HARRV Models Including Sector and Market Regressors Sharon
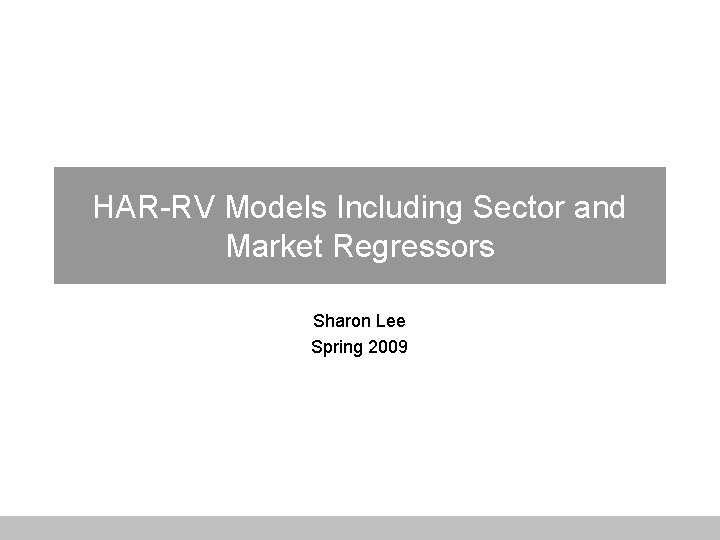
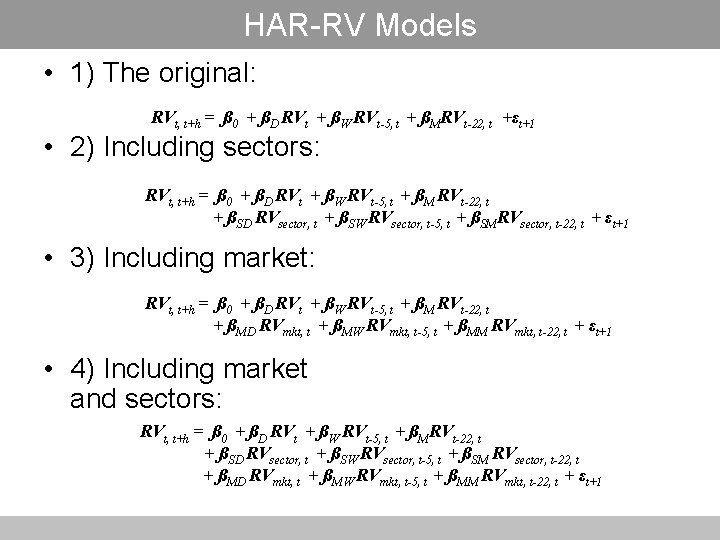
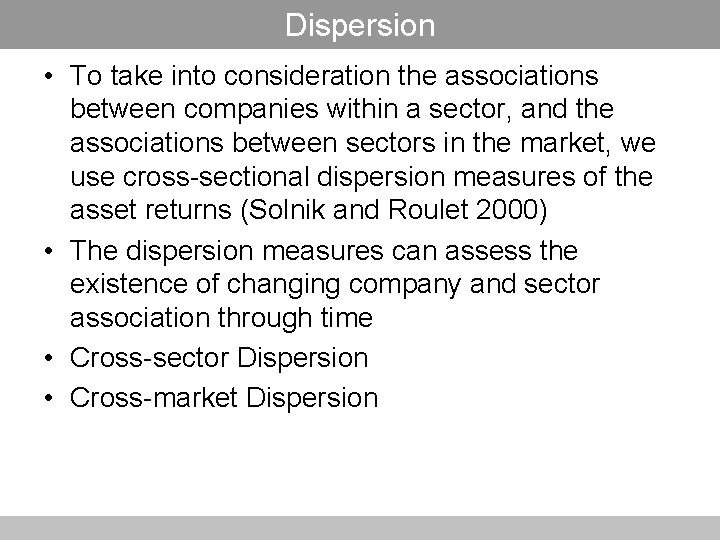
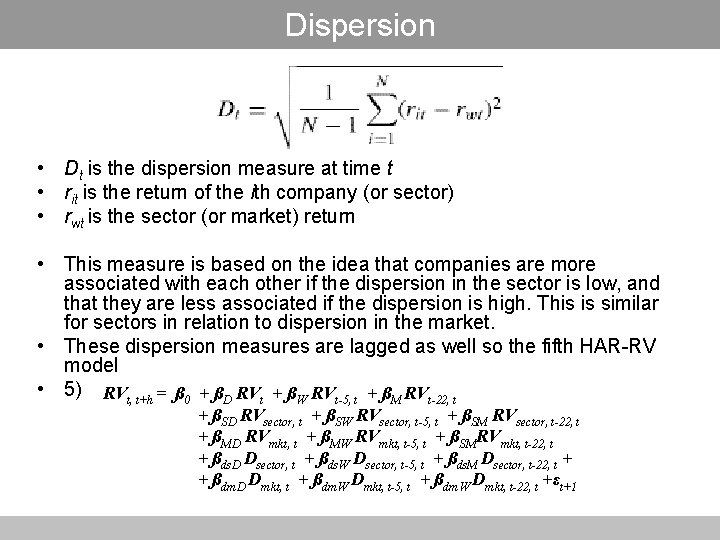
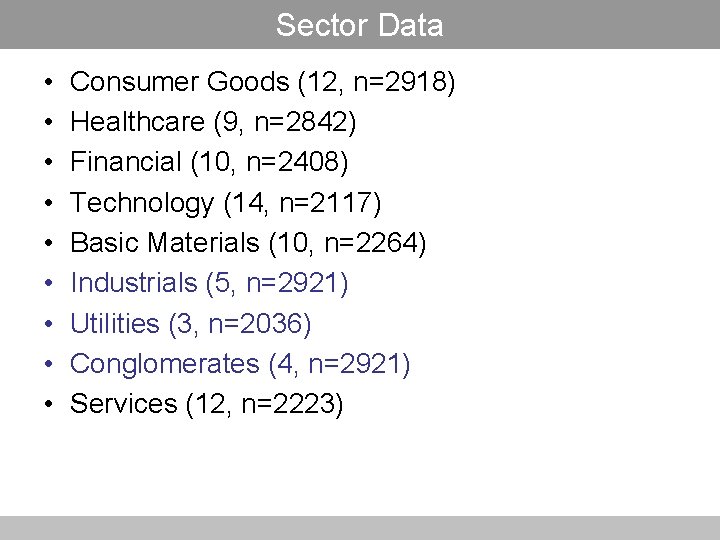
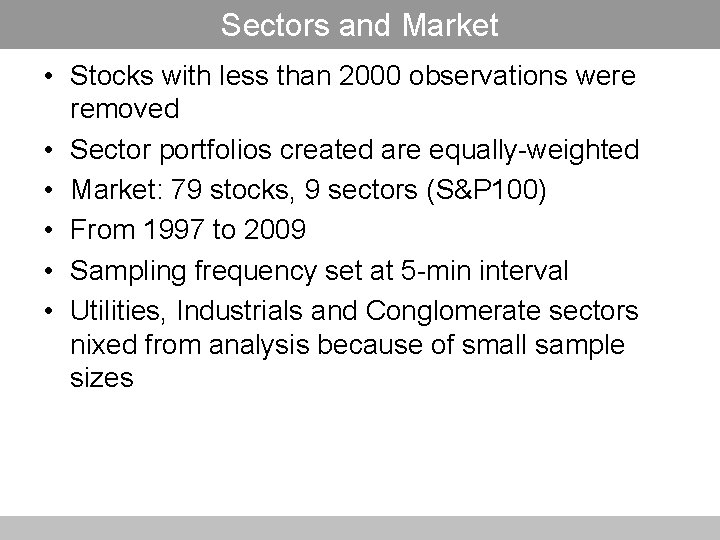
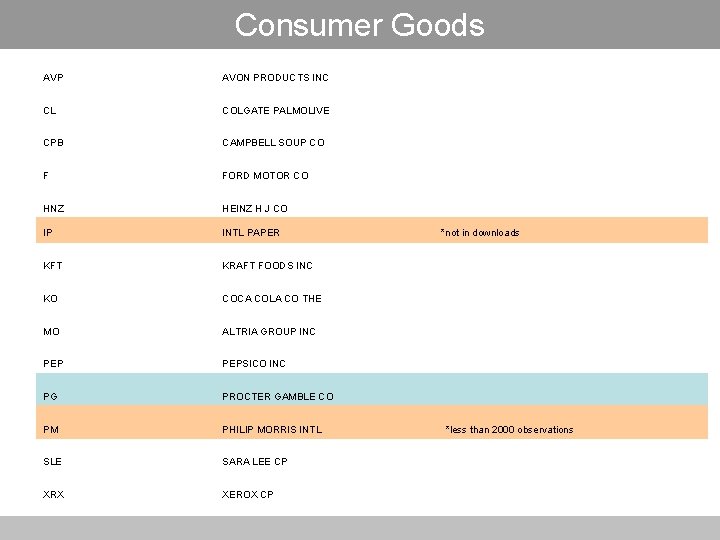
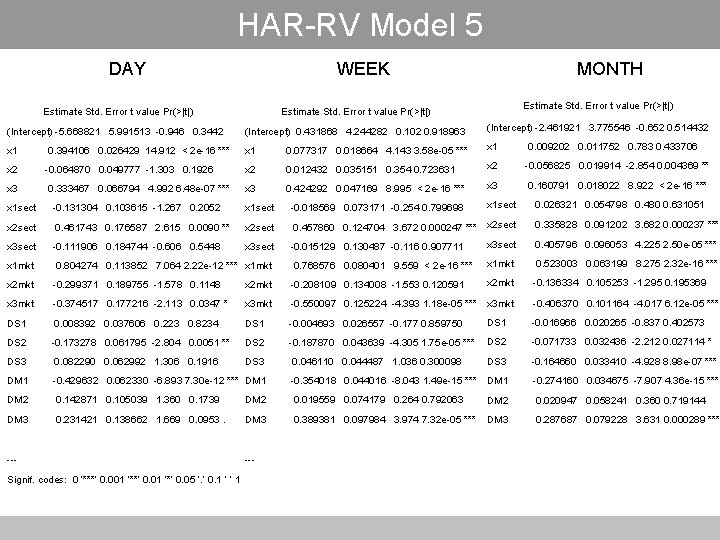
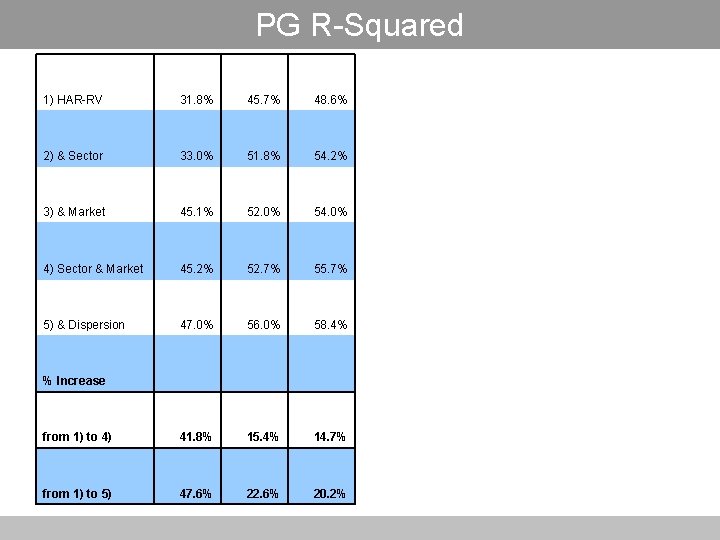
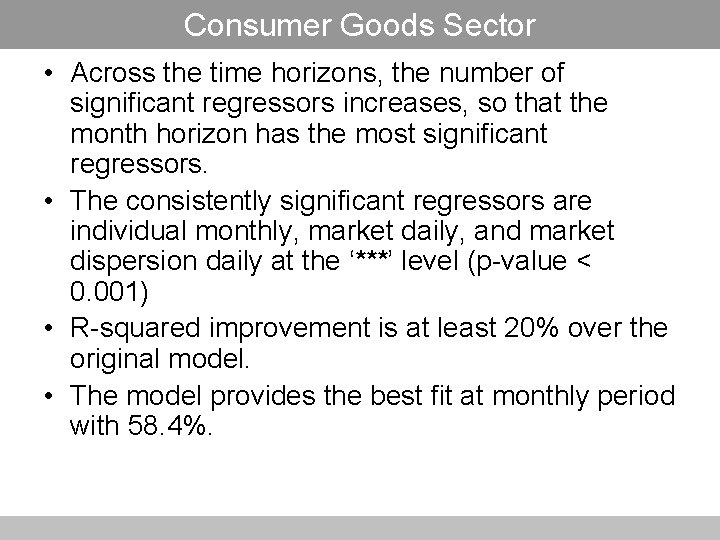
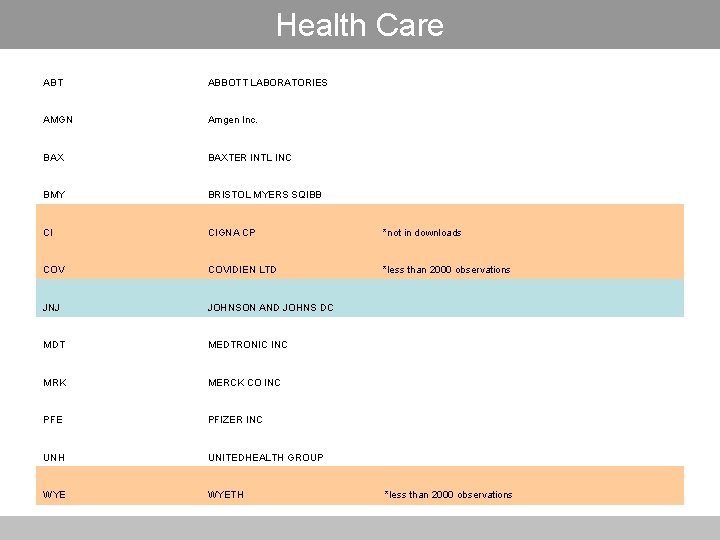
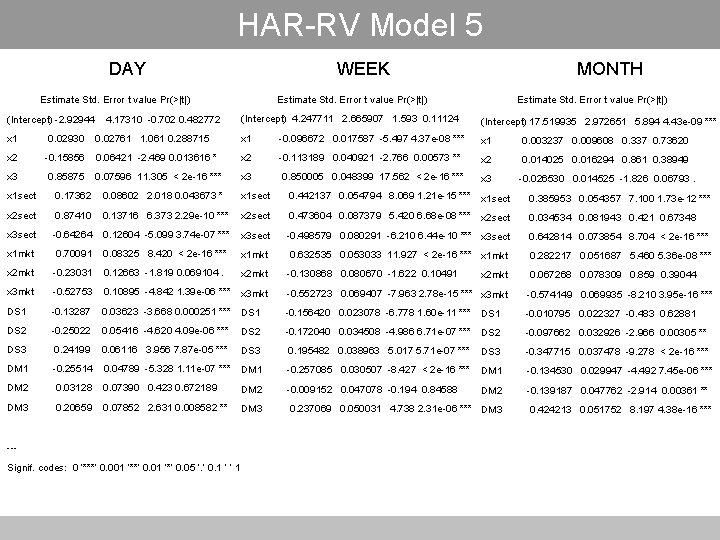
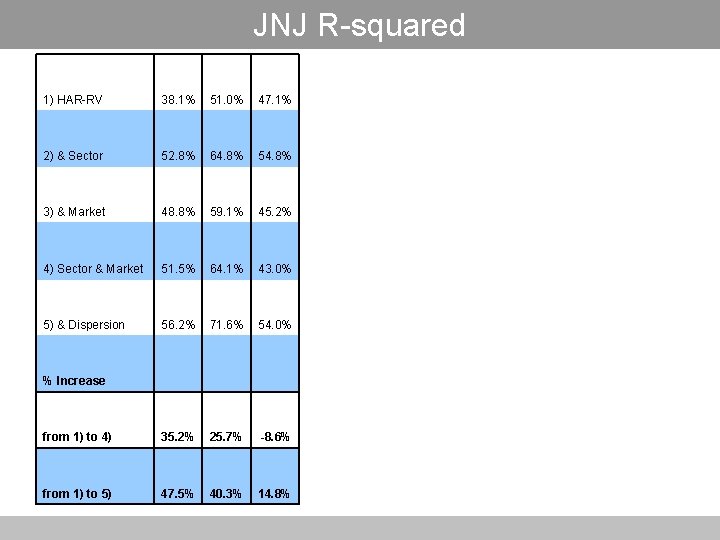
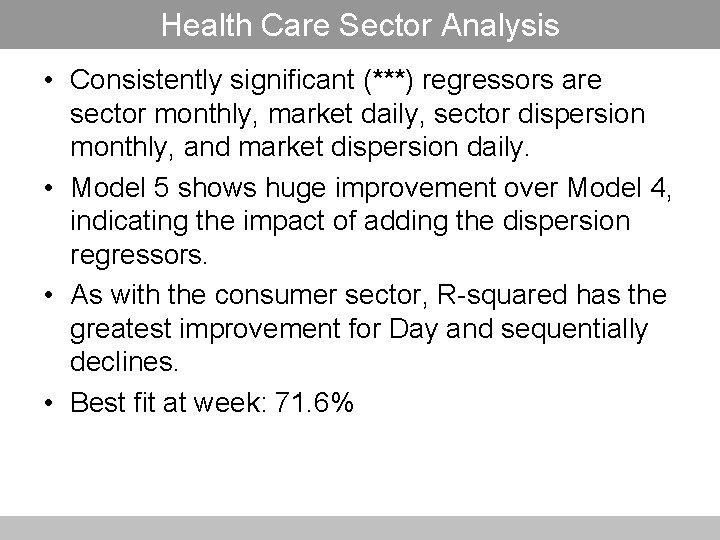
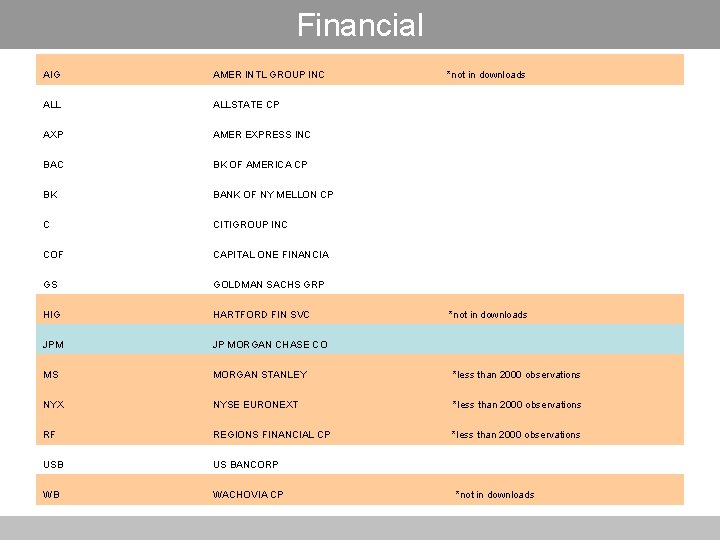
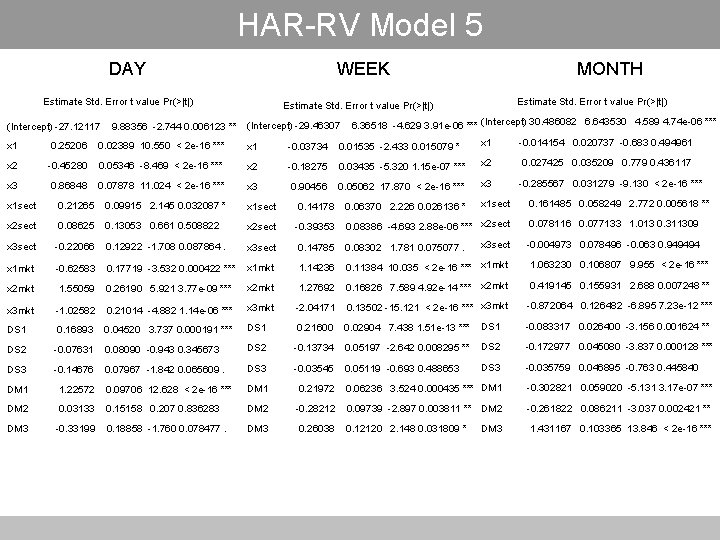
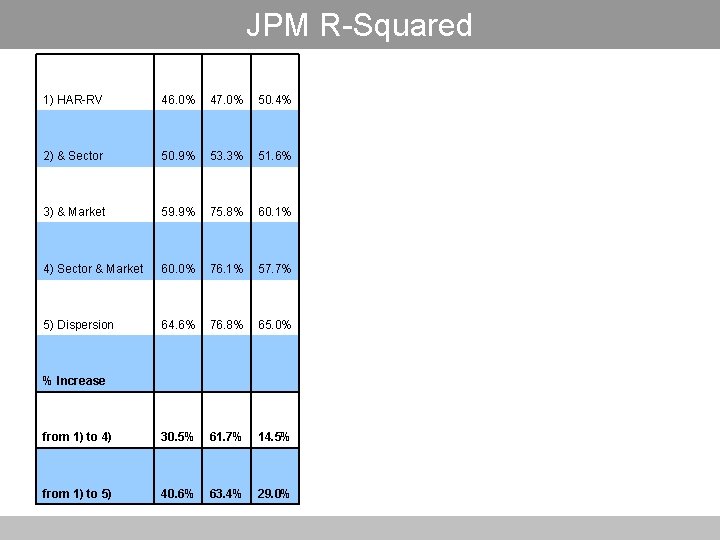
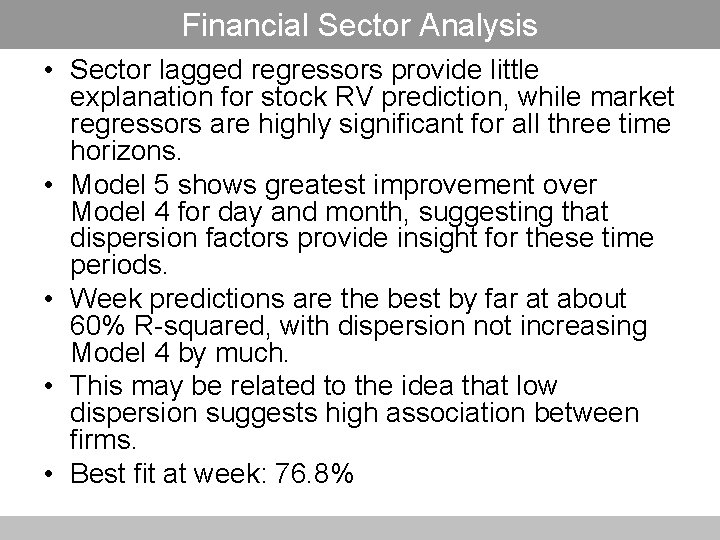
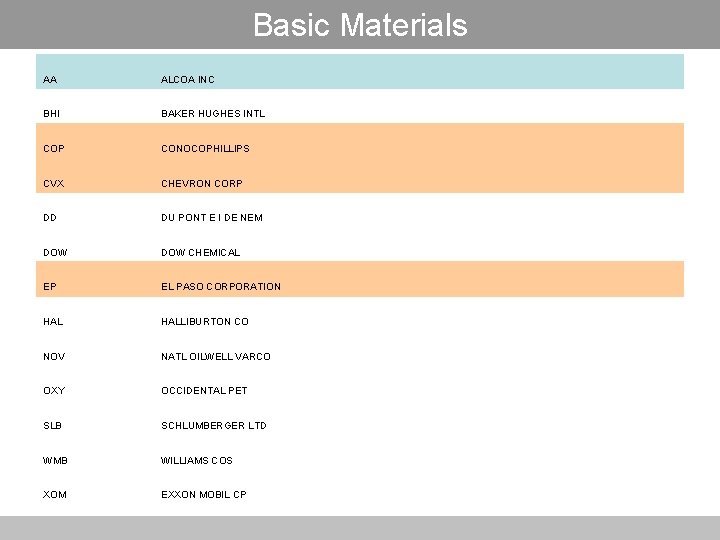
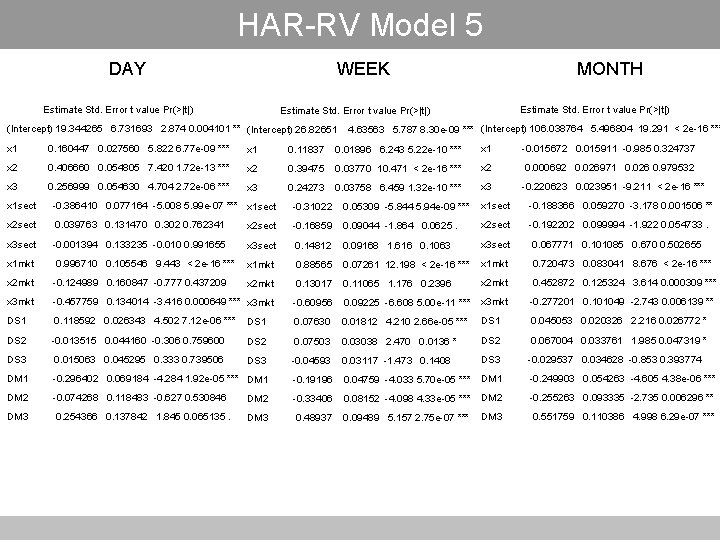
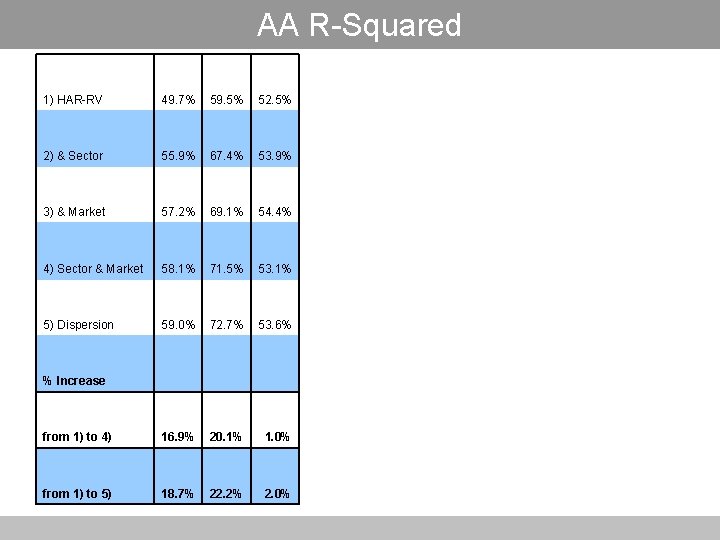
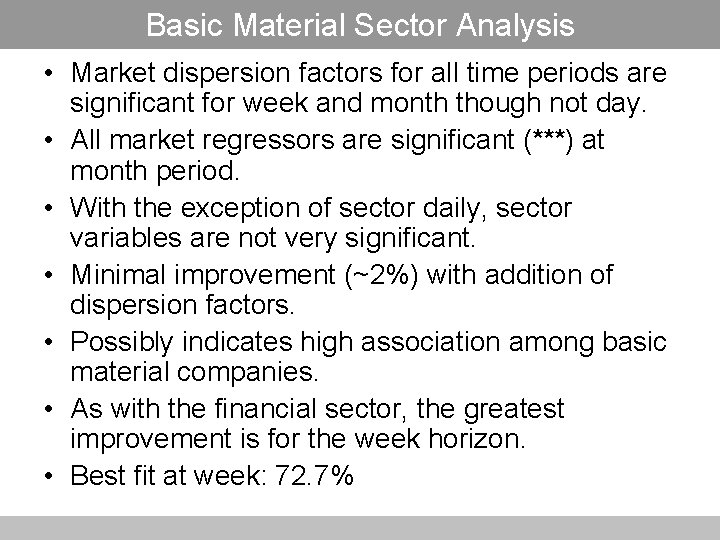
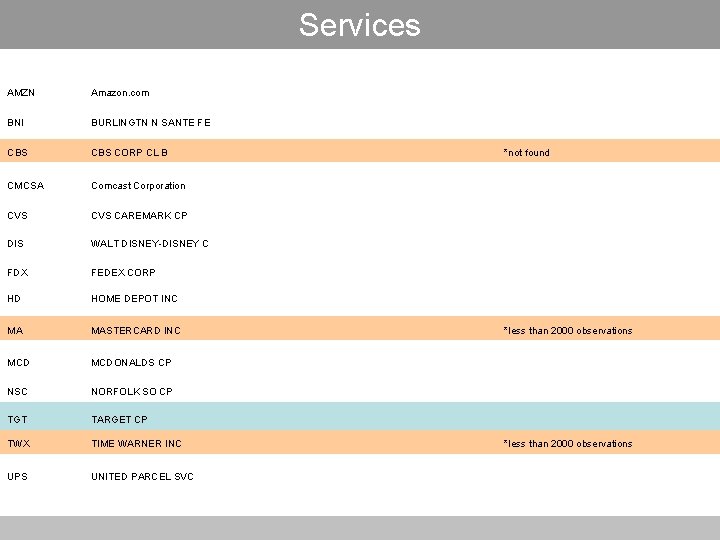
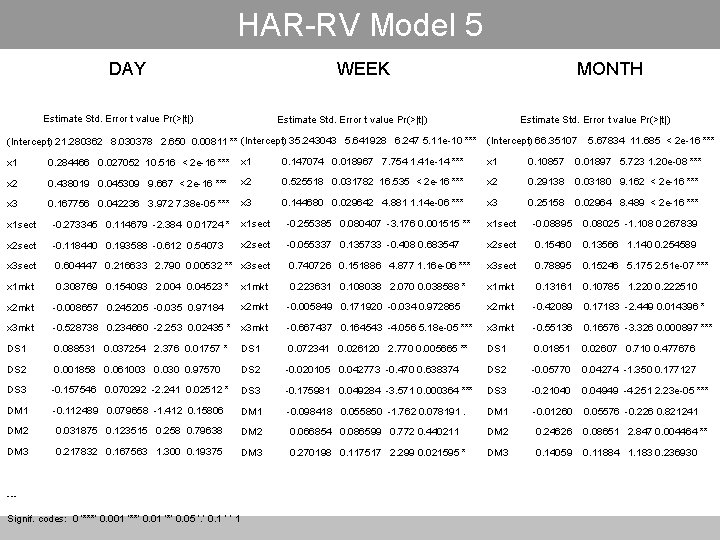
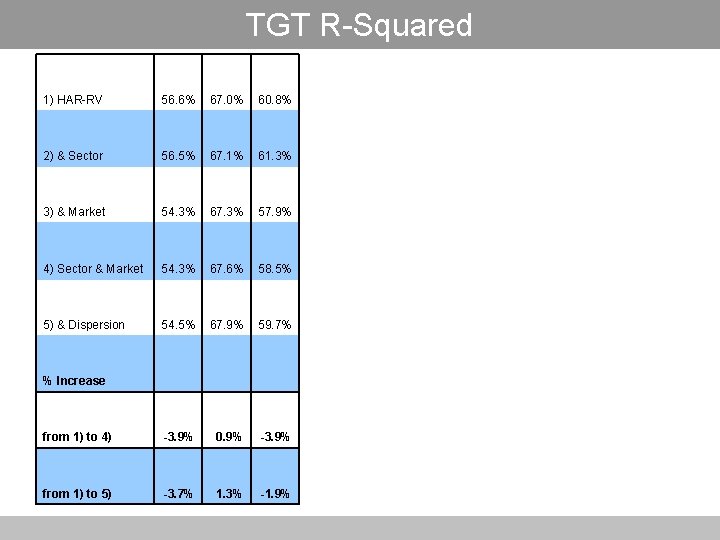
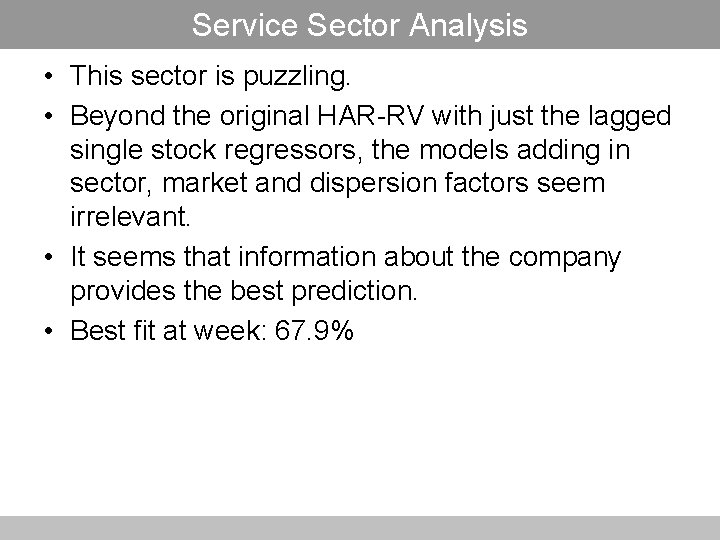
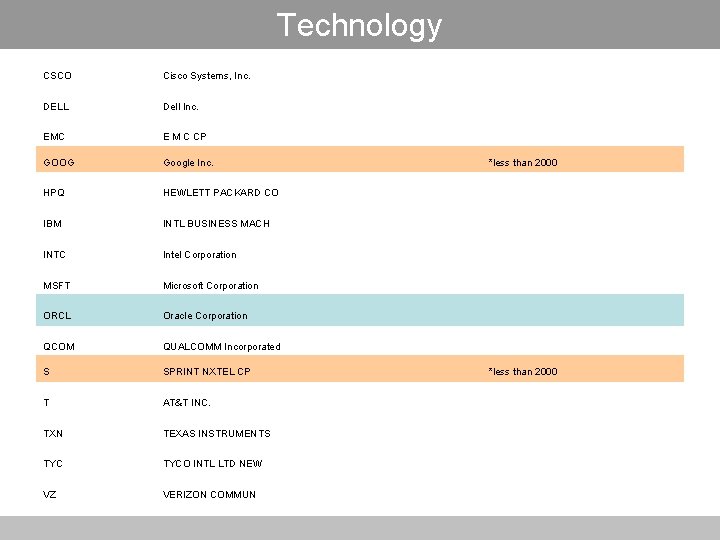
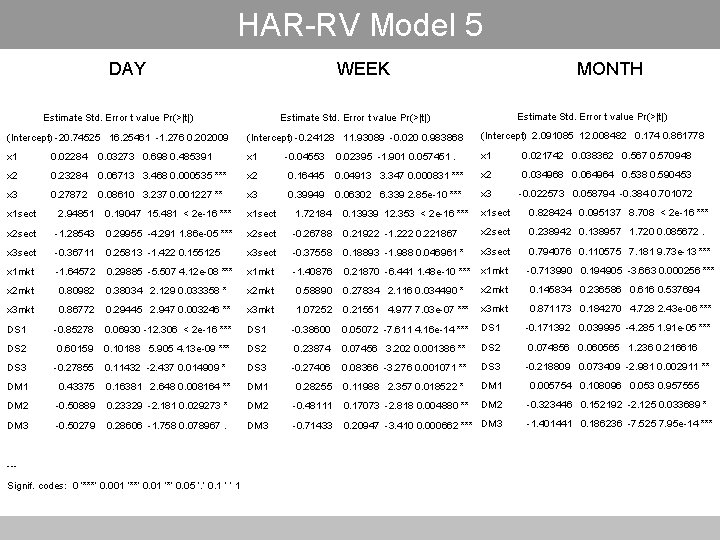
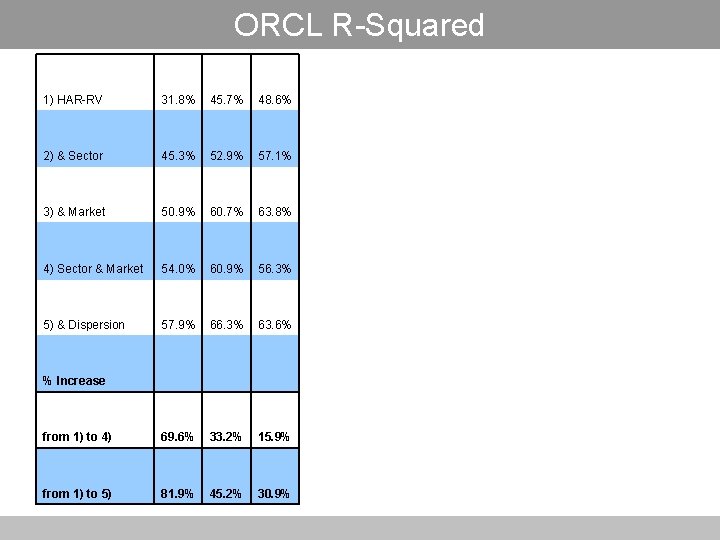
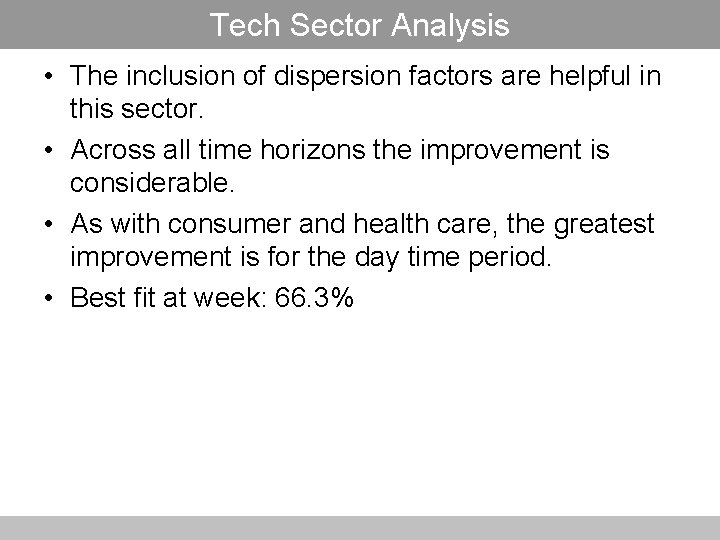
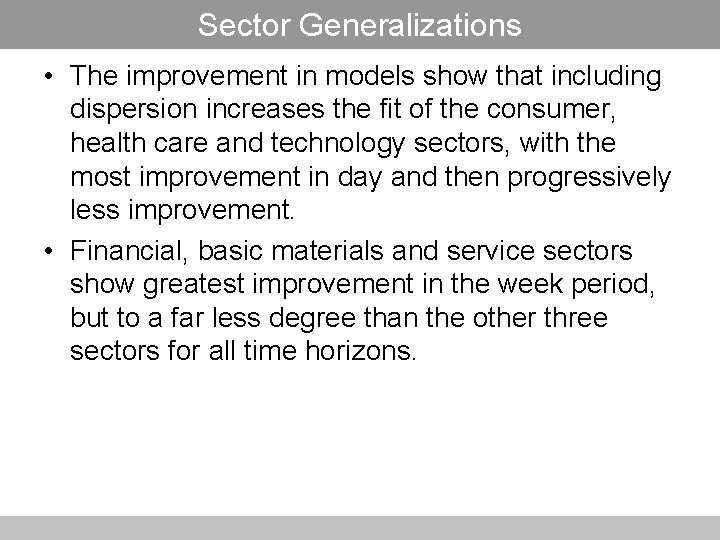
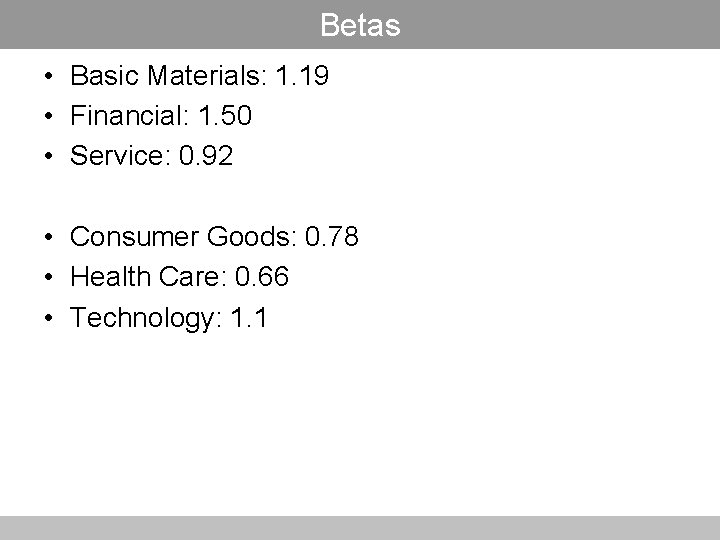
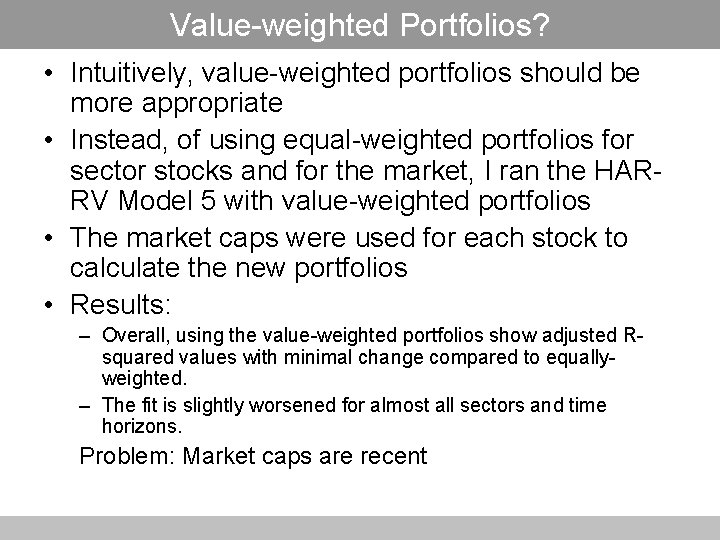
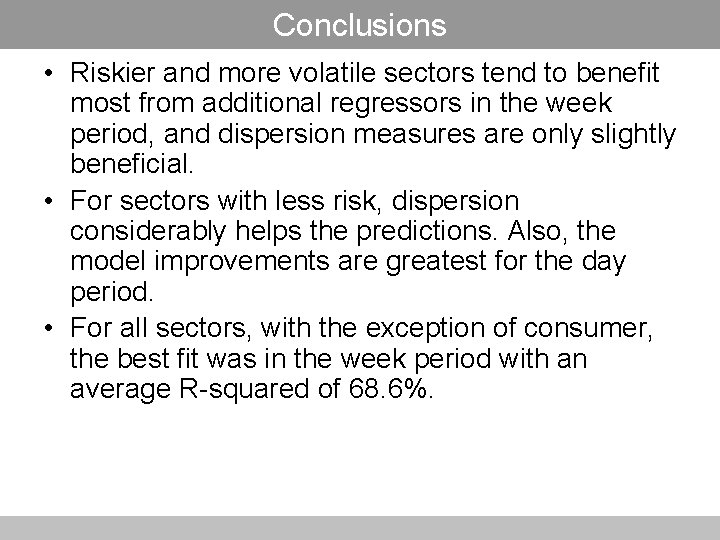
- Slides: 34
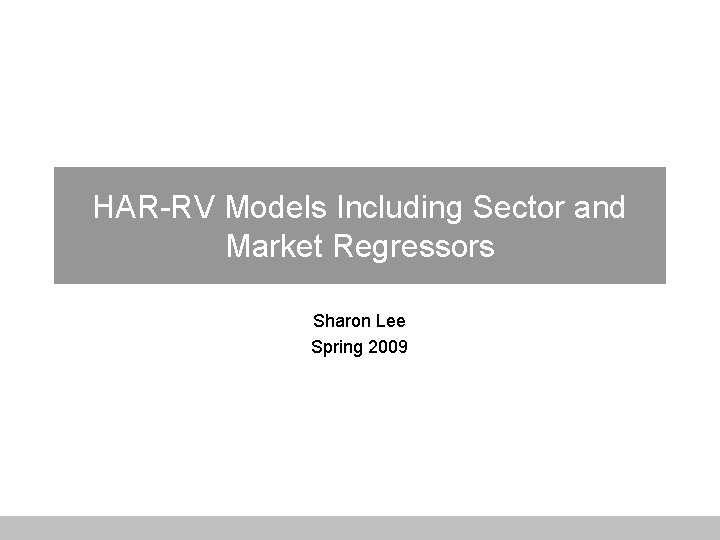
HAR-RV Models Including Sector and Market Regressors Sharon Lee Spring 2009
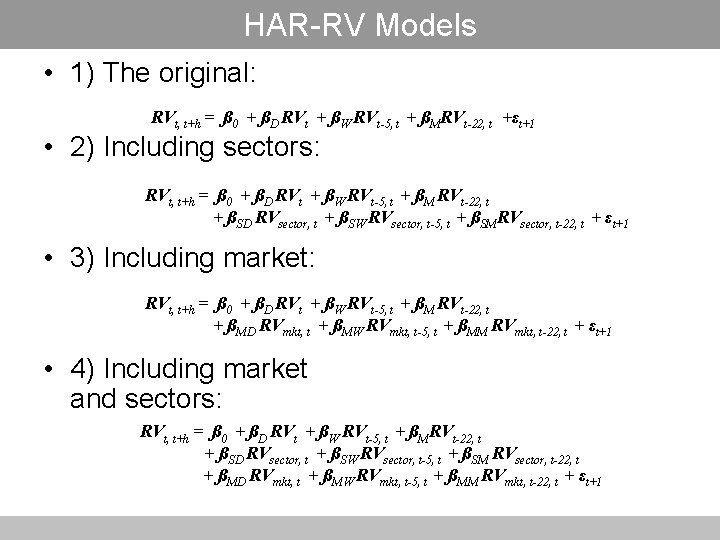
HAR-RV Models • 1) The original: RVt, t+h = ß 0 + ßD RVt + ßW RVt-5, t + ßMRVt-22, t +εt+1 • 2) Including sectors: RVt, t+h = ß 0 + ßD RVt + ßW RVt-5, t + ßM RVt-22, t + ßSD RVsector, t + ßSW RVsector, t-5, t + ßSMRVsector, t-22, t + εt+1 • 3) Including market: RVt, t+h = ß 0 + ßD RVt + ßW RVt-5, t + ßM RVt-22, t + ßMD RVmkt, t + ßMW RVmkt, t-5, t + ßMM RVmkt, t-22, t + εt+1 • 4) Including market and sectors: RVt, t+h = ß 0 + ßD RVt + ßW RVt-5, t + ßMRVt-22, t + ßSD RVsector, t + ßSW RVsector, t-5, t + ßSM RVsector, t-22, t + ßMD RVmkt, t + ßMW RVmkt, t-5, t + ßMM RVmkt, t-22, t + εt+1
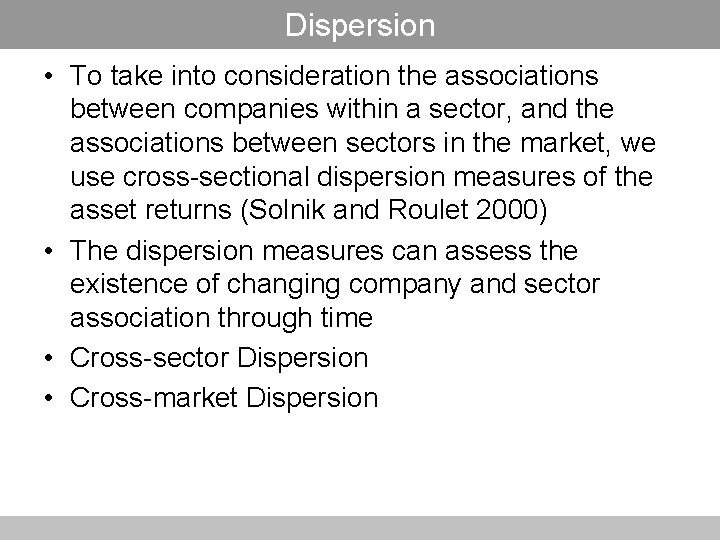
Dispersion • To take into consideration the associations between companies within a sector, and the associations between sectors in the market, we use cross-sectional dispersion measures of the asset returns (Solnik and Roulet 2000) • The dispersion measures can assess the existence of changing company and sector association through time • Cross-sector Dispersion • Cross-market Dispersion
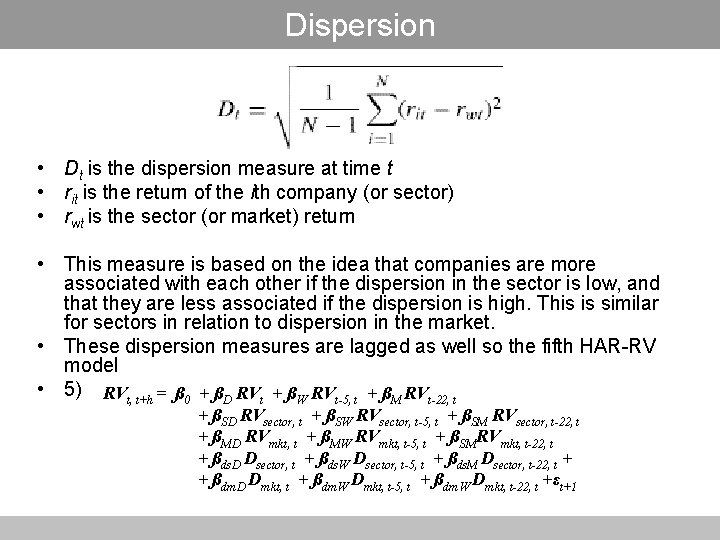
Dispersion • Dt is the dispersion measure at time t • rit is the return of the ith company (or sector) • rwt is the sector (or market) return • This measure is based on the idea that companies are more associated with each other if the dispersion in the sector is low, and that they are less associated if the dispersion is high. This is similar for sectors in relation to dispersion in the market. • These dispersion measures are lagged as well so the fifth HAR-RV model • 5) RVt, t+h = ß 0 + ßD RVt + ßW RVt-5, t + ßM RVt-22, t + ßSD RVsector, t + ßSW RVsector, t-5, t + ßSM RVsector, t-22, t + ßMD RVmkt, t + ßMW RVmkt, t-5, t + ßSMRVmkt, t-22, t + ßds. D Dsector, t + ßds. W Dsector, t-5, t + ßds. M Dsector, t-22, t + + ßdm. D Dmkt, t + ßdm. W Dmkt, t-5, t + ßdm. W Dmkt, t-22, t +εt+1
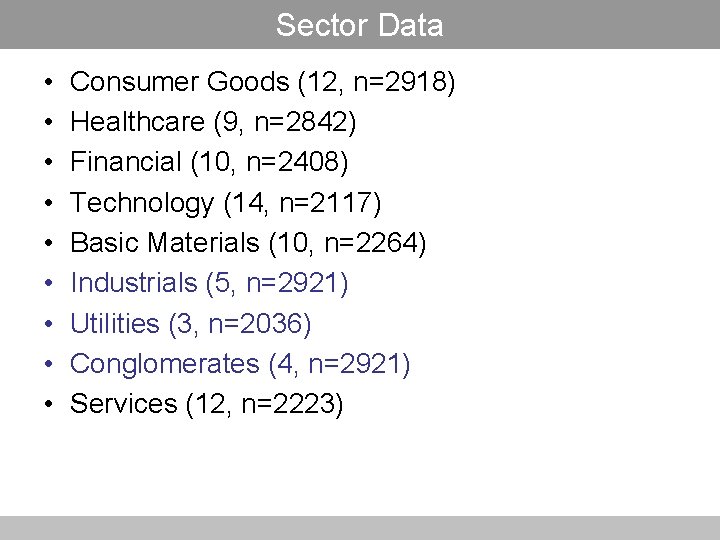
Sector Data • • • Consumer Goods (12, n=2918) Healthcare (9, n=2842) Financial (10, n=2408) Technology (14, n=2117) Basic Materials (10, n=2264) Industrials (5, n=2921) Utilities (3, n=2036) Conglomerates (4, n=2921) Services (12, n=2223)
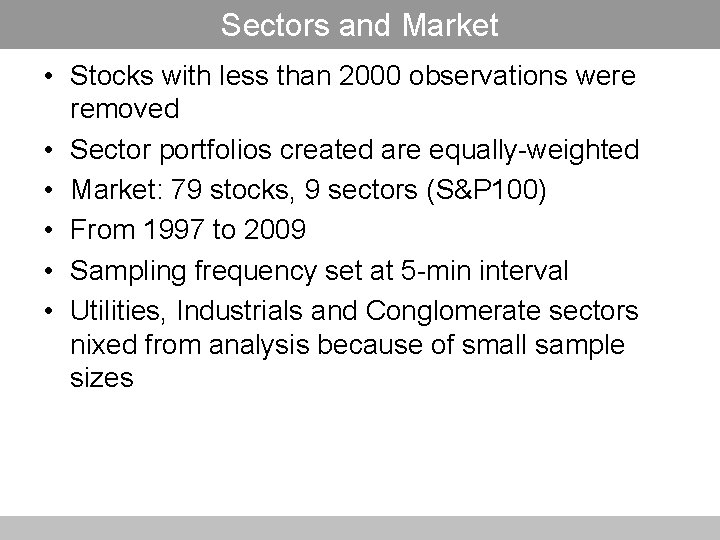
Sectors and Market • Stocks with less than 2000 observations were removed • Sector portfolios created are equally-weighted • Market: 79 stocks, 9 sectors (S&P 100) • From 1997 to 2009 • Sampling frequency set at 5 -min interval • Utilities, Industrials and Conglomerate sectors nixed from analysis because of small sample sizes
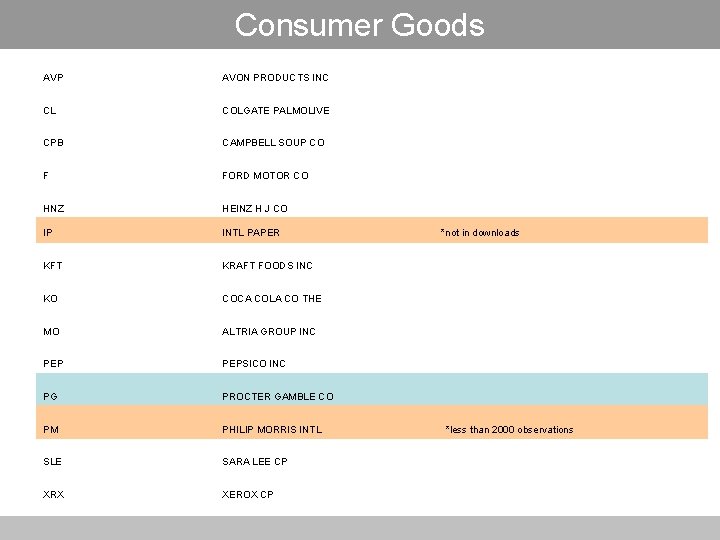
Consumer Goods AVP AVON PRODUCTS INC CL COLGATE PALMOLIVE CPB CAMPBELL SOUP CO F FORD MOTOR CO HNZ HEINZ H J CO IP INTL PAPER KFT KRAFT FOODS INC KO COCA COLA CO THE MO ALTRIA GROUP INC PEPSICO INC PG PROCTER GAMBLE CO PM PHILIP MORRIS INTL SLE SARA LEE CP XRX XEROX CP *not in downloads *less than 2000 observations
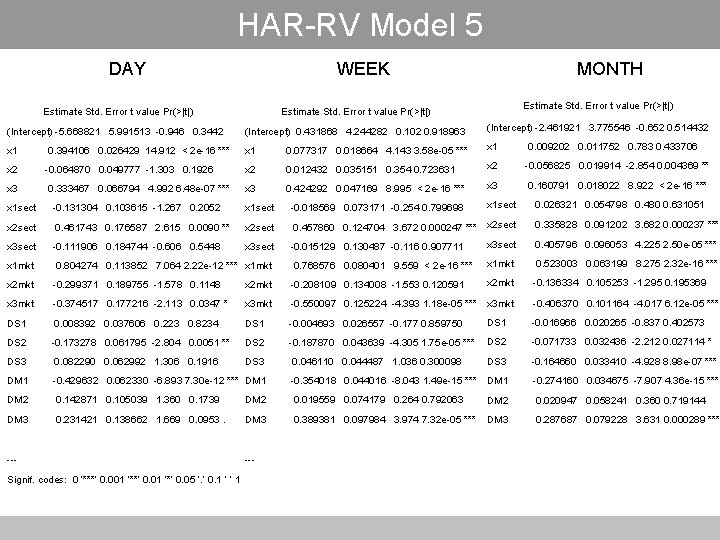
HAR-RV Model 5 DAY WEEK MONTH Estimate Std. Error t value Pr(>|t|) (Intercept) -5. 668821 5. 991513 -0. 946 0. 3442 (Intercept) 0. 431868 4. 244282 0. 102 0. 918963 (Intercept) -2. 461921 3. 775546 -0. 652 0. 514432 x 1 0. 394106 0. 026429 14. 912 < 2 e-16 *** x 1 0. 077317 0. 018664 4. 143 3. 58 e-05 *** x 1 0. 009202 0. 011752 0. 783 0. 433706 x 2 -0. 064870 0. 049777 -1. 303 0. 1926 x 2 0. 012432 0. 035151 0. 354 0. 723631 x 2 -0. 056825 0. 019914 -2. 854 0. 004369 ** x 3 0. 333467 0. 066794 4. 992 6. 48 e-07 *** x 3 0. 424292 0. 047169 8. 995 < 2 e-16 *** x 3 0. 160791 0. 018022 8. 922 < 2 e-16 *** x 1 sect 0. 026321 0. 054798 0. 480 0. 631051 x 1 sect -0. 131304 0. 103615 -1. 267 0. 2052 x 1 sect -0. 018569 0. 073171 -0. 254 0. 799698 x 2 sect 0. 461743 0. 176587 2. 615 0. 0090 ** x 2 sect 0. 457860 0. 124704 3. 672 0. 000247 *** x 2 sect 0. 335828 0. 091202 3. 682 0. 000237 *** x 3 sect -0. 111906 0. 184744 -0. 606 0. 5448 x 3 sect -0. 015129 0. 130487 -0. 116 0. 907711 x 3 sect 0. 405796 0. 096053 4. 225 2. 50 e-05 *** 0. 768576 0. 080401 9. 559 < 2 e-16 *** x 1 mkt 0. 523003 0. 063199 8. 275 2. 32 e-16 *** x 1 mkt 0. 804274 0. 113852 7. 064 2. 22 e-12 *** x 1 mkt x 2 mkt -0. 299371 0. 189755 -1. 578 0. 1148 x 2 mkt -0. 208109 0. 134008 -1. 553 0. 120591 x 2 mkt -0. 136334 0. 105253 -1. 295 0. 195369 x 3 mkt -0. 374517 0. 177216 -2. 113 0. 0347 * x 3 mkt -0. 550097 0. 125224 -4. 393 1. 18 e-05 *** x 3 mkt -0. 406370 0. 101164 -4. 017 6. 12 e-05 *** DS 1 0. 008392 0. 037606 0. 223 0. 8234 DS 1 -0. 004693 0. 026557 -0. 177 0. 859750 DS 1 -0. 016966 0. 020265 -0. 837 0. 402573 DS 2 -0. 173278 0. 061795 -2. 804 0. 0051 ** DS 2 -0. 187870 0. 043639 -4. 305 1. 75 e-05 *** DS 2 -0. 071733 0. 032436 -2. 212 0. 027114 * DS 3 0. 082290 0. 062992 1. 306 0. 1916 DS 3 0. 046110 0. 044487 1. 036 0. 300098 DS 3 -0. 164660 0. 033410 -4. 928 8. 98 e-07 *** DM 1 -0. 429632 0. 062330 -6. 893 7. 30 e-12 *** DM 1 -0. 354018 0. 044016 -8. 043 1. 49 e-15 *** DM 1 -0. 274160 0. 034675 -7. 907 4. 36 e-15 *** DM 2 0. 142871 0. 105039 1. 360 0. 1739 DM 2 0. 019559 0. 074179 0. 264 0. 792063 DM 2 0. 020947 0. 058241 0. 360 0. 719144 DM 3 0. 231421 0. 138662 1. 669 0. 0953. DM 3 0. 389381 0. 097984 3. 974 7. 32 e-05 *** DM 3 0. 287687 0. 079228 3. 631 0. 000289 *** --Signif. codes: 0 ‘***’ 0. 001 ‘**’ 0. 01 ‘*’ 0. 05 ‘. ’ 0. 1 ‘ ’ 1 ---
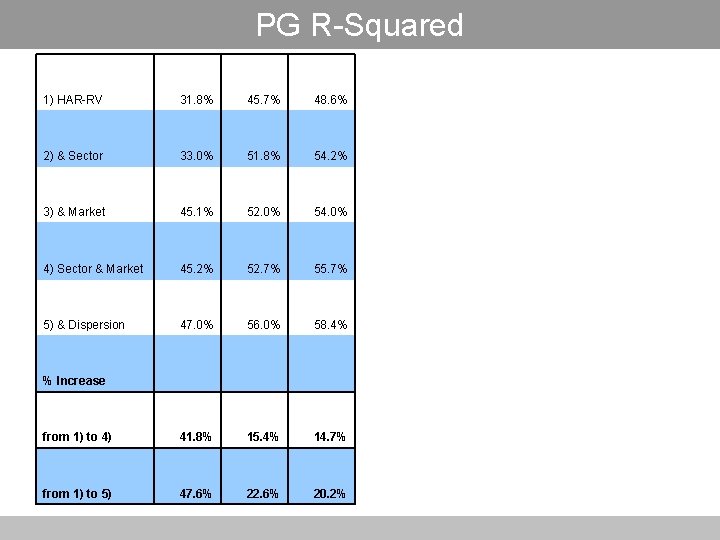
PG R-Squared 1) HAR-RV 31. 8% 45. 7% 48. 6% 2) & Sector 33. 0% 51. 8% 54. 2% 3) & Market 45. 1% 52. 0% 54. 0% 4) Sector & Market 45. 2% 52. 7% 55. 7% 5) & Dispersion 47. 0% 56. 0% 58. 4% from 1) to 4) 41. 8% 15. 4% 14. 7% from 1) to 5) 47. 6% 22. 6% 20. 2% % Increase
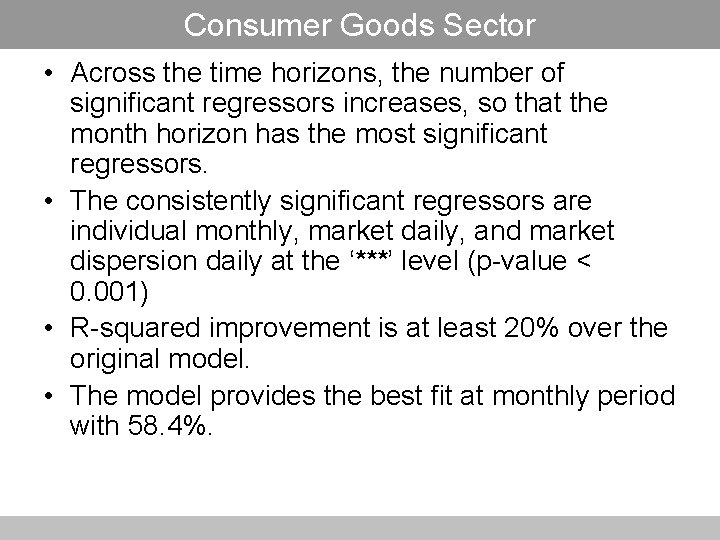
Consumer Goods Sector • Across the time horizons, the number of significant regressors increases, so that the month horizon has the most significant regressors. • The consistently significant regressors are individual monthly, market daily, and market dispersion daily at the ‘***’ level (p-value < 0. 001) • R-squared improvement is at least 20% over the original model. • The model provides the best fit at monthly period with 58. 4%.
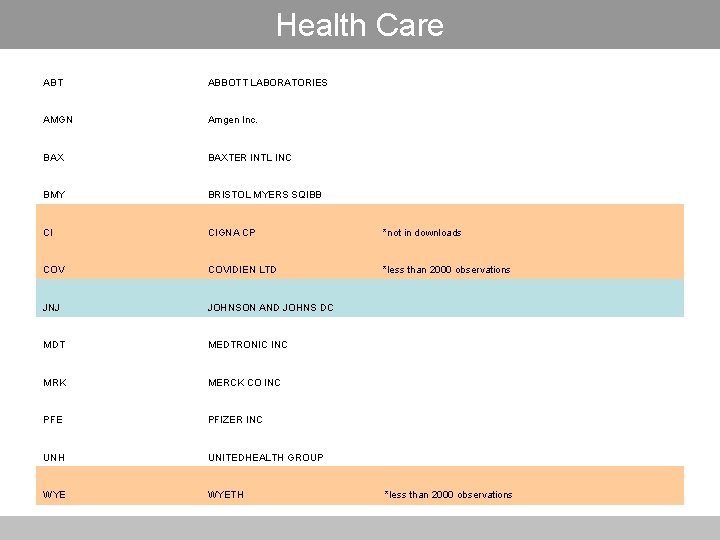
Health Care ABT ABBOTT LABORATORIES AMGN Amgen Inc. BAXTER INTL INC BMY BRISTOL MYERS SQIBB CI CIGNA CP *not in downloads COVIDIEN LTD *less than 2000 observations JNJ JOHNSON AND JOHNS DC MDT MEDTRONIC INC MRK MERCK CO INC PFE PFIZER INC UNH UNITEDHEALTH GROUP WYETH *less than 2000 observations
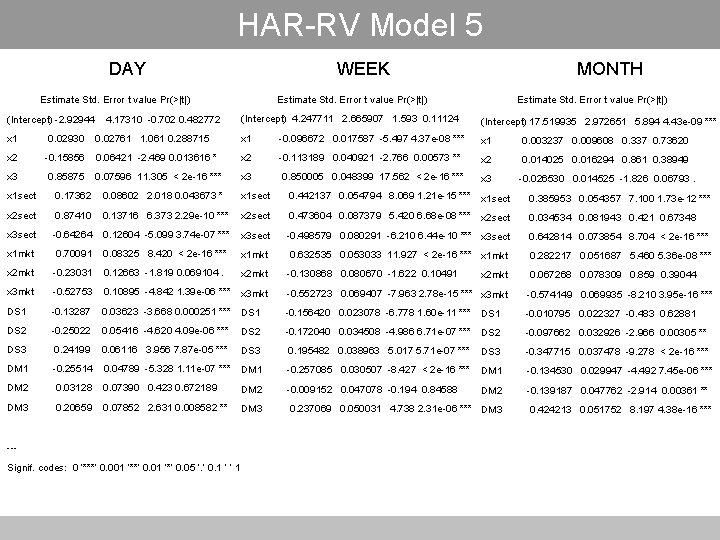
HAR-RV Model 5 DAY WEEK Estimate Std. Error t value Pr(>|t|) (Intercept) -2. 92944 4. 17310 -0. 702 0. 482772 MONTH Estimate Std. Error t value Pr(>|t|) (Intercept) 4. 247711 2. 665907 1. 593 0. 11124 (Intercept) 17. 519935 2. 972651 5. 894 4. 43 e-09 *** x 1 0. 02930 0. 02761 1. 061 0. 288715 x 1 -0. 096672 0. 017587 -5. 497 4. 37 e-08 *** x 1 0. 003237 0. 009608 0. 337 0. 73620 x 2 -0. 15856 0. 06421 -2. 469 0. 013616 * x 2 -0. 113189 0. 040921 -2. 766 0. 00573 ** x 2 0. 014025 0. 016294 0. 861 0. 38949 x 3 0. 85875 0. 07596 11. 305 < 2 e-16 *** x 3 0. 850005 0. 048399 17. 562 < 2 e-16 *** x 3 -0. 026530 0. 014525 -1. 826 0. 06793. x 1 sect 0. 17362 0. 08602 2. 018 0. 043673 * x 1 sect 0. 442137 0. 054794 8. 069 1. 21 e-15 *** x 1 sect 0. 385953 0. 054357 7. 100 1. 73 e-12 *** x 2 sect 0. 87410 0. 13716 6. 373 2. 29 e-10 *** x 2 sect 0. 473604 0. 087379 5. 420 6. 68 e-08 *** x 2 sect 0. 034534 0. 081943 0. 421 0. 67348 x 3 sect -0. 64264 0. 12604 -5. 099 3. 74 e-07 *** x 3 sect -0. 498579 0. 080291 -6. 210 6. 44 e-10 *** x 3 sect 0. 642814 0. 073854 8. 704 < 2 e-16 *** x 1 mkt 0. 70091 0. 08325 8. 420 < 2 e-16 *** x 1 mkt 0. 632535 0. 053033 11. 927 < 2 e-16 *** x 1 mkt 0. 282217 0. 051687 5. 460 5. 36 e-08 *** x 2 mkt -0. 23031 0. 12663 -1. 819 0. 069104. x 2 mkt -0. 130868 0. 080670 -1. 622 0. 10491 0. 067268 0. 078309 0. 859 0. 39044 x 3 mkt -0. 52753 0. 10895 -4. 842 1. 39 e-06 *** x 3 mkt -0. 552723 0. 069407 -7. 963 2. 78 e-15 *** x 3 mkt -0. 574149 0. 069935 -8. 210 3. 95 e-16 *** DS 1 -0. 13287 0. 03623 -3. 668 0. 000251 *** DS 1 -0. 156420 0. 023078 -6. 778 1. 60 e-11 *** DS 1 -0. 010795 0. 022327 -0. 483 0. 62881 DS 2 -0. 25022 0. 05416 -4. 620 4. 09 e-06 *** DS 2 -0. 172040 0. 034508 -4. 986 6. 71 e-07 *** DS 2 -0. 097662 0. 032926 -2. 966 0. 00305 ** DS 3 0. 24199 0. 06116 3. 956 7. 87 e-05 *** DS 3 0. 195482 0. 038963 5. 017 5. 71 e-07 *** DS 3 -0. 347715 0. 037478 -9. 278 < 2 e-16 *** DM 1 -0. 25514 0. 04789 -5. 328 1. 11 e-07 *** DM 1 -0. 257085 0. 030507 -8. 427 < 2 e-16 *** DM 1 -0. 134530 0. 029947 -4. 492 7. 45 e-06 *** DM 2 0. 03128 0. 07390 0. 423 0. 672189 DM 2 -0. 009152 0. 047078 -0. 194 0. 84588 DM 2 -0. 139187 0. 047762 -2. 914 0. 00361 ** DM 3 0. 20659 0. 07852 2. 631 0. 008582 ** DM 3 0. 237069 0. 050031 4. 738 2. 31 e-06 *** DM 3 --Signif. codes: 0 ‘***’ 0. 001 ‘**’ 0. 01 ‘*’ 0. 05 ‘. ’ 0. 1 ‘ ’ 1 x 2 mkt 0. 424213 0. 051752 8. 197 4. 38 e-16 ***
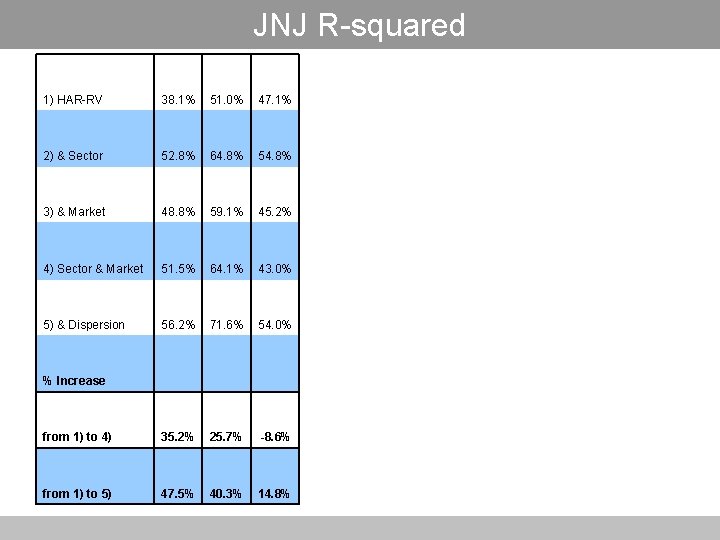
JNJ R-squared 1) HAR-RV 38. 1% 51. 0% 47. 1% 2) & Sector 52. 8% 64. 8% 54. 8% 3) & Market 48. 8% 59. 1% 45. 2% 4) Sector & Market 51. 5% 64. 1% 43. 0% 5) & Dispersion 56. 2% 71. 6% 54. 0% from 1) to 4) 35. 2% 25. 7% -8. 6% from 1) to 5) 47. 5% 40. 3% 14. 8% % Increase
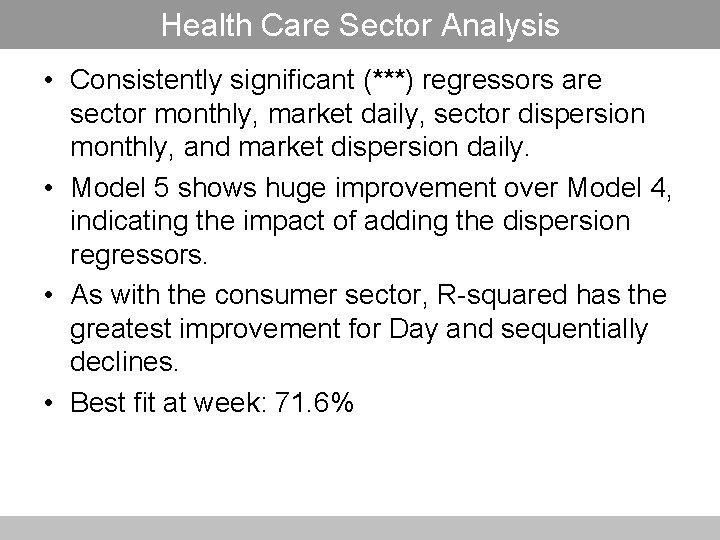
Health Care Sector Analysis • Consistently significant (***) regressors are sector monthly, market daily, sector dispersion monthly, and market dispersion daily. • Model 5 shows huge improvement over Model 4, indicating the impact of adding the dispersion regressors. • As with the consumer sector, R-squared has the greatest improvement for Day and sequentially declines. • Best fit at week: 71. 6%
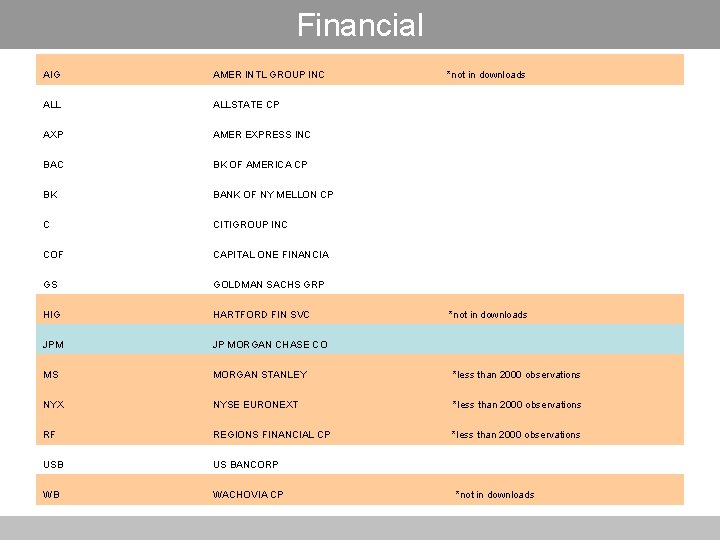
Financial AIG AMER INTL GROUP INC *not in downloads ALLSTATE CP AXP AMER EXPRESS INC BAC BK OF AMERICA CP BK BANK OF NY MELLON CP C CITIGROUP INC COF CAPITAL ONE FINANCIA GS GOLDMAN SACHS GRP HIG HARTFORD FIN SVC JPM JP MORGAN CHASE CO MS MORGAN STANLEY *less than 2000 observations NYX NYSE EURONEXT *less than 2000 observations RF REGIONS FINANCIAL CP *less than 2000 observations USB US BANCORP WB WACHOVIA CP *not in downloads
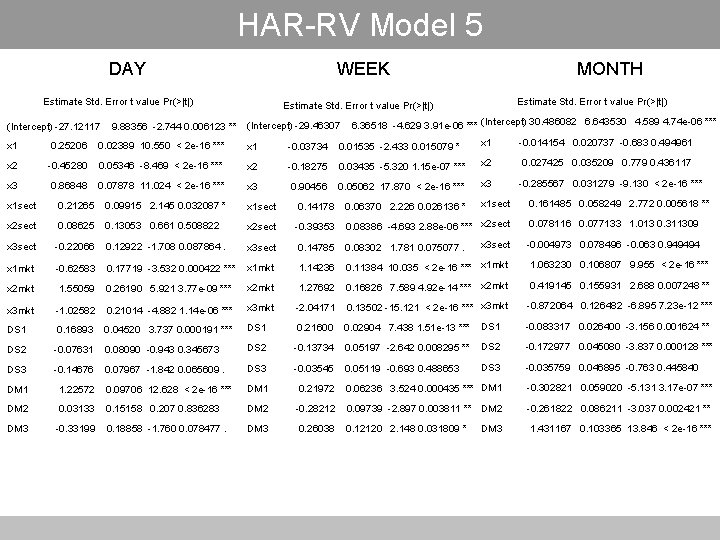
HAR-RV Model 5 DAY WEEK Estimate Std. Error t value Pr(>|t|) (Intercept) -27. 12117 9. 88356 -2. 744 0. 006123 ** MONTH Estimate Std. Error t value Pr(>|t|) (Intercept) -29. 46307 6. 36518 -4. 629 3. 91 e-06 *** (Intercept) 30. 486082 6. 643530 4. 589 4. 74 e-06 *** x 1 0. 25206 0. 02389 10. 550 < 2 e-16 *** x 1 -0. 03734 0. 01535 -2. 433 0. 015079 * x 1 -0. 014154 0. 020737 -0. 683 0. 494961 x 2 -0. 45280 0. 05346 -8. 469 < 2 e-16 *** x 2 -0. 18275 0. 03435 -5. 320 1. 15 e-07 *** x 2 0. 027425 0. 035209 0. 779 0. 436117 x 3 0. 86848 0. 07878 11. 024 < 2 e-16 *** x 3 0. 90456 0. 05062 17. 870 < 2 e-16 *** x 3 -0. 285567 0. 031279 -9. 130 < 2 e-16 *** x 1 sect 0. 161485 0. 058249 2. 772 0. 005618 ** x 1 sect 0. 21265 0. 09915 2. 145 0. 032087 * x 1 sect 0. 14178 0. 06370 2. 226 0. 026136 * x 2 sect 0. 08625 0. 13053 0. 661 0. 508822 x 2 sect -0. 39353 0. 08386 -4. 693 2. 88 e-06 *** x 2 sect 0. 078116 0. 077133 1. 013 0. 311309 x 3 sect -0. 22066 0. 12922 -1. 708 0. 087864. x 3 sect 0. 14785 0. 08302 1. 781 0. 075077. x 3 sect -0. 004973 0. 078496 -0. 063 0. 949494 x 1 mkt -0. 62583 0. 17719 -3. 532 0. 000422 *** x 1 mkt 1. 14236 0. 11384 10. 035 < 2 e-16 *** x 1 mkt 1. 063230 0. 106807 9. 955 < 2 e-16 *** x 2 mkt 1. 55059 0. 26190 5. 921 3. 77 e-09 *** x 2 mkt 1. 27692 0. 16826 7. 589 4. 92 e-14 *** x 2 mkt 0. 419145 0. 155931 2. 688 0. 007248 ** x 3 mkt -1. 02582 0. 21014 -4. 882 1. 14 e-06 *** x 3 mkt -2. 04171 0. 13502 -15. 121 < 2 e-16 *** x 3 mkt -0. 872064 0. 126482 -6. 895 7. 23 e-12 *** DS 1 0. 16893 0. 04520 3. 737 0. 000191 *** DS 1 0. 21600 0. 02904 7. 438 1. 51 e-13 *** DS 1 -0. 083317 0. 026400 -3. 156 0. 001624 ** DS 2 -0. 07631 0. 08090 -0. 943 0. 345673 DS 2 -0. 13734 0. 05197 -2. 642 0. 008295 ** DS 2 -0. 172977 0. 045080 -3. 837 0. 000128 *** DS 3 -0. 14676 0. 07967 -1. 842 0. 065609. DS 3 -0. 03545 0. 05119 -0. 693 0. 488653 DS 3 -0. 035759 0. 046895 -0. 763 0. 445840 DM 1 1. 22572 0. 09706 12. 628 < 2 e-16 *** DM 1 0. 21972 0. 06236 3. 524 0. 000435 *** DM 1 -0. 302821 0. 059020 -5. 131 3. 17 e-07 *** DM 2 0. 03133 0. 15158 0. 207 0. 836283 DM 2 -0. 28212 0. 09739 -2. 897 0. 003811 ** DM 2 -0. 261822 0. 086211 -3. 037 0. 002421 ** DM 3 -0. 33199 0. 18858 -1. 760 0. 078477. DM 3 0. 26038 0. 12120 2. 148 0. 031809 * 1. 431167 0. 103365 13. 846 < 2 e-16 *** DM 3
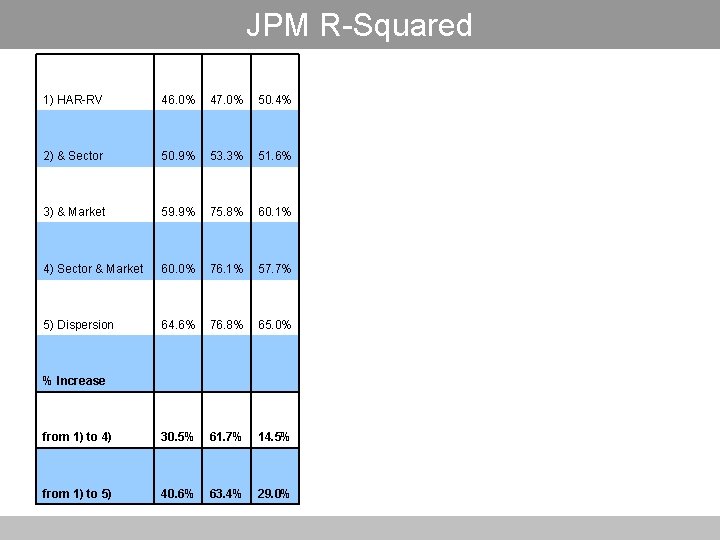
JPM R-Squared 1) HAR-RV 46. 0% 47. 0% 50. 4% 2) & Sector 50. 9% 53. 3% 51. 6% 3) & Market 59. 9% 75. 8% 60. 1% 4) Sector & Market 60. 0% 76. 1% 57. 7% 5) Dispersion 64. 6% 76. 8% 65. 0% from 1) to 4) 30. 5% 61. 7% 14. 5% from 1) to 5) 40. 6% 63. 4% 29. 0% % Increase
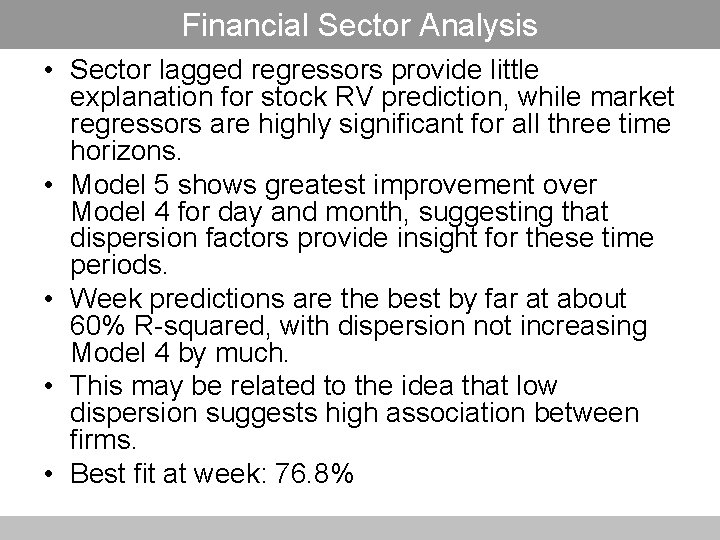
Financial Sector Analysis • Sector lagged regressors provide little explanation for stock RV prediction, while market regressors are highly significant for all three time horizons. • Model 5 shows greatest improvement over Model 4 for day and month, suggesting that dispersion factors provide insight for these time periods. • Week predictions are the best by far at about 60% R-squared, with dispersion not increasing Model 4 by much. • This may be related to the idea that low dispersion suggests high association between firms. • Best fit at week: 76. 8%
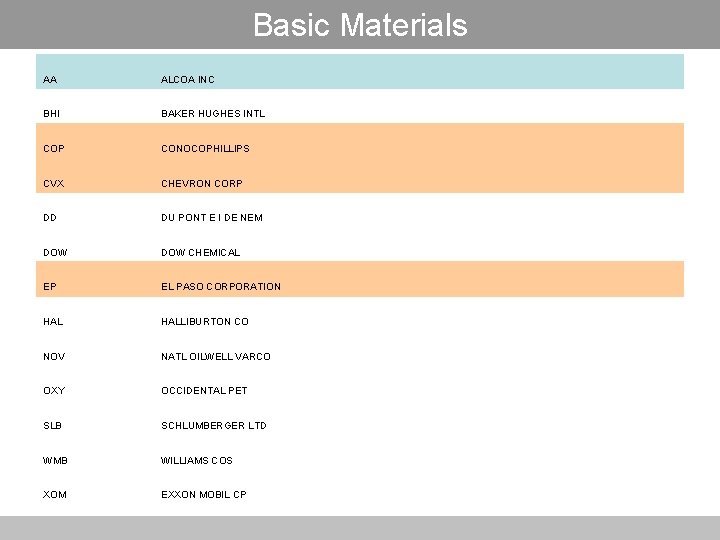
Basic Materials AA ALCOA INC BHI BAKER HUGHES INTL COP CONOCOPHILLIPS CVX CHEVRON CORP DD DU PONT E I DE NEM DOW CHEMICAL EP EL PASO CORPORATION HALLIBURTON CO NOV NATL OILWELL VARCO OXY OCCIDENTAL PET SLB SCHLUMBERGER LTD WMB WILLIAMS COS XOM EXXON MOBIL CP
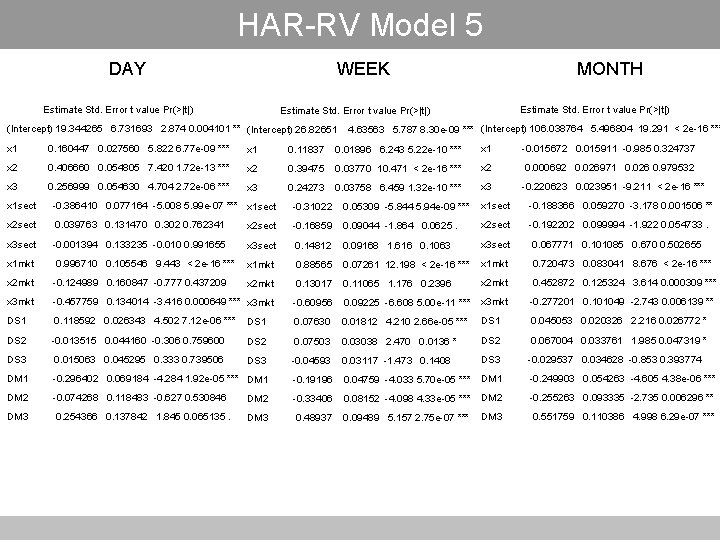
HAR-RV Model 5 DAY WEEK Estimate Std. Error t value Pr(>|t|) MONTH Estimate Std. Error t value Pr(>|t|) (Intercept) 19. 344265 6. 731693 2. 874 0. 004101 ** (Intercept) 26. 82651 4. 63563 5. 787 8. 30 e-09 *** (Intercept) 106. 038764 5. 496804 19. 291 < 2 e-16 *** x 1 0. 160447 0. 027560 5. 822 6. 77 e-09 *** x 1 0. 11837 0. 01896 6. 243 5. 22 e-10 *** x 1 -0. 015672 0. 015911 -0. 985 0. 324737 x 2 0. 406660 0. 054805 7. 420 1. 72 e-13 *** x 2 0. 39475 0. 03770 10. 471 < 2 e-16 *** x 2 0. 000692 0. 026971 0. 026 0. 979532 x 3 0. 256999 0. 054630 4. 704 2. 72 e-06 *** x 3 0. 24273 0. 03758 6. 459 1. 32 e-10 *** x 3 -0. 220623 0. 023951 -9. 211 < 2 e-16 *** x 1 sect -0. 386410 0. 077164 -5. 008 5. 99 e-07 *** x 1 sect -0. 31022 0. 05309 -5. 844 5. 94 e-09 *** x 1 sect -0. 188366 0. 059270 -3. 178 0. 001506 ** x 2 sect 0. 039763 0. 131470 0. 302 0. 762341 x 2 sect -0. 16859 0. 09044 -1. 864 0. 0625. x 2 sect -0. 192202 0. 099994 -1. 922 0. 054733. x 3 sect -0. 001394 0. 133235 -0. 010 0. 991655 x 3 sect 0. 14812 0. 09168 1. 616 0. 1063 x 3 sect 0. 067771 0. 101085 0. 670 0. 502655 x 1 mkt 0. 996710 0. 105546 9. 443 < 2 e-16 *** x 1 mkt 0. 88565 0. 07261 12. 198 < 2 e-16 *** x 1 mkt 0. 720473 0. 083041 8. 676 < 2 e-16 *** x 2 mkt -0. 124989 0. 160847 -0. 777 0. 437209 x 2 mkt 0. 13017 0. 11065 1. 176 0. 2396 x 2 mkt 0. 452872 0. 125324 3. 614 0. 000309 *** x 3 mkt -0. 457759 0. 134014 -3. 416 0. 000649 *** x 3 mkt -0. 60956 0. 09225 -6. 608 5. 00 e-11 *** x 3 mkt -0. 277201 0. 101049 -2. 743 0. 006139 ** DS 1 0. 118592 0. 026343 4. 502 7. 12 e-06 *** DS 1 0. 07630 0. 01812 4. 210 2. 66 e-05 *** DS 1 0. 045053 0. 020326 2. 216 0. 026772 * DS 2 -0. 013515 0. 044160 -0. 306 0. 759600 DS 2 0. 07503 0. 03038 2. 470 0. 0136 * DS 2 0. 067004 0. 033761 1. 985 0. 047319 * DS 3 0. 015063 0. 045295 0. 333 0. 739506 DS 3 -0. 04593 0. 03117 -1. 473 0. 1408 DS 3 -0. 029537 0. 034628 -0. 853 0. 393774 DM 1 -0. 296402 0. 069184 -4. 284 1. 92 e-05 *** DM 1 -0. 19196 0. 04759 -4. 033 5. 70 e-05 *** DM 1 -0. 249903 0. 054263 -4. 605 4. 38 e-06 *** DM 2 -0. 074268 0. 118483 -0. 627 0. 530846 DM 2 -0. 33406 0. 08152 -4. 098 4. 33 e-05 *** DM 2 -0. 255263 0. 093335 -2. 735 0. 006296 ** DM 3 0. 254366 0. 137842 1. 845 0. 065135. DM 3 0. 48937 0. 09489 5. 157 2. 75 e-07 *** DM 3 0. 551759 0. 110386 4. 998 6. 29 e-07 ***
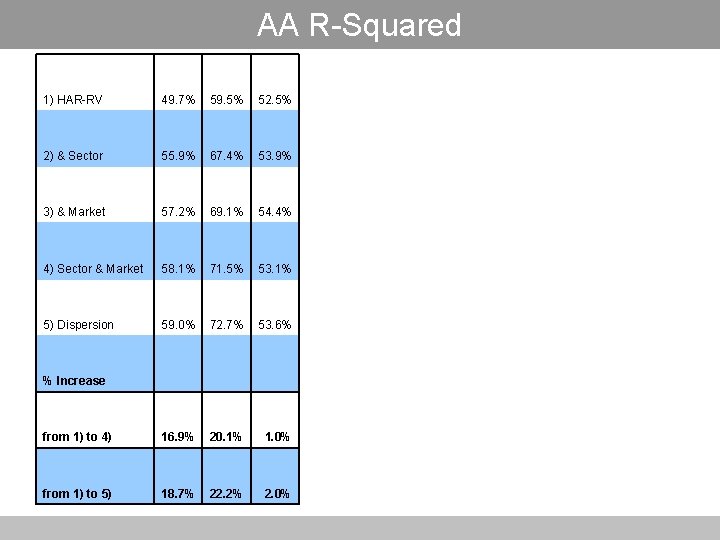
AA R-Squared 1) HAR-RV 49. 7% 59. 5% 52. 5% 2) & Sector 55. 9% 67. 4% 53. 9% 3) & Market 57. 2% 69. 1% 54. 4% 4) Sector & Market 58. 1% 71. 5% 53. 1% 5) Dispersion 59. 0% 72. 7% 53. 6% from 1) to 4) 16. 9% 20. 1% 1. 0% from 1) to 5) 18. 7% 22. 2% 2. 0% % Increase
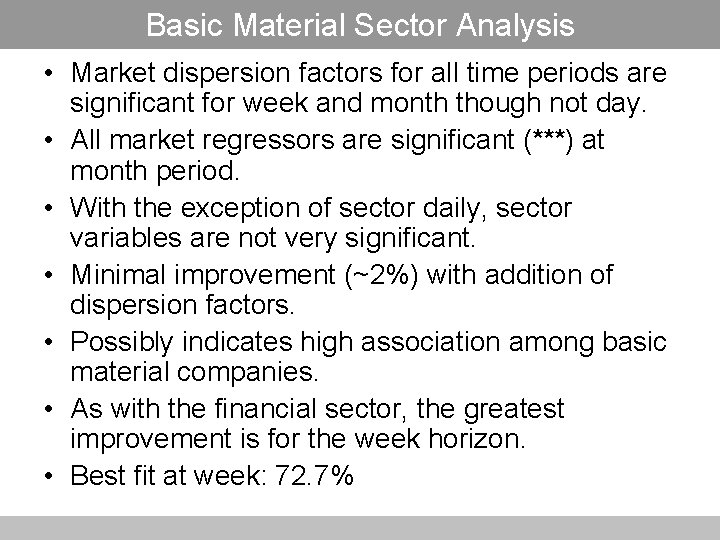
Basic Material Sector Analysis • Market dispersion factors for all time periods are significant for week and month though not day. • All market regressors are significant (***) at month period. • With the exception of sector daily, sector variables are not very significant. • Minimal improvement (~2%) with addition of dispersion factors. • Possibly indicates high association among basic material companies. • As with the financial sector, the greatest improvement is for the week horizon. • Best fit at week: 72. 7%
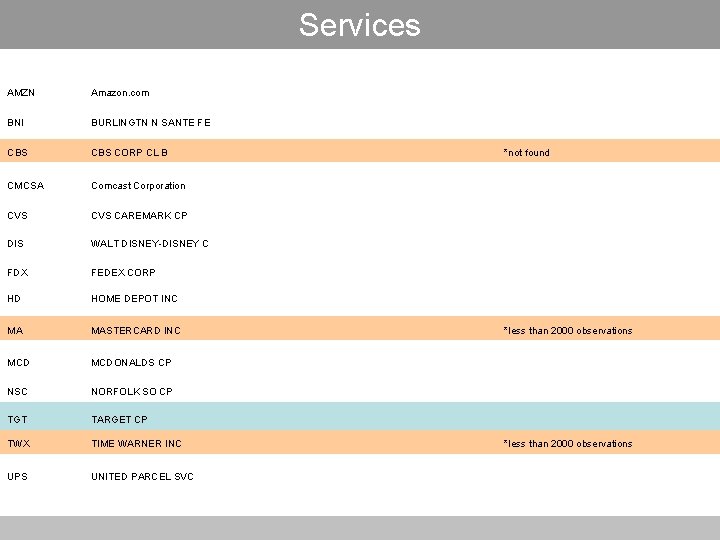
Services AMZN Amazon. com BNI BURLINGTN N SANTE FE CBS CORP CL B CMCSA Comcast Corporation CVS CAREMARK CP DIS WALT DISNEY-DISNEY C FDX FEDEX CORP HD HOME DEPOT INC MA MASTERCARD INC MCDONALDS CP NSC NORFOLK SO CP TGT TARGET CP TWX TIME WARNER INC UPS UNITED PARCEL SVC *not found *less than 2000 observations
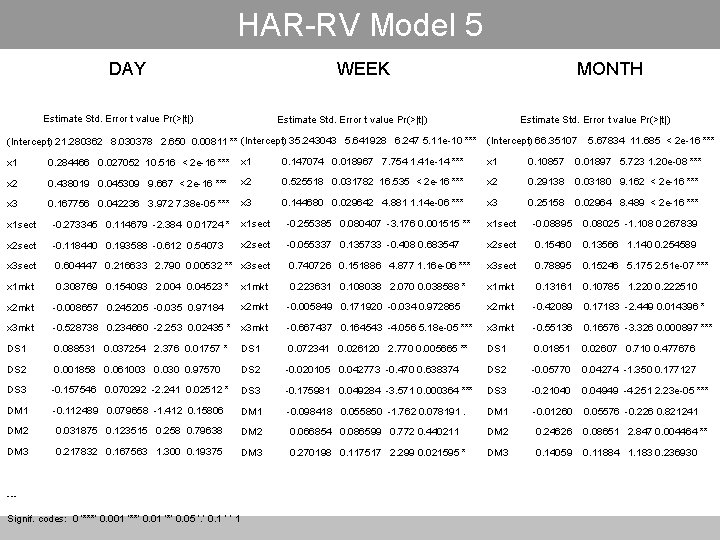
HAR-RV Model 5 DAY WEEK Estimate Std. Error t value Pr(>|t|) MONTH Estimate Std. Error t value Pr(>|t|) (Intercept) 21. 280362 8. 030378 2. 650 0. 00811 ** (Intercept) 35. 243043 5. 641928 6. 247 5. 11 e-10 *** Estimate Std. Error t value Pr(>|t|) (Intercept) 66. 35107 5. 67834 11. 685 < 2 e-16 *** x 1 0. 284466 0. 027052 10. 516 < 2 e-16 *** x 1 0. 147074 0. 018967 7. 754 1. 41 e-14 *** x 1 0. 10857 0. 01897 5. 723 1. 20 e-08 *** x 2 0. 438019 0. 045309 9. 667 < 2 e-16 *** x 2 0. 525518 0. 031782 16. 535 < 2 e-16 *** x 2 0. 29138 0. 03180 9. 162 < 2 e-16 *** x 3 0. 167756 0. 042236 3. 972 7. 38 e-05 *** x 3 0. 144680 0. 029642 4. 881 1. 14 e-06 *** x 3 0. 25158 0. 02964 8. 489 < 2 e-16 *** x 1 sect -0. 273345 0. 114679 -2. 384 0. 01724 * x 1 sect -0. 255385 0. 080407 -3. 176 0. 001515 ** x 1 sect -0. 08895 0. 08025 -1. 108 0. 267839 x 2 sect -0. 118440 0. 193588 -0. 612 0. 54073 x 2 sect -0. 055337 0. 135733 -0. 408 0. 683547 x 2 sect 0. 15460 0. 13566 1. 140 0. 254589 x 3 sect 0. 604447 0. 216633 2. 790 0. 00532 ** x 3 sect 0. 740726 0. 151886 4. 877 1. 16 e-06 *** x 3 sect 0. 78895 0. 15246 5. 175 2. 51 e-07 *** x 1 mkt 0. 308769 0. 154093 2. 004 0. 04523 * x 1 mkt 0. 223631 0. 108038 2. 070 0. 038588 * x 1 mkt 0. 13161 0. 10785 1. 220 0. 222510 x 2 mkt -0. 008657 0. 245205 -0. 035 0. 97184 x 2 mkt -0. 005849 0. 171920 -0. 034 0. 972865 x 2 mkt -0. 42089 0. 17183 -2. 449 0. 014396 * x 3 mkt -0. 528738 0. 234660 -2. 253 0. 02435 * x 3 mkt -0. 667437 0. 164543 -4. 056 5. 18 e-05 *** x 3 mkt -0. 55136 0. 16576 -3. 326 0. 000897 *** DS 1 0. 088531 0. 037254 2. 376 0. 01757 * DS 1 0. 072341 0. 026120 2. 770 0. 005665 ** DS 1 0. 01851 0. 02607 0. 710 0. 477676 DS 2 0. 001858 0. 061003 0. 030 0. 97570 DS 2 -0. 020105 0. 042773 -0. 470 0. 638374 DS 2 -0. 05770 0. 04274 -1. 350 0. 177127 DS 3 -0. 157546 0. 070292 -2. 241 0. 02512 * DS 3 -0. 175981 0. 049284 -3. 571 0. 000364 *** DS 3 -0. 21040 0. 04949 -4. 251 2. 23 e-05 *** DM 1 -0. 112489 0. 079658 -1. 412 0. 15806 DM 1 -0. 098418 0. 055850 -1. 762 0. 078191. DM 1 -0. 01260 0. 05576 -0. 226 0. 821241 DM 2 0. 031875 0. 123515 0. 258 0. 79638 DM 2 0. 066854 0. 086599 0. 772 0. 440211 DM 2 0. 24626 0. 08651 2. 847 0. 004464 ** DM 3 0. 217832 0. 167563 1. 300 0. 19375 DM 3 0. 270198 0. 117517 2. 299 0. 021595 * DM 3 0. 14059 0. 11884 1. 183 0. 236930 --Signif. codes: 0 ‘***’ 0. 001 ‘**’ 0. 01 ‘*’ 0. 05 ‘. ’ 0. 1 ‘ ’ 1
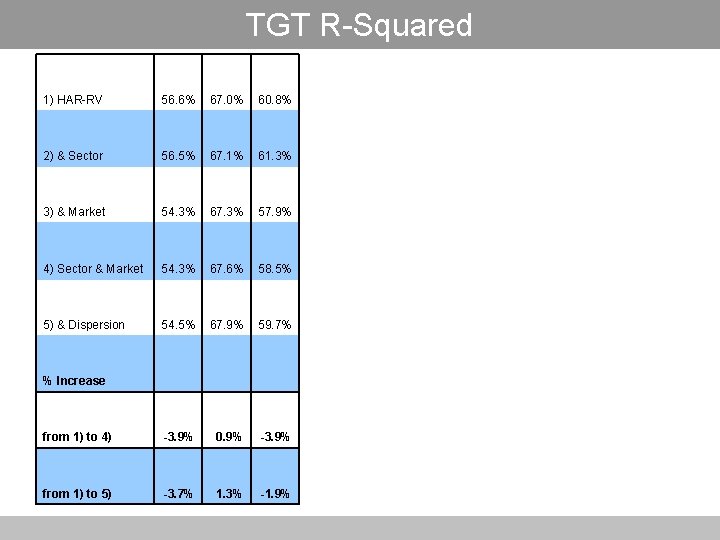
TGT R-Squared 1) HAR-RV 56. 6% 67. 0% 60. 8% 2) & Sector 56. 5% 67. 1% 61. 3% 3) & Market 54. 3% 67. 3% 57. 9% 4) Sector & Market 54. 3% 67. 6% 58. 5% 5) & Dispersion 54. 5% 67. 9% 59. 7% from 1) to 4) -3. 9% 0. 9% -3. 9% from 1) to 5) -3. 7% 1. 3% -1. 9% % Increase
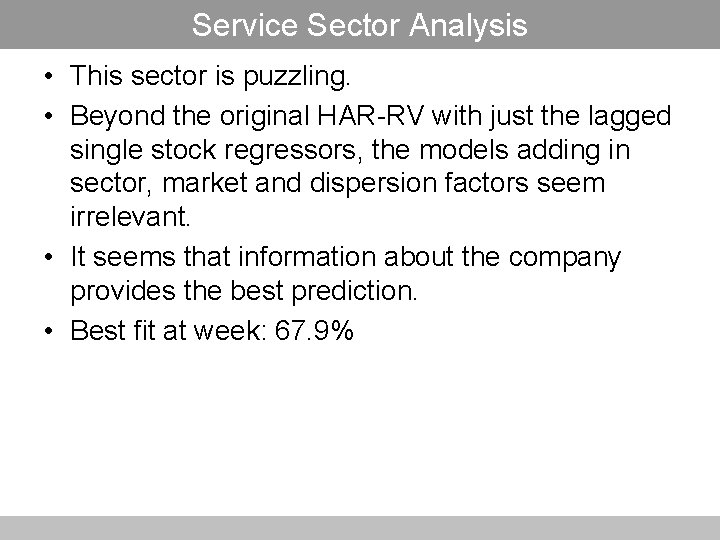
Service Sector Analysis • This sector is puzzling. • Beyond the original HAR-RV with just the lagged single stock regressors, the models adding in sector, market and dispersion factors seem irrelevant. • It seems that information about the company provides the best prediction. • Best fit at week: 67. 9%
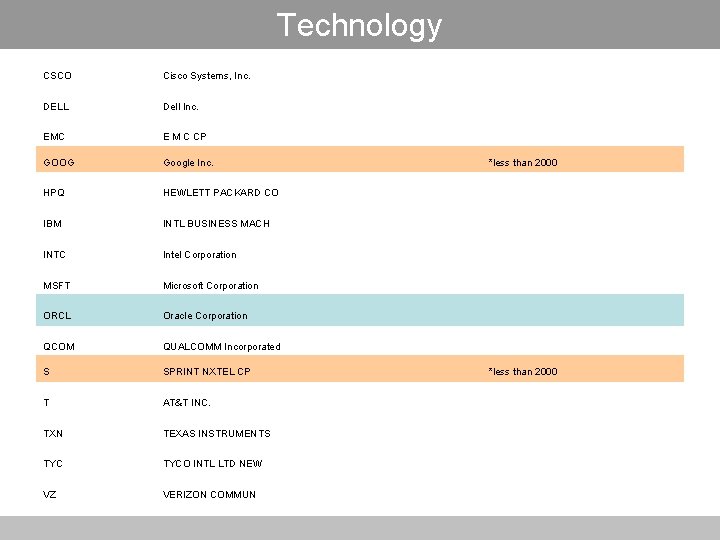
Technology CSCO Cisco Systems, Inc. DELL Dell Inc. EMC E M C CP GOOG Google Inc. HPQ HEWLETT PACKARD CO IBM INTL BUSINESS MACH INTC Intel Corporation MSFT Microsoft Corporation ORCL Oracle Corporation QCOM QUALCOMM Incorporated S SPRINT NXTEL CP T AT&T INC. TXN TEXAS INSTRUMENTS TYCO INTL LTD NEW VZ VERIZON COMMUN *less than 2000
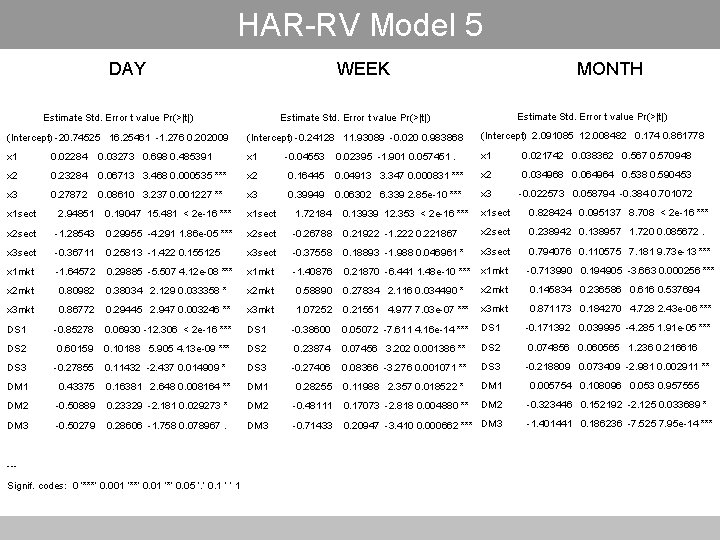
HAR-RV Model 5 DAY WEEK MONTH Estimate Std. Error t value Pr(>|t|) (Intercept) -20. 74525 16. 25461 -1. 276 0. 202009 (Intercept) -0. 24128 11. 93089 -0. 020 0. 983868 (Intercept) 2. 091085 12. 008482 0. 174 0. 861778 x 1 0. 02284 0. 03273 0. 698 0. 485391 x 1 -0. 04553 0. 02395 -1. 901 0. 057451. x 1 0. 021742 0. 038362 0. 567 0. 570948 x 2 0. 23284 0. 06713 3. 468 0. 000535 *** x 2 0. 16445 0. 04913 3. 347 0. 000831 *** x 2 0. 034968 0. 064964 0. 538 0. 590453 x 3 0. 27872 0. 08610 3. 237 0. 001227 ** x 3 0. 39949 0. 06302 6. 339 2. 85 e-10 *** x 3 -0. 022573 0. 058794 -0. 384 0. 701072 x 1 sect 2. 94851 0. 19047 15. 481 < 2 e-16 *** x 1 sect 1. 72184 0. 13939 12. 353 < 2 e-16 *** x 1 sect 0. 828424 0. 095137 8. 708 < 2 e-16 *** x 2 sect -1. 28543 0. 29955 -4. 291 1. 86 e-05 *** x 2 sect -0. 26788 0. 21922 -1. 222 0. 221867 x 2 sect 0. 238942 0. 138957 1. 720 0. 085672. x 3 sect -0. 36711 0. 25813 -1. 422 0. 155125 x 3 sect -0. 37558 0. 18893 -1. 988 0. 046961 * x 3 sect 0. 794076 0. 110575 7. 181 9. 73 e-13 *** x 1 mkt -1. 64572 0. 29885 -5. 507 4. 12 e-08 *** x 1 mkt -1. 40876 0. 21870 -6. 441 1. 48 e-10 *** x 1 mkt -0. 713990 0. 194905 -3. 663 0. 000256 *** x 2 mkt 0. 80982 0. 38034 2. 129 0. 033358 * x 2 mkt 0. 58890 0. 27834 2. 116 0. 034490 * x 2 mkt 0. 145834 0. 236586 0. 616 0. 537694 x 3 mkt 0. 86772 0. 29445 2. 947 0. 003246 ** x 3 mkt 1. 07252 0. 21551 4. 977 7. 03 e-07 *** x 3 mkt 0. 871173 0. 184270 4. 728 2. 43 e-06 *** DS 1 -0. 85278 0. 06930 -12. 306 < 2 e-16 *** DS 1 -0. 38600 0. 05072 -7. 611 4. 16 e-14 *** DS 1 -0. 171392 0. 039995 -4. 285 1. 91 e-05 *** DS 2 0. 60159 0. 10188 5. 905 4. 13 e-09 *** DS 2 0. 23874 0. 07456 3. 202 0. 001386 ** DS 2 0. 074856 0. 060565 1. 236 0. 216616 DS 3 -0. 27855 0. 11432 -2. 437 0. 014909 * DS 3 -0. 27406 0. 08366 -3. 276 0. 001071 ** DS 3 -0. 218809 0. 073409 -2. 981 0. 002911 ** DM 1 0. 43375 0. 16381 2. 648 0. 008164 ** DM 1 0. 28255 0. 11988 2. 357 0. 018522 * DM 1 0. 005754 0. 108096 0. 053 0. 957555 DM 2 -0. 50889 0. 23329 -2. 181 0. 029273 * DM 2 -0. 48111 0. 17073 -2. 818 0. 004880 ** DM 2 -0. 323446 0. 152192 -2. 125 0. 033689 * DM 3 -0. 50279 0. 28606 -1. 758 0. 078967. DM 3 -0. 71433 0. 20947 -3. 410 0. 000662 *** DM 3 --Signif. codes: 0 ‘***’ 0. 001 ‘**’ 0. 01 ‘*’ 0. 05 ‘. ’ 0. 1 ‘ ’ 1 -1. 401441 0. 186236 -7. 525 7. 95 e-14 ***
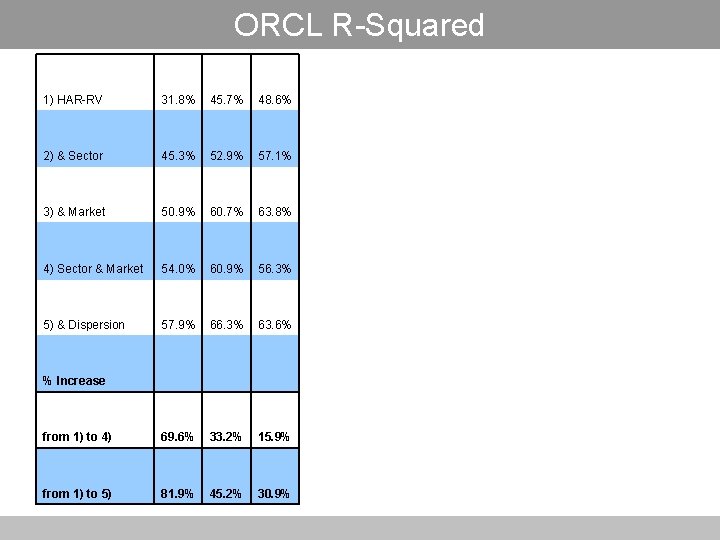
ORCL R-Squared 1) HAR-RV 31. 8% 45. 7% 48. 6% 2) & Sector 45. 3% 52. 9% 57. 1% 3) & Market 50. 9% 60. 7% 63. 8% 4) Sector & Market 54. 0% 60. 9% 56. 3% 5) & Dispersion 57. 9% 66. 3% 63. 6% from 1) to 4) 69. 6% 33. 2% 15. 9% from 1) to 5) 81. 9% 45. 2% 30. 9% % Increase
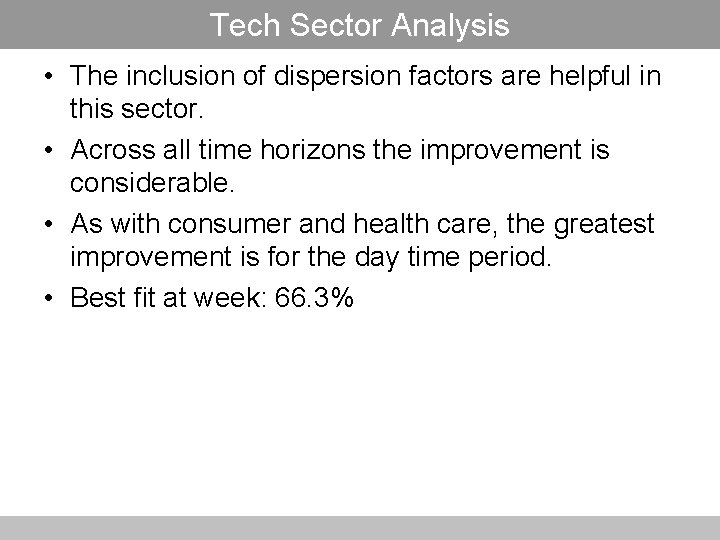
Tech Sector Analysis • The inclusion of dispersion factors are helpful in this sector. • Across all time horizons the improvement is considerable. • As with consumer and health care, the greatest improvement is for the day time period. • Best fit at week: 66. 3%
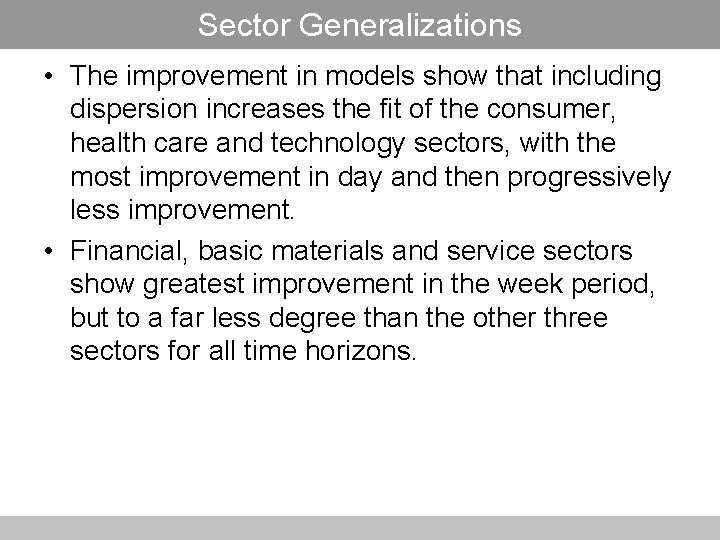
Sector Generalizations • The improvement in models show that including dispersion increases the fit of the consumer, health care and technology sectors, with the most improvement in day and then progressively less improvement. • Financial, basic materials and service sectors show greatest improvement in the week period, but to a far less degree than the other three sectors for all time horizons.
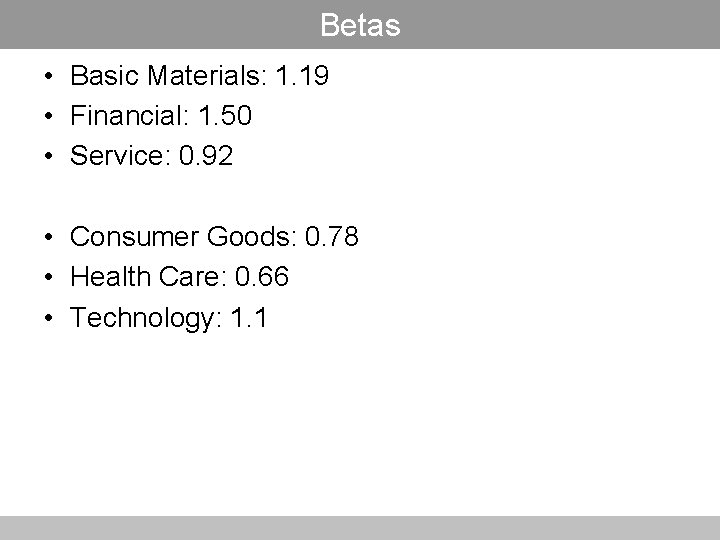
Betas • Basic Materials: 1. 19 • Financial: 1. 50 • Service: 0. 92 • Consumer Goods: 0. 78 • Health Care: 0. 66 • Technology: 1. 1
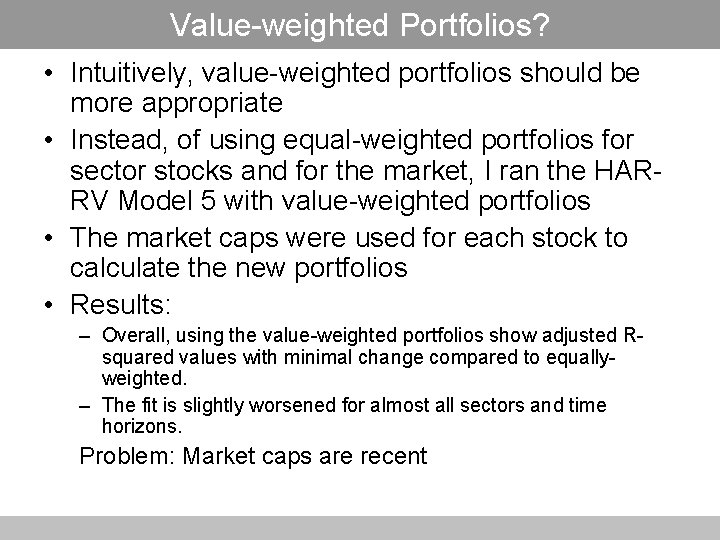
Value-weighted Portfolios? • Intuitively, value-weighted portfolios should be more appropriate • Instead, of using equal-weighted portfolios for sector stocks and for the market, I ran the HARRV Model 5 with value-weighted portfolios • The market caps were used for each stock to calculate the new portfolios • Results: – Overall, using the value-weighted portfolios show adjusted Rsquared values with minimal change compared to equallyweighted. – The fit is slightly worsened for almost all sectors and time horizons. Problem: Market caps are recent
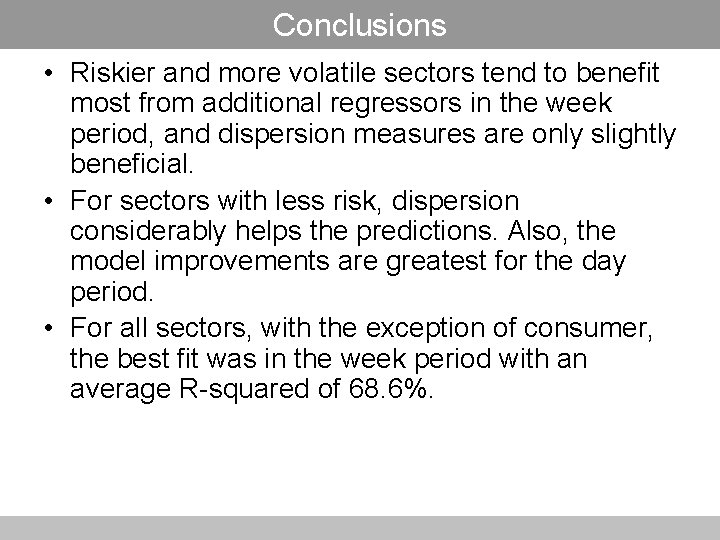
Conclusions • Riskier and more volatile sectors tend to benefit most from additional regressors in the week period, and dispersion measures are only slightly beneficial. • For sectors with less risk, dispersion considerably helps the predictions. Also, the model improvements are greatest for the day period. • For all sectors, with the exception of consumer, the best fit was in the week period with an average R-squared of 68. 6%.
Harrv
Harrv
Harrv
Multicollinearity
Stochastic regressors
Stochastic regressors
Difference between segmentation targeting and positioning
Market leader challenger follower nicher examples
Public sector governance models
What is the difference between model and semi modals
Invertebrates including snails slugs and mussels
Invertebrates including snails slugs and mussels
Four market models
Karen and sharon sanders
Intersex and identity sharon e. preves
Sharon draper biography
Primary target market and secondary target market
A model of business buyer behavior
Money market
Market identification
What is ipo
Importance of money
Printed words including dialogue
It encompasses several different aspects, including
Ice quotations
The mutcd recommends the use of emergency vehicles
Animals including humans year 6
Animals including humans
Animals including humans year 4
The committee debate these questions carefully
Printed words including dialogue
How many faces does square pyramid have
Including samuel discussion questions
Sharon buquet
What is the horror genre analyzing the text answers