Estimation of the Uncertainty of Predicted Thermophysical Property
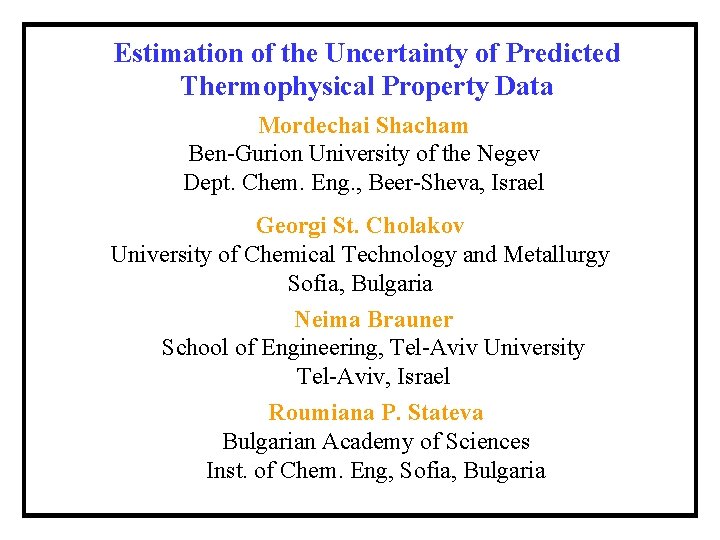
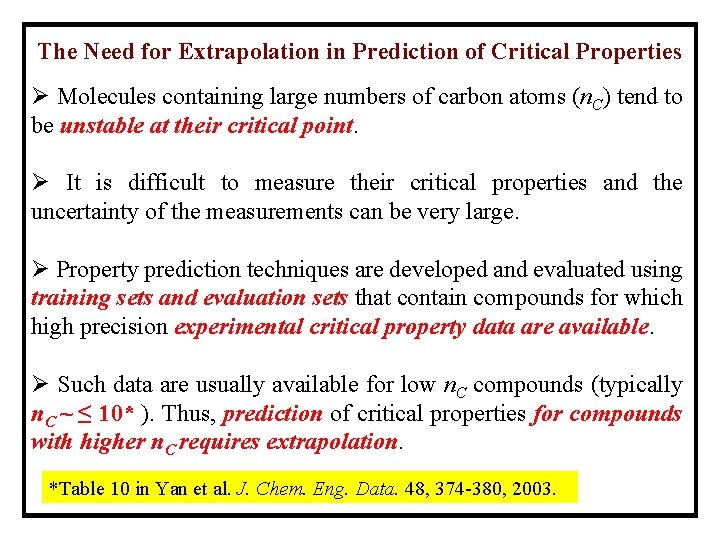
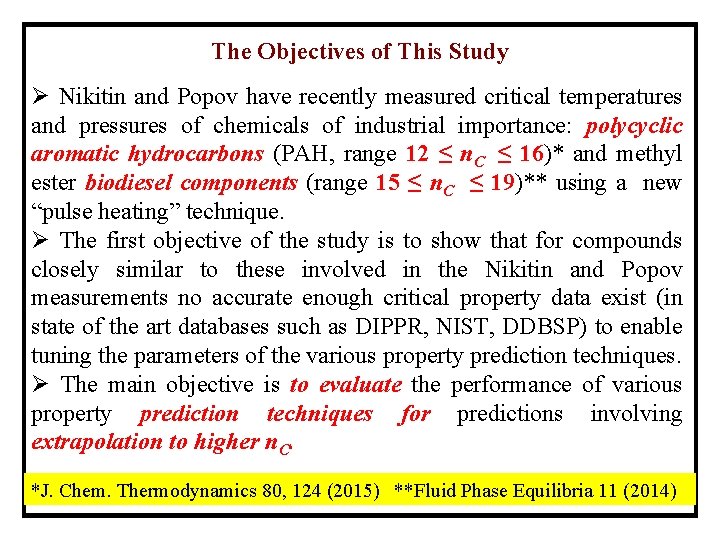
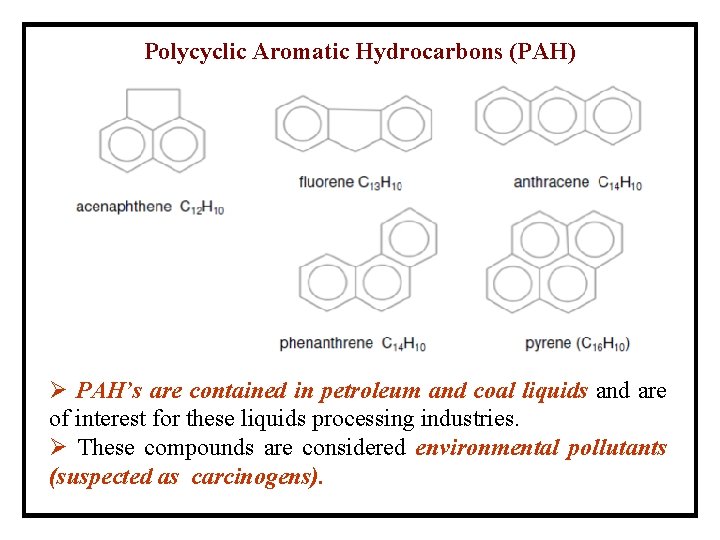
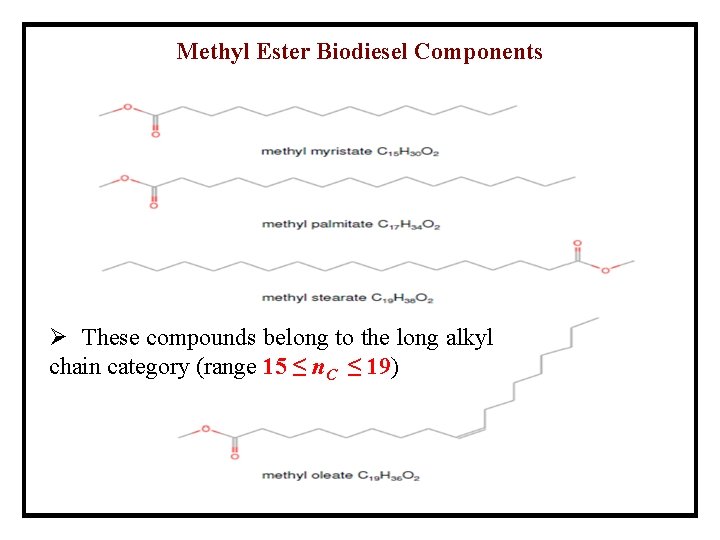
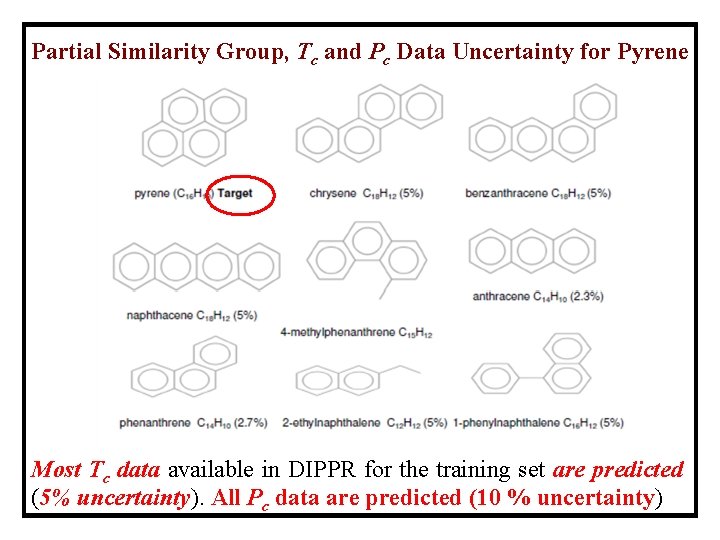
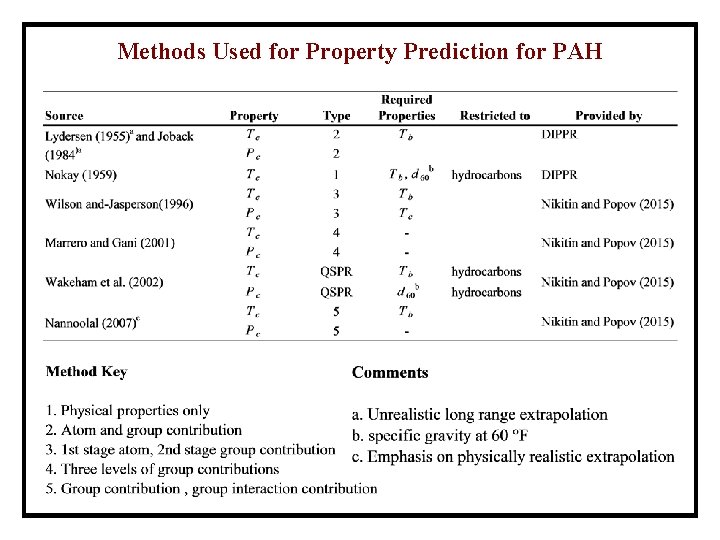
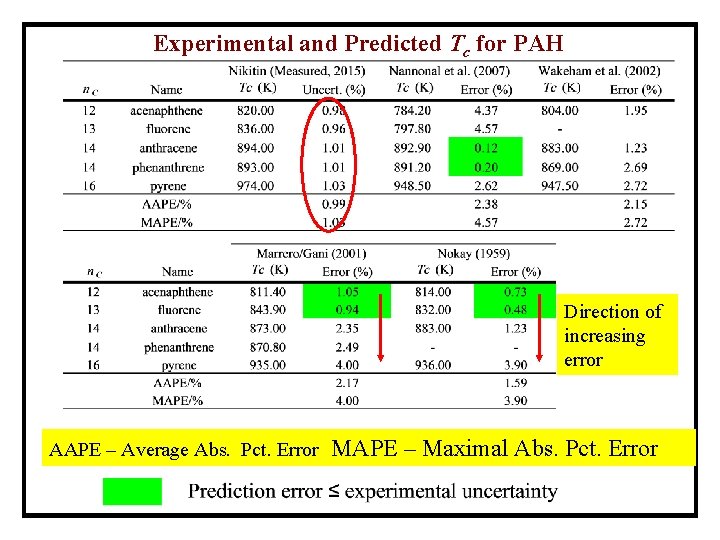
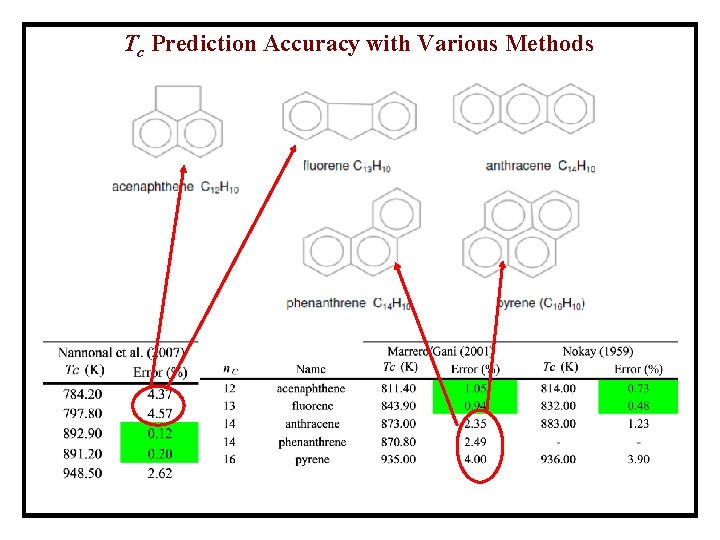
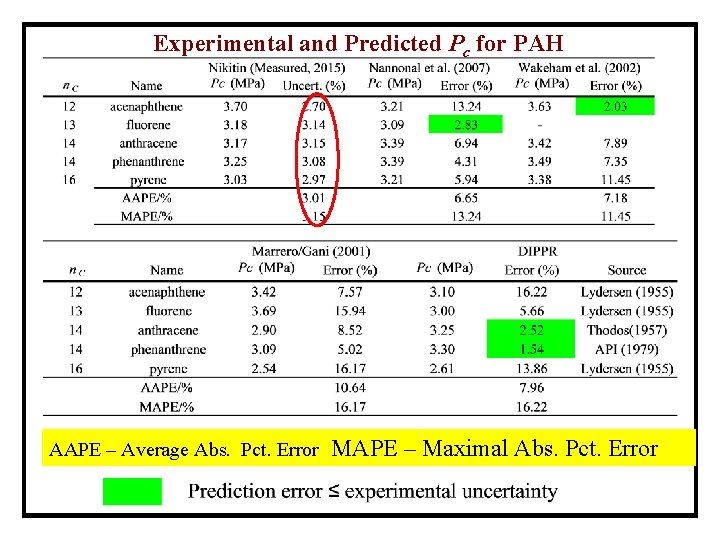
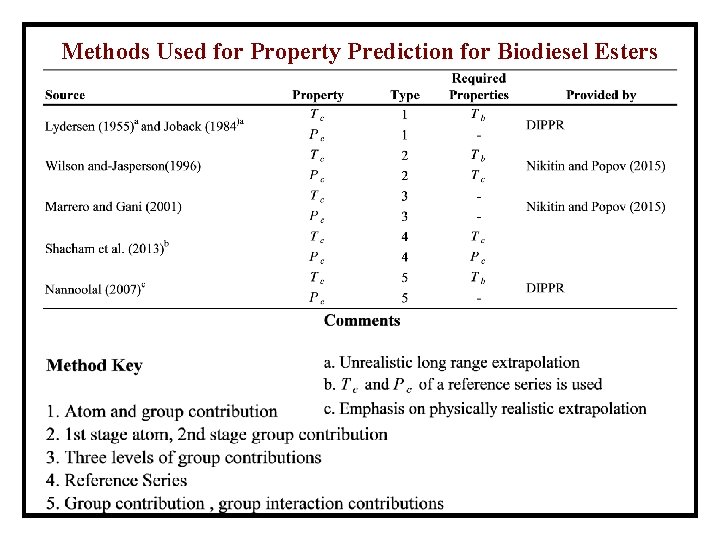
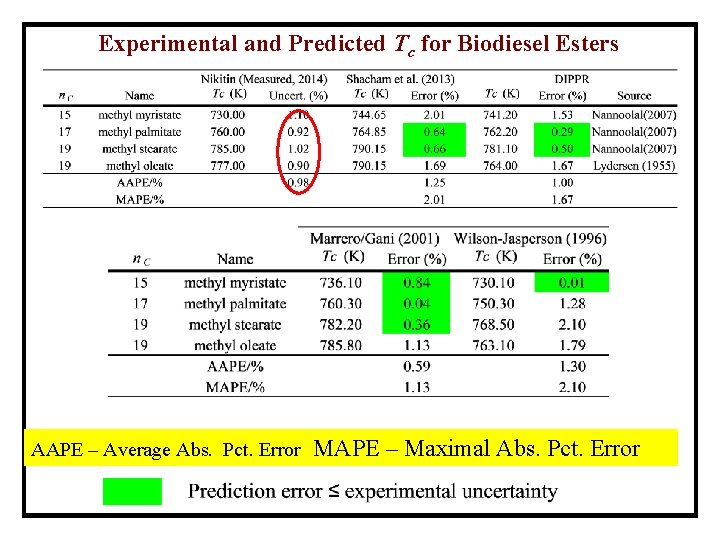
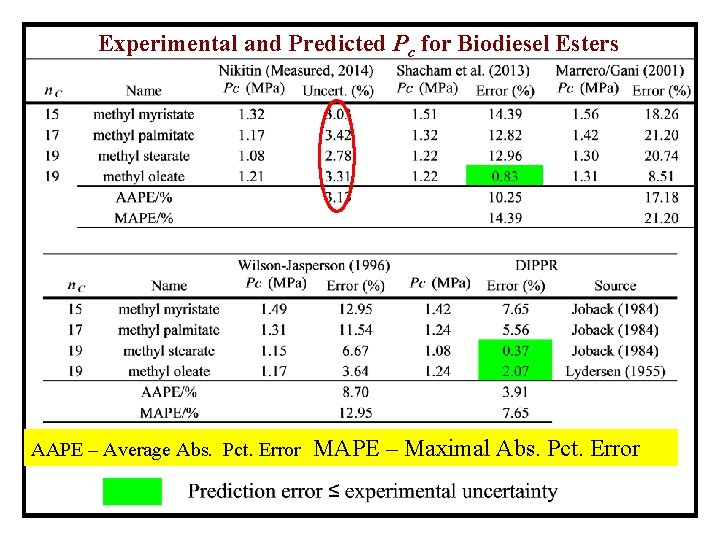
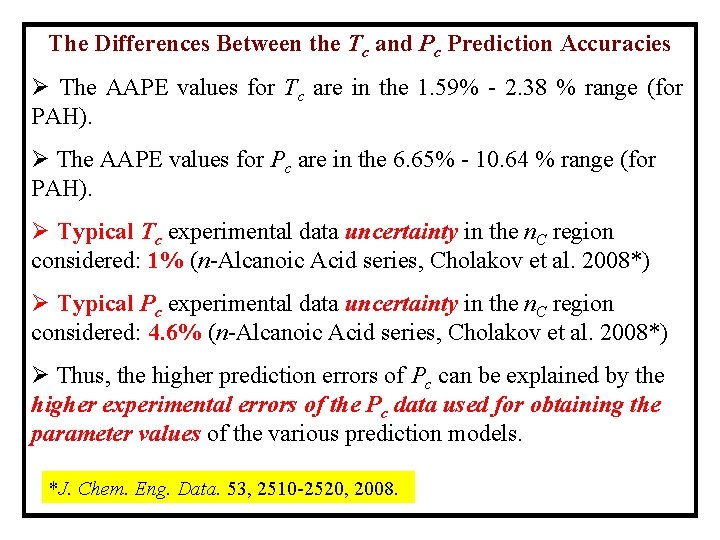
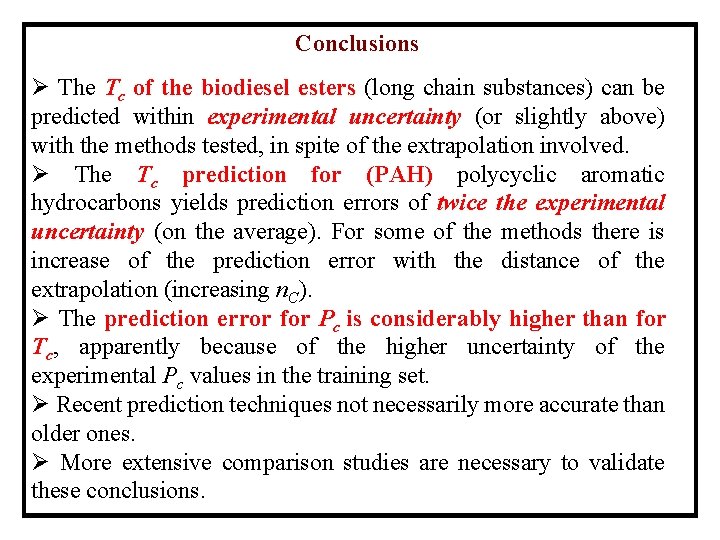
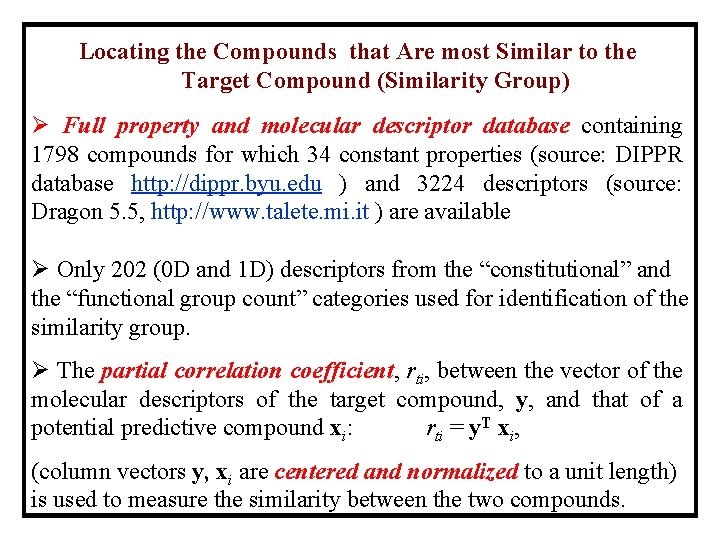
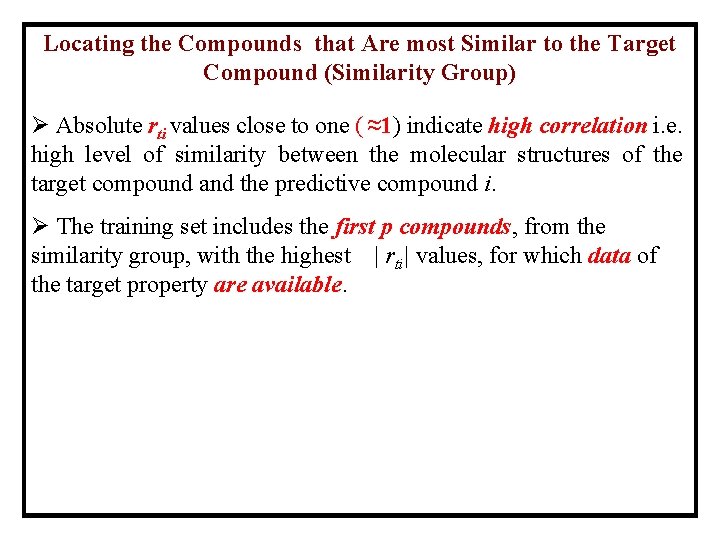
- Slides: 17
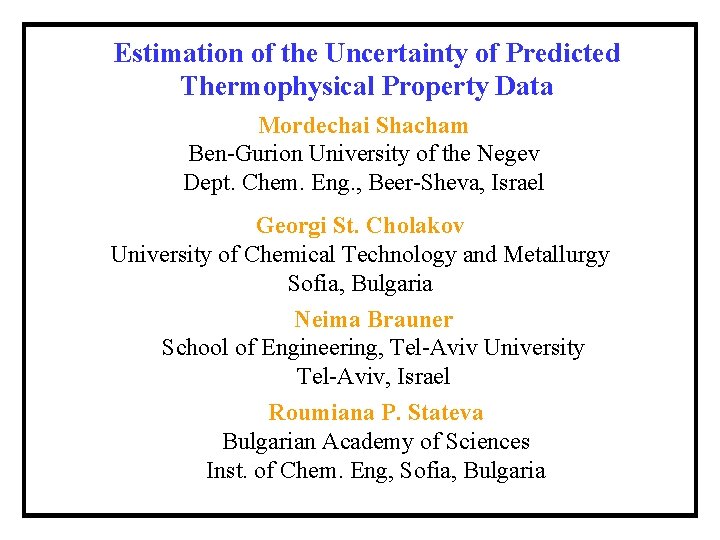
Estimation of the Uncertainty of Predicted Thermophysical Property Data Mordechai Shacham Ben-Gurion University of the Negev Dept. Chem. Eng. , Beer-Sheva, Israel Georgi St. Cholakov University of Chemical Technology and Metallurgy Sofia, Bulgaria Neima Brauner School of Engineering, Tel-Aviv University Tel-Aviv, Israel Roumiana P. Stateva Bulgarian Academy of Sciences Inst. of Chem. Eng, Sofia, Bulgaria
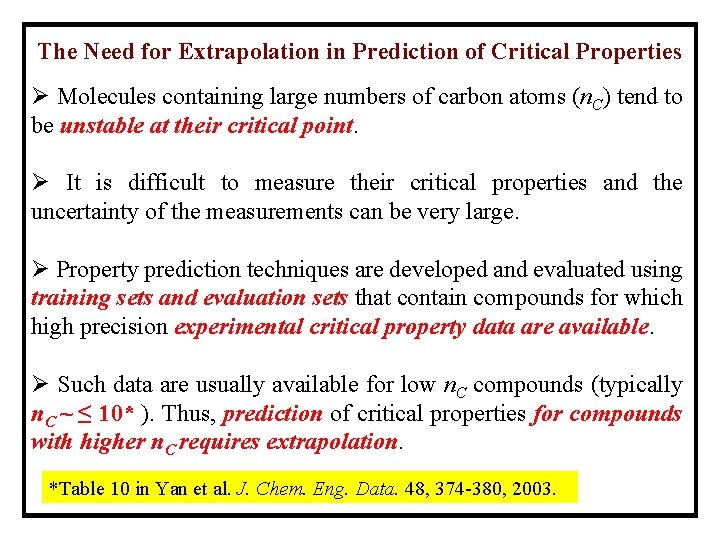
The Need for Extrapolation in Prediction of Critical Properties Ø Molecules containing large numbers of carbon atoms (n. C) tend to be unstable at their critical point. Ø It is difficult to measure their critical properties and the uncertainty of the measurements can be very large. Ø Property prediction techniques are developed and evaluated using training sets and evaluation sets that contain compounds for which high precision experimental critical property data are available. Ø Such data are usually available for low n. C compounds (typically n. C ~ ≤ 10* ). Thus, prediction of critical properties for compounds with higher n. C requires extrapolation. *Table 10 in Yan et al. J. Chem. Eng. Data. 48, 374 -380, 2003.
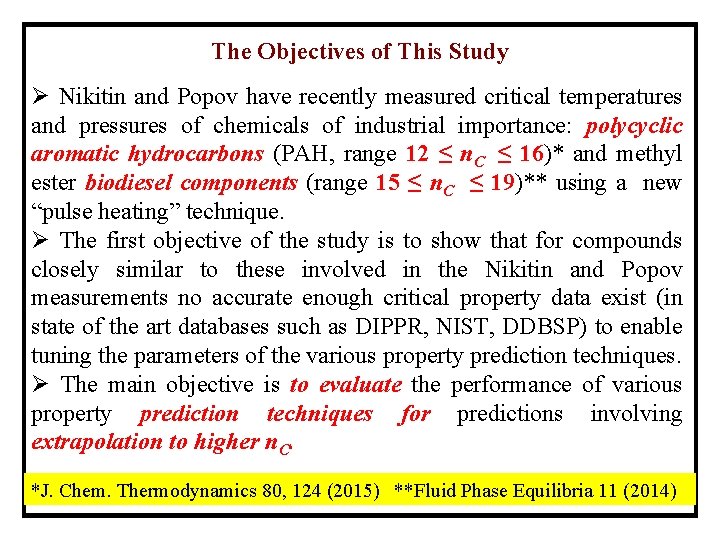
The Objectives of This Study Ø Nikitin and Popov have recently measured critical temperatures and pressures of chemicals of industrial importance: polycyclic aromatic hydrocarbons (PAH, range 12 ≤ n. C ≤ 16)* and methyl ester biodiesel components (range 15 ≤ n. C ≤ 19)** using a new “pulse heating” technique. Ø The first objective of the study is to show that for compounds closely similar to these involved in the Nikitin and Popov measurements no accurate enough critical property data exist (in state of the art databases such as DIPPR, NIST, DDBSP) to enable tuning the parameters of the various property prediction techniques. Ø The main objective is to evaluate the performance of various property prediction techniques for predictions involving extrapolation to higher n. C. *J. Chem. Thermodynamics 80, 124 (2015) **Fluid Phase Equilibria 11 (2014)
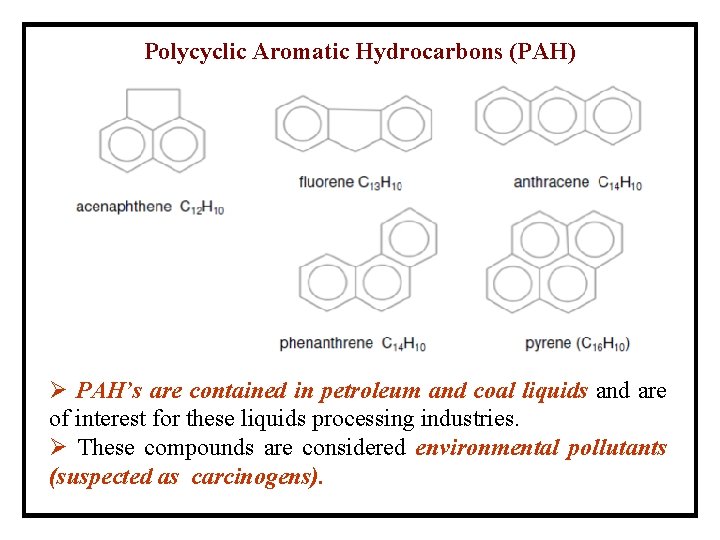
Polycyclic Aromatic Hydrocarbons (PAH) Ø PAH’s are contained in petroleum and coal liquids and are of interest for these liquids processing industries. Ø These compounds are considered environmental pollutants (suspected as carcinogens).
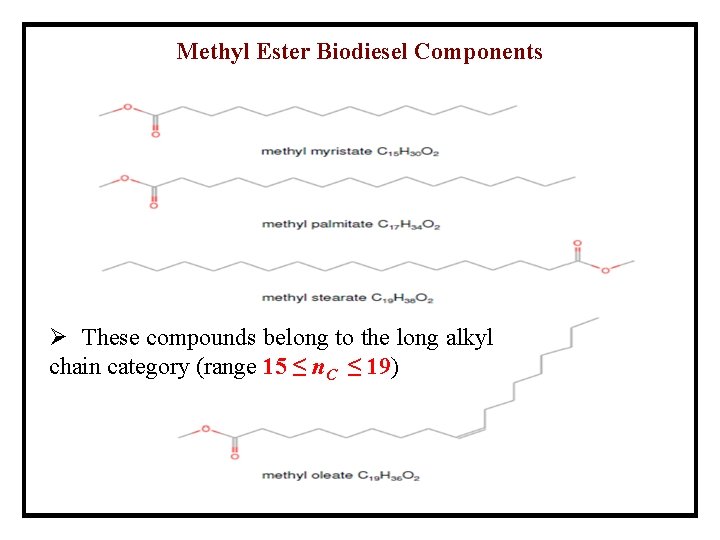
Methyl Ester Biodiesel Components Ø These compounds belong to the long alkyl chain category (range 15 ≤ n. C ≤ 19)
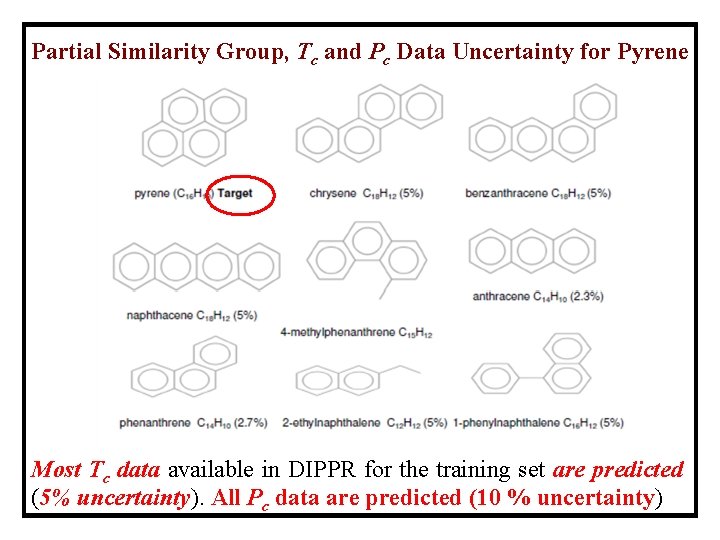
Partial Similarity Group, Tc and Pc Data Uncertainty for Pyrene Most Tc data available in DIPPR for the training set are predicted (5% uncertainty). All Pc data are predicted (10 % uncertainty)
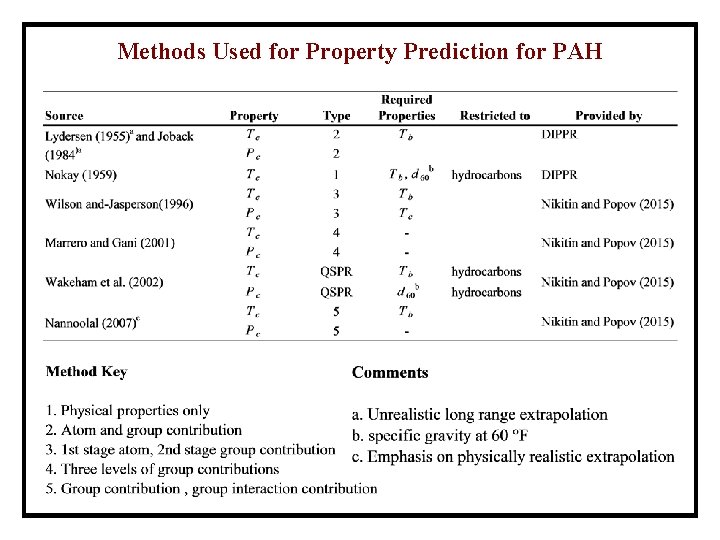
Methods Used for Property Prediction for PAH
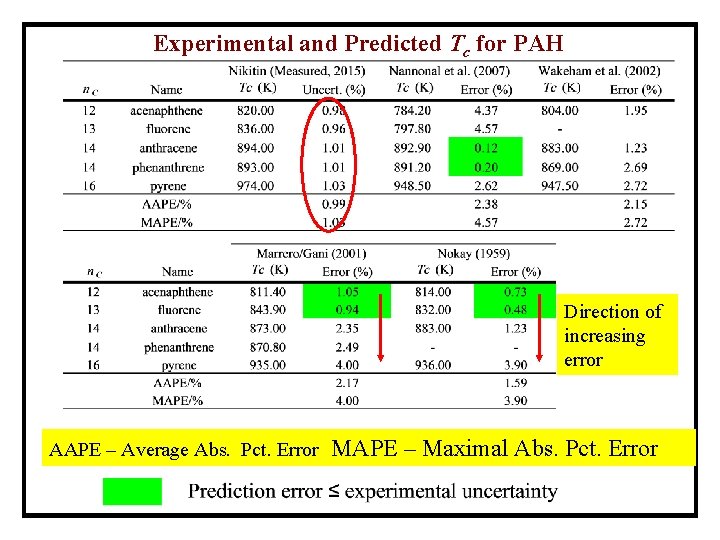
Experimental and Predicted Tc for PAH Direction of increasing error AAPE – Average Abs. Pct. Error MAPE – Maximal Abs. Pct. Error
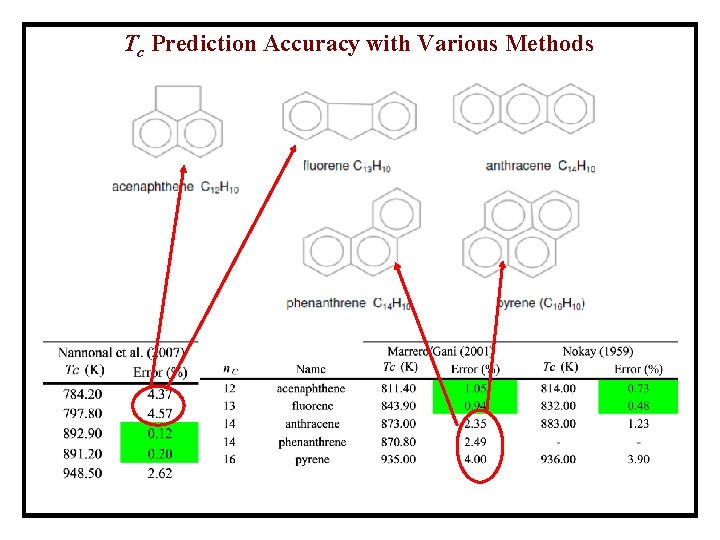
Tc Prediction Accuracy with Various Methods
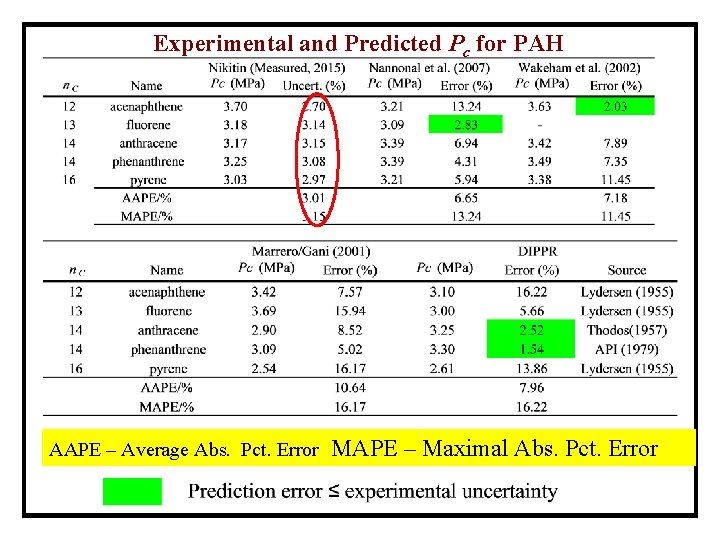
Experimental and Predicted Pc for PAH AAPE – Average Abs. Pct. Error MAPE – Maximal Abs. Pct. Error
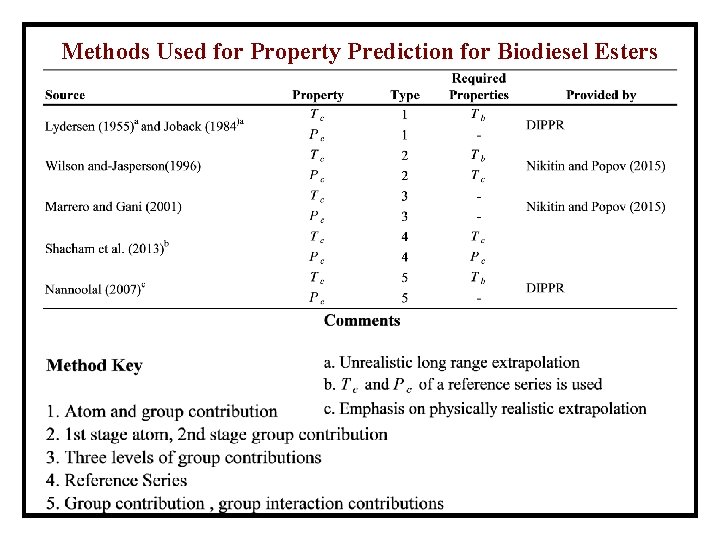
Methods Used for Property Prediction for Biodiesel Esters
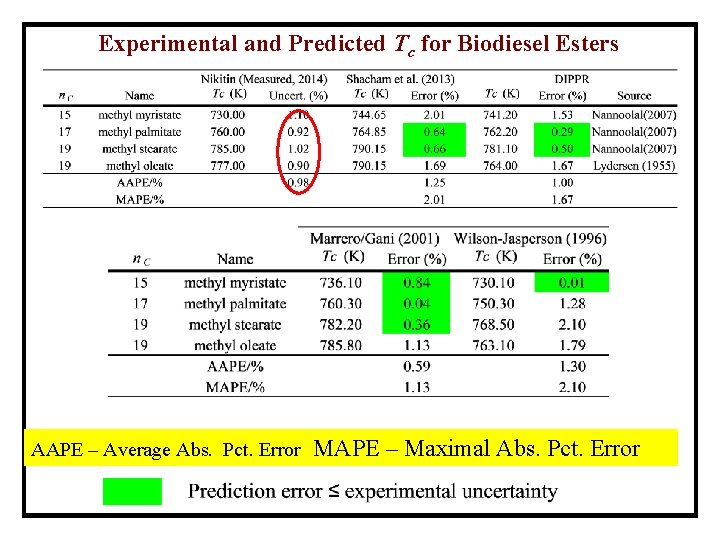
Experimental and Predicted Tc for Biodiesel Esters AAPE – Average Abs. Pct. Error MAPE – Maximal Abs. Pct. Error
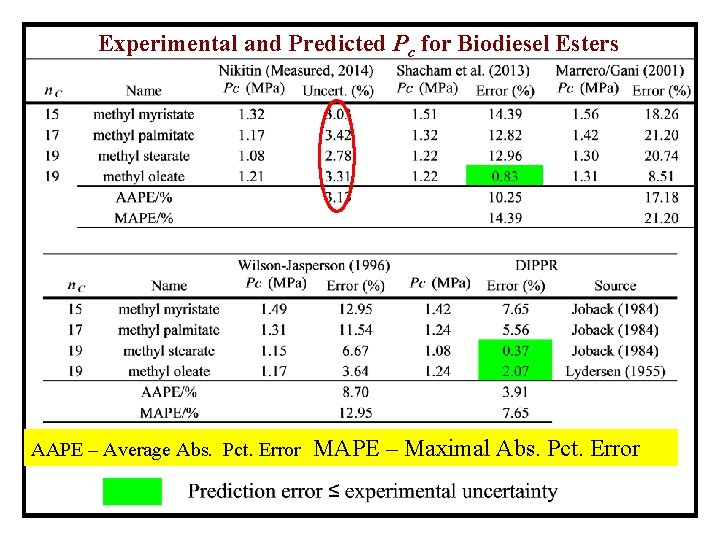
Experimental and Predicted Pc for Biodiesel Esters AAPE – Average Abs. Pct. Error MAPE – Maximal Abs. Pct. Error
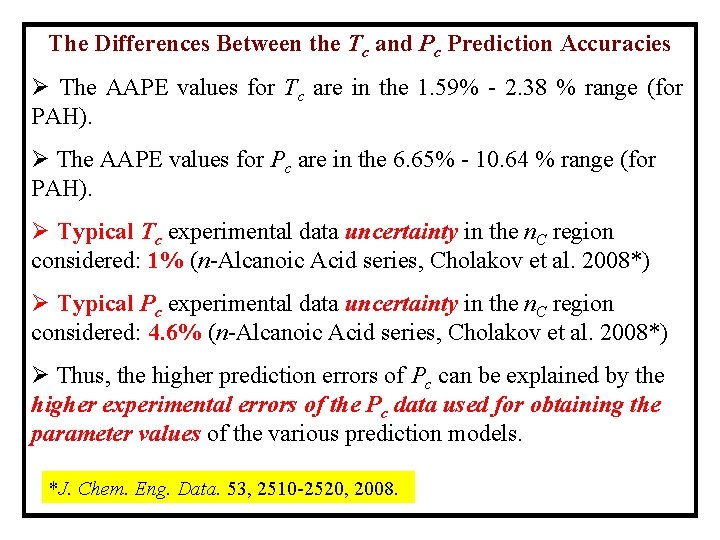
The Differences Between the Tc and Pc Prediction Accuracies Ø The AAPE values for Tc are in the 1. 59% - 2. 38 % range (for PAH). Ø The AAPE values for Pc are in the 6. 65% - 10. 64 % range (for PAH). Ø Typical Tc experimental data uncertainty in the n. C region considered: 1% (n-Alcanoic Acid series, Cholakov et al. 2008*) Ø Typical Pc experimental data uncertainty in the n. C region considered: 4. 6% (n-Alcanoic Acid series, Cholakov et al. 2008*) Ø Thus, the higher prediction errors of Pc can be explained by the higher experimental errors of the Pc data used for obtaining the parameter values of the various prediction models. *J. Chem. Eng. Data. 53, 2510 -2520, 2008.
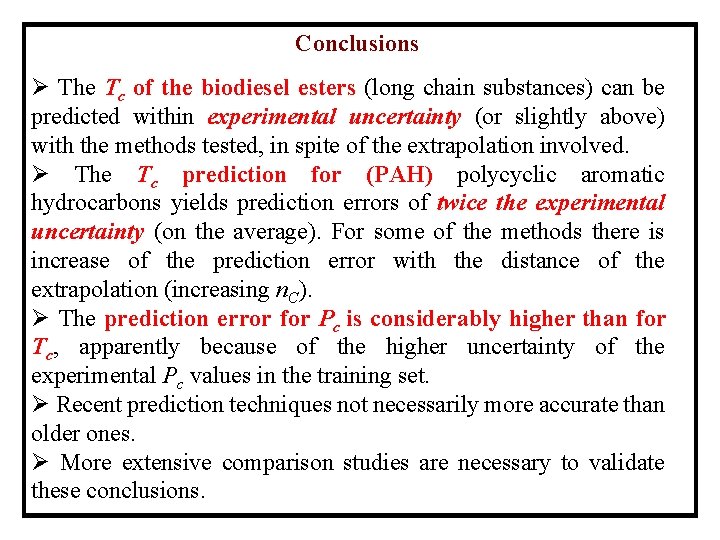
Conclusions Ø The Tc of the biodiesel esters (long chain substances) can be predicted within experimental uncertainty (or slightly above) with the methods tested, in spite of the extrapolation involved. Ø The Tc prediction for (PAH) polycyclic aromatic hydrocarbons yields prediction errors of twice the experimental uncertainty (on the average). For some of the methods there is increase of the prediction error with the distance of the extrapolation (increasing n. C). Ø The prediction error for Pc is considerably higher than for Tc, apparently because of the higher uncertainty of the experimental Pc values in the training set. Ø Recent prediction techniques not necessarily more accurate than older ones. Ø More extensive comparison studies are necessary to validate these conclusions.
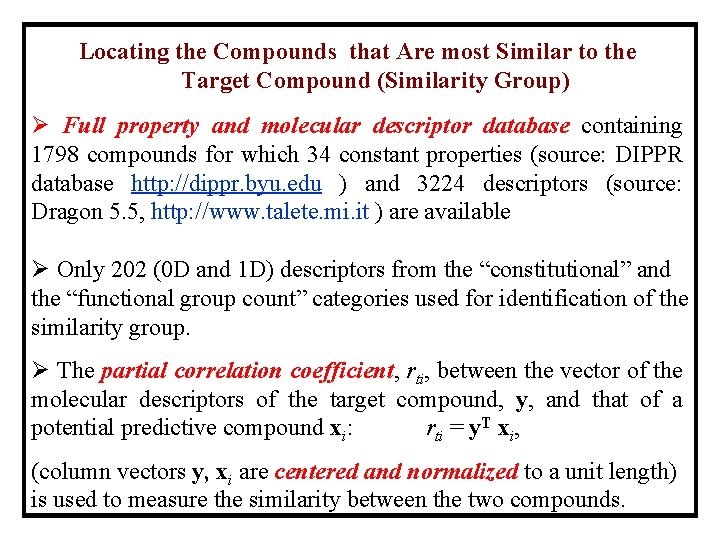
Locating the Compounds that Are most Similar to the Target Compound (Similarity Group) Ø Full property and molecular descriptor database containing 1798 compounds for which 34 constant properties (source: DIPPR database http: //dippr. byu. edu ) and 3224 descriptors (source: Dragon 5. 5, http: //www. talete. mi. it ) are available Ø Only 202 (0 D and 1 D) descriptors from the “constitutional” and the “functional group count” categories used for identification of the similarity group. Ø The partial correlation coefficient, rti, between the vector of the molecular descriptors of the target compound, y, and that of a potential predictive compound xi: rti = y. T xi, (column vectors y, xi are centered and normalized to a unit length) is used to measure the similarity between the two compounds.
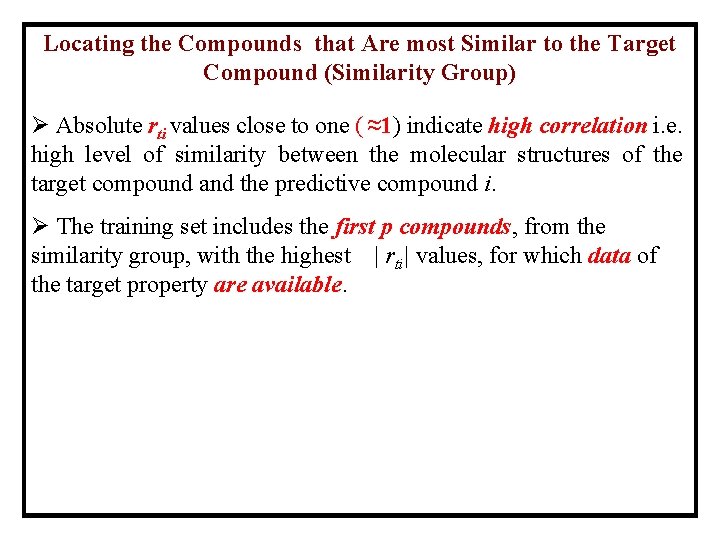
Locating the Compounds that Are most Similar to the Target Compound (Similarity Group) Ø Absolute rti values close to one ( ≈1) indicate high correlation i. e. high level of similarity between the molecular structures of the target compound and the predictive compound i. Ø The training set includes the first p compounds, from the similarity group, with the highest | rti| values, for which data of the target property are available.
Thermophysical properties of dental materials
Predicted outcome value theory
Predicted service
Chemical and physical properties
Associative vs commutative
Obstructed heritage in hindu law
Thế nào là sự mỏi cơ
Bổ thể
Vẽ hình chiếu đứng bằng cạnh của vật thể
độ dài liên kết
Thiếu nhi thế giới liên hoan
Chúa yêu trần thế
điện thế nghỉ
Một số thể thơ truyền thống
Trời xanh đây là của chúng ta thể thơ
Bảng số nguyên tố lớn hơn 1000
Vẽ hình chiếu vuông góc của vật thể sau
đặc điểm cơ thể của người tối cổ