Developing Novel Data Architectures for Comparative Effectiveness Research
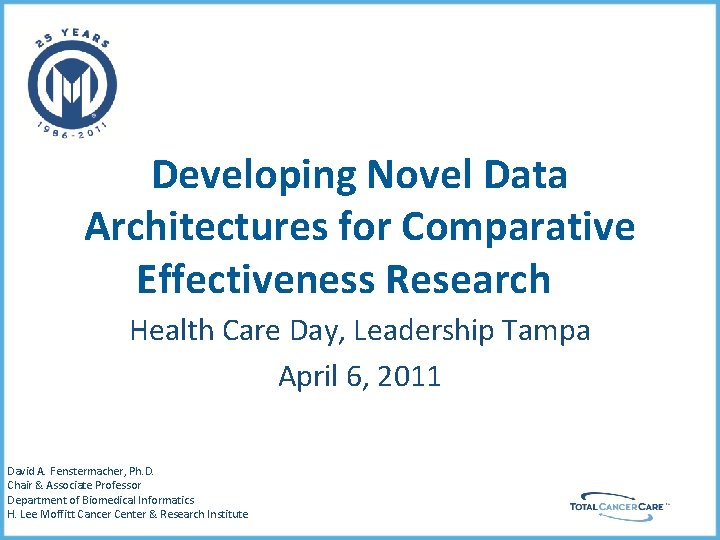
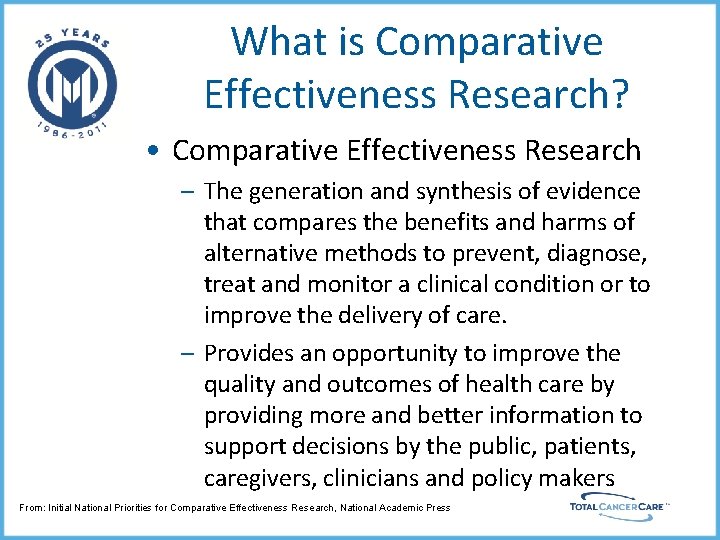
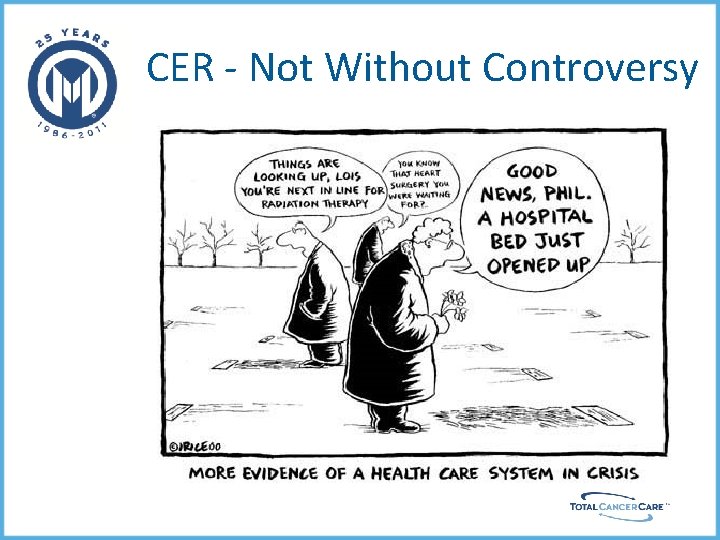
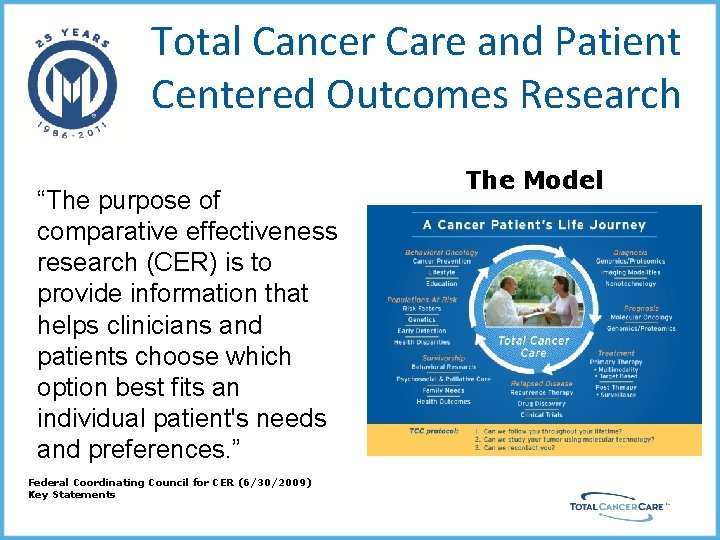
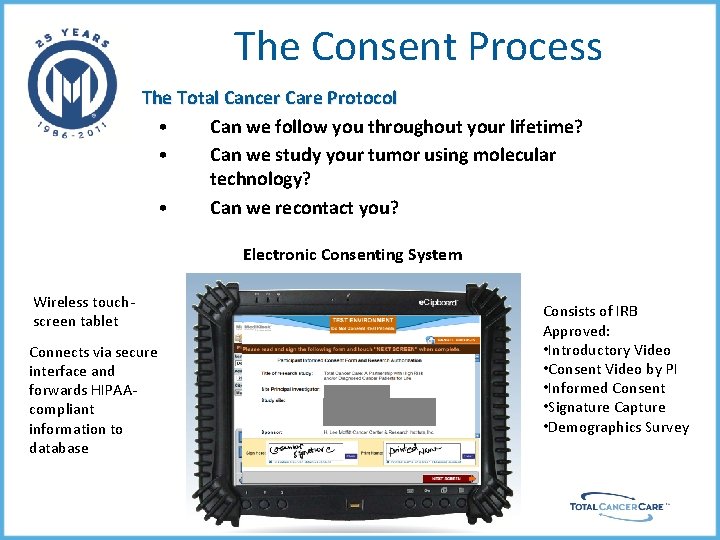
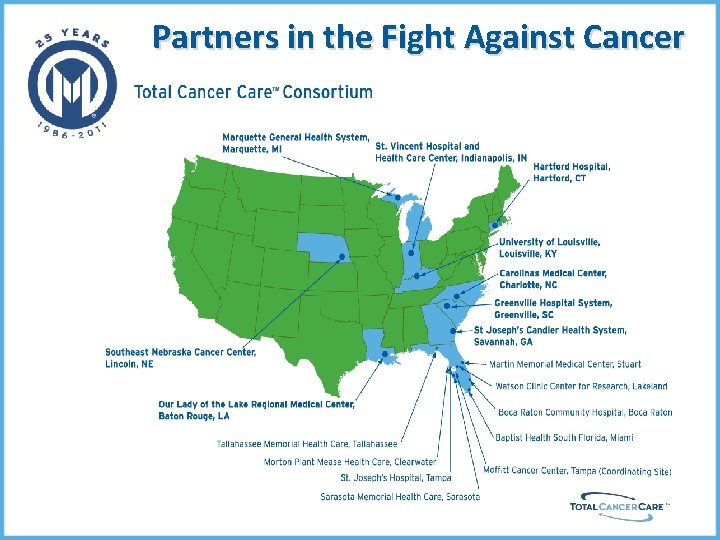
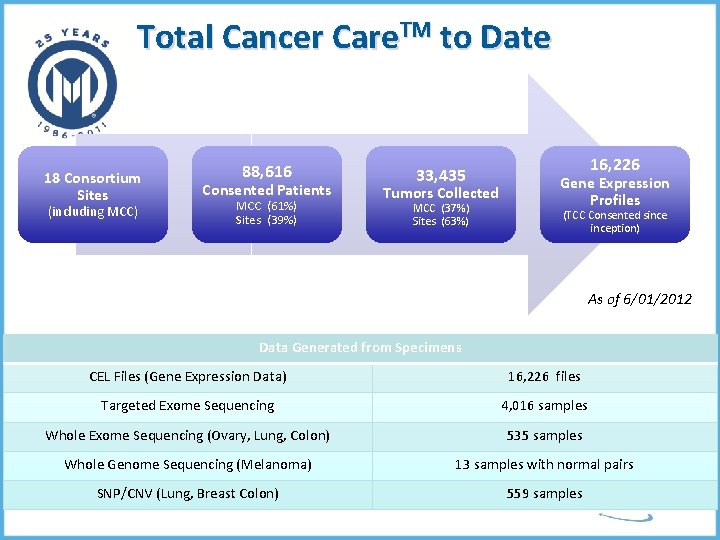
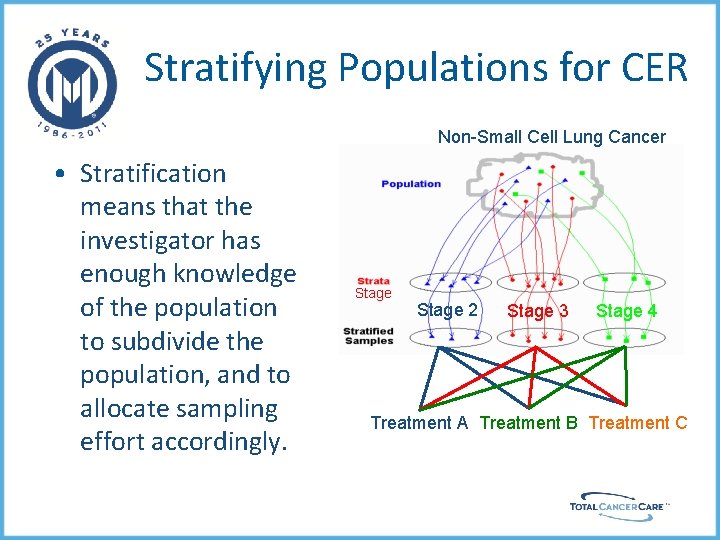
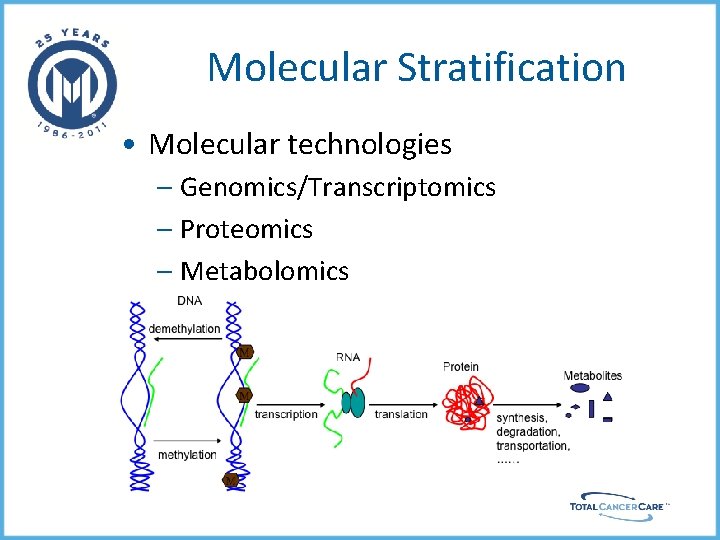
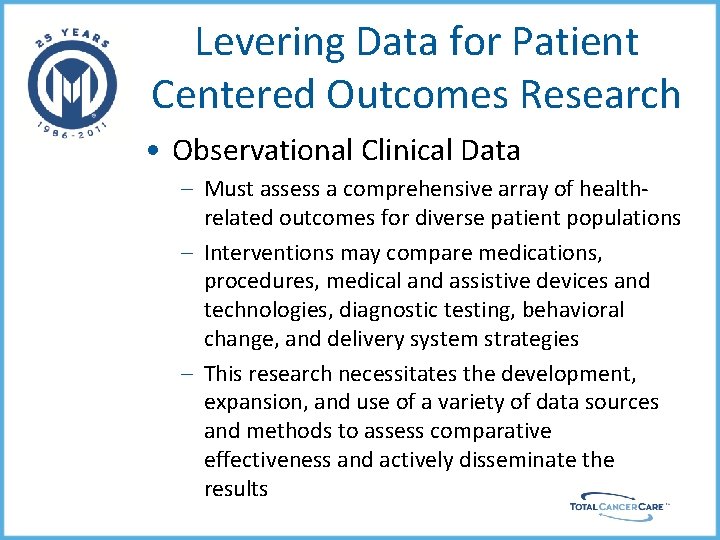
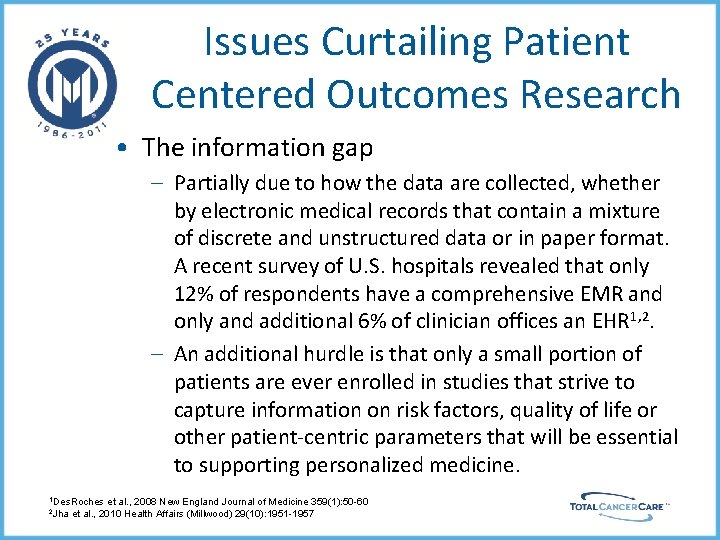
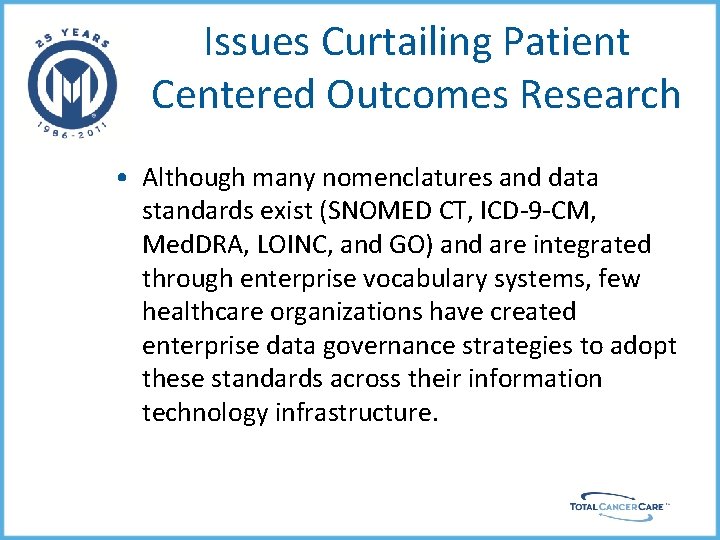
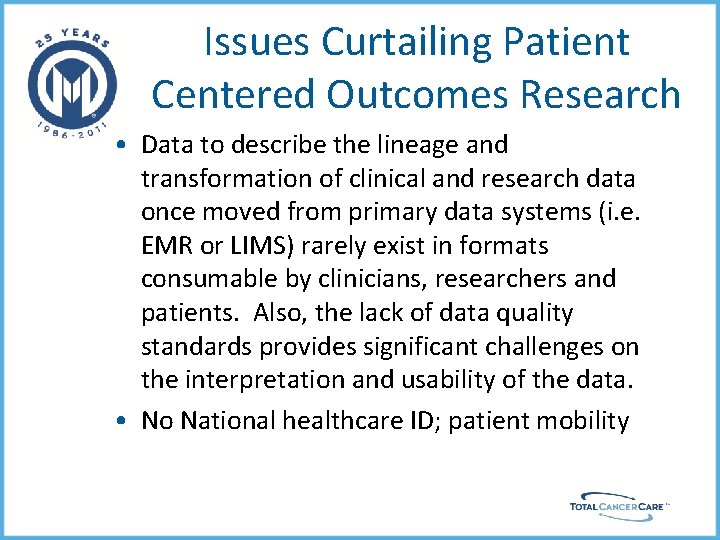
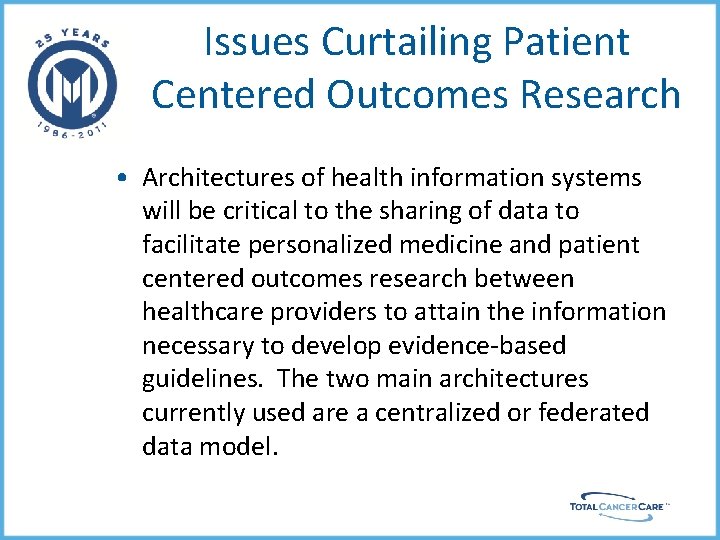
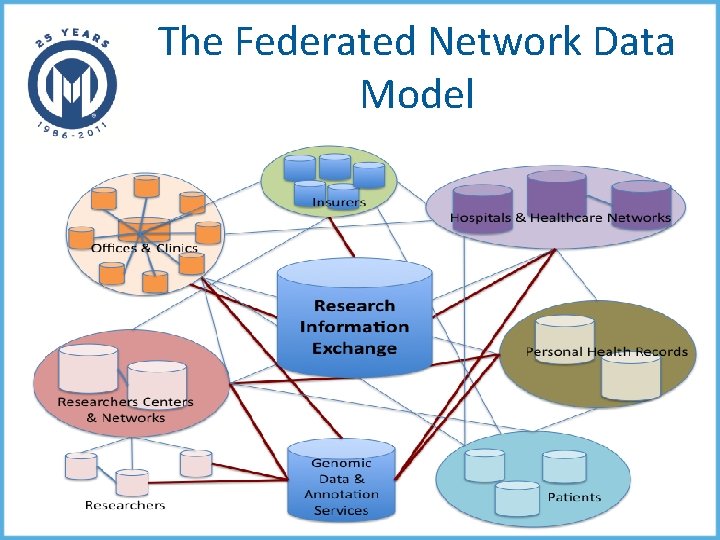
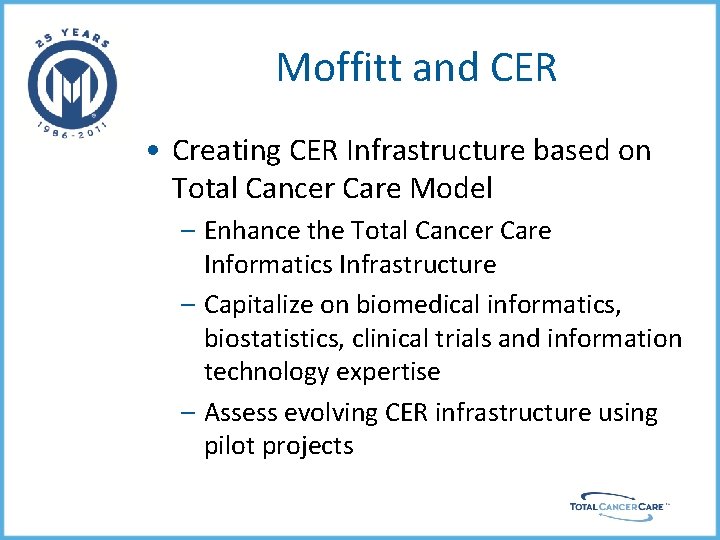
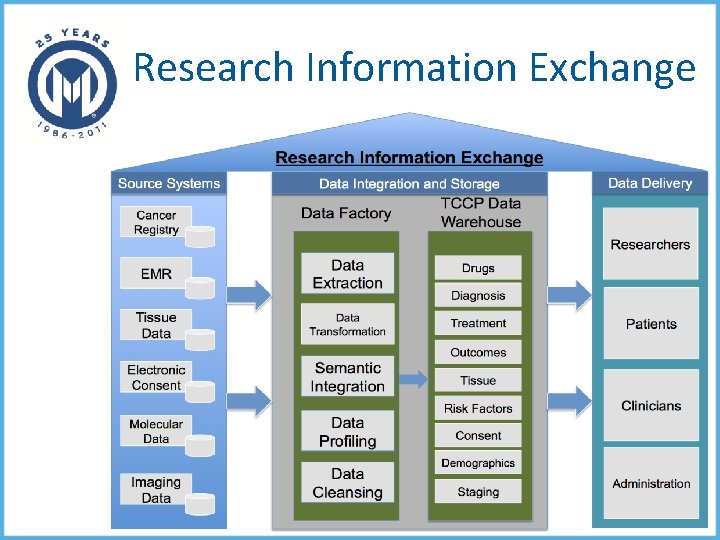
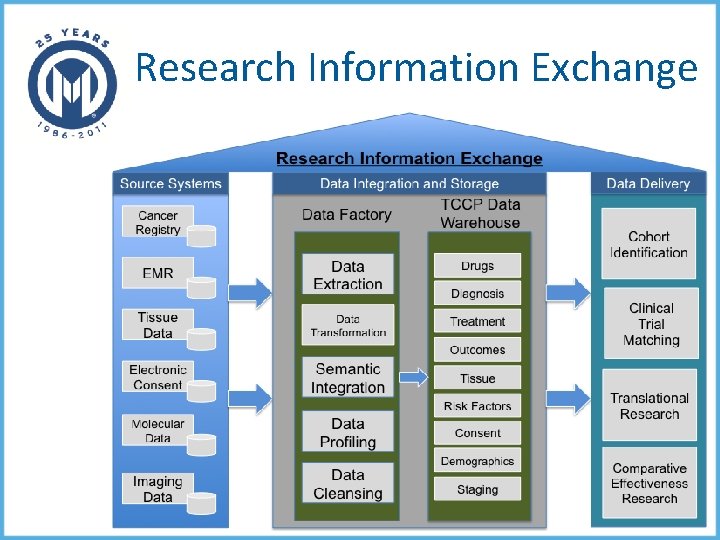
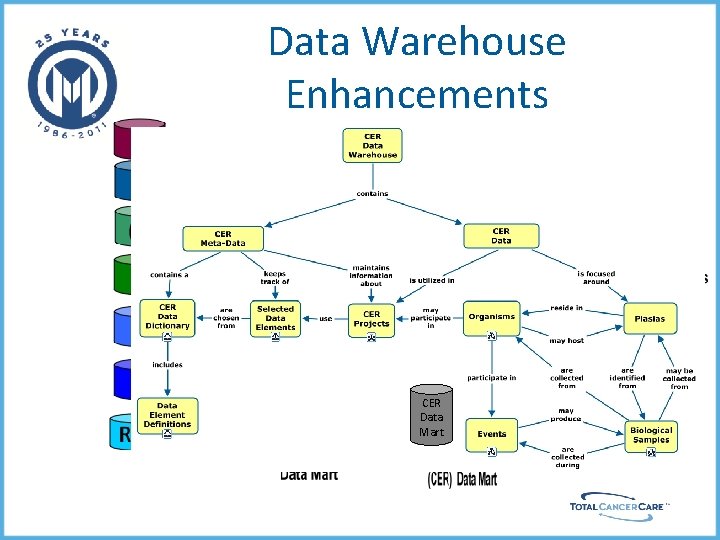
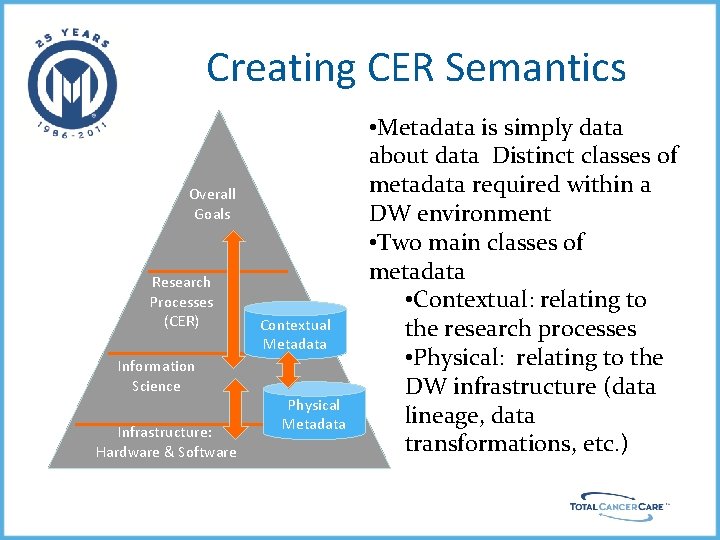
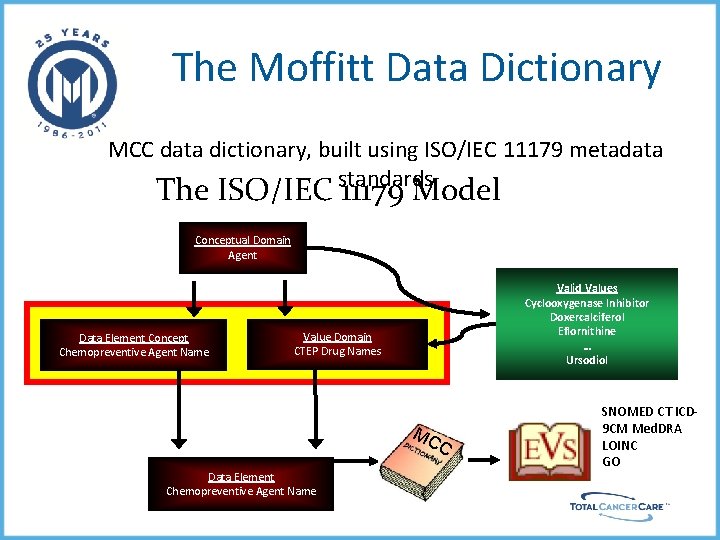
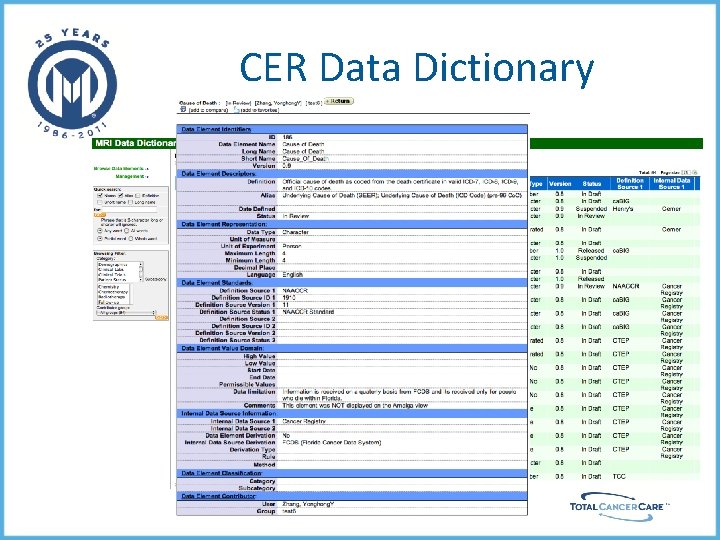
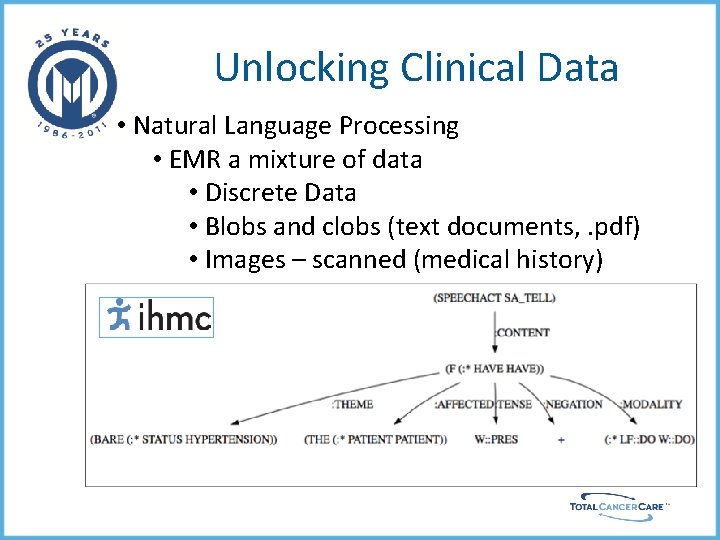
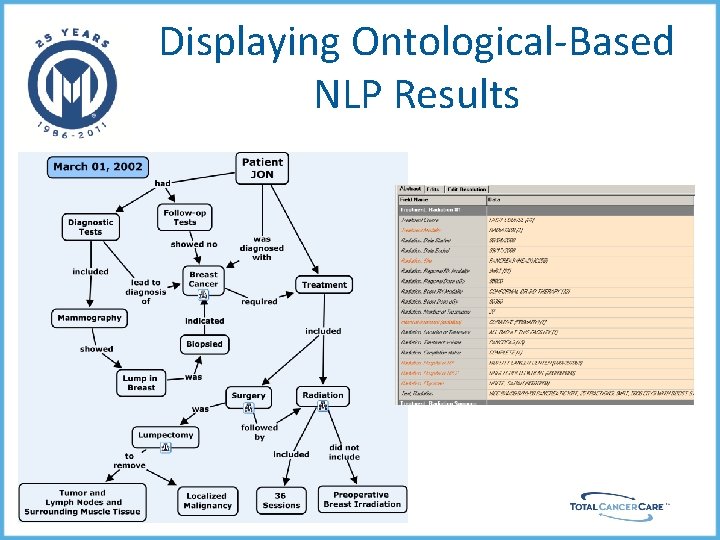
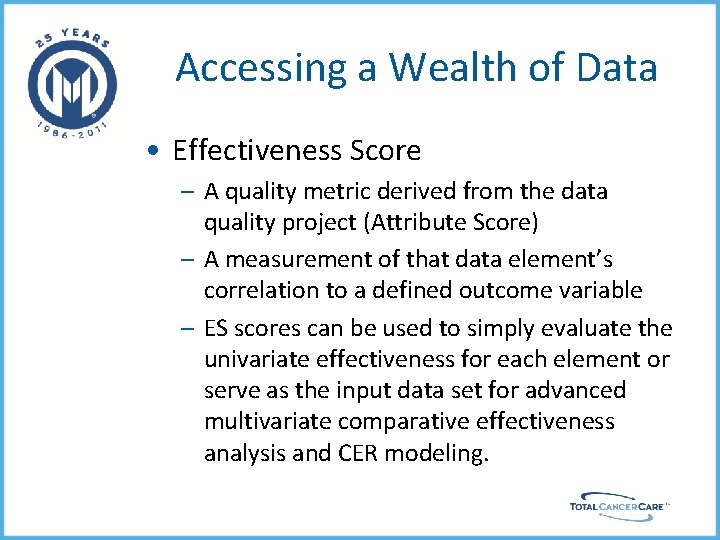
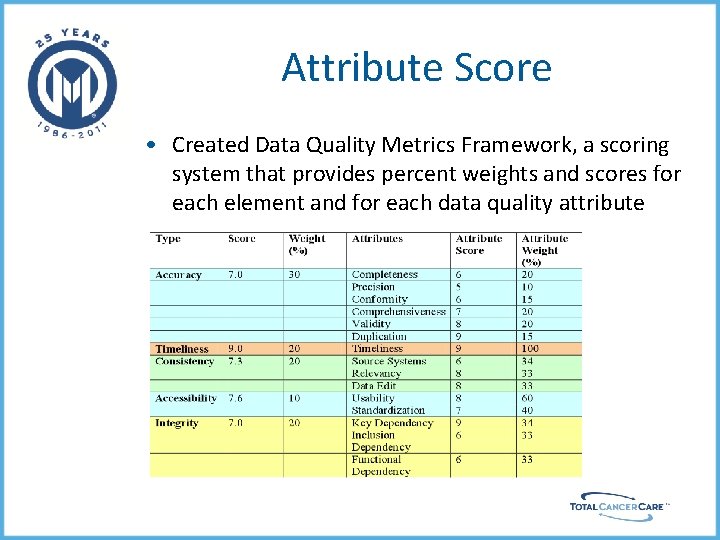
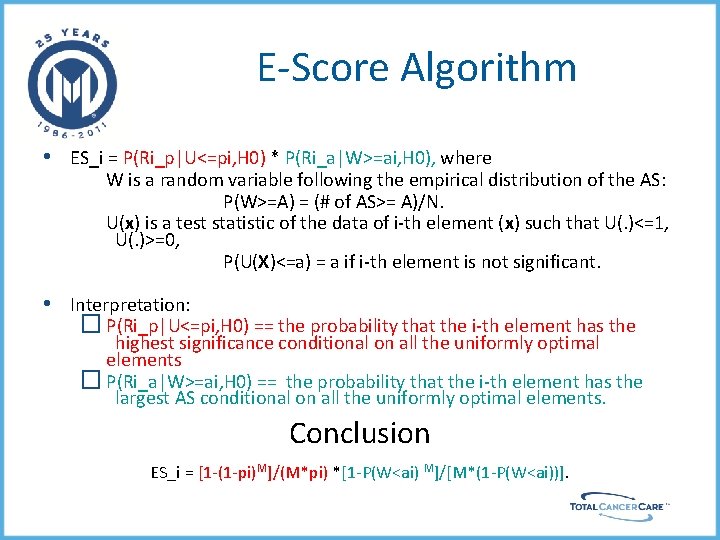
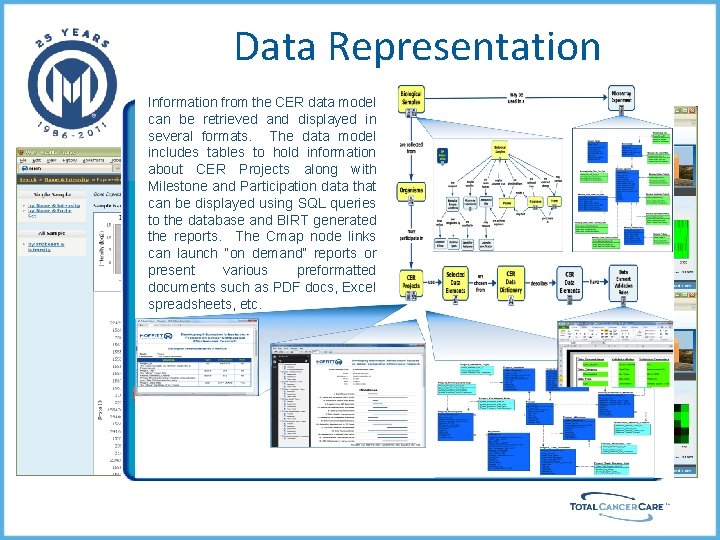
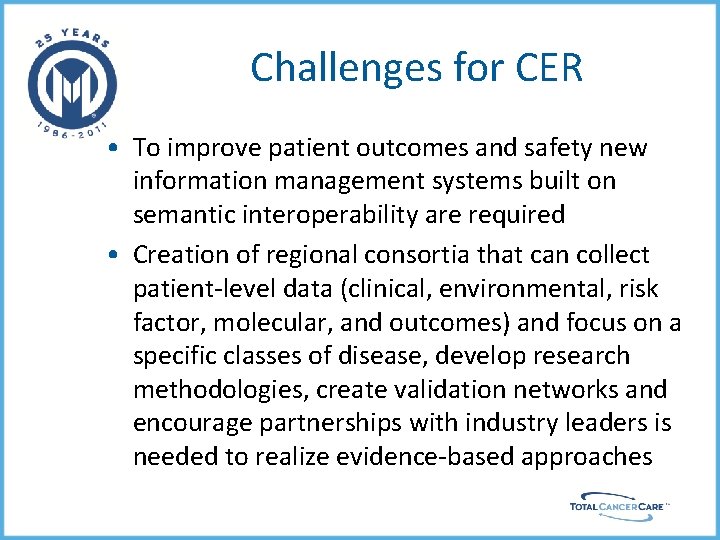
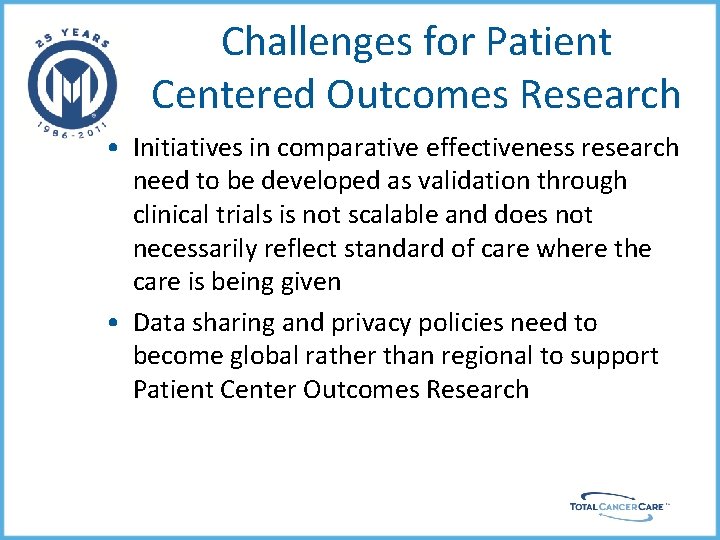
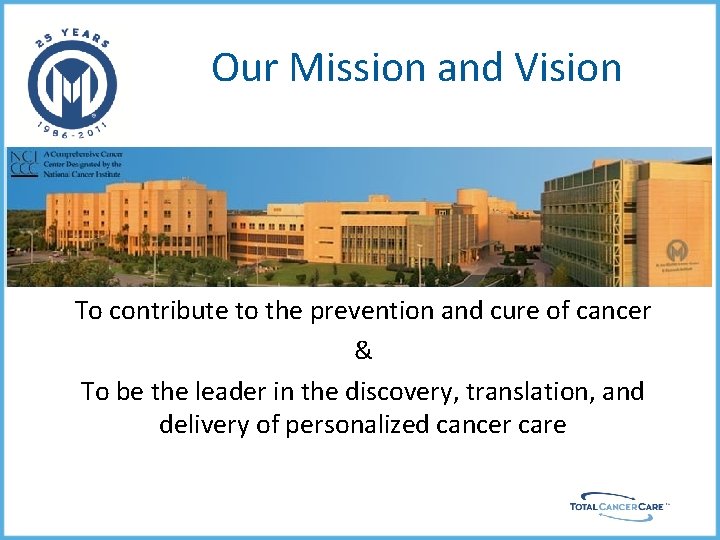
- Slides: 31
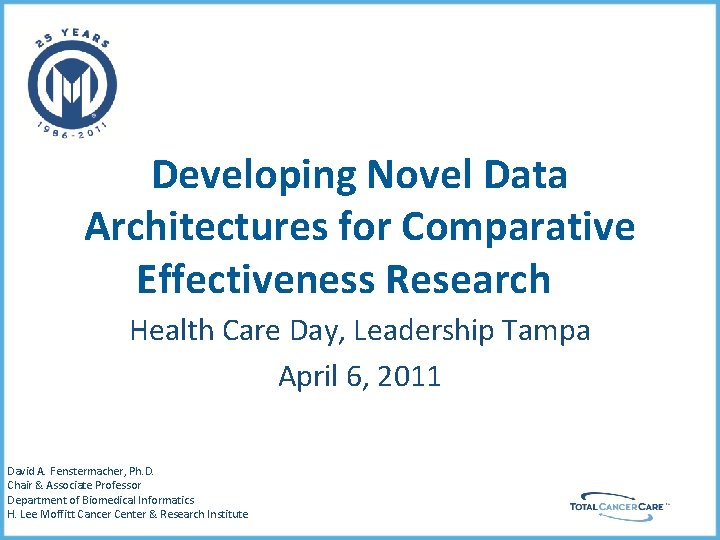
Developing Novel Data Architectures for Comparative Effectiveness Research Health Care Day, Leadership Tampa April 6, 2011 David A. Fenstermacher, Ph. D. Chair & Associate Professor Department of Biomedical Informatics H. Lee Moffitt Cancer Center & Research Institute
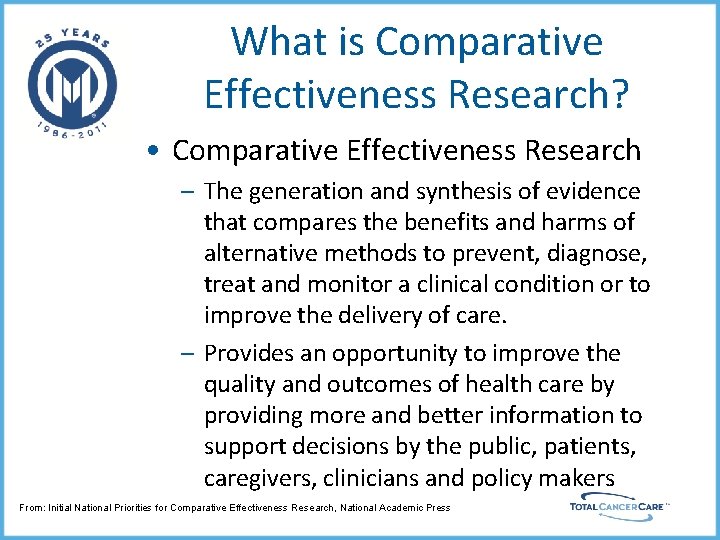
What is Comparative Effectiveness Research? • Comparative Effectiveness Research – The generation and synthesis of evidence that compares the benefits and harms of alternative methods to prevent, diagnose, treat and monitor a clinical condition or to improve the delivery of care. – Provides an opportunity to improve the quality and outcomes of health care by providing more and better information to support decisions by the public, patients, caregivers, clinicians and policy makers From: Initial National Priorities for Comparative Effectiveness Research, National Academic Press
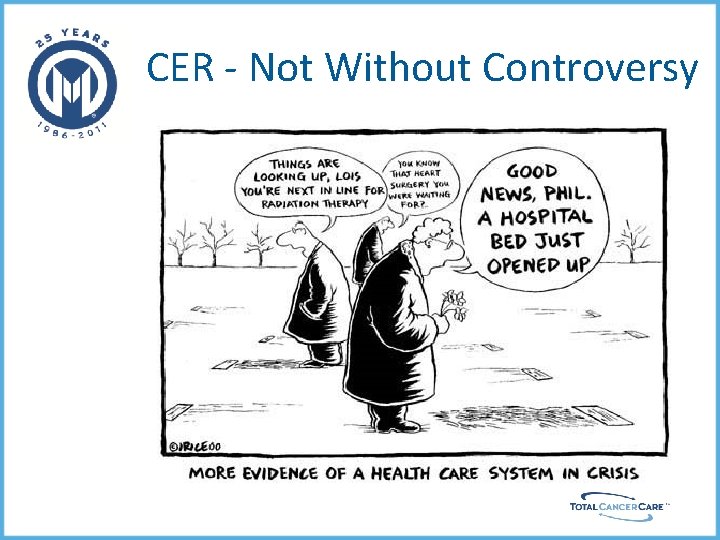
CER - Not Without Controversy
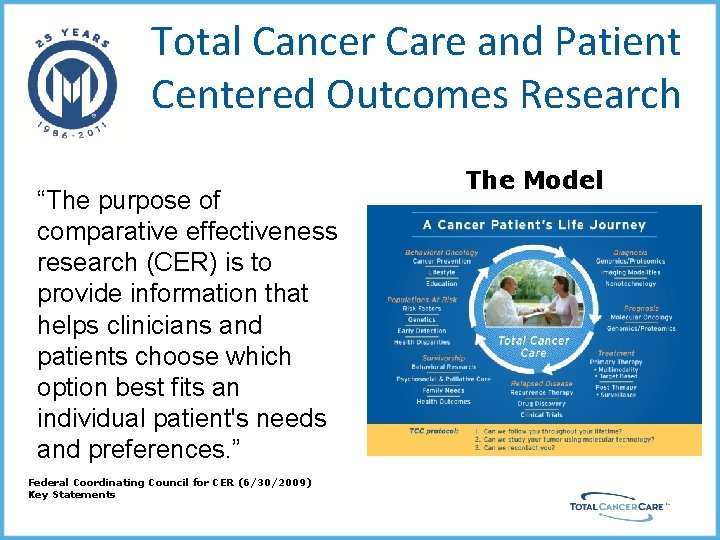
Total Cancer Care and Patient Centered Outcomes Research “The purpose of comparative effectiveness research (CER) is to provide information that helps clinicians and patients choose which option best fits an individual patient's needs and preferences. ” Federal Coordinating Council for CER (6/30/2009) Key Statements The Model
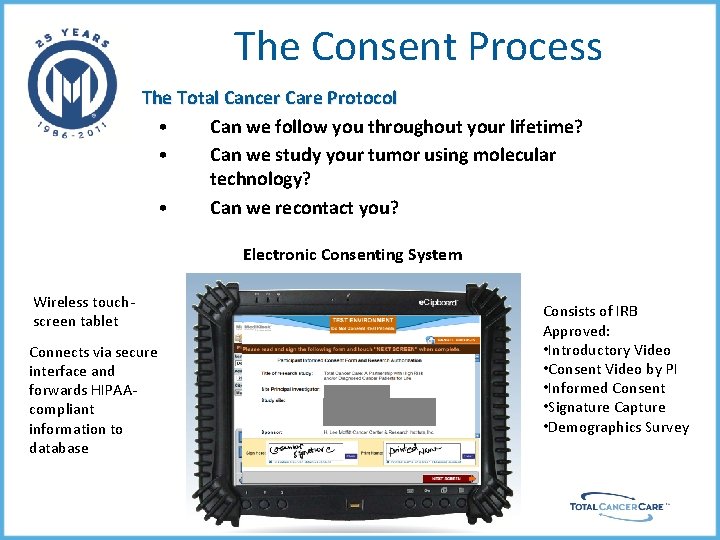
The Consent Process The Total Cancer Care Protocol • Can we follow you throughout your lifetime? • Can we study your tumor using molecular technology? • Can we recontact you? Electronic Consenting System Wireless touch- screen tablet Connects via secure interface and forwards HIPAAcompliant information to database Consists of IRB Approved: • Introductory Video • Consent Video by PI • Informed Consent • Signature Capture • Demographics Survey
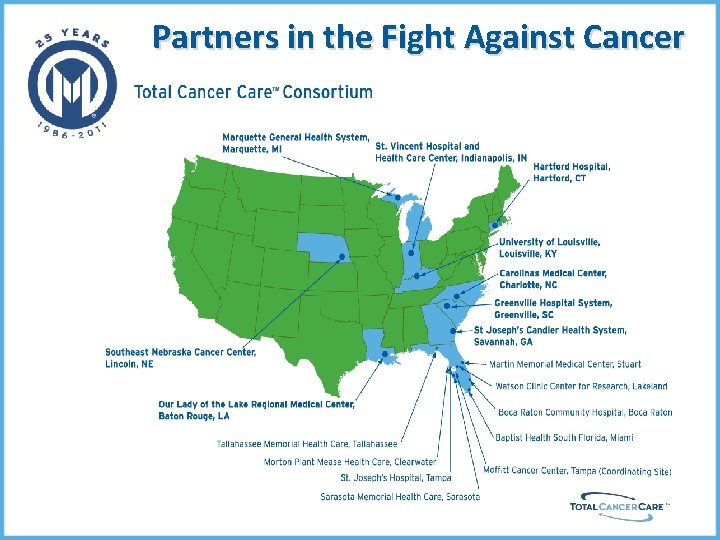
Partners in the Fight Against Cancer
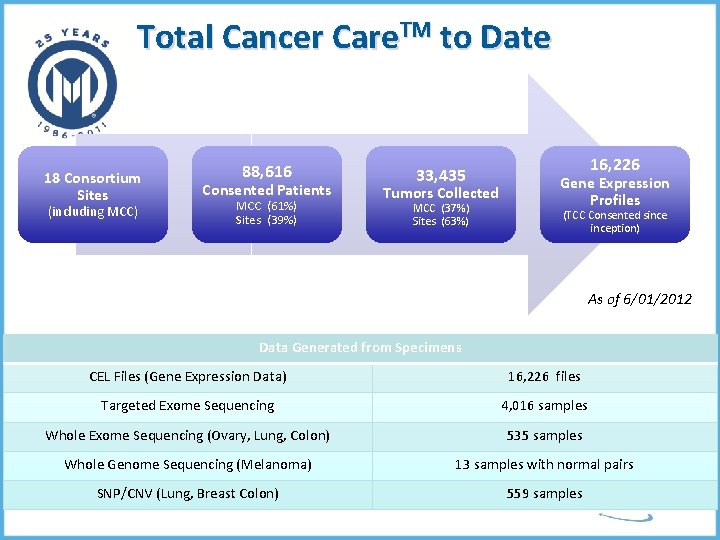
Total Cancer Care. TM to Date 18 Consortium Sites (including MCC) 88, 616 Consented Patients MCC (61%) Sites (39%) 33, 435 Tumors Collected MCC (37%) Sites (63%) 16, 226 Gene Expression Profiles (TCC Consented sinception) As of 6/01/2012 Data Generated from Specimens CEL Files (Gene Expression Data) 16, 226 files Targeted Exome Sequencing 4, 016 samples Whole Exome Sequencing (Ovary, Lung, Colon) 535 samples Whole Genome Sequencing (Melanoma) 13 samples with normal pairs SNP/CNV (Lung, Breast Colon) 559 samples
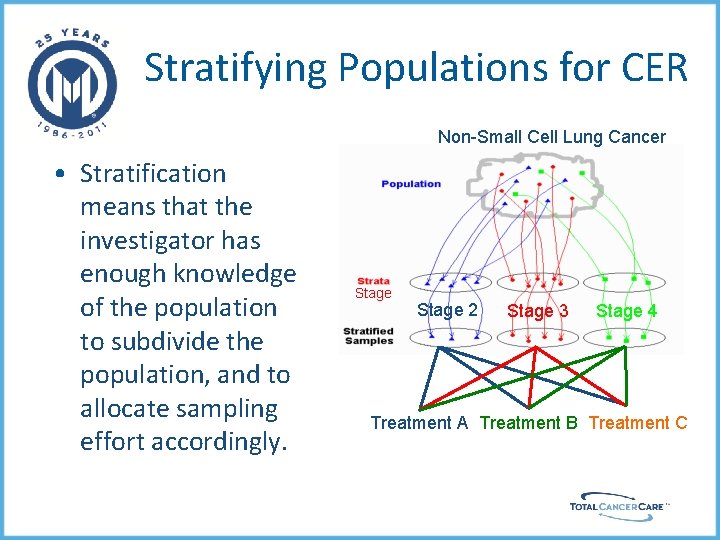
Stratifying Populations for CER Non-Small Cell Lung Cancer • Stratification means that the investigator has enough knowledge of the population to subdivide the population, and to allocate sampling effort accordingly. Stage 2 Stage 3 Stage 4 Treatment A Treatment B Treatment C
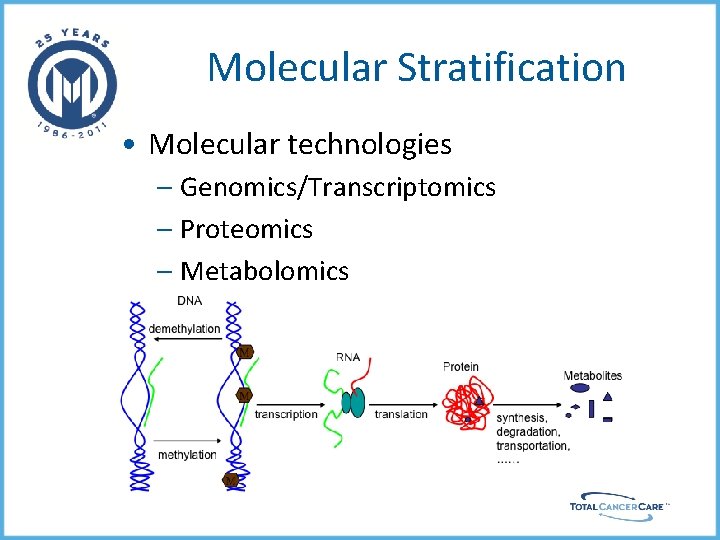
Molecular Stratification • Molecular technologies – Genomics/Transcriptomics – Proteomics – Metabolomics
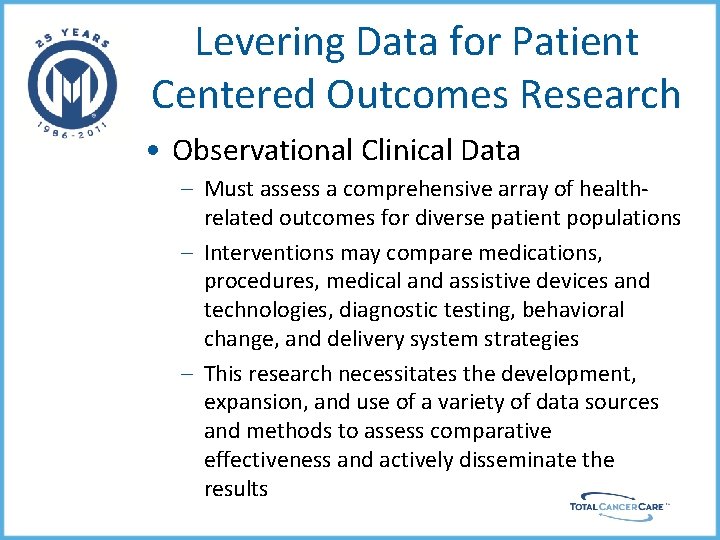
Levering Data for Patient Centered Outcomes Research • Observational Clinical Data – Must assess a comprehensive array of healthrelated outcomes for diverse patient populations – Interventions may compare medications, procedures, medical and assistive devices and technologies, diagnostic testing, behavioral change, and delivery system strategies – This research necessitates the development, expansion, and use of a variety of data sources and methods to assess comparative effectiveness and actively disseminate the results
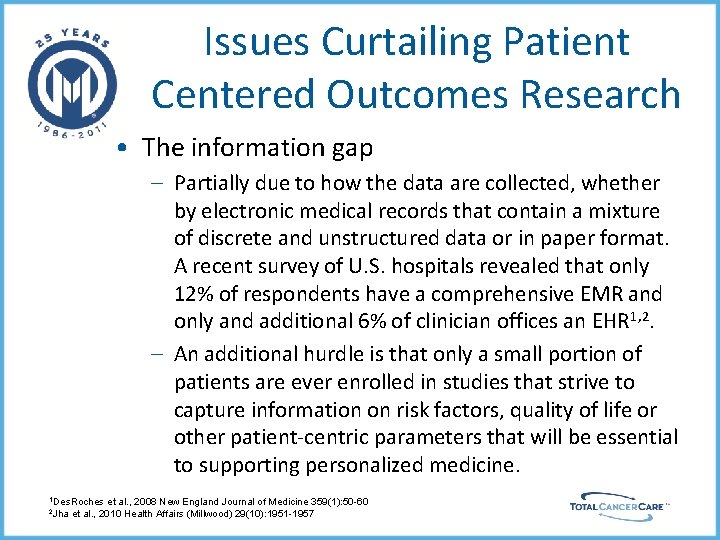
Issues Curtailing Patient Centered Outcomes Research • The information gap – Partially due to how the data are collected, whether by electronic medical records that contain a mixture of discrete and unstructured data or in paper format. A recent survey of U. S. hospitals revealed that only 12% of respondents have a comprehensive EMR and only and additional 6% of clinician offices an EHR 1, 2. – An additional hurdle is that only a small portion of patients are ever enrolled in studies that strive to capture information on risk factors, quality of life or other patient-centric parameters that will be essential to supporting personalized medicine. 1 Des. Roches et al. , 2008 New England Journal of Medicine 359(1): 50 -60 2 Jha et al. , 2010 Health Affairs (Millwood) 29(10): 1951 -1957
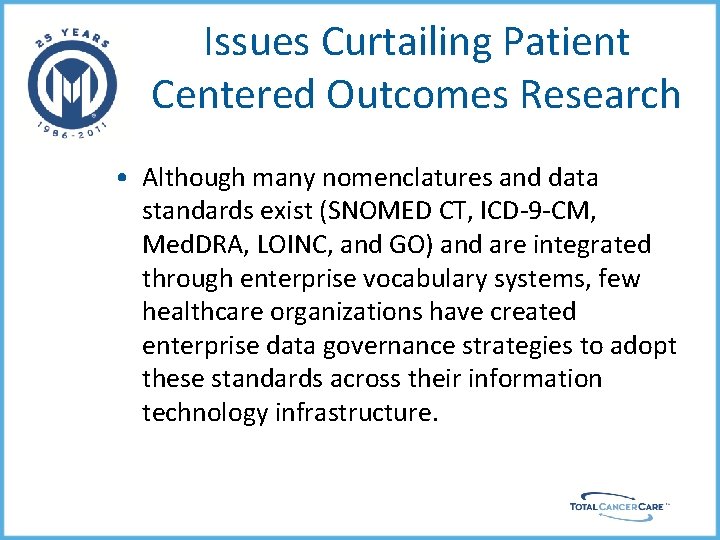
Issues Curtailing Patient Centered Outcomes Research • Although many nomenclatures and data standards exist (SNOMED CT, ICD-9 -CM, Med. DRA, LOINC, and GO) and are integrated through enterprise vocabulary systems, few healthcare organizations have created enterprise data governance strategies to adopt these standards across their information technology infrastructure.
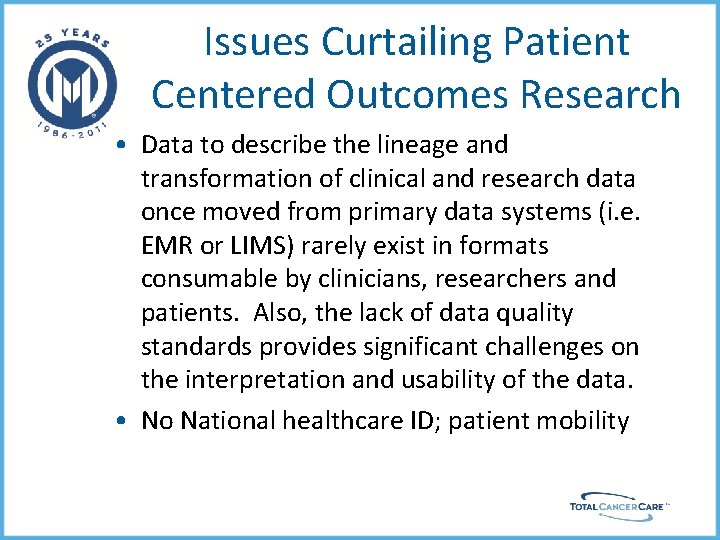
Issues Curtailing Patient Centered Outcomes Research • Data to describe the lineage and transformation of clinical and research data once moved from primary data systems (i. e. EMR or LIMS) rarely exist in formats consumable by clinicians, researchers and patients. Also, the lack of data quality standards provides significant challenges on the interpretation and usability of the data. • No National healthcare ID; patient mobility
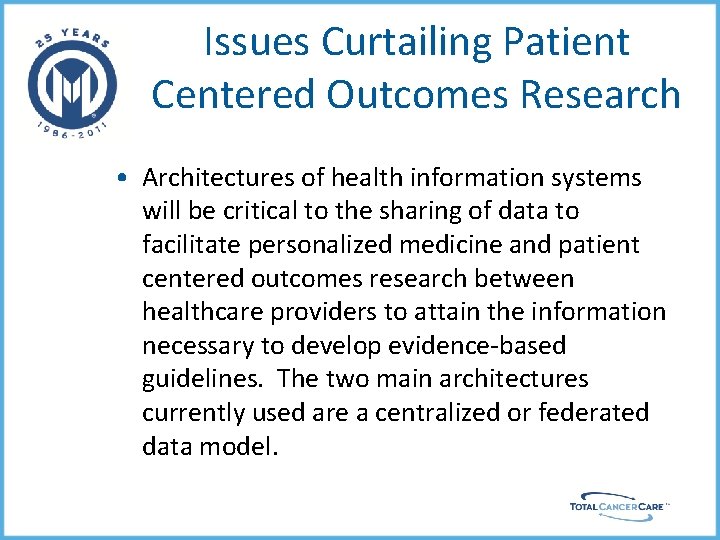
Issues Curtailing Patient Centered Outcomes Research • Architectures of health information systems will be critical to the sharing of data to facilitate personalized medicine and patient centered outcomes research between healthcare providers to attain the information necessary to develop evidence-based guidelines. The two main architectures currently used are a centralized or federated data model.
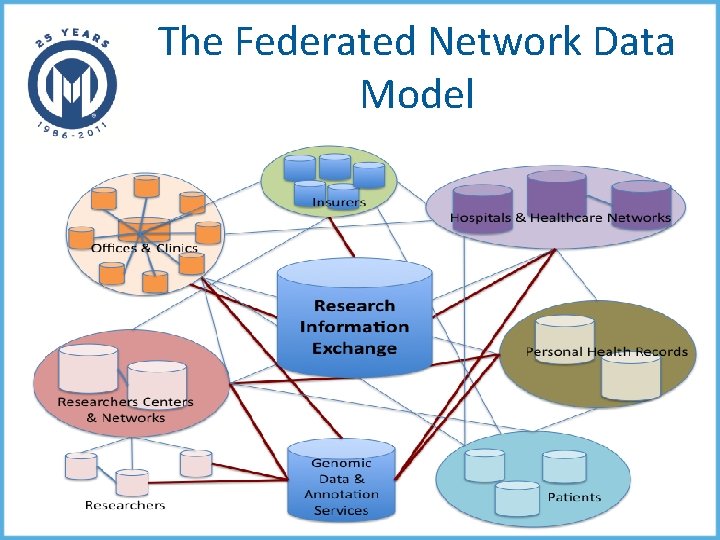
The Federated Network Data Model
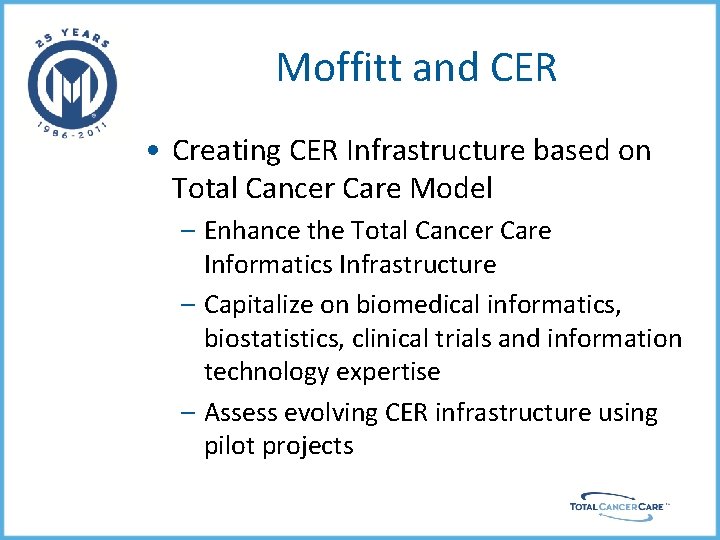
Moffitt and CER • Creating CER Infrastructure based on Total Cancer Care Model – Enhance the Total Cancer Care Informatics Infrastructure – Capitalize on biomedical informatics, biostatistics, clinical trials and information technology expertise – Assess evolving CER infrastructure using pilot projects
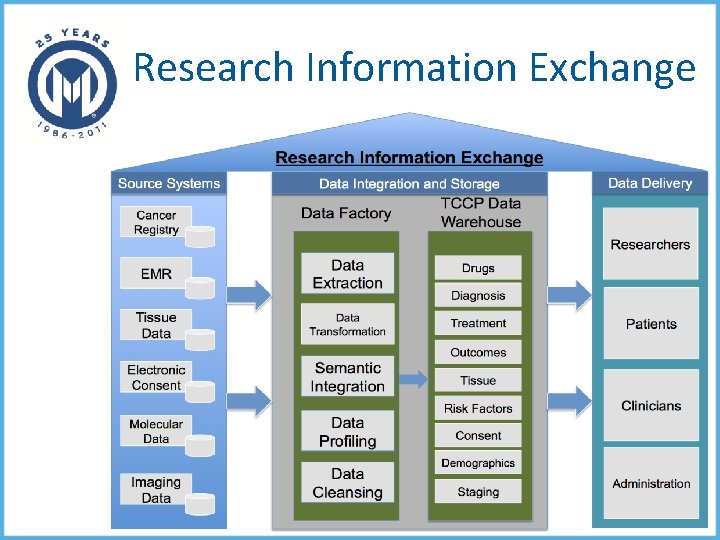
Research Information Exchange
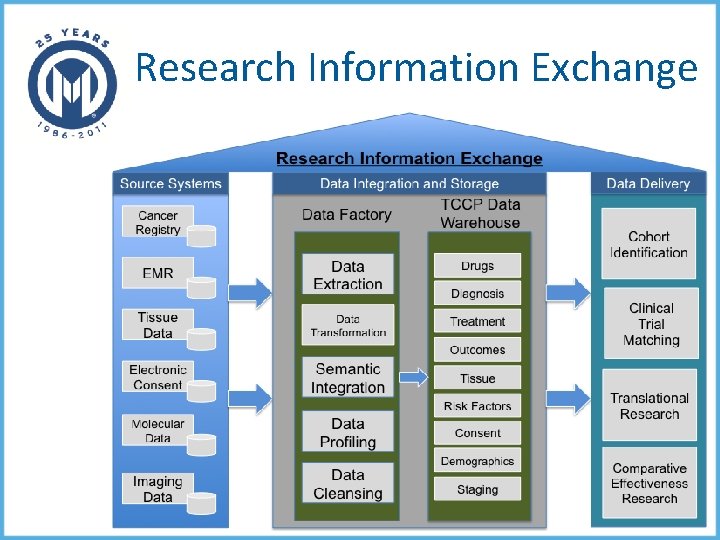
Research Information Exchange
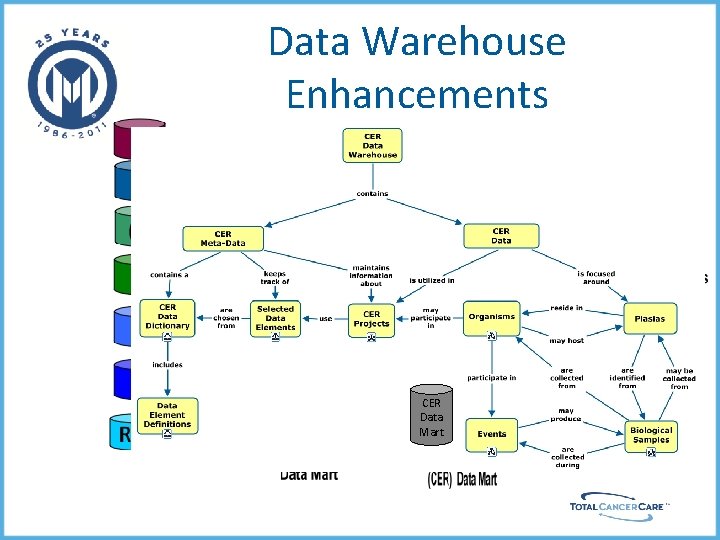
Data Warehouse Enhancements CER Data Mart
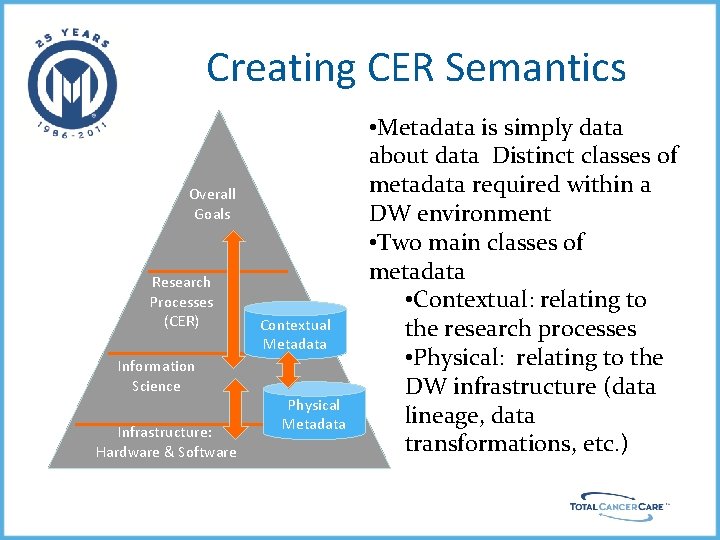
Creating CER Semantics Overall Goals Research Processes (CER) Contextual Metadata Information Science Infrastructure: Hardware & Software Physical Metadata • Metadata is simply data about data Distinct classes of metadata required within a DW environment • Two main classes of metadata • Contextual: relating to the research processes • Physical: relating to the DW infrastructure (data lineage, data transformations, etc. )
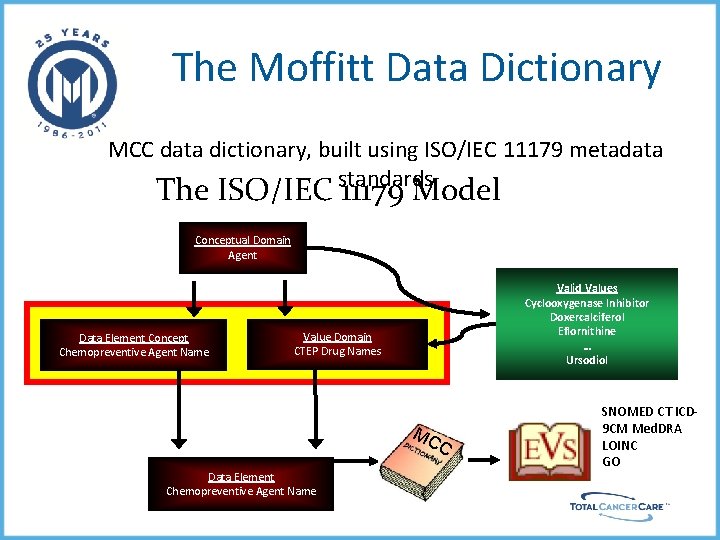
The Moffitt Data Dictionary MCC data dictionary, built using ISO/IEC 11179 metadata standards The ISO/IEC 11179 Model Conceptual Domain Agent Data Element Concept Chemopreventive Agent Name Valid Values Cyclooxygenase Inhibitor Doxercalciferol Eflornithine … Ursodiol Value Domain CTEP Drug Names MC C Data Element Chemopreventive Agent Name SNOMED CT ICD 9 CM Med. DRA LOINC GO
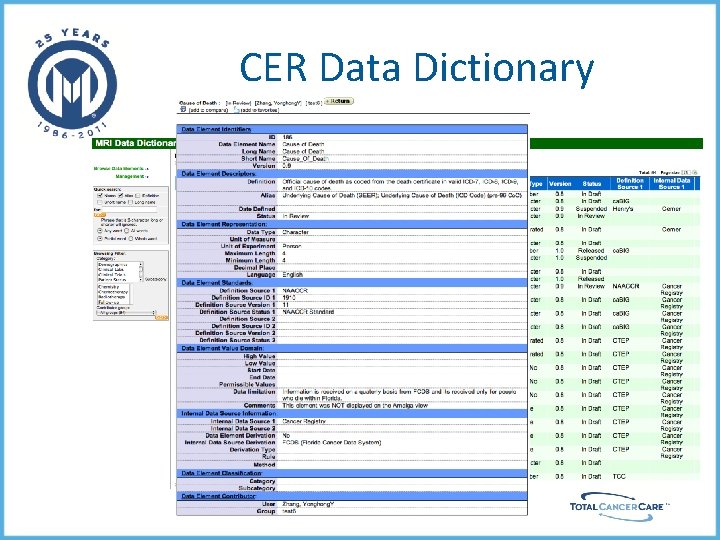
CER Data Dictionary
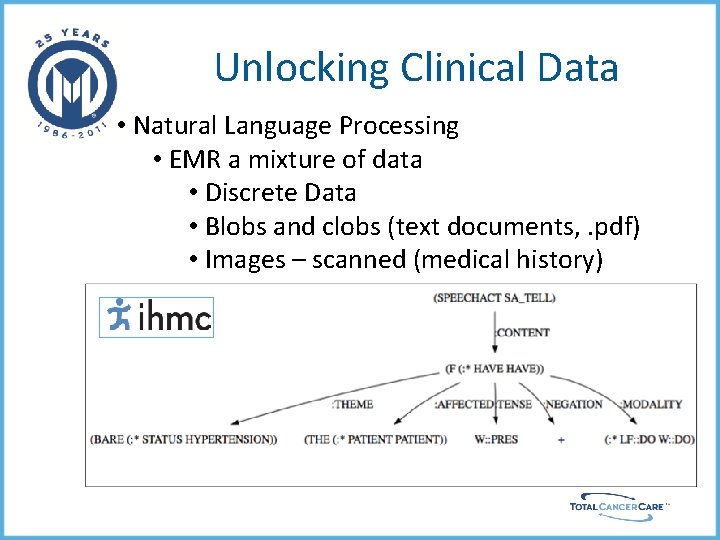
Unlocking Clinical Data • Natural Language Processing • EMR a mixture of data • Discrete Data • Blobs and clobs (text documents, . pdf) • Images – scanned (medical history)
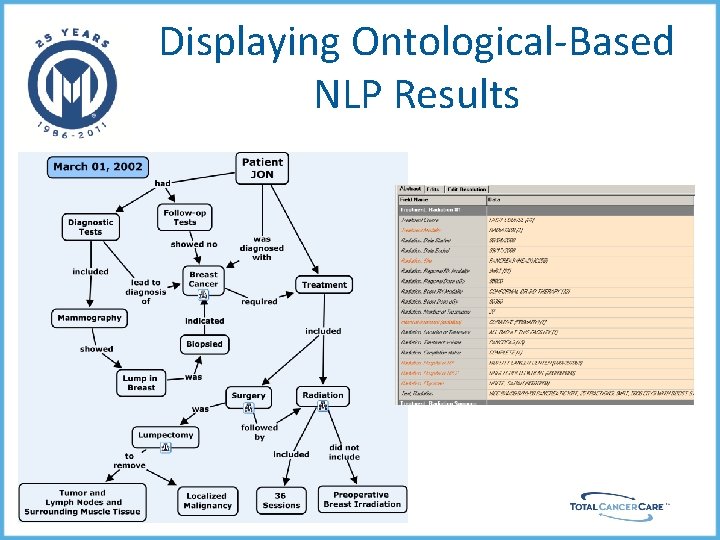
Displaying Ontological-Based NLP Results
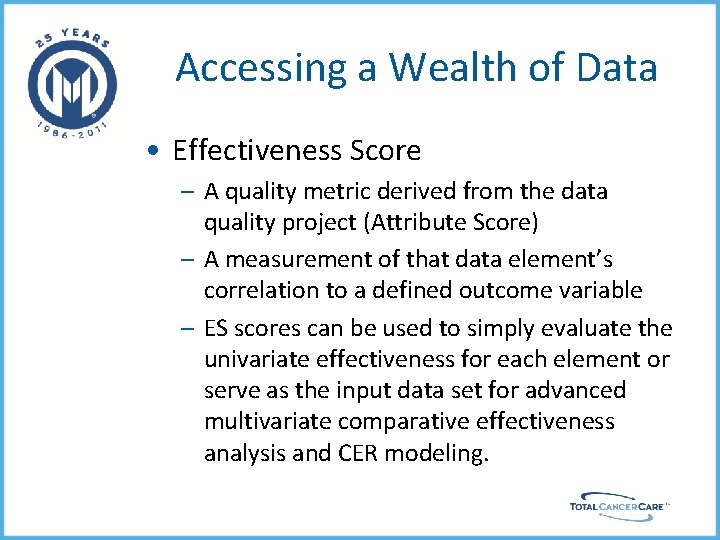
Accessing a Wealth of Data • Effectiveness Score – A quality metric derived from the data quality project (Attribute Score) – A measurement of that data element’s correlation to a defined outcome variable – ES scores can be used to simply evaluate the univariate effectiveness for each element or serve as the input data set for advanced multivariate comparative effectiveness analysis and CER modeling.
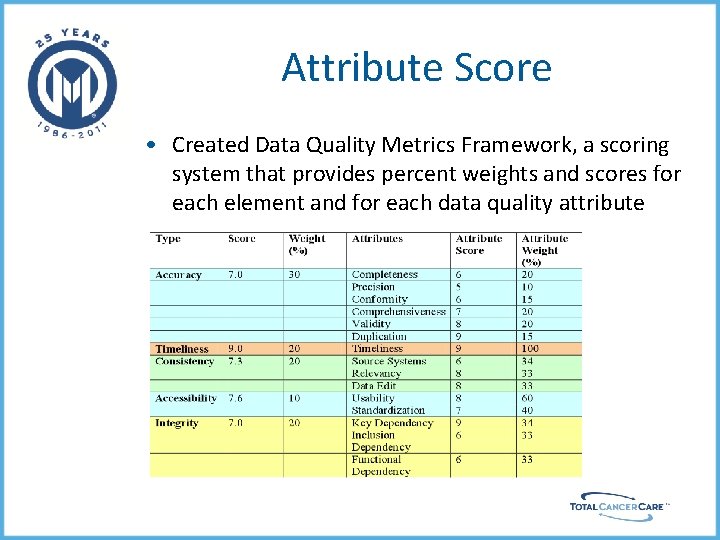
Attribute Score • Created Data Quality Metrics Framework, a scoring system that provides percent weights and scores for each element and for each data quality attribute
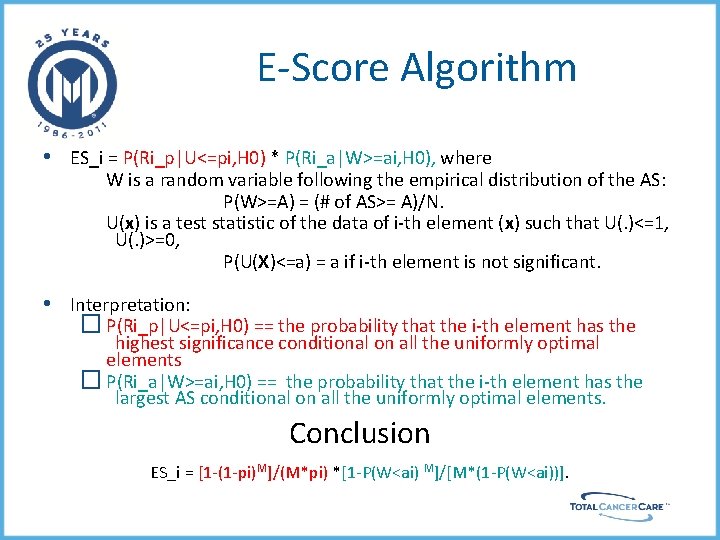
E-Score Algorithm • ES_i = P(Ri_p|U<=pi, H 0) * P(Ri_a|W>=ai, H 0), where W is a random variable following the empirical distribution of the AS: P(W>=A) = (# of AS>= A)/N. U(x) is a test statistic of the data of i-th element (x) such that U(. )<=1, U(. )>=0, P(U(X)<=a) = a if i-th element is not significant. • Interpretation: � P(Ri_p|U<=pi, H 0) == the probability that the i-th element has the highest significance conditional on all the uniformly optimal elements � P(Ri_a|W>=ai, H 0) == the probability that the i-th element has the largest AS conditional on all the uniformly optimal elements. Conclusion ES_i = [1 -(1 -pi)M]/(M*pi) *[1 -P(W<ai) M]/[M*(1 -P(W<ai))].
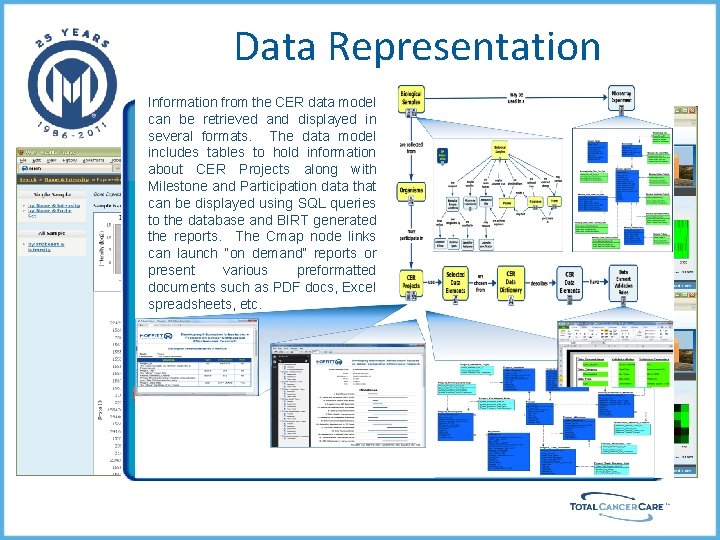
Data Representation Information from the CER data model can be retrieved and displayed in several formats. The data model includes tables to hold information about CER Projects along with Milestone and Participation data that can be displayed using SQL queries to the database and BIRT generated the reports. The Cmap node links can launch “on demand” reports or present various preformatted documents such as PDF docs, Excel spreadsheets, etc.
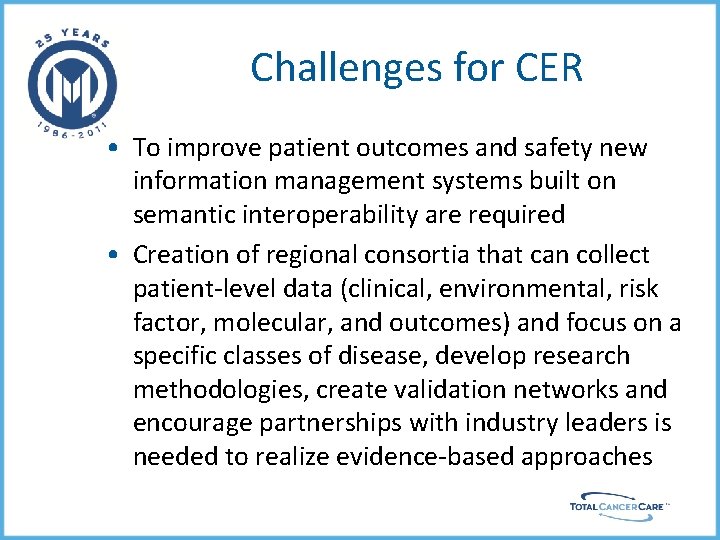
Challenges for CER • To improve patient outcomes and safety new information management systems built on semantic interoperability are required • Creation of regional consortia that can collect patient-level data (clinical, environmental, risk factor, molecular, and outcomes) and focus on a specific classes of disease, develop research methodologies, create validation networks and encourage partnerships with industry leaders is needed to realize evidence-based approaches
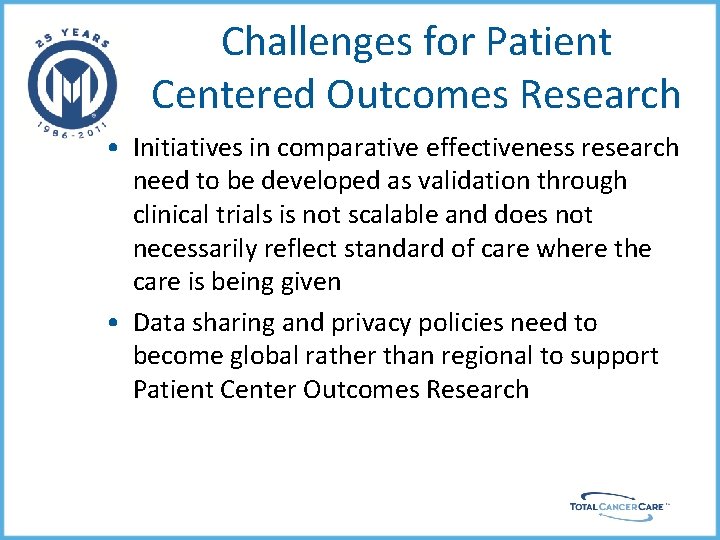
Challenges for Patient Centered Outcomes Research • Initiatives in comparative effectiveness research need to be developed as validation through clinical trials is not scalable and does not necessarily reflect standard of care where the care is being given • Data sharing and privacy policies need to become global rather than regional to support Patient Center Outcomes Research
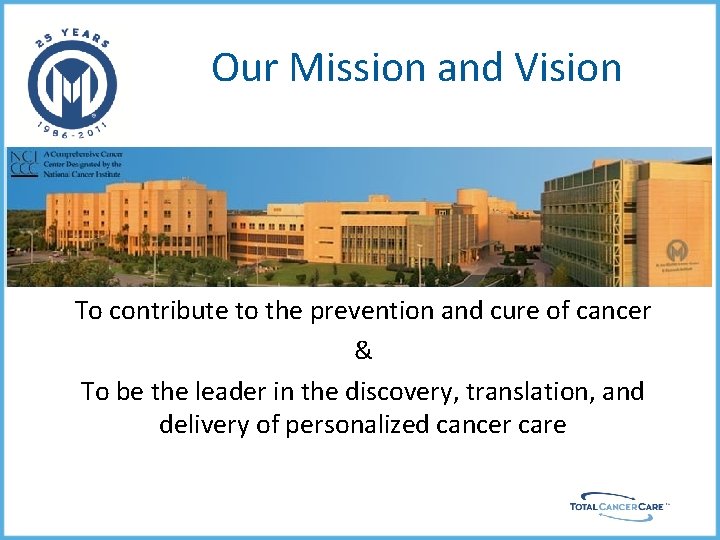
Our Mission and Vision To contribute to the prevention and cure of cancer & To be the leader in the discovery, translation, and delivery of personalized cancer care
Define product architecture
Database storage architecture
Ansi sparc
Switched backbone
Autoencoders
George schlossnagle
Architecture
Gui architectures
Database system architectures
Cdn architectures
Scalable web architectures
Three tier architecture of data warehouse
Computer architecture attributes
Website client server architecture
Distributed systems architectures
Backbone network architectures
Gpu cache coherence
Why systolic architectures
Causal-comparative research design
Comparative education in developing countries
Developing a global vision through marketing research
Developing a global vision through marketing research
Developing effective research proposals
Developing a global vision through marketing research
Developing a global vision through marketing research
Research question examples
Meter data management roadmap
Novel data science applications
Kontinuitetshantering i praktiken
Novell typiska drag
Nationell inriktning för artificiell intelligens
Returpilarna