IGARSS 2011 Forest mapping using multitemporal polarimetric SAR
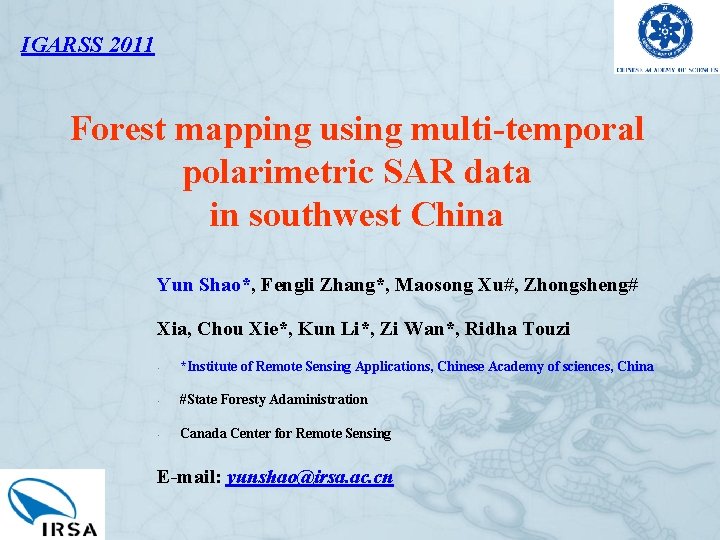
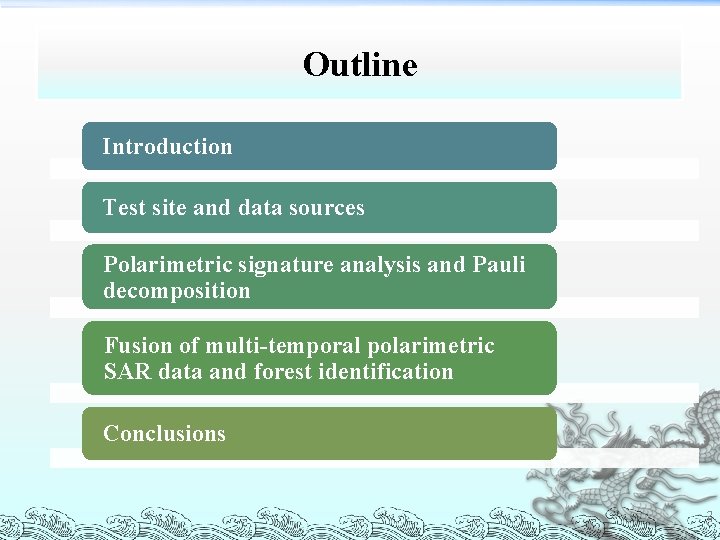
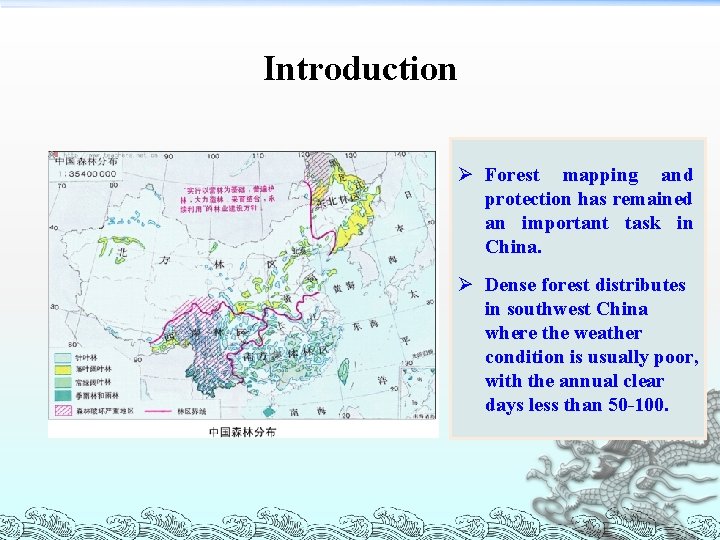
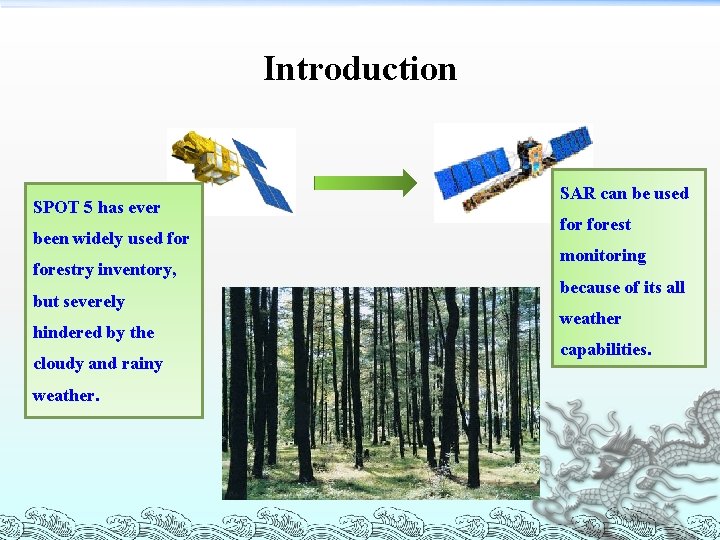
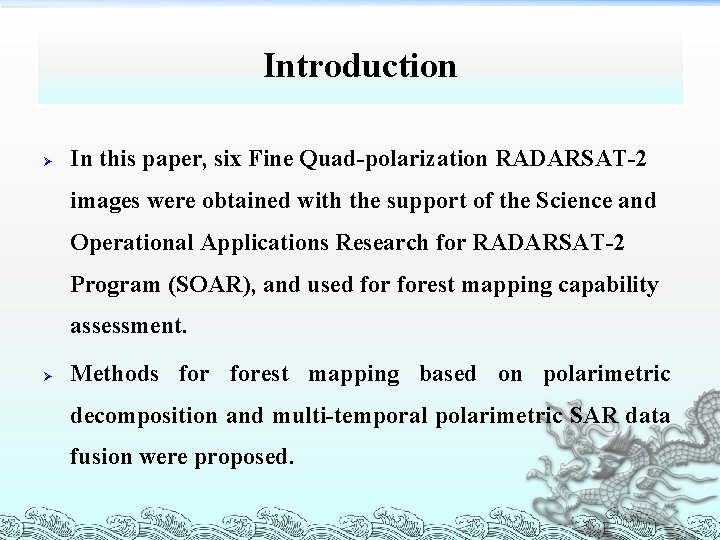
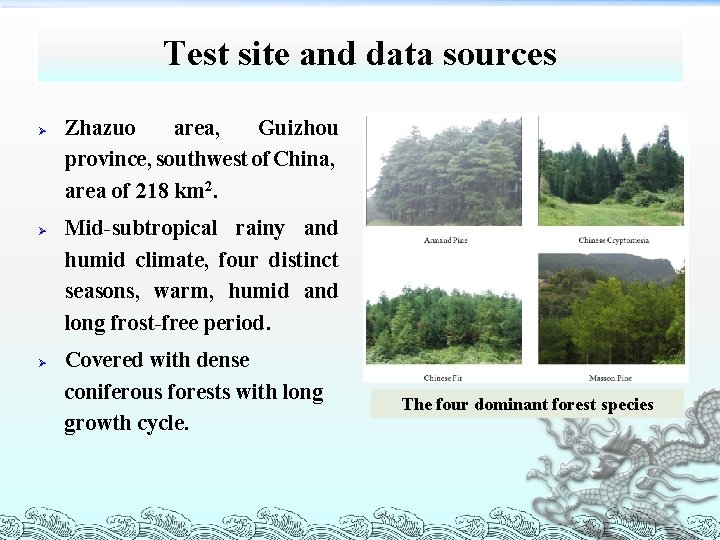
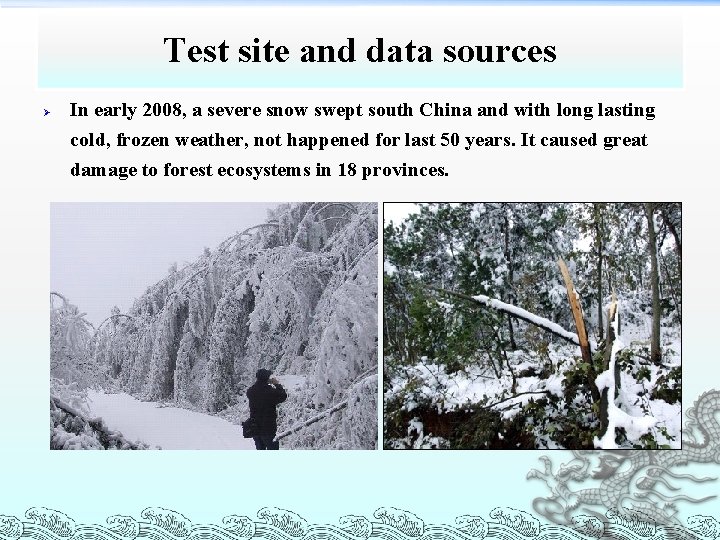
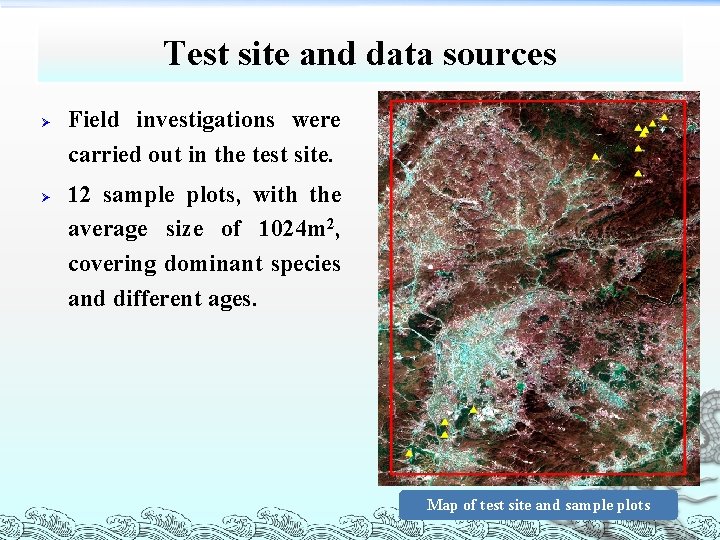
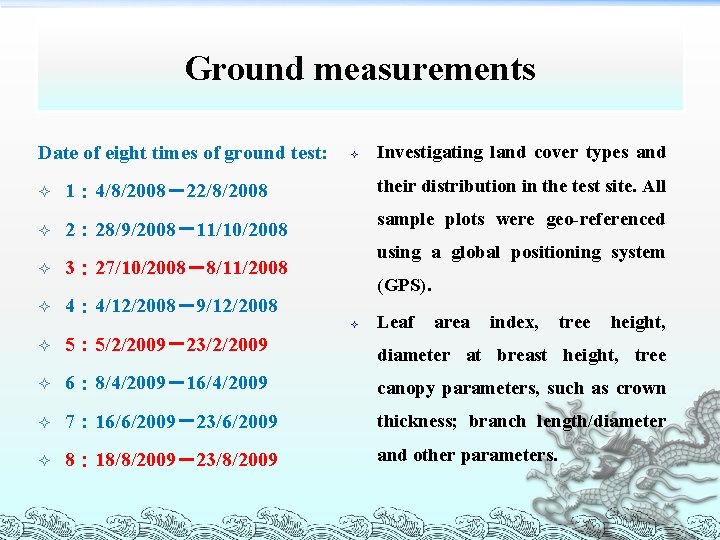
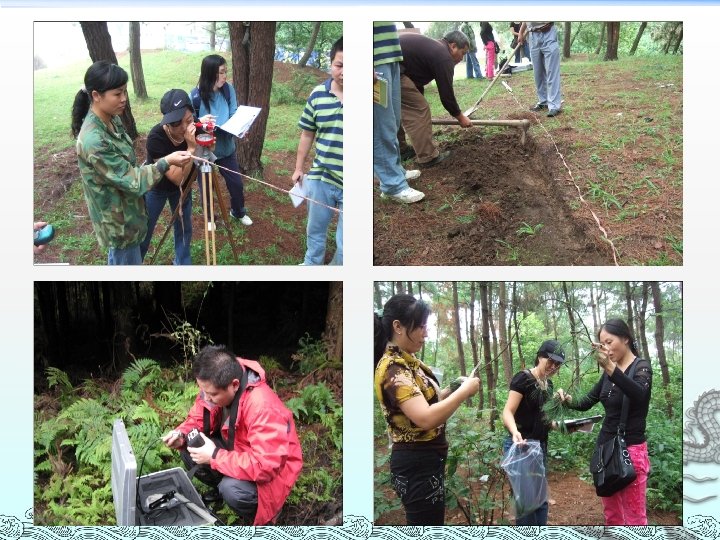
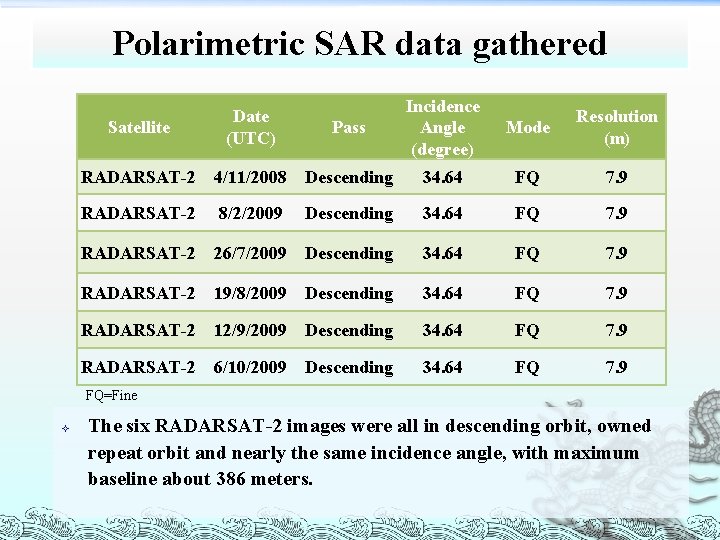
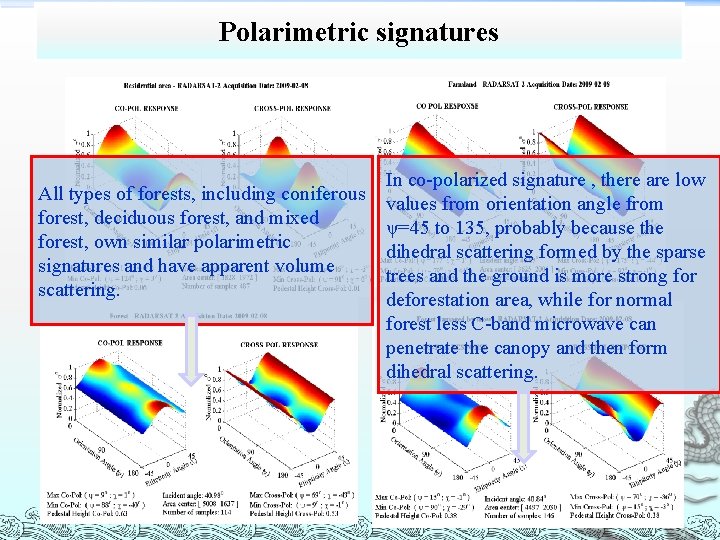
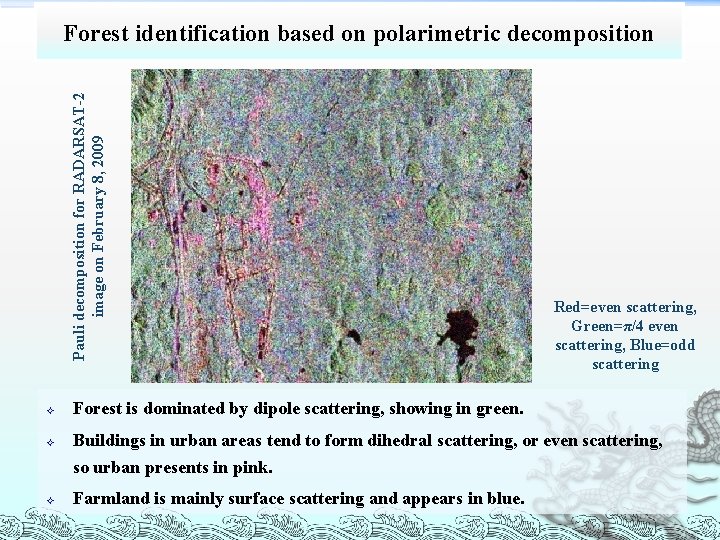
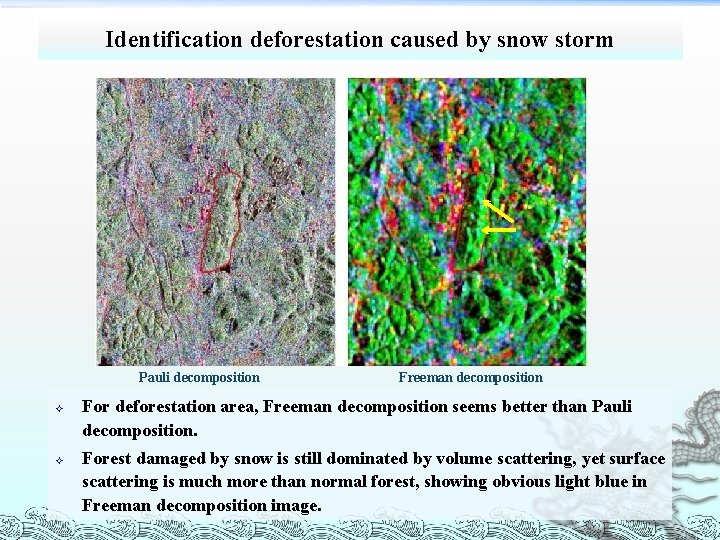
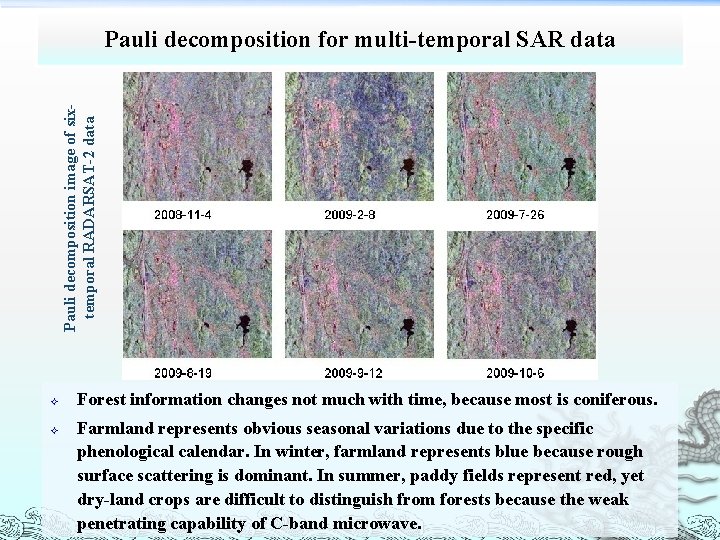
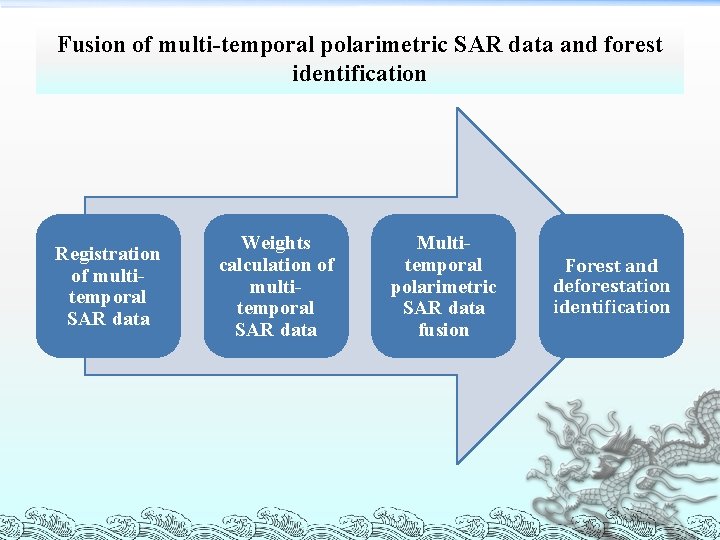
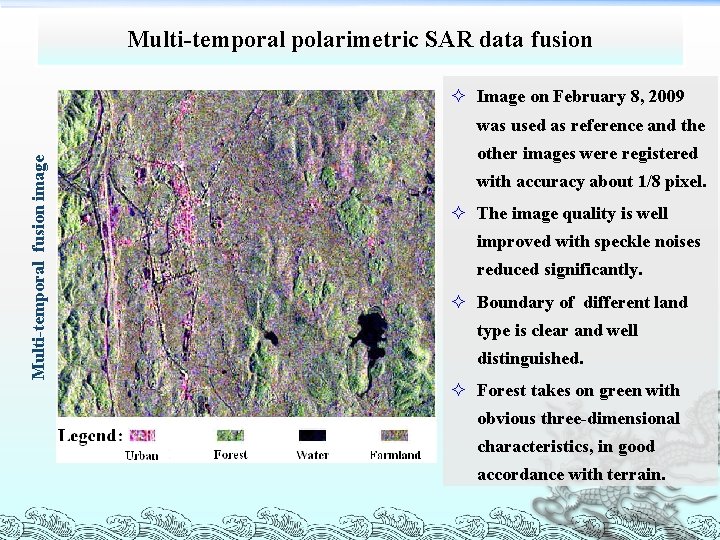
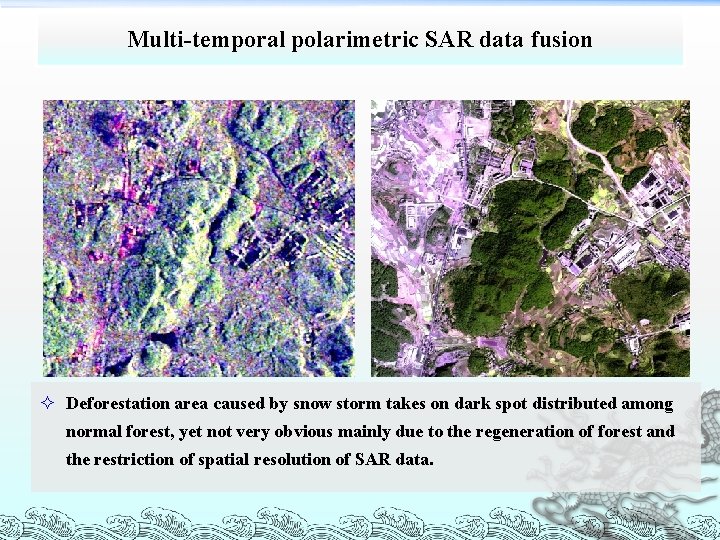
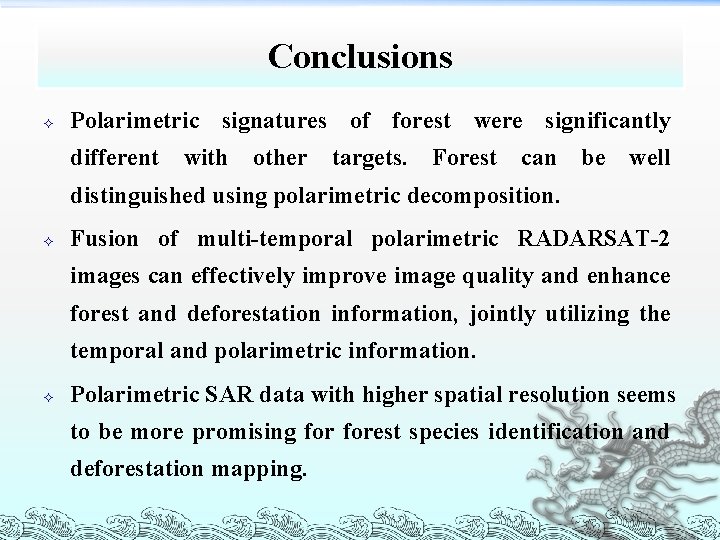
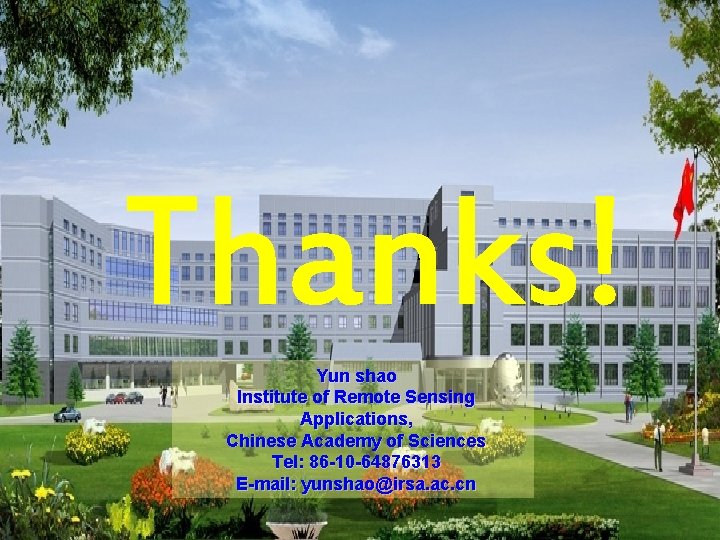
- Slides: 20
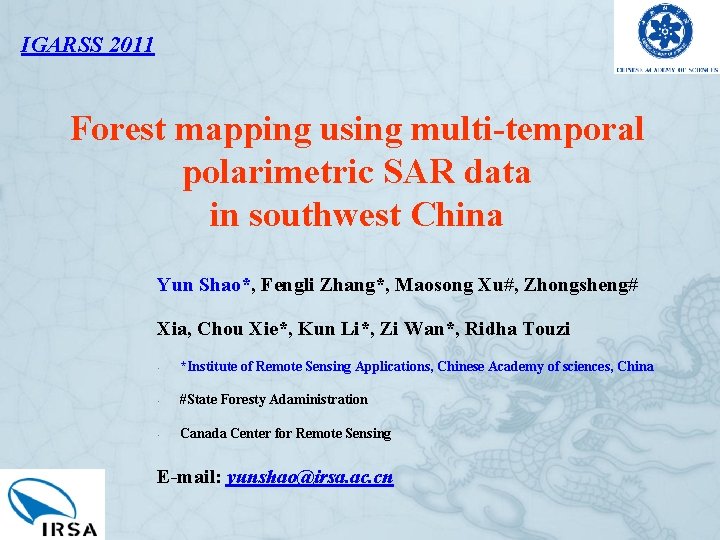
IGARSS 2011 Forest mapping using multi-temporal polarimetric SAR data in southwest China Yun Shao*, Fengli Zhang*, Maosong Xu#, Zhongsheng# Xia, Chou Xie*, Kun Li*, Zi Wan*, Ridha Touzi • *Institute of Remote Sensing Applications, Chinese Academy of sciences, China • #State Foresty Adaministration • Canada Center for Remote Sensing E-mail: yunshao@irsa. ac. cn
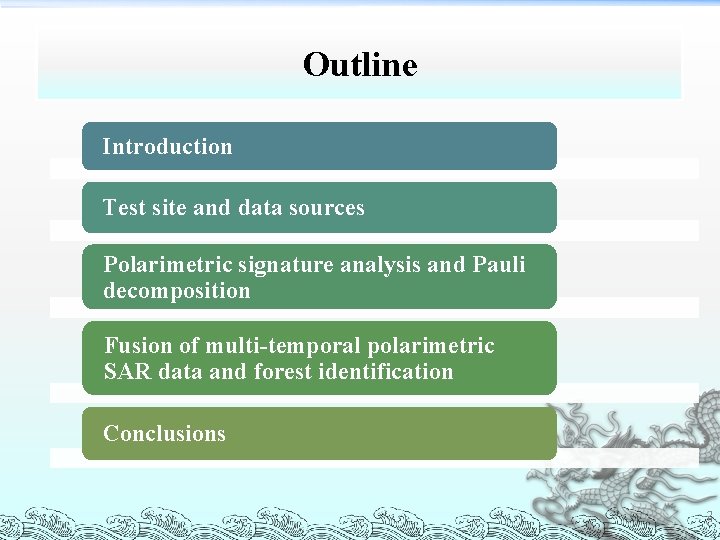
Outline Introduction Test site and data sources Polarimetric signature analysis and Pauli decomposition Fusion of multi-temporal polarimetric SAR data and forest identification Conclusions 2
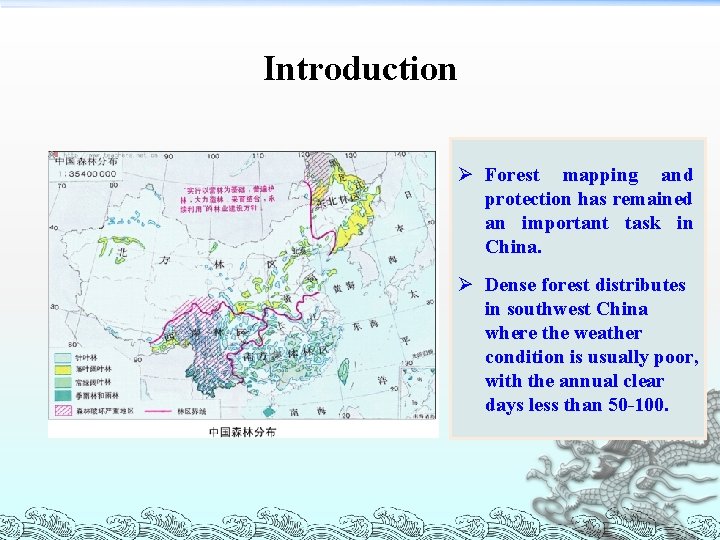
Introduction Ø Forest mapping and protection has remained an important task in China. Ø Dense forest distributes in southwest China where the weather condition is usually poor, with the annual clear days less than 50 -100.
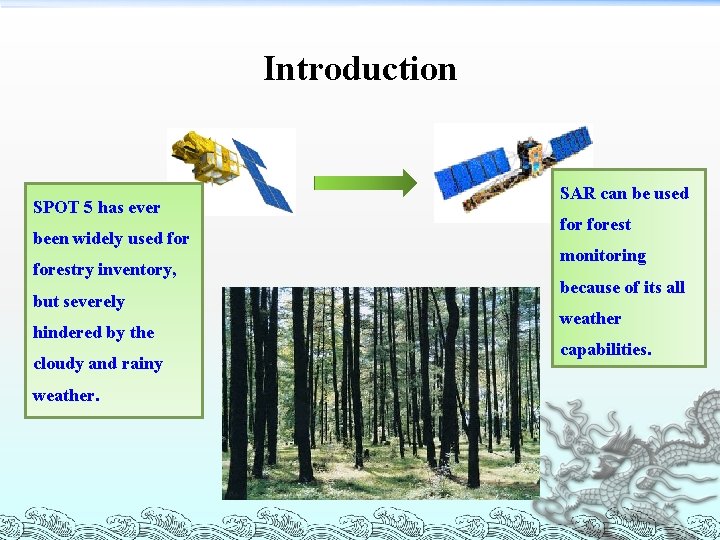
Introduction SPOT 5 has ever been widely used forestry inventory, but severely hindered by the cloudy and rainy weather. SAR can be used forest monitoring because of its all weather capabilities.
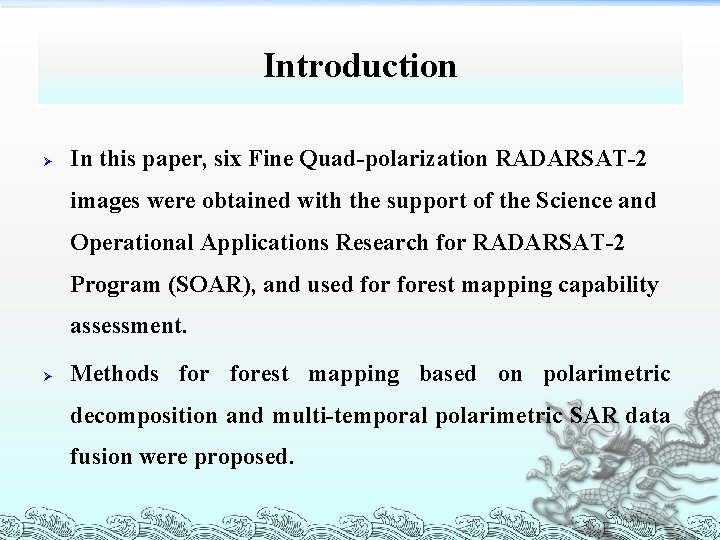
Introduction Ø In this paper, six Fine Quad-polarization RADARSAT-2 images were obtained with the support of the Science and Operational Applications Research for RADARSAT-2 Program (SOAR), and used forest mapping capability assessment. Ø Methods forest mapping based on polarimetric decomposition and multi-temporal polarimetric SAR data fusion were proposed.
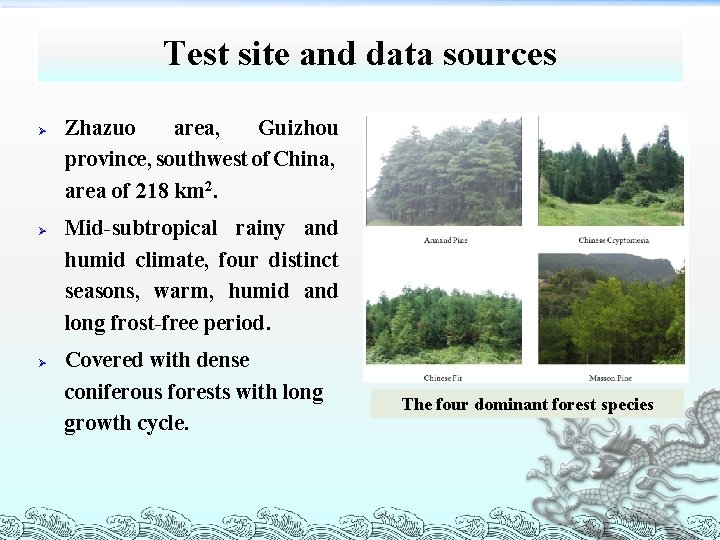
Test site and data sources Ø Ø Ø Zhazuo area, Guizhou province, southwest of China, area of 218 km 2. Mid-subtropical rainy and humid climate, four distinct seasons, warm, humid and long frost-free period. Covered with dense coniferous forests with long growth cycle. The four dominant forest species
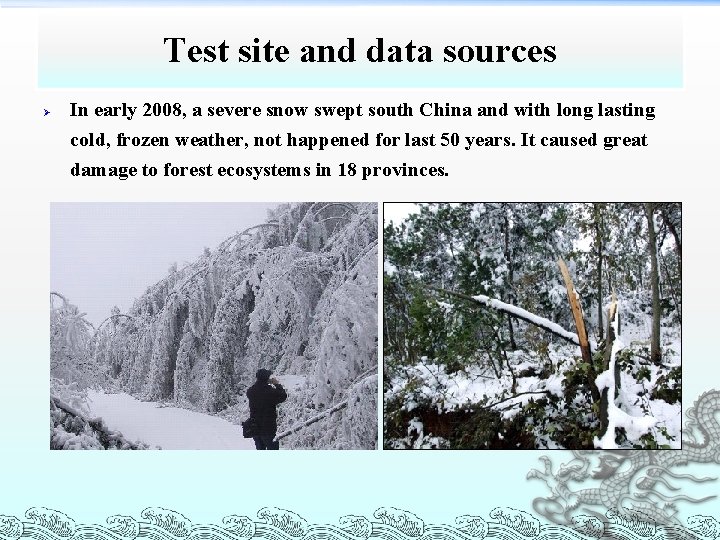
Test site and data sources Ø In early 2008, a severe snow swept south China and with long lasting cold, frozen weather, not happened for last 50 years. It caused great damage to forest ecosystems in 18 provinces.
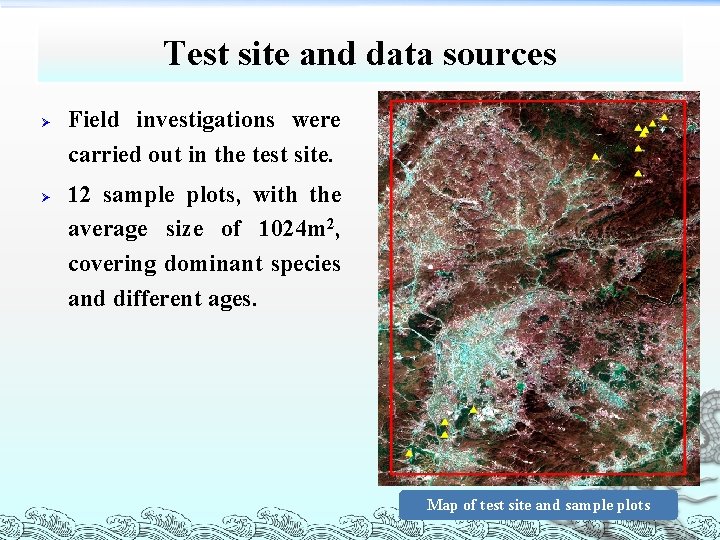
Test site and data sources Ø Ø Field investigations were carried out in the test site. 12 sample plots, with the average size of 1024 m 2, covering dominant species and different ages. Map of test site and sample plots
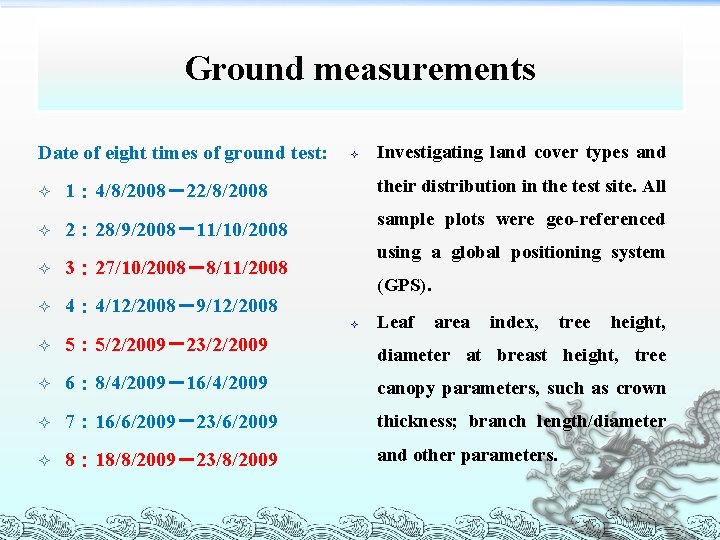
Ground measurements Date of eight times of ground test: ² 1: 4/8/2008-22/8/2008 ² 2: 28/9/2008-11/10/2008 ² 3: 27/10/2008-8/11/2008 ² 4: 4/12/2008-9/12/2008 ² Investigating land cover types and their distribution in the test site. All sample plots were geo-referenced using a global positioning system (GPS). ² Leaf area index, tree height, ² 5: 5/2/2009-23/2/2009 ² 6: 8/4/2009-16/4/2009 canopy parameters, such as crown ² 7: 16/6/2009-23/6/2009 thickness; branch length/diameter ² 8: 18/8/2009-23/8/2009 and other parameters. diameter at breast height, tree
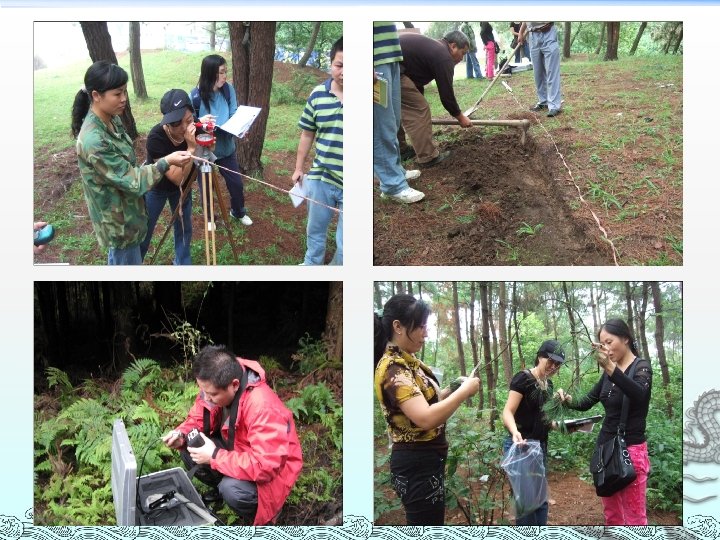
10
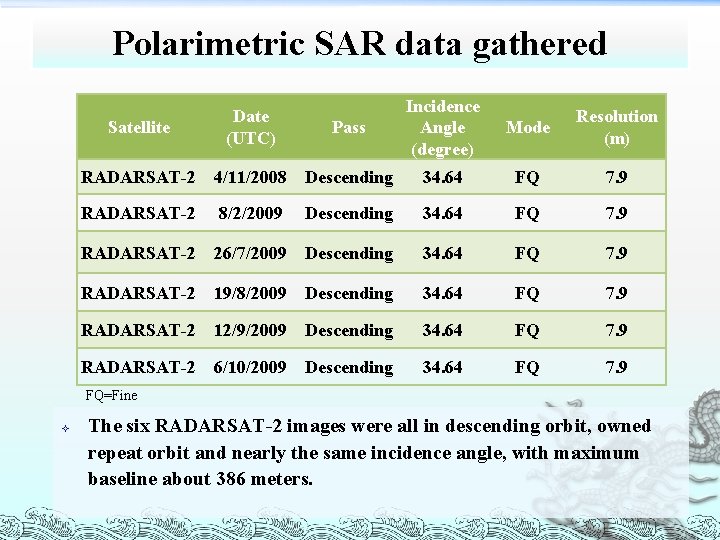
Polarimetric SAR data gathered Incidence Angle (degree) Mode Resolution (m) RADARSAT-2 4/11/2008 Descending 34. 64 FQ 7. 9 RADARSAT-2 26/7/2009 Descending 34. 64 FQ 7. 9 RADARSAT-2 19/8/2009 Descending 34. 64 FQ 7. 9 RADARSAT-2 12/9/2009 Descending 34. 64 FQ 7. 9 RADARSAT-2 6/10/2009 Descending 34. 64 FQ 7. 9 Satellite Date (UTC) 8/2/2009 Pass FQ=Fine ² The six RADARSAT-2 images were all in descending orbit, owned repeat orbit and nearly the same incidence angle, with maximum baseline about 386 meters.
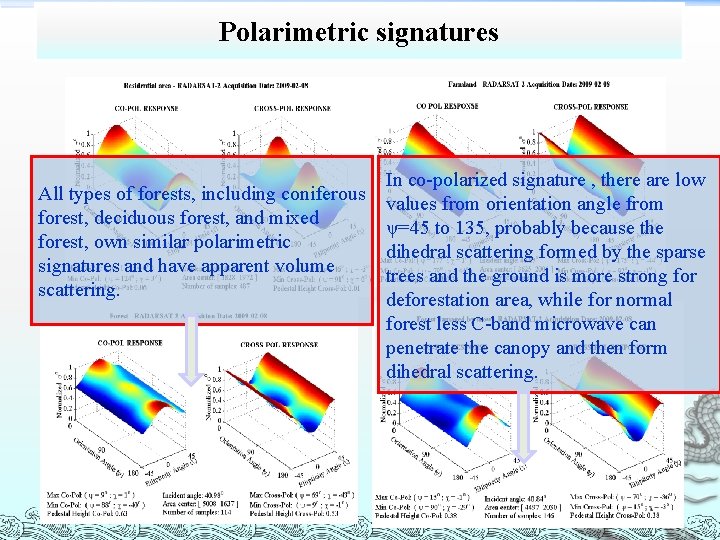
Polarimetric signatures All types of forests, including coniferous forest, deciduous forest, and mixed forest, own similar polarimetric signatures and have apparent volume scattering. In co-polarized signature , there are low values from orientation angle from ψ=45 to 135, probably because the dihedral scattering formed by the sparse trees and the ground is more strong for deforestation area, while for normal forest less C-band microwave can penetrate the canopy and then form dihedral scattering.
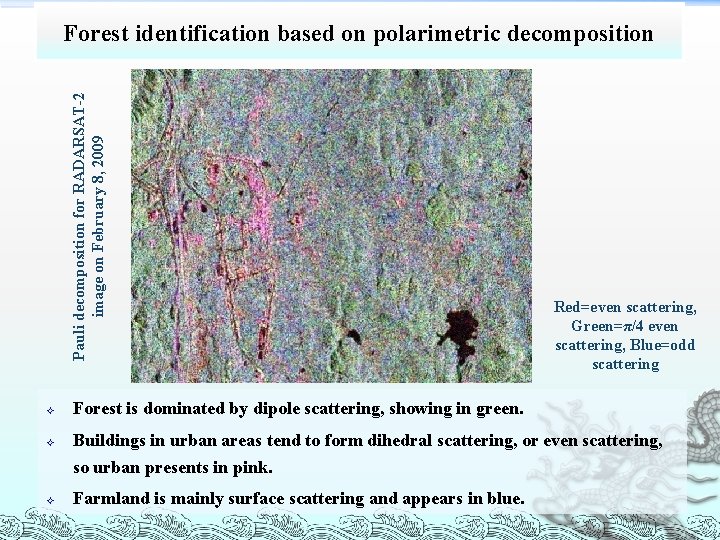
Pauli decomposition for RADARSAT-2 image on February 8, 2009 Forest identification based on polarimetric decomposition ² ² ² Red=even scattering, Green=π/4 even scattering, Blue=odd scattering Forest is dominated by dipole scattering, showing in green. Buildings in urban areas tend to form dihedral scattering, or even scattering, so urban presents in pink. Farmland is mainly surface scattering and appears in blue.
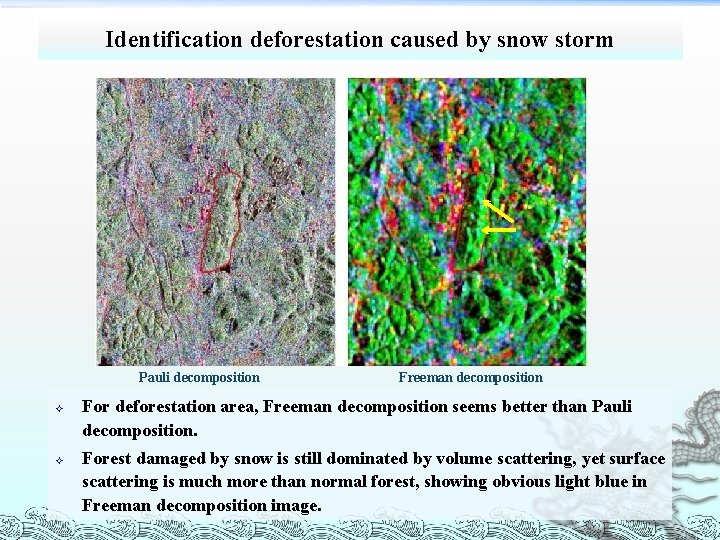
Identification deforestation caused by snow storm Pauli decomposition ² ² Freeman decomposition For deforestation area, Freeman decomposition seems better than Pauli decomposition. Forest damaged by snow is still dominated by volume scattering, yet surface scattering is much more than normal forest, showing obvious light blue in Freeman decomposition image.
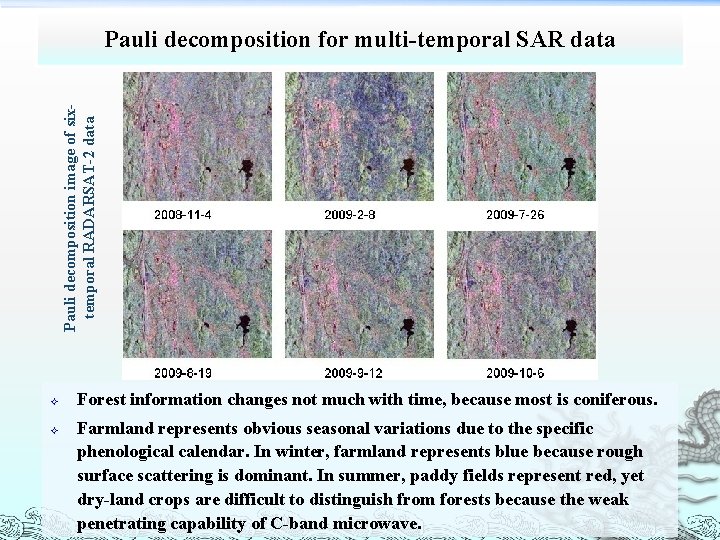
Pauli decomposition image of sixtemporal RADARSAT-2 data Pauli decomposition for multi-temporal SAR data ² ² Forest information changes not much with time, because most is coniferous. Farmland represents obvious seasonal variations due to the specific phenological calendar. In winter, farmland represents blue because rough surface scattering is dominant. In summer, paddy fields represent red, yet dry-land crops are difficult to distinguish from forests because the weak penetrating capability of C-band microwave.
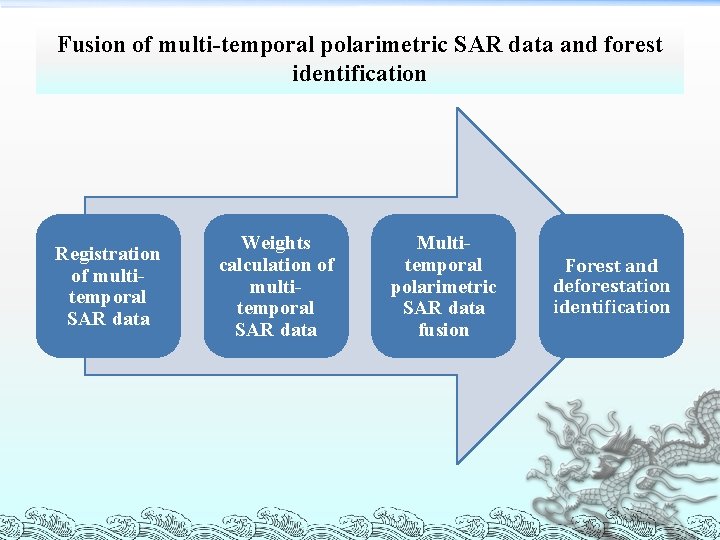
Fusion of multi-temporal polarimetric SAR data and forest identification Registration of multitemporal SAR data Weights calculation of multitemporal SAR data Multitemporal polarimetric SAR data fusion Forest and deforestation identification
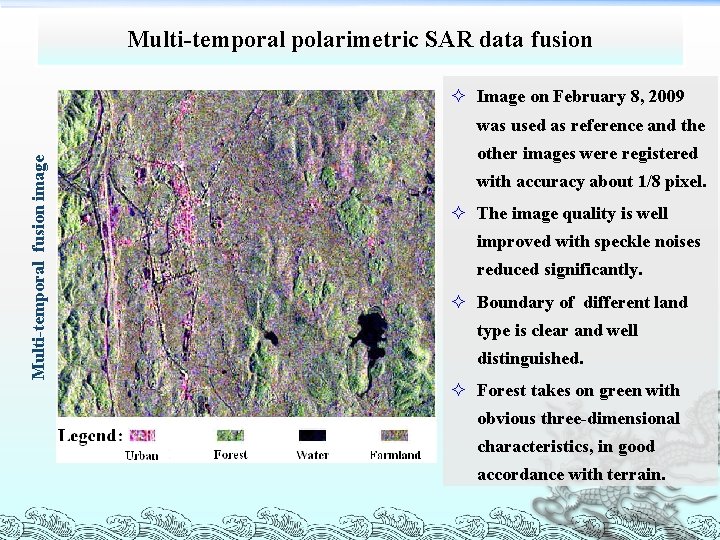
Multi-temporal polarimetric SAR data fusion ² Image on February 8, 2009 Multi-temporal fusion image was used as reference and the other images were registered with accuracy about 1/8 pixel. ² The image quality is well improved with speckle noises reduced significantly. ² Boundary of different land type is clear and well distinguished. ² Forest takes on green with obvious three-dimensional characteristics, in good accordance with terrain.
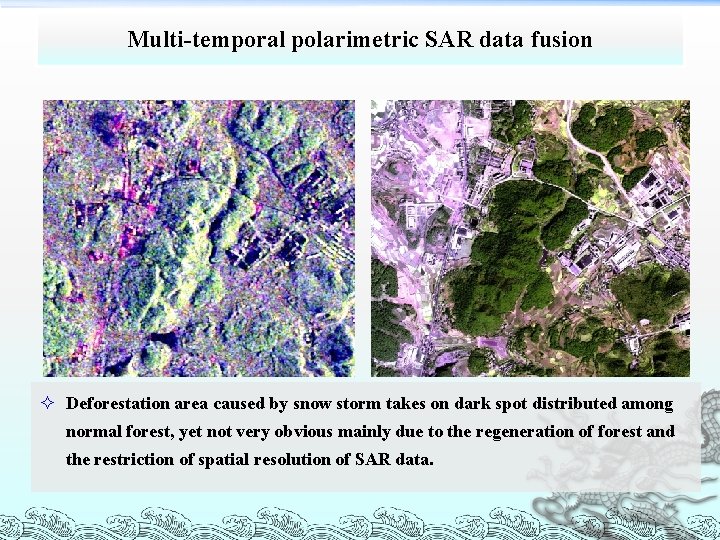
Multi-temporal polarimetric SAR data fusion ² Deforestation area caused by snow storm takes on dark spot distributed among normal forest, yet not very obvious mainly due to the regeneration of forest and the restriction of spatial resolution of SAR data.
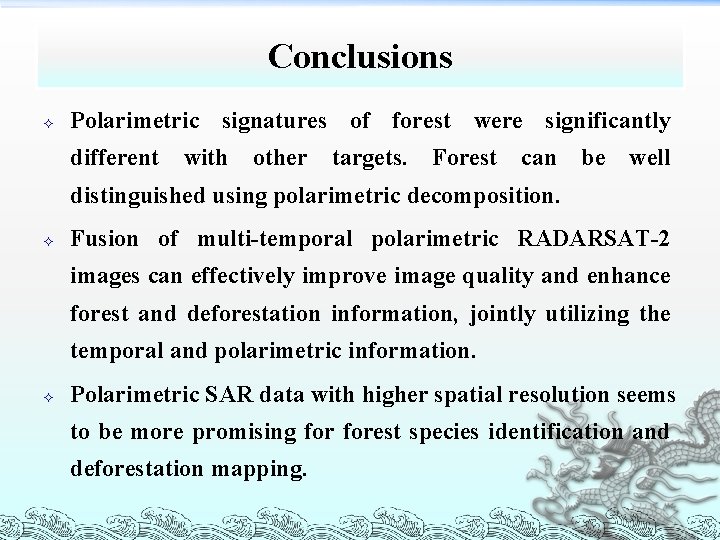
Conclusions ² Polarimetric signatures of forest were significantly different with other targets. Forest can be well distinguished using polarimetric decomposition. ² Fusion of multi-temporal polarimetric RADARSAT-2 images can effectively improve image quality and enhance forest and deforestation information, jointly utilizing the temporal and polarimetric information. ² Polarimetric SAR data with higher spatial resolution seems to be more promising forest species identification and deforestation mapping.
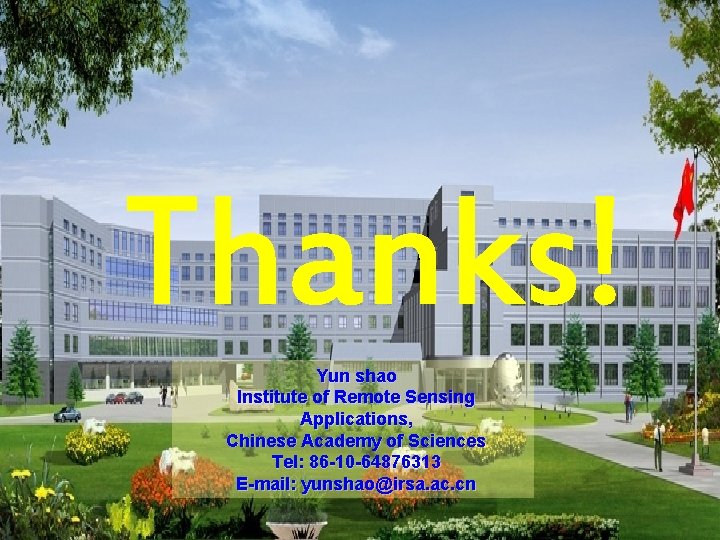
Yun shao Institute of Remote Sensing Applications, Chinese Academy of Sciences Tel: 86 -10 -64876313 E-mail: yunshao@irsa. ac. cn
Head sar body sar
Memory parameters
Forward mapping vs backward mapping
Prinsip analisis dan desain
Utility mapping using gis
Structure activity relationship of sympathomimetic drugs
Sar of cholinergic agents
Definition of sar
Sar soil
Sar vs qsar difference
Definition of sar
Diagnosis banding denture stomatitis
Sar of cephalosporins
Nova sar
Sar of penicillin
Sar of penicillin
Ravenscroft camps
Sar of penicillin
Hudlim til sår
Sar image formation
Leptiri na sar planini