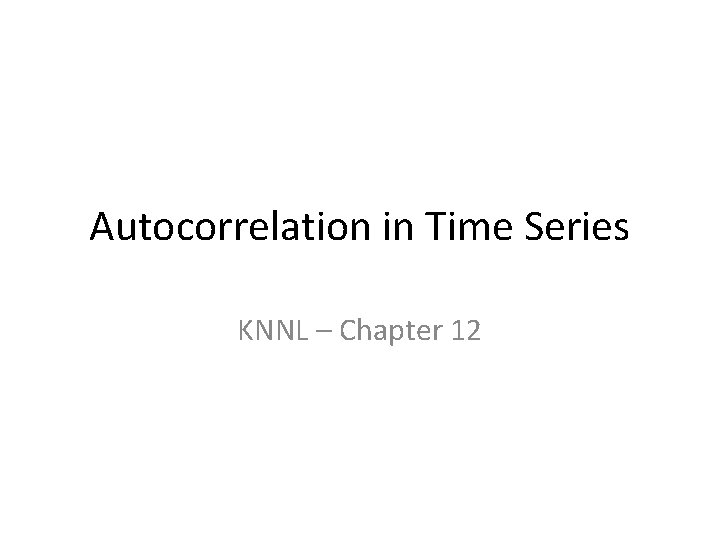
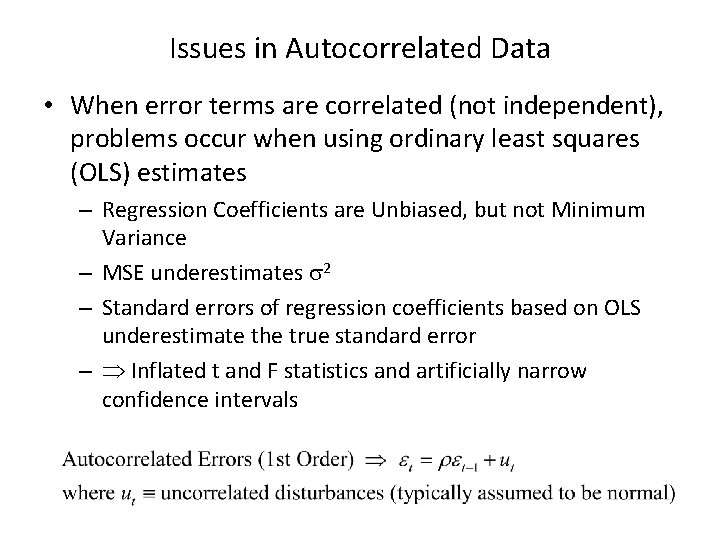
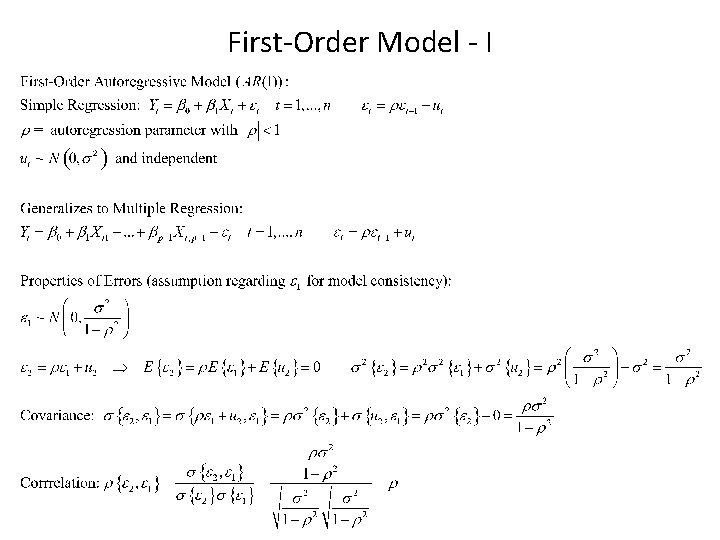
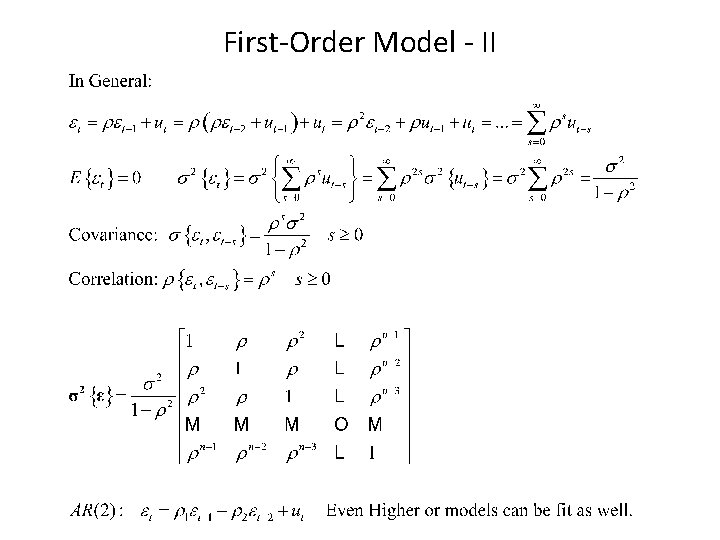
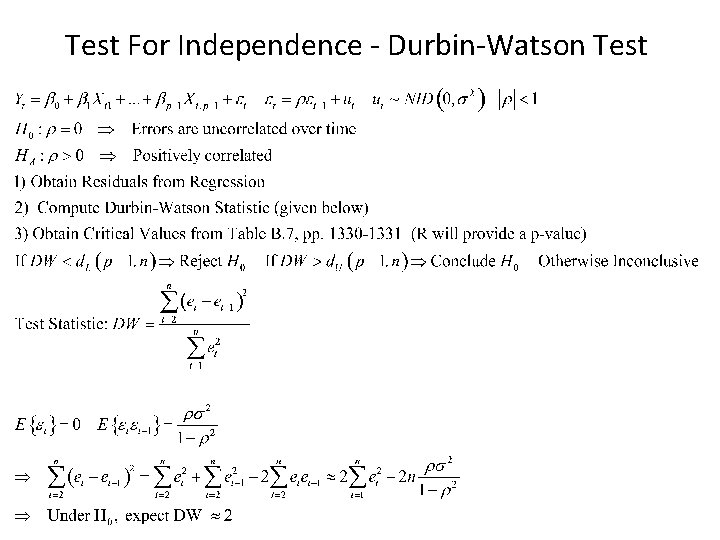
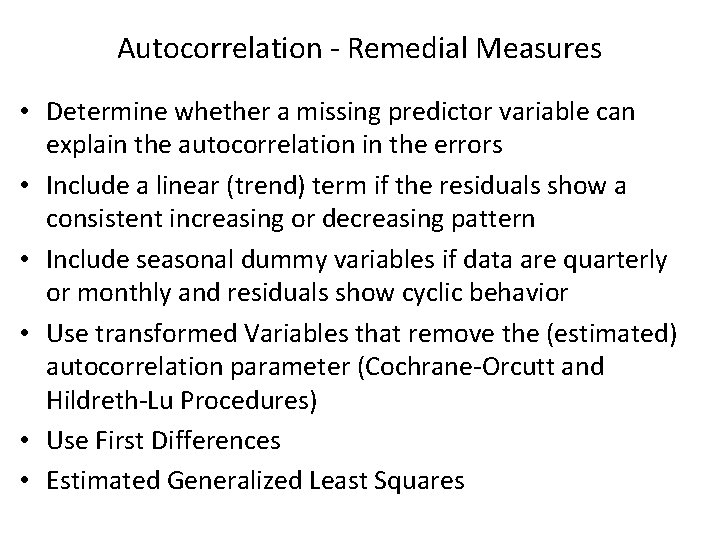
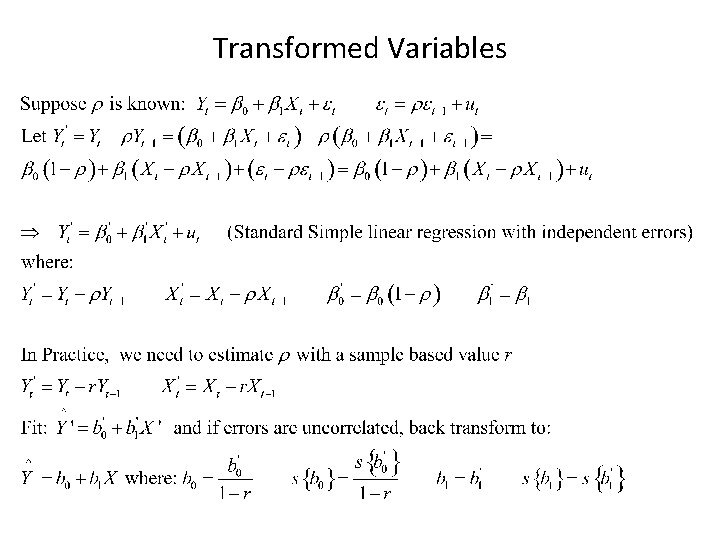
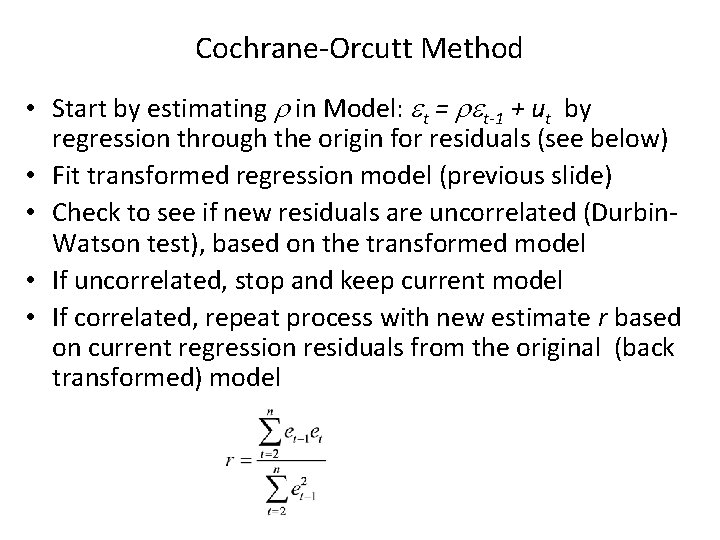
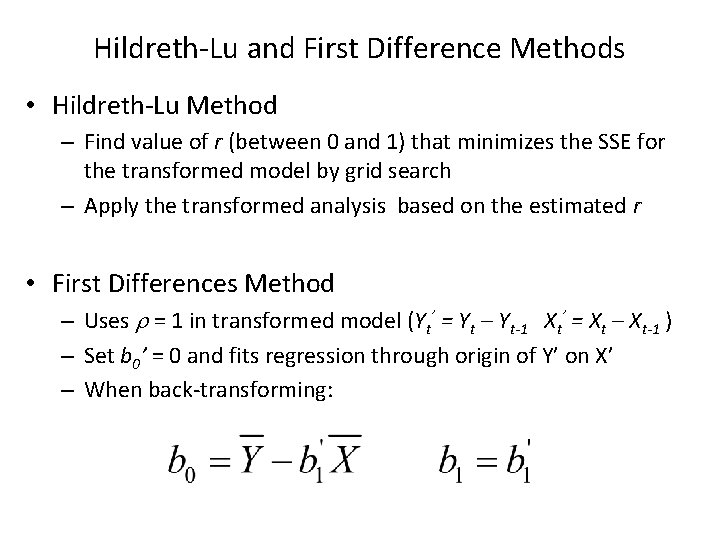
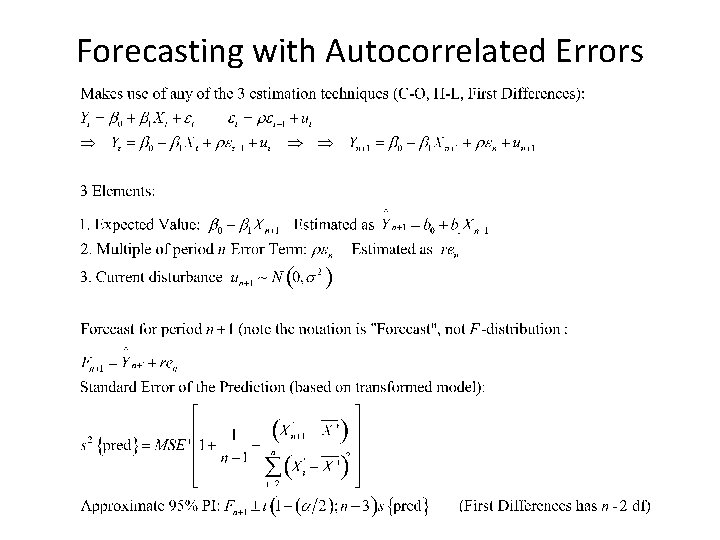
- Slides: 10
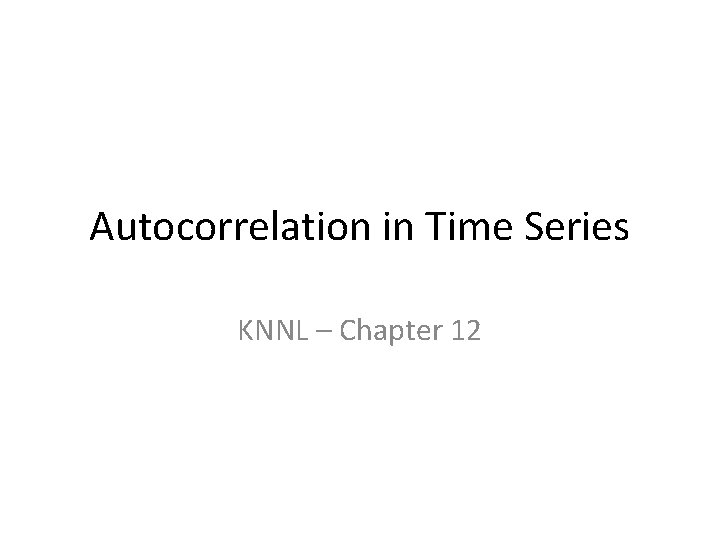
Autocorrelation in Time Series KNNL – Chapter 12
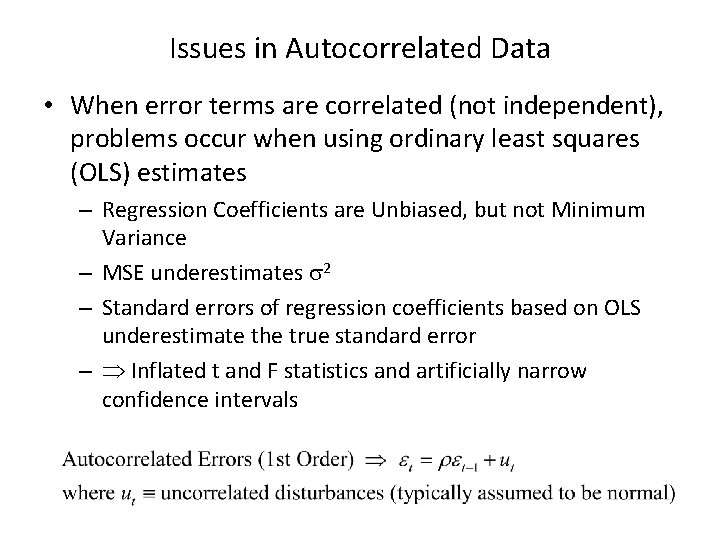
Issues in Autocorrelated Data • When error terms are correlated (not independent), problems occur when using ordinary least squares (OLS) estimates – Regression Coefficients are Unbiased, but not Minimum Variance – MSE underestimates s 2 – Standard errors of regression coefficients based on OLS underestimate the true standard error – Inflated t and F statistics and artificially narrow confidence intervals
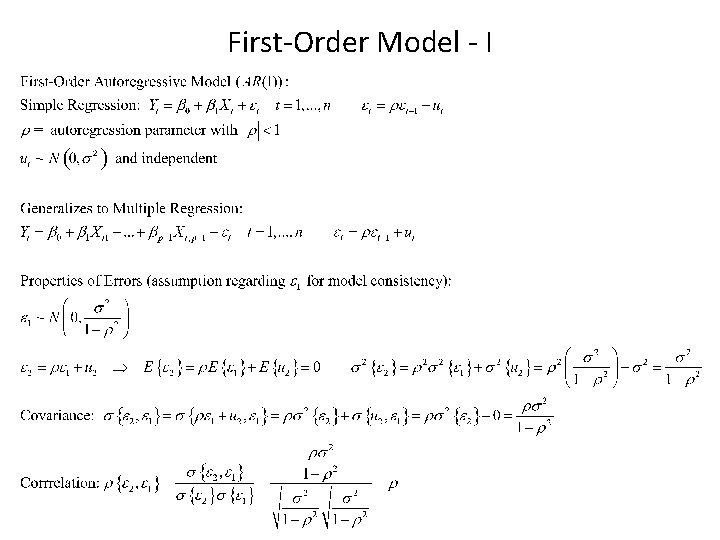
First-Order Model - I
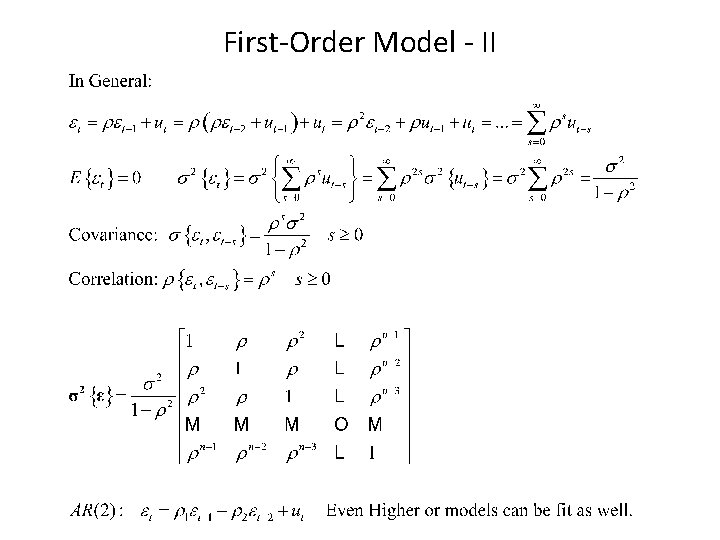
First-Order Model - II
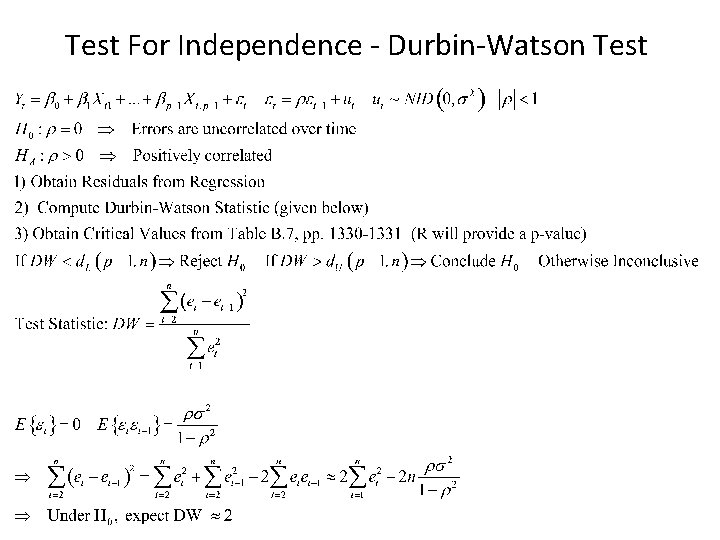
Test For Independence - Durbin-Watson Test
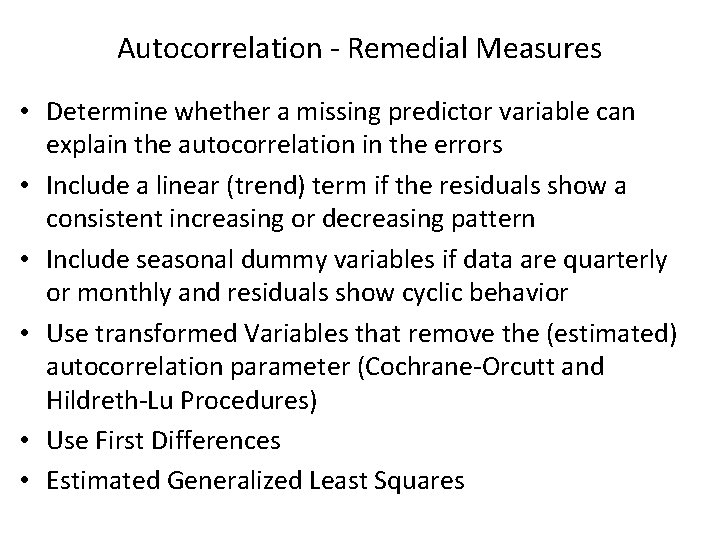
Autocorrelation - Remedial Measures • Determine whether a missing predictor variable can explain the autocorrelation in the errors • Include a linear (trend) term if the residuals show a consistent increasing or decreasing pattern • Include seasonal dummy variables if data are quarterly or monthly and residuals show cyclic behavior • Use transformed Variables that remove the (estimated) autocorrelation parameter (Cochrane-Orcutt and Hildreth-Lu Procedures) • Use First Differences • Estimated Generalized Least Squares
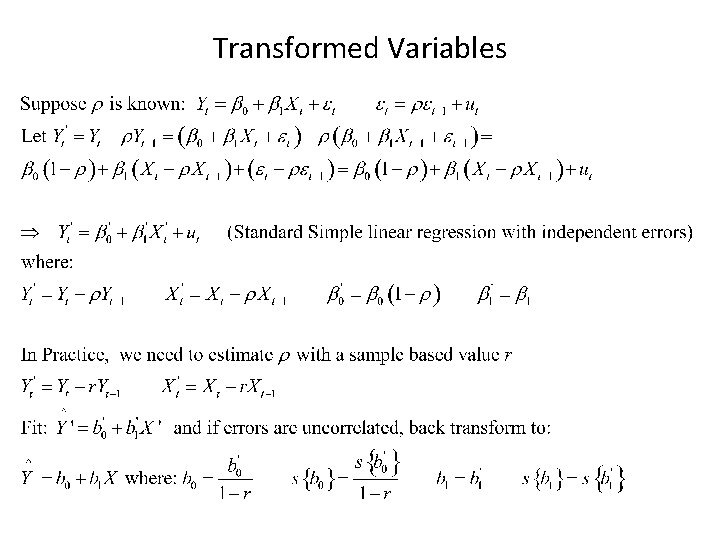
Transformed Variables
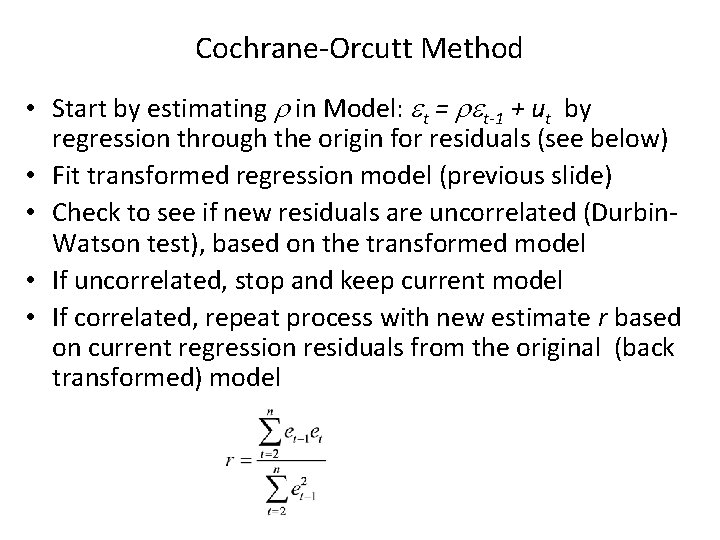
Cochrane-Orcutt Method • Start by estimating r in Model: et = ret-1 + ut by regression through the origin for residuals (see below) • Fit transformed regression model (previous slide) • Check to see if new residuals are uncorrelated (Durbin. Watson test), based on the transformed model • If uncorrelated, stop and keep current model • If correlated, repeat process with new estimate r based on current regression residuals from the original (back transformed) model
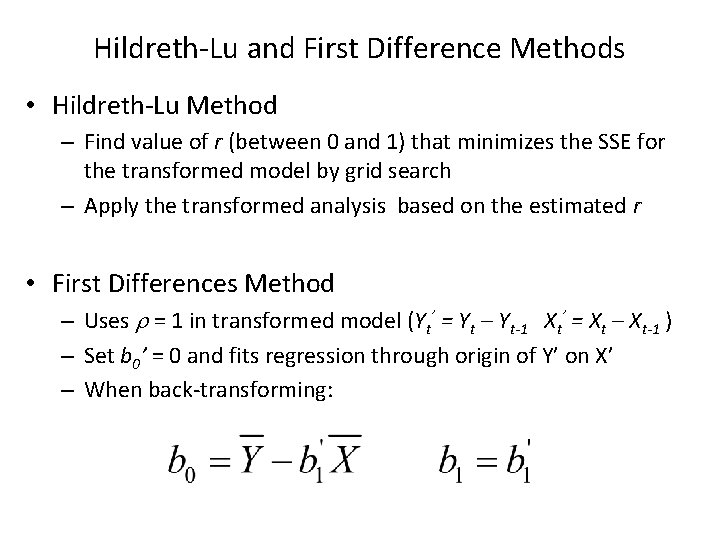
Hildreth-Lu and First Difference Methods • Hildreth-Lu Method – Find value of r (between 0 and 1) that minimizes the SSE for the transformed model by grid search – Apply the transformed analysis based on the estimated r • First Differences Method – Uses r = 1 in transformed model (Yt’ = Yt – Yt-1 Xt’ = Xt – Xt-1 ) – Set b 0’ = 0 and fits regression through origin of Y’ on X’ – When back-transforming:
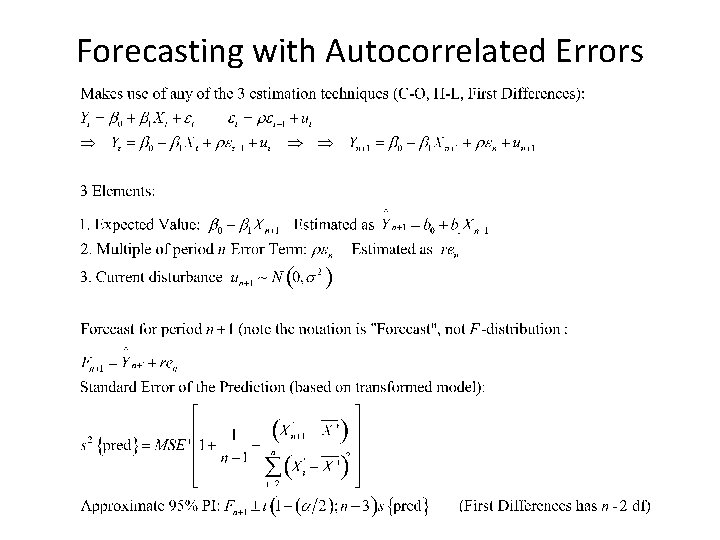
Forecasting with Autocorrelated Errors