Evaluation of ECHAM 5 General Circulation Model using
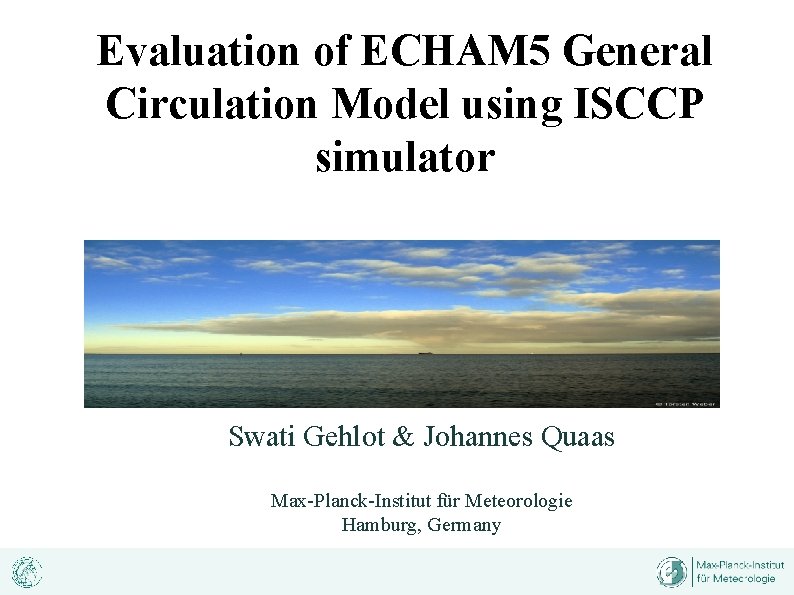
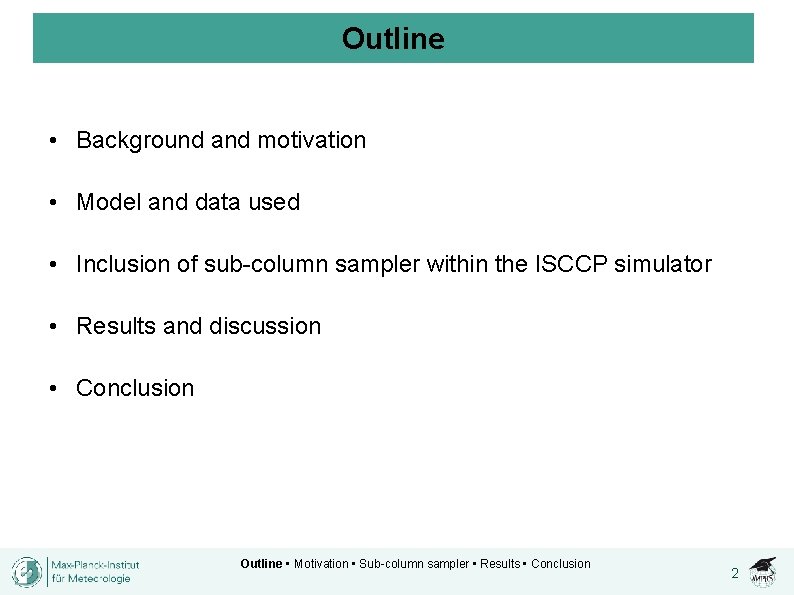
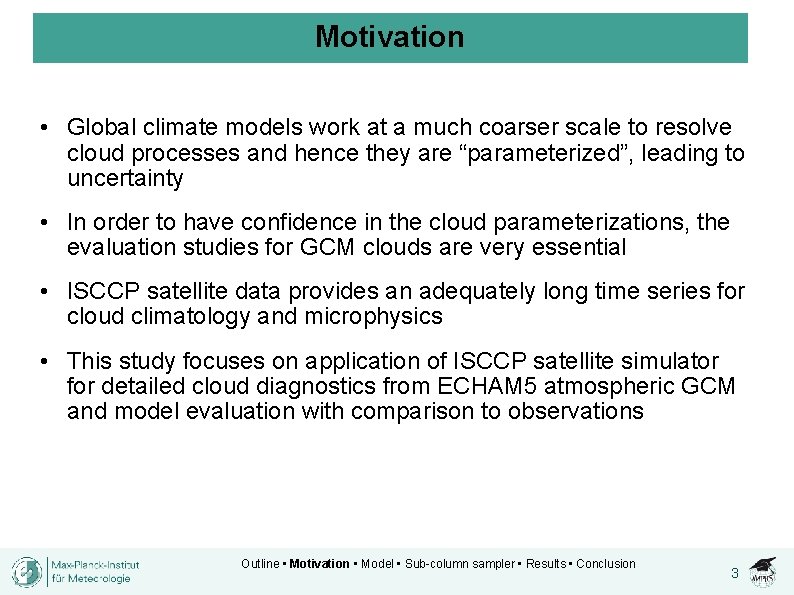
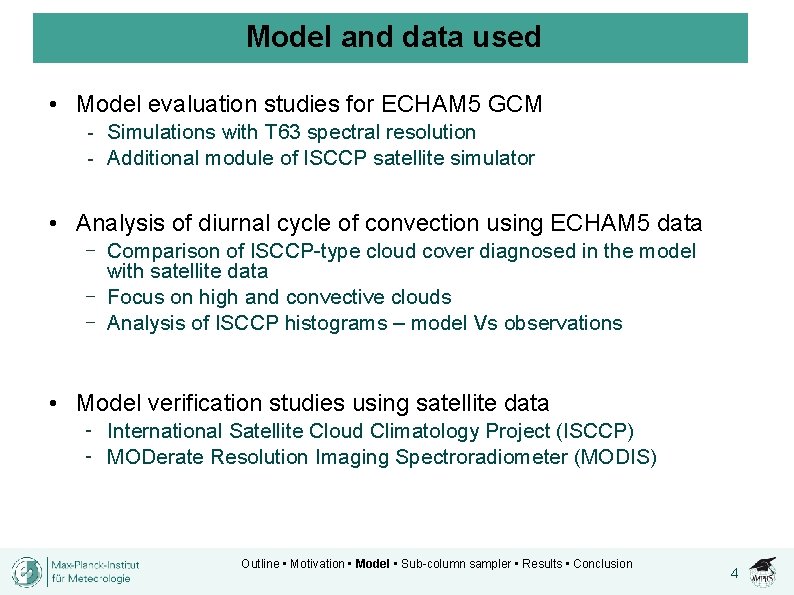
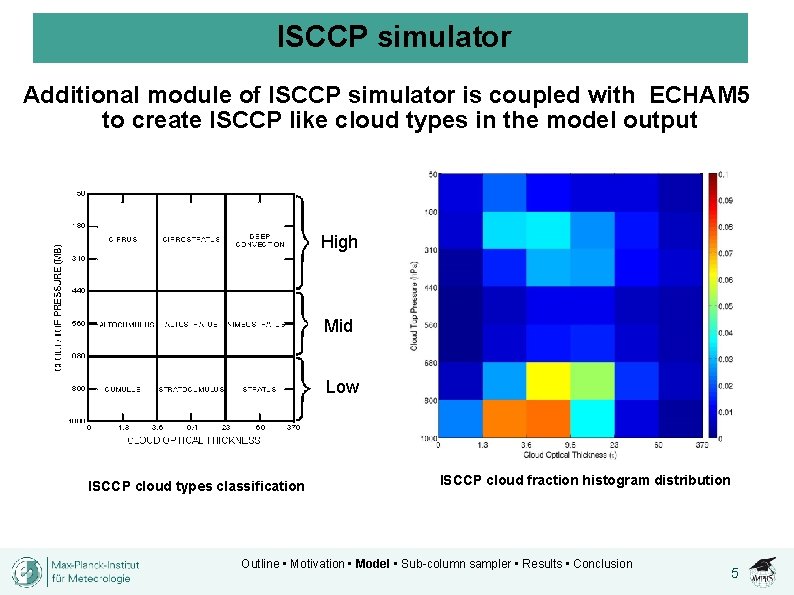
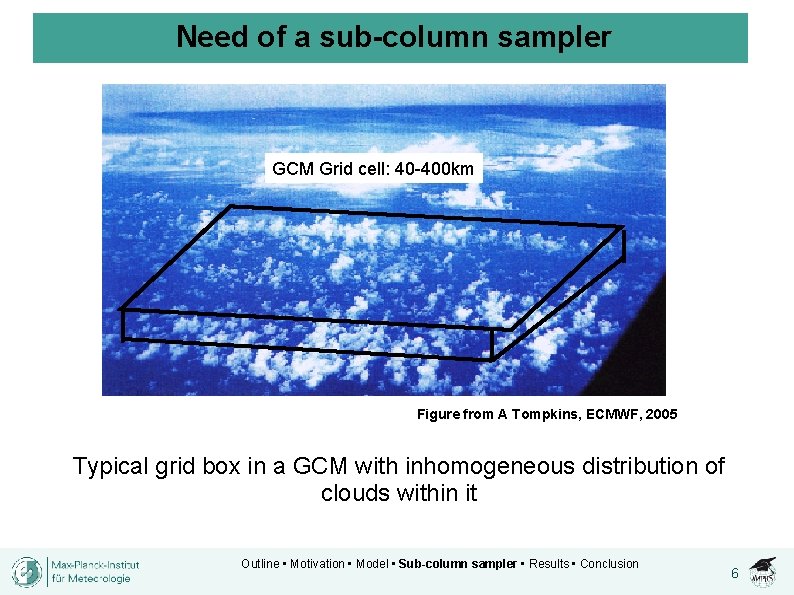
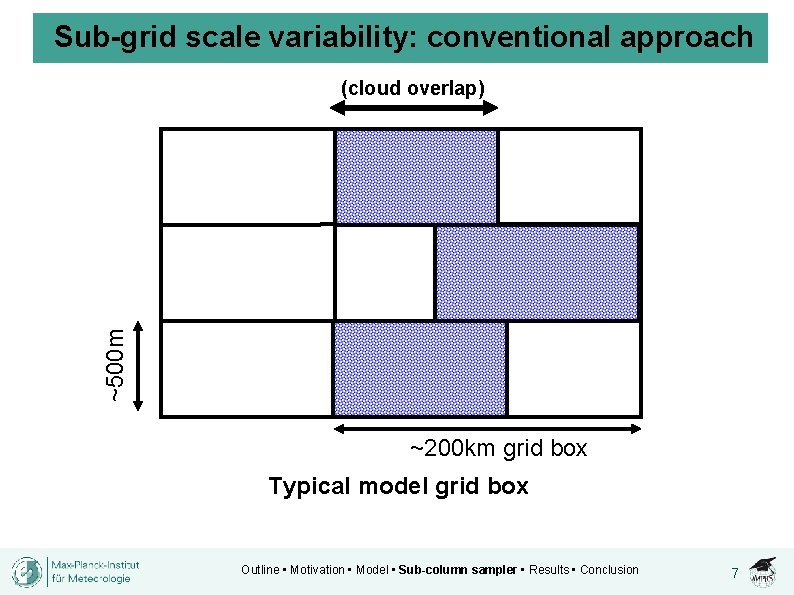
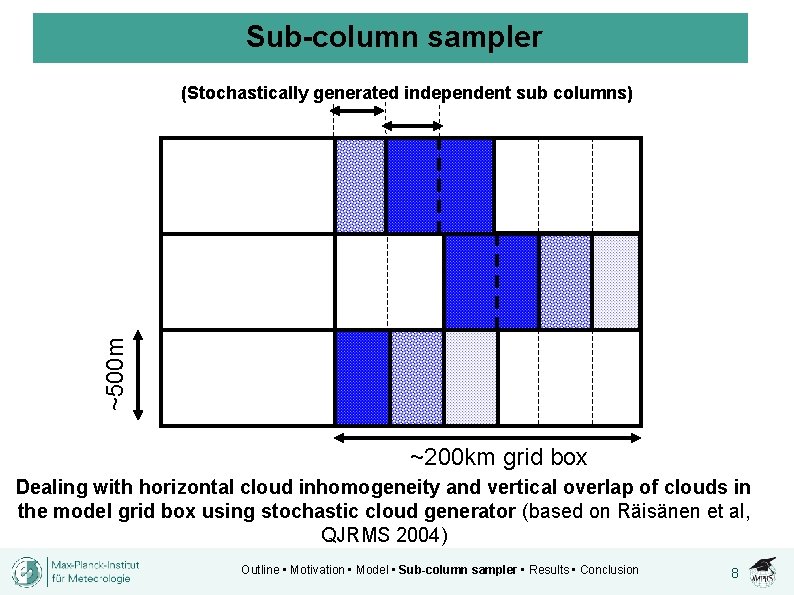
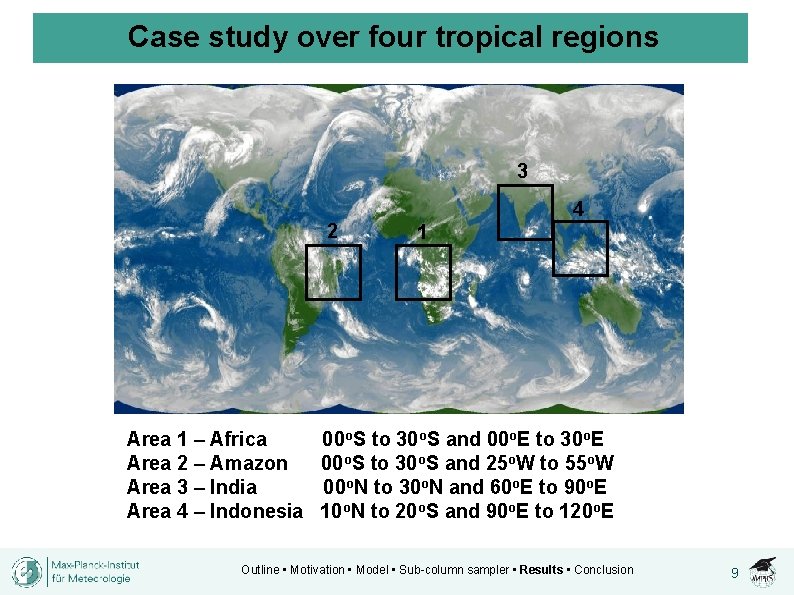
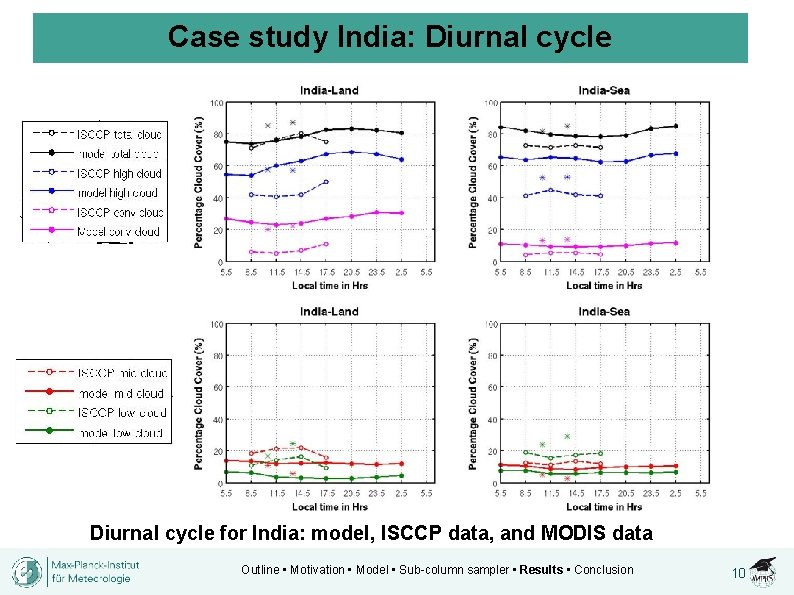
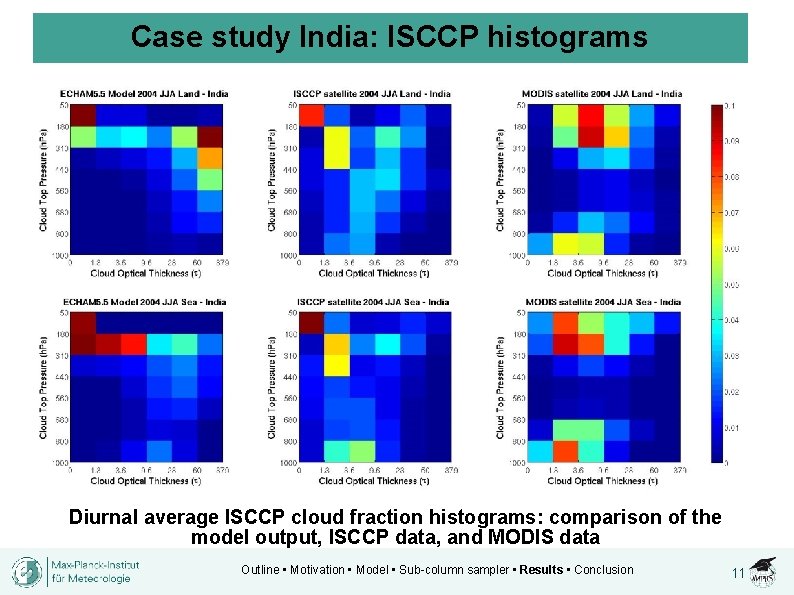
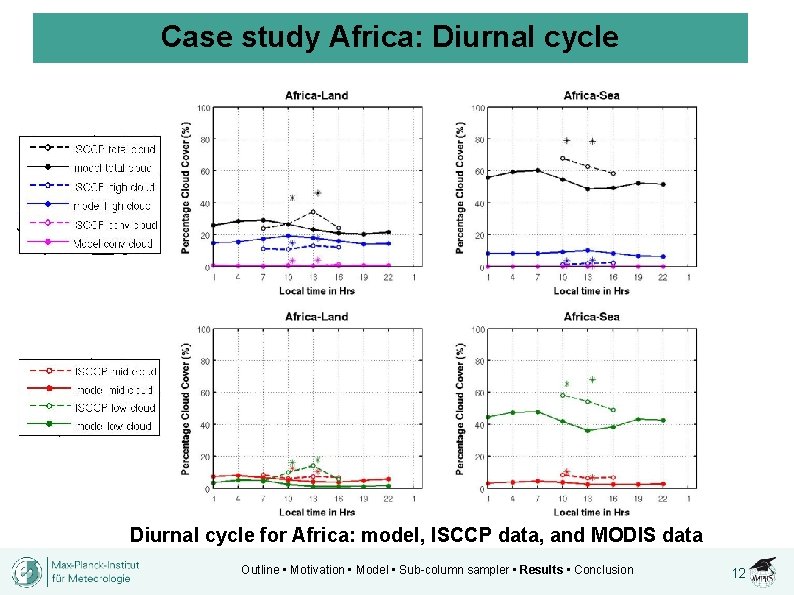
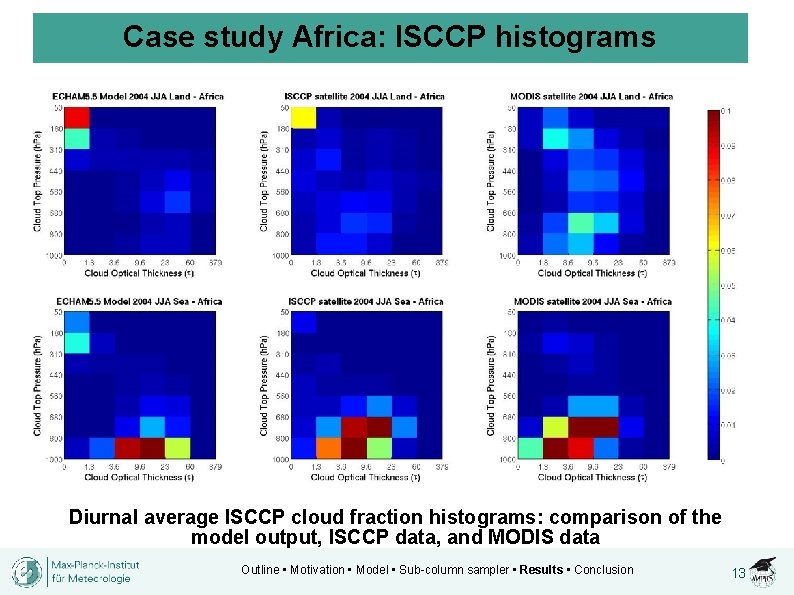
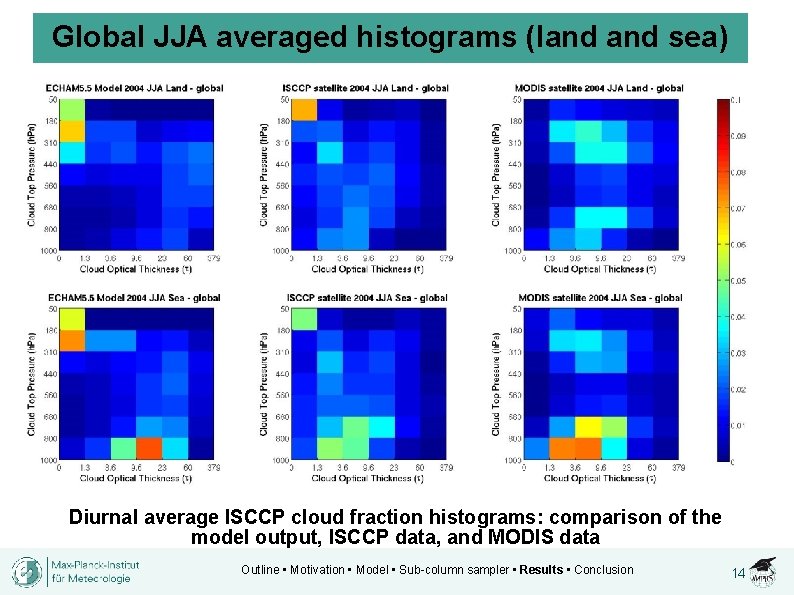
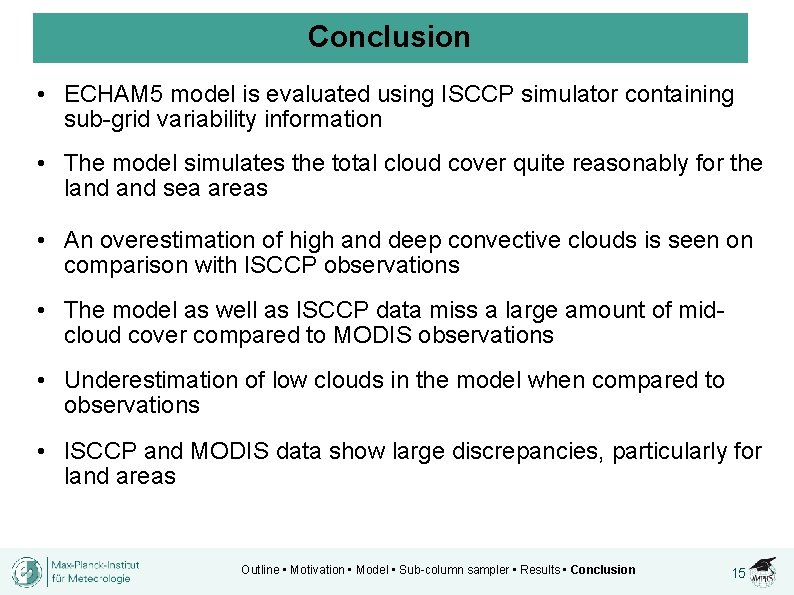
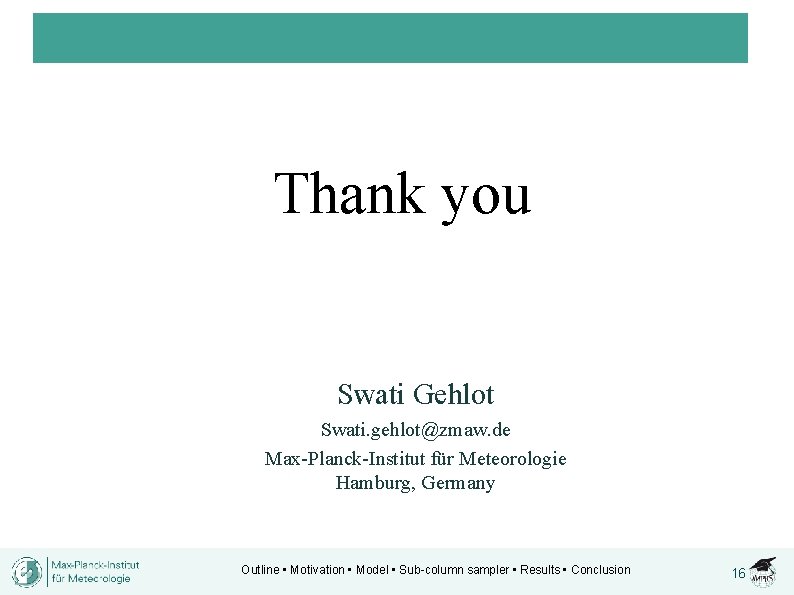
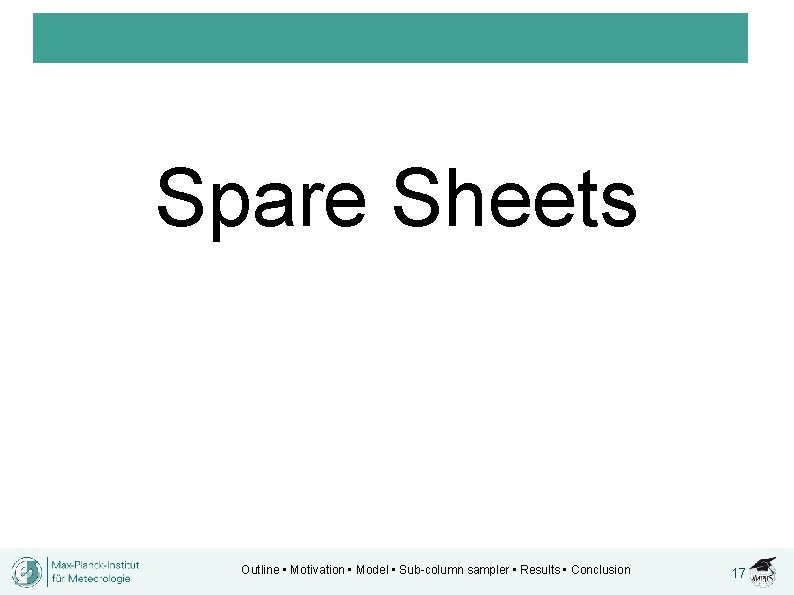
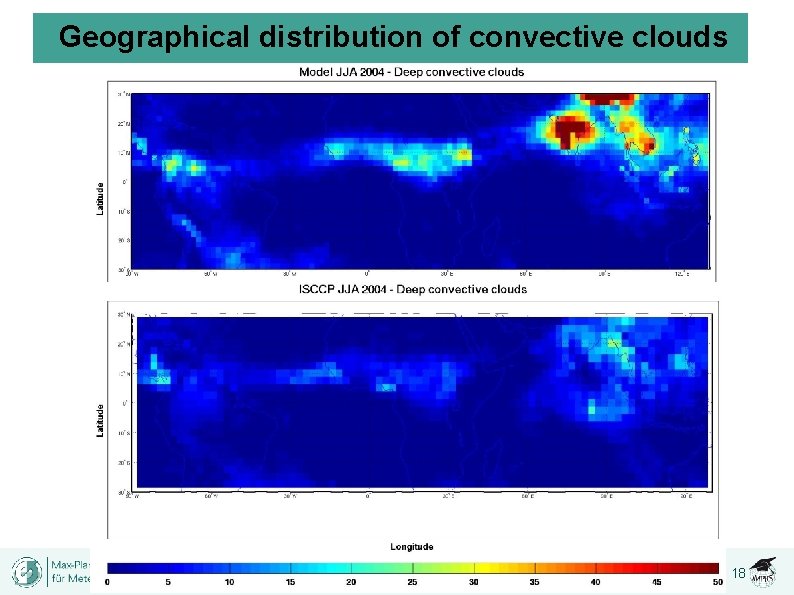
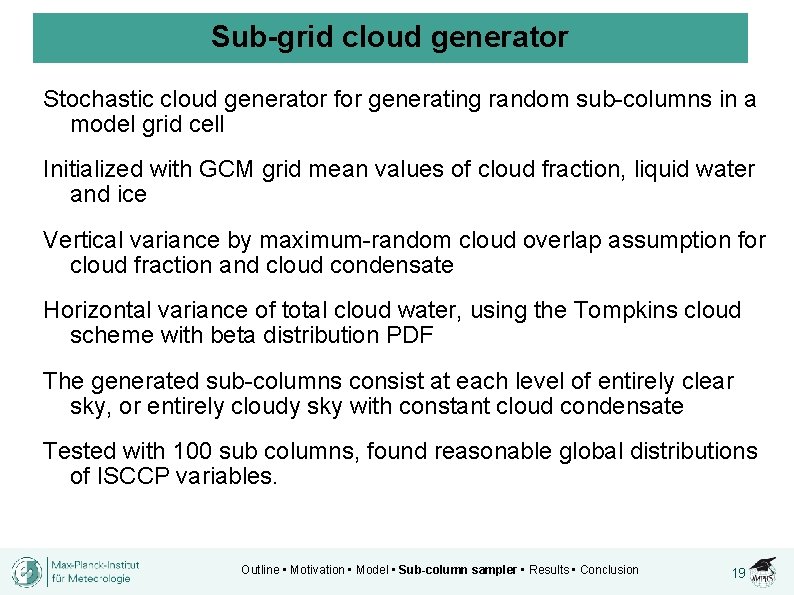
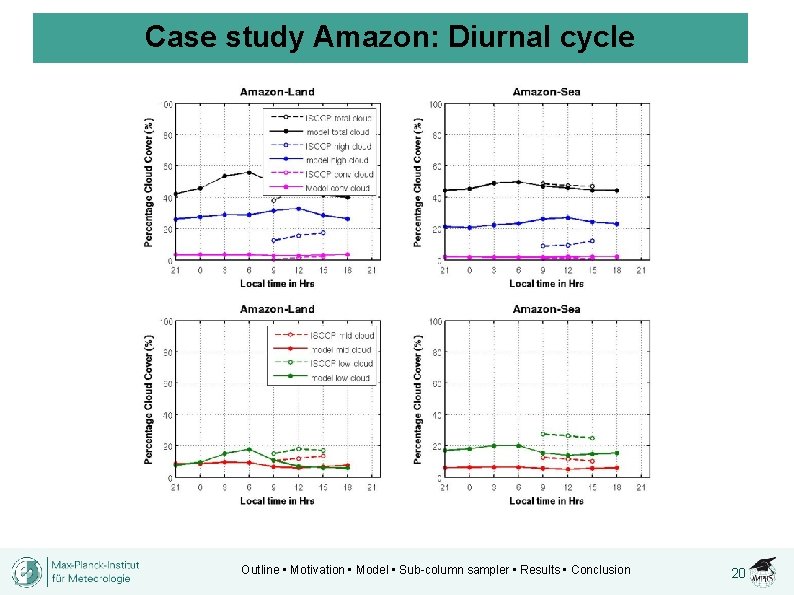
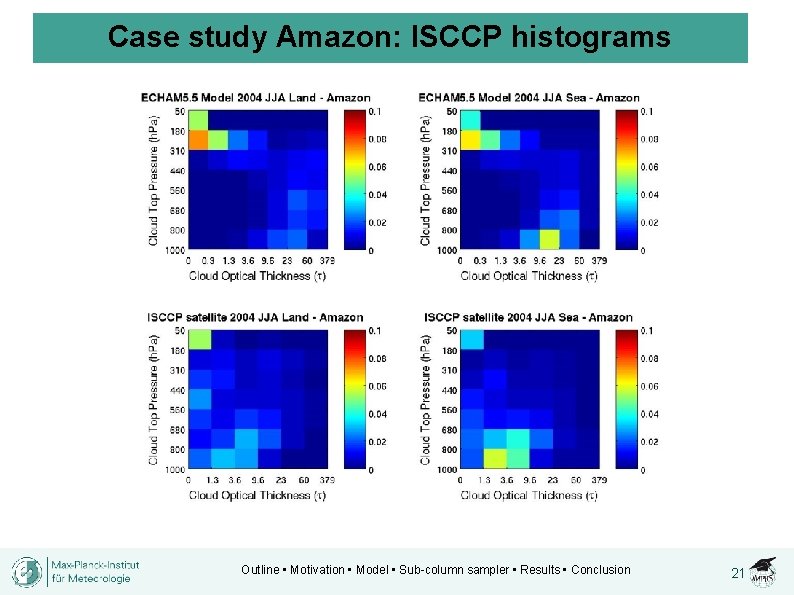
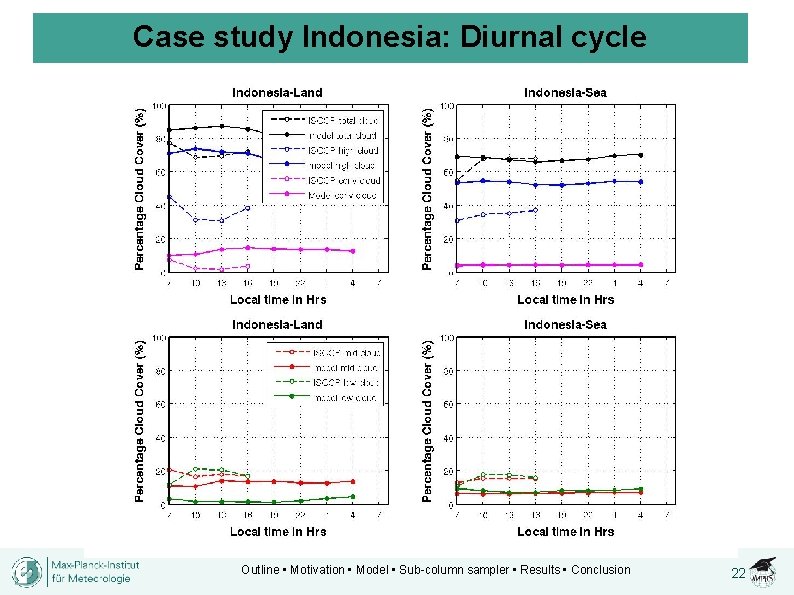
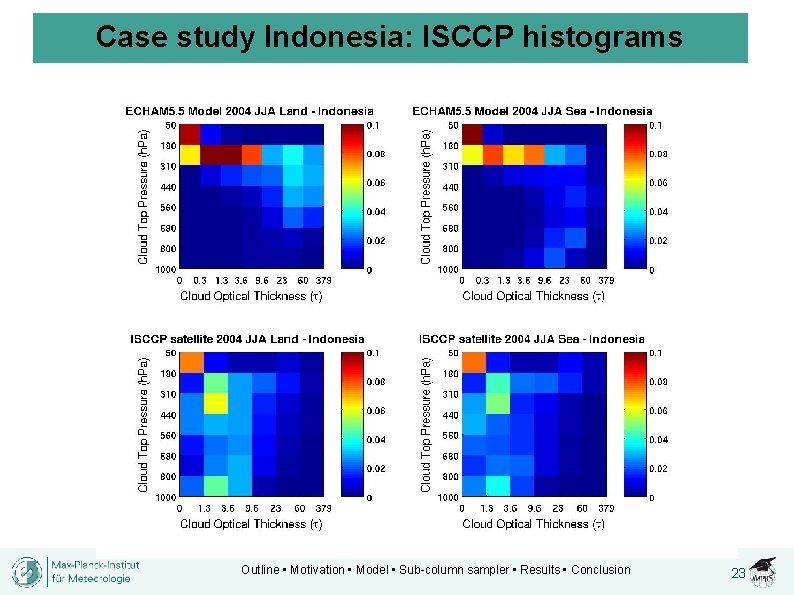
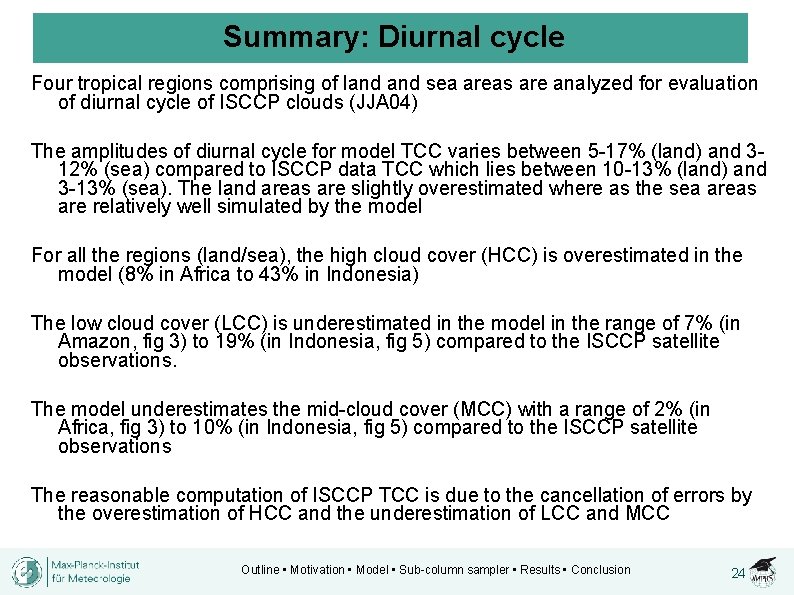
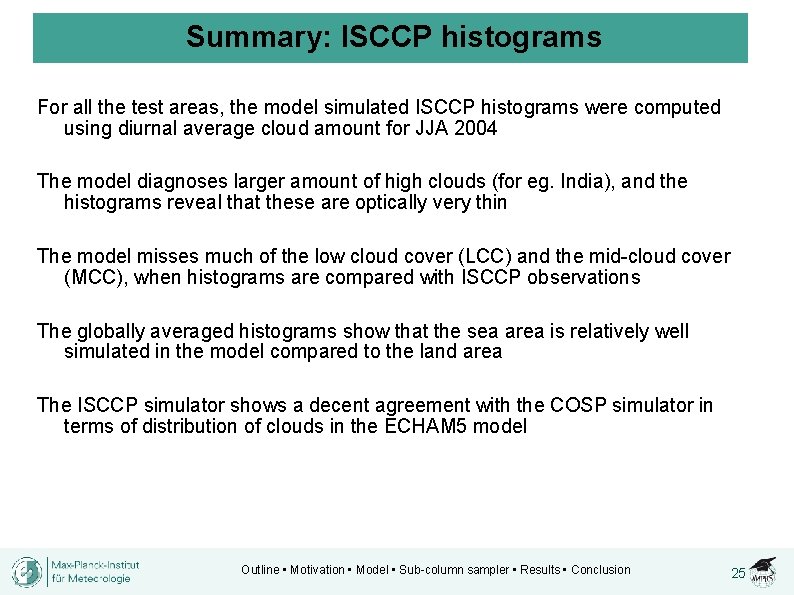
- Slides: 25
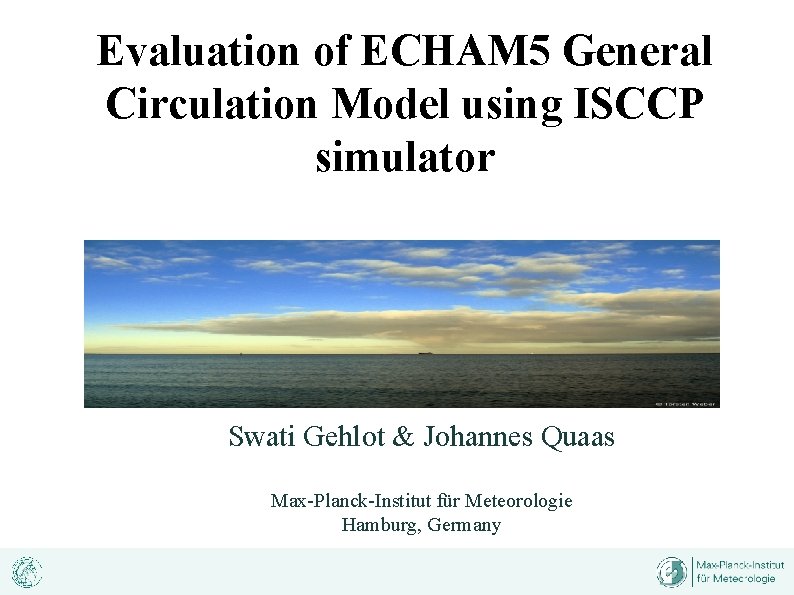
Evaluation of ECHAM 5 General Circulation Model using ISCCP simulator Swati Gehlot & Johannes Quaas Max-Planck-Institut für Meteorologie Hamburg, Germany
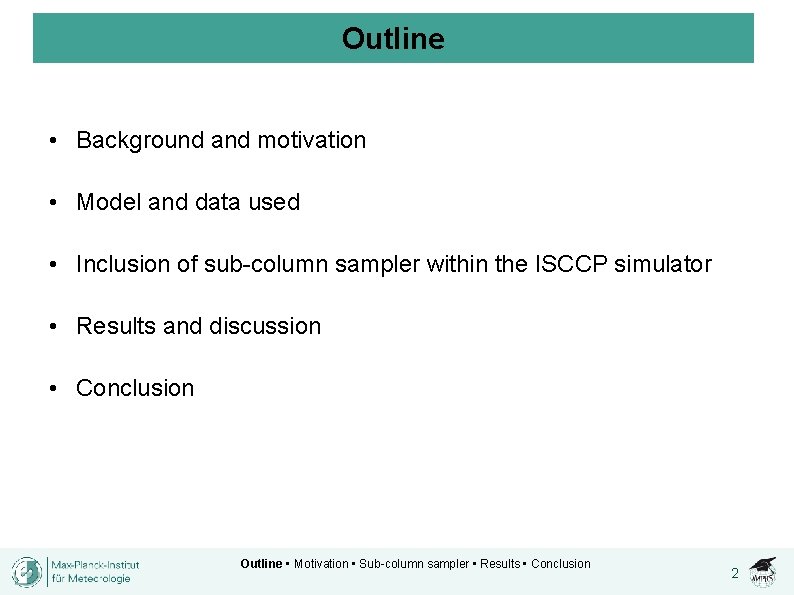
Outline • Background and motivation • Model and data used • Inclusion of sub-column sampler within the ISCCP simulator • Results and discussion • Conclusion Outline • Motivation • Sub-column sampler • Results • Conclusion 2
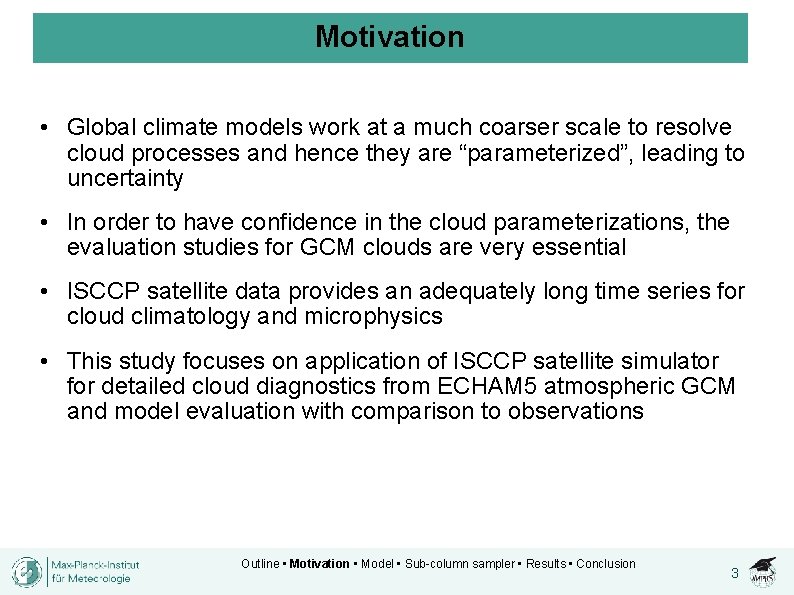
Motivation • Global climate models work at a much coarser scale to resolve cloud processes and hence they are “parameterized”, leading to uncertainty • In order to have confidence in the cloud parameterizations, the evaluation studies for GCM clouds are very essential • ISCCP satellite data provides an adequately long time series for cloud climatology and microphysics • This study focuses on application of ISCCP satellite simulator for detailed cloud diagnostics from ECHAM 5 atmospheric GCM and model evaluation with comparison to observations Outline • Motivation • Model • Sub-column sampler • Results • Conclusion 3
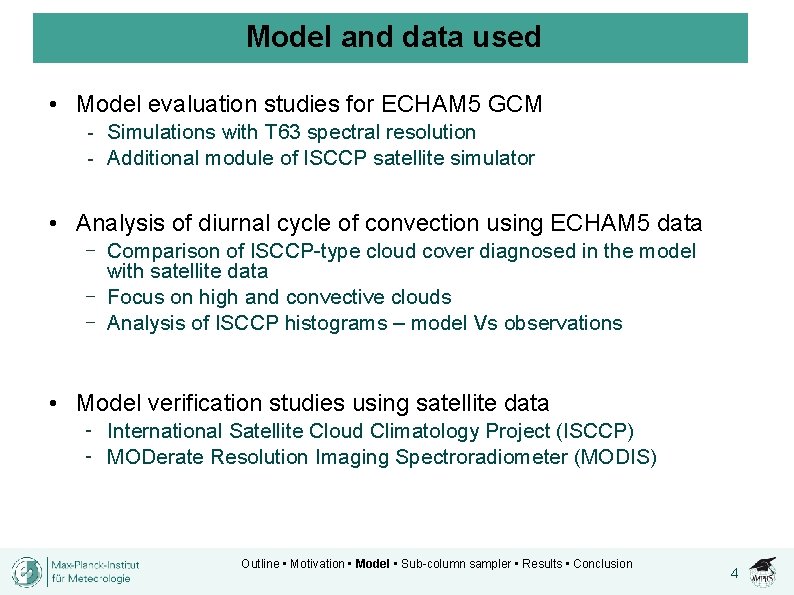
Model and data used • Model evaluation studies for ECHAM 5 GCM ‐ Simulations with T 63 spectral resolution ‐ Additional module of ISCCP satellite simulator • Analysis of diurnal cycle of convection using ECHAM 5 data - Comparison of ISCCP-type cloud cover diagnosed in the model with satellite data - Focus on high and convective clouds - Analysis of ISCCP histograms – model Vs observations • Model verification studies using satellite data ‑ International Satellite Cloud Climatology Project (ISCCP) ‑ MODerate Resolution Imaging Spectroradiometer (MODIS) Outline • Motivation • Model • Sub-column sampler • Results • Conclusion 4
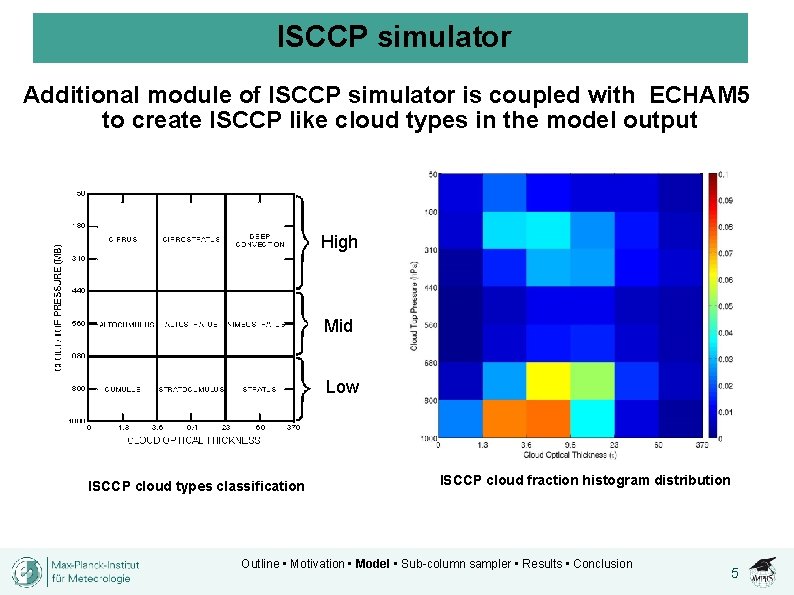
ISCCP simulator Additional module of ISCCP simulator is coupled with ECHAM 5 to create ISCCP like cloud types in the model output High Mid Low ISCCP cloud types classification ISCCP cloud fraction histogram distribution Outline • Motivation • Model • Sub-column sampler • Results • Conclusion 5
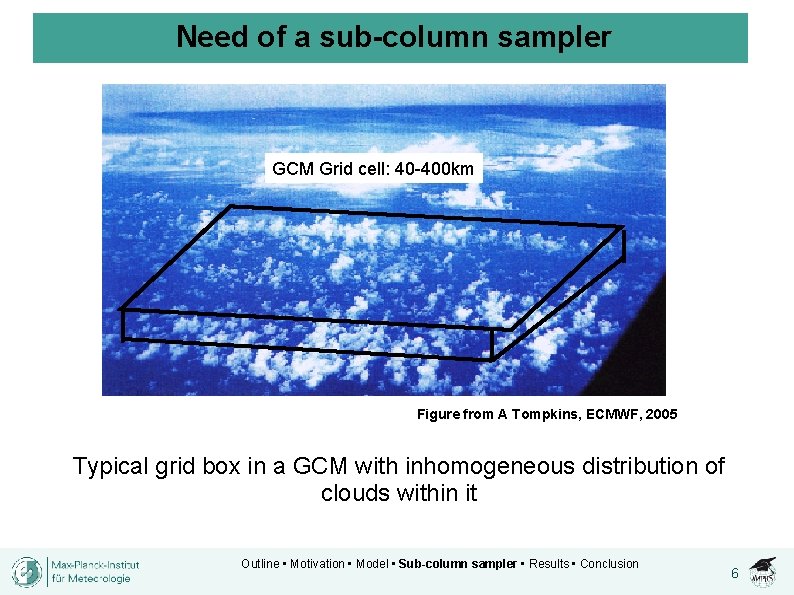
Need of a sub-column sampler GCM Grid cell: 40 -400 km Figure from A Tompkins, ECMWF, 2005 Typical grid box in a GCM with inhomogeneous distribution of clouds within it Outline • Motivation • Model • Sub-column sampler • Results • Conclusion 6
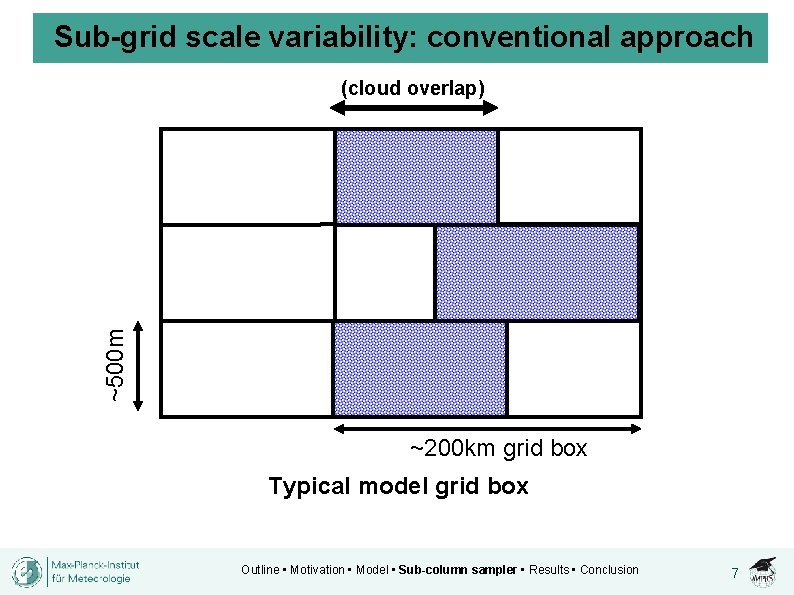
Sub-grid scale variability: conventional approach ~500 m (cloud overlap) ~200 km grid box Typical model grid box Outline • Motivation • Model • Sub-column sampler • Results • Conclusion 7
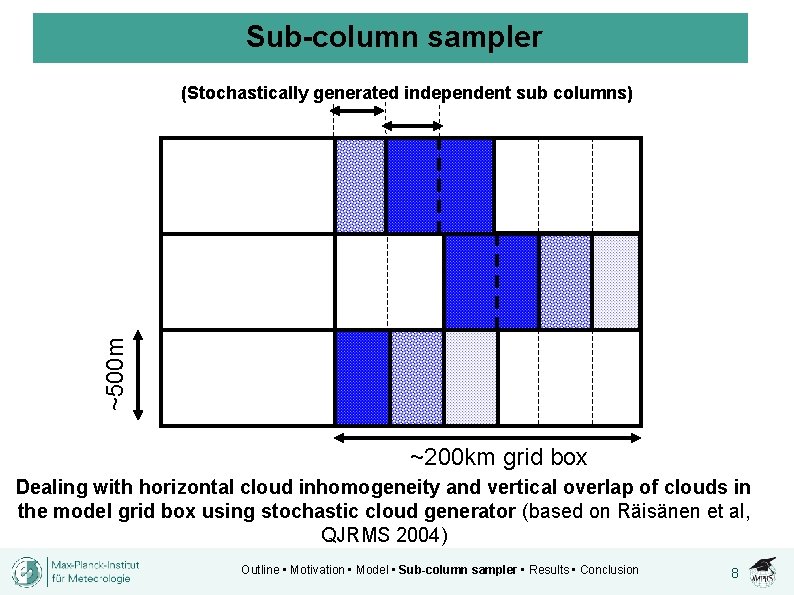
Sub-column sampler ~500 m (Stochastically generated independent sub columns) ~200 km grid box Dealing with horizontal cloud inhomogeneity and vertical overlap of clouds in the model grid box using stochastic cloud generator (based on Räisänen et al, QJRMS 2004) Outline • Motivation • Model • Sub-column sampler • Results • Conclusion 8
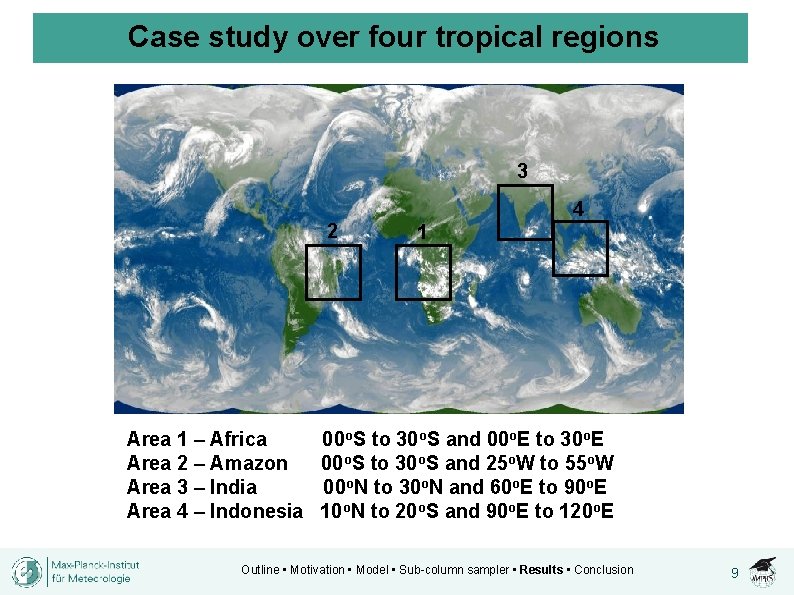
Case study over four tropical regions 3 2 Area 1 – Africa Area 2 – Amazon Area 3 – India Area 4 – Indonesia 4 1 00 o. S to 30 o. S and 00 o. E to 30 o. E 00 o. S to 30 o. S and 25 o. W to 55 o. W 00 o. N to 30 o. N and 60 o. E to 90 o. E 10 o. N to 20 o. S and 90 o. E to 120 o. E Outline • Motivation • Model • Sub-column sampler • Results • Conclusion 9
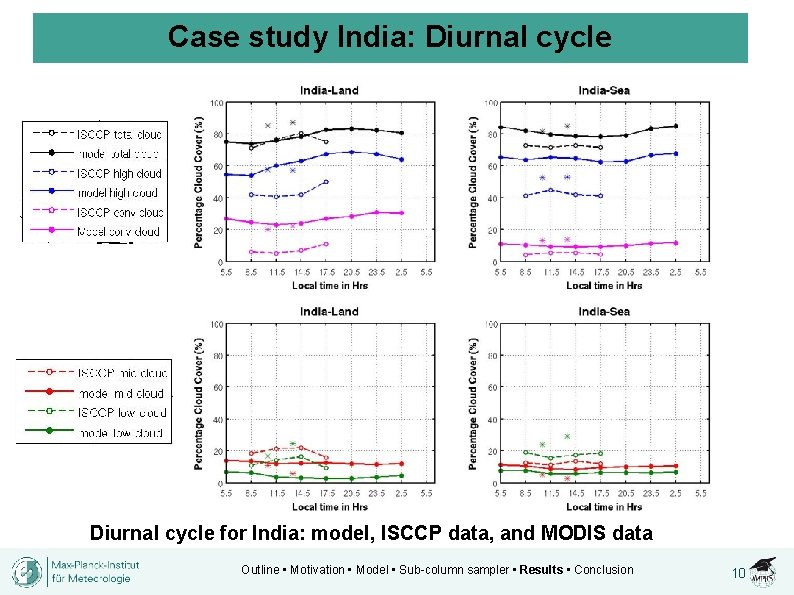
Case study India: Diurnal cycle for India: model, ISCCP data, and MODIS data Outline • Motivation • Model • Sub-column sampler • Results • Conclusion 10
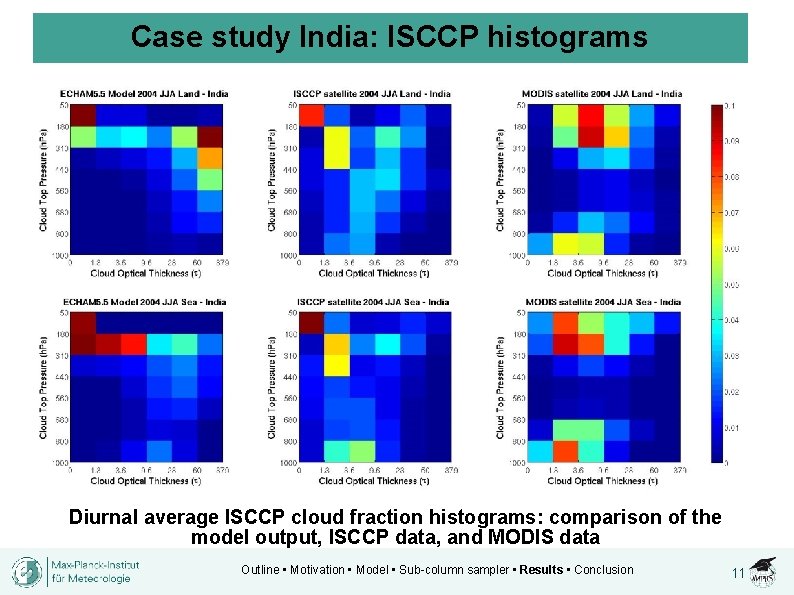
Case study India: ISCCP histograms Diurnal average ISCCP cloud fraction histograms: comparison of the model output, ISCCP data, and MODIS data Outline • Motivation • Model • Sub-column sampler • Results • Conclusion 11
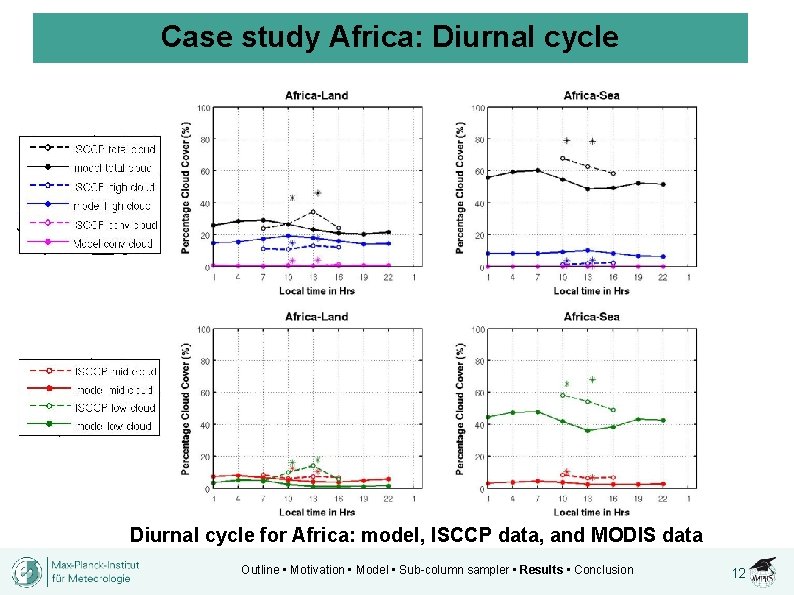
Case study Africa: Diurnal cycle for Africa: model, ISCCP data, and MODIS data Outline • Motivation • Model • Sub-column sampler • Results • Conclusion 12
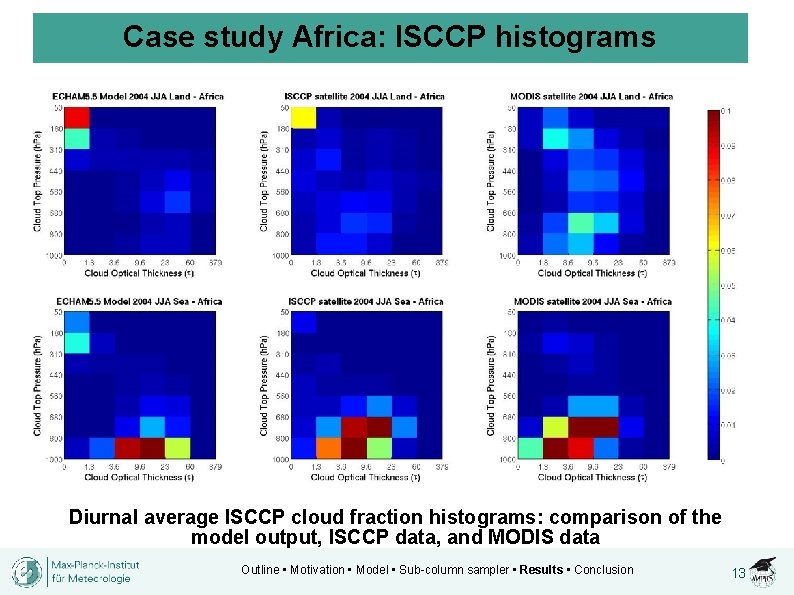
Case study Africa: ISCCP histograms Diurnal average ISCCP cloud fraction histograms: comparison of the model output, ISCCP data, and MODIS data Outline • Motivation • Model • Sub-column sampler • Results • Conclusion 13
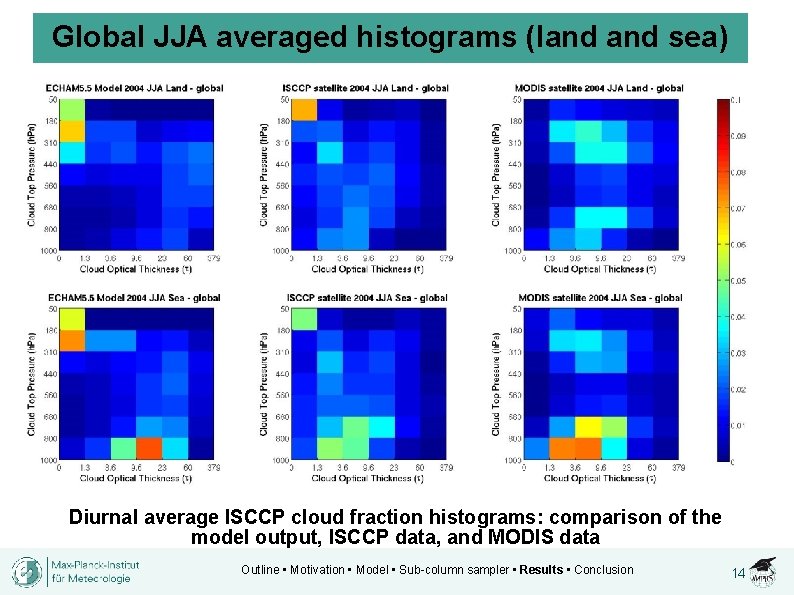
Global JJA averaged histograms (land sea) Diurnal average ISCCP cloud fraction histograms: comparison of the model output, ISCCP data, and MODIS data Outline • Motivation • Model • Sub-column sampler • Results • Conclusion 14
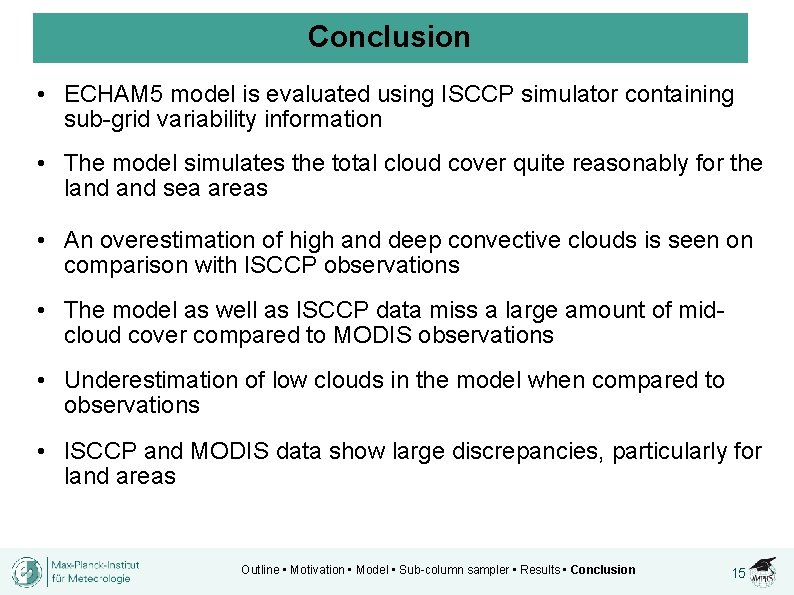
Conclusion • ECHAM 5 model is evaluated using ISCCP simulator containing sub-grid variability information • The model simulates the total cloud cover quite reasonably for the land sea areas • An overestimation of high and deep convective clouds is seen on comparison with ISCCP observations • The model as well as ISCCP data miss a large amount of midcloud cover compared to MODIS observations • Underestimation of low clouds in the model when compared to observations • ISCCP and MODIS data show large discrepancies, particularly for land areas Outline • Motivation • Model • Sub-column sampler • Results • Conclusion 15
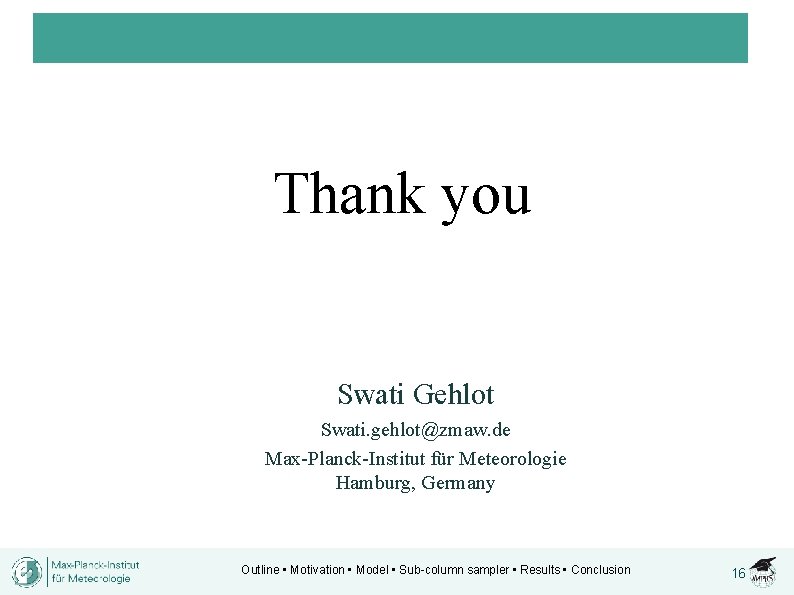
Thank you Swati Gehlot Swati. gehlot@zmaw. de Max-Planck-Institut für Meteorologie Hamburg, Germany Outline • Motivation • Model • Sub-column sampler • Results • Conclusion 16
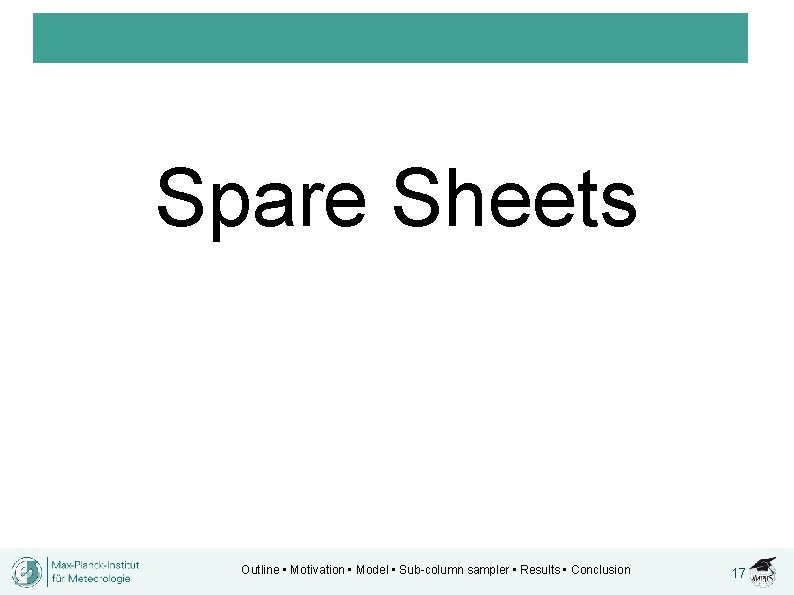
Spare Sheets Outline • Motivation • Model • Sub-column sampler • Results • Conclusion 17
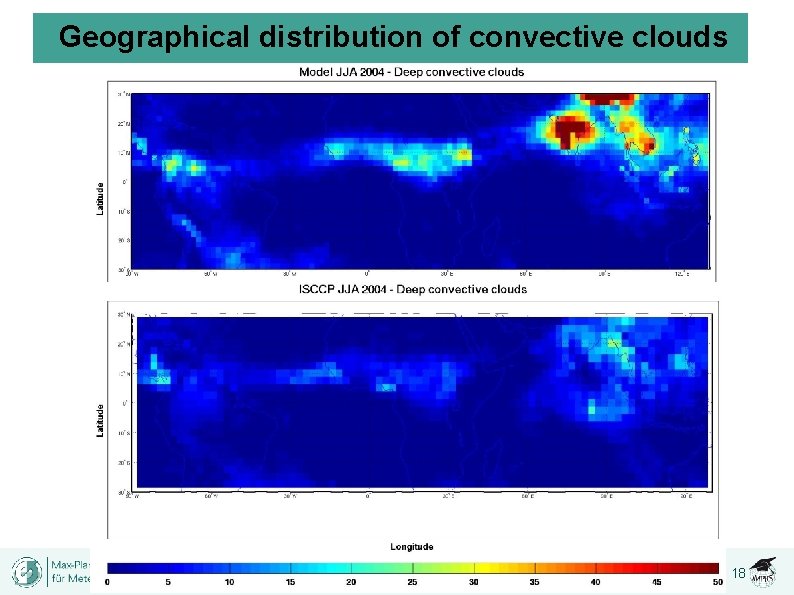
Geographical distribution of convective clouds Outline • Motivation • Model • Sub-column sampler • Results • Conclusion 18
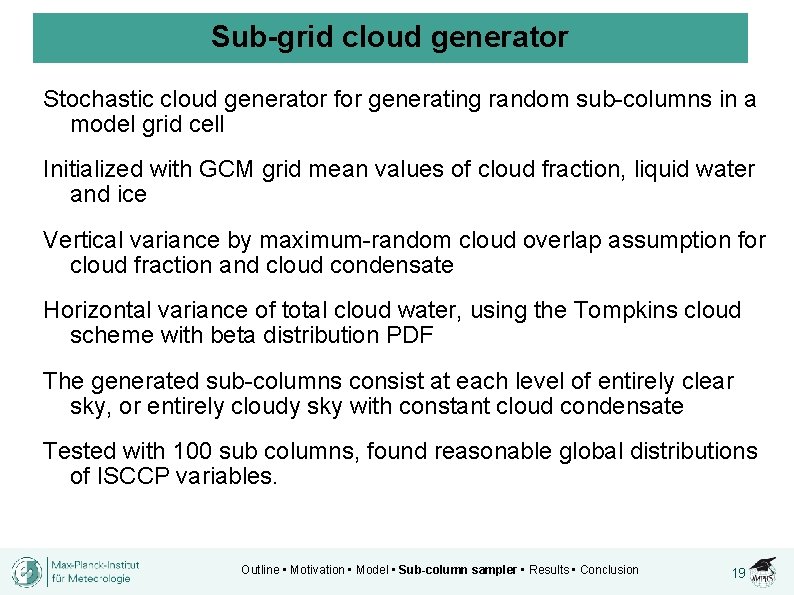
Sub-grid cloud generator Stochastic cloud generator for generating random sub-columns in a model grid cell Initialized with GCM grid mean values of cloud fraction, liquid water and ice Vertical variance by maximum-random cloud overlap assumption for cloud fraction and cloud condensate Horizontal variance of total cloud water, using the Tompkins cloud scheme with beta distribution PDF The generated sub-columns consist at each level of entirely clear sky, or entirely cloudy sky with constant cloud condensate Tested with 100 sub columns, found reasonable global distributions of ISCCP variables. Outline • Motivation • Model • Sub-column sampler • Results • Conclusion 19
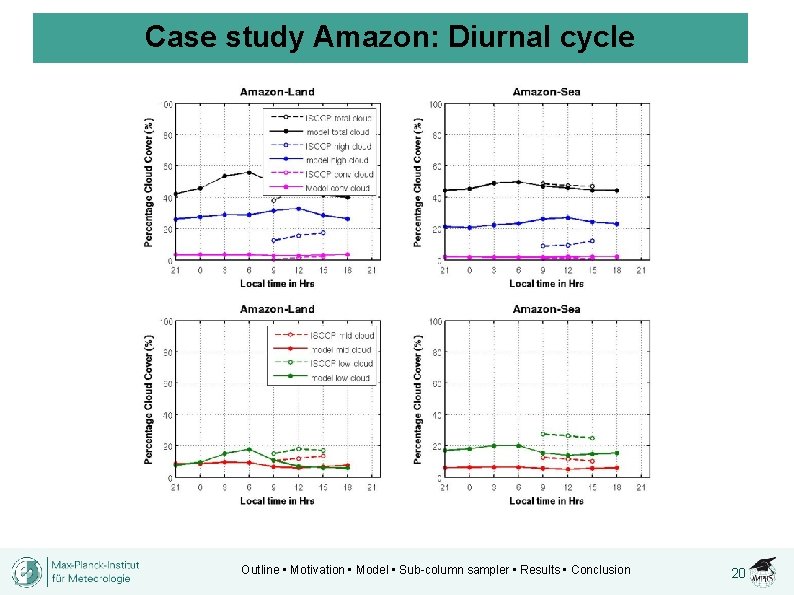
Case study Amazon: Diurnal cycle Outline • Motivation • Model • Sub-column sampler • Results • Conclusion 20
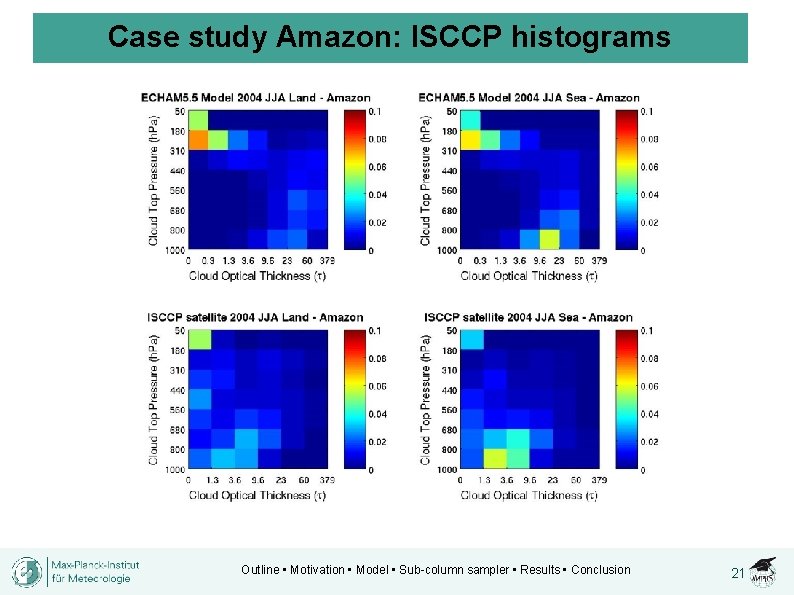
Case study Amazon: ISCCP histograms Outline • Motivation • Model • Sub-column sampler • Results • Conclusion 21
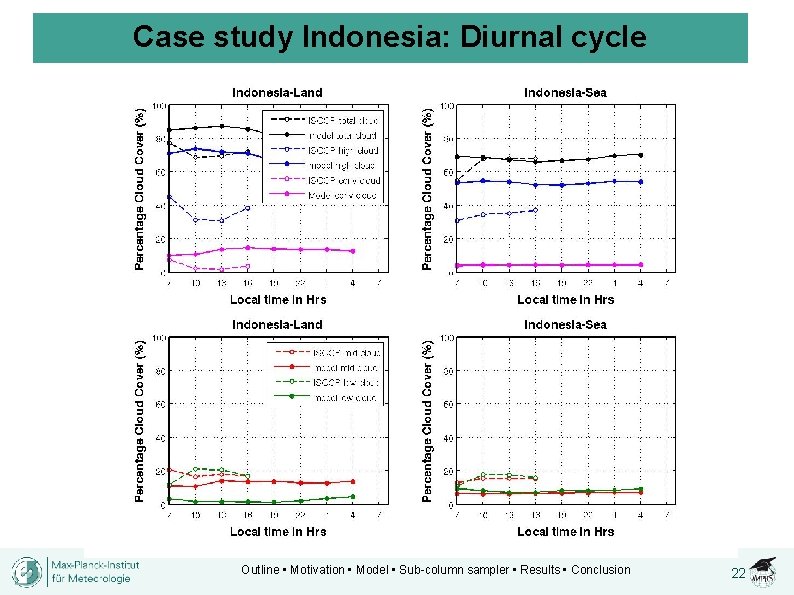
Case study Indonesia: Diurnal cycle Outline • Motivation • Model • Sub-column sampler • Results • Conclusion 22
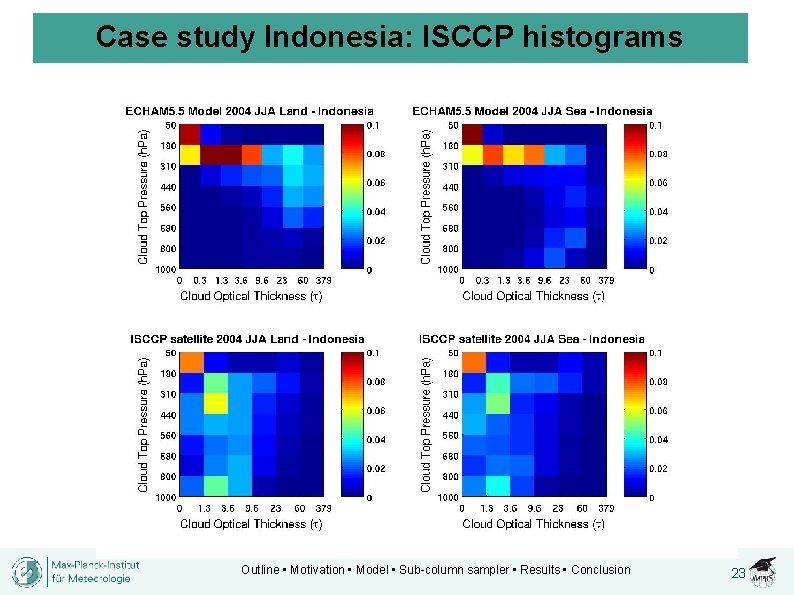
Case study Indonesia: ISCCP histograms Outline • Motivation • Model • Sub-column sampler • Results • Conclusion 23
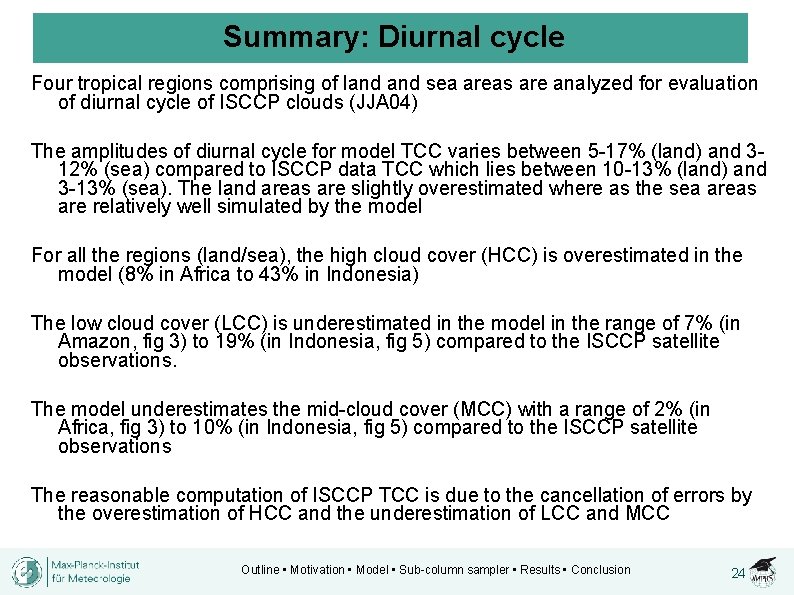
Summary: Diurnal cycle Four tropical regions comprising of land sea areas are analyzed for evaluation of diurnal cycle of ISCCP clouds (JJA 04) The amplitudes of diurnal cycle for model TCC varies between 5 -17% (land) and 312% (sea) compared to ISCCP data TCC which lies between 10 -13% (land) and 3 -13% (sea). The land areas are slightly overestimated where as the sea areas are relatively well simulated by the model For all the regions (land/sea), the high cloud cover (HCC) is overestimated in the model (8% in Africa to 43% in Indonesia) The low cloud cover (LCC) is underestimated in the model in the range of 7% (in Amazon, fig 3) to 19% (in Indonesia, fig 5) compared to the ISCCP satellite observations. The model underestimates the mid-cloud cover (MCC) with a range of 2% (in Africa, fig 3) to 10% (in Indonesia, fig 5) compared to the ISCCP satellite observations The reasonable computation of ISCCP TCC is due to the cancellation of errors by the overestimation of HCC and the underestimation of LCC and MCC Outline • Motivation • Model • Sub-column sampler • Results • Conclusion 24
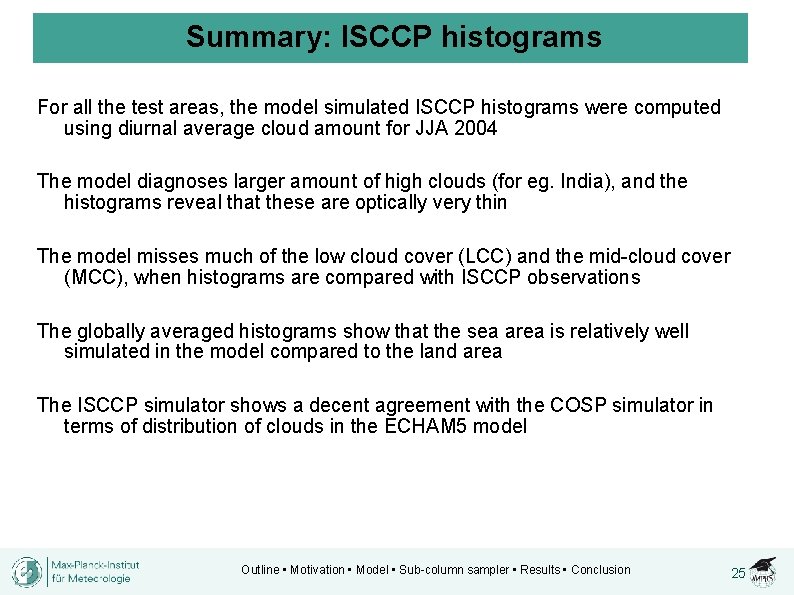
Summary: ISCCP histograms For all the test areas, the model simulated ISCCP histograms were computed using diurnal average cloud amount for JJA 2004 The model diagnoses larger amount of high clouds (for eg. India), and the histograms reveal that these are optically very thin The model misses much of the low cloud cover (LCC) and the mid-cloud cover (MCC), when histograms are compared with ISCCP observations The globally averaged histograms show that the sea area is relatively well simulated in the model compared to the land area The ISCCP simulator shows a decent agreement with the COSP simulator in terms of distribution of clouds in the ECHAM 5 model Outline • Motivation • Model • Sub-column sampler • Results • Conclusion 25
Single circulation and double circulation
Single circulation and double circulation
Structure of bronchiole
Single cell model of atmospheric circulation
Circulates air between 60-90 latitudes
Zonation and succession
Performance evaluation using variances from standard costs
Diferencia entre gran plano general y plano general
Where did general lee surrender to general grant?
Chapter 37 respiration circulation and excretion
Two circulation syndrome
Blood flow circuit
Blood
Large rotating air mass
Hepatic protal system
Rubaliser
Teeth of herbivorous animals
Intervening obstacle ap human geography
Types of vertical circulation
Hepatic acinus
Circulation extracorporelle
Secretion of bicarbonate ions
Flow chart of fetal circulation
To which sub phylum
Systemic circulation system
Estuary circulation