Evaluating the Use of Autonomous Recording Units for
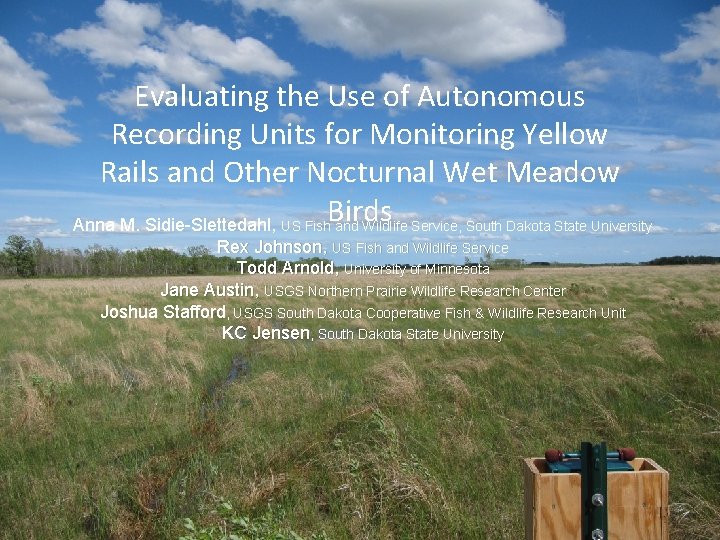
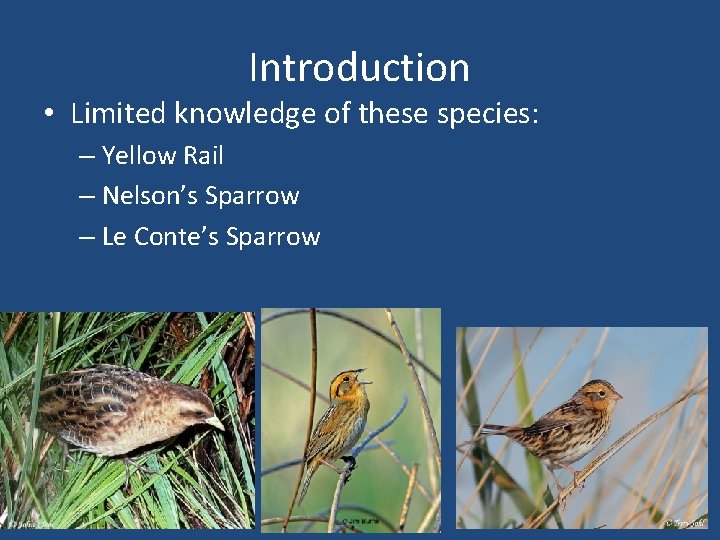
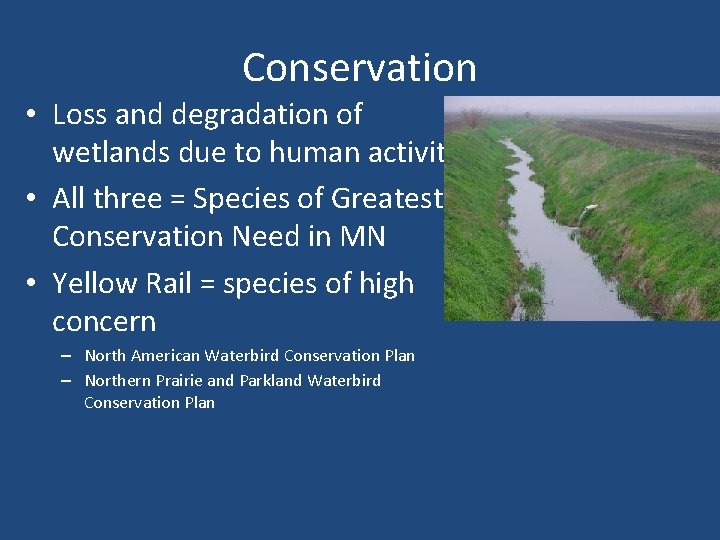
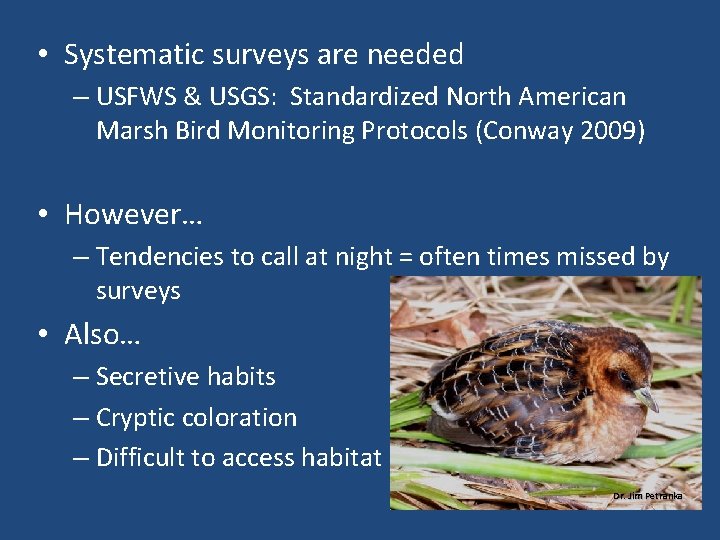
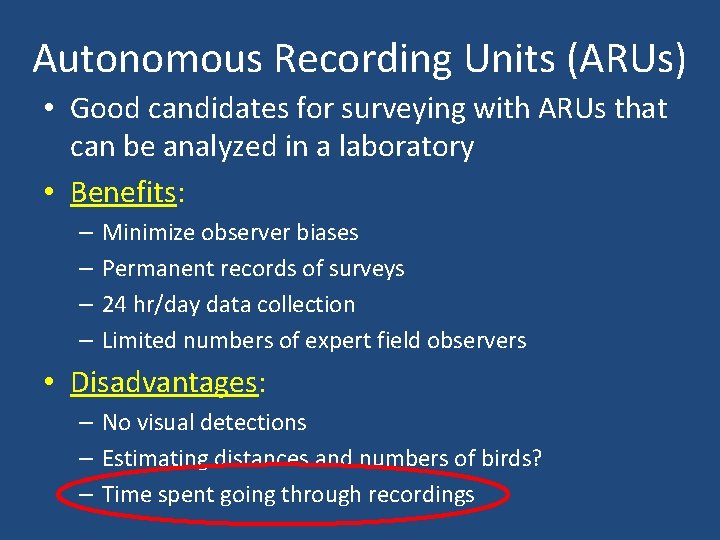
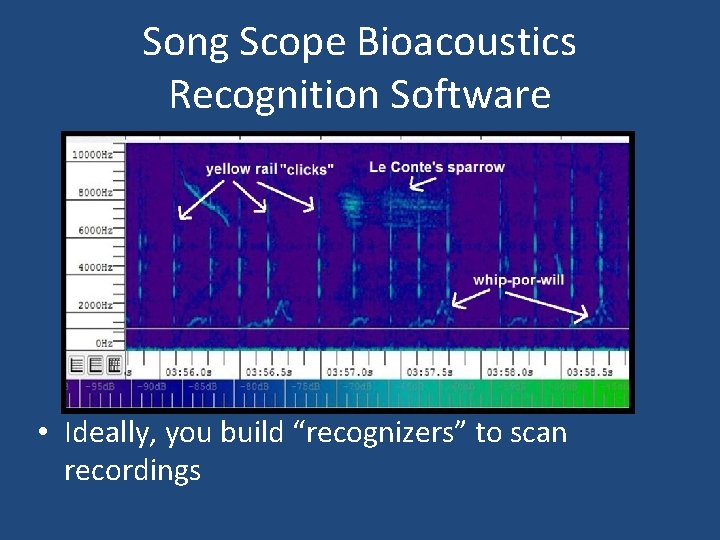
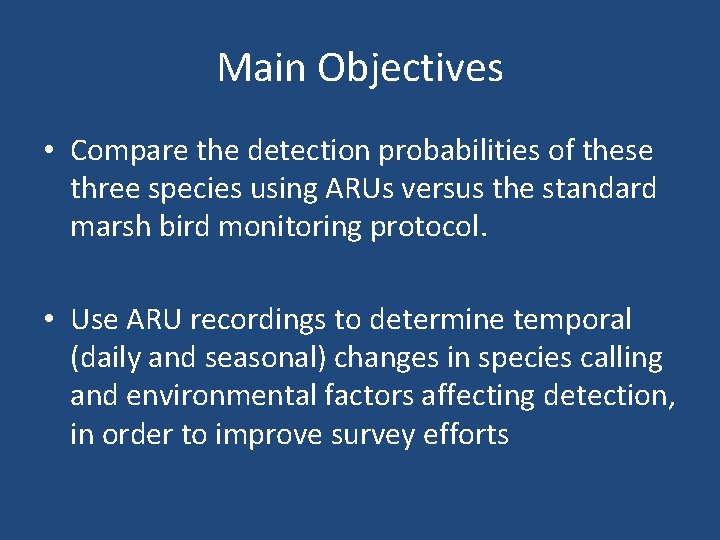
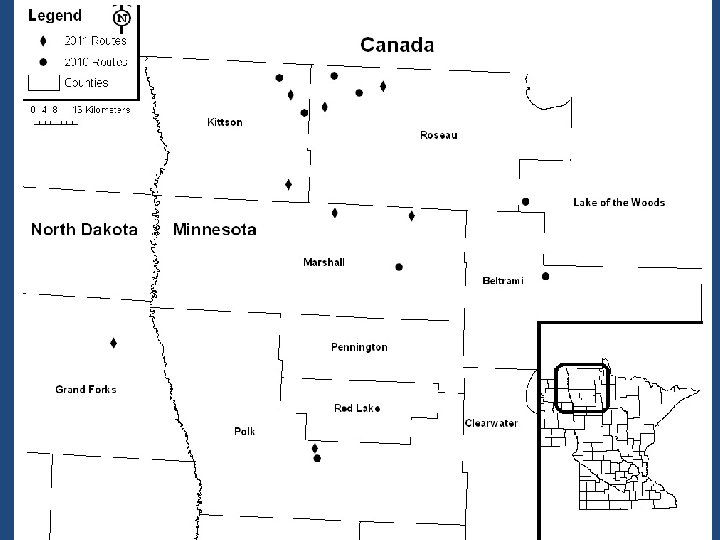
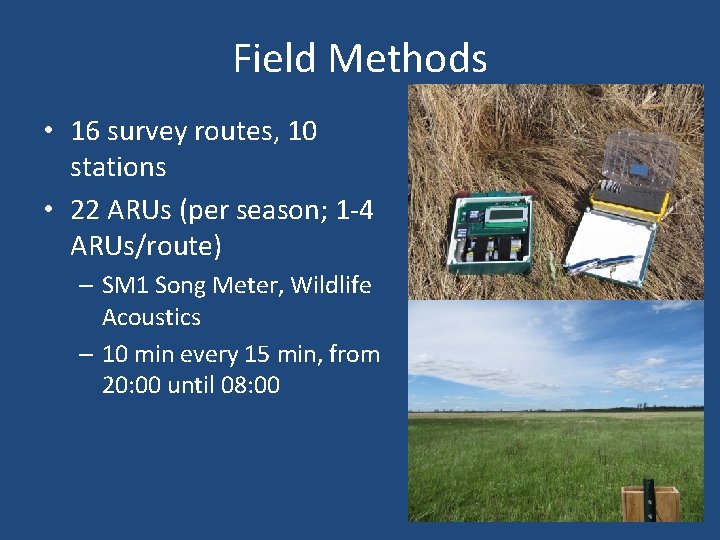
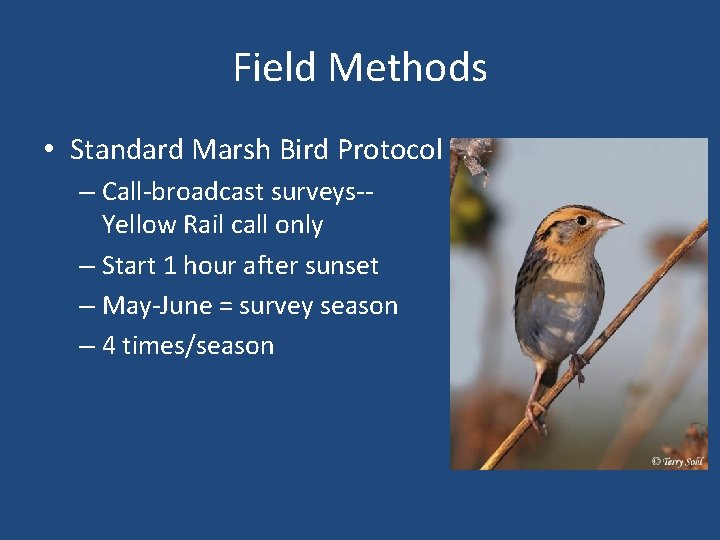
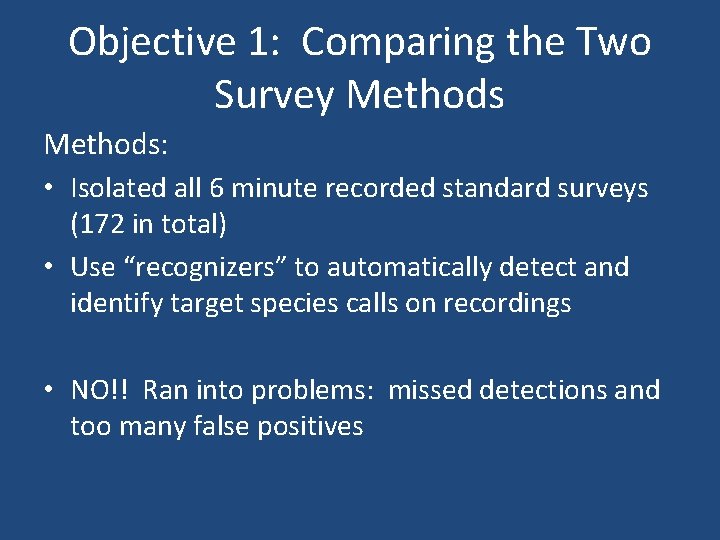
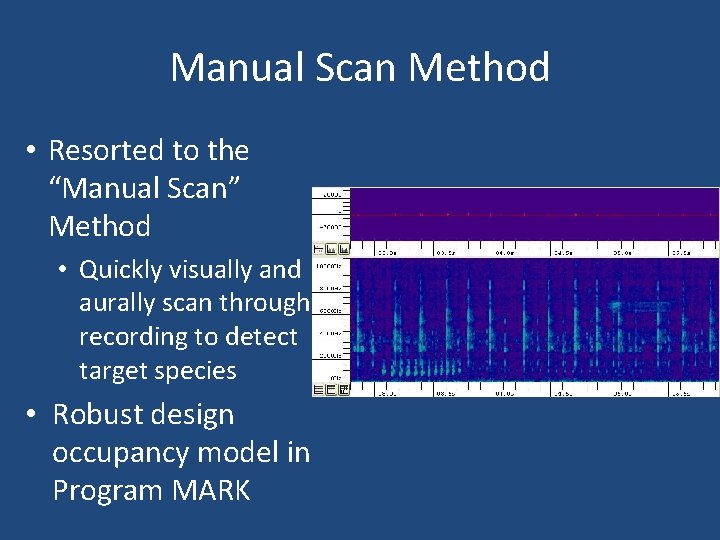
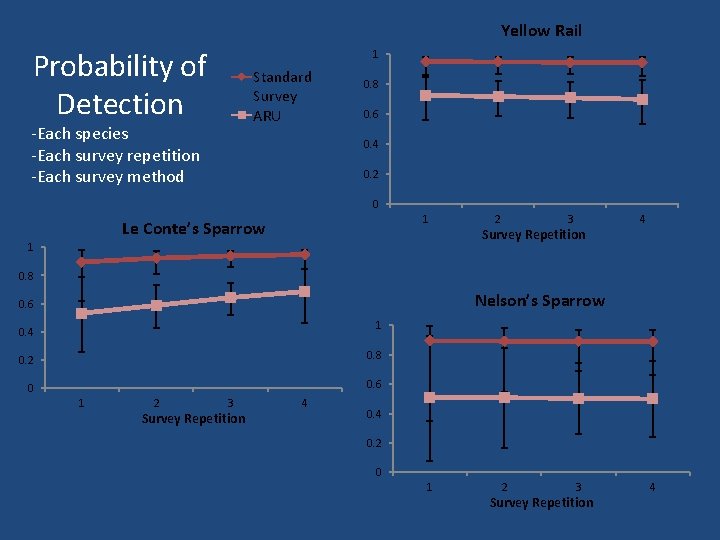
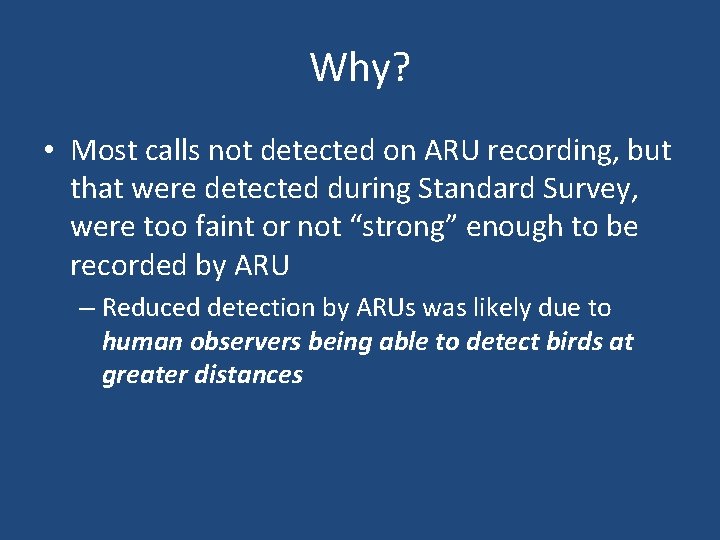
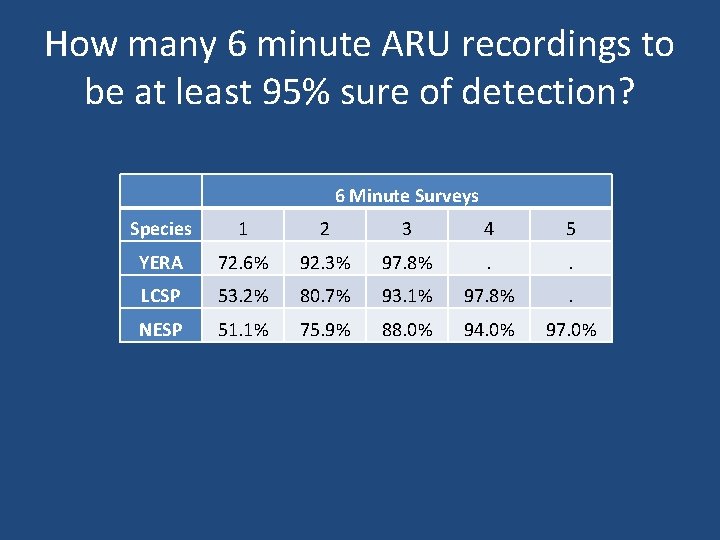
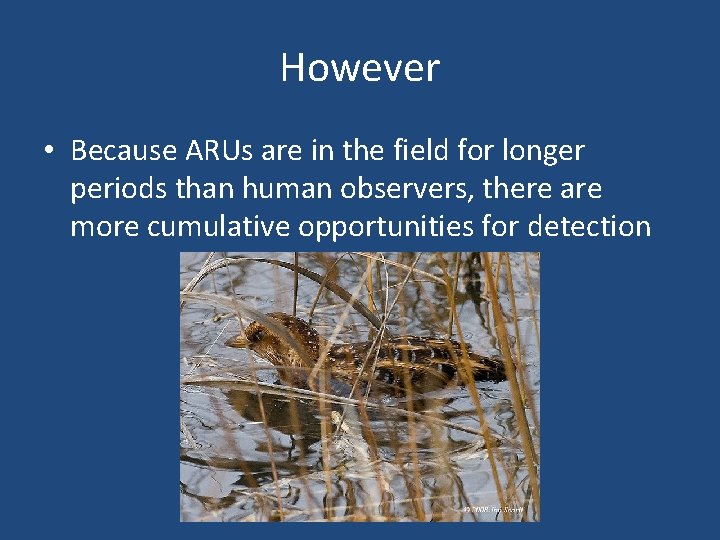
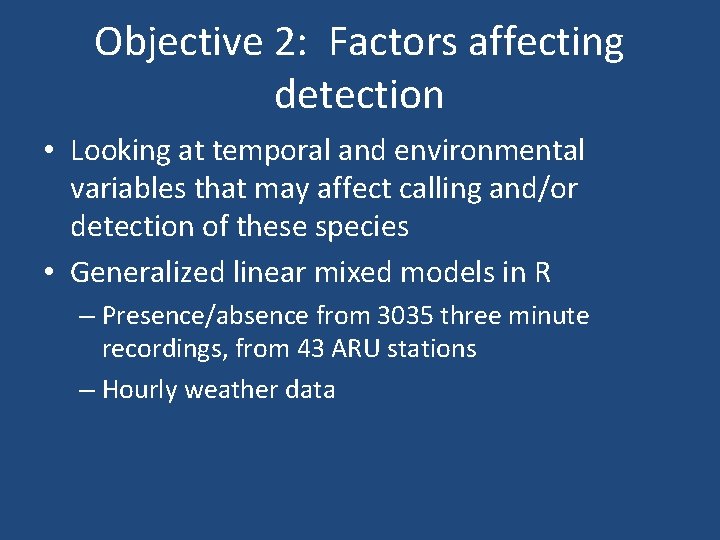
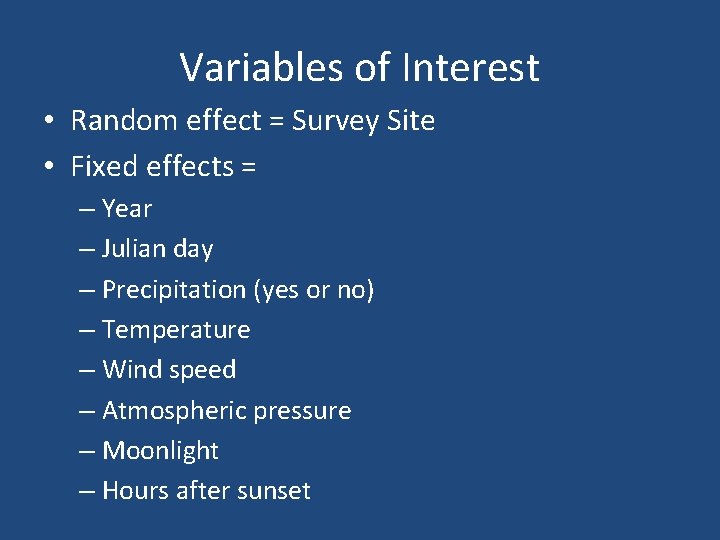
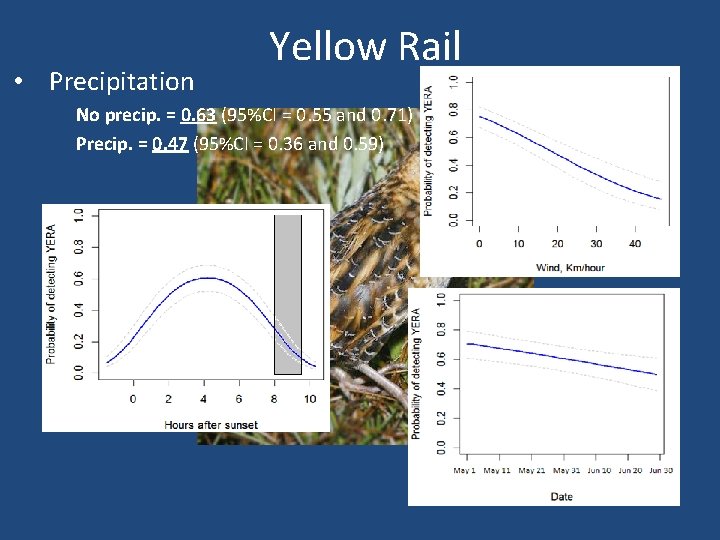
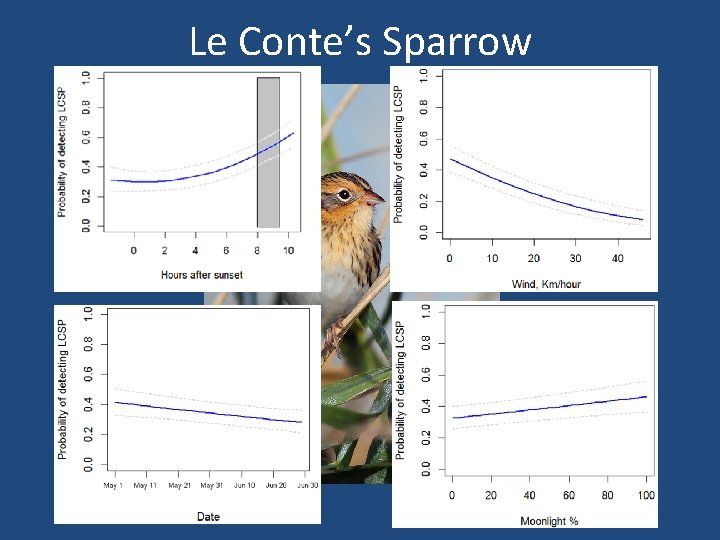
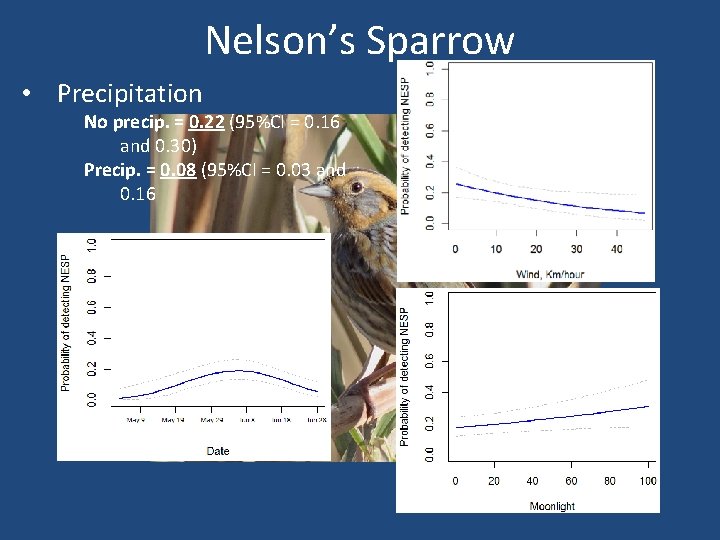
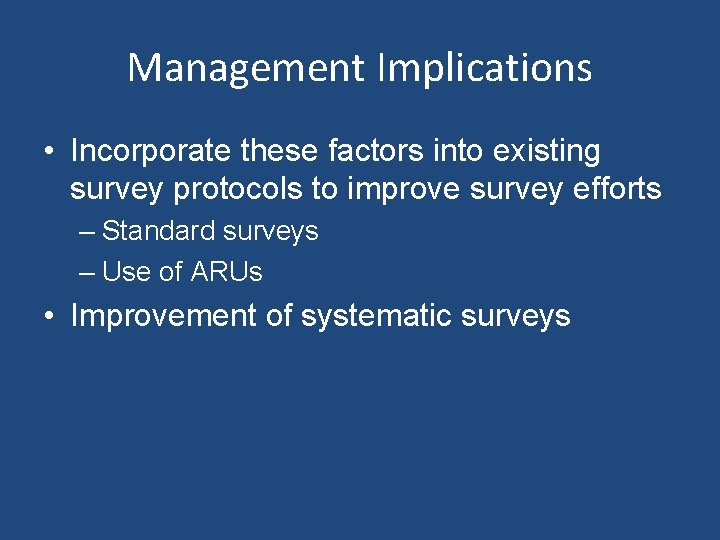
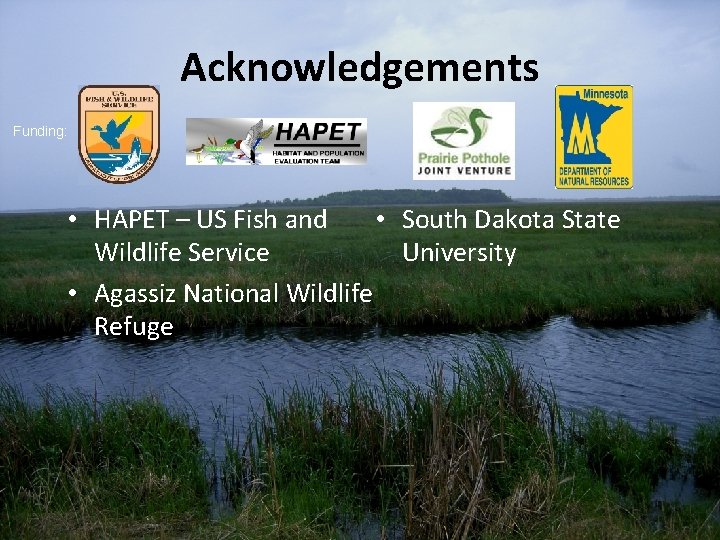
- Slides: 23
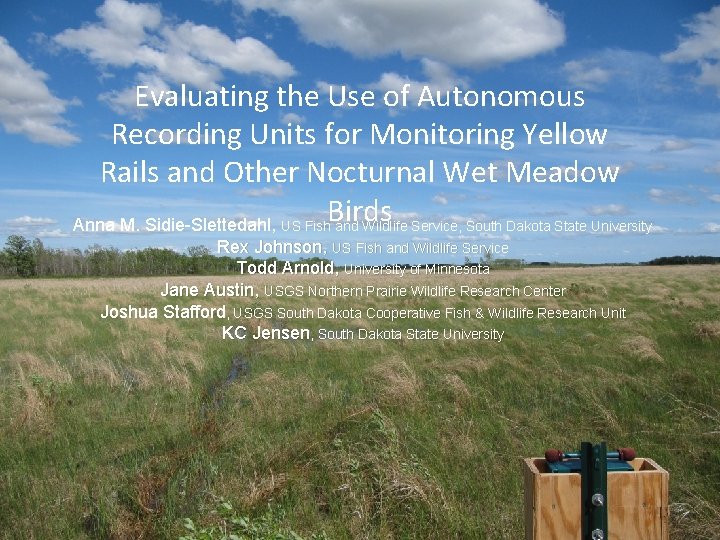
Evaluating the Use of Autonomous Recording Units for Monitoring Yellow Rails and Other Nocturnal Wet Meadow Birds Anna M. Sidie-Slettedahl, US Fish and Wildlife Service, South Dakota State University Rex Johnson, US Fish and Wildlife Service Todd Arnold, University of Minnesota Jane Austin, USGS Northern Prairie Wildlife Research Center Joshua Stafford, USGS South Dakota Cooperative Fish & Wildlife Research Unit KC Jensen, South Dakota State University
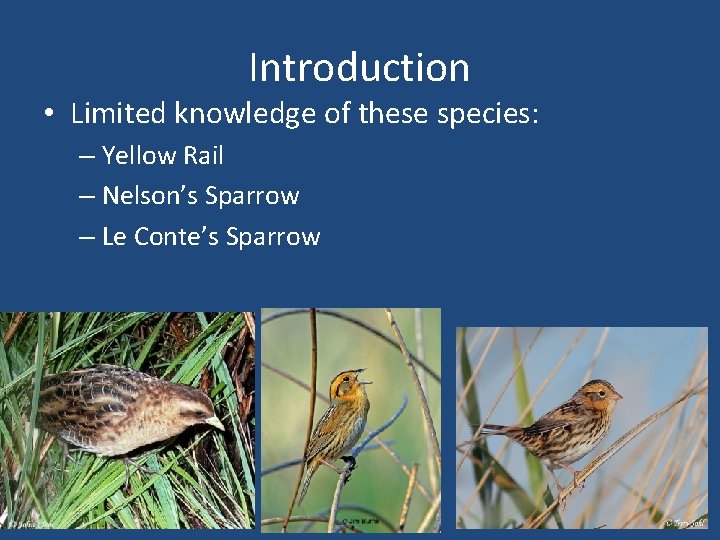
Introduction • Limited knowledge of these species: – Yellow Rail – Nelson’s Sparrow – Le Conte’s Sparrow
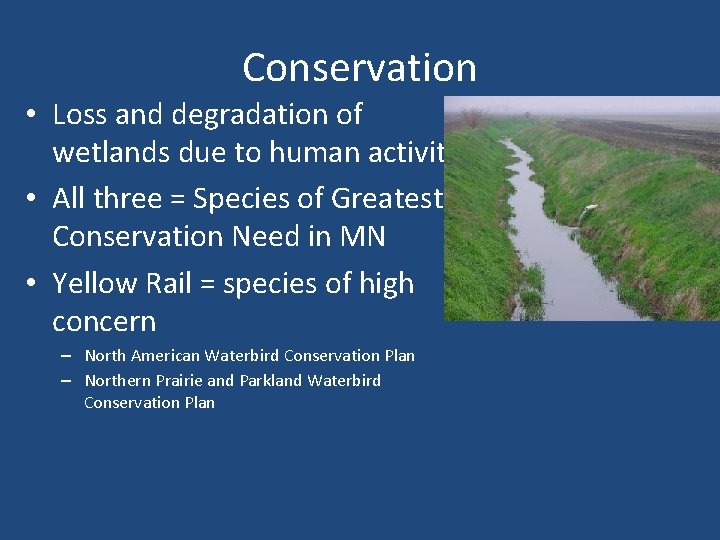
Conservation • Loss and degradation of wetlands due to human activity • All three = Species of Greatest Conservation Need in MN • Yellow Rail = species of high concern – North American Waterbird Conservation Plan – Northern Prairie and Parkland Waterbird Conservation Plan
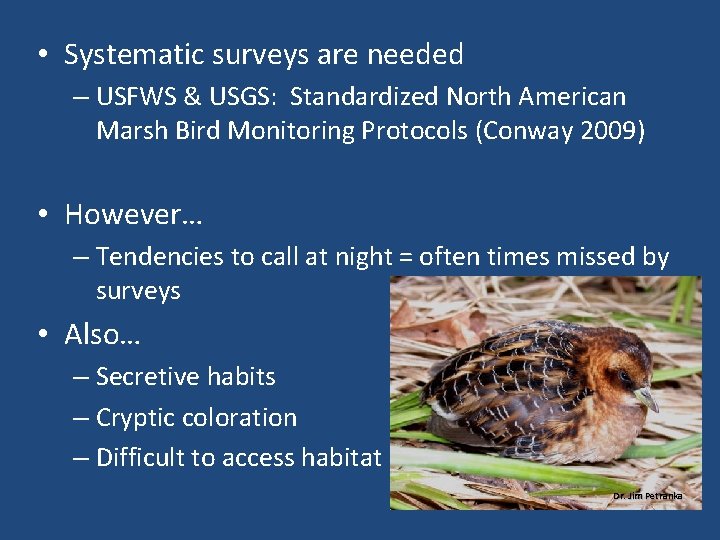
• Systematic surveys are needed – USFWS & USGS: Standardized North American Marsh Bird Monitoring Protocols (Conway 2009) • However… – Tendencies to call at night = often times missed by surveys • Also… – Secretive habits – Cryptic coloration – Difficult to access habitat Dr. Jim Petranka
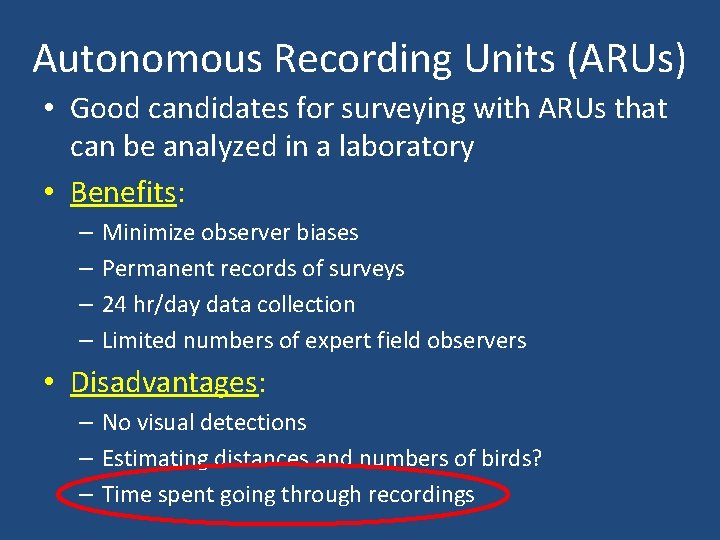
Autonomous Recording Units (ARUs) • Good candidates for surveying with ARUs that can be analyzed in a laboratory • Benefits: – – Minimize observer biases Permanent records of surveys 24 hr/day data collection Limited numbers of expert field observers • Disadvantages: – No visual detections – Estimating distances and numbers of birds? – Time spent going through recordings
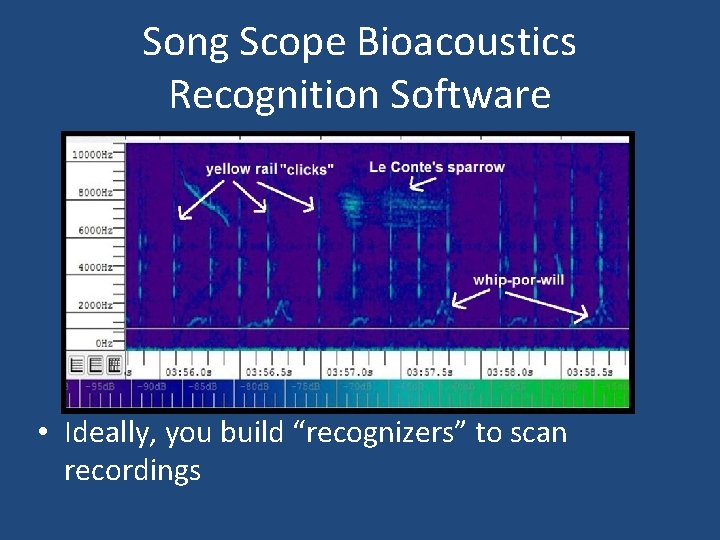
Song Scope Bioacoustics Recognition Software • Ideally, you build “recognizers” to scan recordings
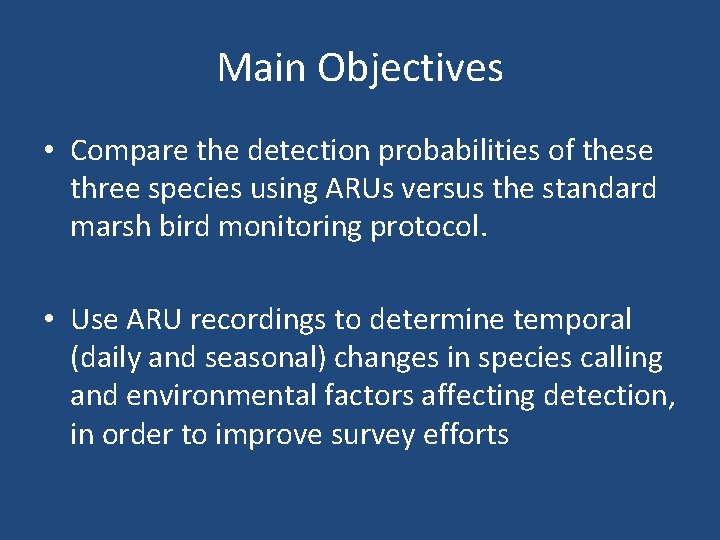
Main Objectives • Compare the detection probabilities of these three species using ARUs versus the standard marsh bird monitoring protocol. • Use ARU recordings to determine temporal (daily and seasonal) changes in species calling and environmental factors affecting detection, in order to improve survey efforts
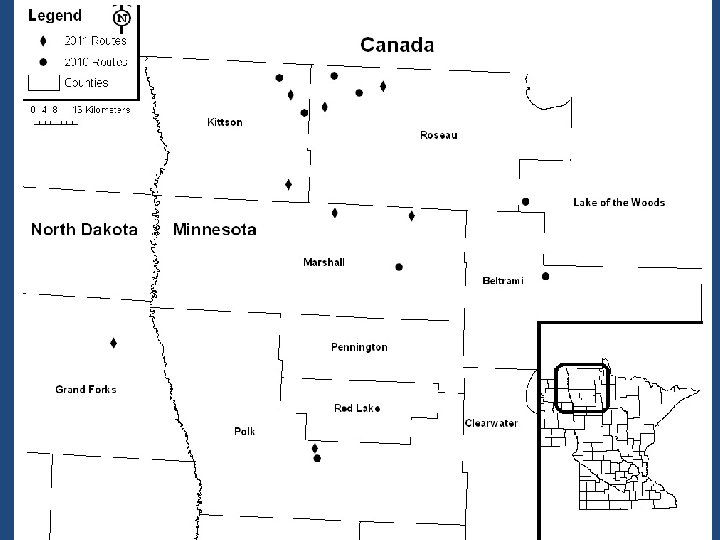
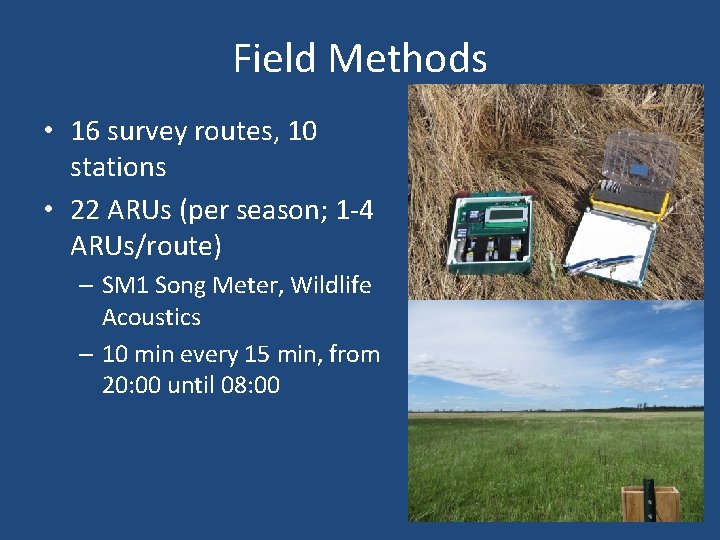
Field Methods • 16 survey routes, 10 stations • 22 ARUs (per season; 1 -4 ARUs/route) – SM 1 Song Meter, Wildlife Acoustics – 10 min every 15 min, from 20: 00 until 08: 00
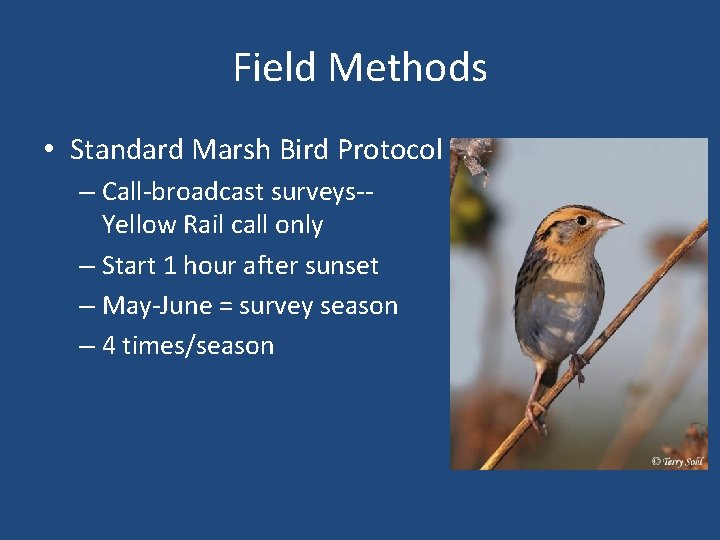
Field Methods • Standard Marsh Bird Protocol – Call-broadcast surveys-Yellow Rail call only – Start 1 hour after sunset – May-June = survey season – 4 times/season
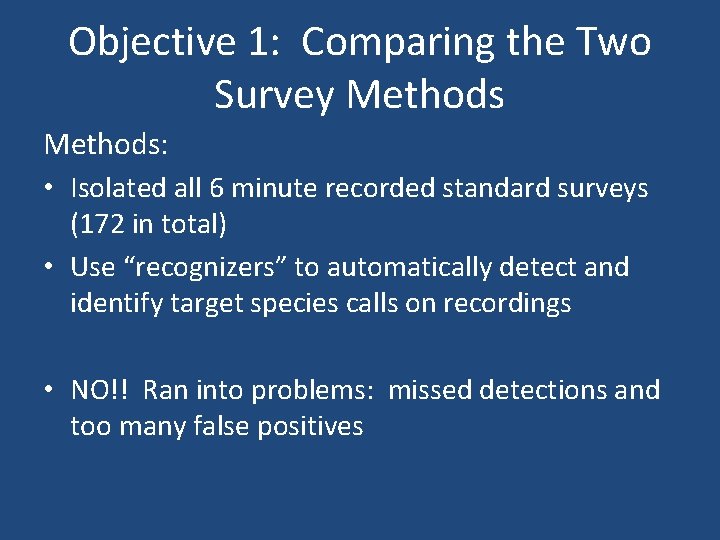
Objective 1: Comparing the Two Survey Methods: • Isolated all 6 minute recorded standard surveys (172 in total) • Use “recognizers” to automatically detect and identify target species calls on recordings • NO!! Ran into problems: missed detections and too many false positives
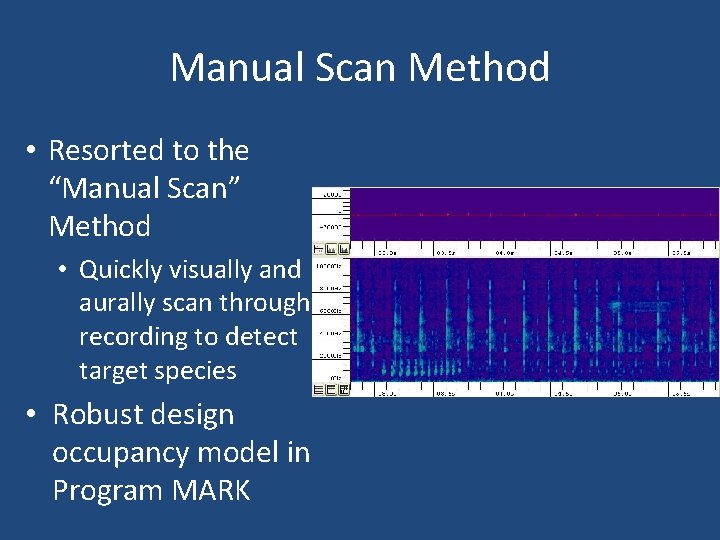
Manual Scan Method • Resorted to the “Manual Scan” Method • Quickly visually and aurally scan through recording to detect target species • Robust design occupancy model in Program MARK
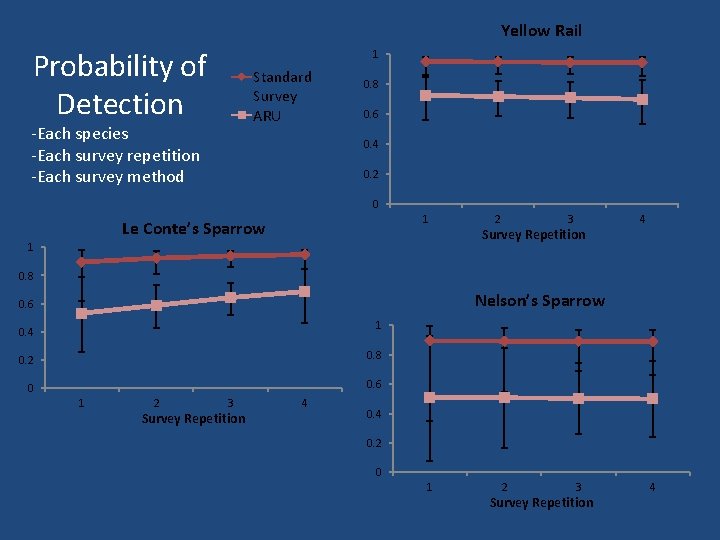
Yellow Rail Probability of Detection 1 Standard Survey ARU -Each species -Each survey repetition -Each survey method 0. 8 0. 6 0. 4 0. 2 0 1 Le Conte’s Sparrow 1 2 3 Survey Repetition 4 0. 8 Nelson’s Sparrow 0. 6 1 0. 4 0. 2 0. 8 0 0. 6 1 2 3 Survey Repetition 4 0. 2 0 1 2 3 Survey Repetition 4
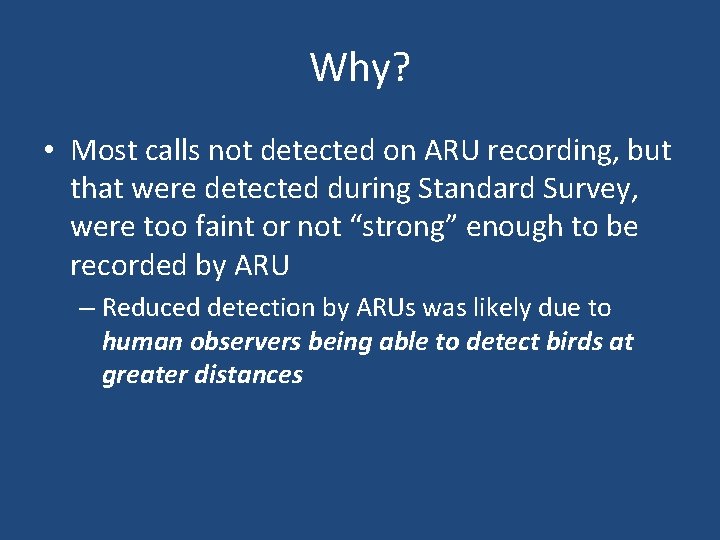
Why? • Most calls not detected on ARU recording, but that were detected during Standard Survey, were too faint or not “strong” enough to be recorded by ARU – Reduced detection by ARUs was likely due to human observers being able to detect birds at greater distances
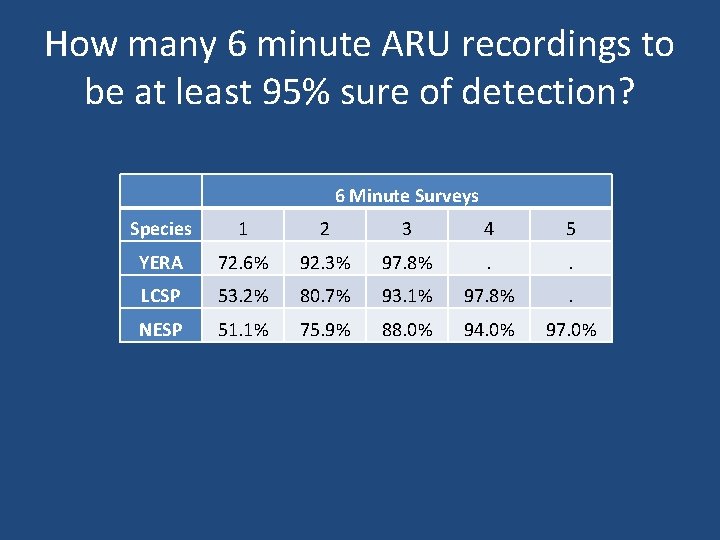
How many 6 minute ARU recordings to be at least 95% sure of detection? 6 Minute Surveys Species 1 2 3 4 5 YERA 72. 6% 92. 3% 97. 8% . . LCSP 53. 2% 80. 7% 93. 1% 97. 8% . NESP 51. 1% 75. 9% 88. 0% 94. 0% 97. 0%
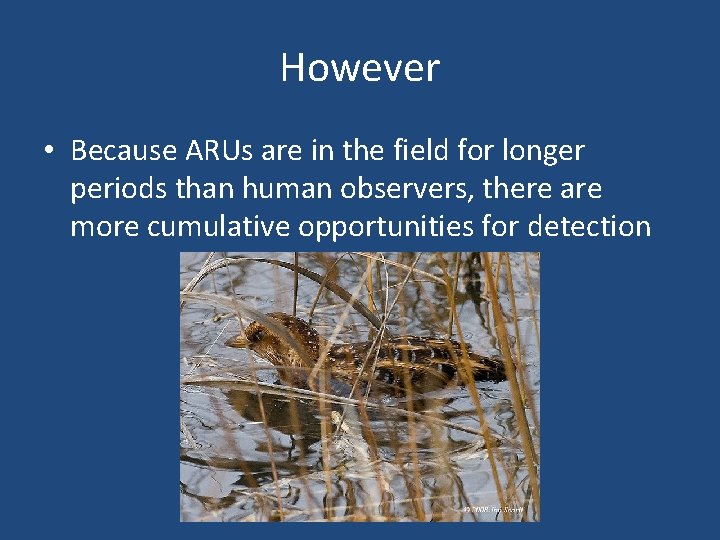
However • Because ARUs are in the field for longer periods than human observers, there are more cumulative opportunities for detection
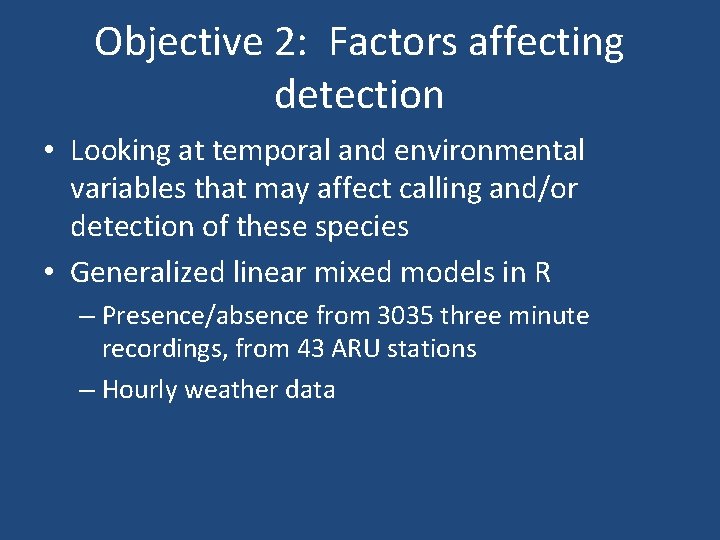
Objective 2: Factors affecting detection • Looking at temporal and environmental variables that may affect calling and/or detection of these species • Generalized linear mixed models in R – Presence/absence from 3035 three minute recordings, from 43 ARU stations – Hourly weather data
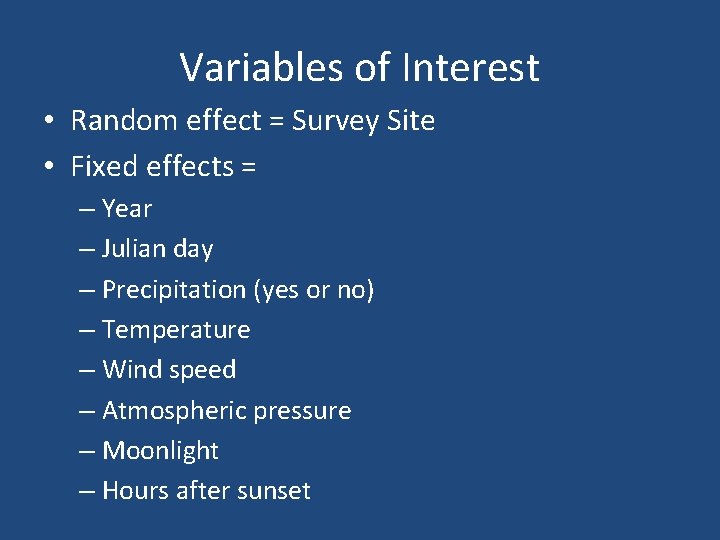
Variables of Interest • Random effect = Survey Site • Fixed effects = – Year – Julian day – Precipitation (yes or no) – Temperature – Wind speed – Atmospheric pressure – Moonlight – Hours after sunset
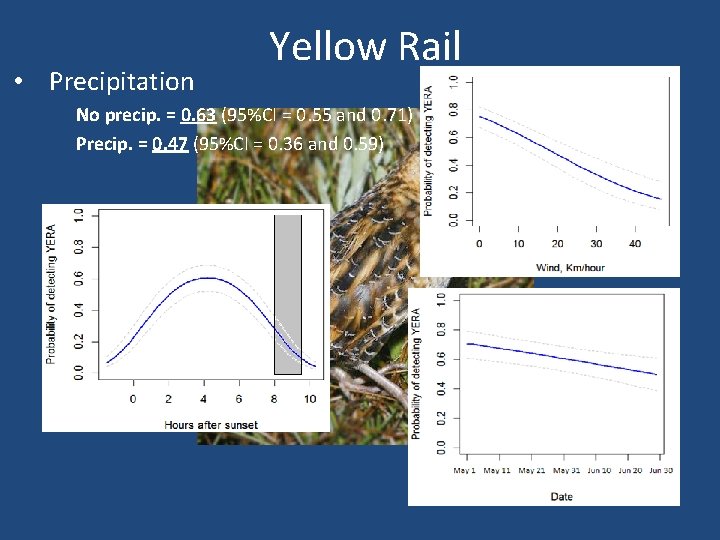
• Precipitation Yellow Rail No precip. = 0. 63 (95%CI = 0. 55 and 0. 71) Precip. = 0. 47 (95%CI = 0. 36 and 0. 59)
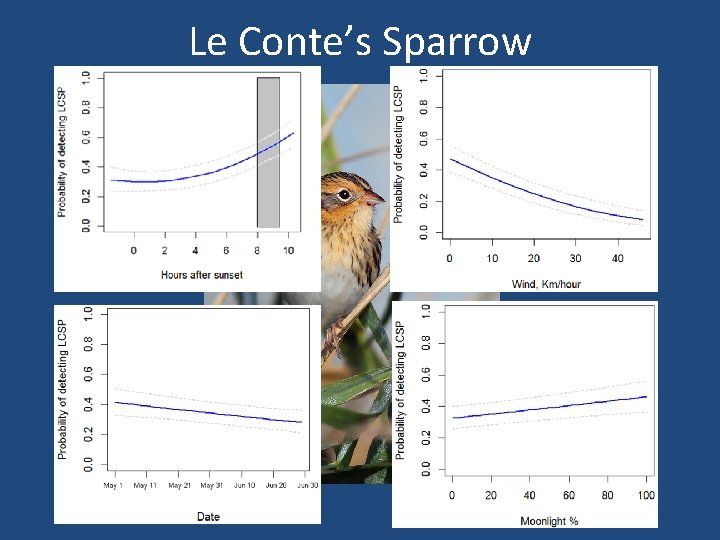
Le Conte’s Sparrow
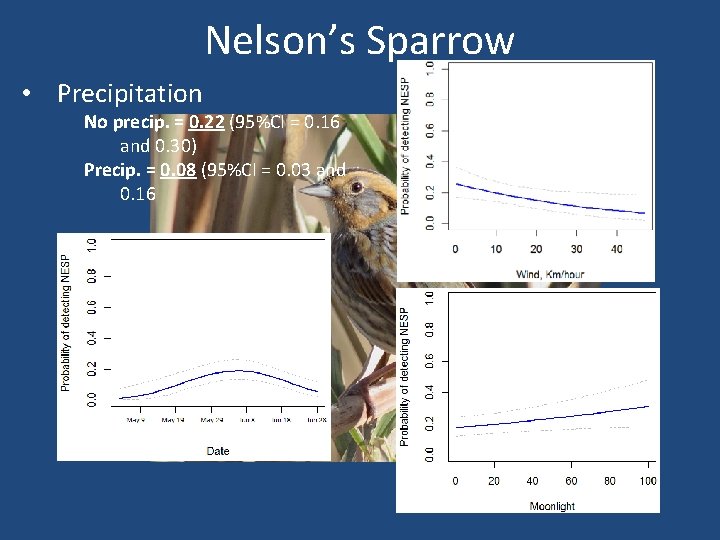
Nelson’s Sparrow • Precipitation No precip. = 0. 22 (95%CI = 0. 16 and 0. 30) Precip. = 0. 08 (95%CI = 0. 03 and 0. 16
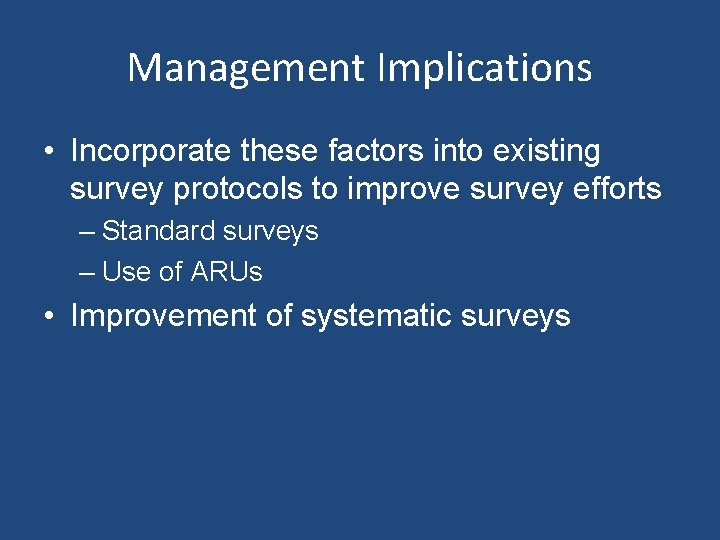
Management Implications • Incorporate these factors into existing survey protocols to improve survey efforts – Standard surveys – Use of ARUs • Improvement of systematic surveys
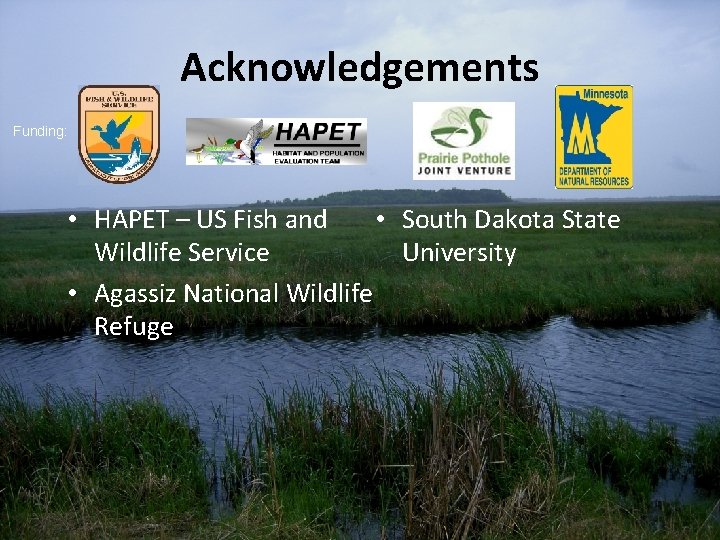
Acknowledgements Funding: • HAPET – US Fish and • South Dakota State Wildlife Service University • Agassiz National Wildlife Refuge
Autonomous recording units
Botox units per ml
Variable costing income statement
Example of partial interval recording
Autonomous stage of learning
Autonomous benthic explorer
Autonomous work teams
Autonomous data harvesting
Atonic vs autonomous bladder
Frontal lobe function
Autonomous expenditure formula
Mengkonfigurasi router pada stub as
Tax multiplier formula
New year equation
Autonomous ode definition
Autonomous consumption
Relative income hypothesis
Demand for a commodity refers to a
Autonomous to lightweight mode upgrade tool
Autonomous investment
Autonomous differential equation
Autonomous expenditure formula
Induced vs autonomous expenditure
Autonomous expenditure