PCA for analysis of complex multivariate data Interpretation
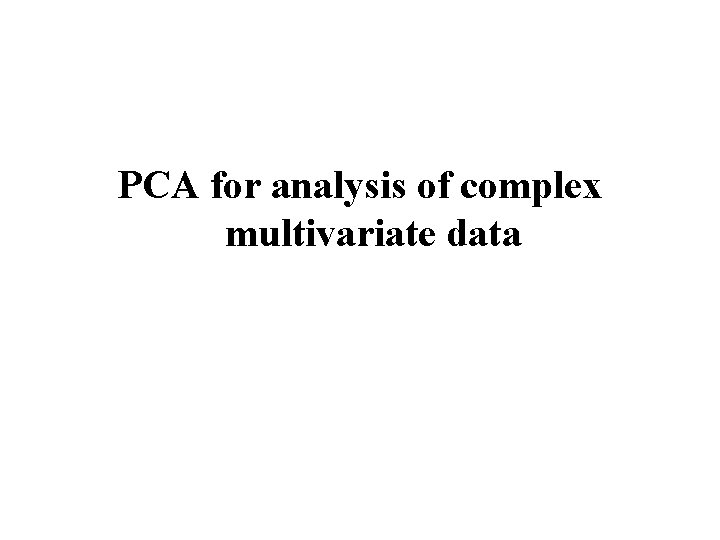
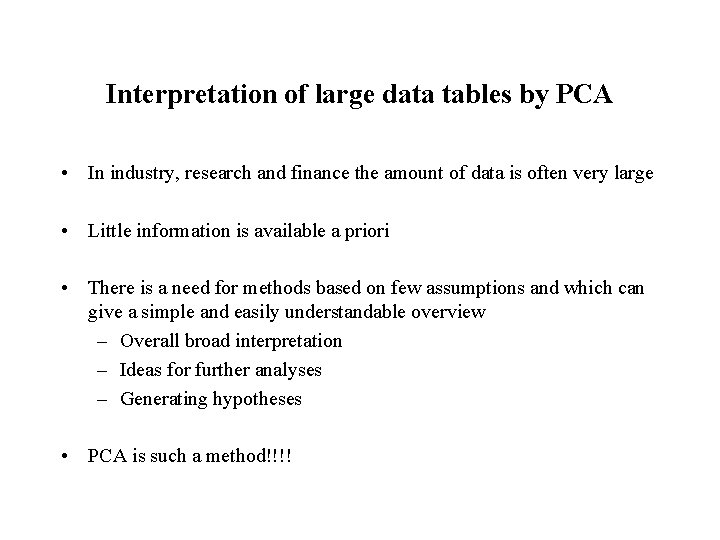
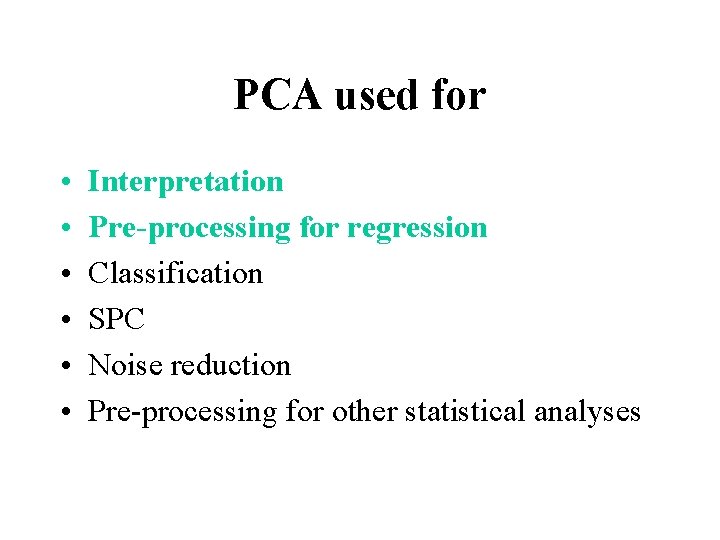
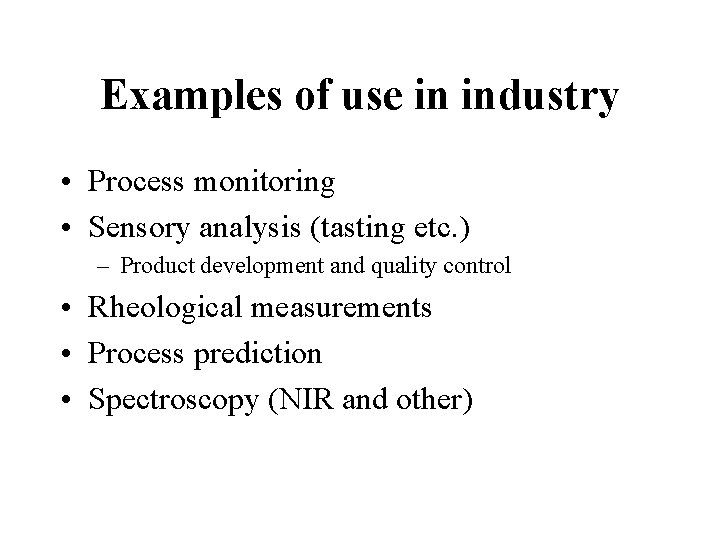
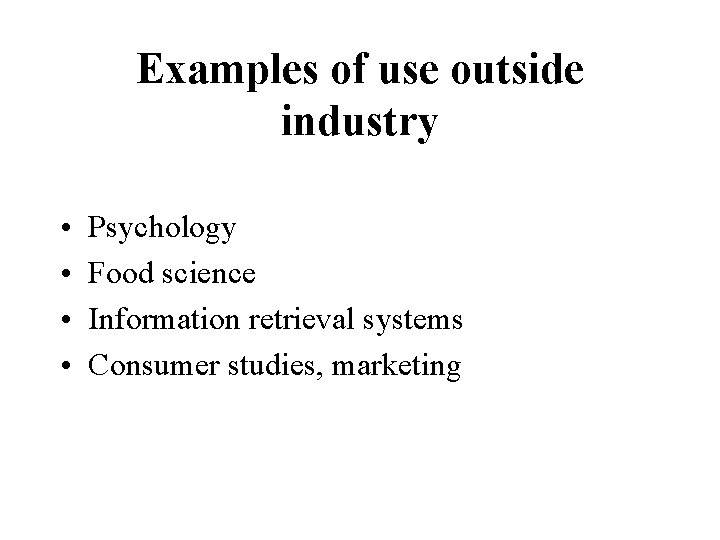
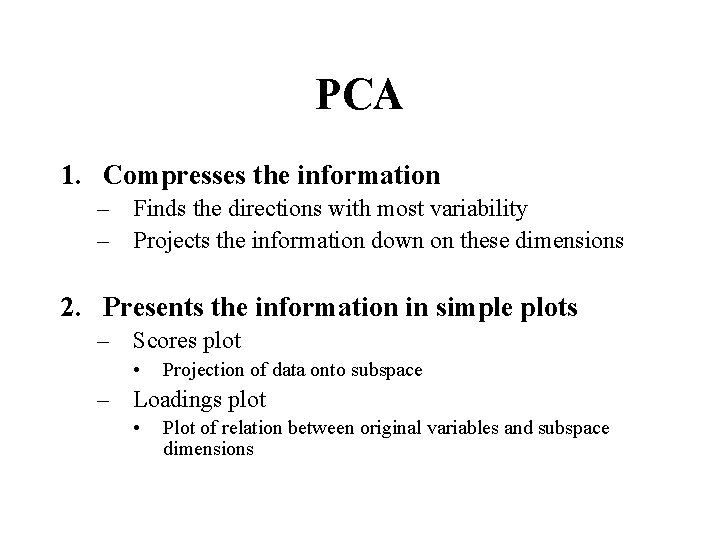
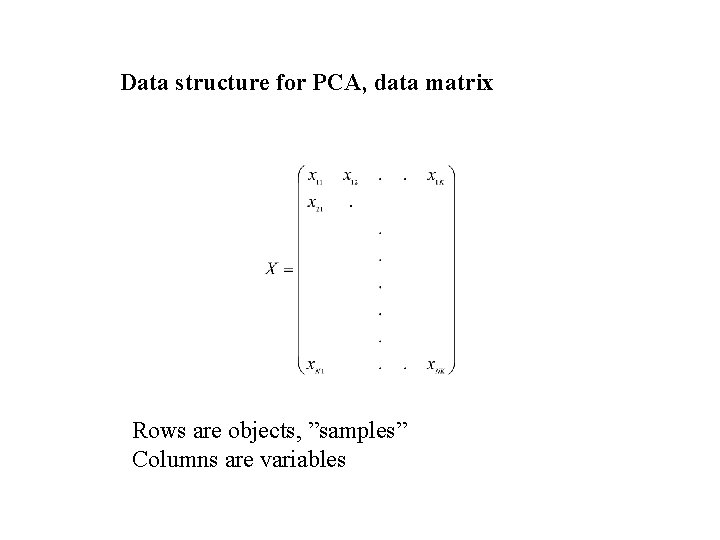
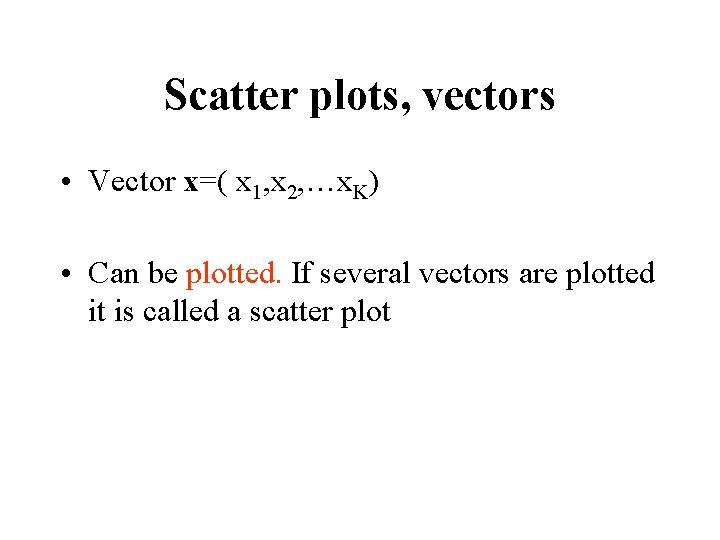
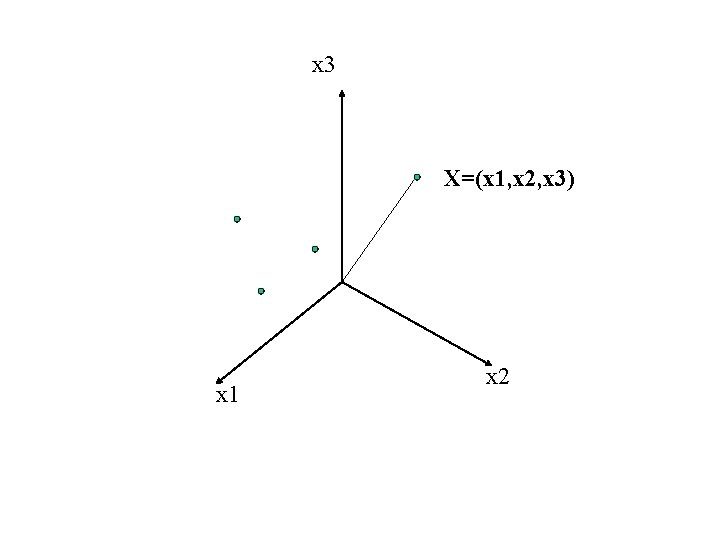
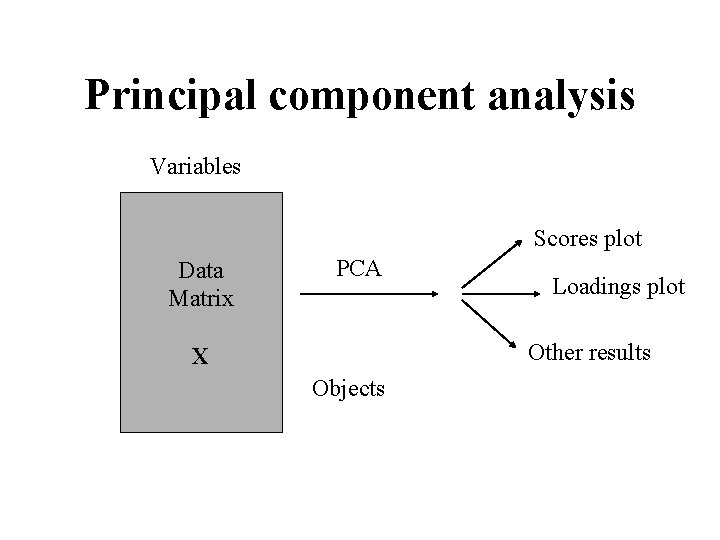
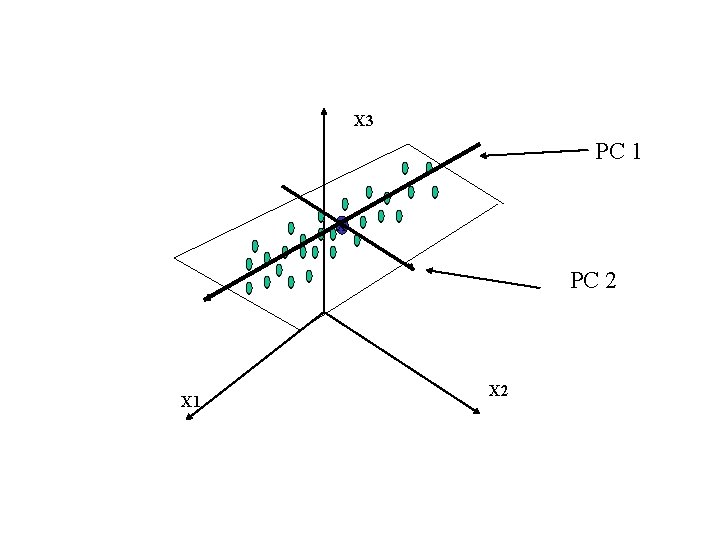
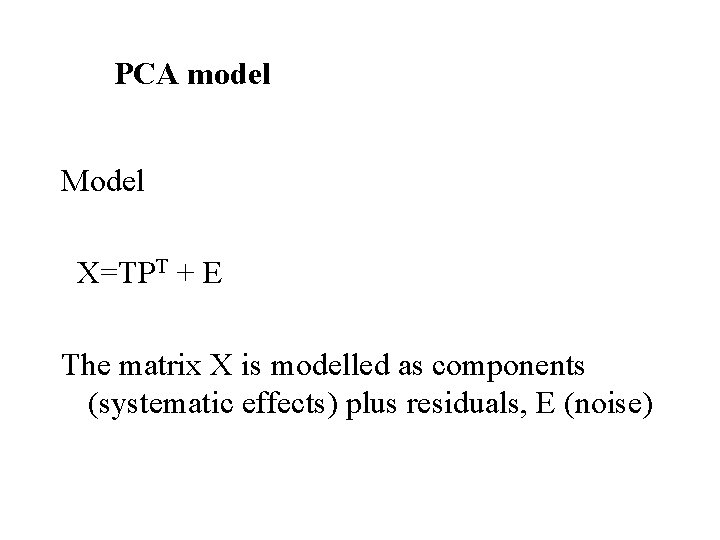
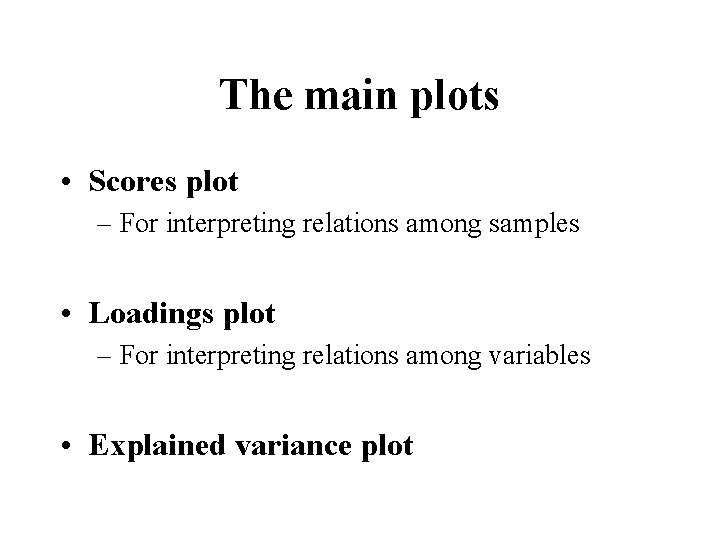
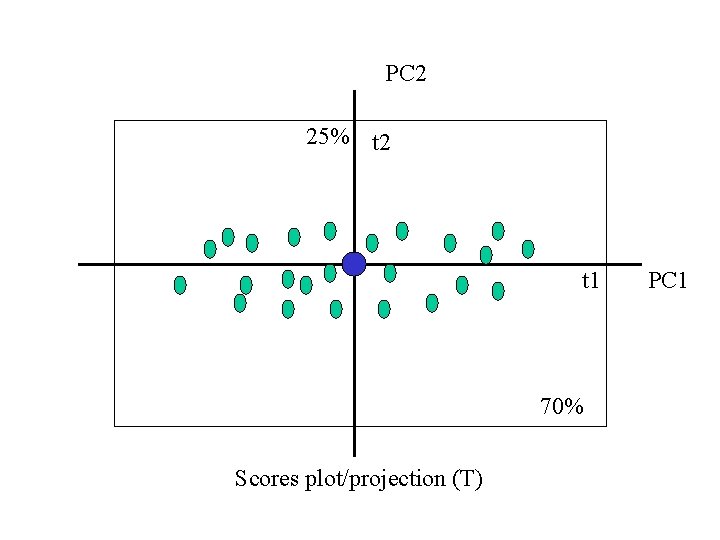
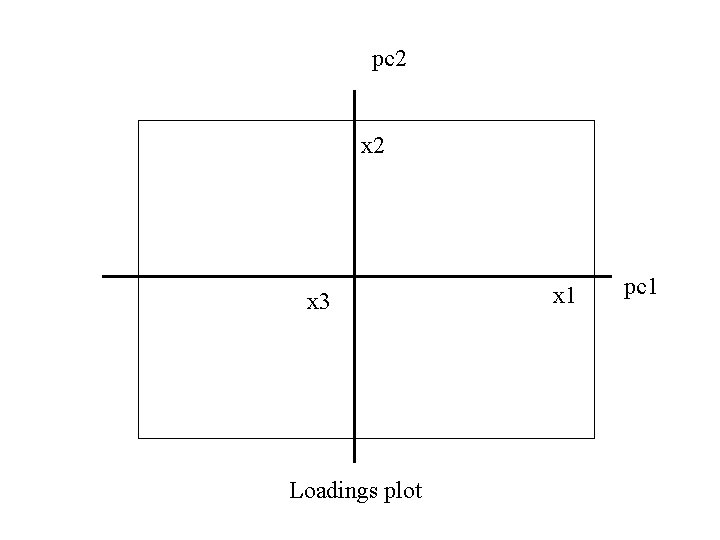
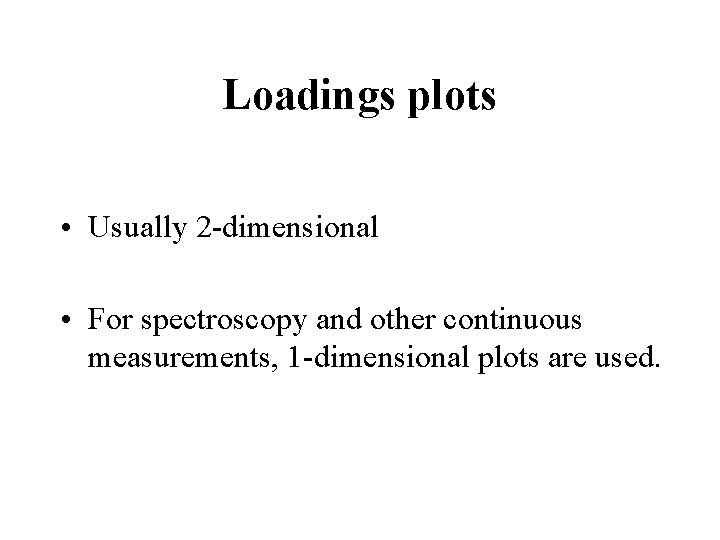
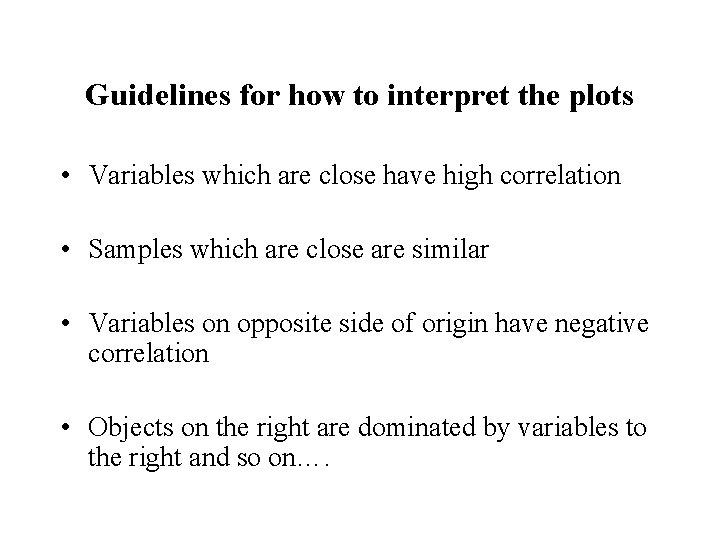
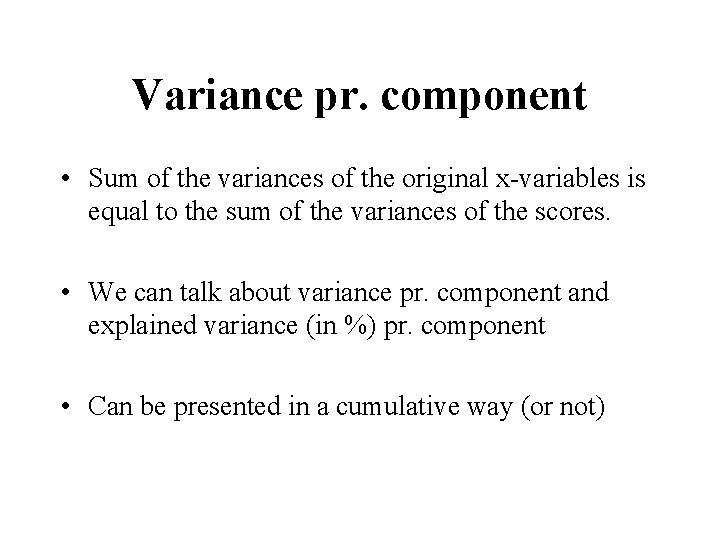
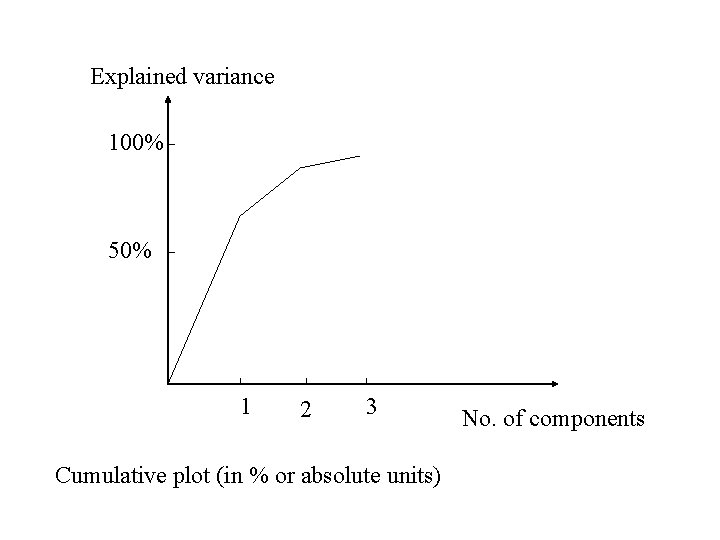
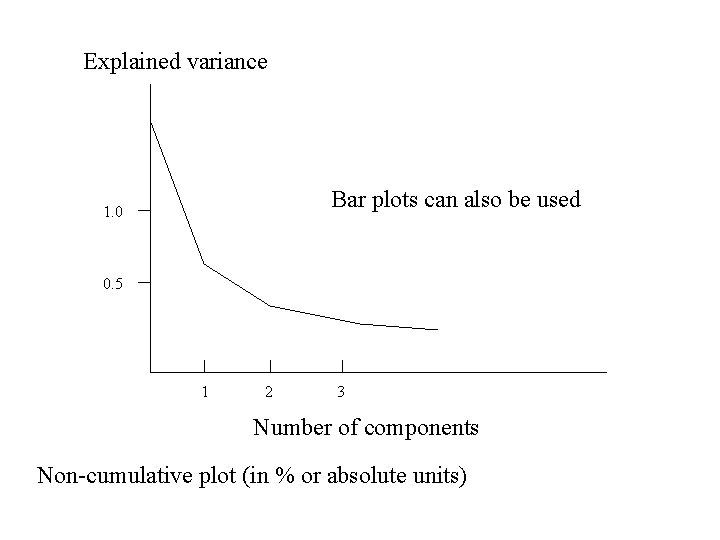
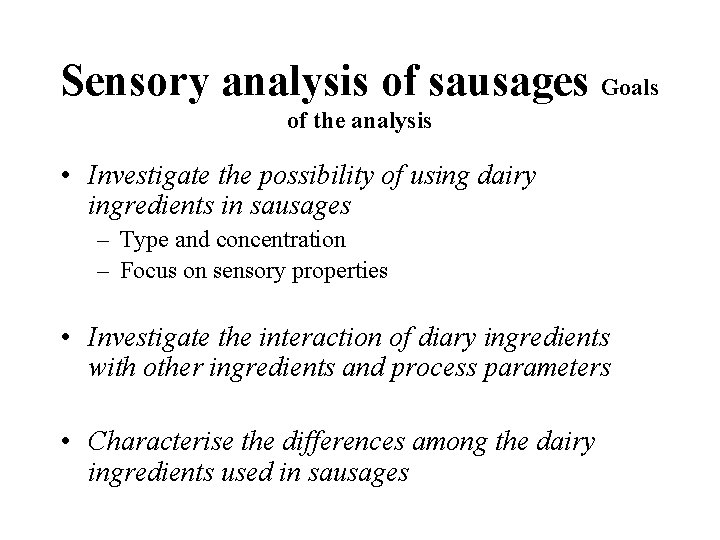
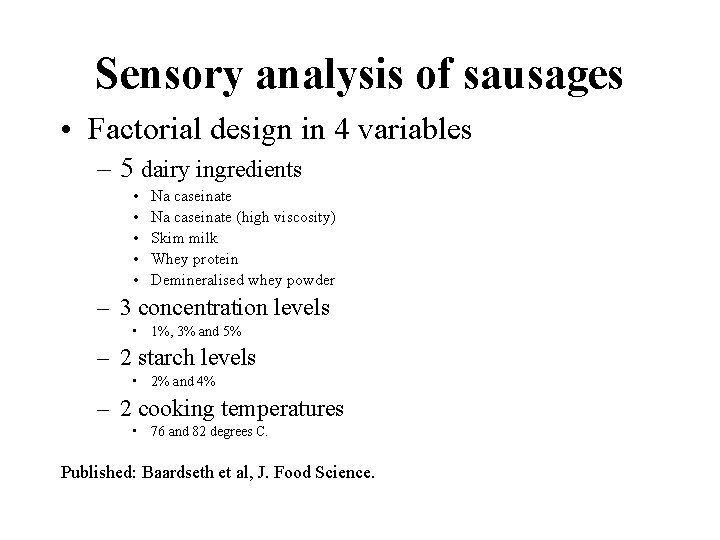
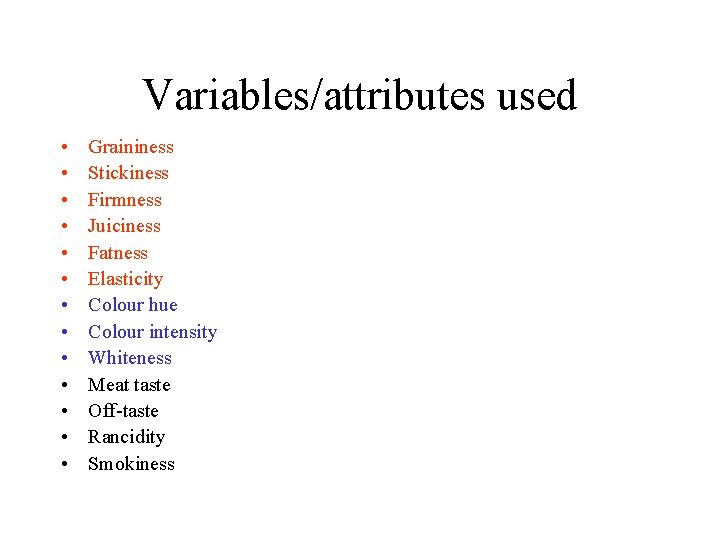
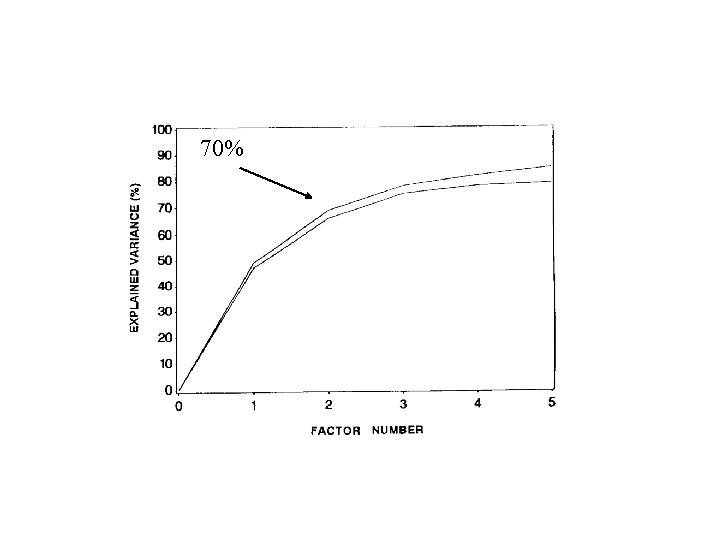
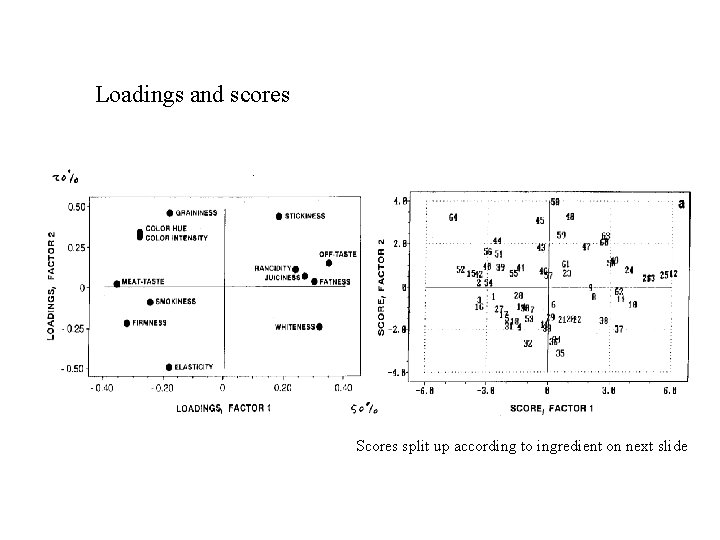
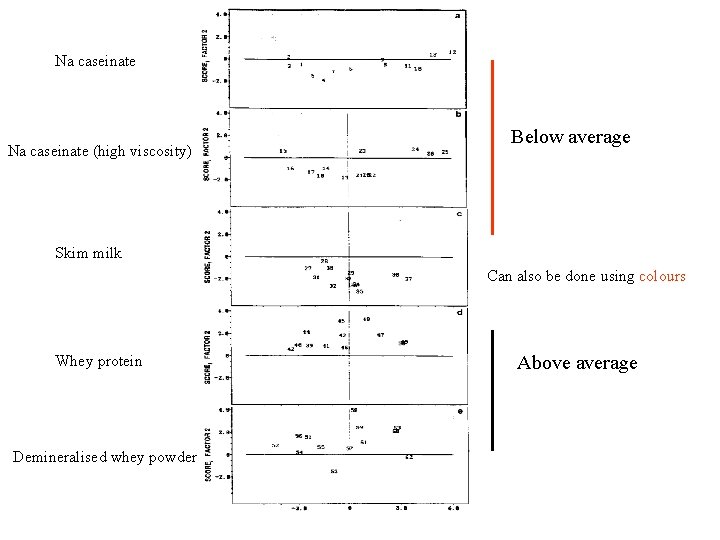
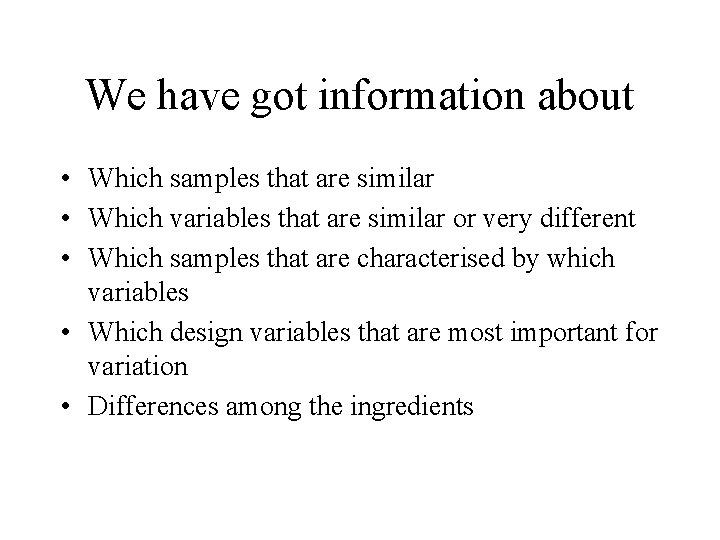
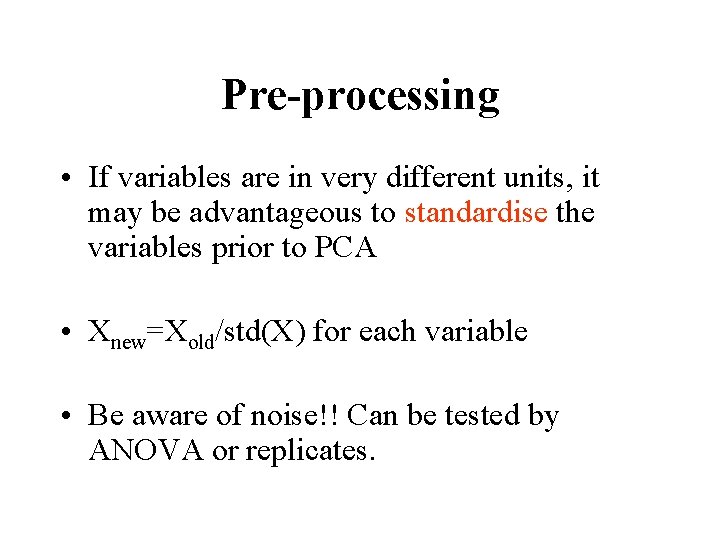
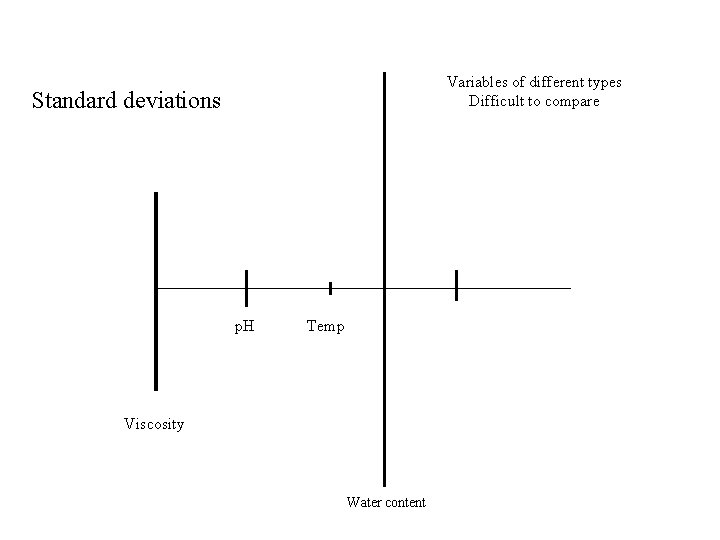
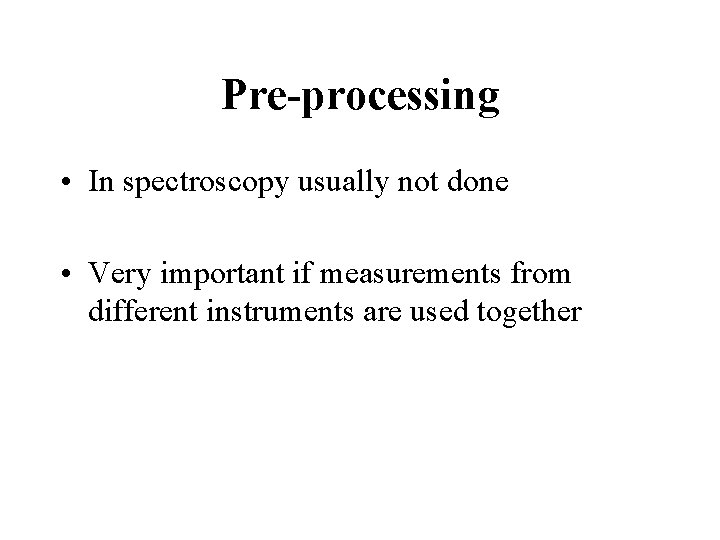
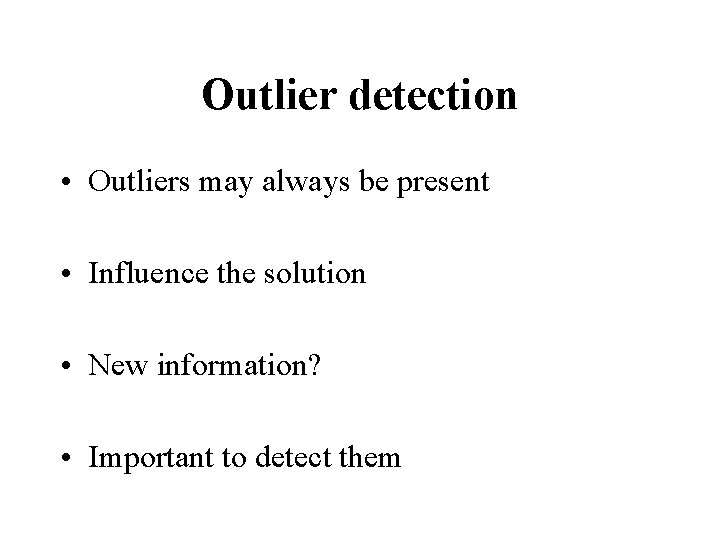
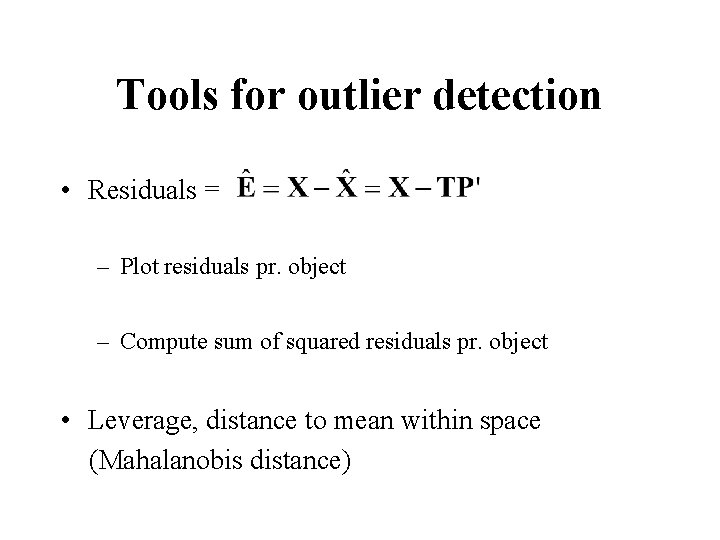
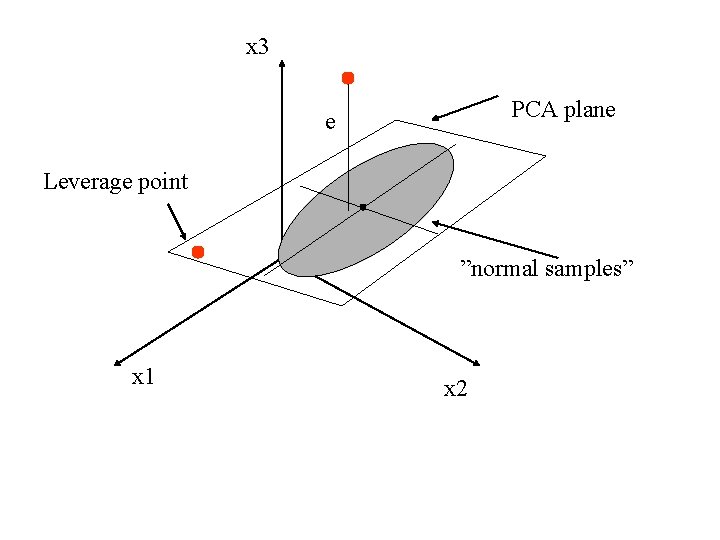
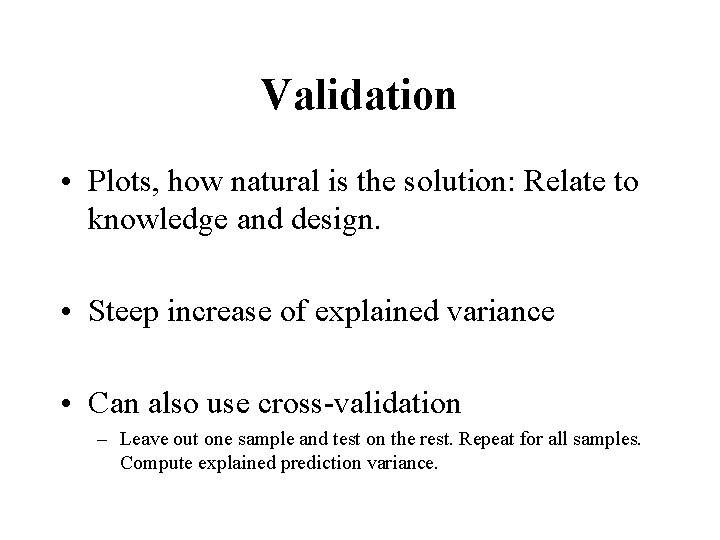
- Slides: 34
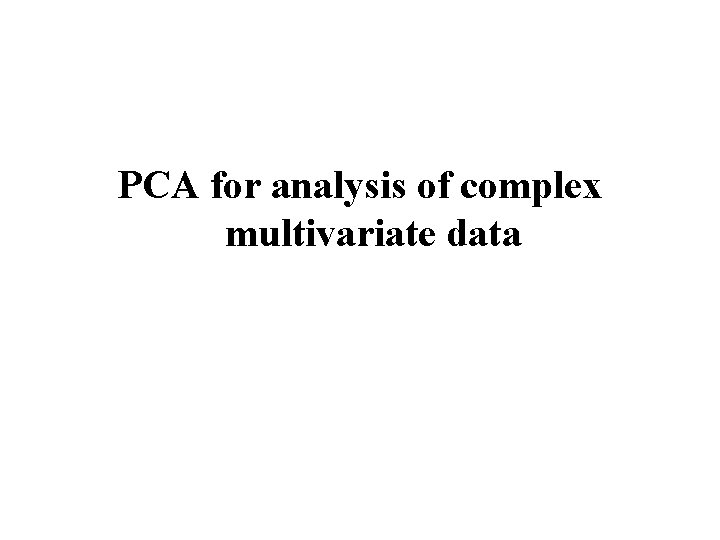
PCA for analysis of complex multivariate data
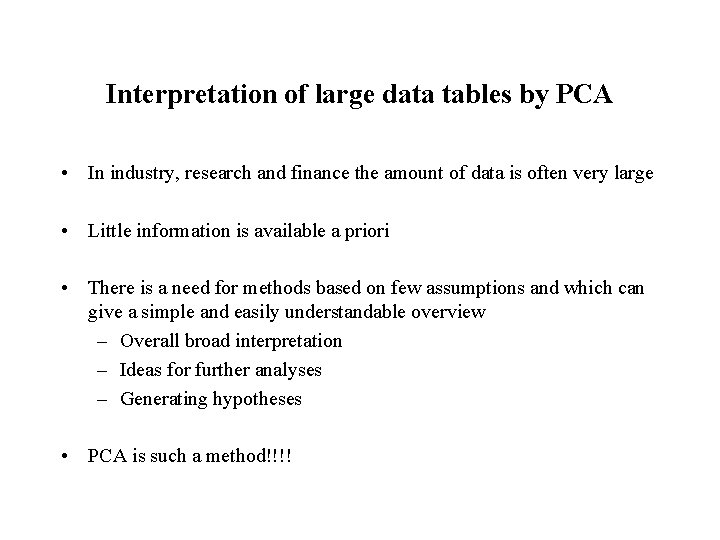
Interpretation of large data tables by PCA • In industry, research and finance the amount of data is often very large • Little information is available a priori • There is a need for methods based on few assumptions and which can give a simple and easily understandable overview – Overall broad interpretation – Ideas for further analyses – Generating hypotheses • PCA is such a method!!!!
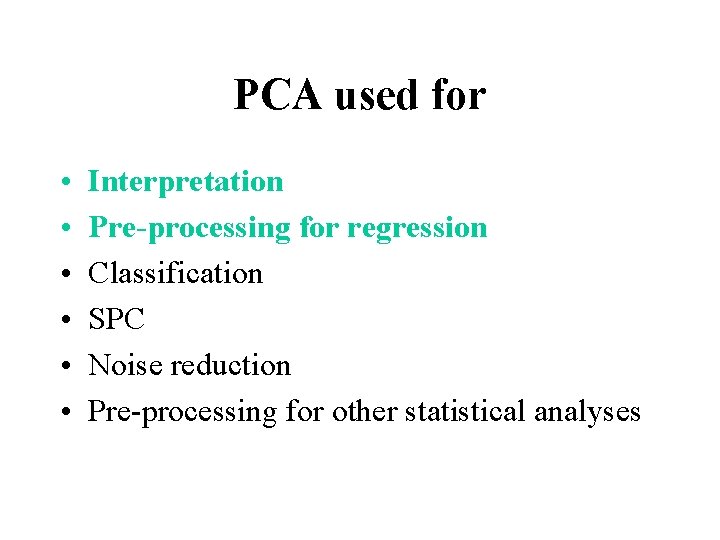
PCA used for • • • Interpretation Pre-processing for regression Classification SPC Noise reduction Pre-processing for other statistical analyses
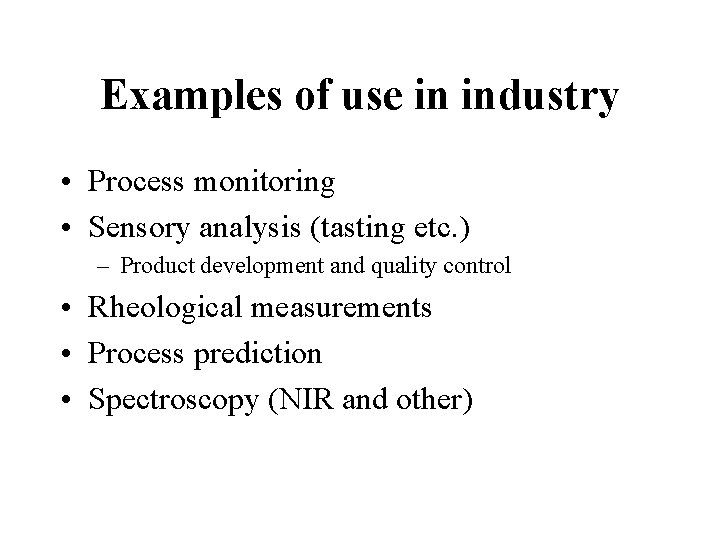
Examples of use in industry • Process monitoring • Sensory analysis (tasting etc. ) – Product development and quality control • Rheological measurements • Process prediction • Spectroscopy (NIR and other)
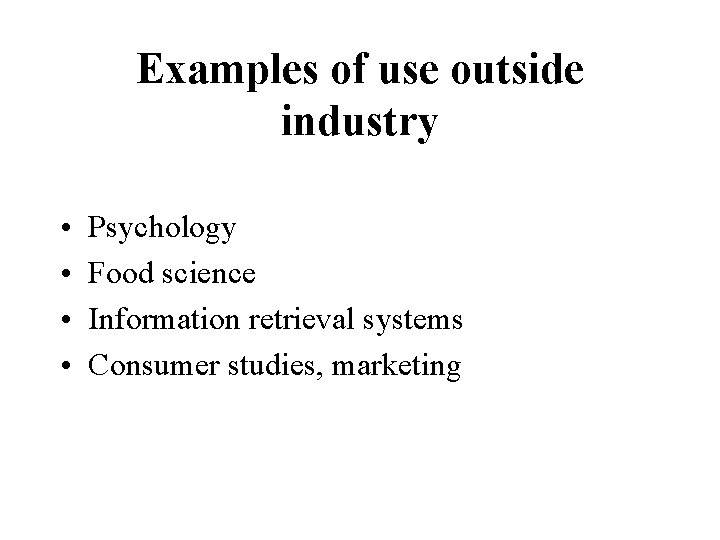
Examples of use outside industry • • Psychology Food science Information retrieval systems Consumer studies, marketing
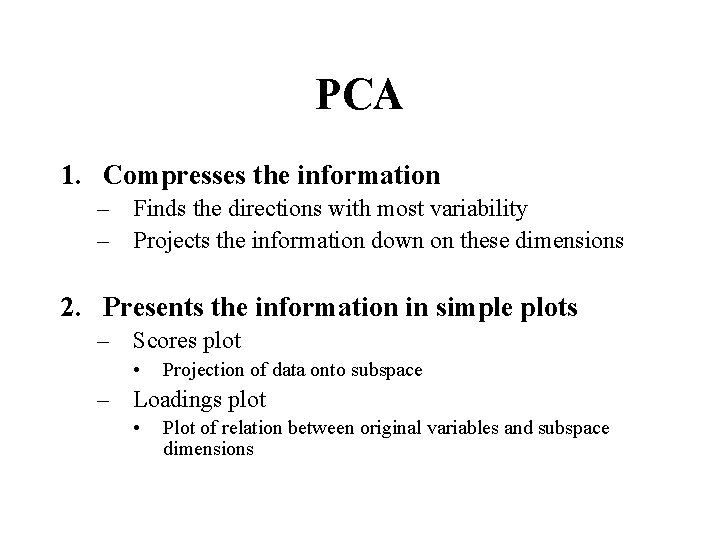
PCA 1. Compresses the information – Finds the directions with most variability – Projects the information down on these dimensions 2. Presents the information in simple plots – Scores plot • Projection of data onto subspace – Loadings plot • Plot of relation between original variables and subspace dimensions
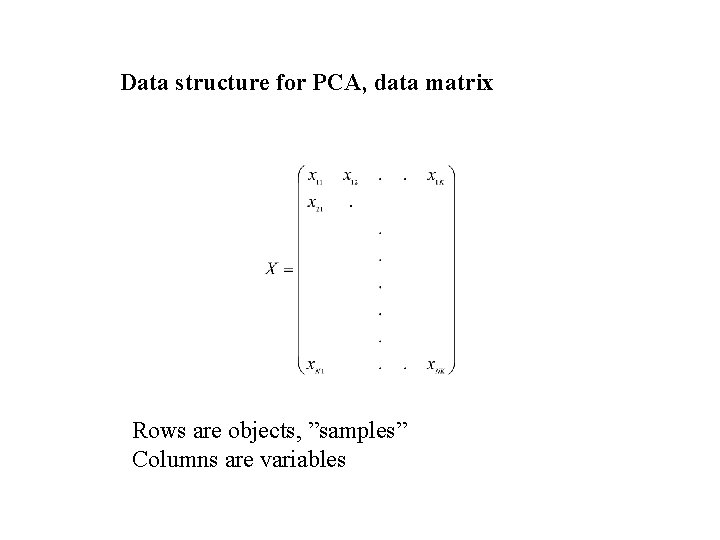
Data structure for PCA, data matrix Rows are objects, ”samples” Columns are variables
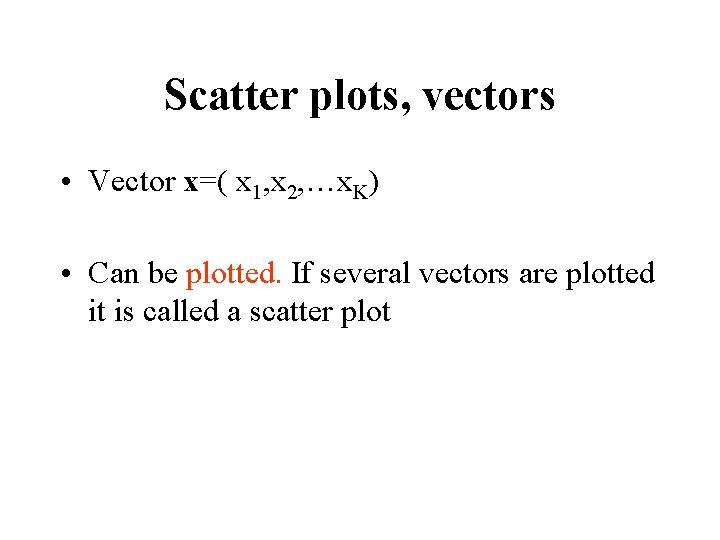
Scatter plots, vectors • Vector x=( x 1, x 2, …x. K) • Can be plotted. If several vectors are plotted it is called a scatter plot
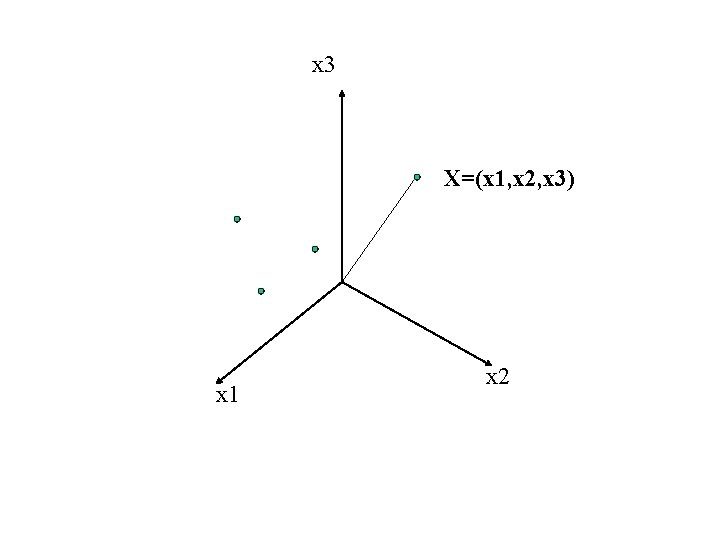
x 3 X=(x 1, x 2, x 3) x 1 x 2
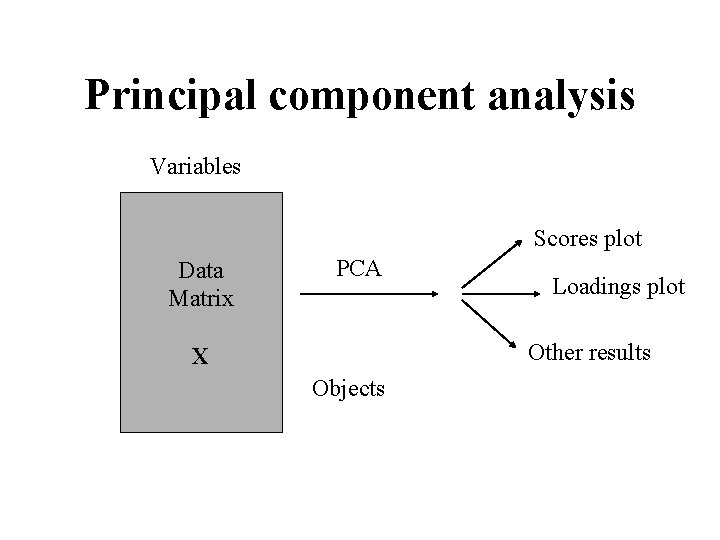
Principal component analysis Variables Scores plot Data Matrix PCA Loadings plot Other results X Objects
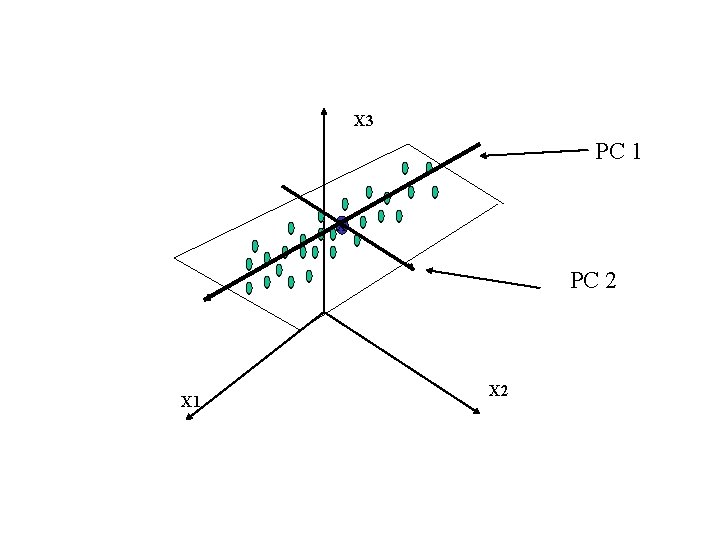
X 3 PC 1 X PC 2 X 1 X 2
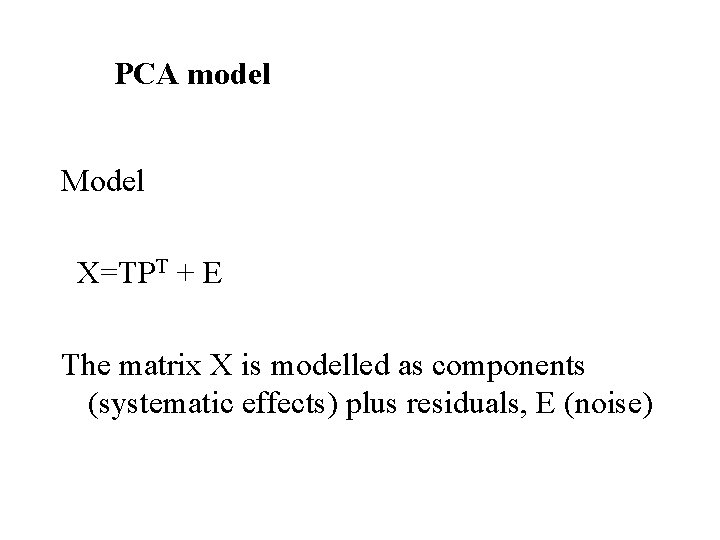
PCA model Model X=TPT + E The matrix X is modelled as components (systematic effects) plus residuals, E (noise)
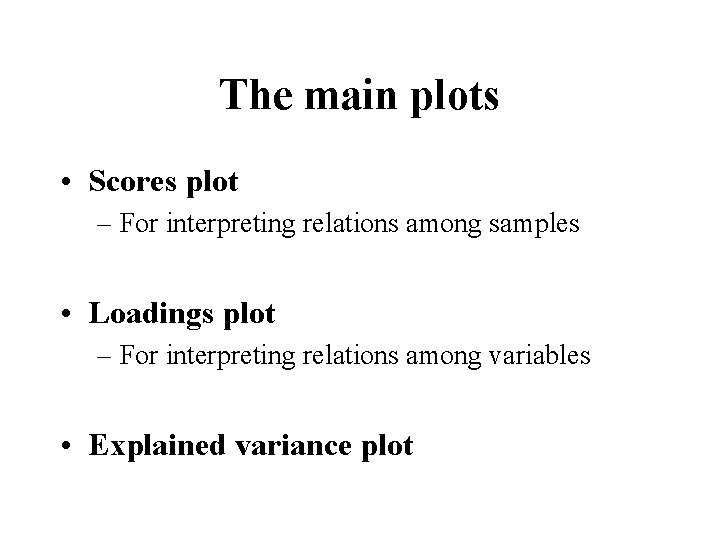
The main plots • Scores plot – For interpreting relations among samples • Loadings plot – For interpreting relations among variables • Explained variance plot
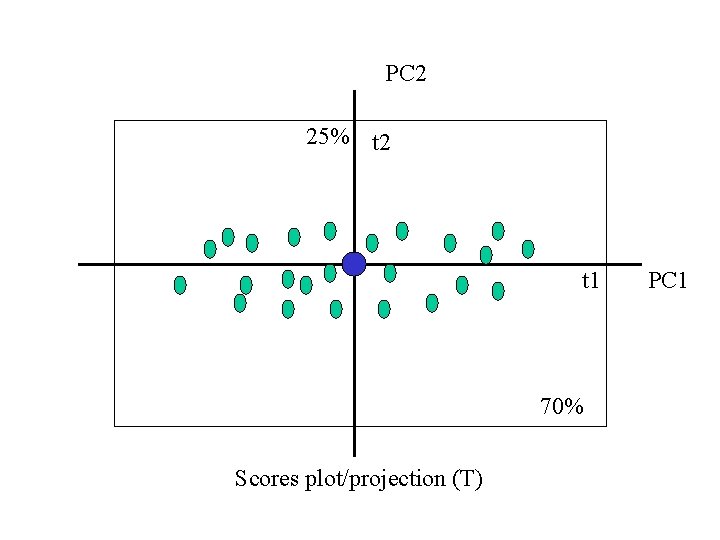
PC 2 25% t 2 t 1 70% Scores plot/projection (T) PC 1
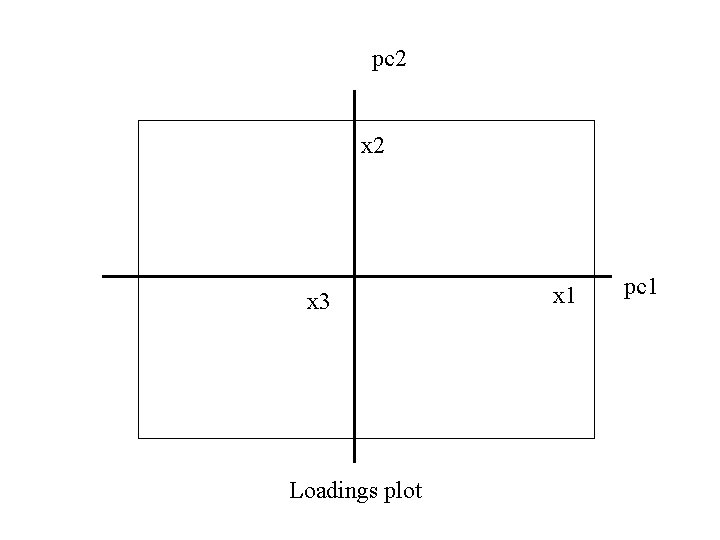
pc 2 x 3 Loadings plot x 1 pc 1
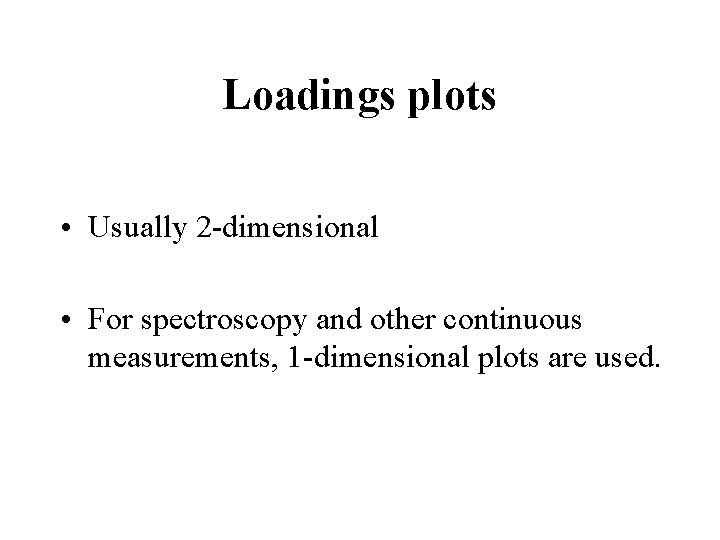
Loadings plots • Usually 2 -dimensional • For spectroscopy and other continuous measurements, 1 -dimensional plots are used.
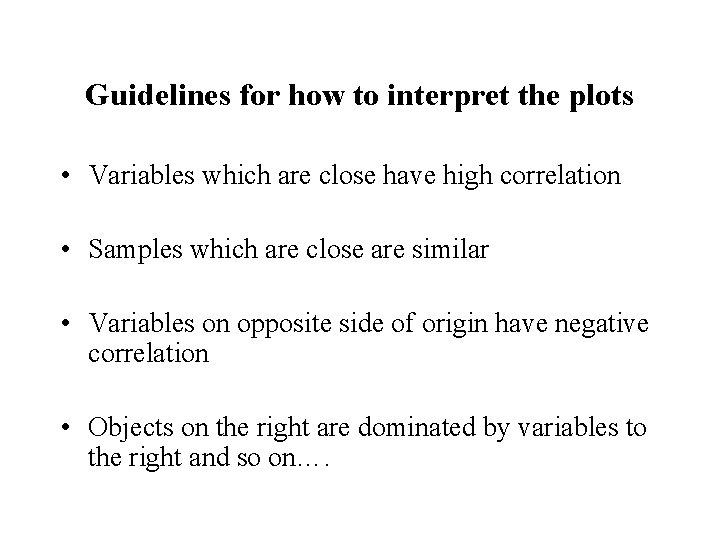
Guidelines for how to interpret the plots • Variables which are close have high correlation • Samples which are close are similar • Variables on opposite side of origin have negative correlation • Objects on the right are dominated by variables to the right and so on….
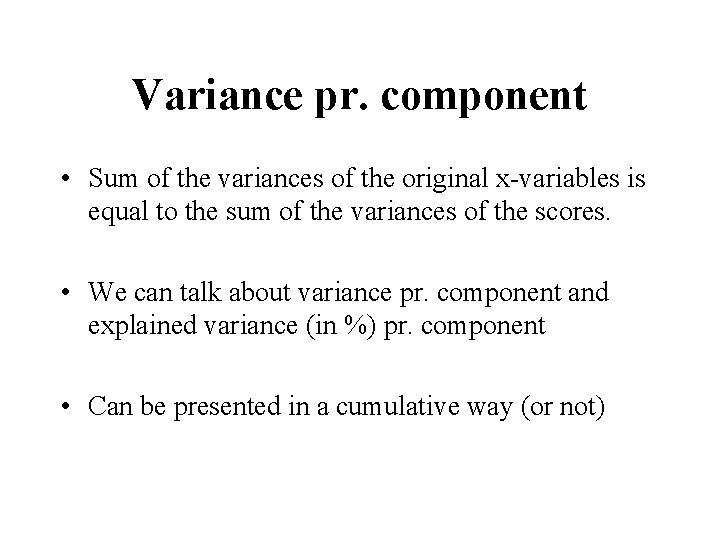
Variance pr. component • Sum of the variances of the original x-variables is equal to the sum of the variances of the scores. • We can talk about variance pr. component and explained variance (in %) pr. component • Can be presented in a cumulative way (or not)
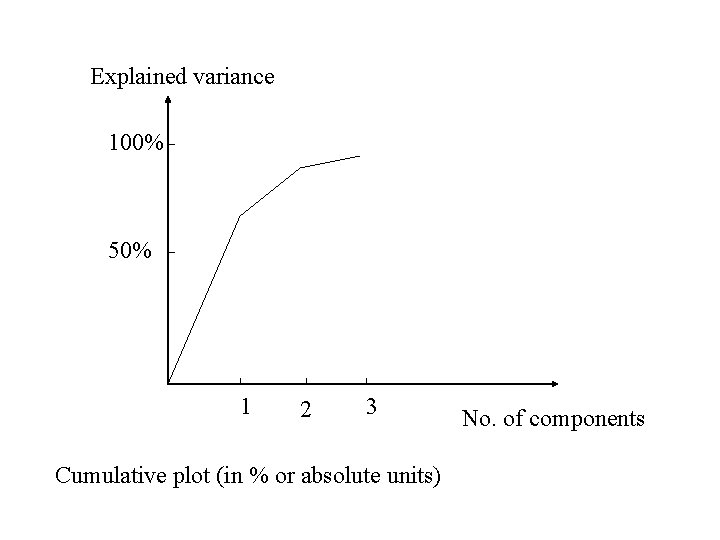
Explained variance 100% 50% 1 2 3 Cumulative plot (in % or absolute units) No. of components
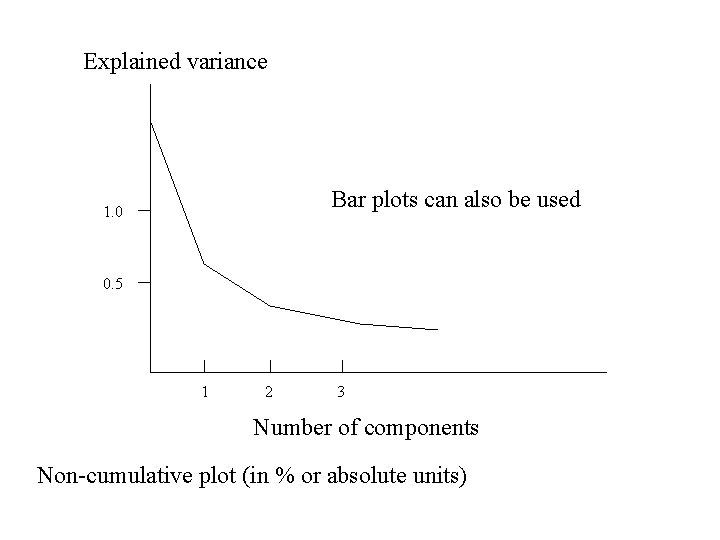
Explained variance Bar plots can also be used 1. 0 0. 5 1 2 3 Number of components Non-cumulative plot (in % or absolute units)
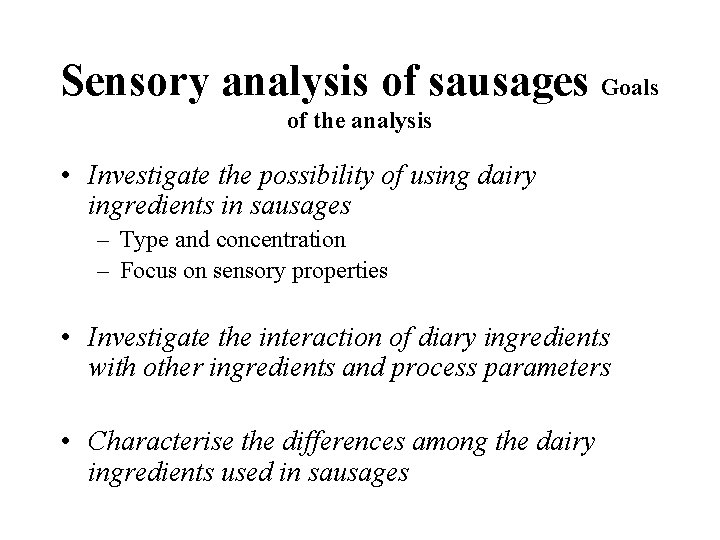
Sensory analysis of sausages Goals of the analysis • Investigate the possibility of using dairy ingredients in sausages – Type and concentration – Focus on sensory properties • Investigate the interaction of diary ingredients with other ingredients and process parameters • Characterise the differences among the dairy ingredients used in sausages
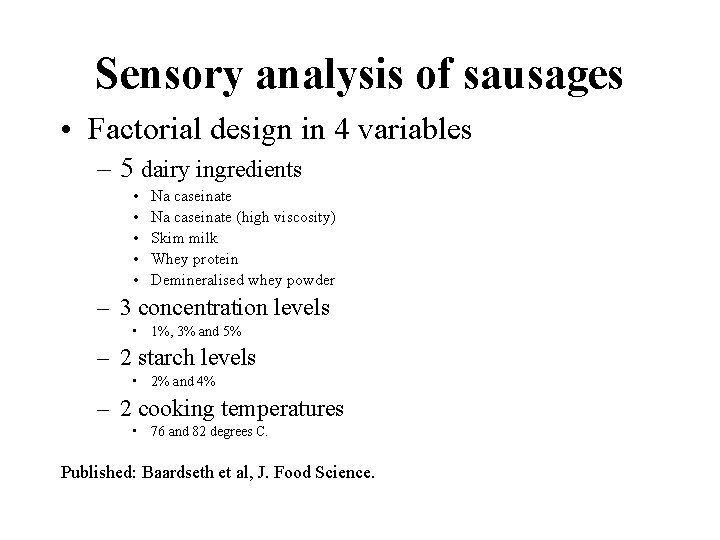
Sensory analysis of sausages • Factorial design in 4 variables – 5 dairy ingredients • • • Na caseinate (high viscosity) Skim milk Whey protein Demineralised whey powder – 3 concentration levels • 1%, 3% and 5% – 2 starch levels • 2% and 4% – 2 cooking temperatures • 76 and 82 degrees C. Published: Baardseth et al, J. Food Science.
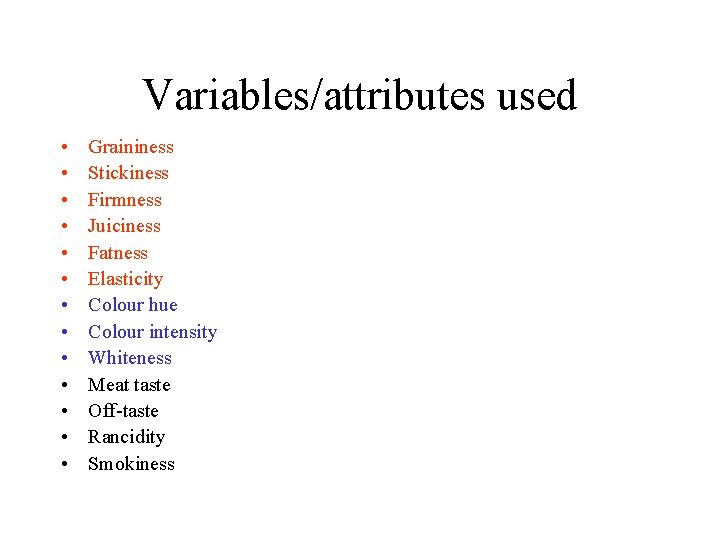
Variables/attributes used • • • • Graininess Stickiness Firmness Juiciness Fatness Elasticity Colour hue Colour intensity Whiteness Meat taste Off-taste Rancidity Smokiness
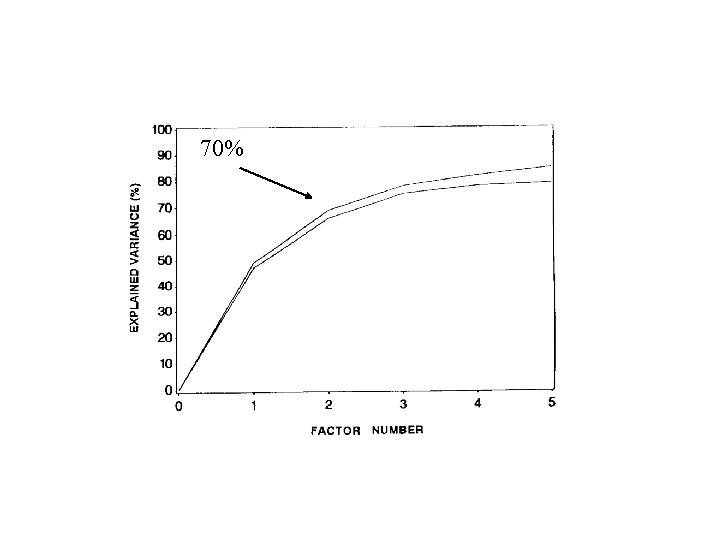
70%
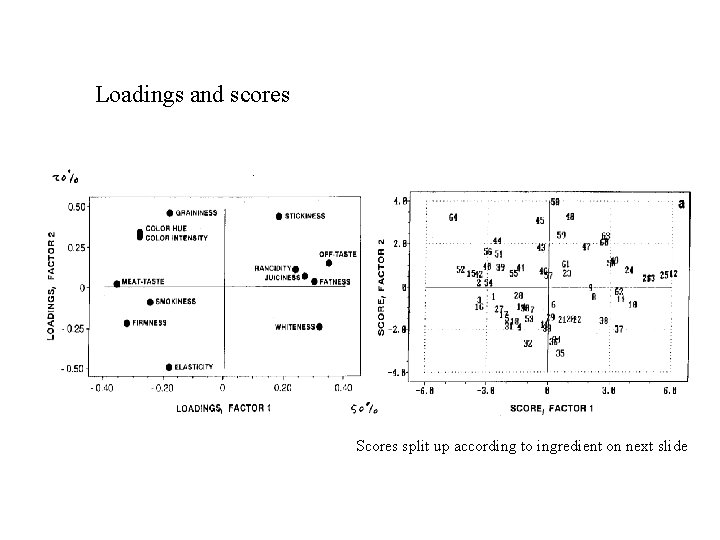
Loadings and scores Scores split up according to ingredient on next slide
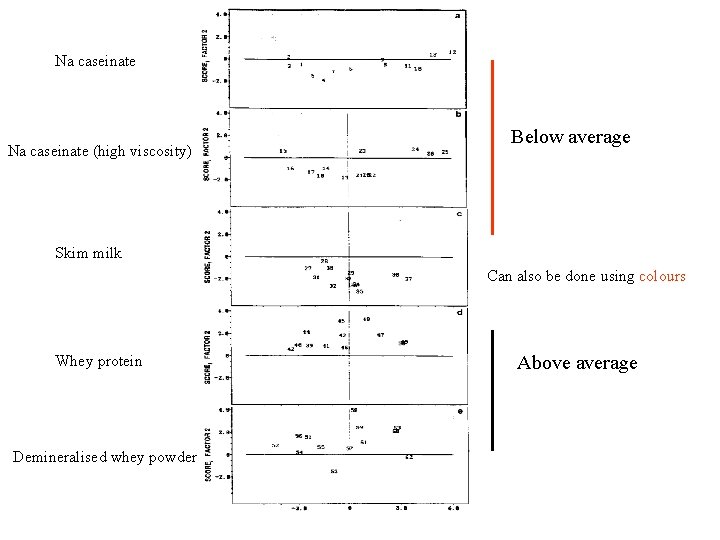
Na caseinate (high viscosity) Below average Skim milk Can also be done using colours Whey protein Demineralised whey powder Above average
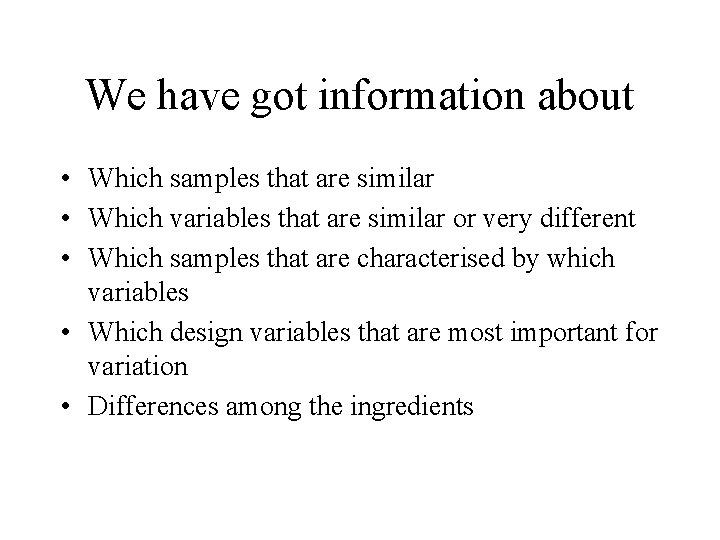
We have got information about • Which samples that are similar • Which variables that are similar or very different • Which samples that are characterised by which variables • Which design variables that are most important for variation • Differences among the ingredients
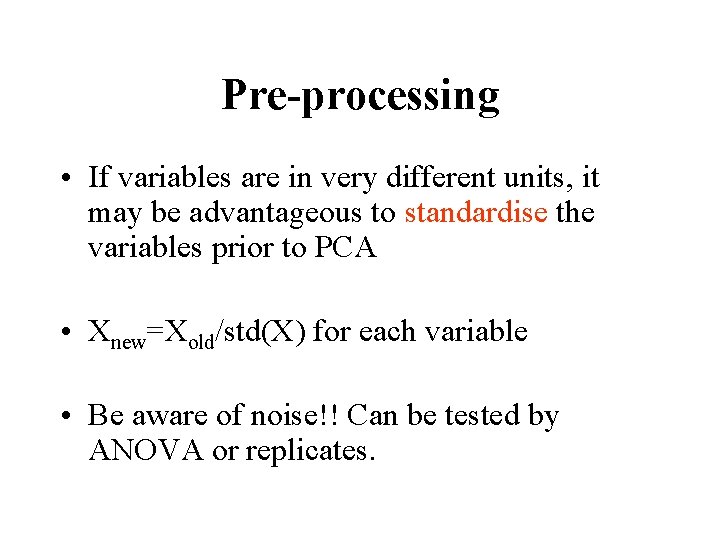
Pre-processing • If variables are in very different units, it may be advantageous to standardise the variables prior to PCA • Xnew=Xold/std(X) for each variable • Be aware of noise!! Can be tested by ANOVA or replicates.
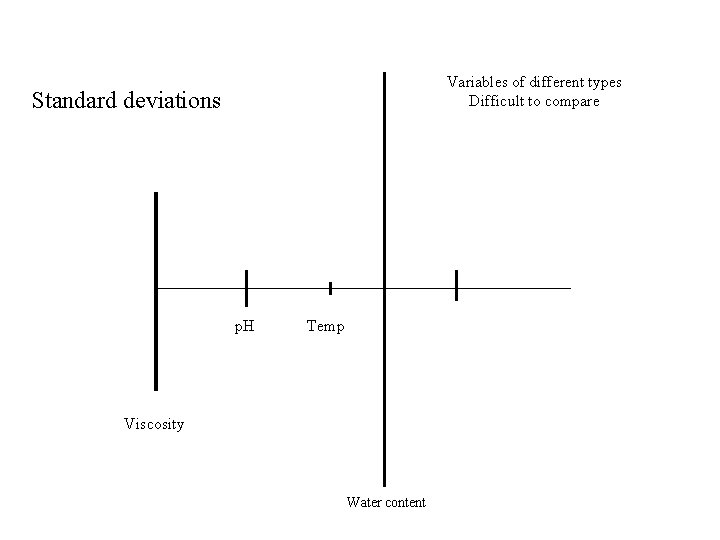
Variables of different types Difficult to compare Standard deviations p. H Temp Viscosity Water content
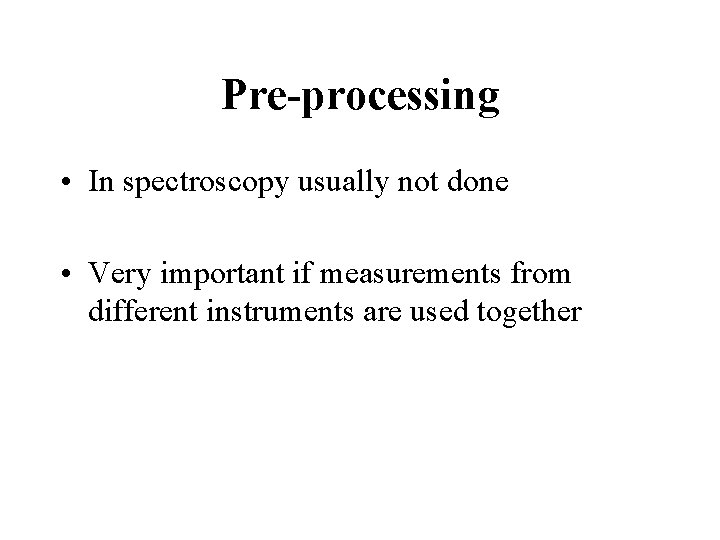
Pre-processing • In spectroscopy usually not done • Very important if measurements from different instruments are used together
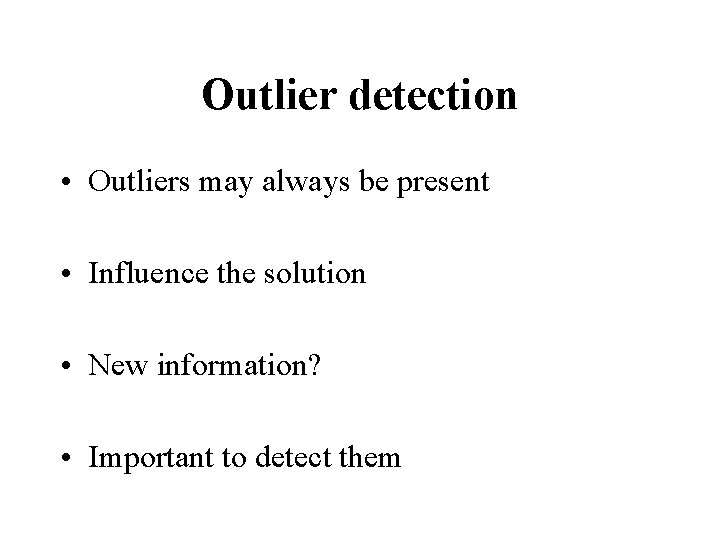
Outlier detection • Outliers may always be present • Influence the solution • New information? • Important to detect them
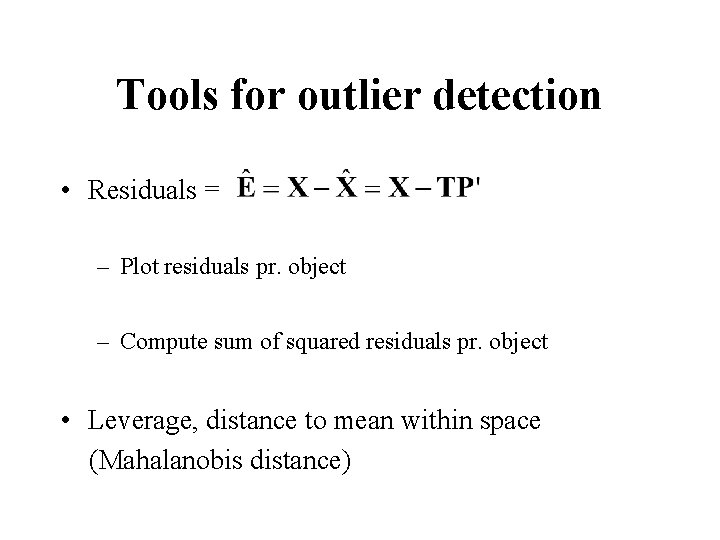
Tools for outlier detection • Residuals = – Plot residuals pr. object – Compute sum of squared residuals pr. object • Leverage, distance to mean within space (Mahalanobis distance)
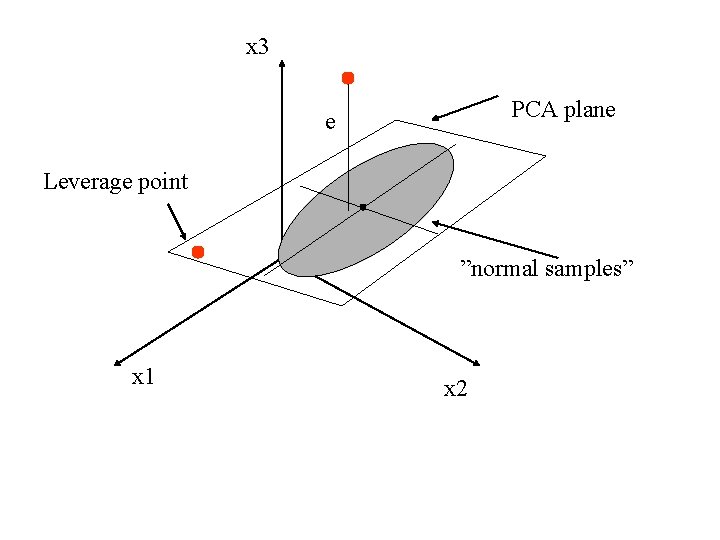
x 3 PCA plane e Leverage point ”normal samples” x 1 x 2
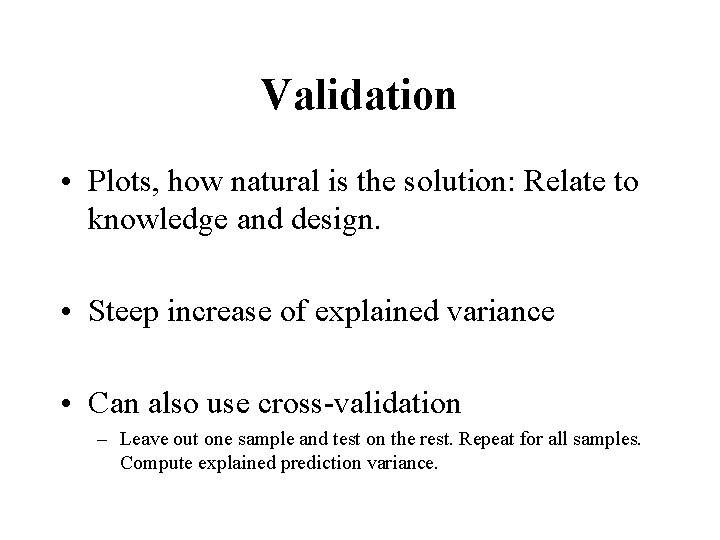
Validation • Plots, how natural is the solution: Relate to knowledge and design. • Steep increase of explained variance • Can also use cross-validation – Leave out one sample and test on the rest. Repeat for all samples. Compute explained prediction variance.
Multivariate analysis
Multi variance
Nature of multivariate analysis
Multivariate analysis of variance and covariance
Multivariate analysis
Multivariate statistical analysis
Multivariate analysis
Multivariate analysis
Multivariate pattern analysis
How does interpretation b differ from interpretation a
Independent component analysis vs pca
Example of analysis and interpretation of data
Example of analysis and interpretation of data
Chapter 4 data presentation and analysis
Analysis and interpretation of data example
Difference between qualitative and quantitative research
Nnrims
Data analysis, interpretation and presentation
Mining complex types of data
Mining complex types of data in data mining
Complex data types in data mining
Simple compound complex and compound-complex sentences quiz
Miliary tb
Complex and compound sentences quiz
Oedipus complex and electra complex
What are defense mechanisms according to freud?
Sublimation defense mechanism
Logistisches wachstum ableitung
Multivariate binomial distribution
Multivariate pdf
Linear regression spss interpretation
Log likelihood multivariate normal
Maximum a posteriori estimation for multivariate gaussian
Multivariate pdf
Multivariate vs bivariate