Hydrologic Data and Modeling Towards Hydrologic Information Science
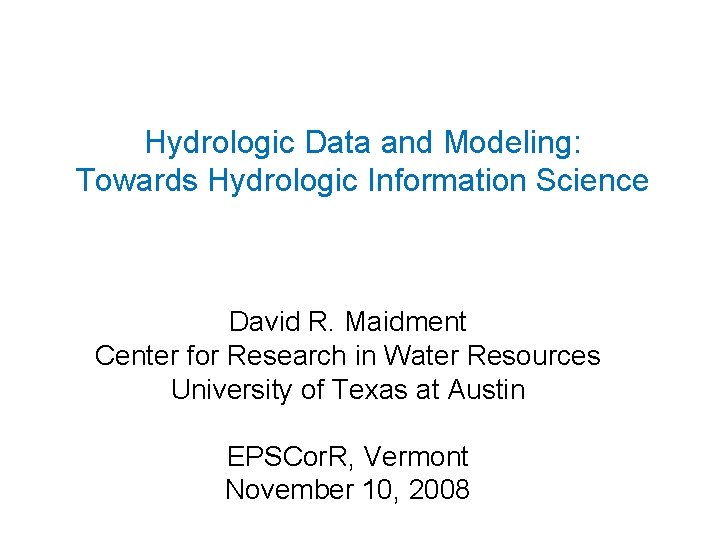
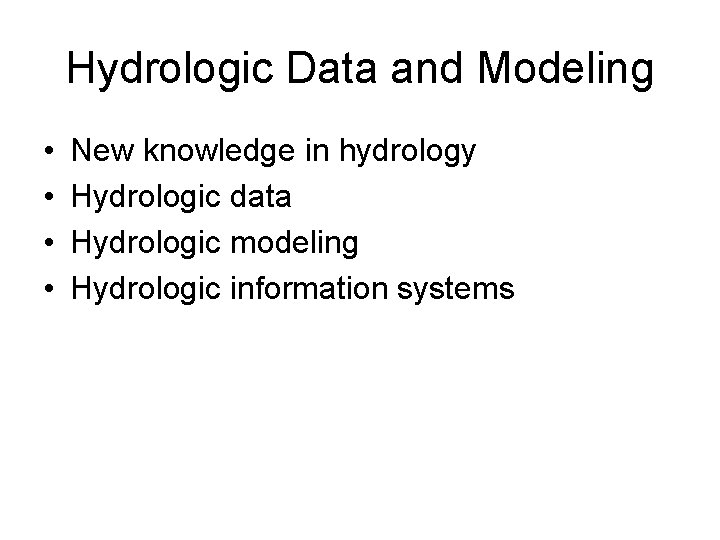
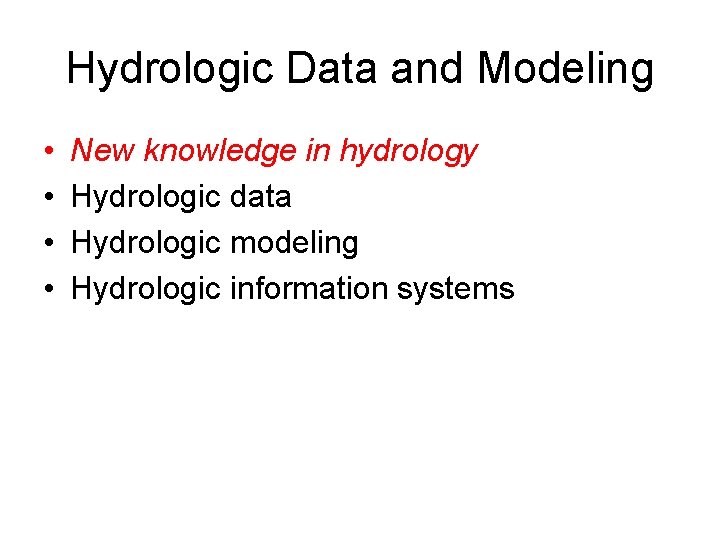
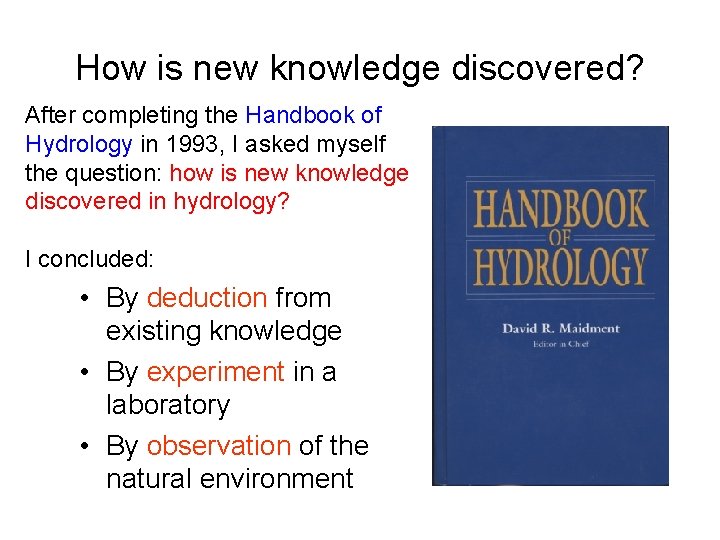
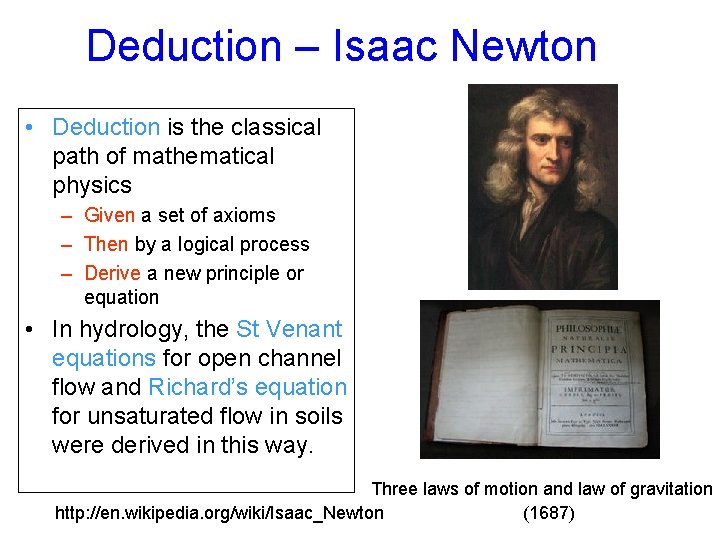
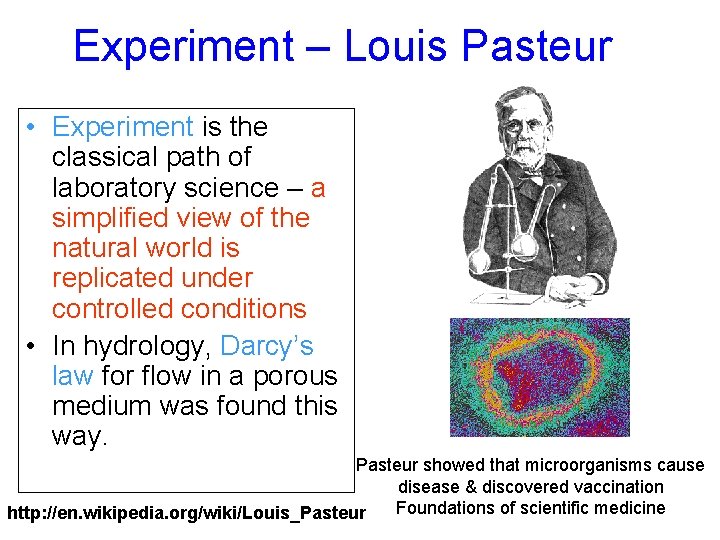
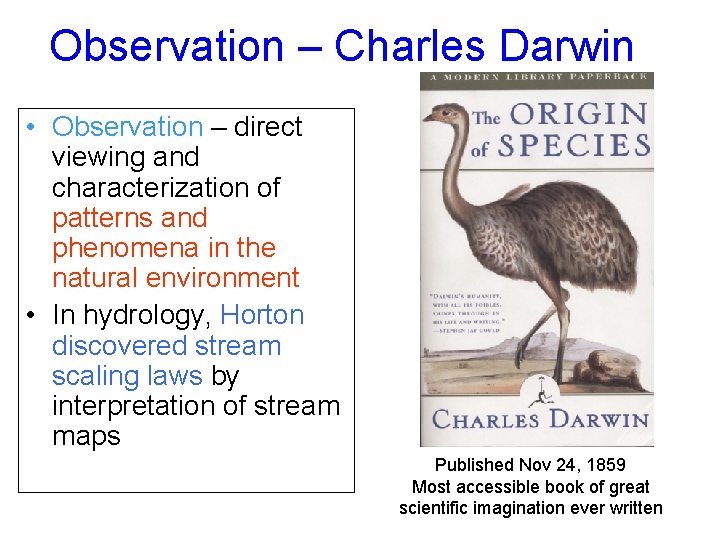
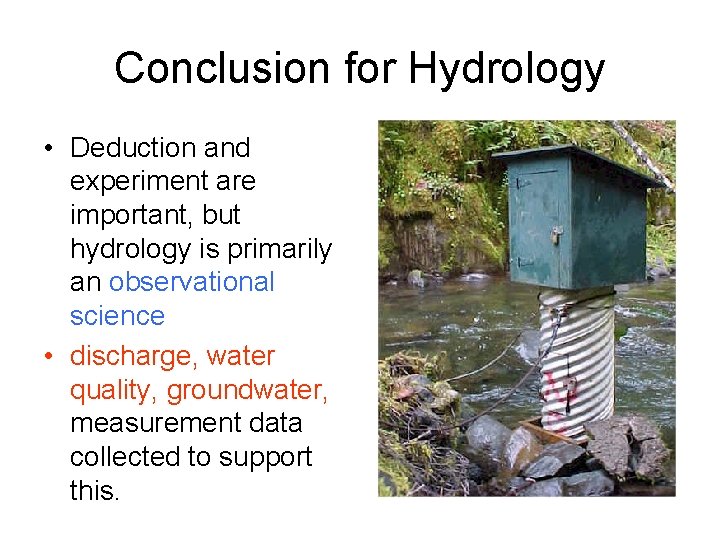
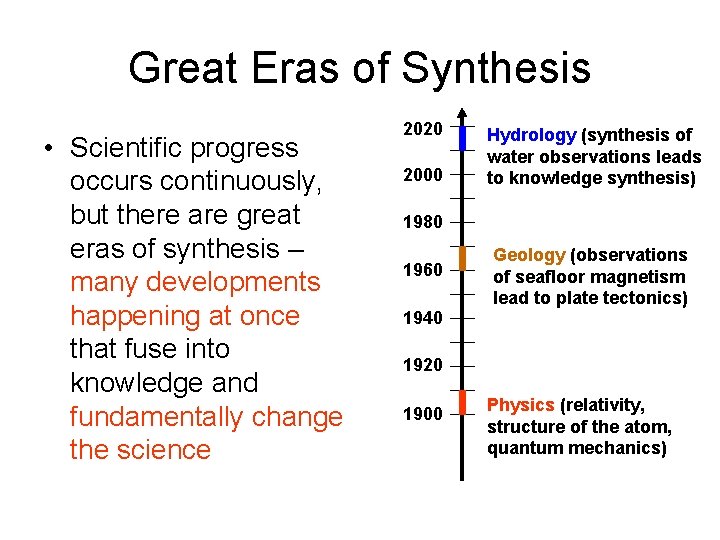
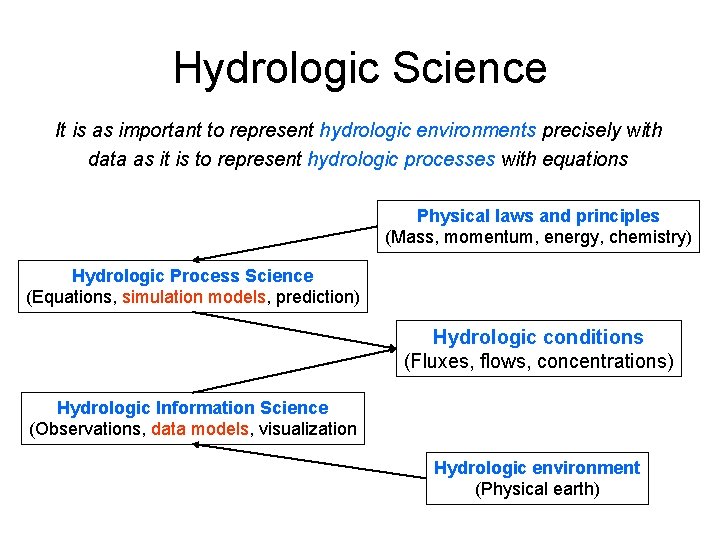
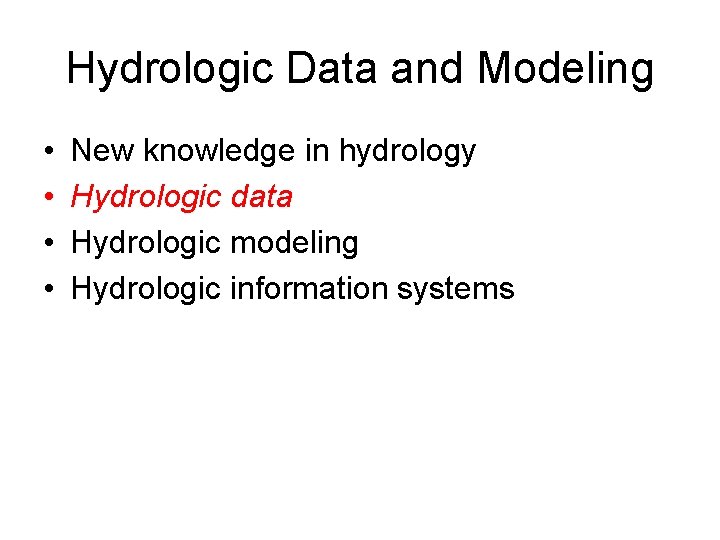
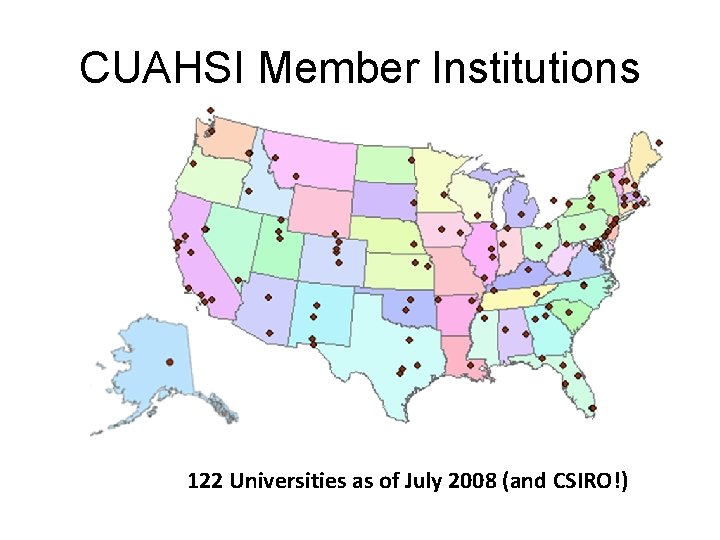
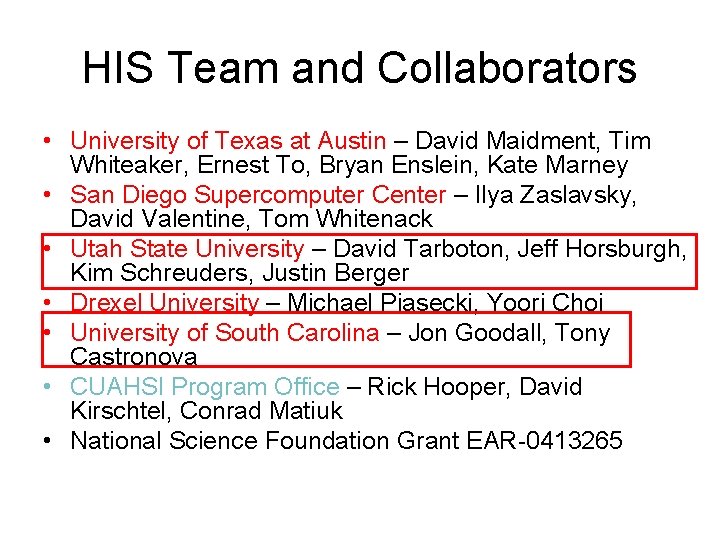
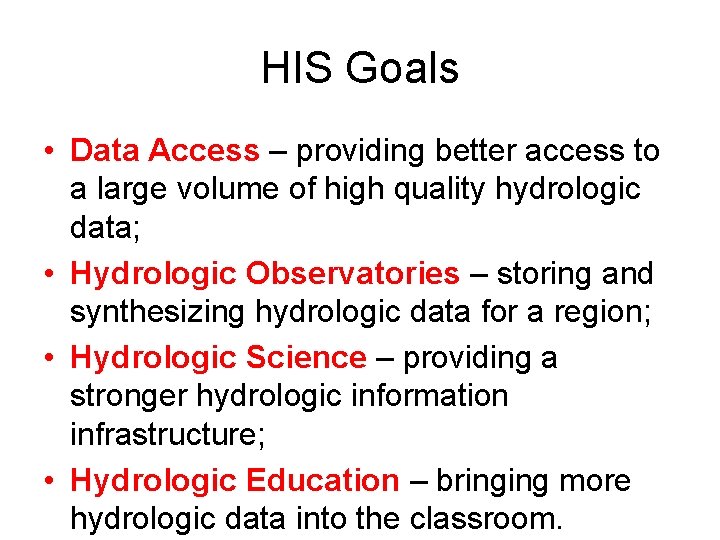
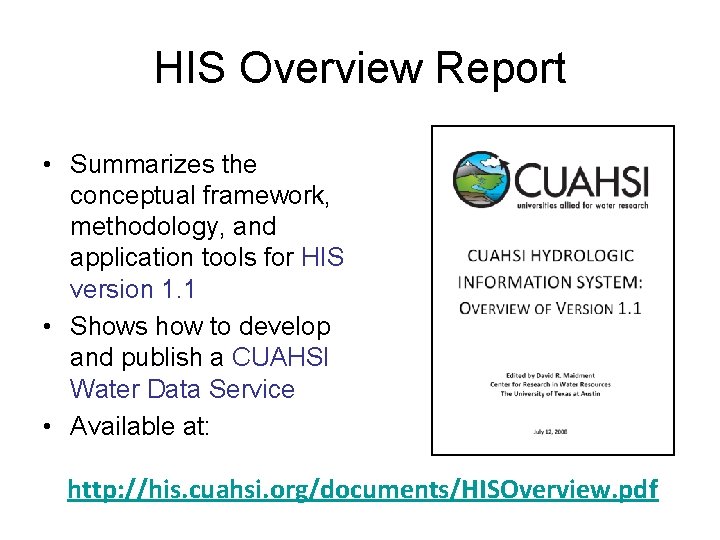
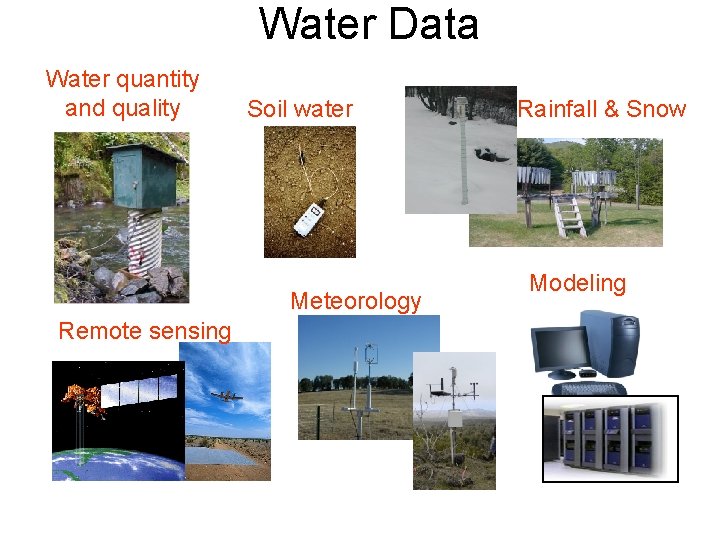
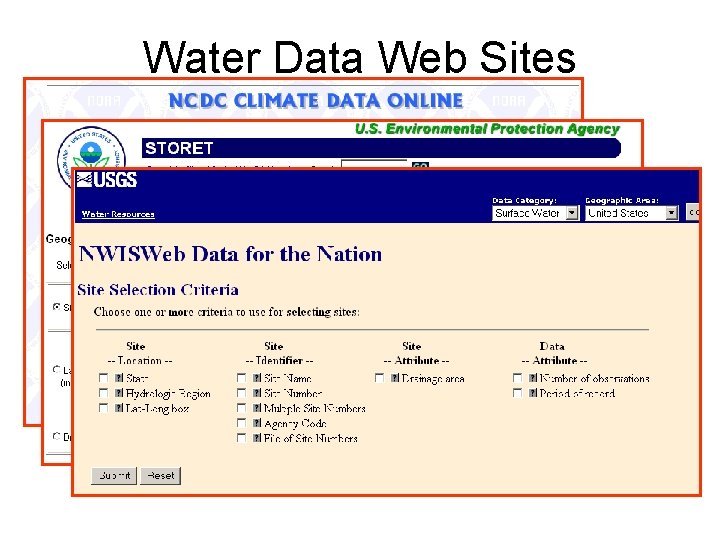
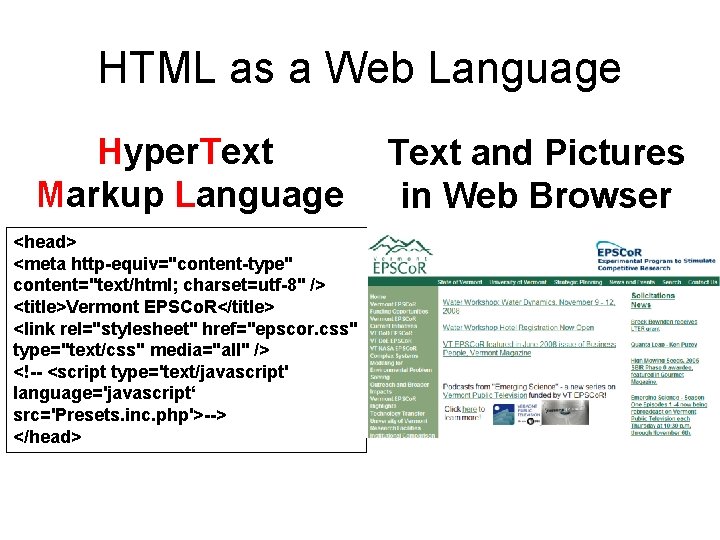
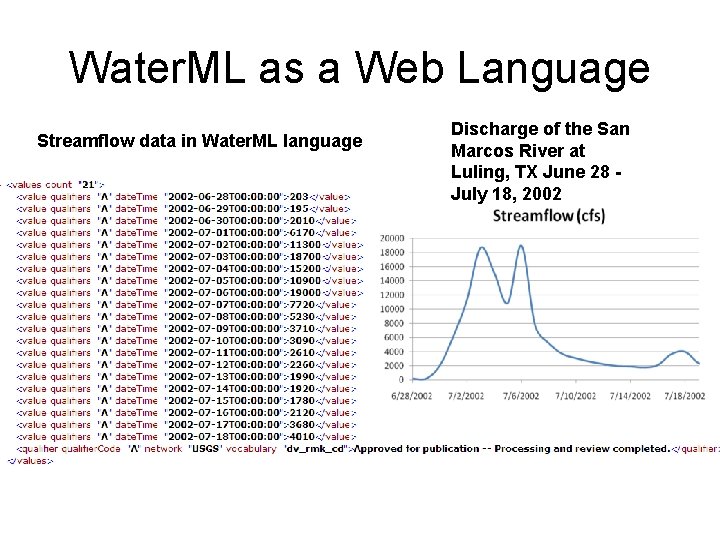
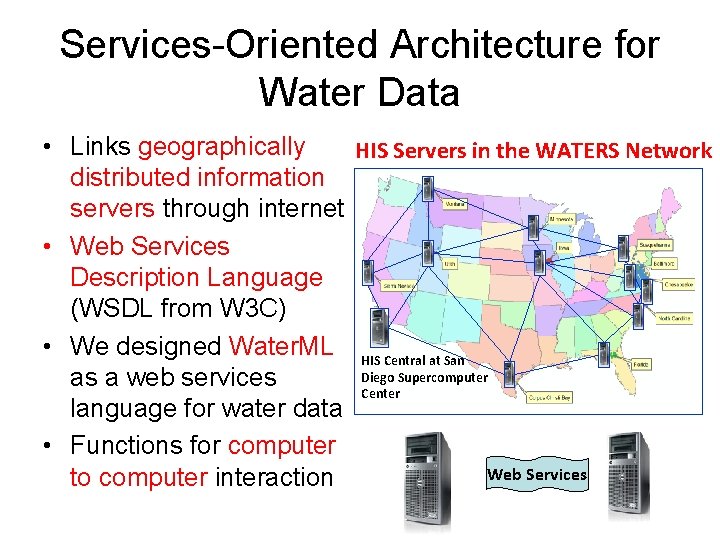
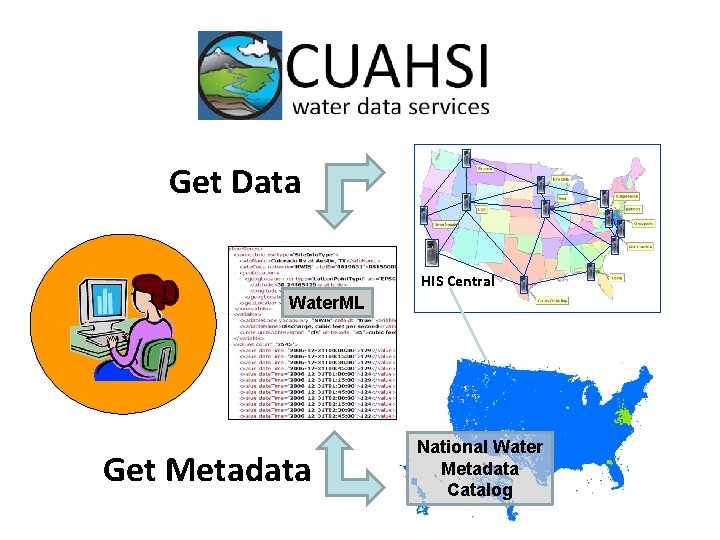
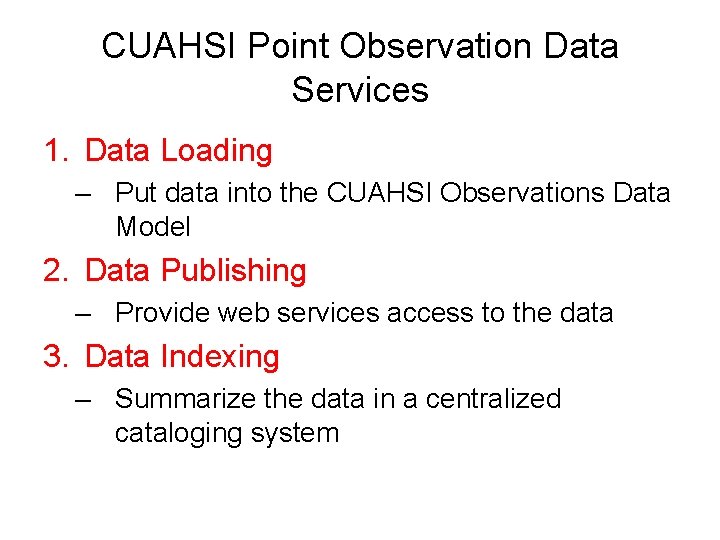
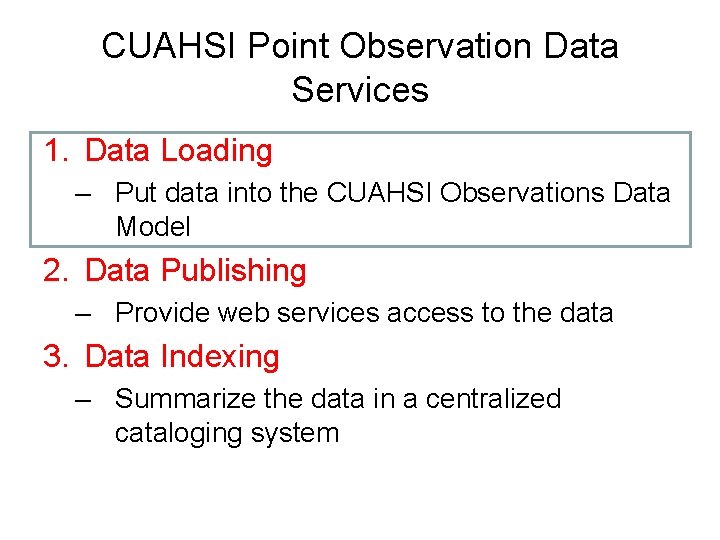
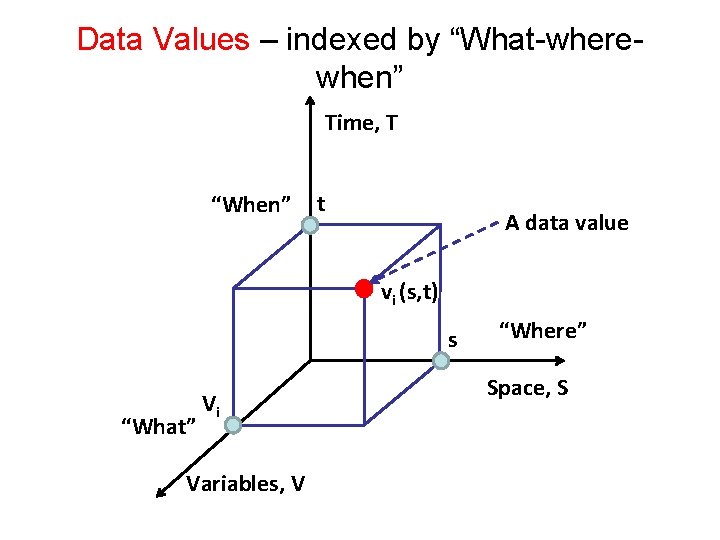
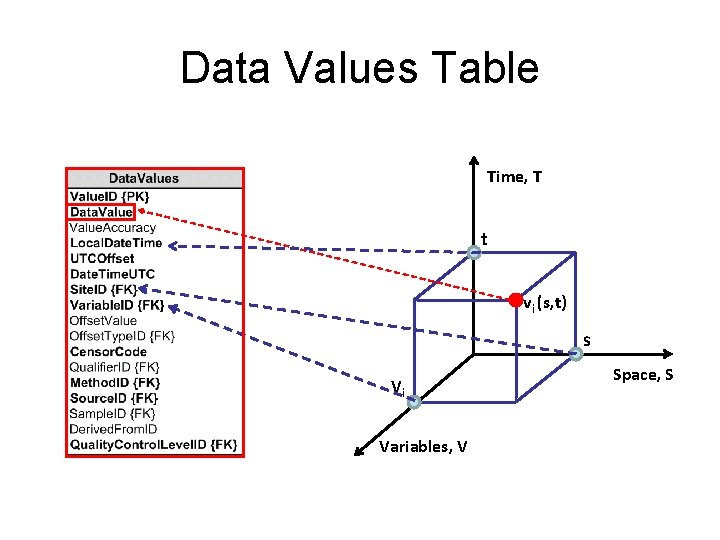
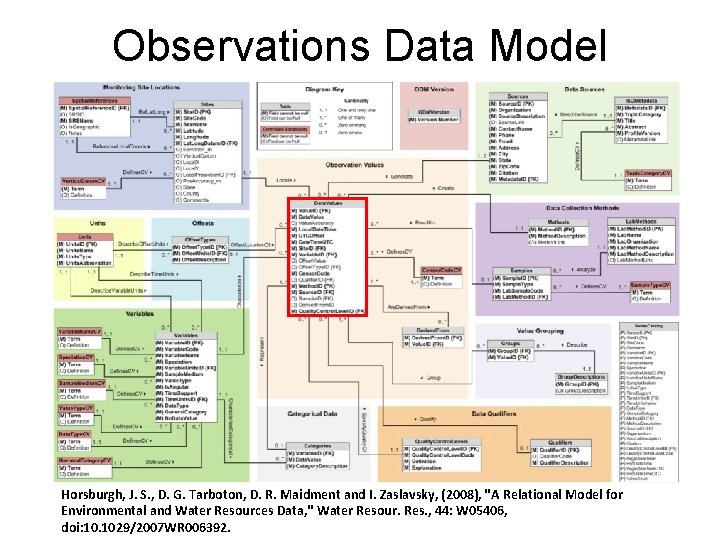
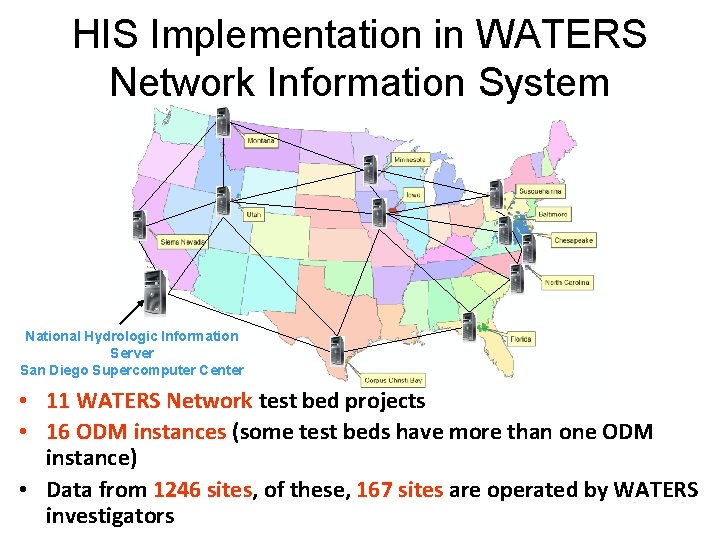
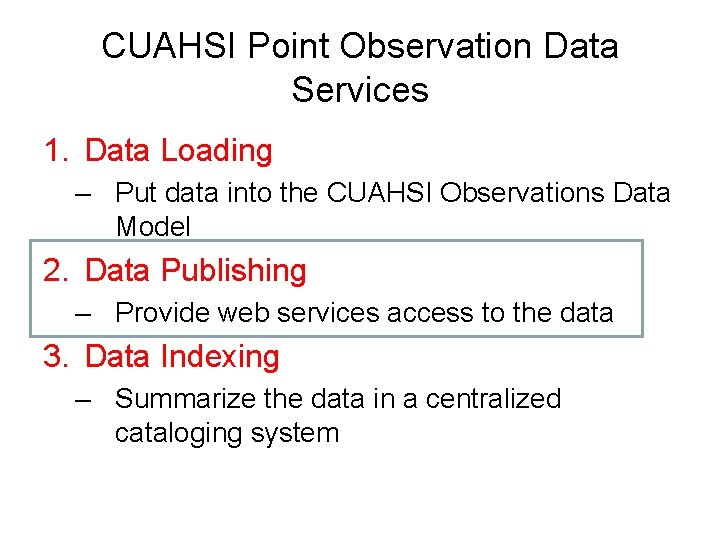
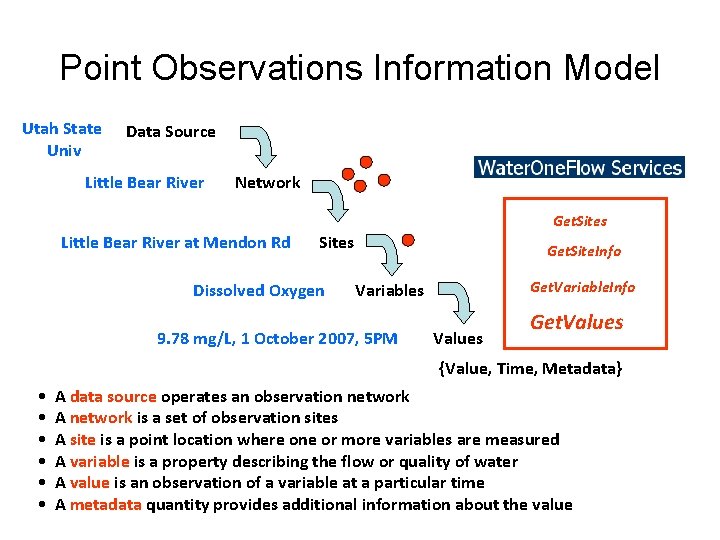
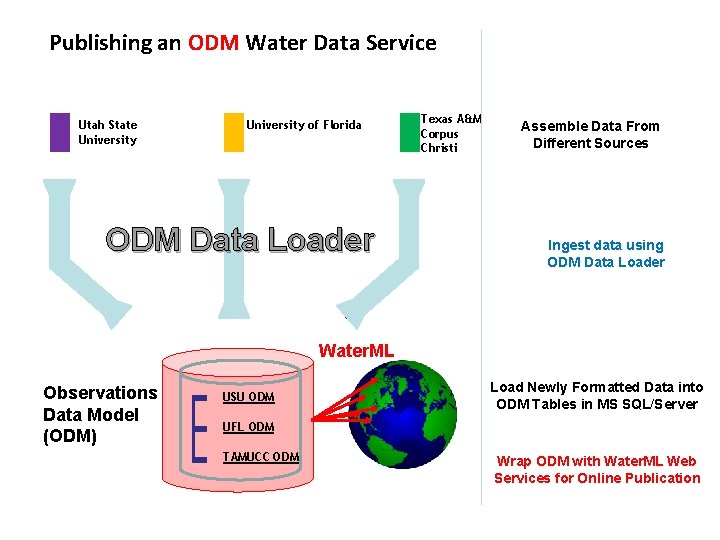
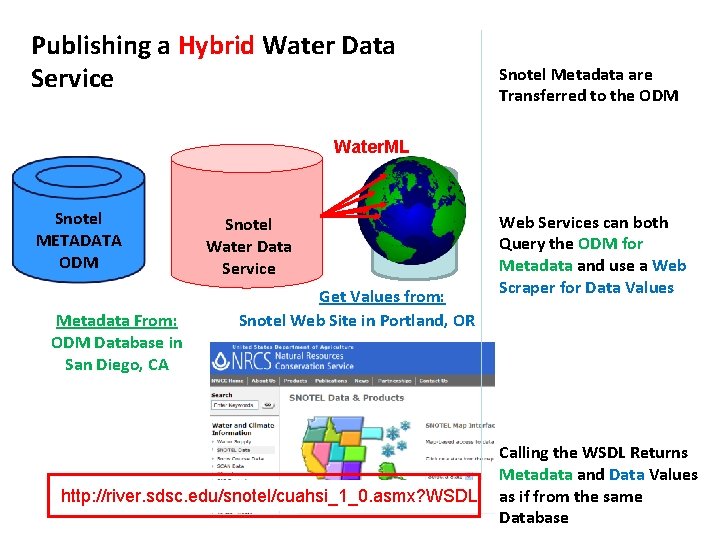
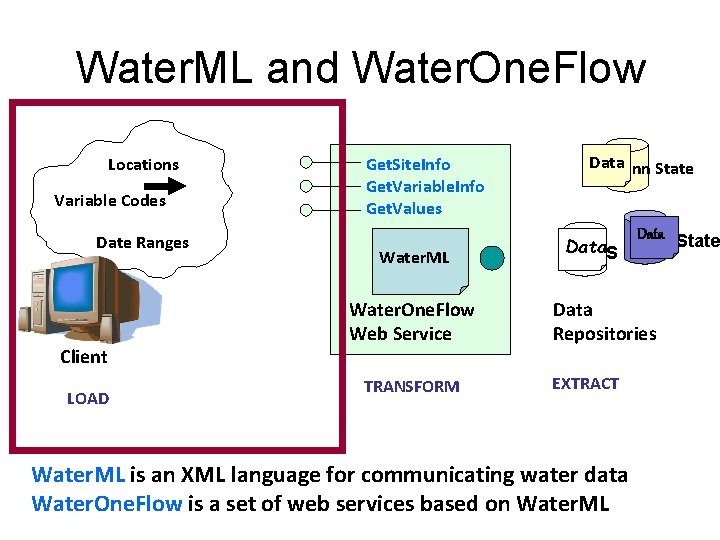
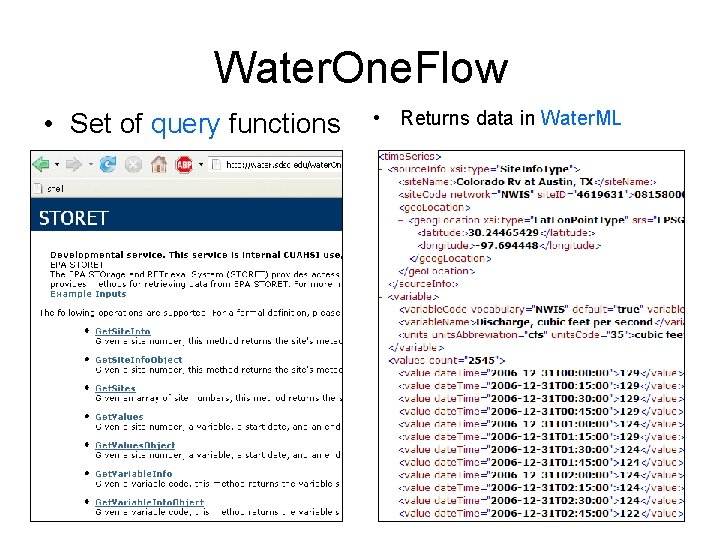
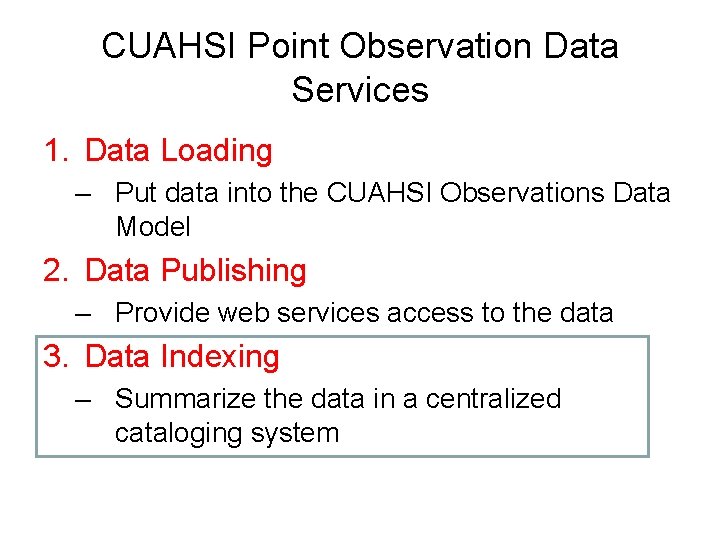
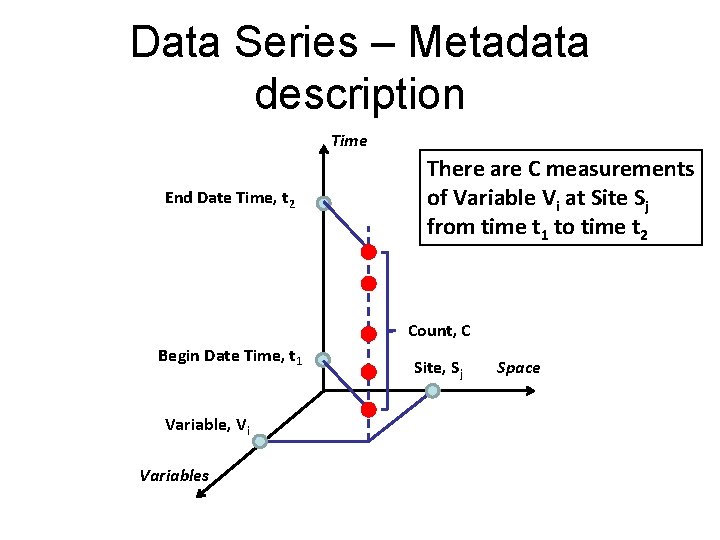
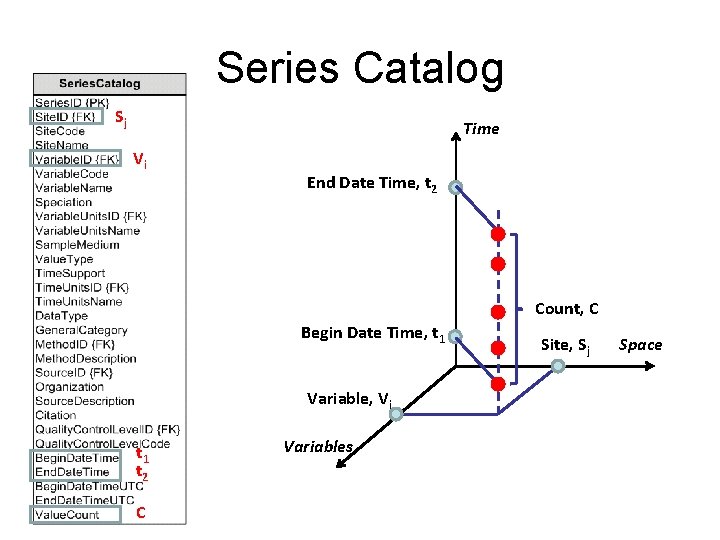
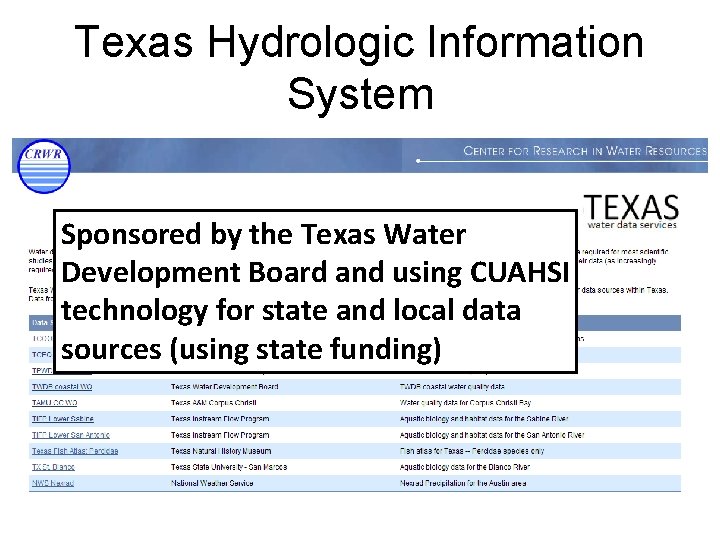
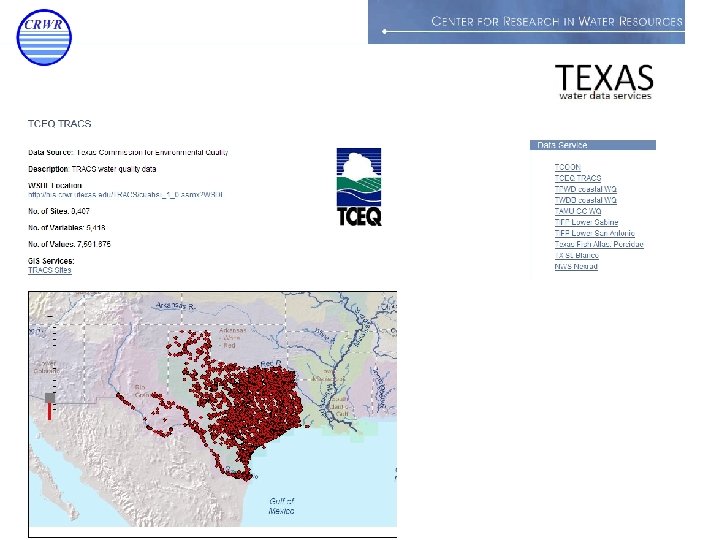
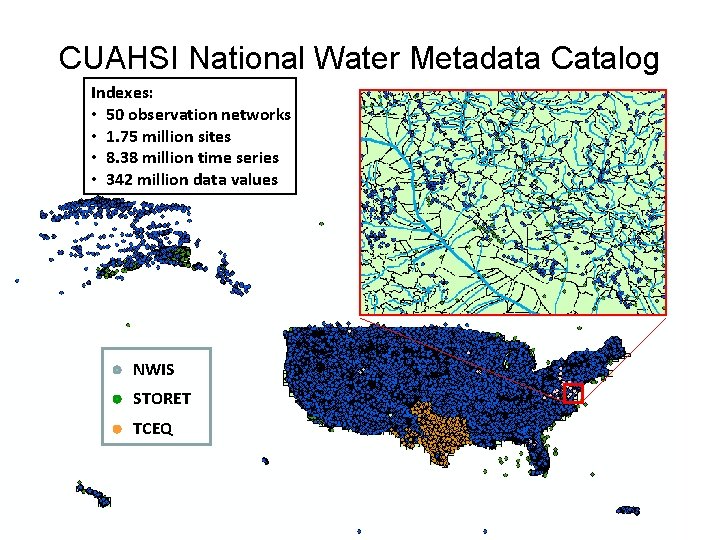
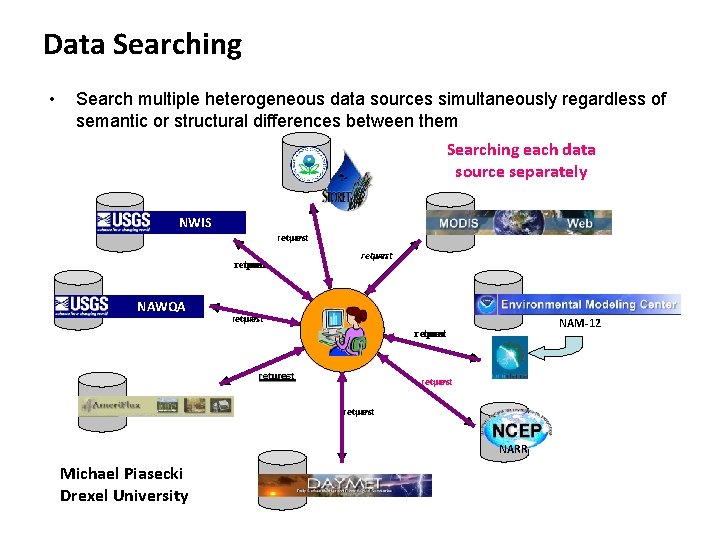
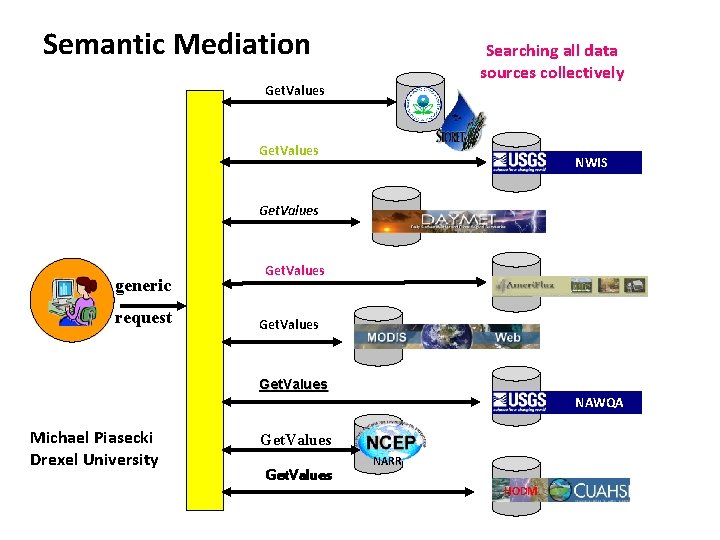
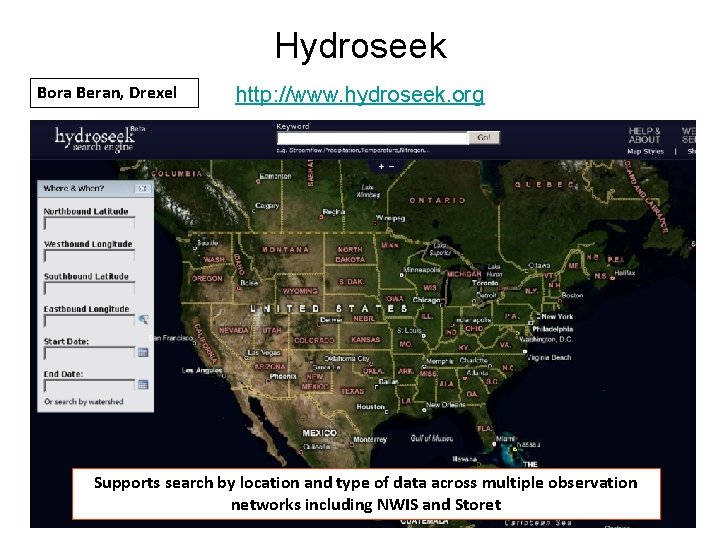
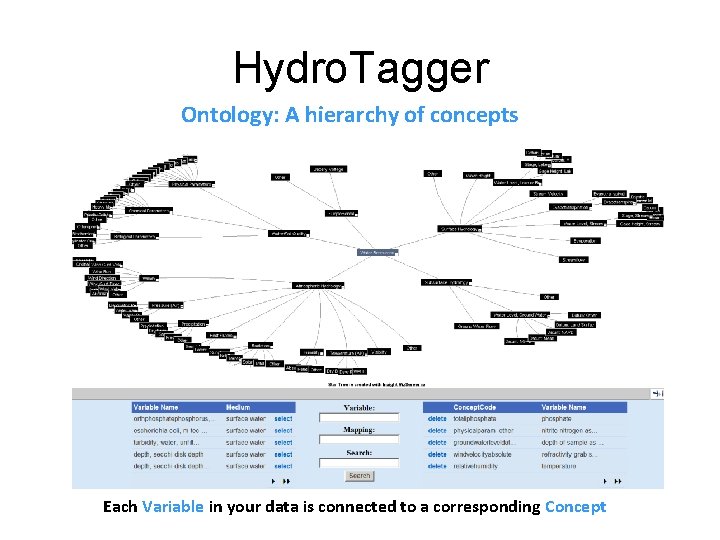
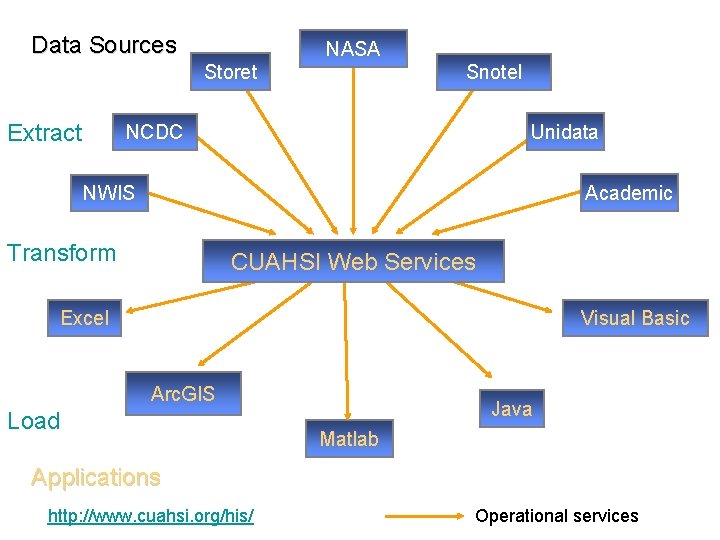
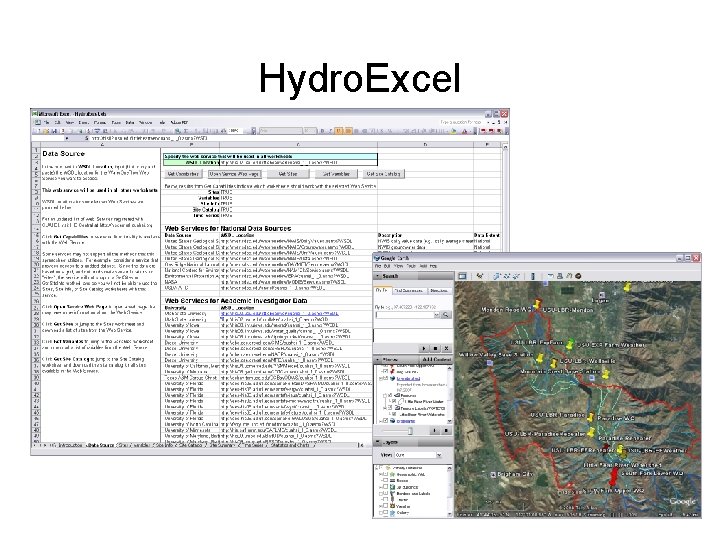
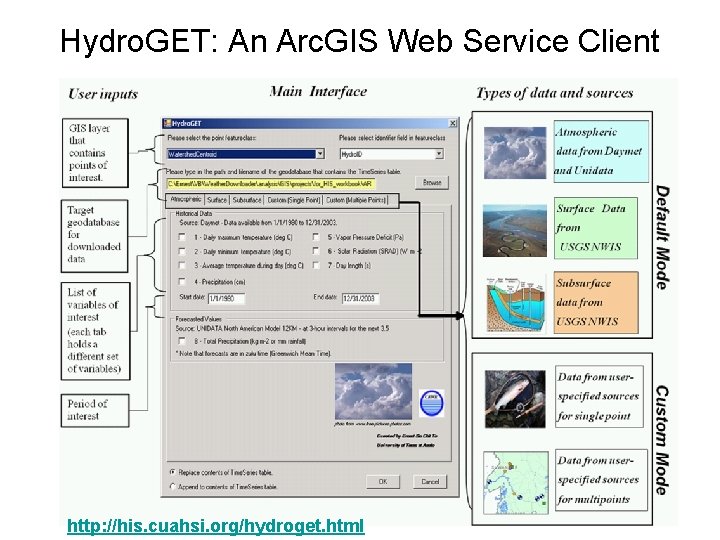
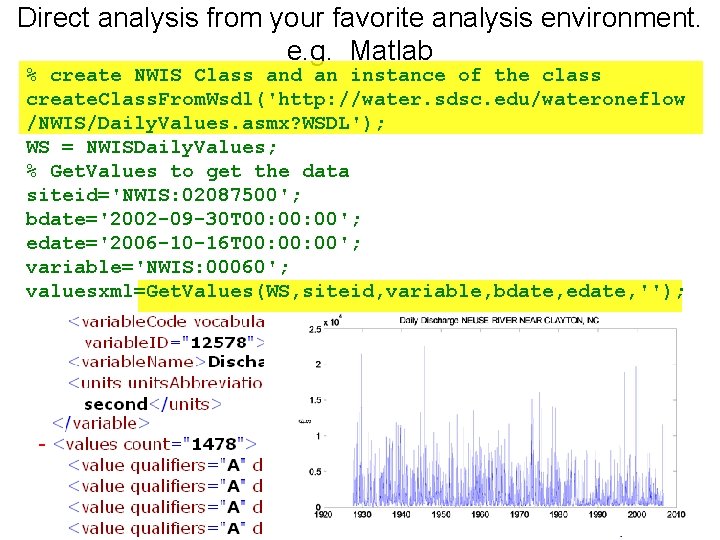
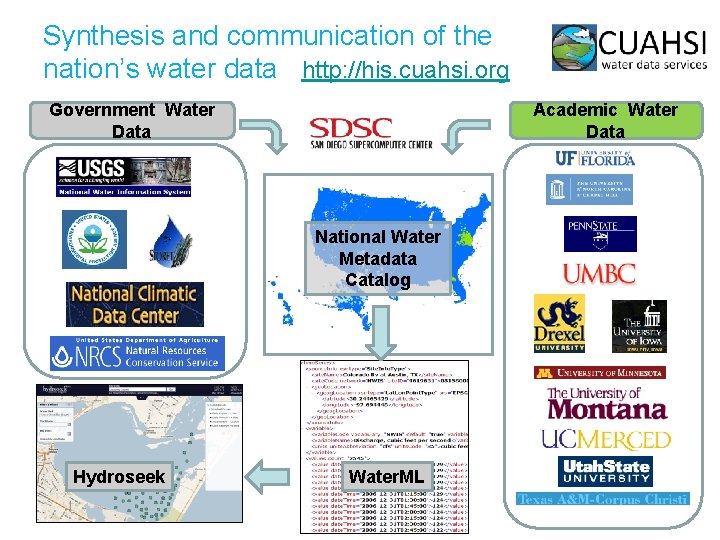
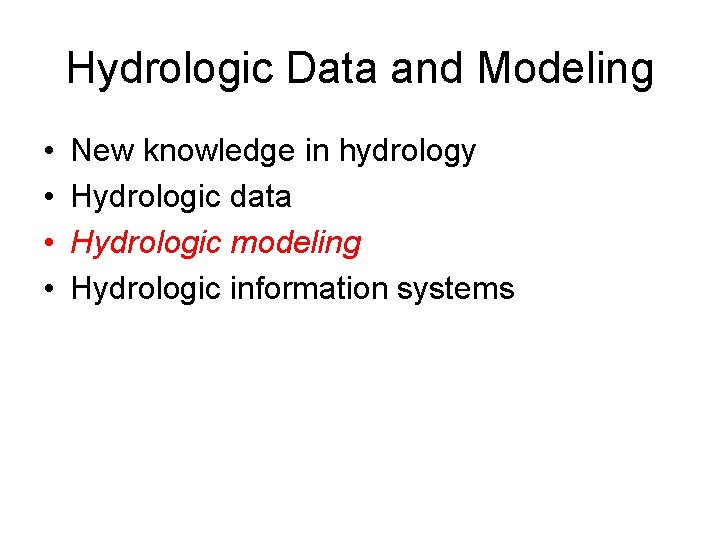
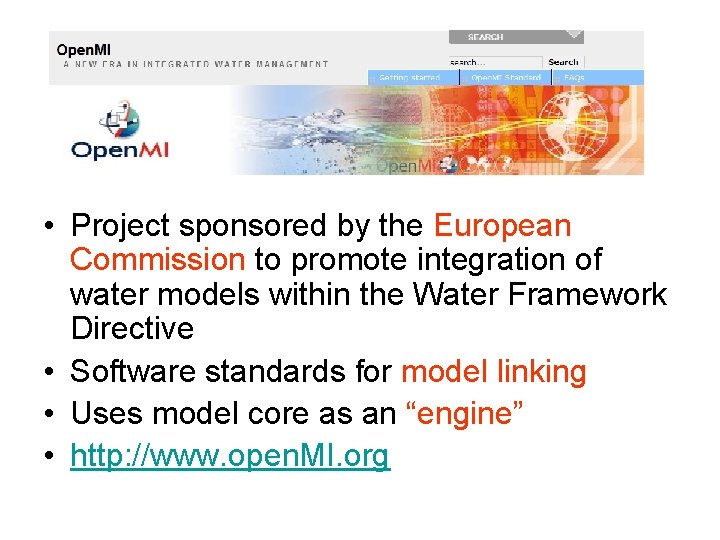
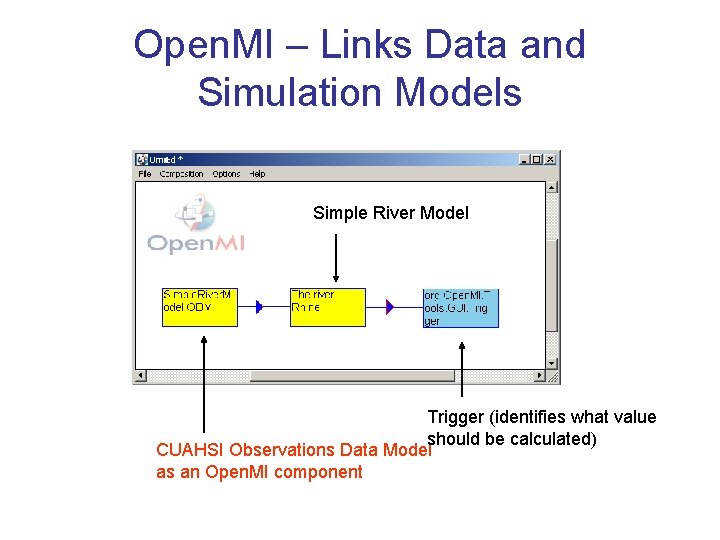
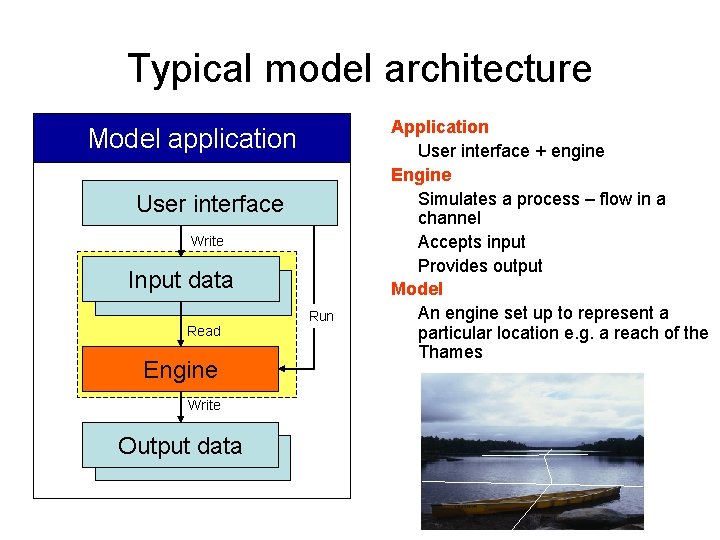
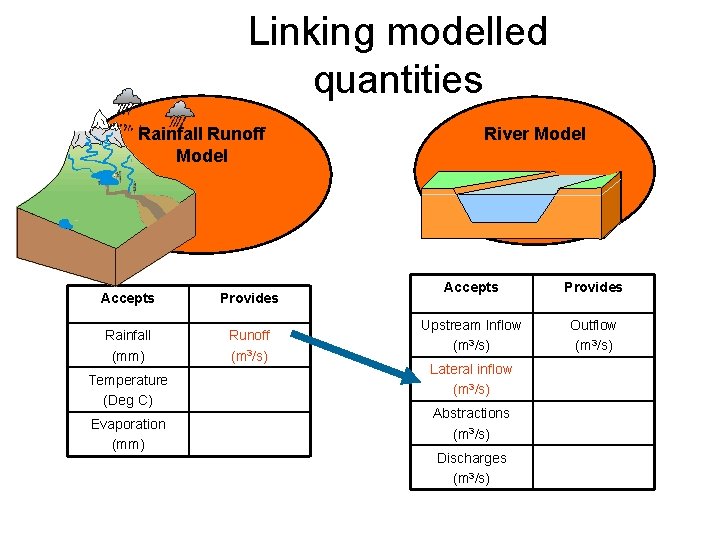
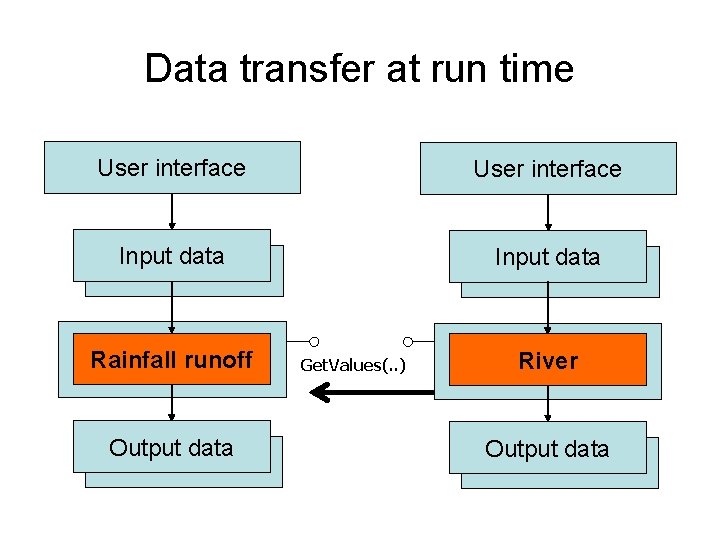
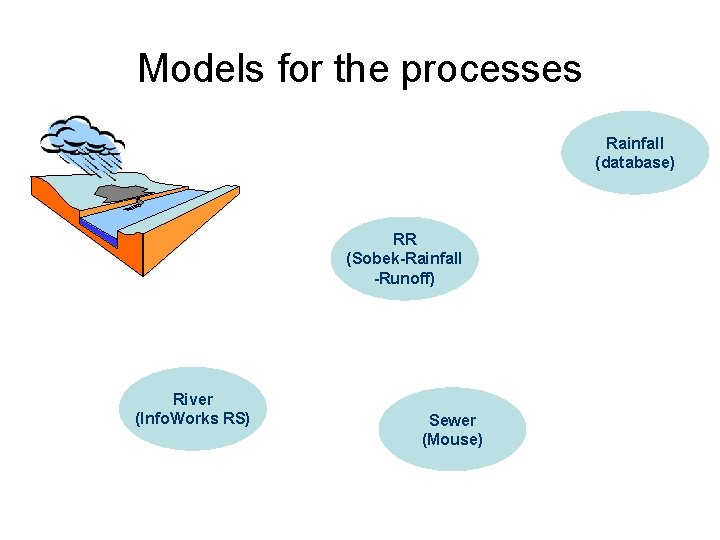
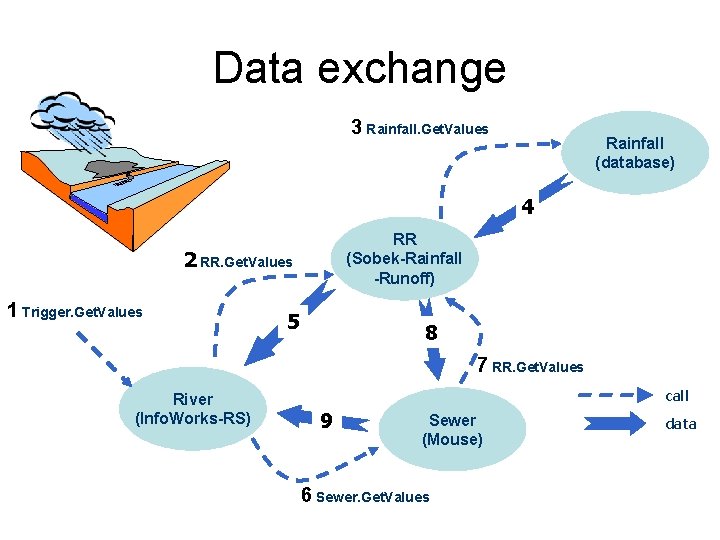
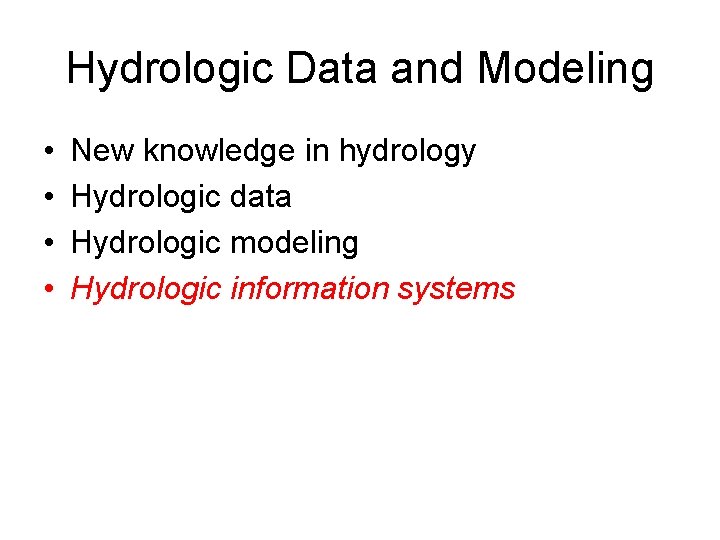
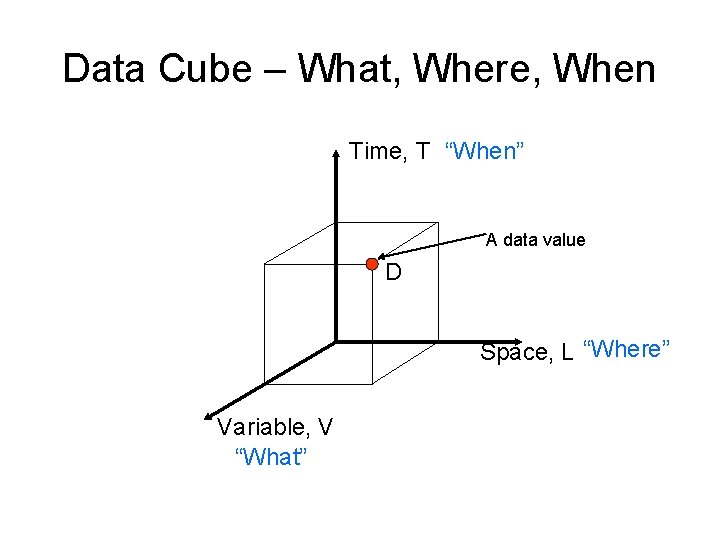
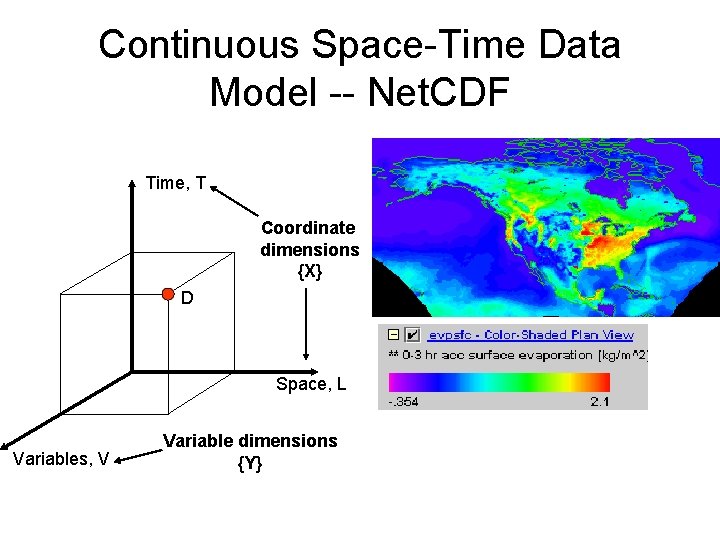
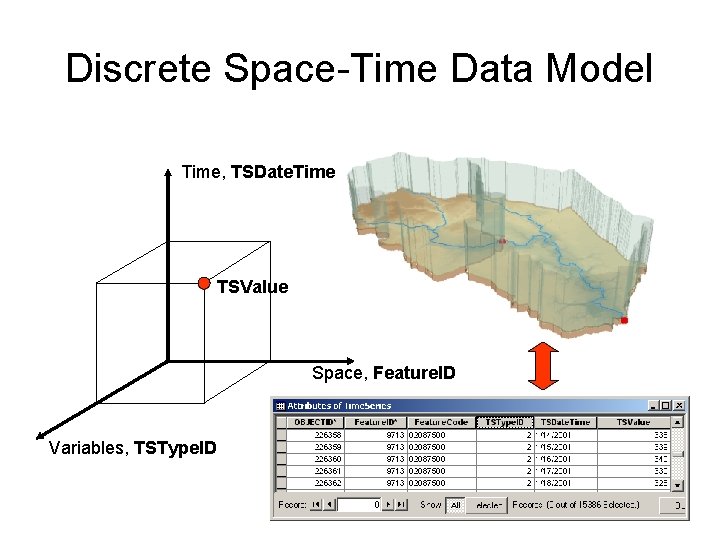
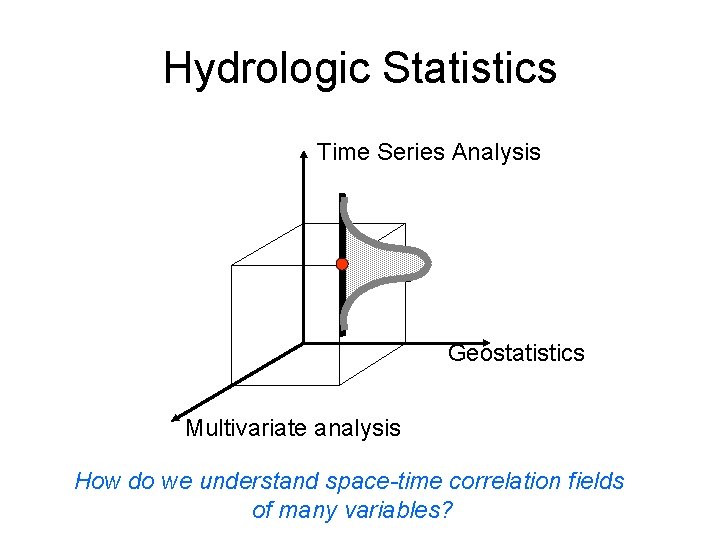
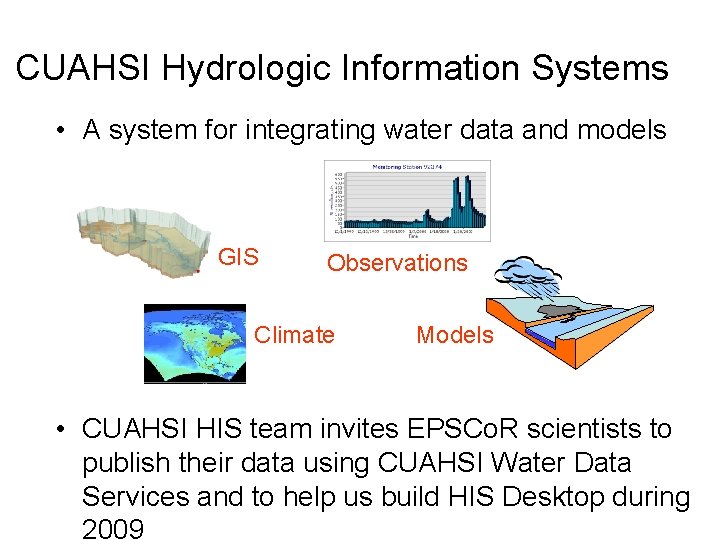
- Slides: 62
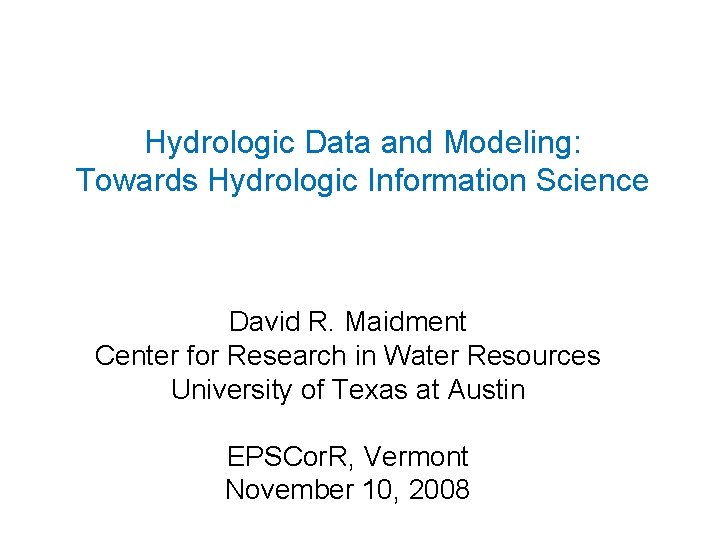
Hydrologic Data and Modeling: Towards Hydrologic Information Science David R. Maidment Center for Research in Water Resources University of Texas at Austin EPSCor. R, Vermont November 10, 2008
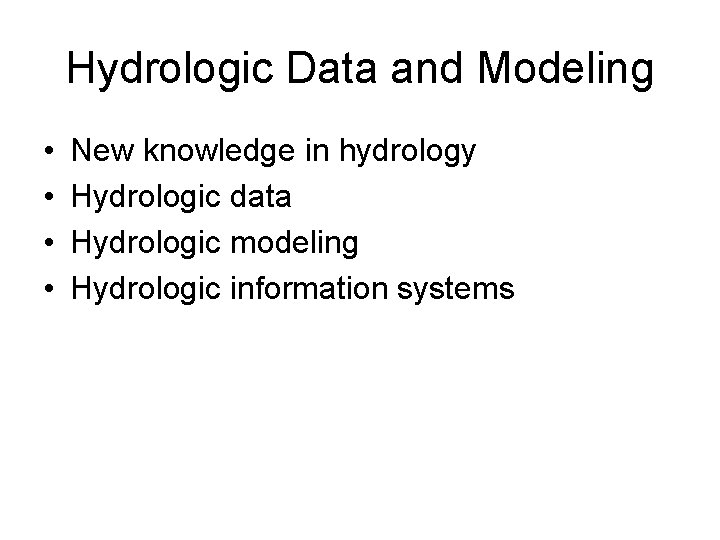
Hydrologic Data and Modeling • • New knowledge in hydrology Hydrologic data Hydrologic modeling Hydrologic information systems
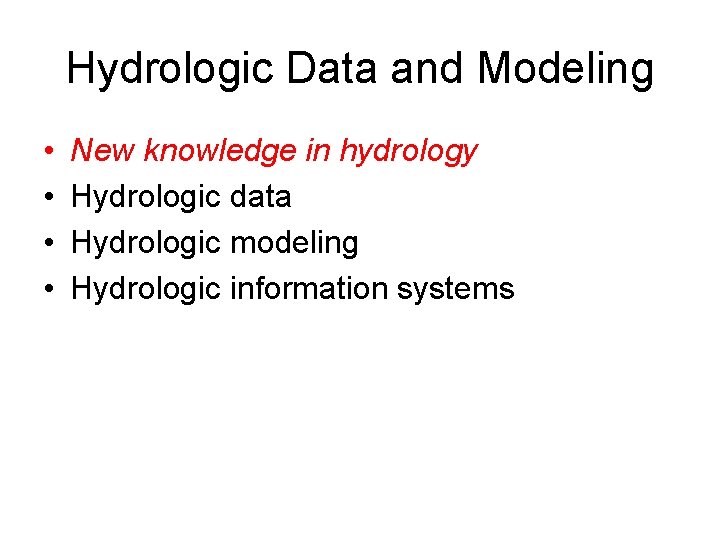
Hydrologic Data and Modeling • • New knowledge in hydrology Hydrologic data Hydrologic modeling Hydrologic information systems
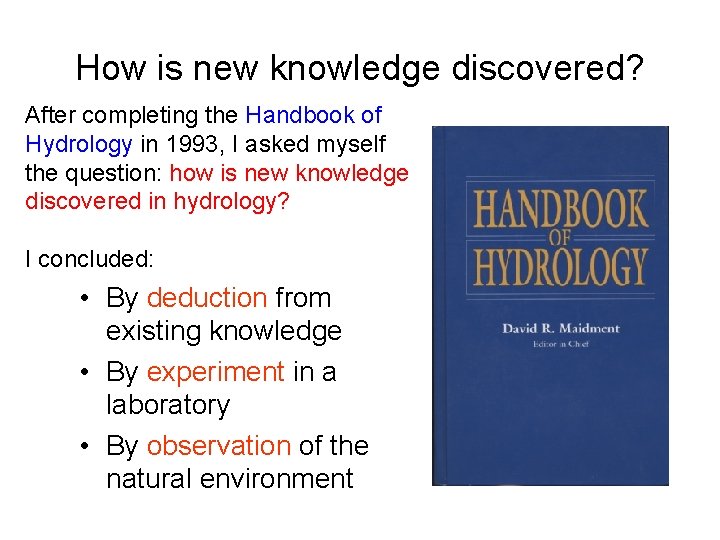
How is new knowledge discovered? After completing the Handbook of Hydrology in 1993, I asked myself the question: how is new knowledge discovered in hydrology? I concluded: • By deduction from existing knowledge • By experiment in a laboratory • By observation of the natural environment
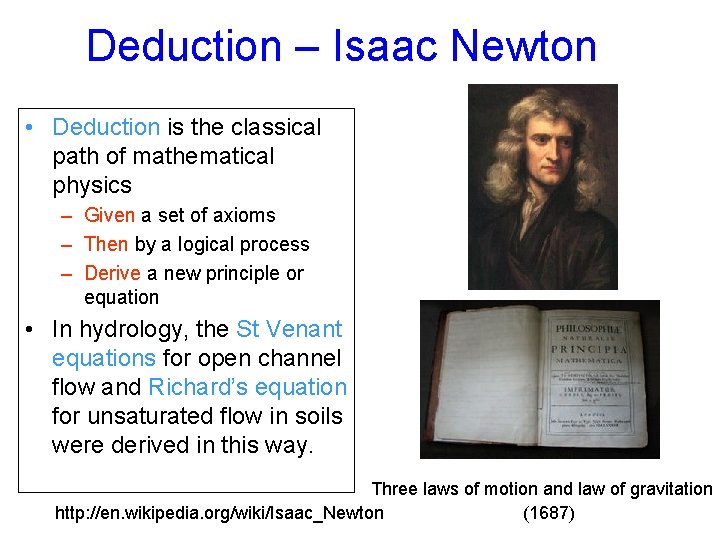
Deduction – Isaac Newton • Deduction is the classical path of mathematical physics – Given a set of axioms – Then by a logical process – Derive a new principle or equation • In hydrology, the St Venant equations for open channel flow and Richard’s equation for unsaturated flow in soils were derived in this way. Three laws of motion and law of gravitation http: //en. wikipedia. org/wiki/Isaac_Newton (1687)
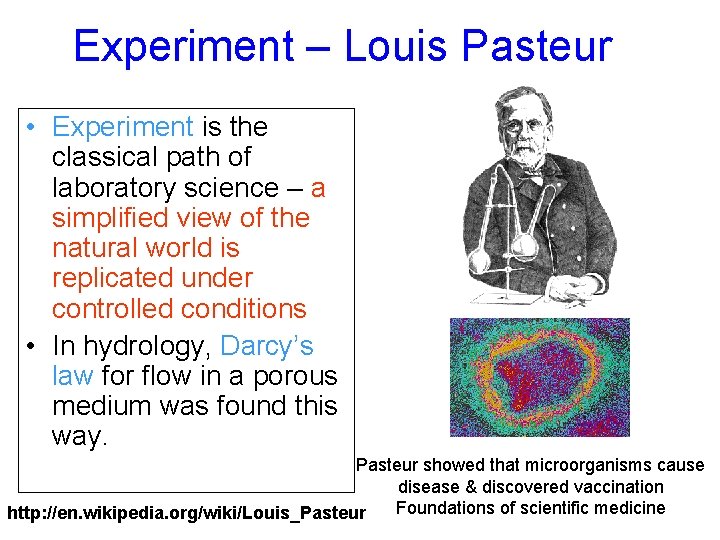
Experiment – Louis Pasteur • Experiment is the classical path of laboratory science – a simplified view of the natural world is replicated under controlled conditions • In hydrology, Darcy’s law for flow in a porous medium was found this way. Pasteur showed that microorganisms cause disease & discovered vaccination Foundations of scientific medicine http: //en. wikipedia. org/wiki/Louis_Pasteur
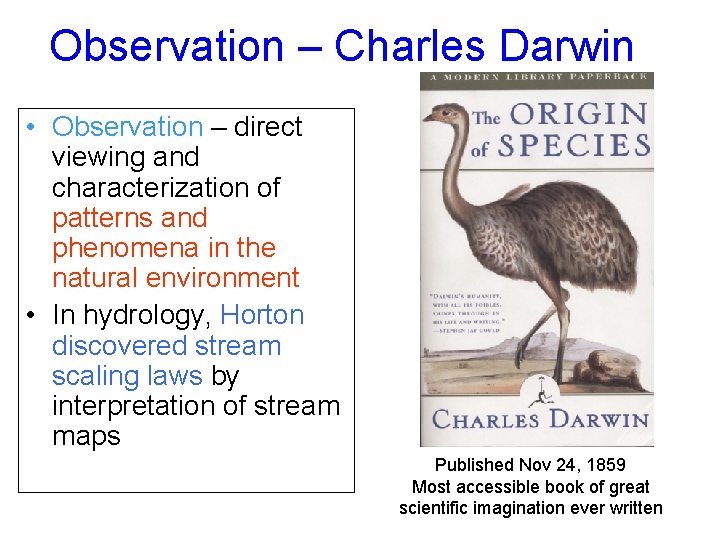
Observation – Charles Darwin • Observation – direct viewing and characterization of patterns and phenomena in the natural environment • In hydrology, Horton discovered stream scaling laws by interpretation of stream maps Published Nov 24, 1859 Most accessible book of great scientific imagination ever written
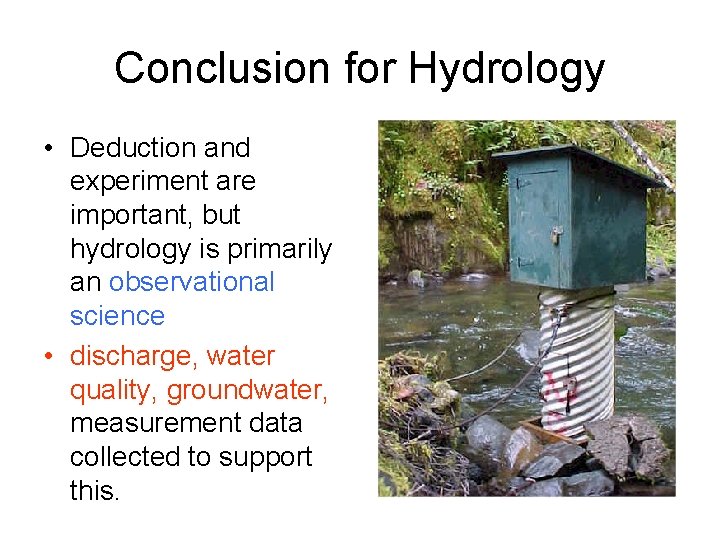
Conclusion for Hydrology • Deduction and experiment are important, but hydrology is primarily an observational science • discharge, water quality, groundwater, measurement data collected to support this.
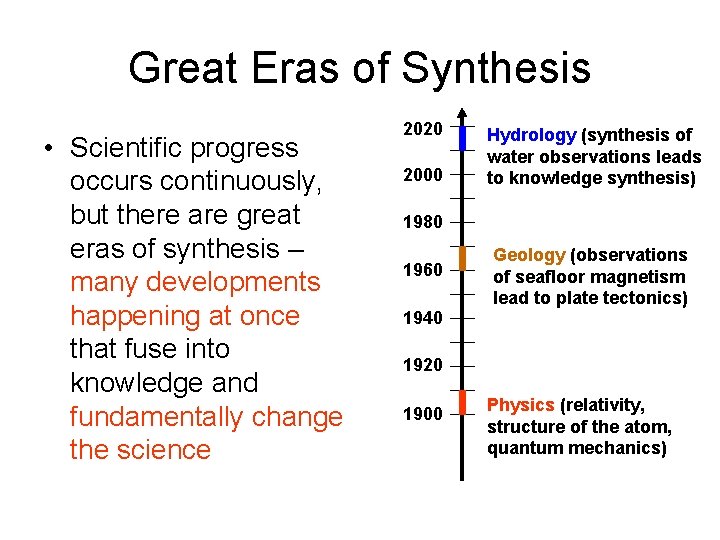
Great Eras of Synthesis • Scientific progress occurs continuously, but there are great eras of synthesis – many developments happening at once that fuse into knowledge and fundamentally change the science 2020 2000 Hydrology (synthesis of water observations leads to knowledge synthesis) 1980 1960 1940 Geology (observations of seafloor magnetism lead to plate tectonics) 1920 1900 Physics (relativity, structure of the atom, quantum mechanics)
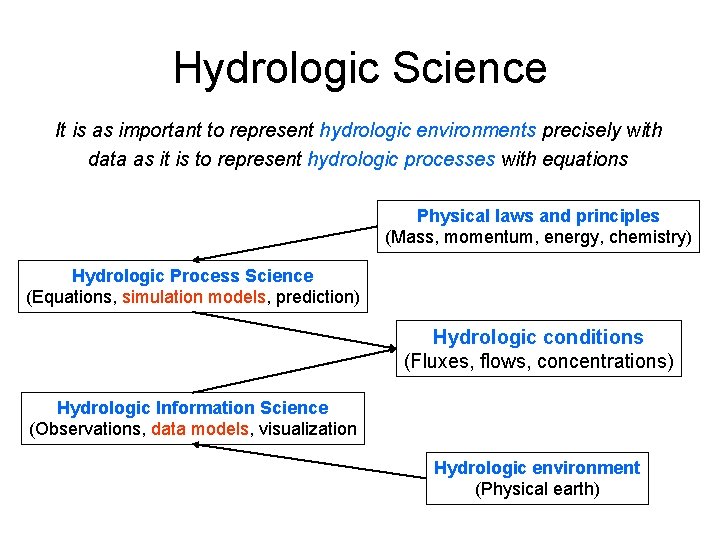
Hydrologic Science It is as important to represent hydrologic environments precisely with data as it is to represent hydrologic processes with equations Physical laws and principles (Mass, momentum, energy, chemistry) Hydrologic Process Science (Equations, simulation models, prediction) Hydrologic conditions (Fluxes, flows, concentrations) Hydrologic Information Science (Observations, data models, visualization Hydrologic environment (Physical earth)
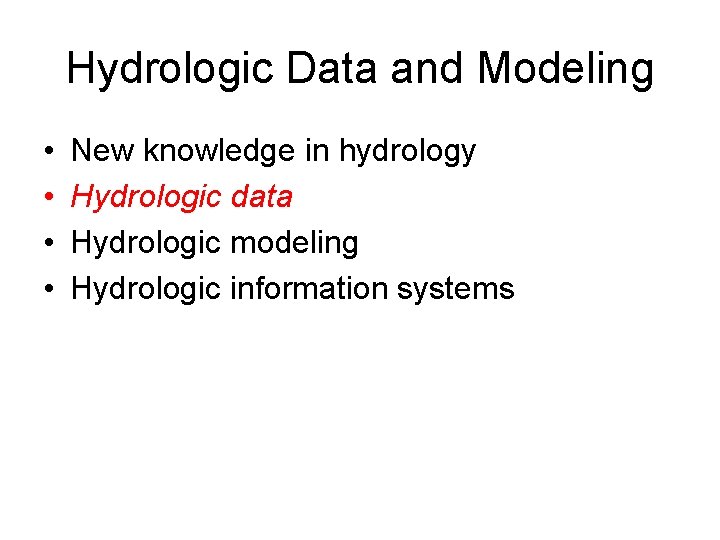
Hydrologic Data and Modeling • • New knowledge in hydrology Hydrologic data Hydrologic modeling Hydrologic information systems
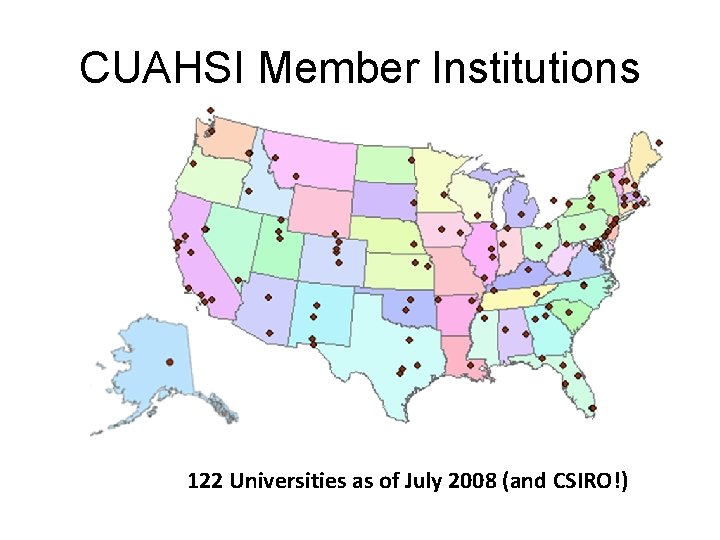
CUAHSI Member Institutions 122 Universities as of July 2008 (and CSIRO!)
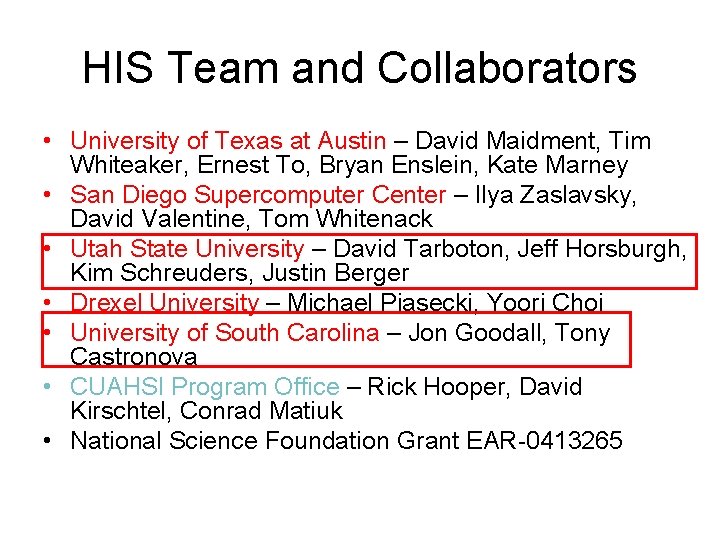
HIS Team and Collaborators • University of Texas at Austin – David Maidment, Tim Whiteaker, Ernest To, Bryan Enslein, Kate Marney • San Diego Supercomputer Center – Ilya Zaslavsky, David Valentine, Tom Whitenack • Utah State University – David Tarboton, Jeff Horsburgh, Kim Schreuders, Justin Berger • Drexel University – Michael Piasecki, Yoori Choi • University of South Carolina – Jon Goodall, Tony Castronova • CUAHSI Program Office – Rick Hooper, David Kirschtel, Conrad Matiuk • National Science Foundation Grant EAR-0413265
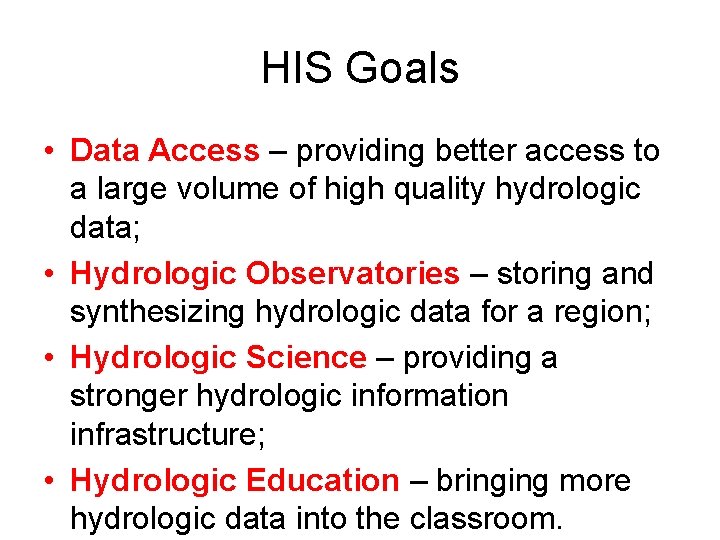
HIS Goals • Data Access – providing better access to a large volume of high quality hydrologic data; • Hydrologic Observatories – storing and synthesizing hydrologic data for a region; • Hydrologic Science – providing a stronger hydrologic information infrastructure; • Hydrologic Education – bringing more hydrologic data into the classroom.
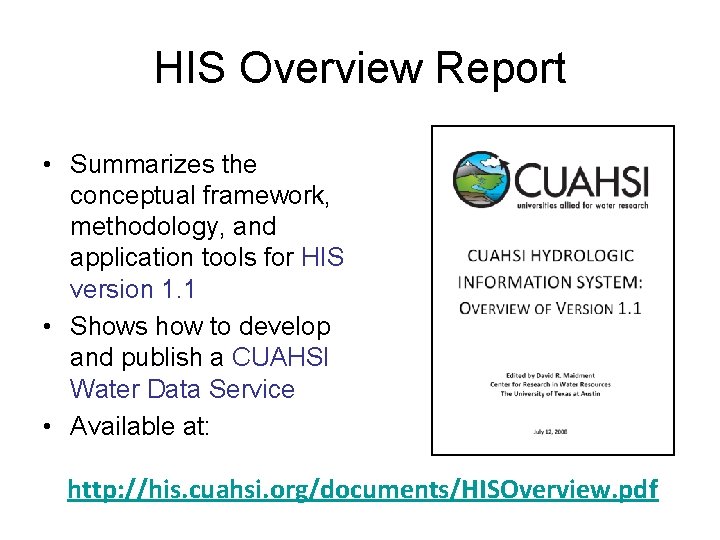
HIS Overview Report • Summarizes the conceptual framework, methodology, and application tools for HIS version 1. 1 • Shows how to develop and publish a CUAHSI Water Data Service • Available at: http: //his. cuahsi. org/documents/HISOverview. pdf
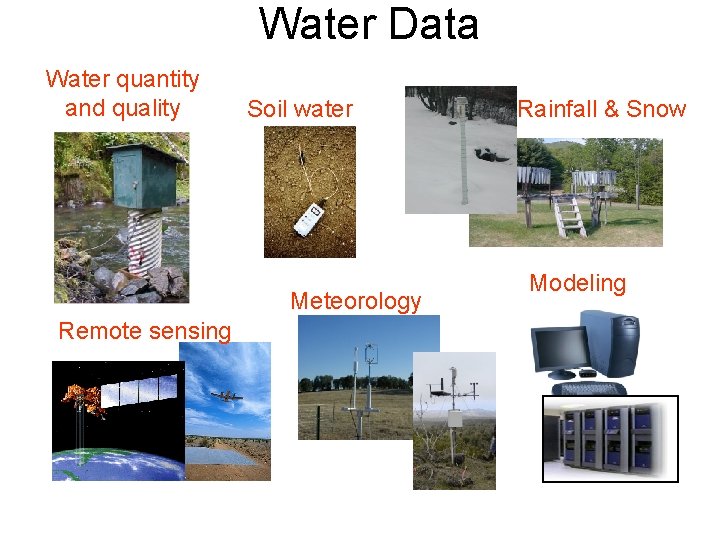
Water Data Water quantity and quality Soil water Meteorology Remote sensing Rainfall & Snow Modeling
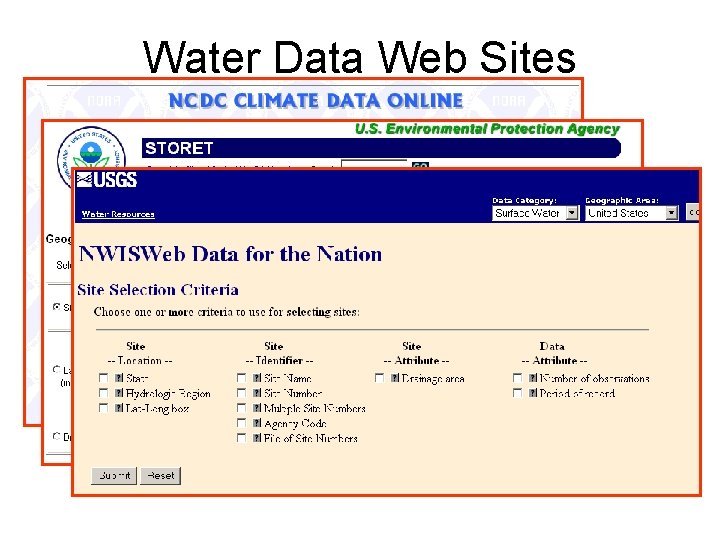
Water Data Web Sites
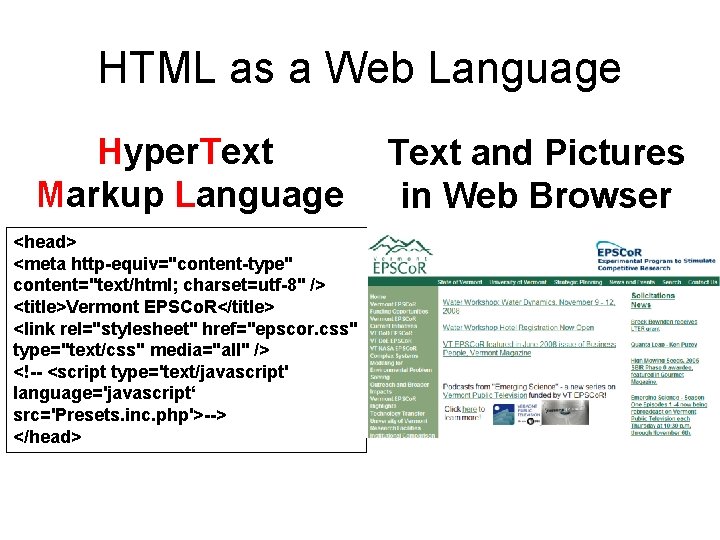
HTML as a Web Language Hyper. Text Markup Language <head> <meta http-equiv="content-type" content="text/html; charset=utf-8" /> <title>Vermont EPSCo. R</title> <link rel="stylesheet" href="epscor. css" type="text/css" media="all" /> <!-- <script type='text/javascript' language='javascript‘ src='Presets. inc. php'>--> </head> Text and Pictures in Web Browser
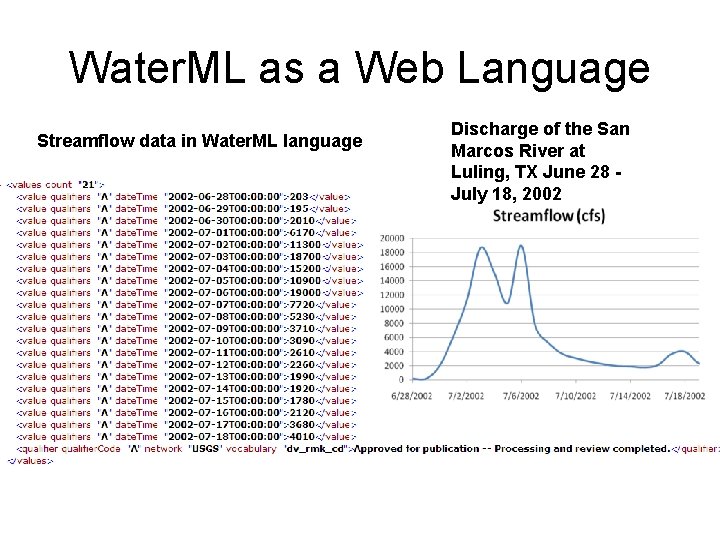
Water. ML as a Web Language Streamflow data in Water. ML language Discharge of the San Marcos River at Luling, TX June 28 July 18, 2002
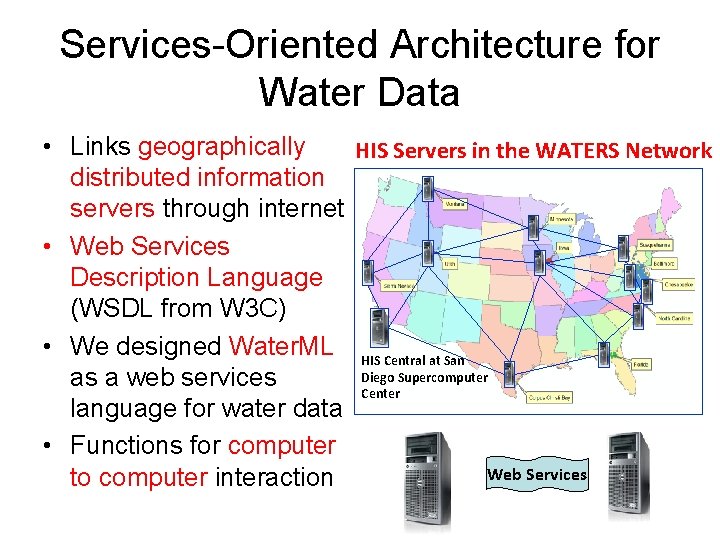
Services-Oriented Architecture for Water Data • Links geographically HIS Servers in the WATERS Network distributed information servers through internet • Web Services Description Language (WSDL from W 3 C) • We designed Water. ML HIS Central at San Diego Supercomputer as a web services Center language for water data • Functions for computer Web Services to computer interaction
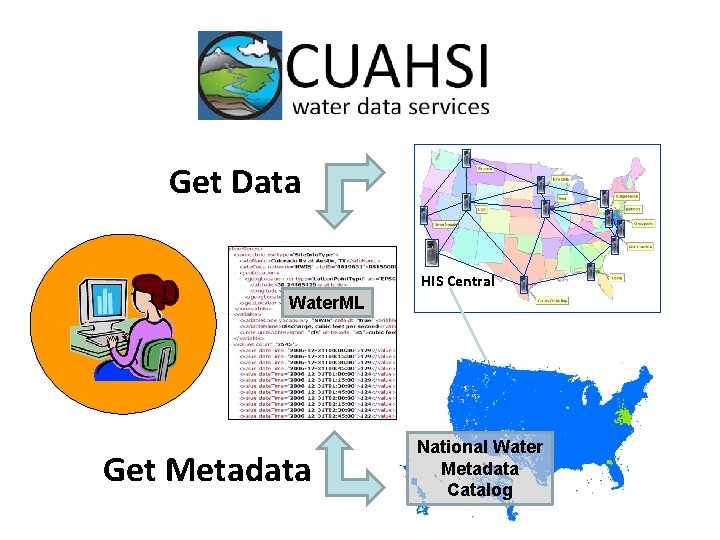
Get Data HIS Central Water. ML Get Metadata National Water Metadata Catalog
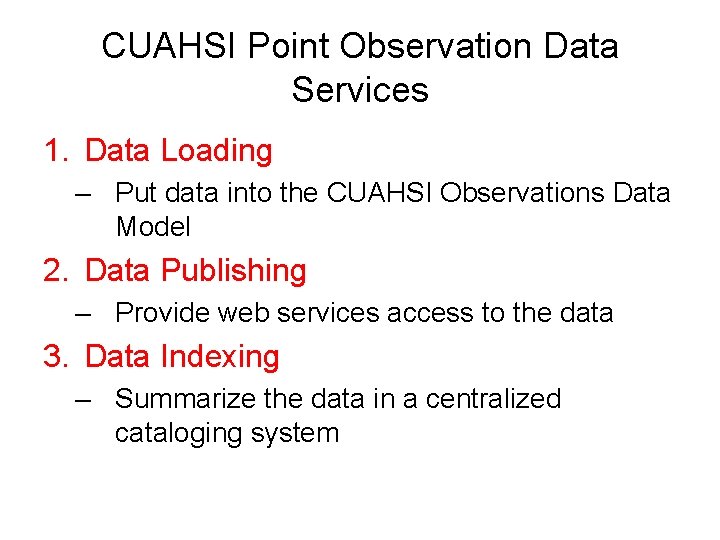
CUAHSI Point Observation Data Services 1. Data Loading – Put data into the CUAHSI Observations Data Model 2. Data Publishing – Provide web services access to the data 3. Data Indexing – Summarize the data in a centralized cataloging system
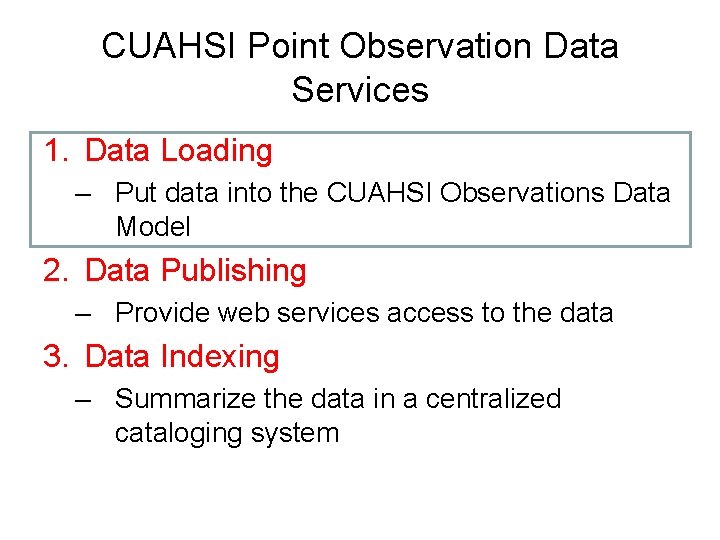
CUAHSI Point Observation Data Services 1. Data Loading – Put data into the CUAHSI Observations Data Model 2. Data Publishing – Provide web services access to the data 3. Data Indexing – Summarize the data in a centralized cataloging system
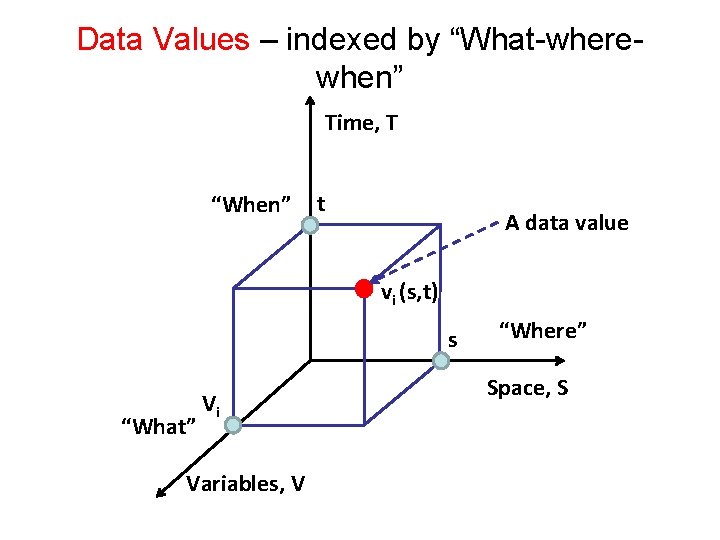
Data Values – indexed by “What-wherewhen” Time, T “When” t A data value vi (s, t) s “What” Vi Variables, V “Where” Space, S
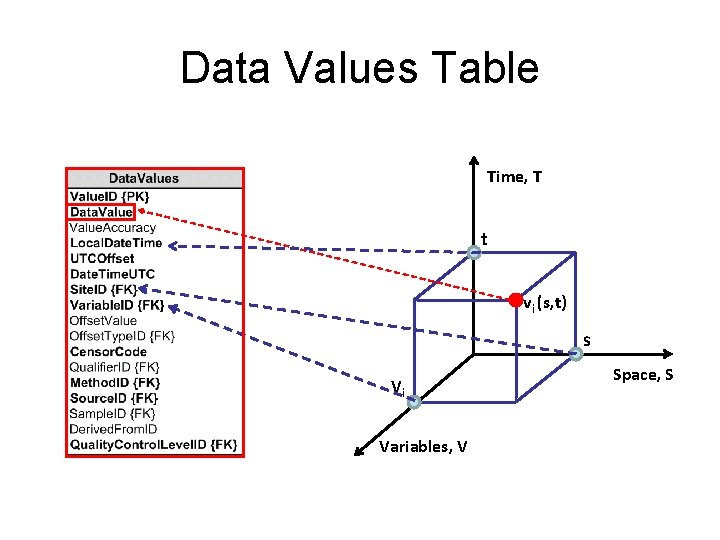
Data Values Table Time, T t vi (s, t) s Vi Variables, V Space, S
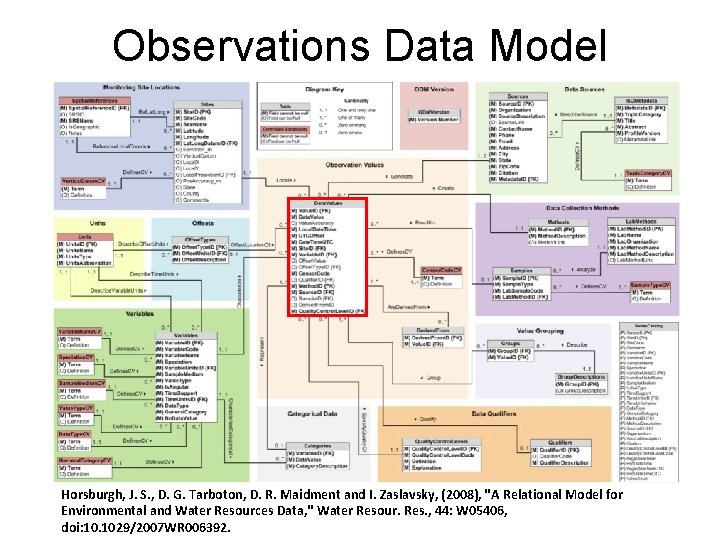
Observations Data Model Horsburgh, J. S. , D. G. Tarboton, D. R. Maidment and I. Zaslavsky, (2008), "A Relational Model for Environmental and Water Resources Data, " Water Resour. Res. , 44: W 05406, doi: 10. 1029/2007 WR 006392.
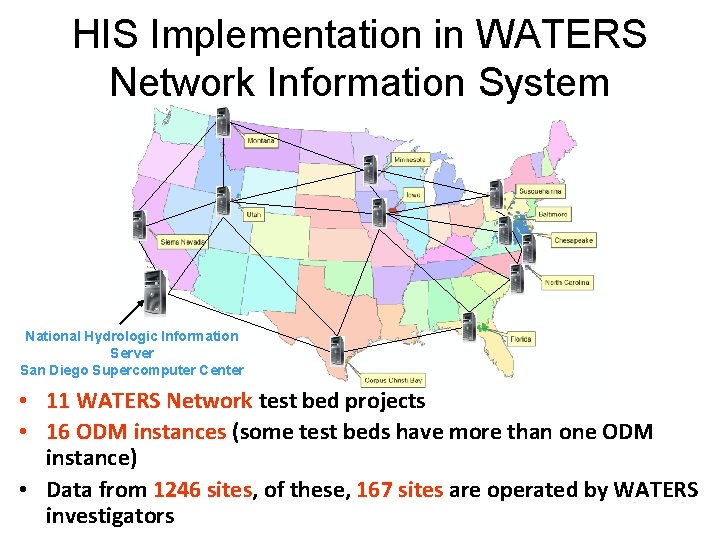
HIS Implementation in WATERS Network Information System National Hydrologic Information Server San Diego Supercomputer Center • 11 WATERS Network test bed projects • 16 ODM instances (some test beds have more than one ODM instance) • Data from 1246 sites, of these, 167 sites are operated by WATERS investigators
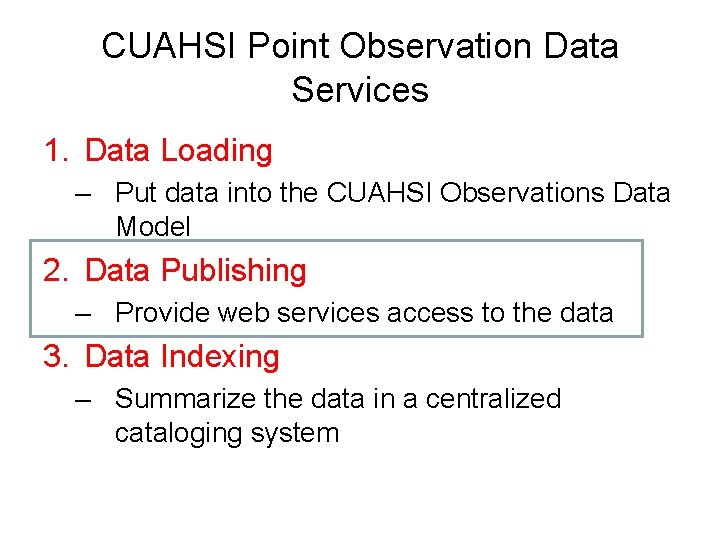
CUAHSI Point Observation Data Services 1. Data Loading – Put data into the CUAHSI Observations Data Model 2. Data Publishing – Provide web services access to the data 3. Data Indexing – Summarize the data in a centralized cataloging system
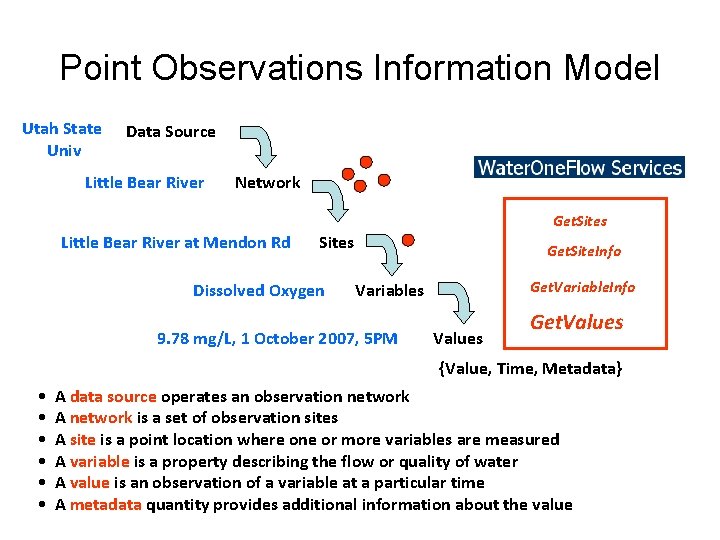
Point Observations Information Model Utah State Univ Data Source Little Bear River Network Get. Sites Little Bear River at Mendon Rd Sites Dissolved Oxygen Get. Site. Info Get. Variable. Info Variables 9. 78 mg/L, 1 October 2007, 5 PM Values Get. Values {Value, Time, Metadata} • • • A data source operates an observation network A network is a set of observation sites A site is a point location where one or more variables are measured A variable is a property describing the flow or quality of water A value is an observation of a variable at a particular time A metadata quantity provides additional information about the value
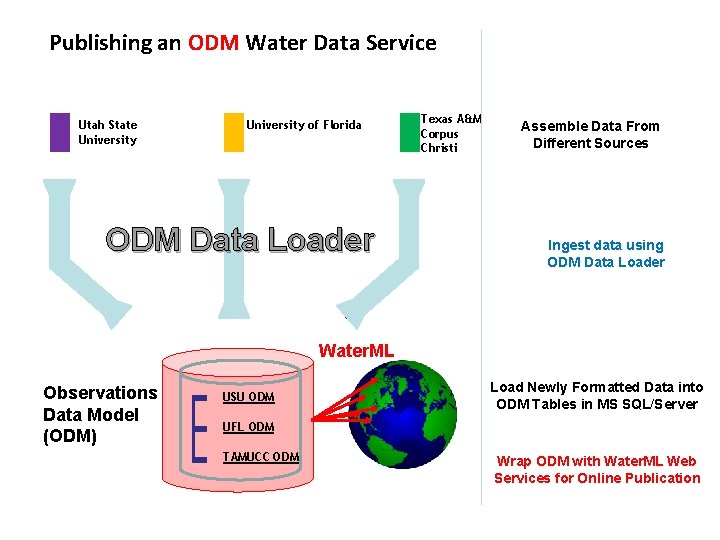
Publishing an ODM Water Data Service Utah State University of Florida ODM Data Loader Texas A&M Corpus Christi Assemble Data From Different Sources Ingest data using ODM Data Loader Water. ML Observations Data Model (ODM) USU ODM Load Newly Formatted Data into ODM Tables in MS SQL/Server UFL ODM TAMUCC ODM Wrap ODM with Water. ML Web Services for Online Publication
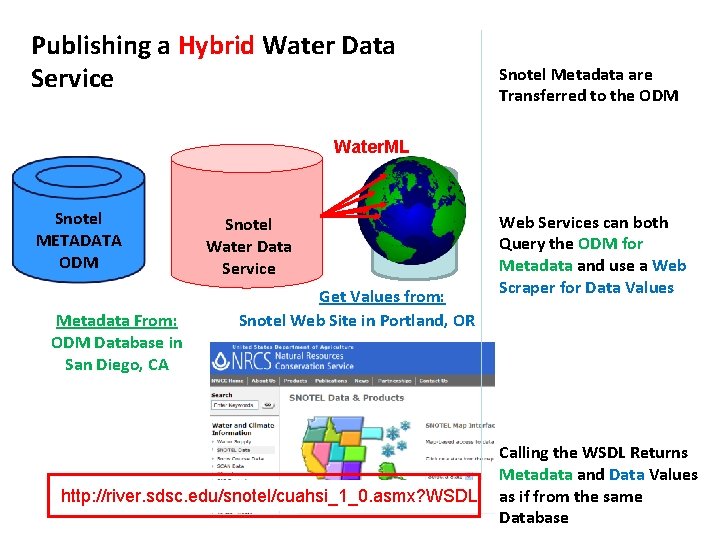
Publishing a Hybrid Water Data Service Snotel Metadata are Transferred to the ODM Water. ML Snotel METADATA ODM Metadata From: ODM Database in San Diego, CA Snotel Water Data Service Snotel Data. Values Get Values from: Snotel Web Site in Portland, OR http: //river. sdsc. edu/snotel/cuahsi_1_0. asmx? WSDL Web Services can both Query the ODM for Metadata and use a Web Scraper for Data Values Calling the WSDL Returns Metadata and Data Values as if from the same Database
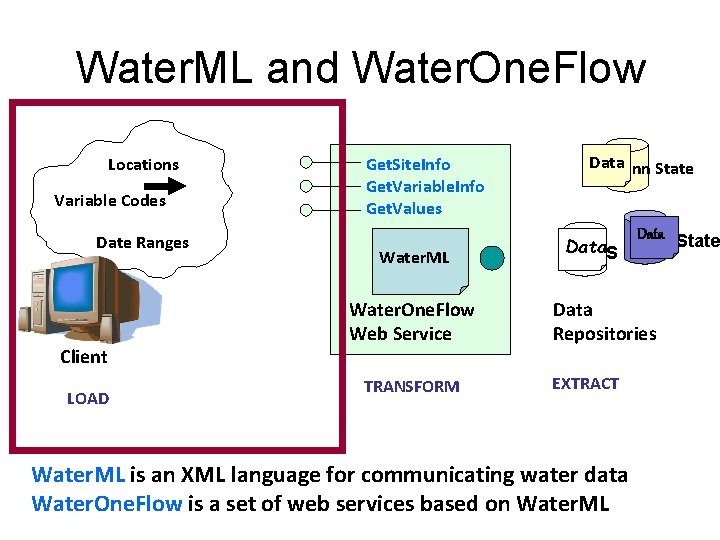
Water. ML and Water. One. Flow Locations Variable Codes Date Ranges Client LOAD Get. Site. Info Get. Variable. Info Get. Values Water. ML Water. One. Flow Web Service TRANSFORM Data Penn State Data NWIS Data State Utah Data Repositories EXTRACT Water. ML is an XML language for communicating water data Water. One. Flow is a set of web services based on Water. ML
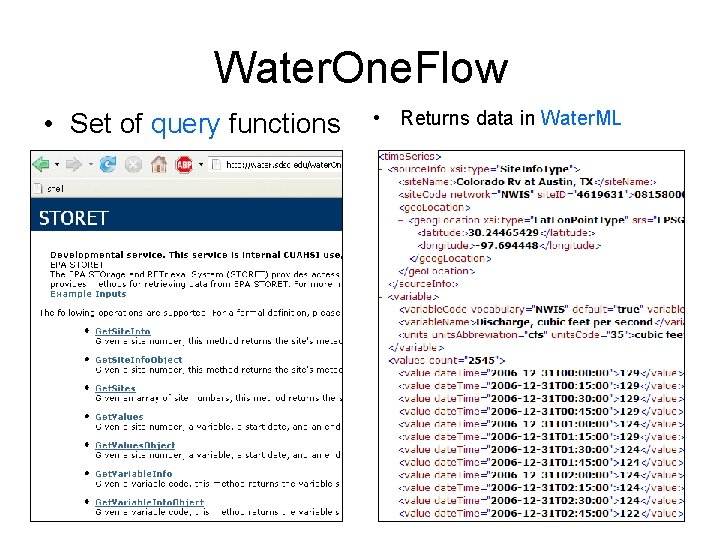
Water. One. Flow • Set of query functions • Returns data in Water. ML
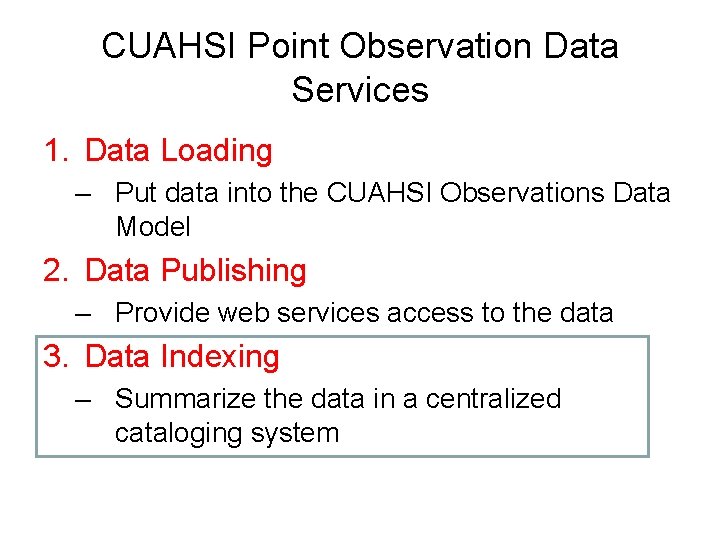
CUAHSI Point Observation Data Services 1. Data Loading – Put data into the CUAHSI Observations Data Model 2. Data Publishing – Provide web services access to the data 3. Data Indexing – Summarize the data in a centralized cataloging system
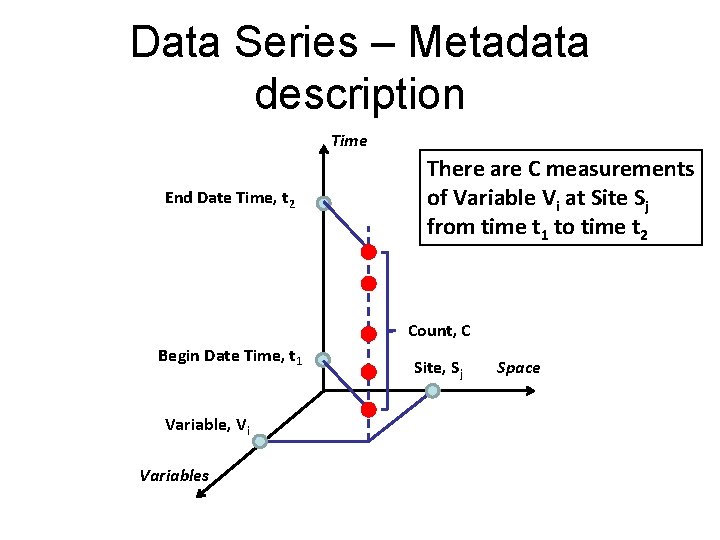
Data Series – Metadata description Time End Date Time, t 2 There are C measurements of Variable Vi at Site Sj from time t 1 to time t 2 Count, C Begin Date Time, t 1 Variable, Vi Variables Site, Sj Space
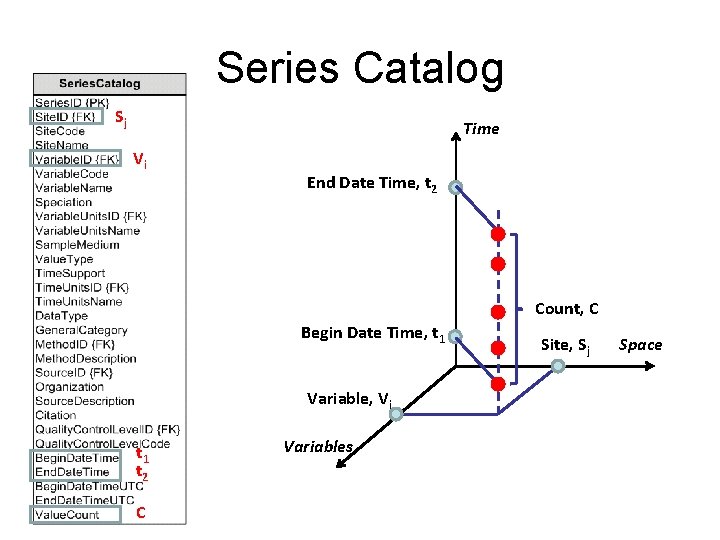
Series Catalog Sj Time Vi End Date Time, t 2 Count, C Begin Date Time, t 1 Variable, Vi t 1 t 2 C Variables Site, Sj Space
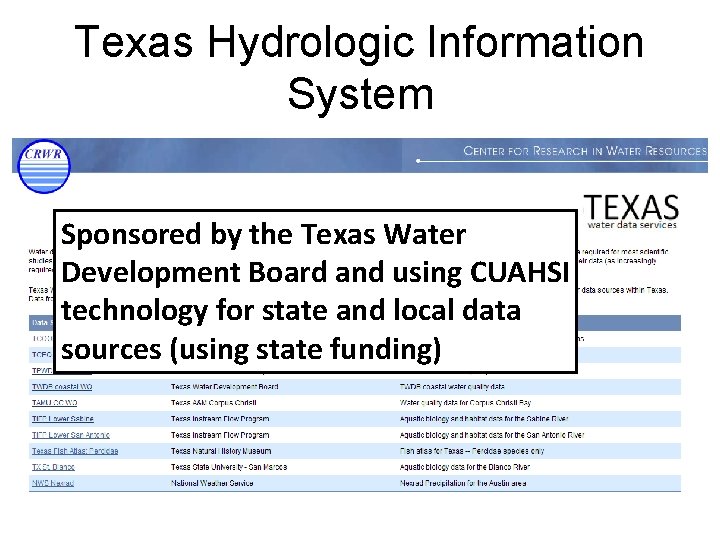
Texas Hydrologic Information System Sponsored by the Texas Water Development Board and using CUAHSI technology for state and local data sources (using state funding)
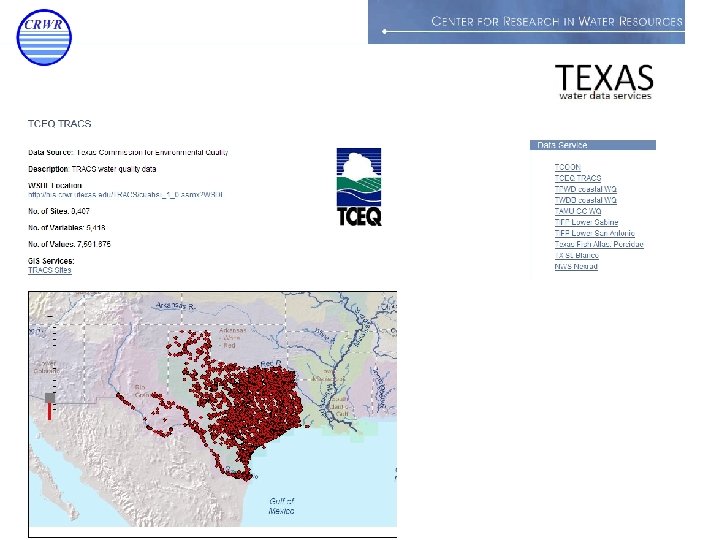
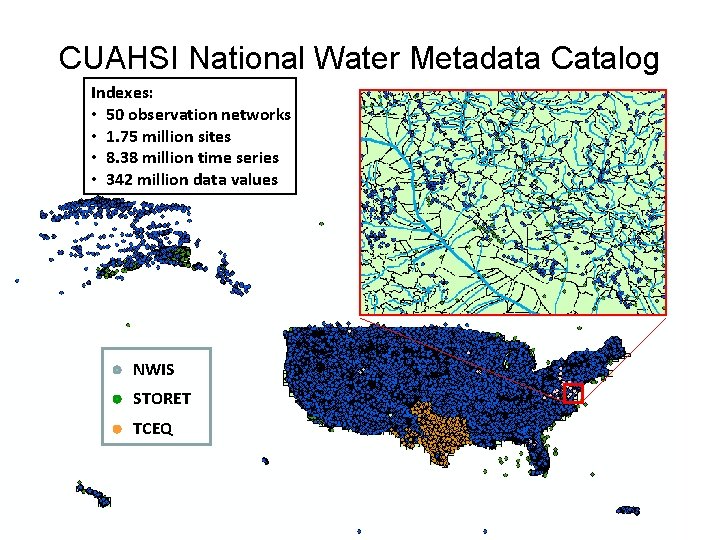
CUAHSI National Water Metadata Catalog Indexes: • 50 observation networks • 1. 75 million sites • 8. 38 million time series • 342 million data values NWIS STORET TCEQ
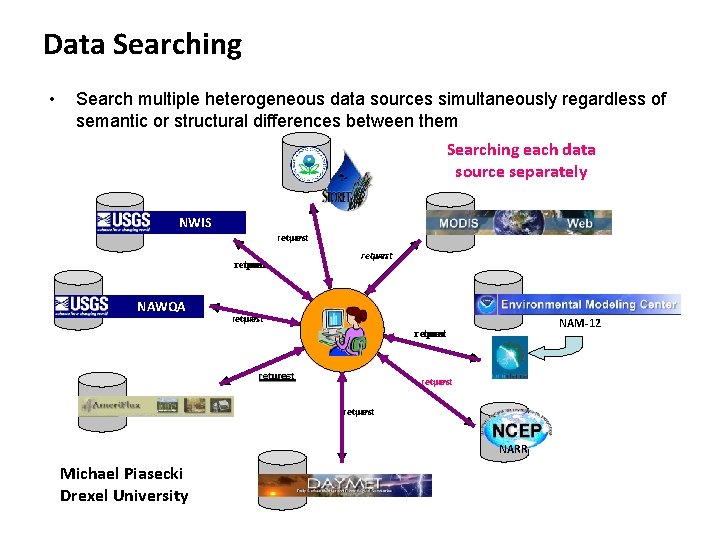
Data Searching • Search multiple heterogeneous data sources simultaneously regardless of semantic or structural differences between them Searching each data source separately NWIS request return NAWQA request return NAM-12 request return NARR Michael Piasecki Drexel University
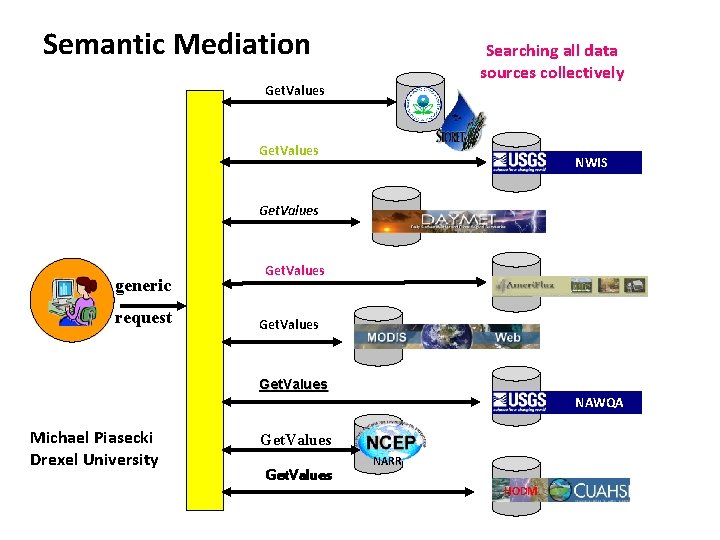
Semantic Mediation Searching all data sources collectively Get. Values NWIS Get. Values generic request Get. Values NAWQA Michael Piasecki Drexel University Get. Values NARR HODM
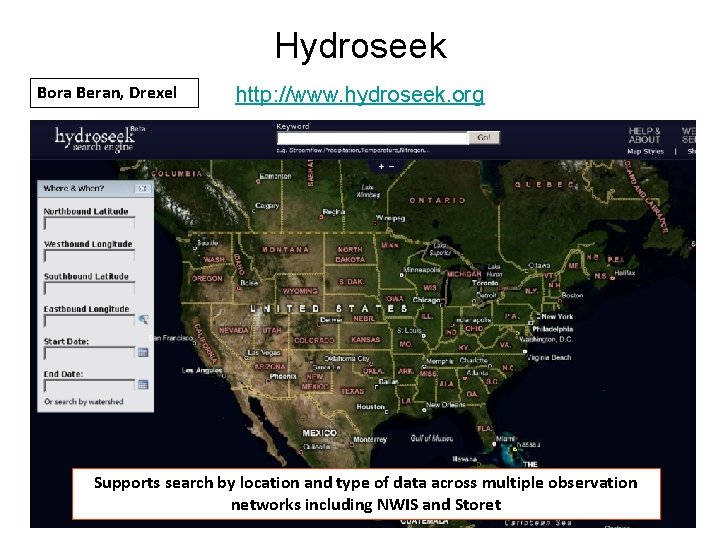
Hydroseek Bora Beran, Drexel http: //www. hydroseek. org Supports search by location and type of data across multiple observation networks including NWIS and Storet
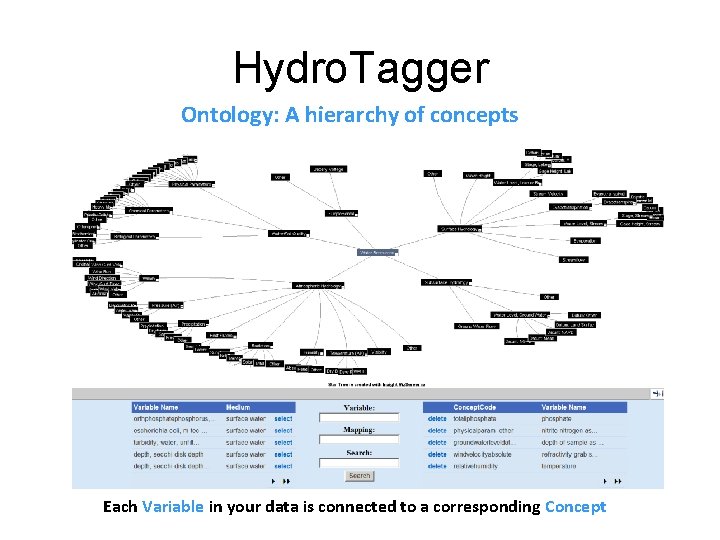
Hydro. Tagger Ontology: A hierarchy of concepts Each Variable in your data is connected to a corresponding Concept
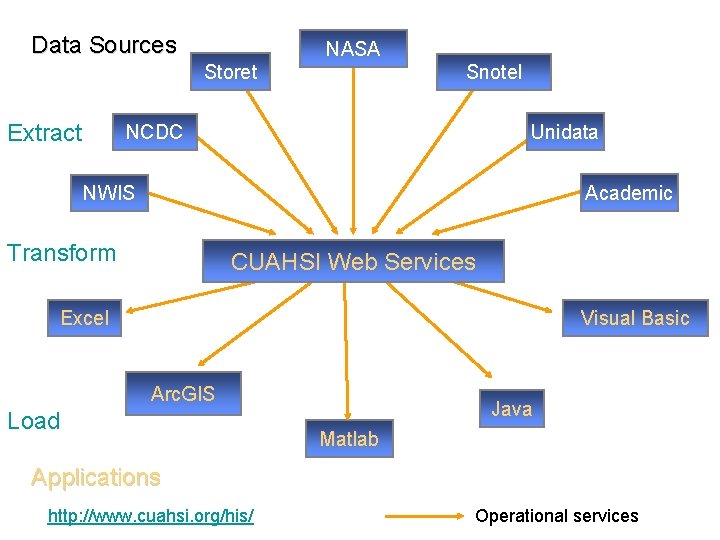
Data Sources Storet Extract NASA Snotel NCDC Unidata NWIS Academic Transform CUAHSI Web Services Excel Visual Basic Arc. GIS Load Java Matlab Applications http: //www. cuahsi. org/his/ Operational services
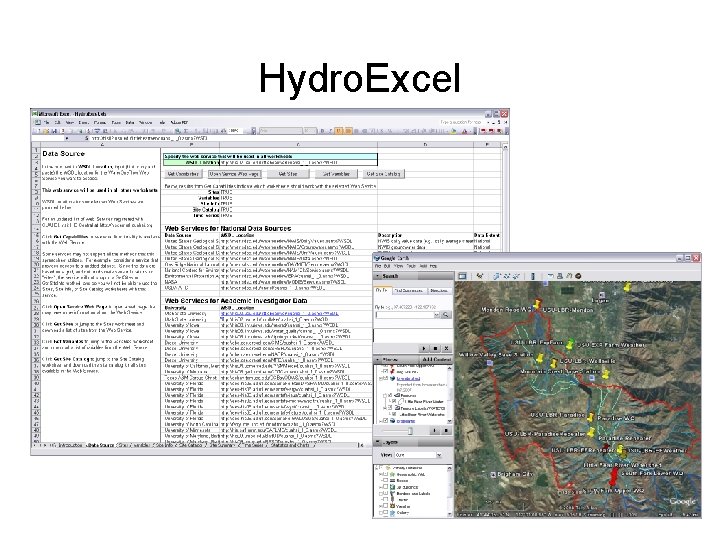
Hydro. Excel
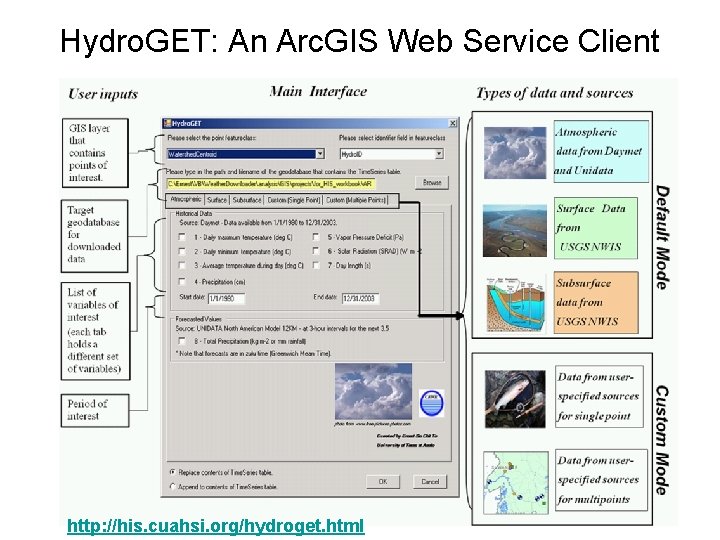
Hydro. GET: An Arc. GIS Web Service Client http: //his. cuahsi. org/hydroget. html
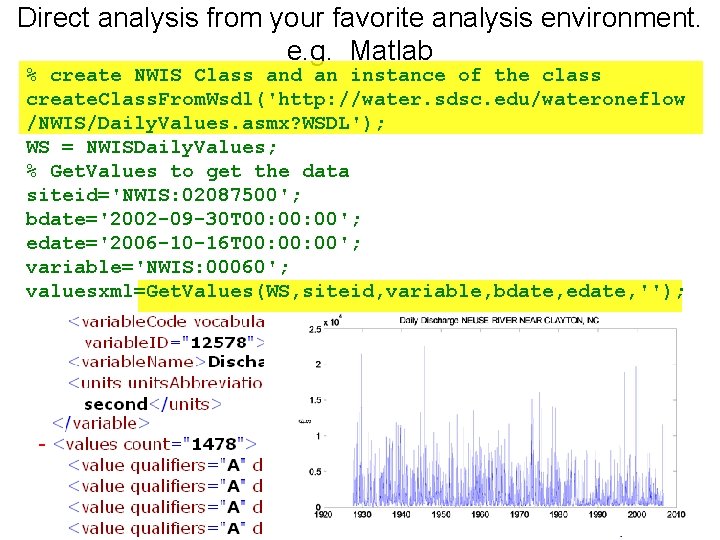
Direct analysis from your favorite analysis environment. e. g. Matlab % create NWIS Class and an instance of the class create. Class. From. Wsdl('http: //water. sdsc. edu/wateroneflow /NWIS/Daily. Values. asmx? WSDL'); WS = NWISDaily. Values; % Get. Values to get the data siteid='NWIS: 02087500'; bdate='2002 -09 -30 T 00: 00'; edate='2006 -10 -16 T 00: 00'; variable='NWIS: 00060'; valuesxml=Get. Values(WS, siteid, variable, bdate, edate, '');
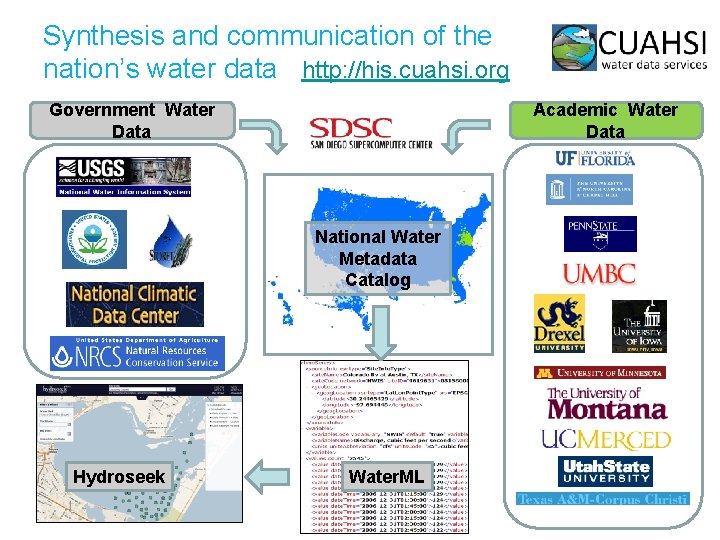
Synthesis and communication of the nation’s water data http: //his. cuahsi. org Government Water Data Academic Water Data National Water Metadata Catalog Hydroseek Water. ML
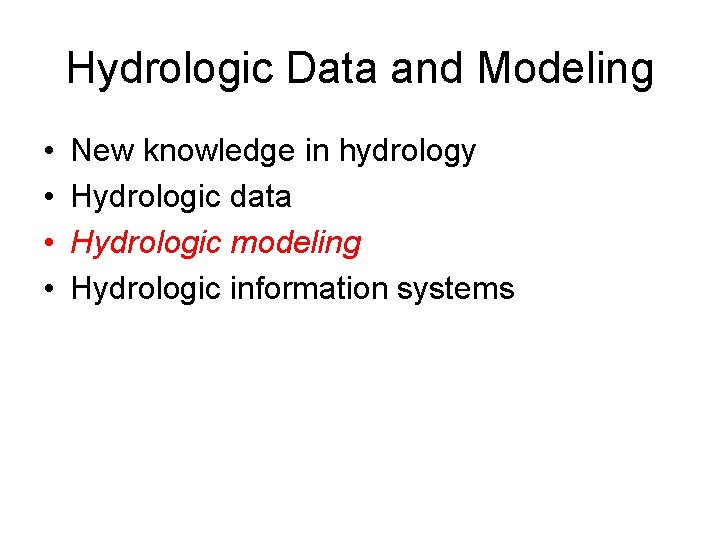
Hydrologic Data and Modeling • • New knowledge in hydrology Hydrologic data Hydrologic modeling Hydrologic information systems
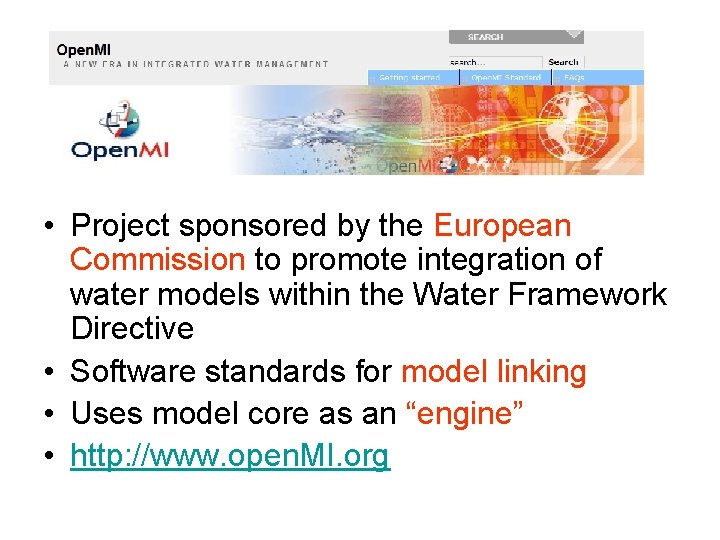
• Project sponsored by the European Commission to promote integration of water models within the Water Framework Directive • Software standards for model linking • Uses model core as an “engine” • http: //www. open. MI. org
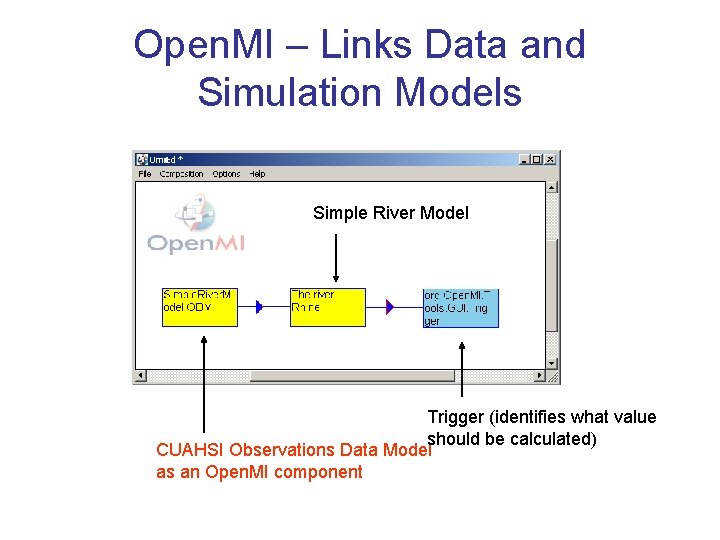
Open. MI – Links Data and Simulation Models Simple River Model Trigger (identifies what value should be calculated) CUAHSI Observations Data Model as an Open. MI component
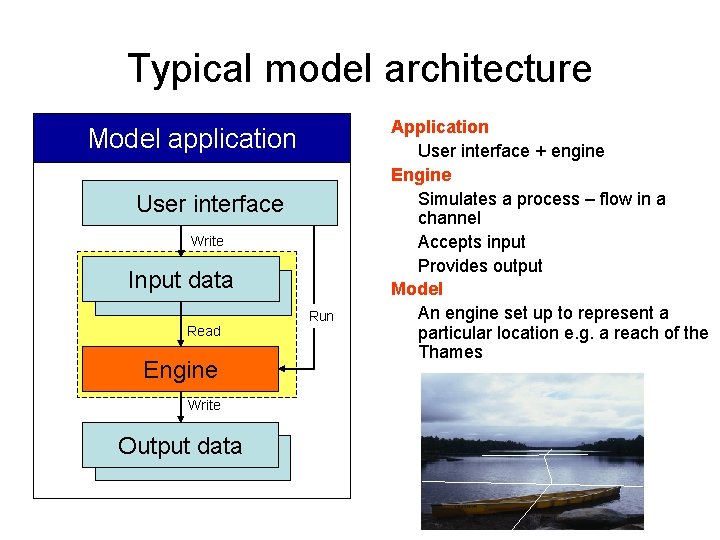
Typical model architecture Model application User interface Write Input data Read Engine Write Output data Run Application User interface + engine Engine Simulates a process – flow in a channel Accepts input Provides output Model An engine set up to represent a particular location e. g. a reach of the Thames
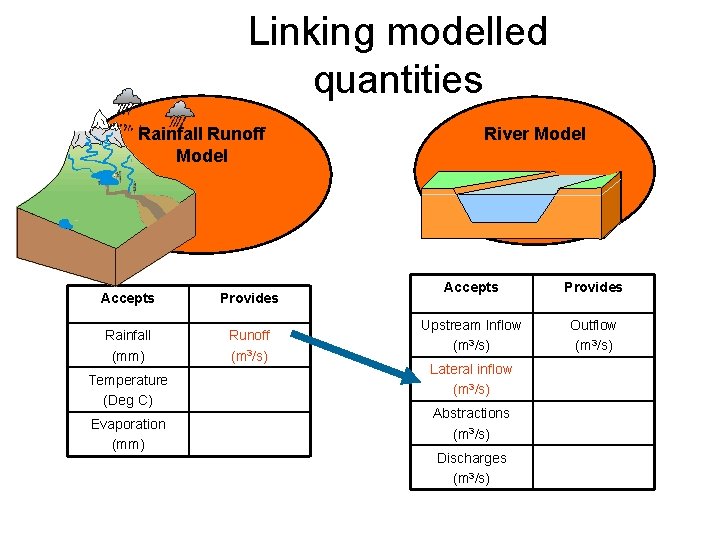
Linking modelled quantities Rainfall Runoff Model Accepts Provides Rainfall (mm) Runoff (m 3/s) Temperature (Deg C) Evaporation (mm) River Model Accepts Provides Upstream Inflow (m 3/s) Outflow (m 3/s) Lateral inflow (m 3/s) Abstractions (m 3/s) Discharges (m 3/s)
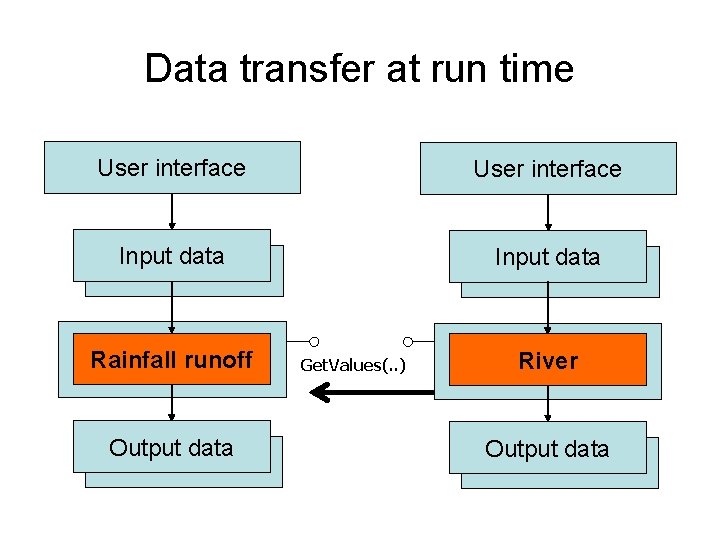
Data transfer at run time User interface Input data Rainfall runoff Output data Get. Values(. . ) River Output data
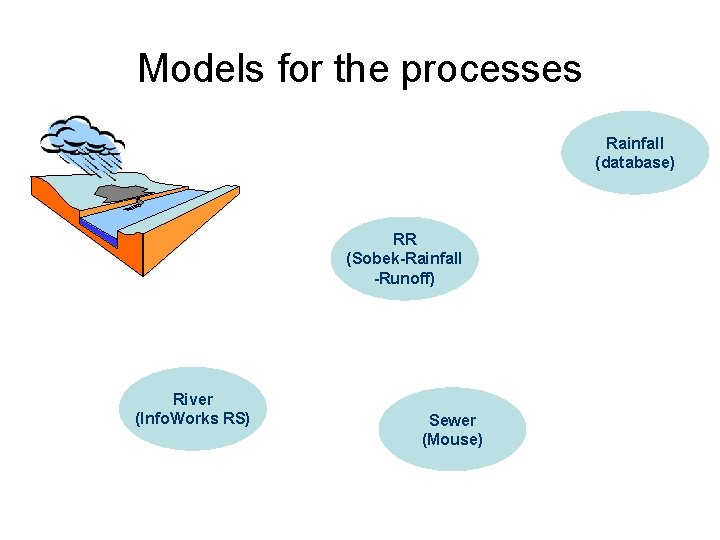
Models for the processes Rainfall (database) RR (Sobek-Rainfall -Runoff) River (Info. Works RS) Sewer (Mouse)
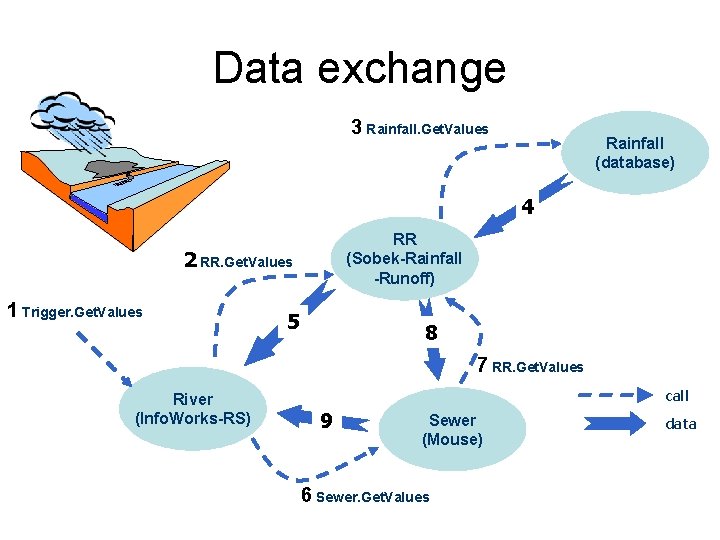
Data exchange 3 Rainfall. Get. Values Rainfall (database) 4 RR (Sobek-Rainfall -Runoff) 2 RR. Get. Values 1 Trigger. Get. Values 5 8 7 RR. Get. Values River (Info. Works-RS) call 9 Sewer (Mouse) 6 Sewer. Get. Values data
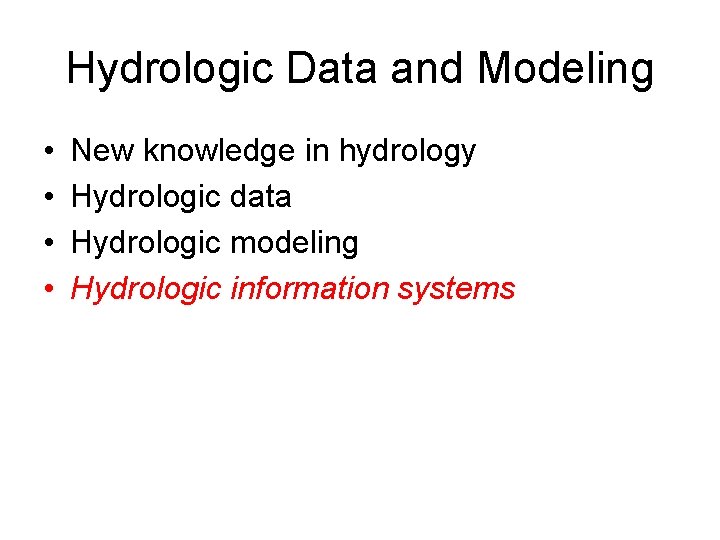
Hydrologic Data and Modeling • • New knowledge in hydrology Hydrologic data Hydrologic modeling Hydrologic information systems
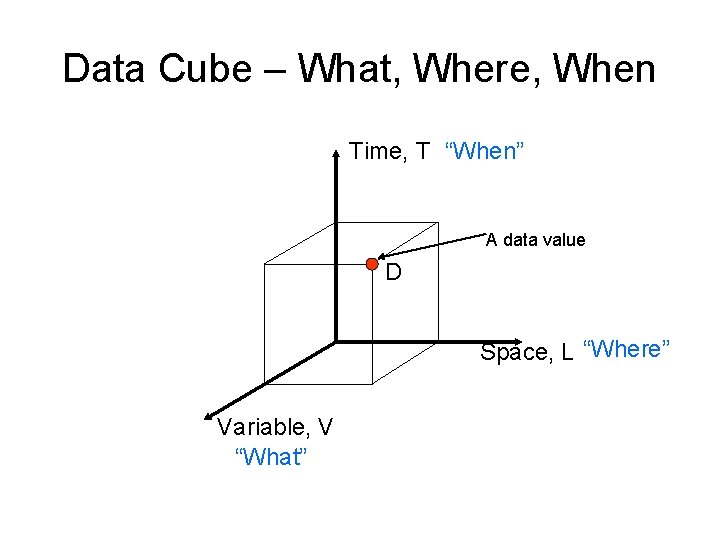
Data Cube – What, Where, When Time, T “When” A data value D Space, L “Where” Variable, V “What”
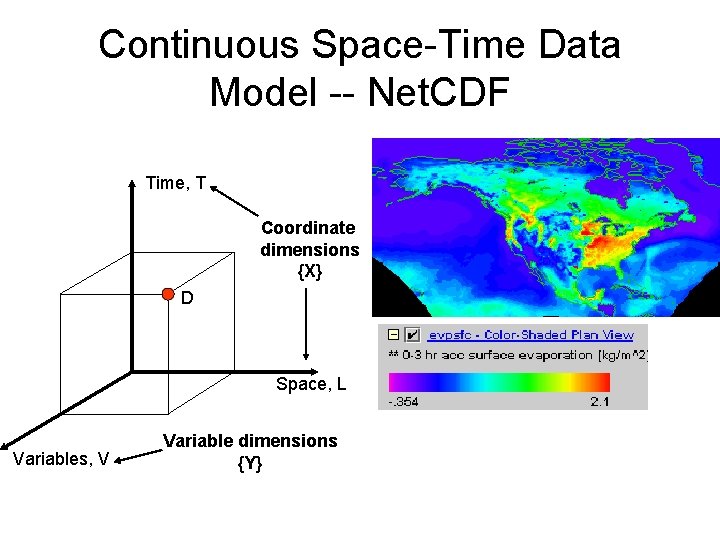
Continuous Space-Time Data Model -- Net. CDF Time, T Coordinate dimensions {X} D Space, L Variables, V Variable dimensions {Y}
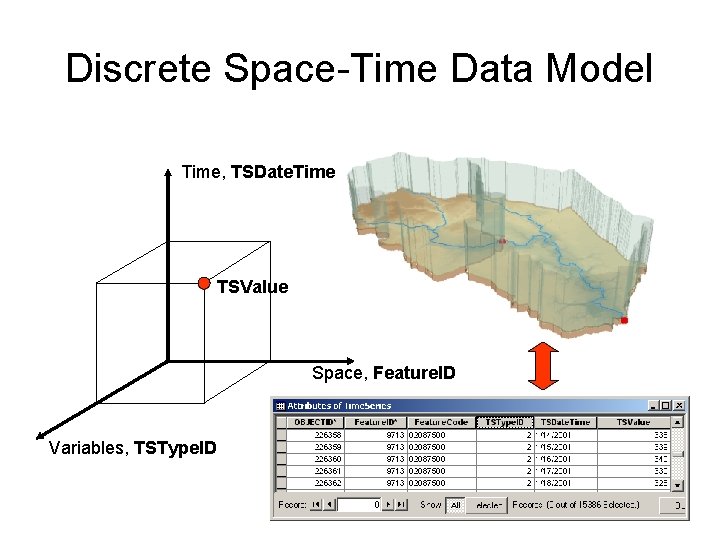
Discrete Space-Time Data Model Time, TSDate. Time TSValue Space, Feature. ID Variables, TSType. ID
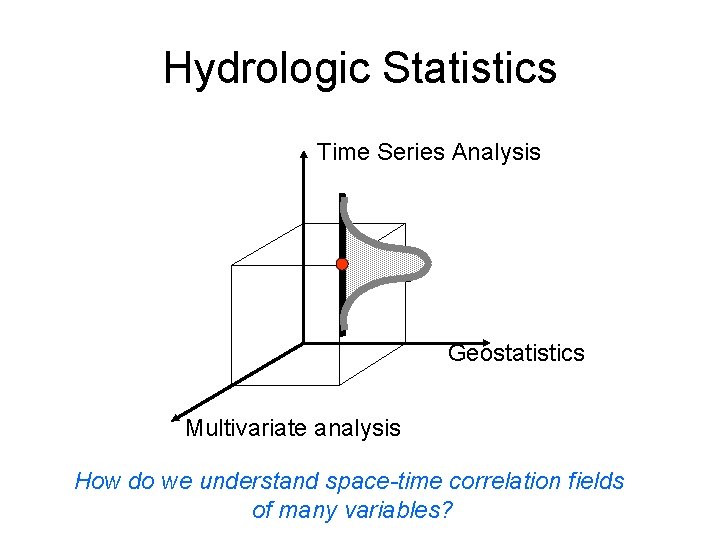
Hydrologic Statistics Time Series Analysis Geostatistics Multivariate analysis How do we understand space-time correlation fields of many variables?
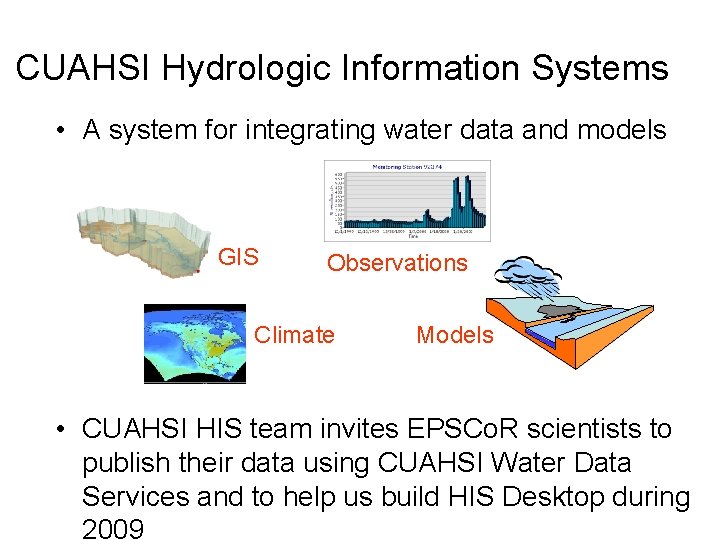
CUAHSI Hydrologic Information Systems • A system for integrating water data and models GIS Observations Climate Models • CUAHSI HIS team invites EPSCo. R scientists to publish their data using CUAHSI Water Data Services and to help us build HIS Desktop during 2009
Modeling and role modeling theory
Relational vs dimensional data modeling
Hydrologic continuity equation
Best practice etl architecture
Eric’s favourite .......... is science
What is data and process modeling
Describe data and process modeling concepts and tools
Hydrologic engineering center
Flood routing meaning
Hydrologic routing
Infiltration
Write the inflow as a formula
Hydrologic abstractions
Rickley hydrologic
Continuity equation hydrology
Hydrologic engineering center
Water cycle the hydrologic cycle
Applied hydrology
River analysis system
Hydrologic engineering center
Business modeling olympiad
Science modeling documents
Management science the art of modeling with spreadsheets
Msoe science olympiad
Jeff renz
National building information modeling standard
Bim ppt
Compare torvald and nora's attitude towards money
Loyalty and devotion to one's country
Modeling relational data with graph convolutional networks
Idefix notation
Data modeling using entity relationship model
Data warehouse modeling tutorial
Modeling data in the organization
Modeling data in the organization
Qlik sense data model best practices
Vhdl data flow modeling
Oltp data model
Sinusoidal examples in real life
Modeling distributions of data
Eclipse data modeling
Data flow modeling in verilog
Modeling data in the organization
Chapter 2 modeling distributions of data
Advanced data modeling
Company er diagram
When is the concept of a weak entity used in data modeling
Chapter 2 modeling distributions of data
Modeling data in the organization
Example of data processing cycle
Erwin data modeler
Tbone codd
Modeling distributions of data
Modeling data in the organization
Cardinality and modality in database
Linear quadratic function
Rapid change
Extra credit
Hard science and soft science
Halliday 1993
Author attitude
Together towards improvement
Sand: towards high-performance serverless computing