Design and Analysis of Experiments Lecture 2 2
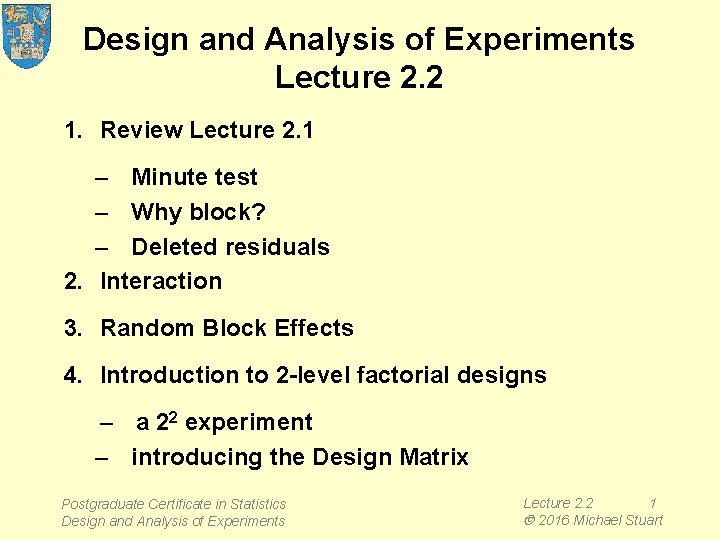
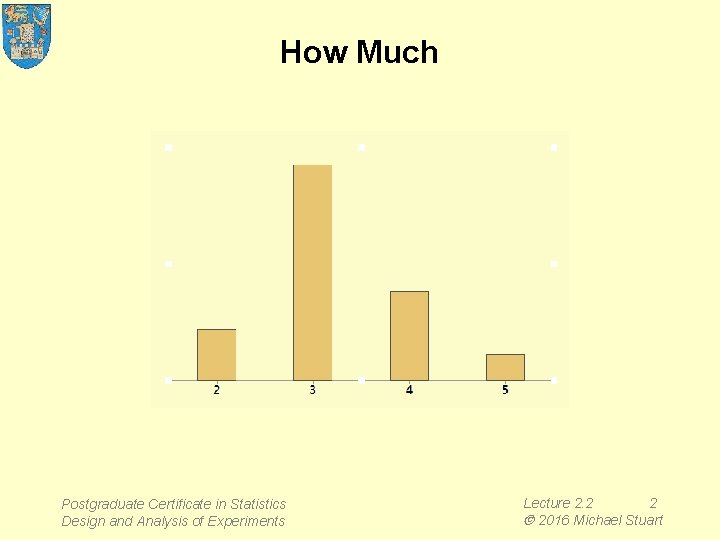
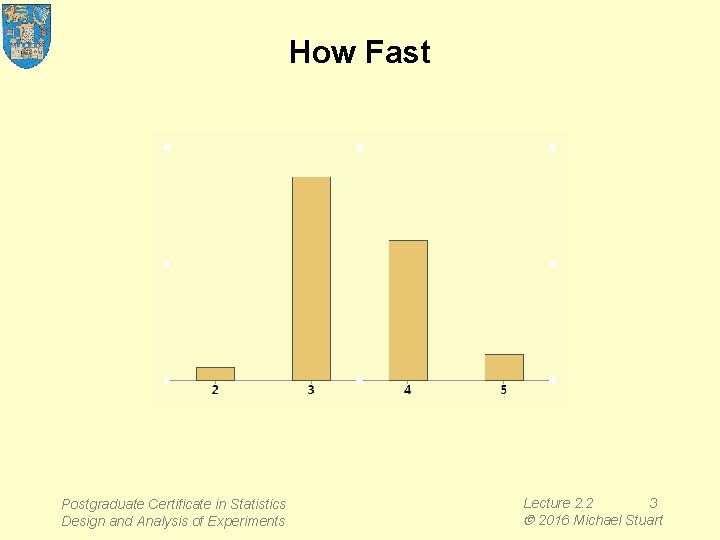
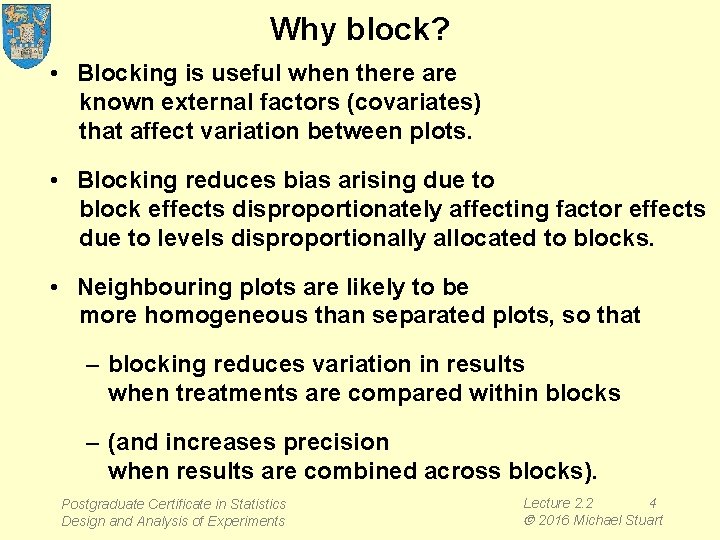
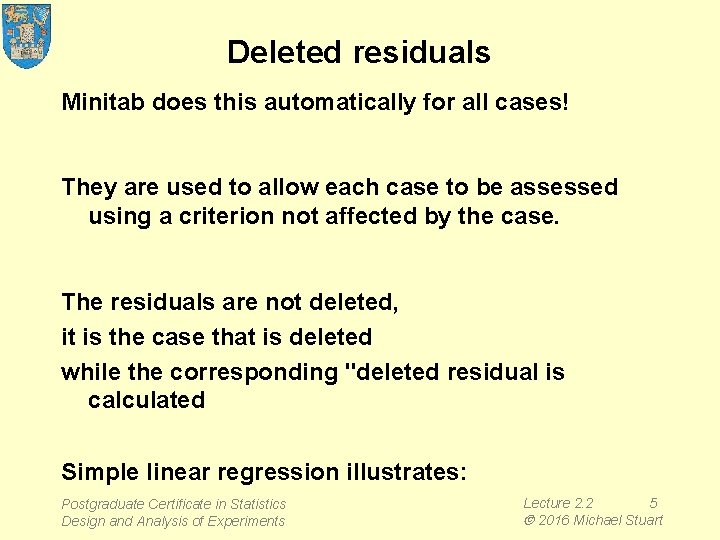
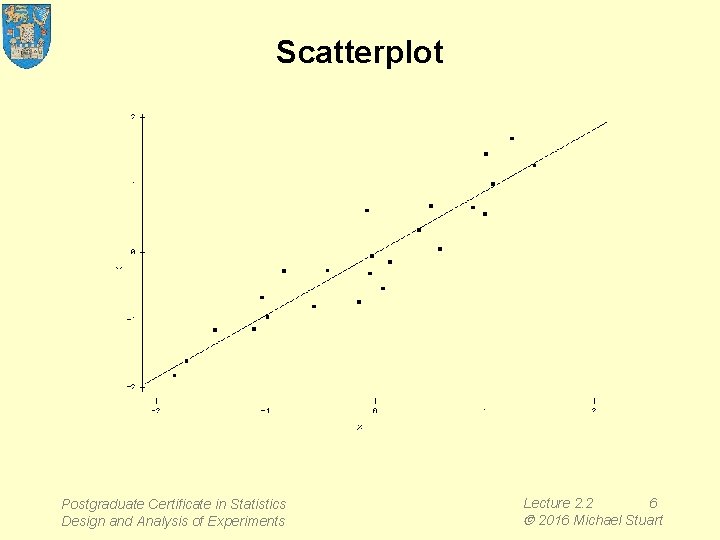
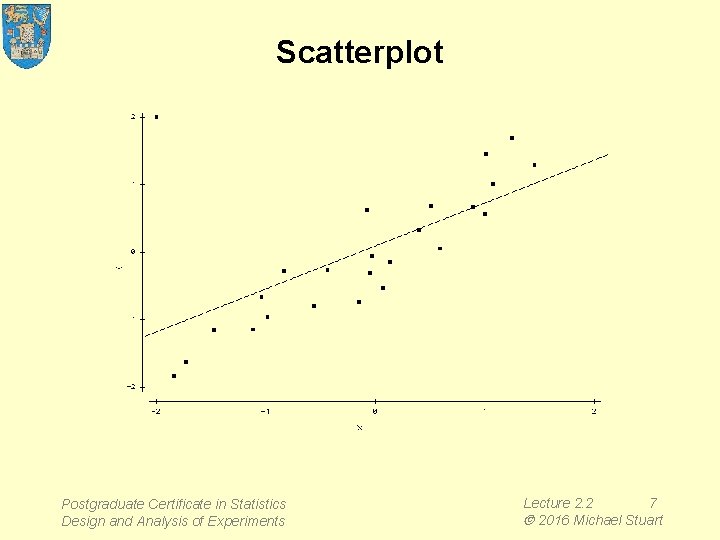
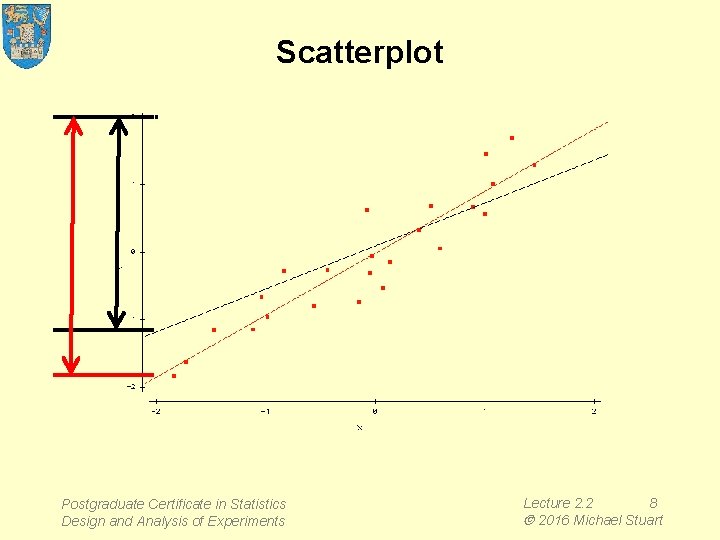
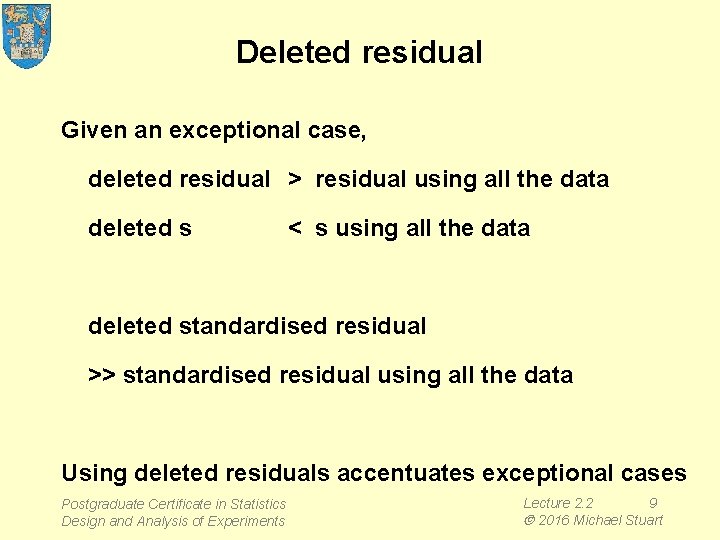
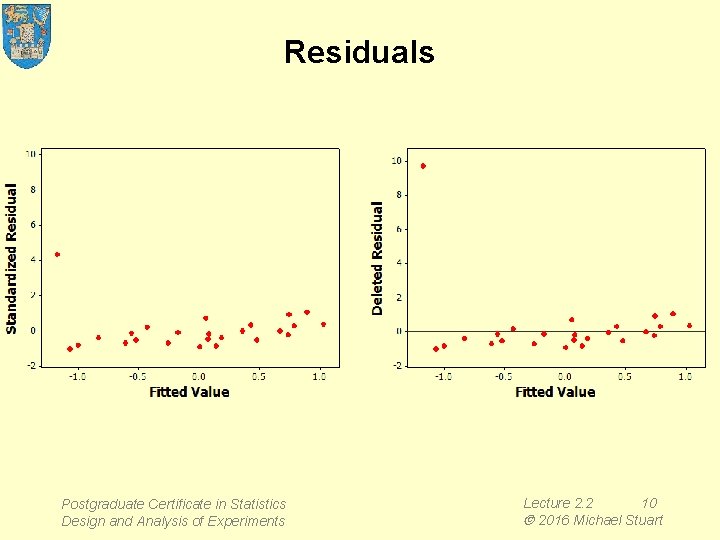
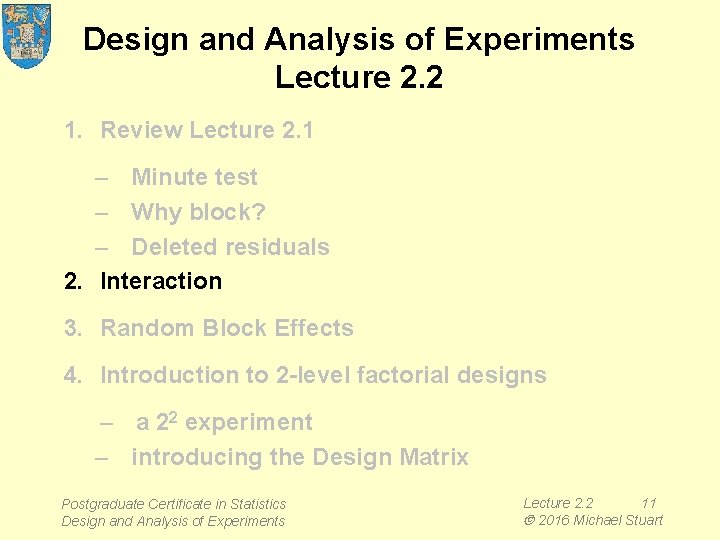
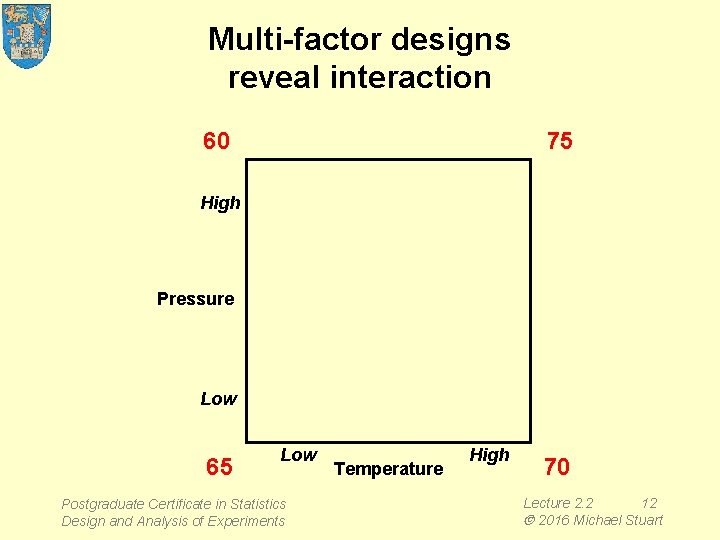
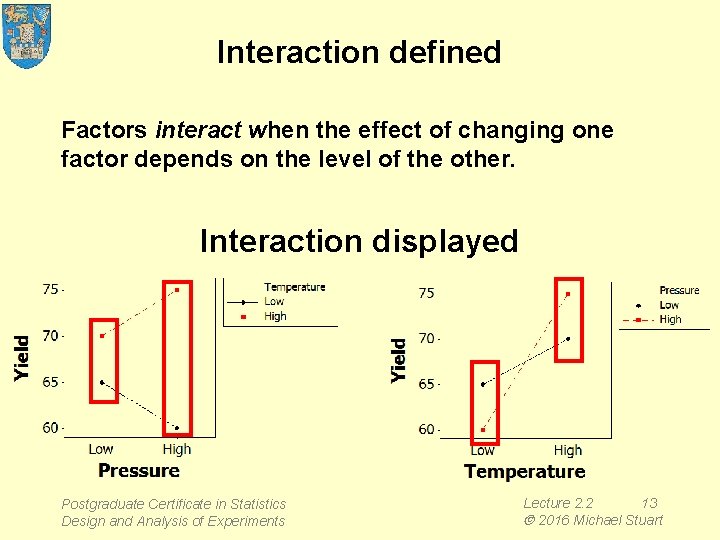
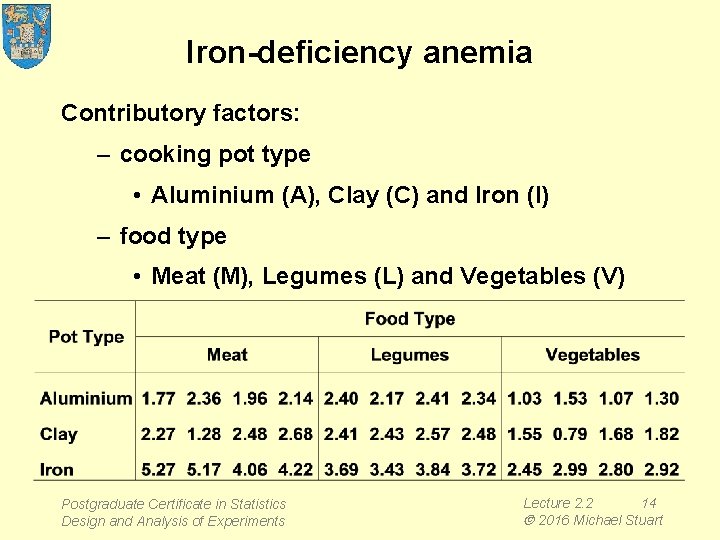
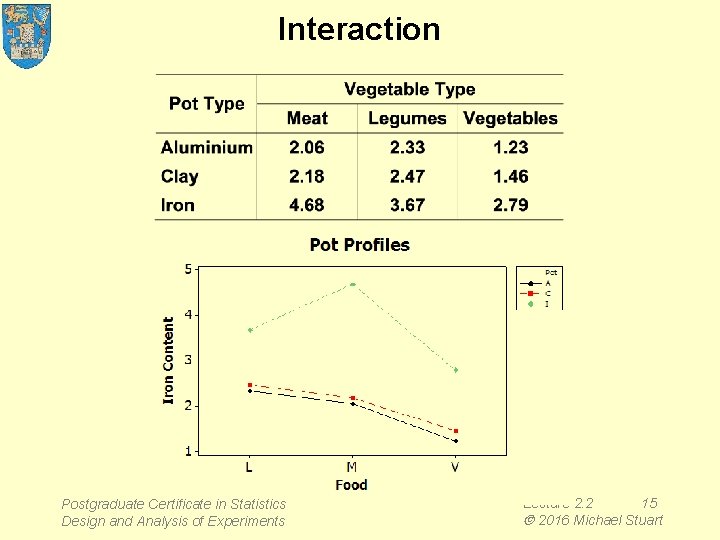
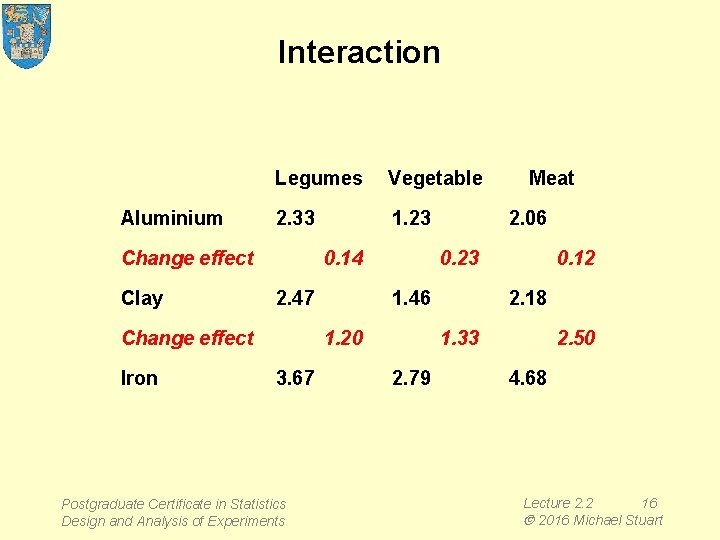
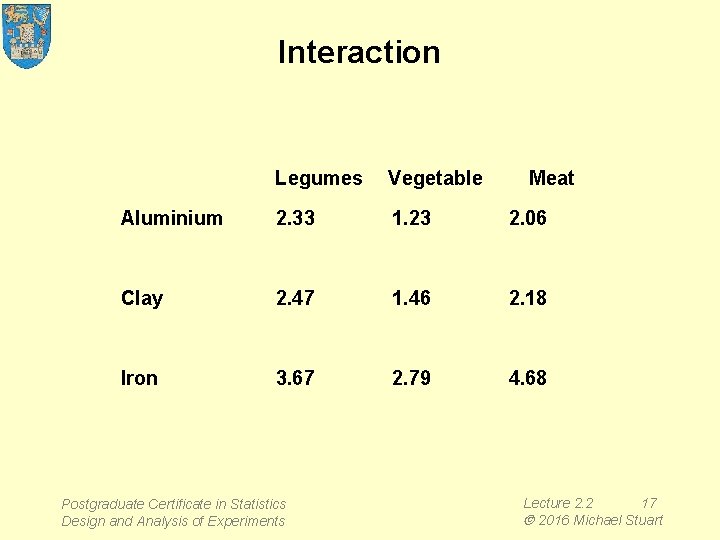
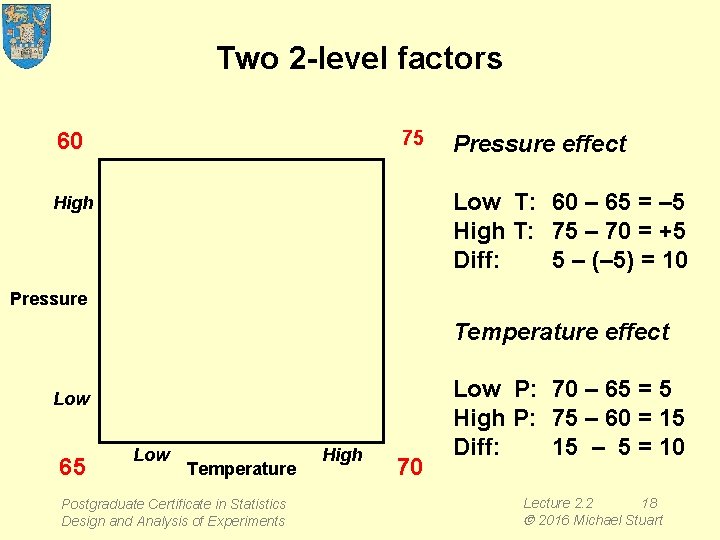
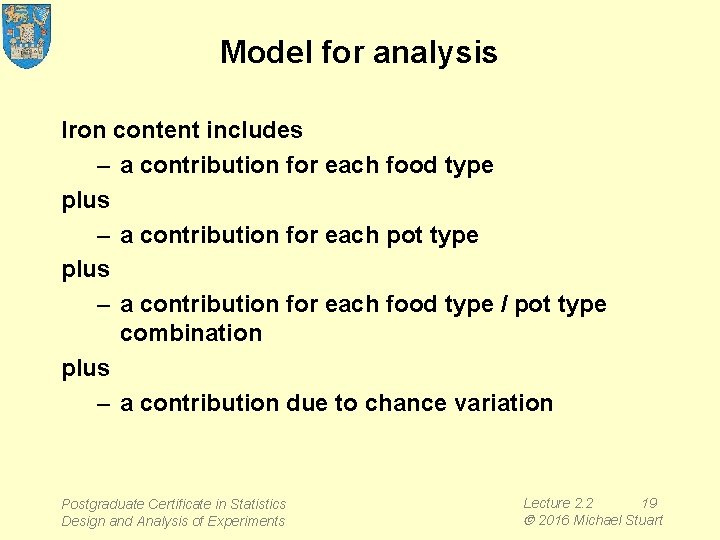
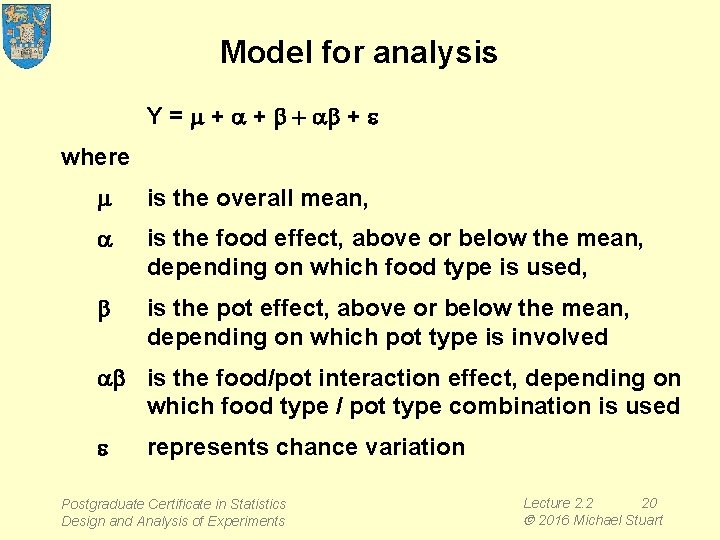
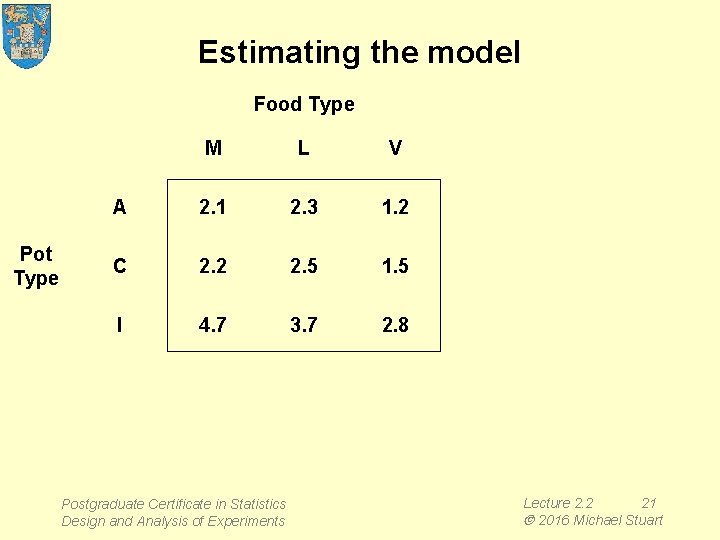
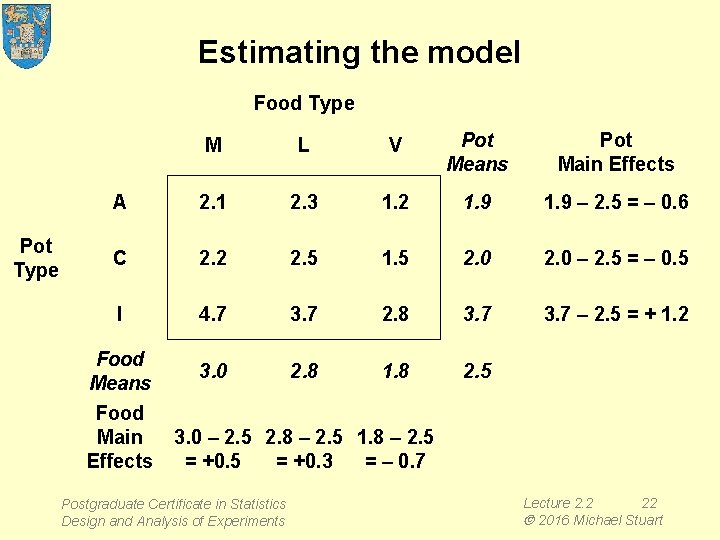
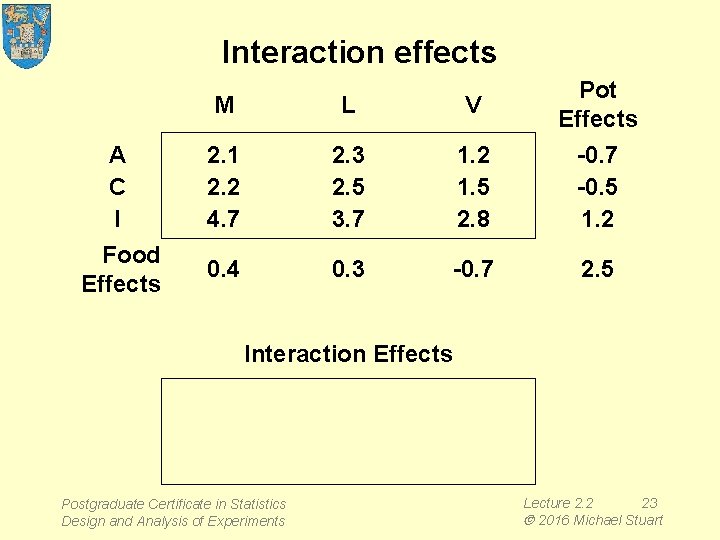
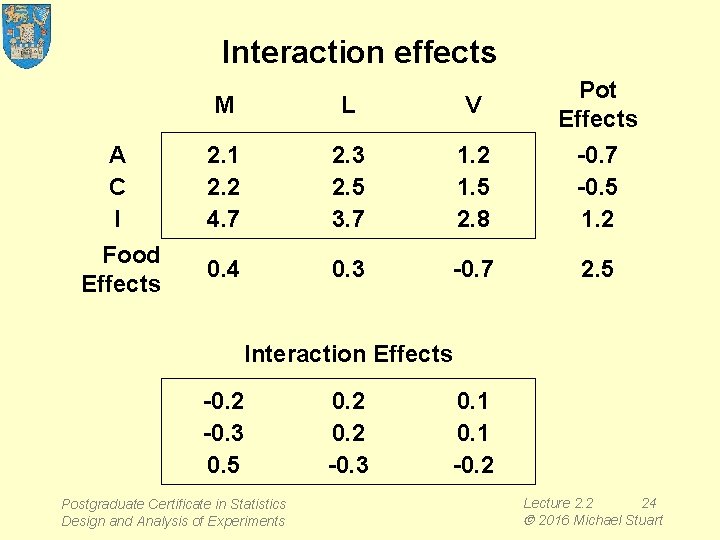
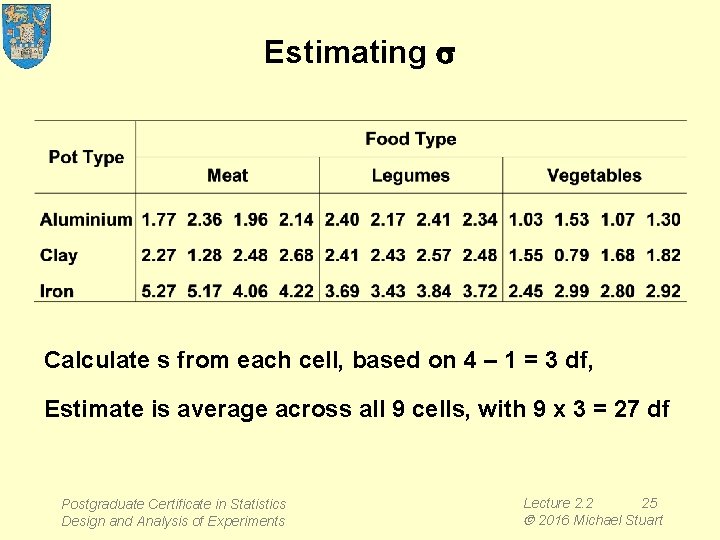
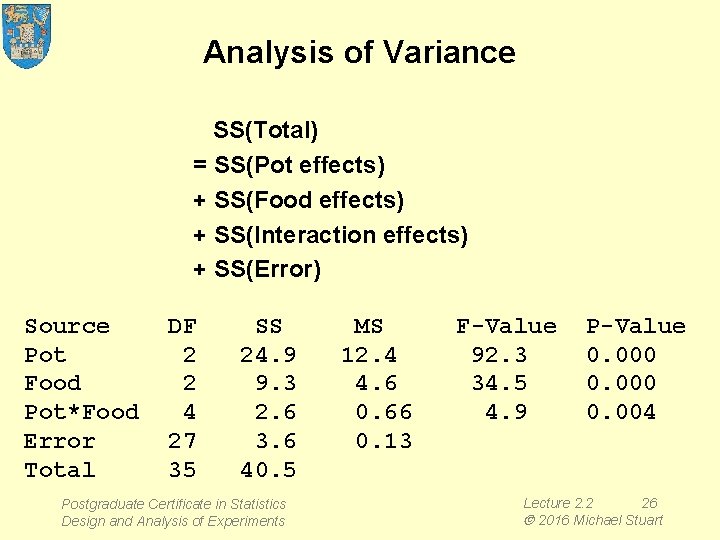
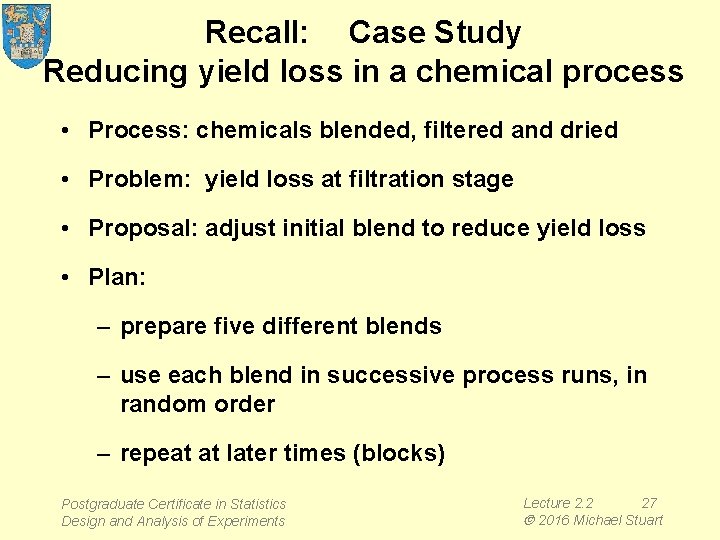
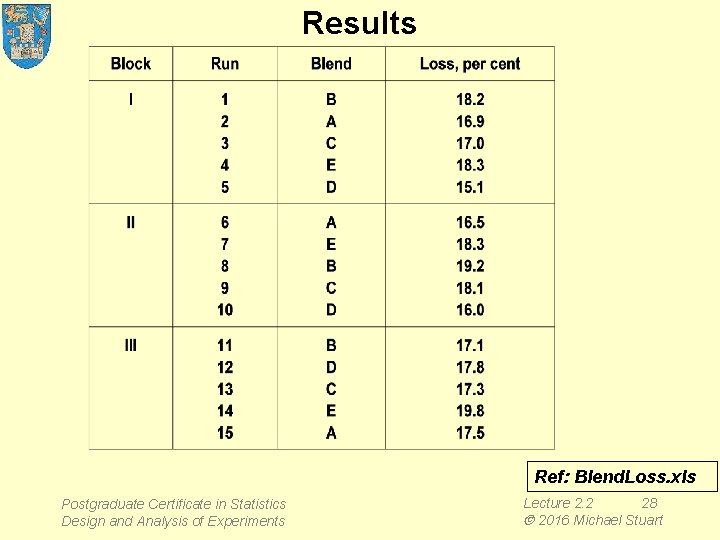
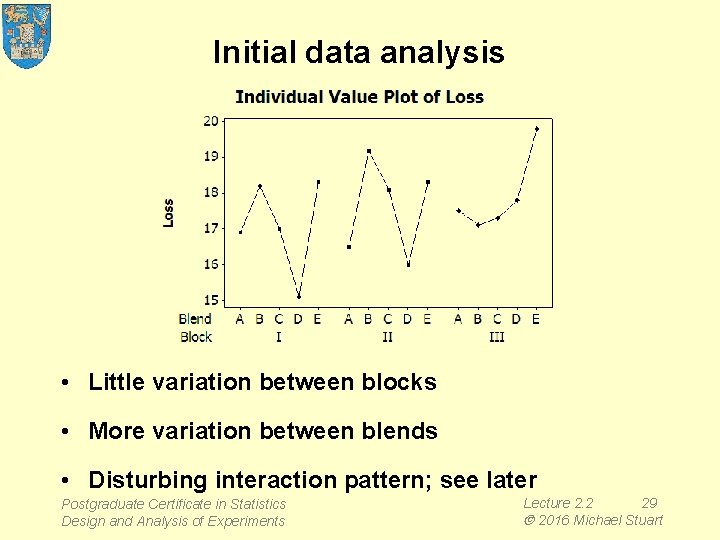
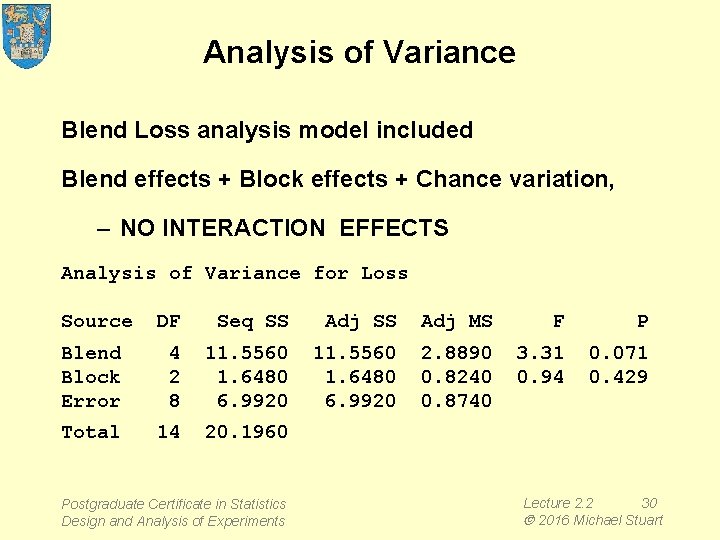
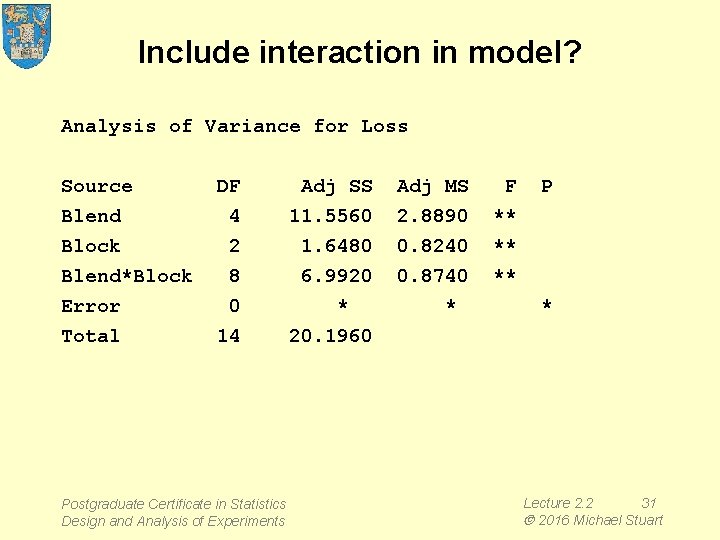
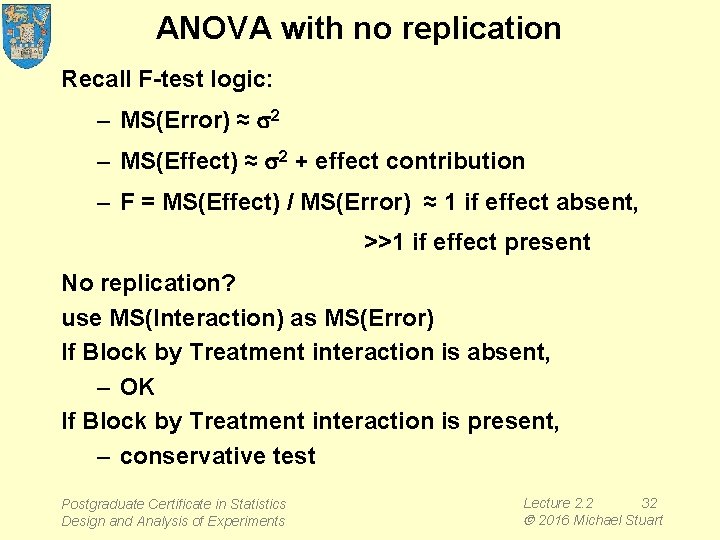
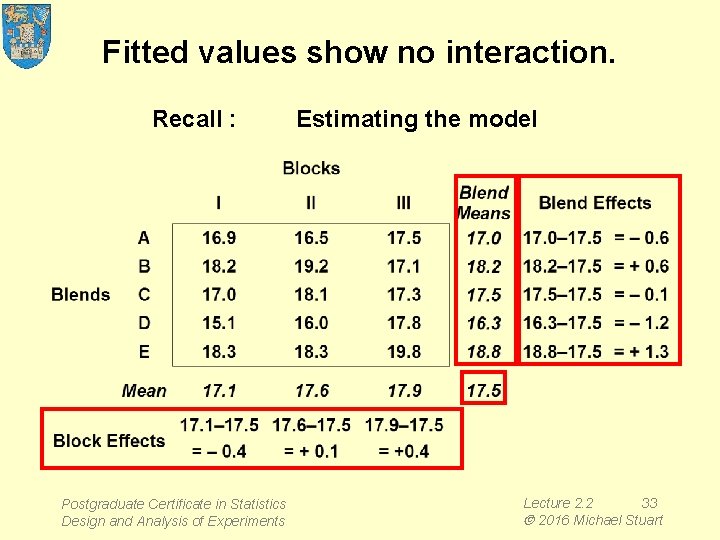
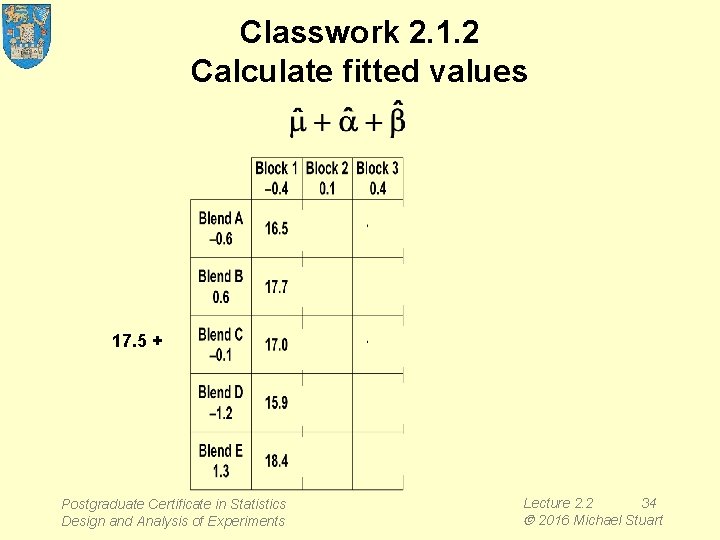
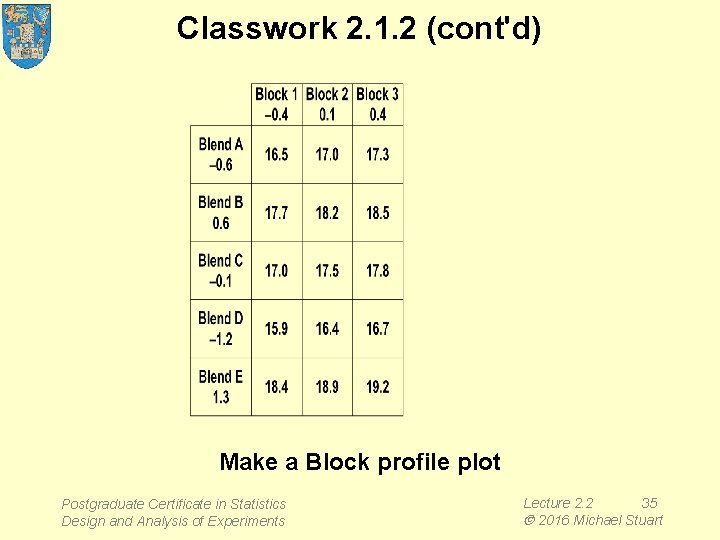
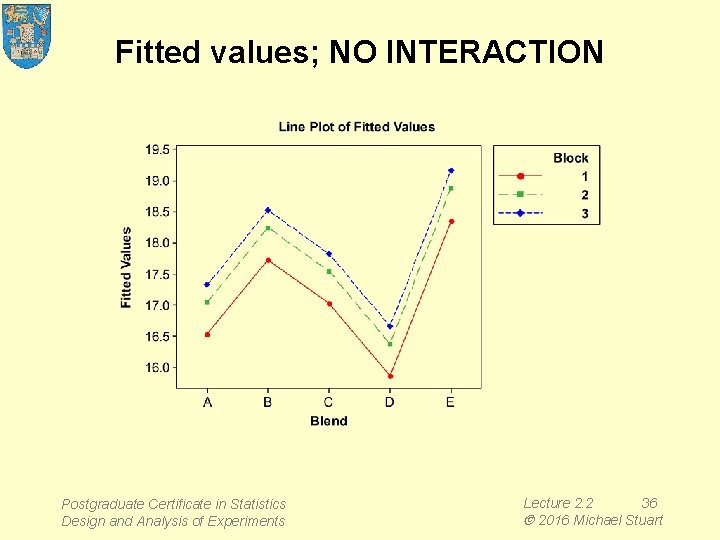
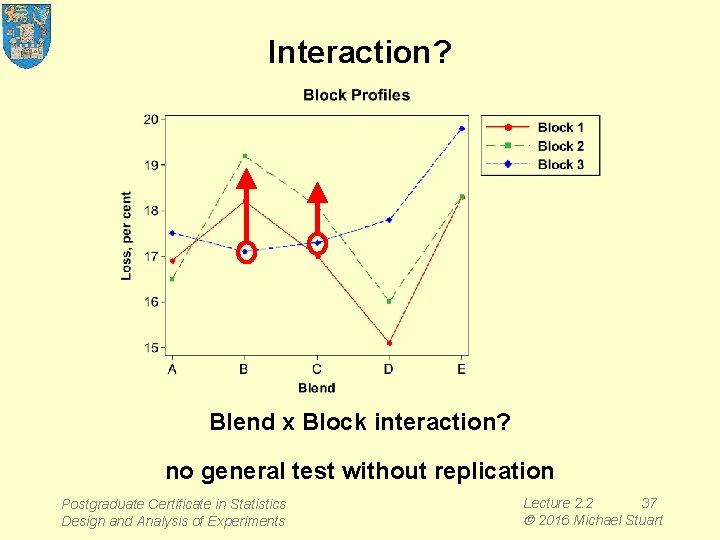
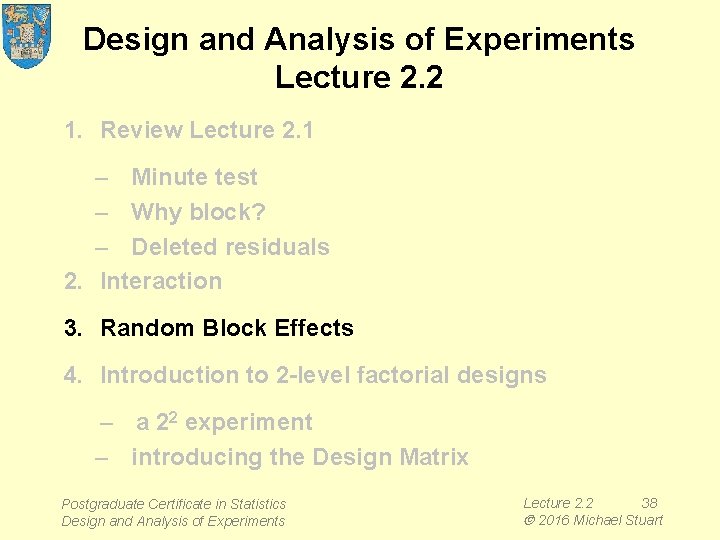
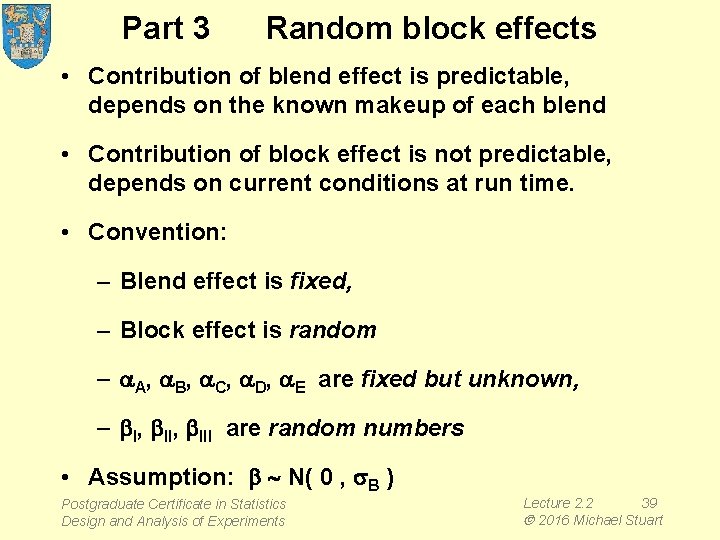
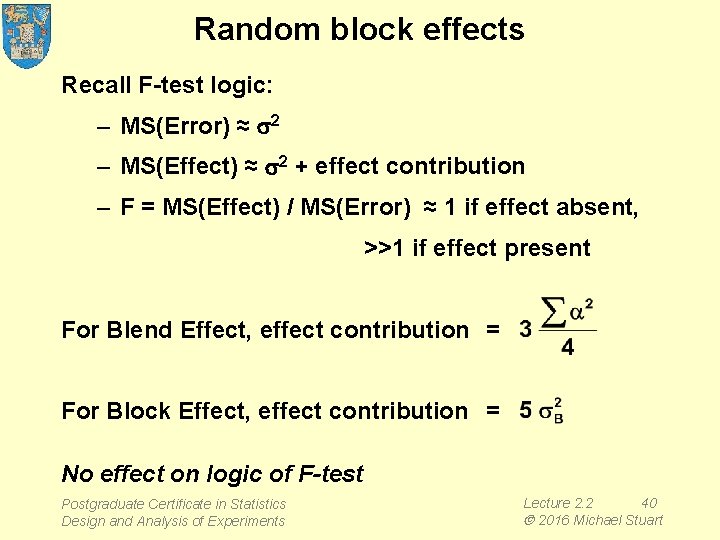
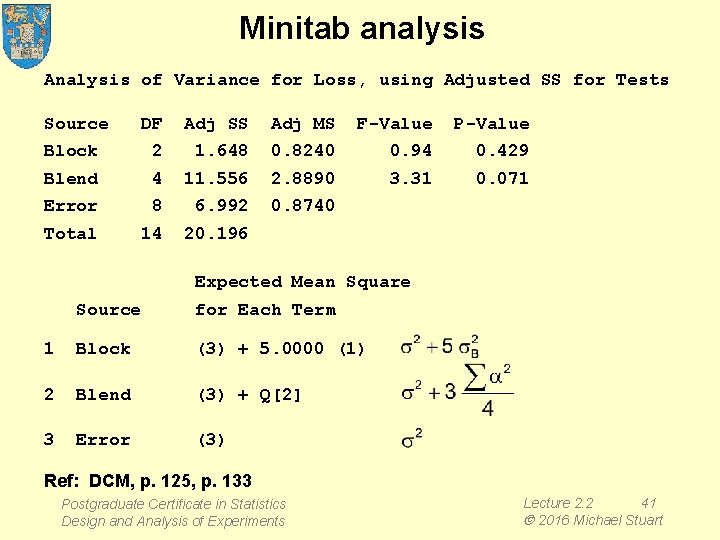
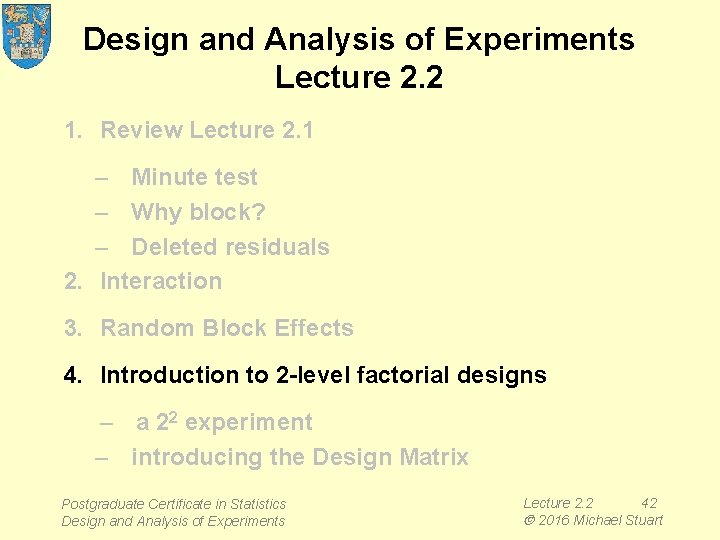
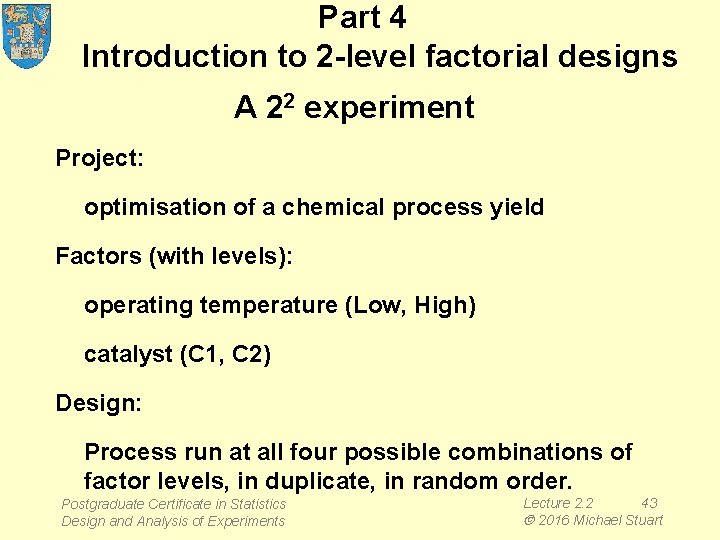
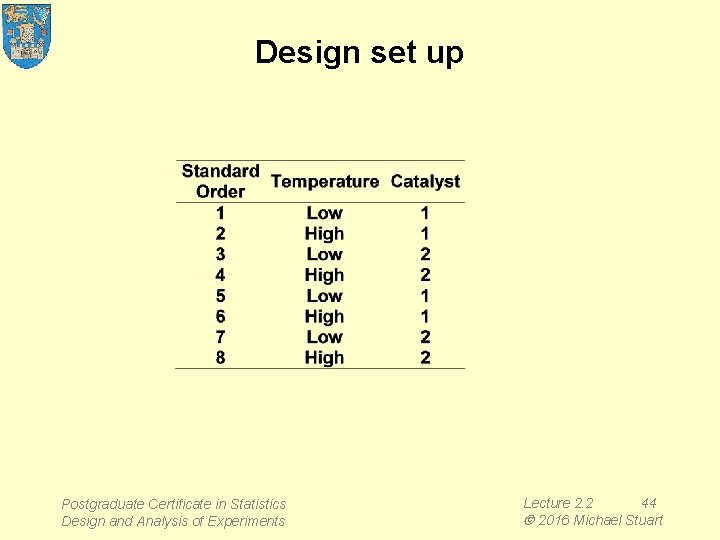
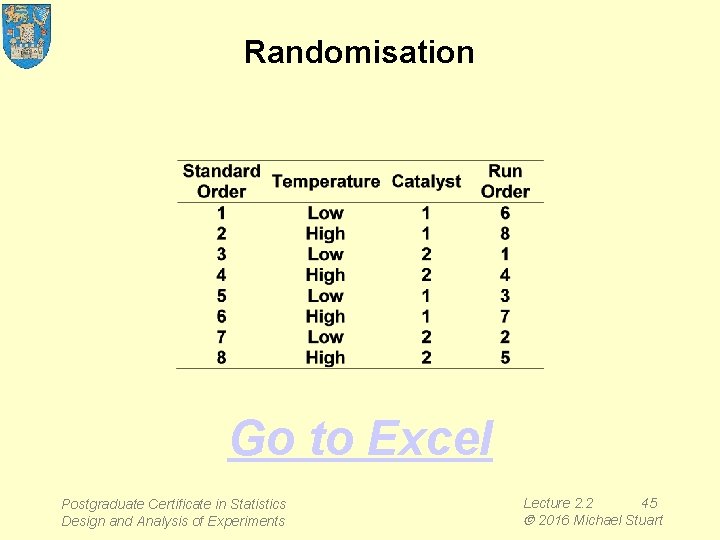
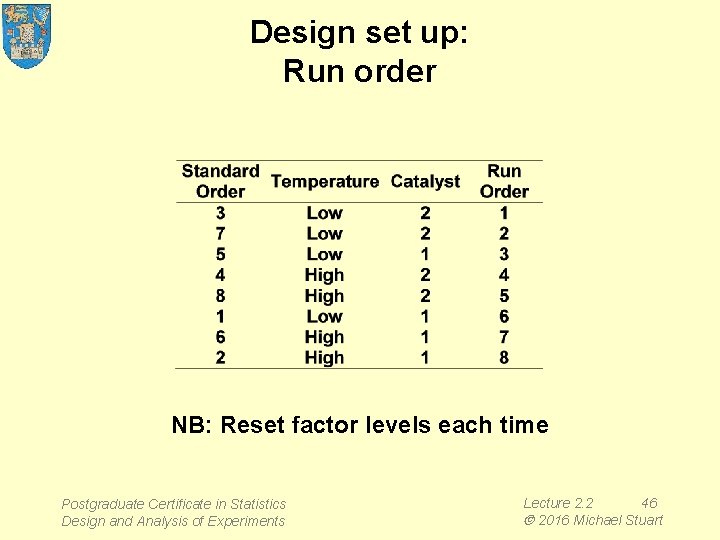
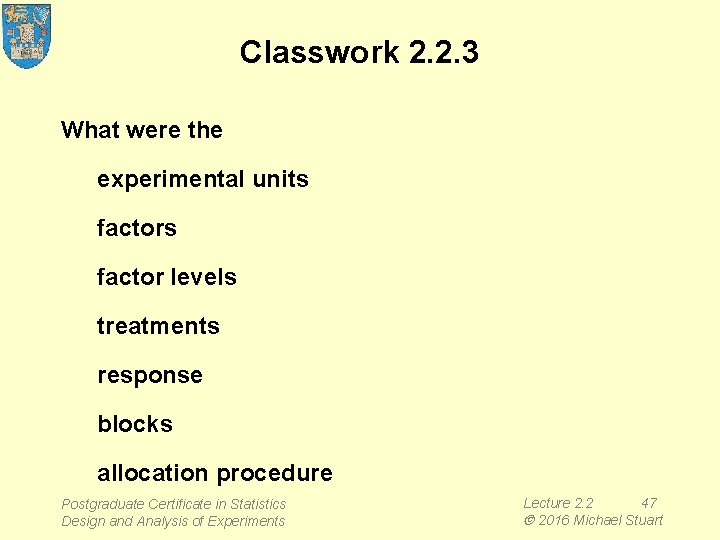
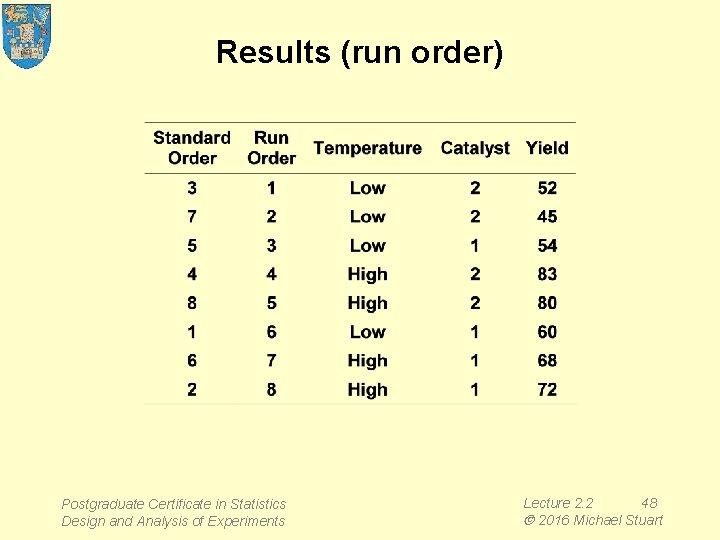
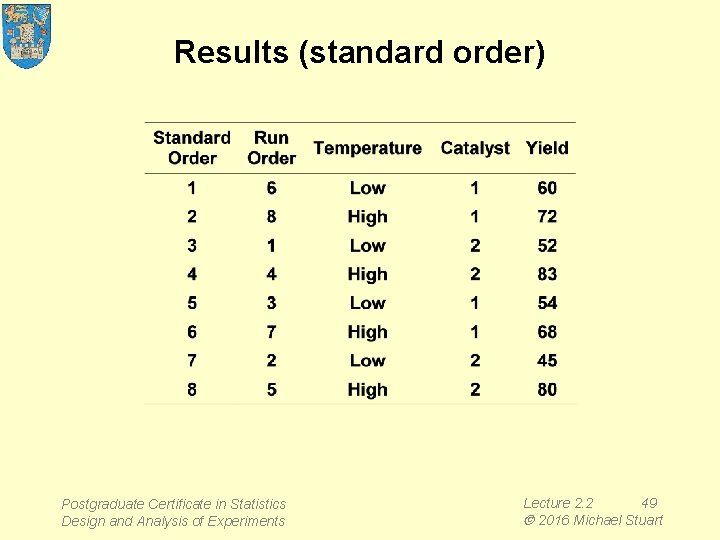
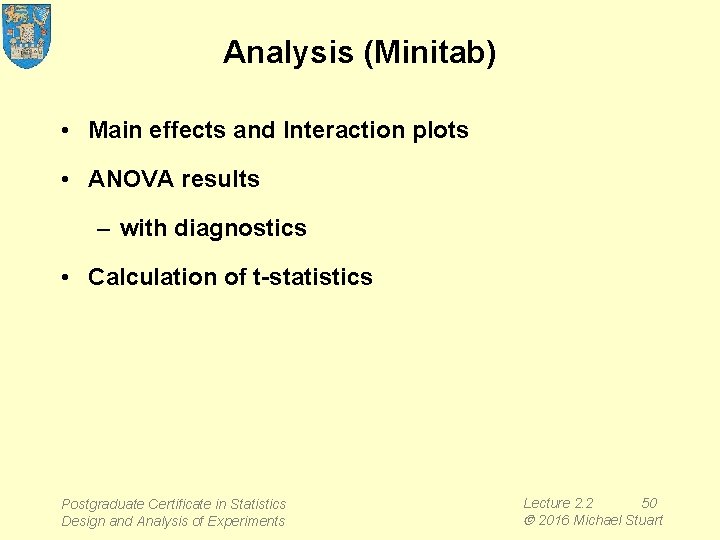
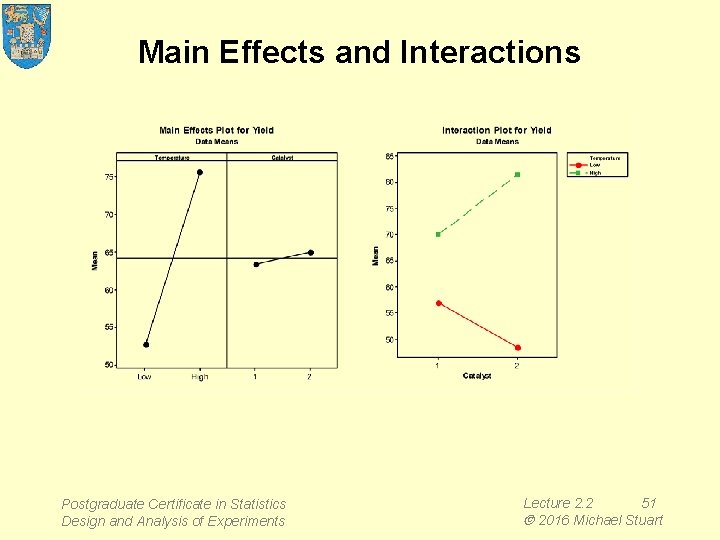
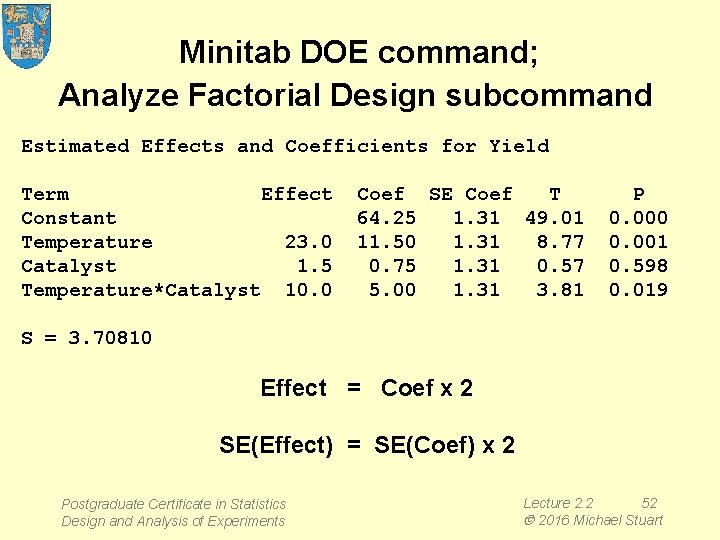
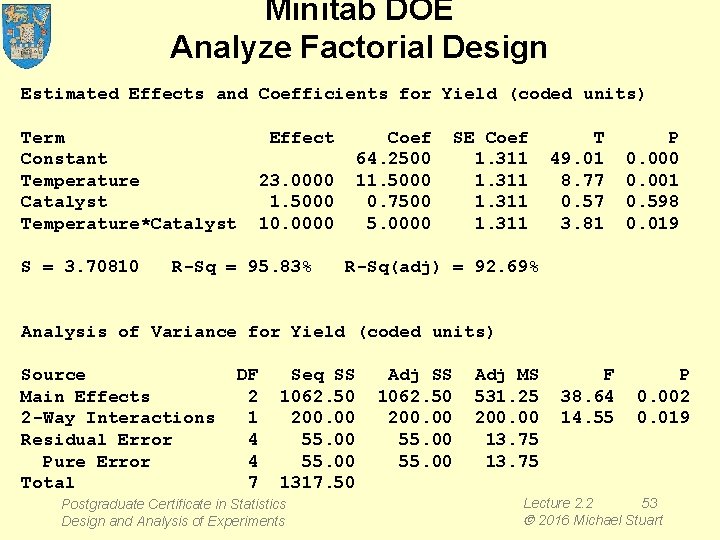
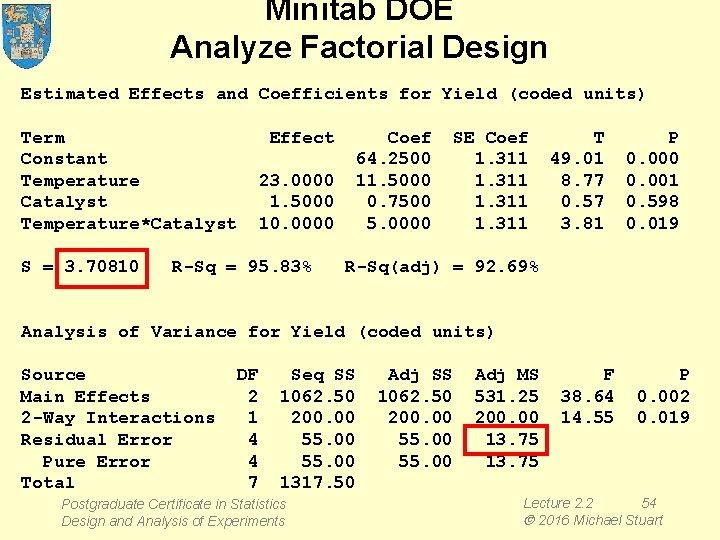
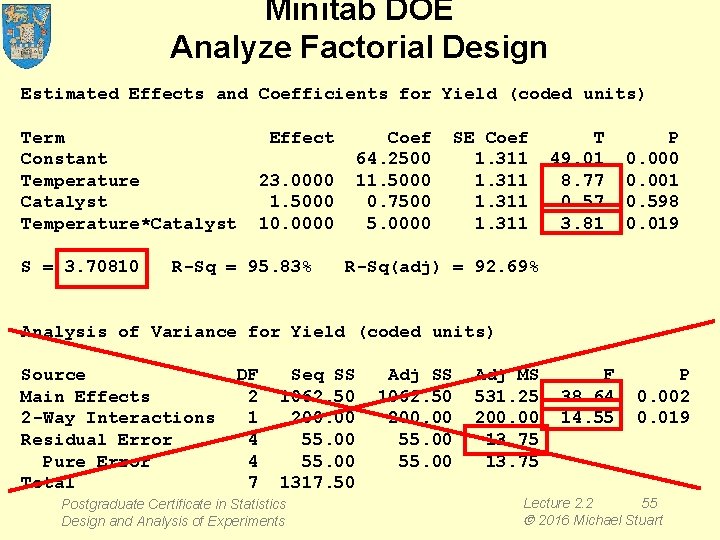
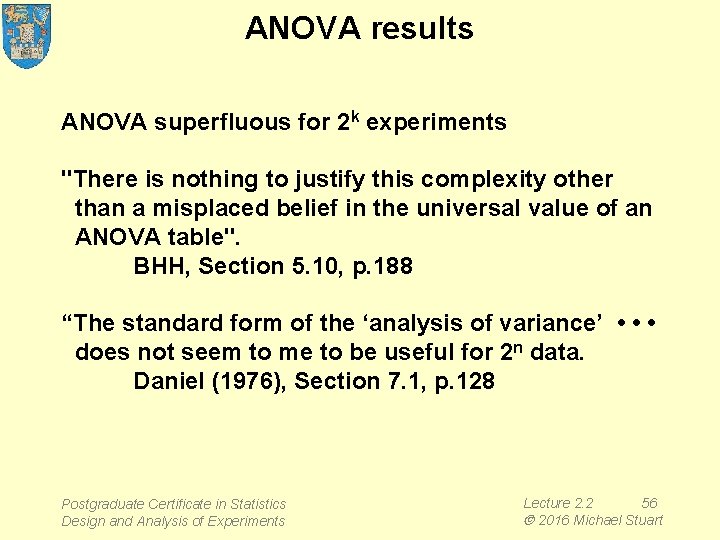
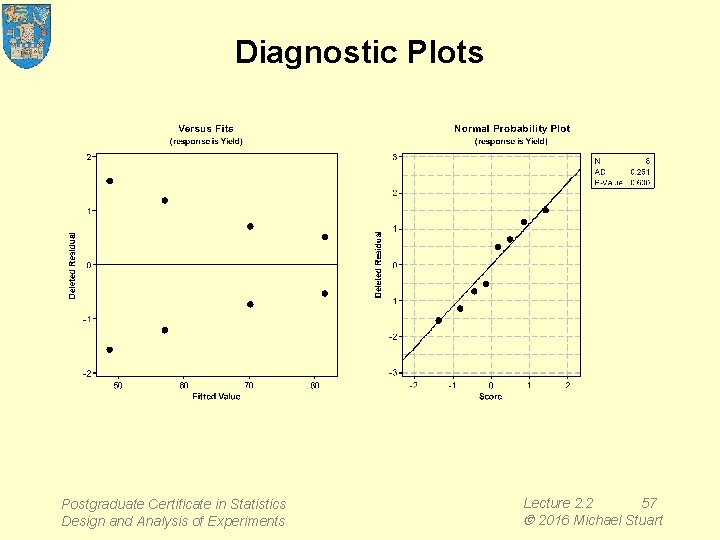
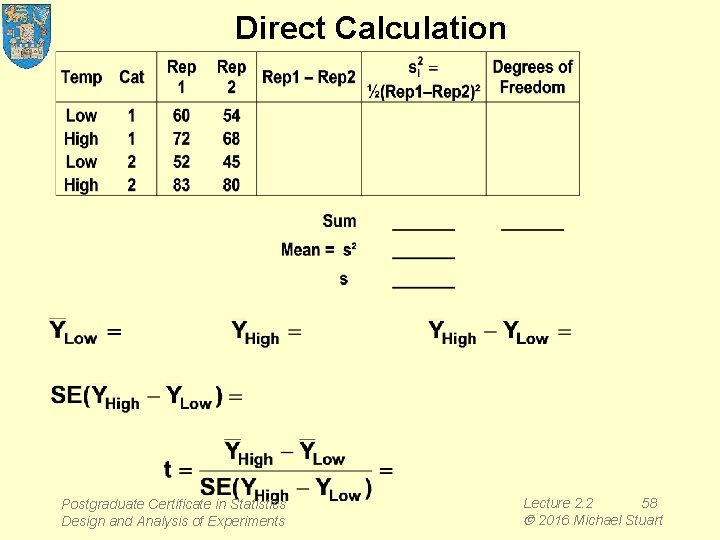
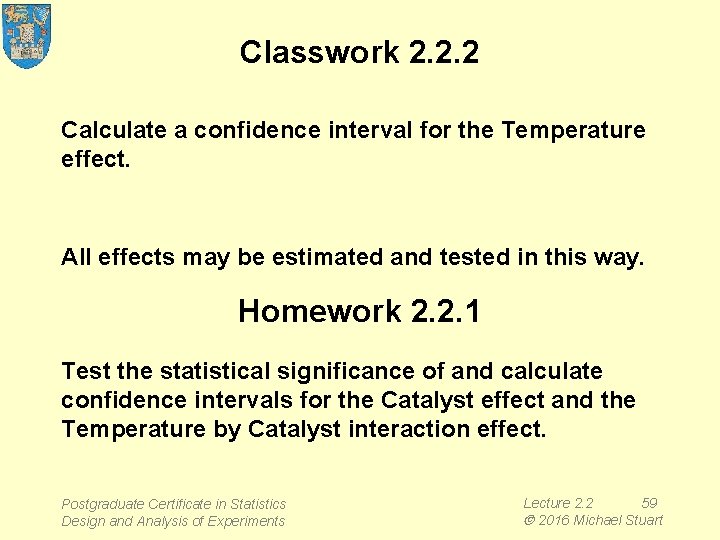
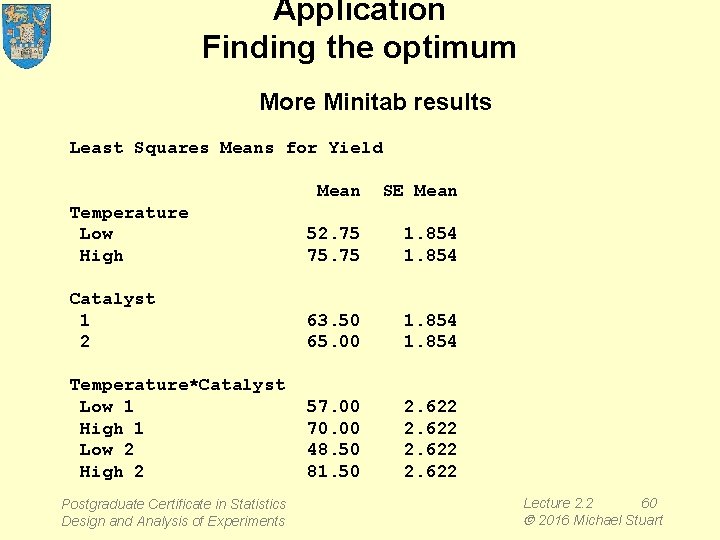
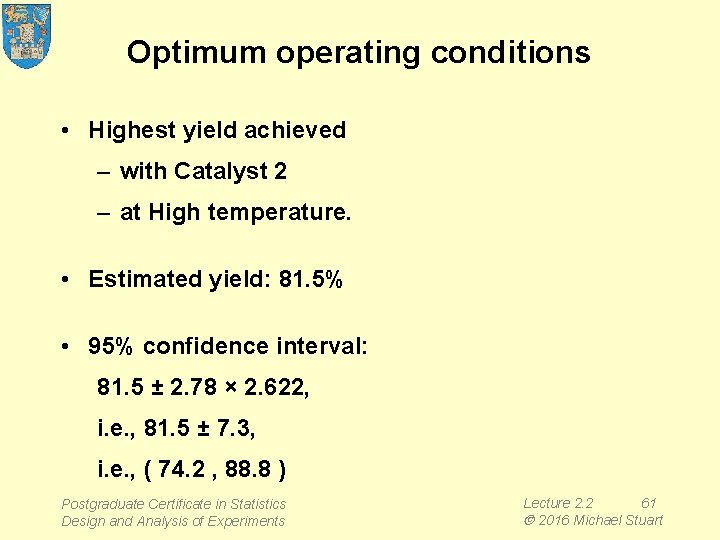
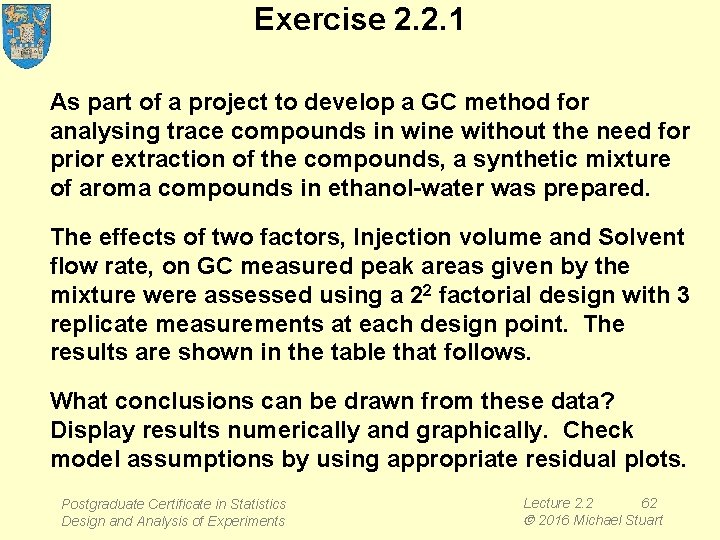
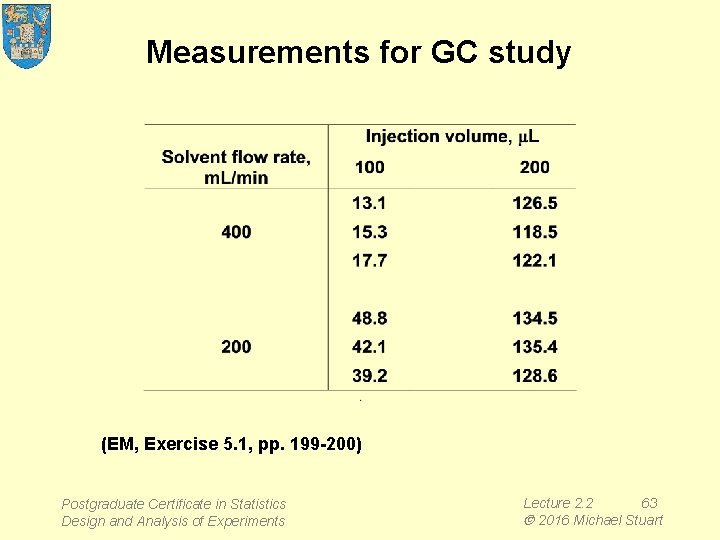
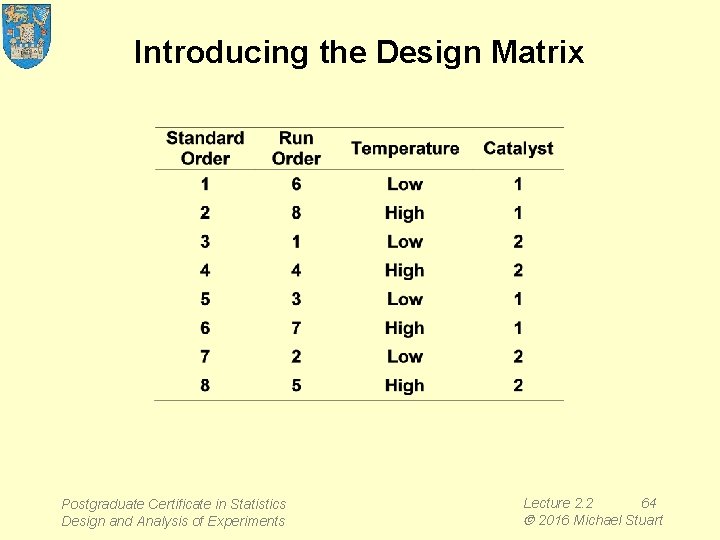
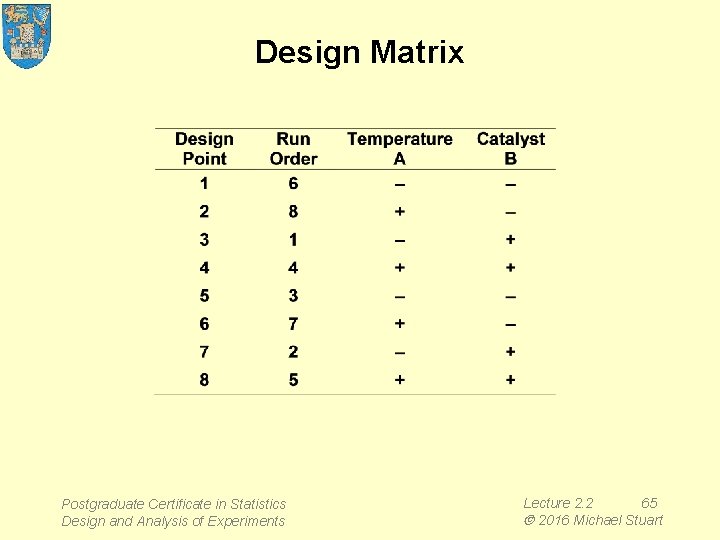
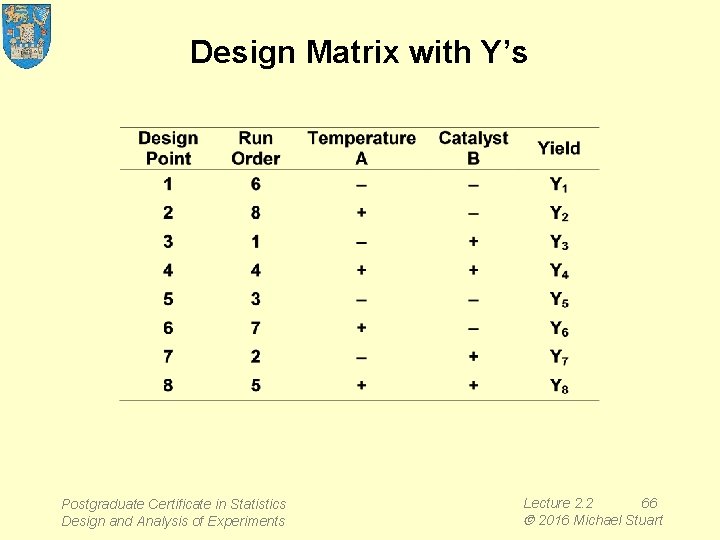
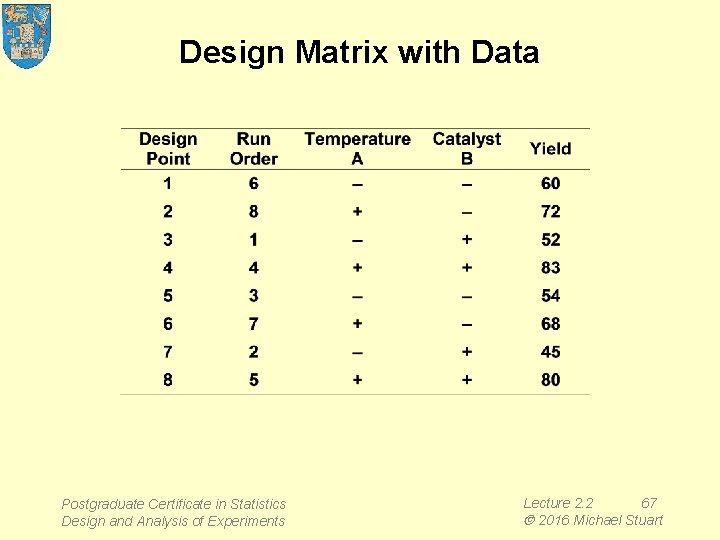
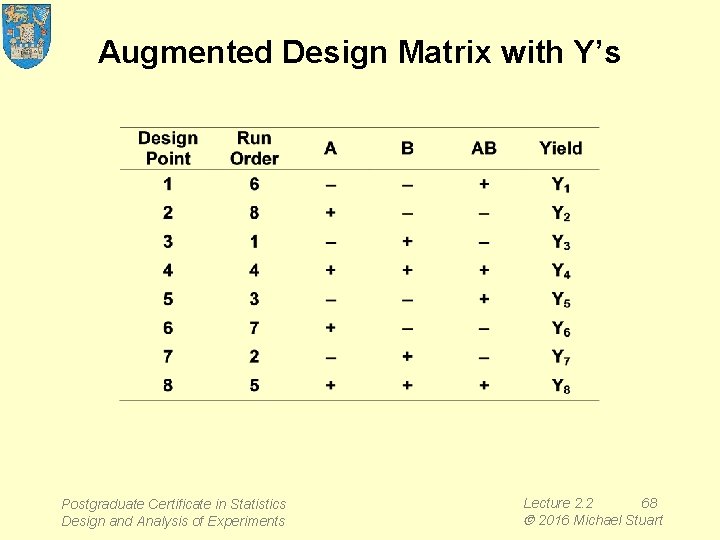
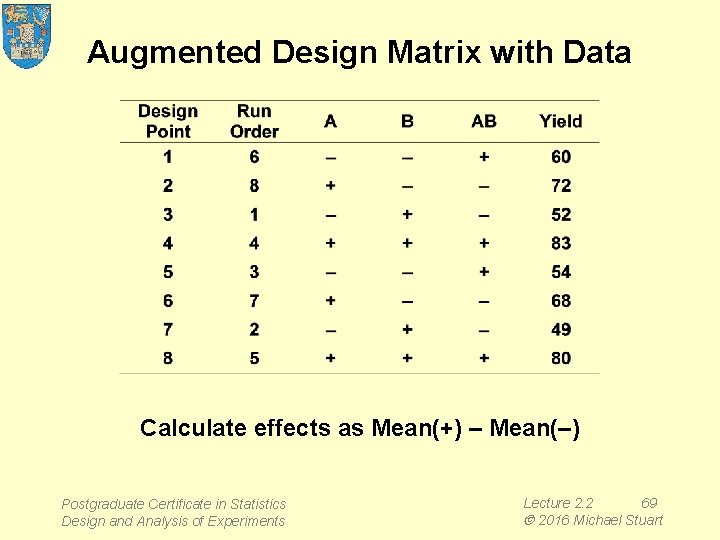
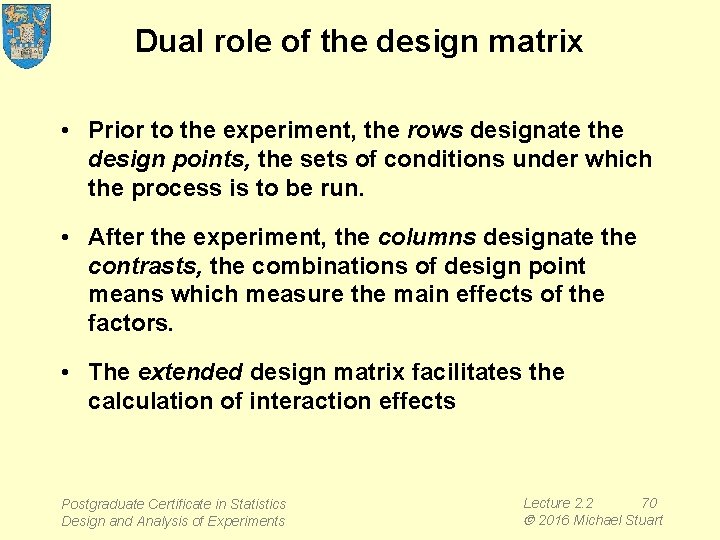
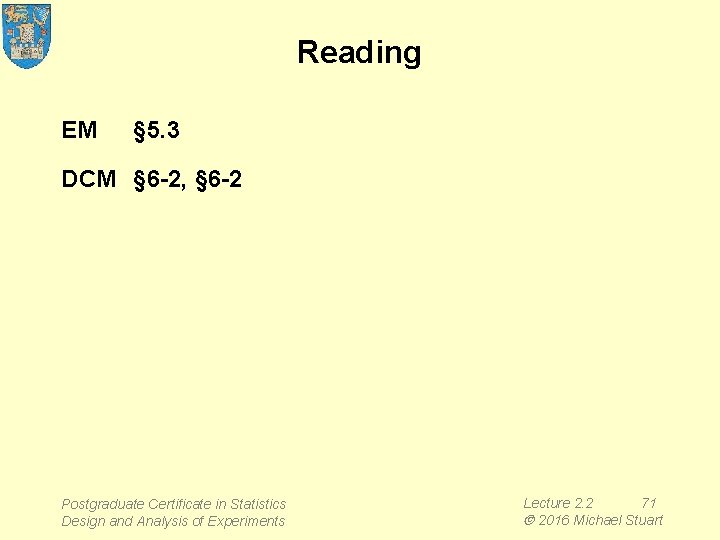
- Slides: 71
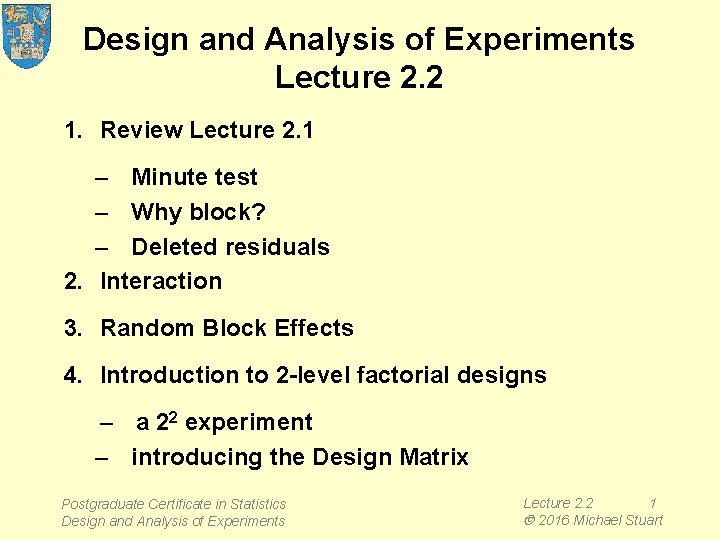
Design and Analysis of Experiments Lecture 2. 2 1. Review Lecture 2. 1 – Minute test – Why block? – Deleted residuals 2. Interaction 3. Random Block Effects 4. Introduction to 2 -level factorial designs – a 22 experiment – introducing the Design Matrix Postgraduate Certificate in Statistics Design and Analysis of Experiments Lecture 2. 2 1 2016 Michael Stuart
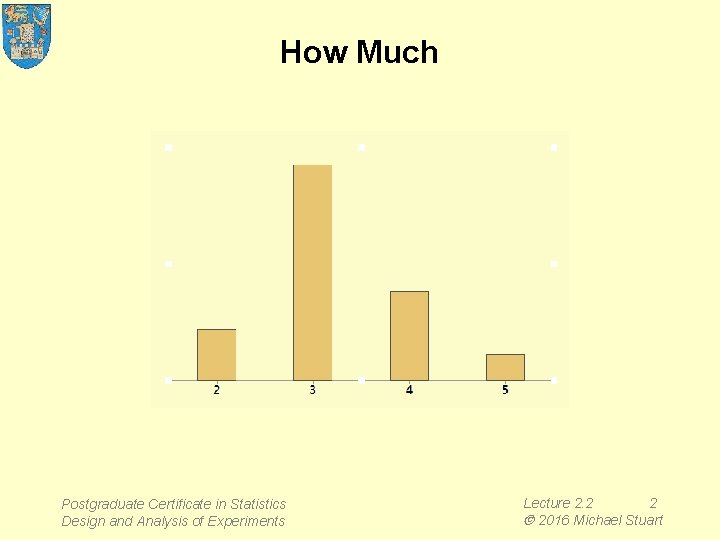
How Much Postgraduate Certificate in Statistics Design and Analysis of Experiments Lecture 2. 2 2 2016 Michael Stuart
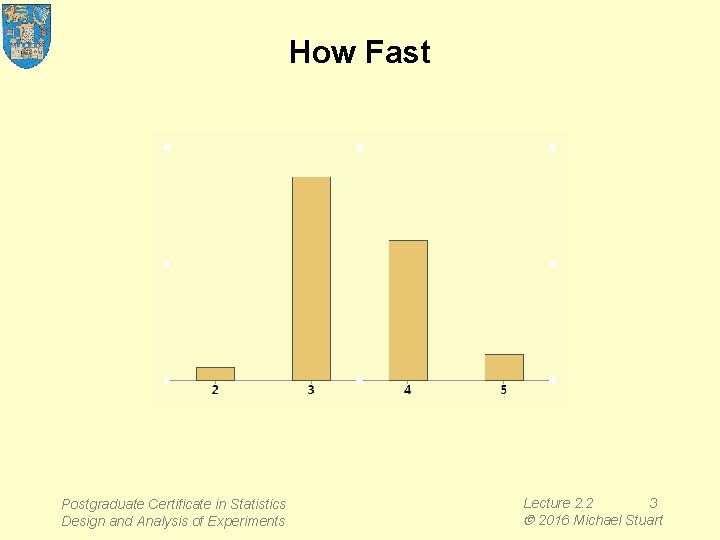
How Fast Postgraduate Certificate in Statistics Design and Analysis of Experiments Lecture 2. 2 3 2016 Michael Stuart
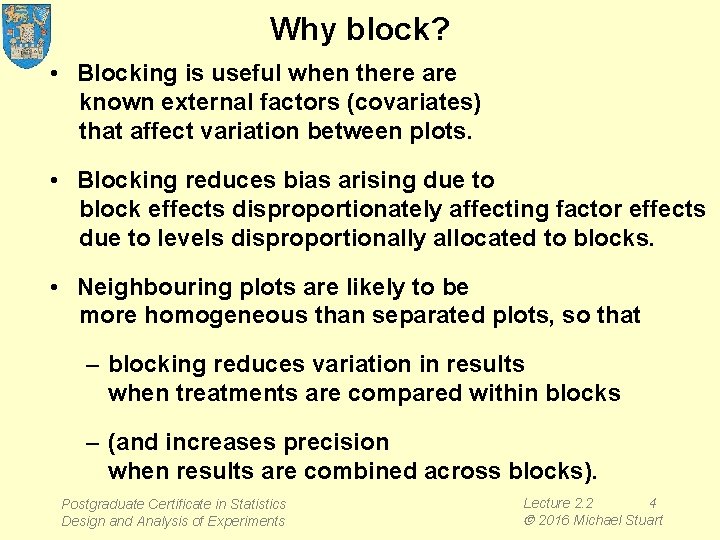
Why block? • Blocking is useful when there are known external factors (covariates) that affect variation between plots. • Blocking reduces bias arising due to block effects disproportionately affecting factor effects due to levels disproportionally allocated to blocks. • Neighbouring plots are likely to be more homogeneous than separated plots, so that – blocking reduces variation in results when treatments are compared within blocks – (and increases precision when results are combined across blocks). Postgraduate Certificate in Statistics Design and Analysis of Experiments Lecture 2. 2 4 2016 Michael Stuart
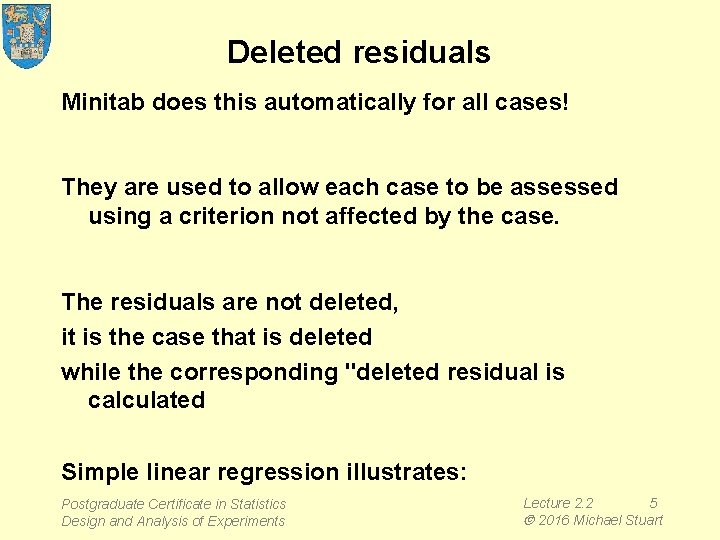
Deleted residuals Minitab does this automatically for all cases! They are used to allow each case to be assessed using a criterion not affected by the case. The residuals are not deleted, it is the case that is deleted while the corresponding "deleted residual is calculated Simple linear regression illustrates: Postgraduate Certificate in Statistics Design and Analysis of Experiments Lecture 2. 2 5 2016 Michael Stuart
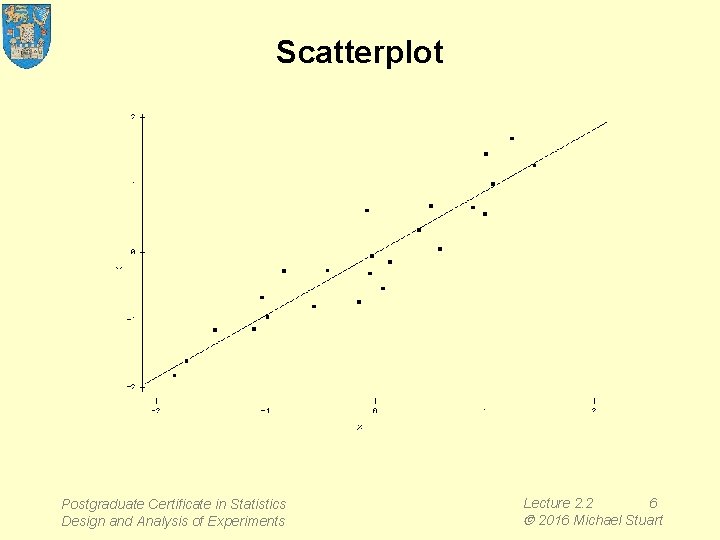
Scatterplot Postgraduate Certificate in Statistics Design and Analysis of Experiments Lecture 2. 2 6 2016 Michael Stuart
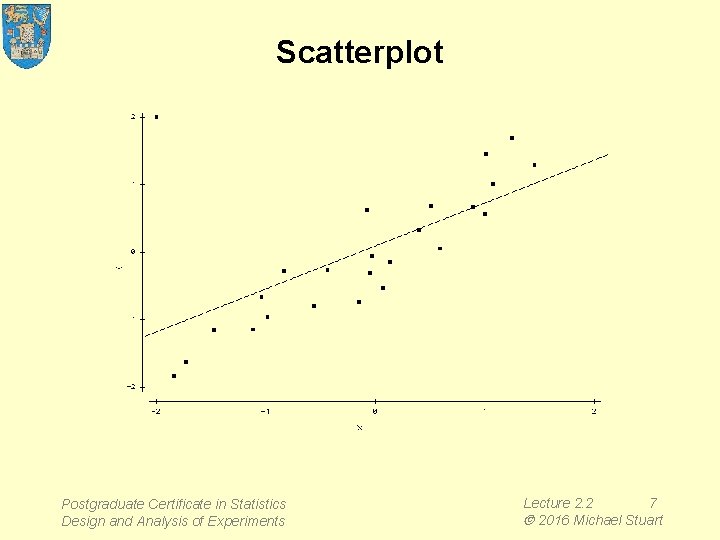
Scatterplot Postgraduate Certificate in Statistics Design and Analysis of Experiments Lecture 2. 2 7 2016 Michael Stuart
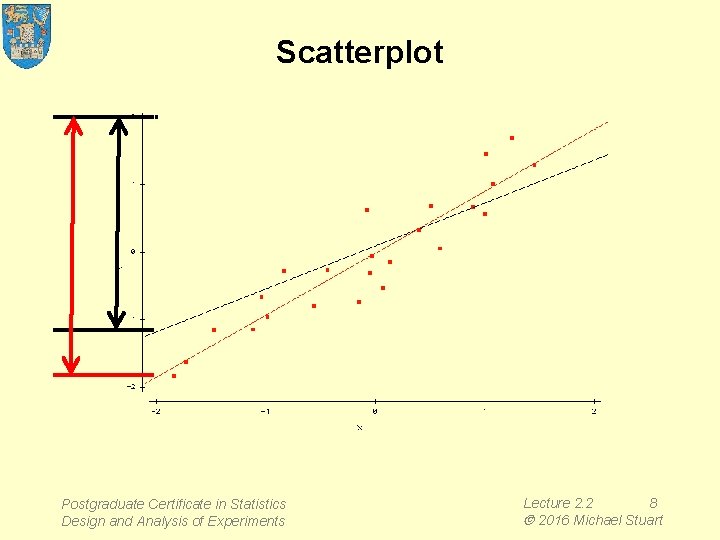
Scatterplot Postgraduate Certificate in Statistics Design and Analysis of Experiments Lecture 2. 2 8 2016 Michael Stuart
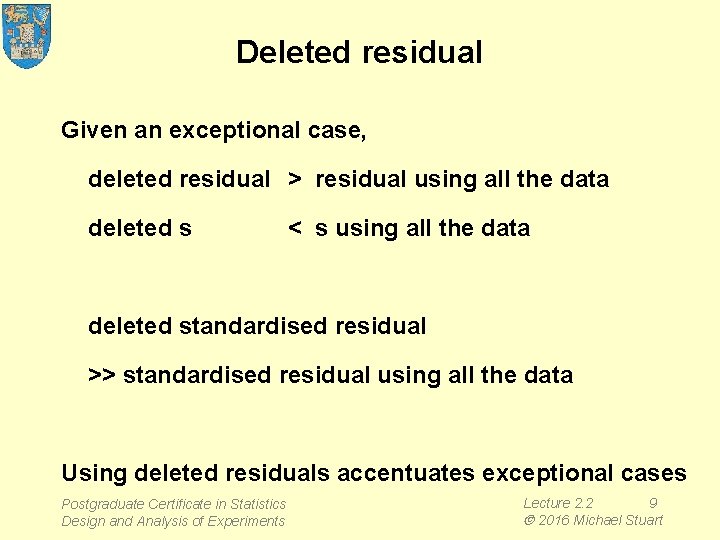
Deleted residual Given an exceptional case, deleted residual > residual using all the data deleted s < s using all the data deleted standardised residual >> standardised residual using all the data Using deleted residuals accentuates exceptional cases Postgraduate Certificate in Statistics Design and Analysis of Experiments Lecture 2. 2 9 2016 Michael Stuart
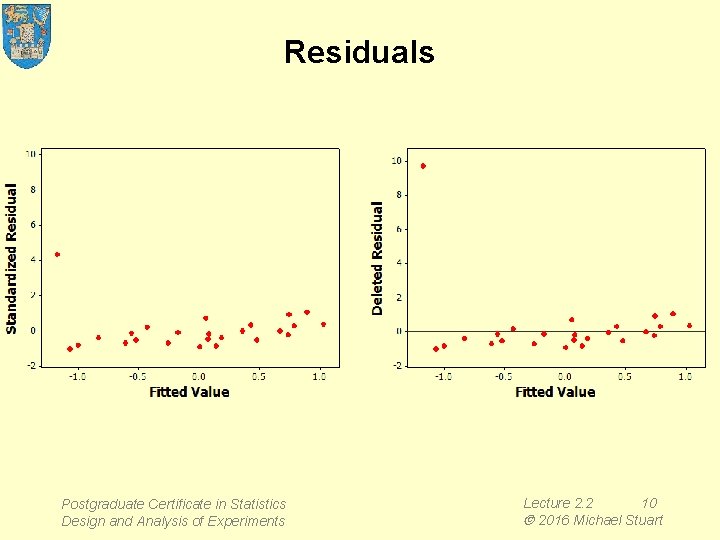
Residuals Postgraduate Certificate in Statistics Design and Analysis of Experiments Lecture 2. 2 10 2016 Michael Stuart
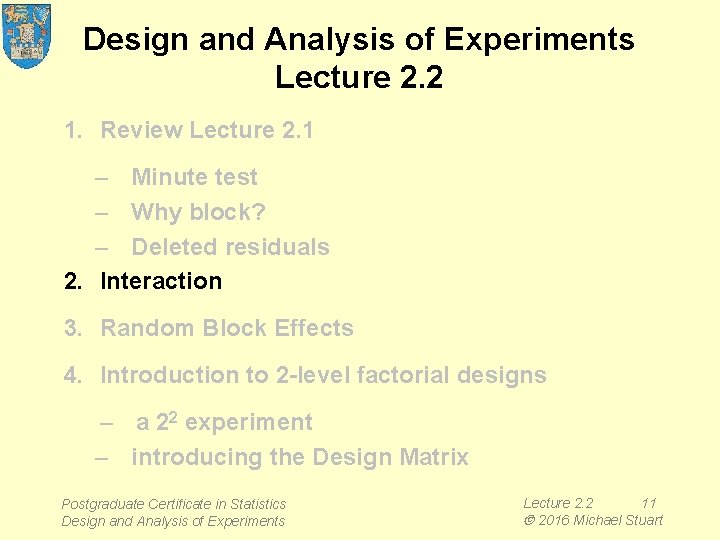
Design and Analysis of Experiments Lecture 2. 2 1. Review Lecture 2. 1 – Minute test – Why block? – Deleted residuals 2. Interaction 3. Random Block Effects 4. Introduction to 2 -level factorial designs – a 22 experiment – introducing the Design Matrix Postgraduate Certificate in Statistics Design and Analysis of Experiments Lecture 2. 2 11 2016 Michael Stuart
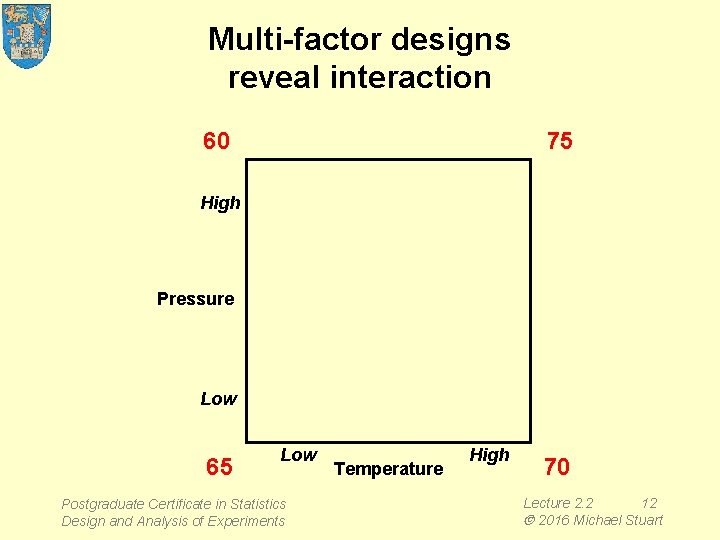
Multi-factor designs reveal interaction 75 60 High Pressure Low 65 Low Postgraduate Certificate in Statistics Design and Analysis of Experiments Temperature High 70 Lecture 2. 2 12 2016 Michael Stuart
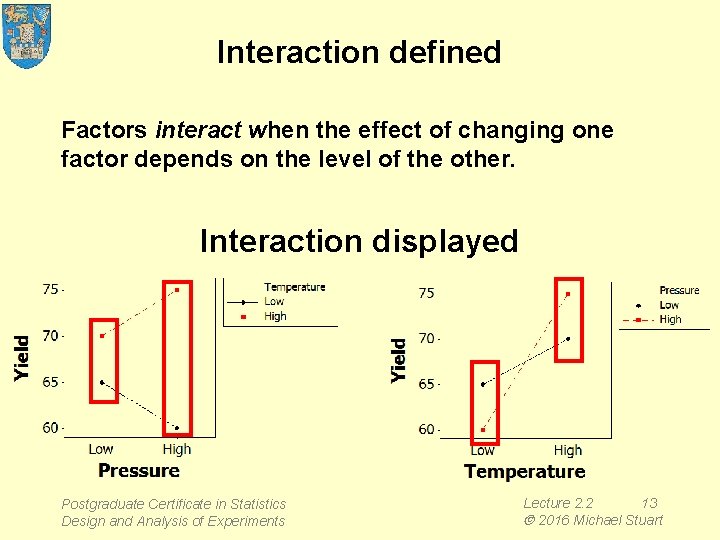
Interaction defined Factors interact when the effect of changing one factor depends on the level of the other. Interaction displayed Postgraduate Certificate in Statistics Design and Analysis of Experiments Lecture 2. 2 13 2016 Michael Stuart
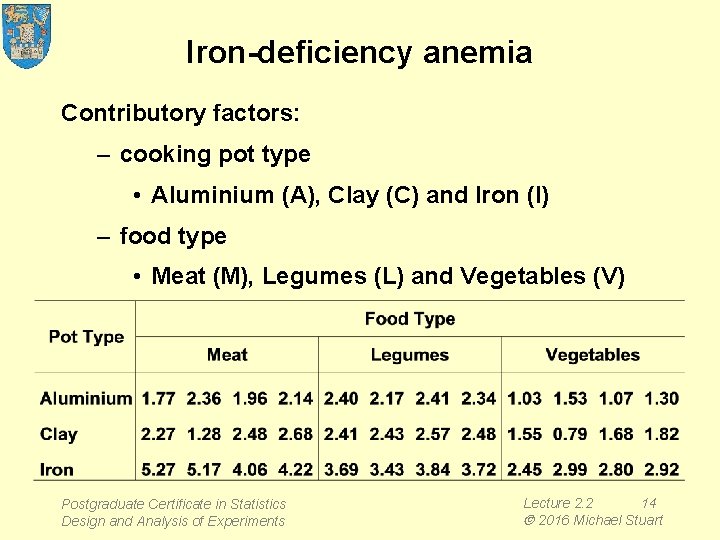
Iron-deficiency anemia Contributory factors: – cooking pot type • Aluminium (A), Clay (C) and Iron (I) – food type • Meat (M), Legumes (L) and Vegetables (V) Postgraduate Certificate in Statistics Design and Analysis of Experiments Lecture 2. 2 14 2016 Michael Stuart
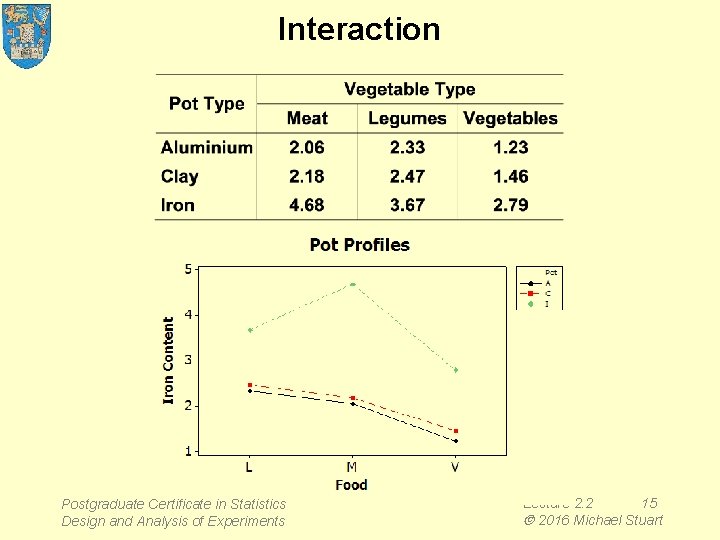
Interaction Postgraduate Certificate in Statistics Design and Analysis of Experiments Lecture 2. 2 15 2016 Michael Stuart
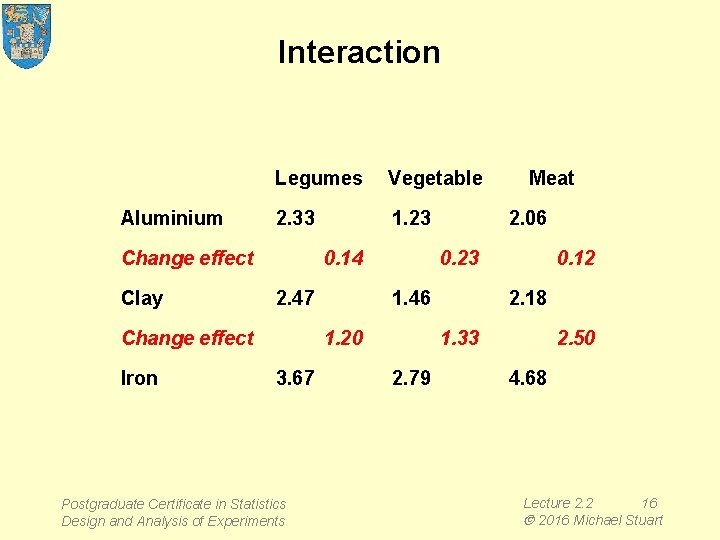
Interaction Aluminium Legumes Vegetable 2. 33 1. 23 Change effect Clay 0. 14 2. 47 Change effect Iron Postgraduate Certificate in Statistics Design and Analysis of Experiments 2. 06 0. 23 1. 46 1. 20 3. 67 Meat 0. 12 2. 18 1. 33 2. 79 2. 50 4. 68 Lecture 2. 2 16 2016 Michael Stuart
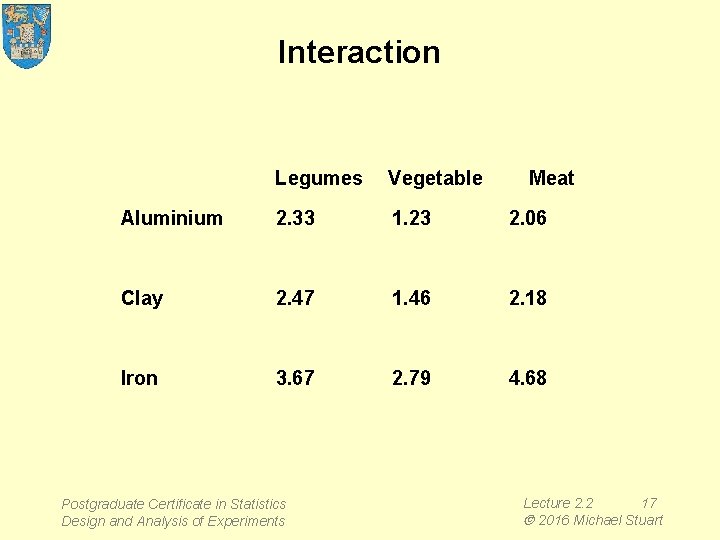
Interaction Aluminium Legumes Vegetable 2. 33 1. 23 Change effect Clay 0. 14 2. 47 Change effect Iron Postgraduate Certificate in Statistics Design and Analysis of Experiments 2. 06 0. 23 1. 46 1. 20 3. 67 Meat 0. 12 2. 18 1. 33 2. 79 2. 50 4. 68 Lecture 2. 2 17 2016 Michael Stuart
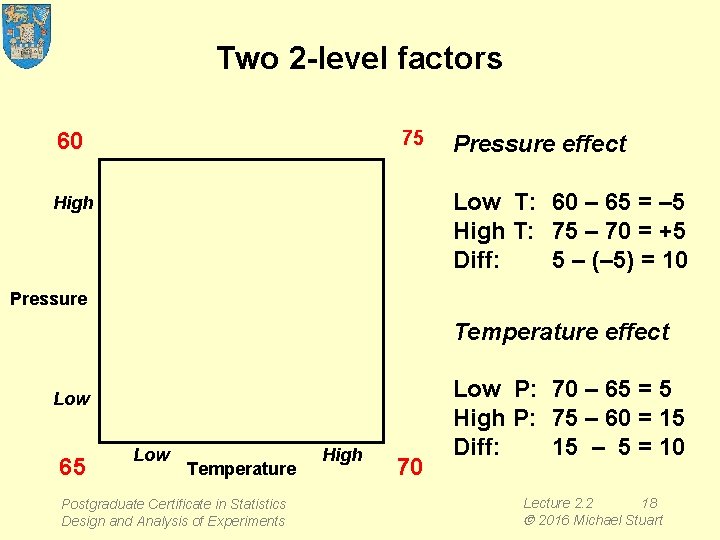
Two 2 -level factors 60 75 Pressure effect Low T: 60 – 65 = – 5 High T: 75 – 70 = +5 Diff: 5 – (– 5) = 10 High Pressure Temperature effect Low 65 Low Temperature Postgraduate Certificate in Statistics Design and Analysis of Experiments High 70 Low P: 70 – 65 = 5 High P: 75 – 60 = 15 Diff: 15 – 5 = 10 Lecture 2. 2 18 2016 Michael Stuart
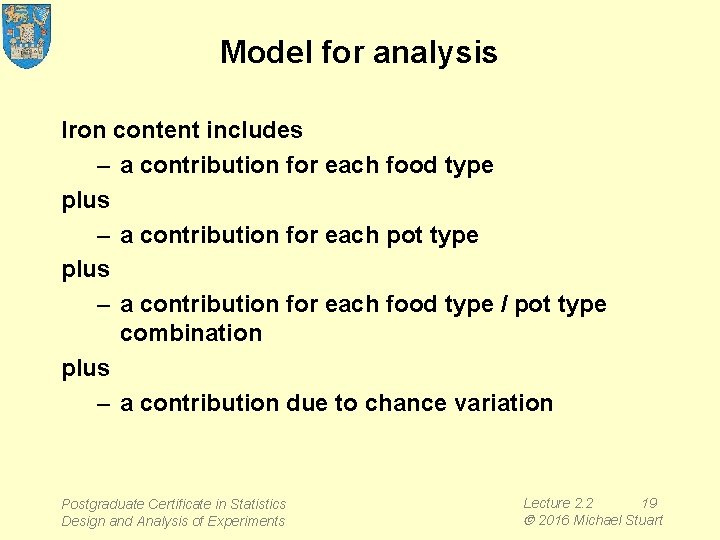
Model for analysis Iron content includes – a contribution for each food type plus – a contribution for each pot type plus – a contribution for each food type / pot type combination plus – a contribution due to chance variation Postgraduate Certificate in Statistics Design and Analysis of Experiments Lecture 2. 2 19 2016 Michael Stuart
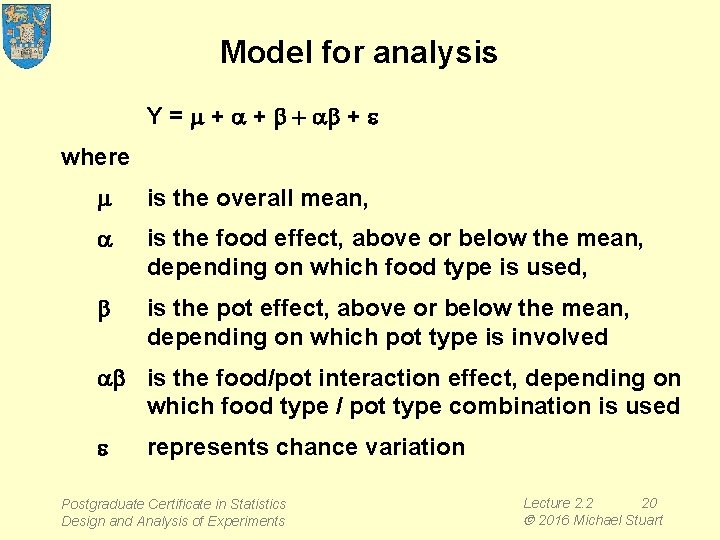
Model for analysis Y = m + a + b + ab + e where m is the overall mean, a is the food effect, above or below the mean, depending on which food type is used, b is the pot effect, above or below the mean, depending on which pot type is involved ab is the food/pot interaction effect, depending on which food type / pot type combination is used e represents chance variation Postgraduate Certificate in Statistics Design and Analysis of Experiments Lecture 2. 2 20 2016 Michael Stuart
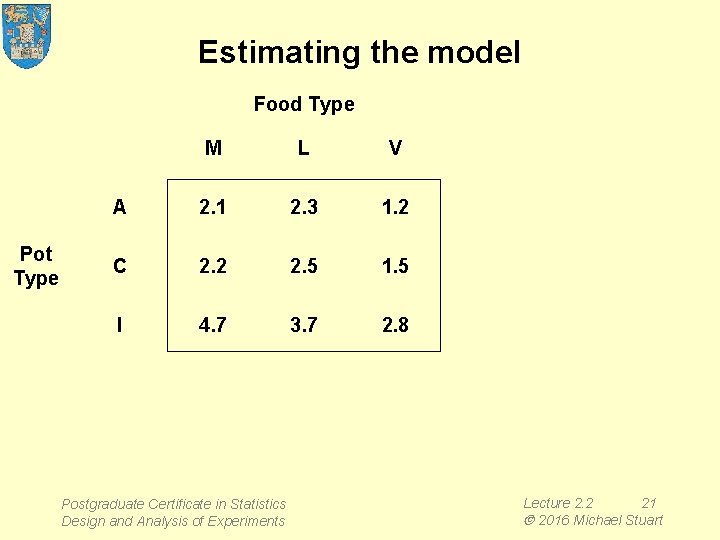
Estimating the model Food Type Pot Type M L V Pot Means Pot Main Effects A 2. 1 2. 3 1. 2 1. 9 – 2. 5 = – 0. 6 C 2. 2 2. 5 1. 5 2. 0 – 2. 5 = – 0. 5 I 4. 7 3. 7 2. 8 3. 7 – 2. 5 = + 1. 2 3. 0 2. 8 1. 8 2. 5 Food Means Food Main Effects 3. 0 – 2. 5 2. 8 – 2. 5 1. 8 – 2. 5 = +0. 3 = – 0. 7 Postgraduate Certificate in Statistics Design and Analysis of Experiments Lecture 2. 2 21 2016 Michael Stuart
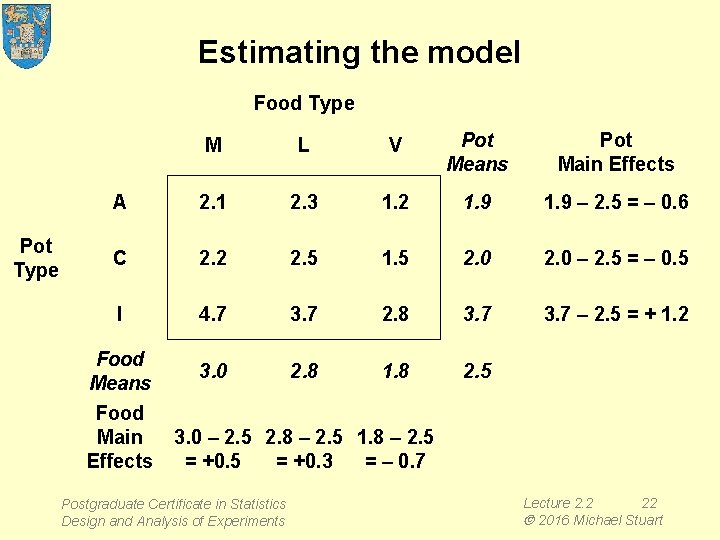
Estimating the model Food Type Pot Type M L V Pot Means Pot Main Effects A 2. 1 2. 3 1. 2 1. 9 – 2. 5 = – 0. 6 C 2. 2 2. 5 1. 5 2. 0 – 2. 5 = – 0. 5 I 4. 7 3. 7 2. 8 3. 7 – 2. 5 = + 1. 2 Food Means 3. 0 2. 8 1. 8 2. 5 Food Main Effects 3. 0 – 2. 5 2. 8 – 2. 5 1. 8 – 2. 5 = +0. 3 = – 0. 7 Postgraduate Certificate in Statistics Design and Analysis of Experiments Lecture 2. 2 22 2016 Michael Stuart
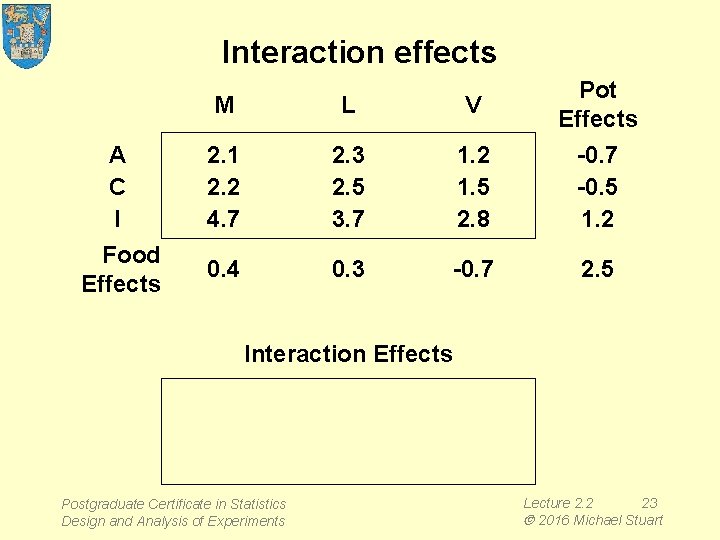
Interaction effects A C I Food Effects M L V 2. 1 2. 2 4. 7 2. 3 2. 5 3. 7 1. 2 1. 5 2. 8 Pot Effects -0. 7 -0. 5 1. 2 0. 4 0. 3 -0. 7 2. 5 Interaction Effects -0. 2 -0. 3 0. 5 Postgraduate Certificate in Statistics Design and Analysis of Experiments 0. 2 -0. 3 0. 1 -0. 2 Lecture 2. 2 23 2016 Michael Stuart
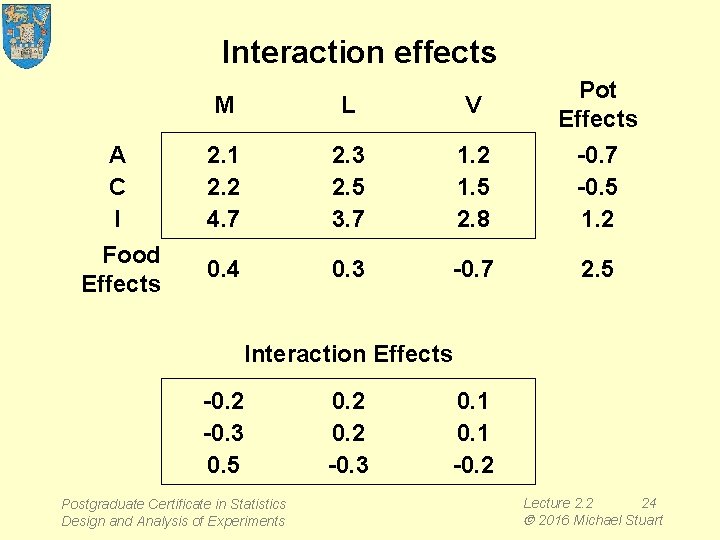
Interaction effects A C I Food Effects M L V 2. 1 2. 2 4. 7 2. 3 2. 5 3. 7 1. 2 1. 5 2. 8 Pot Effects -0. 7 -0. 5 1. 2 0. 4 0. 3 -0. 7 2. 5 Interaction Effects -0. 2 -0. 3 0. 5 Postgraduate Certificate in Statistics Design and Analysis of Experiments 0. 2 -0. 3 0. 1 -0. 2 Lecture 2. 2 24 2016 Michael Stuart
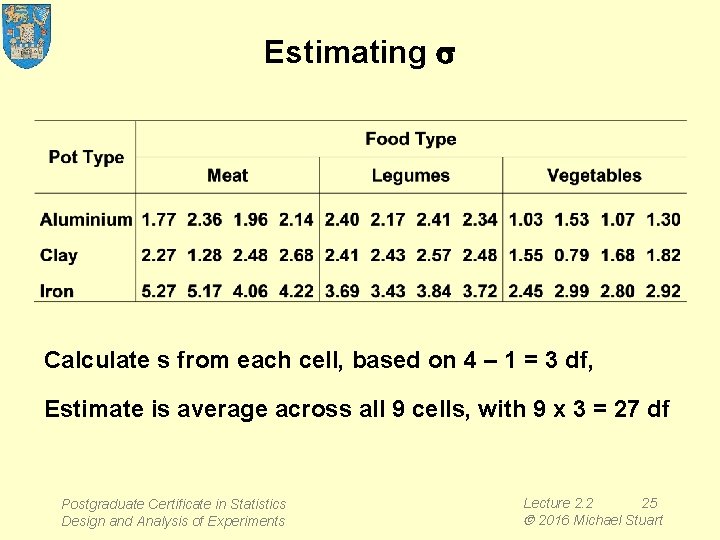
Estimating s Calculate s from each cell, based on 4 – 1 = 3 df, Estimate is average across all 9 cells, with 9 x 3 = 27 df Postgraduate Certificate in Statistics Design and Analysis of Experiments Lecture 2. 2 25 2016 Michael Stuart
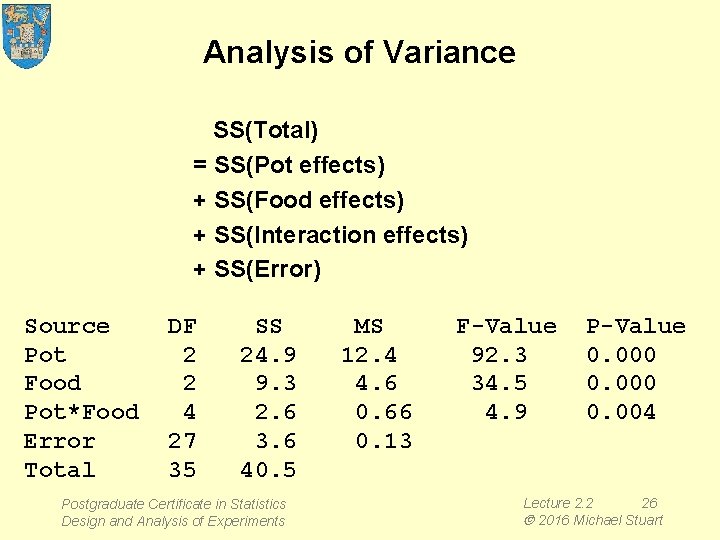
Analysis of Variance SS(Total) = SS(Pot effects) + SS(Food effects) + SS(Interaction effects) + SS(Error) Source Pot Food Pot*Food Error Total DF 2 2 4 27 35 SS 24. 9 9. 3 2. 6 3. 6 40. 5 Postgraduate Certificate in Statistics Design and Analysis of Experiments MS 12. 4 4. 6 0. 66 0. 13 F-Value 92. 3 34. 5 4. 9 P-Value 0. 000 0. 004 Lecture 2. 2 26 2016 Michael Stuart
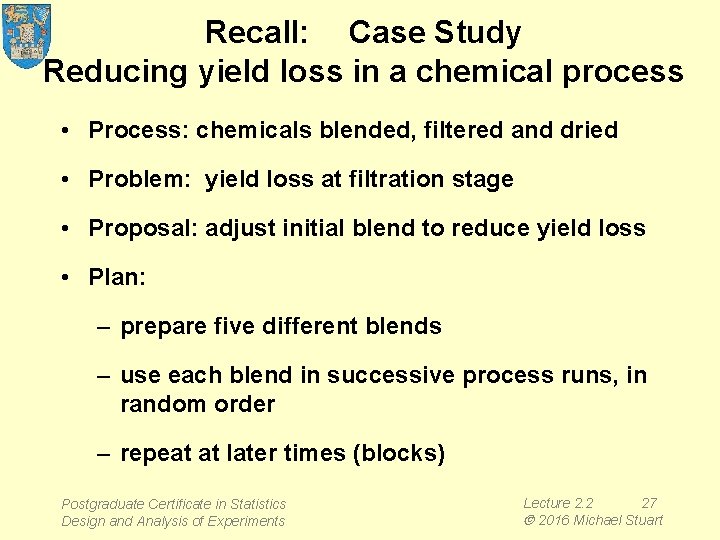
Recall: Case Study Reducing yield loss in a chemical process • Process: chemicals blended, filtered and dried • Problem: yield loss at filtration stage • Proposal: adjust initial blend to reduce yield loss • Plan: – prepare five different blends – use each blend in successive process runs, in random order – repeat at later times (blocks) Postgraduate Certificate in Statistics Design and Analysis of Experiments Lecture 2. 2 27 2016 Michael Stuart
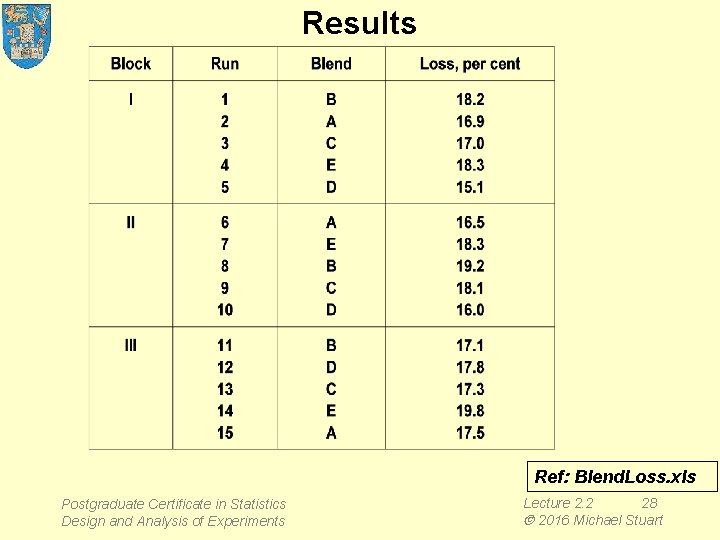
Results Ref: Blend. Loss. xls Postgraduate Certificate in Statistics Design and Analysis of Experiments Lecture 2. 2 28 2016 Michael Stuart
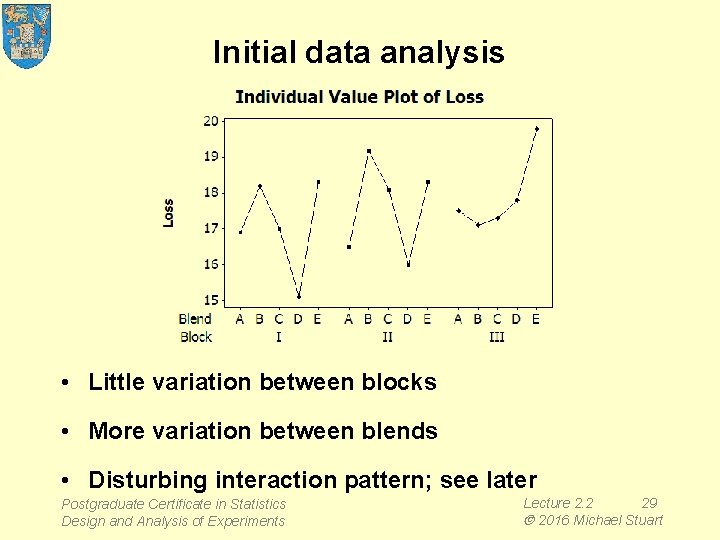
Initial data analysis • Little variation between blocks • More variation between blends • Disturbing interaction pattern; see later Postgraduate Certificate in Statistics Design and Analysis of Experiments Lecture 2. 2 29 2016 Michael Stuart
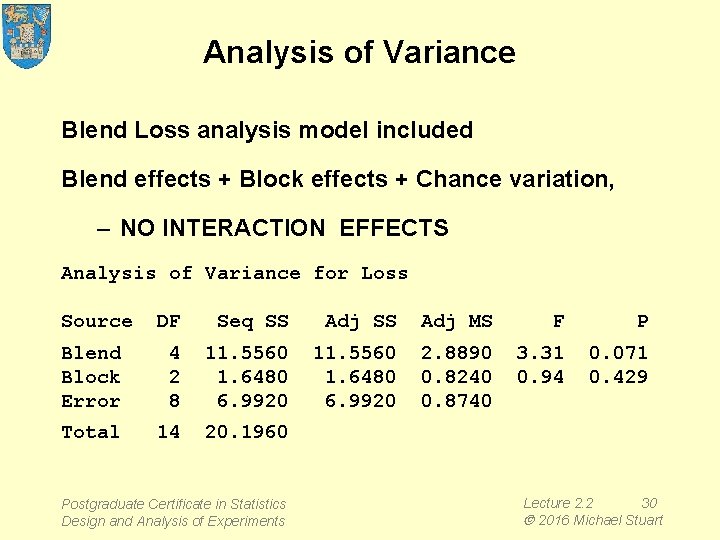
Analysis of Variance Blend Loss analysis model included Blend effects + Block effects + Chance variation, – NO INTERACTION EFFECTS Analysis of Variance for Loss Source DF Seq SS Adj MS F P Blend Block Error 4 2 8 11. 5560 1. 6480 6. 9920 2. 8890 0. 8240 0. 8740 3. 31 0. 94 0. 071 0. 429 Total 14 20. 1960 Postgraduate Certificate in Statistics Design and Analysis of Experiments Lecture 2. 2 30 2016 Michael Stuart
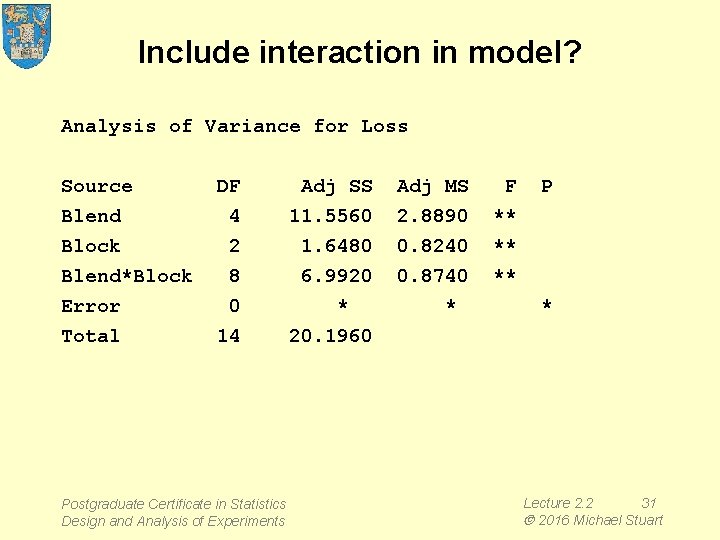
Include interaction in model? Analysis of Variance for Loss Source Blend DF 4 Adj SS 11. 5560 Adj MS 2. 8890 F ** Block Blend*Block Error Total 2 8 0 14 1. 6480 6. 9920 * 20. 1960 0. 8240 0. 8740 * ** ** Postgraduate Certificate in Statistics Design and Analysis of Experiments P * Lecture 2. 2 31 2016 Michael Stuart
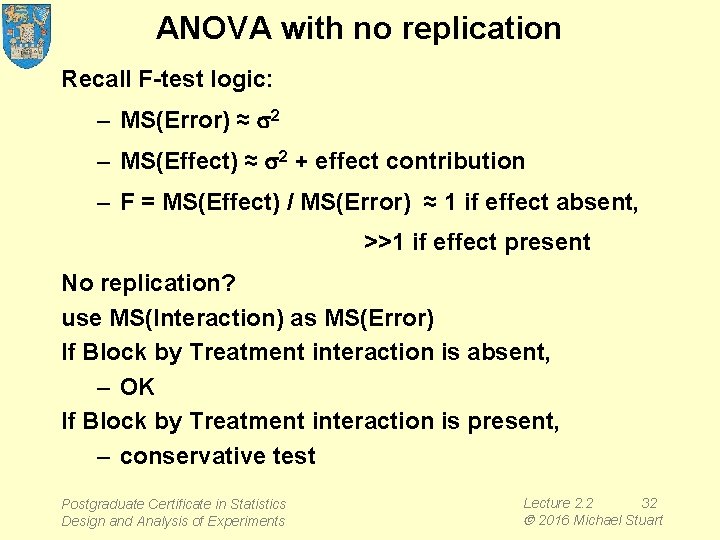
ANOVA with no replication Recall F-test logic: – MS(Error) ≈ s 2 – MS(Effect) ≈ s 2 + effect contribution – F = MS(Effect) / MS(Error) ≈ 1 if effect absent, >>1 if effect present No replication? use MS(Interaction) as MS(Error) If Block by Treatment interaction is absent, – OK If Block by Treatment interaction is present, – conservative test Postgraduate Certificate in Statistics Design and Analysis of Experiments Lecture 2. 2 32 2016 Michael Stuart
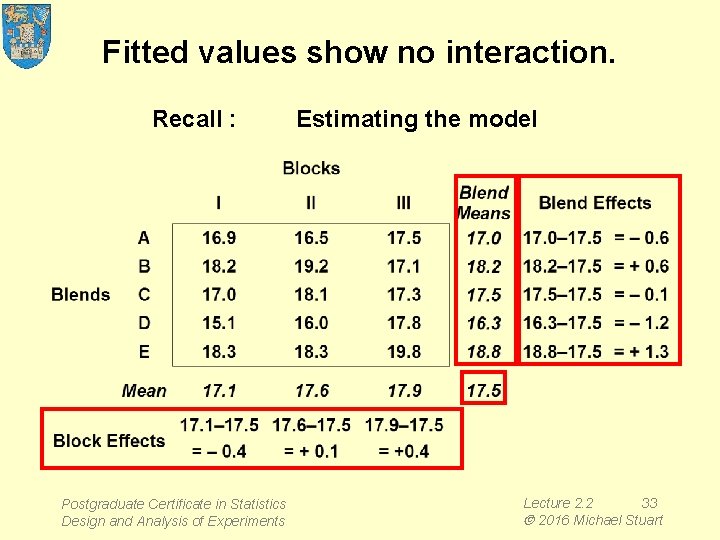
Fitted values show no interaction. Recall : Postgraduate Certificate in Statistics Design and Analysis of Experiments Estimating the model Lecture 2. 2 33 2016 Michael Stuart
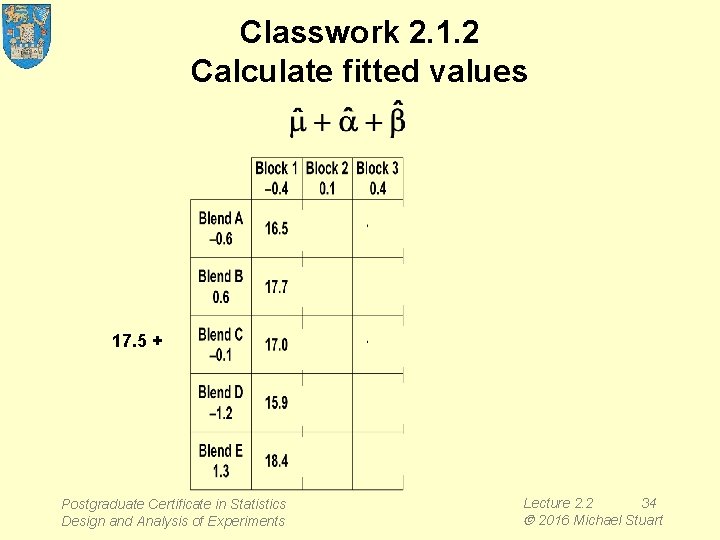
Classwork 2. 1. 2 Calculate fitted values 17. 5 + Postgraduate Certificate in Statistics Design and Analysis of Experiments Lecture 2. 2 34 2016 Michael Stuart
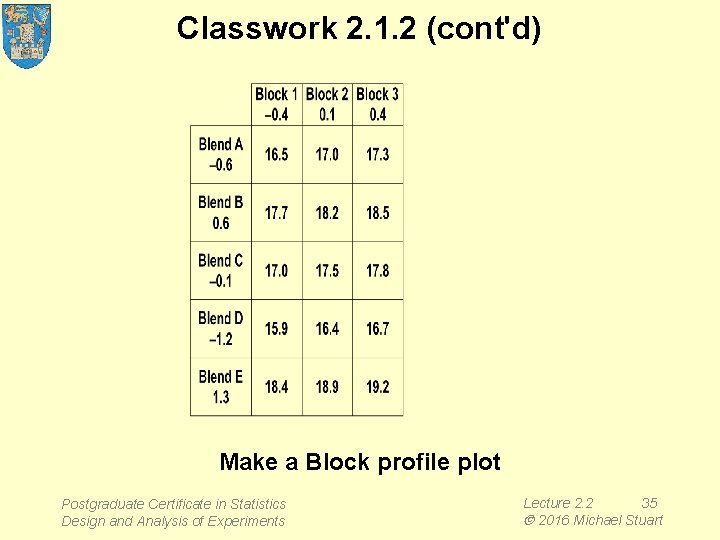
Classwork 2. 1. 2 (cont'd) Make a Block profile plot Postgraduate Certificate in Statistics Design and Analysis of Experiments Lecture 2. 2 35 2016 Michael Stuart
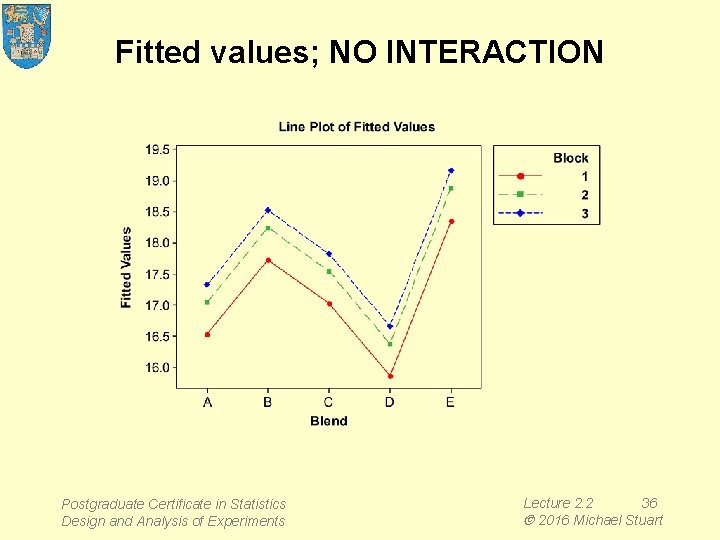
Fitted values; NO INTERACTION Postgraduate Certificate in Statistics Design and Analysis of Experiments Lecture 2. 2 36 2016 Michael Stuart
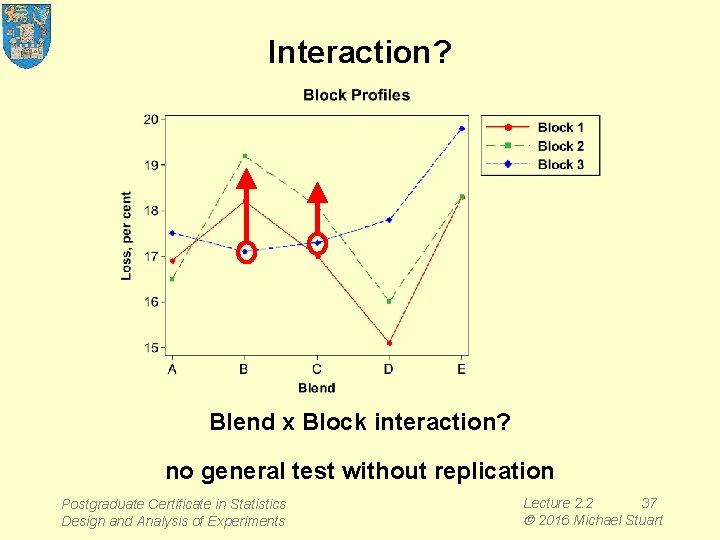
Interaction? Blend x Block interaction? no general test without replication Postgraduate Certificate in Statistics Design and Analysis of Experiments Lecture 2. 2 37 2016 Michael Stuart
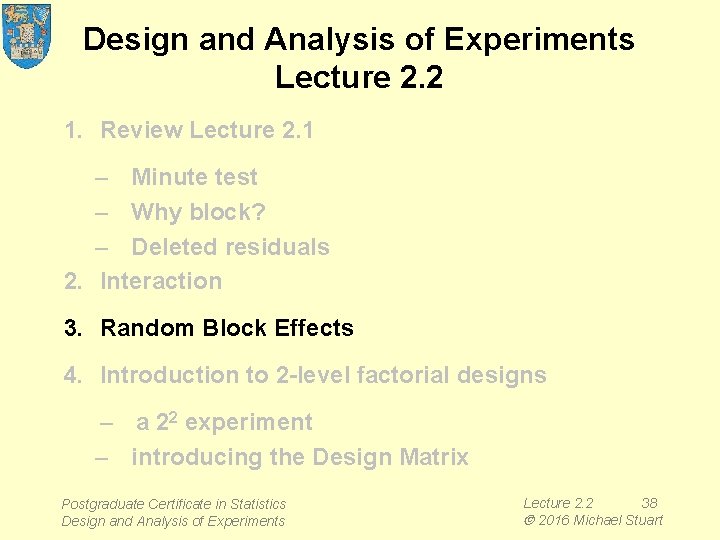
Design and Analysis of Experiments Lecture 2. 2 1. Review Lecture 2. 1 – Minute test – Why block? – Deleted residuals 2. Interaction 3. Random Block Effects 4. Introduction to 2 -level factorial designs – a 22 experiment – introducing the Design Matrix Postgraduate Certificate in Statistics Design and Analysis of Experiments Lecture 2. 2 38 2016 Michael Stuart
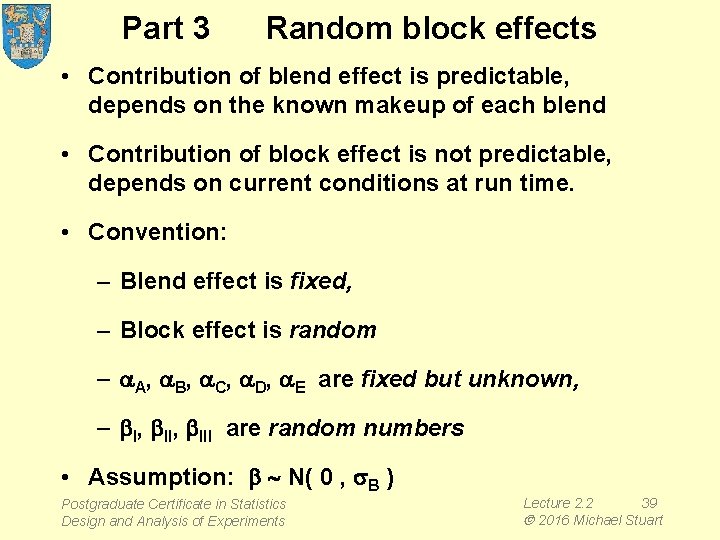
Part 3 Random block effects • Contribution of blend effect is predictable, depends on the known makeup of each blend • Contribution of block effect is not predictable, depends on current conditions at run time. • Convention: – Blend effect is fixed, – Block effect is random a. A, a. B, a. C, a. D, a. E are fixed but unknown, b. I, b. III are random numbers • Assumption: b N( 0 , s. B ) Postgraduate Certificate in Statistics Design and Analysis of Experiments Lecture 2. 2 39 2016 Michael Stuart
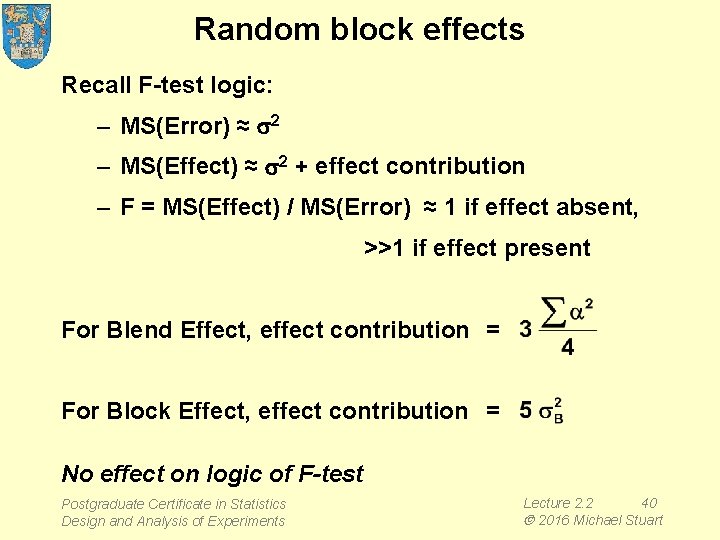
Random block effects Recall F-test logic: – MS(Error) ≈ s 2 – MS(Effect) ≈ s 2 + effect contribution – F = MS(Effect) / MS(Error) ≈ 1 if effect absent, >>1 if effect present For Blend Effect, effect contribution = For Block Effect, effect contribution = No effect on logic of F-test Postgraduate Certificate in Statistics Design and Analysis of Experiments Lecture 2. 2 40 2016 Michael Stuart
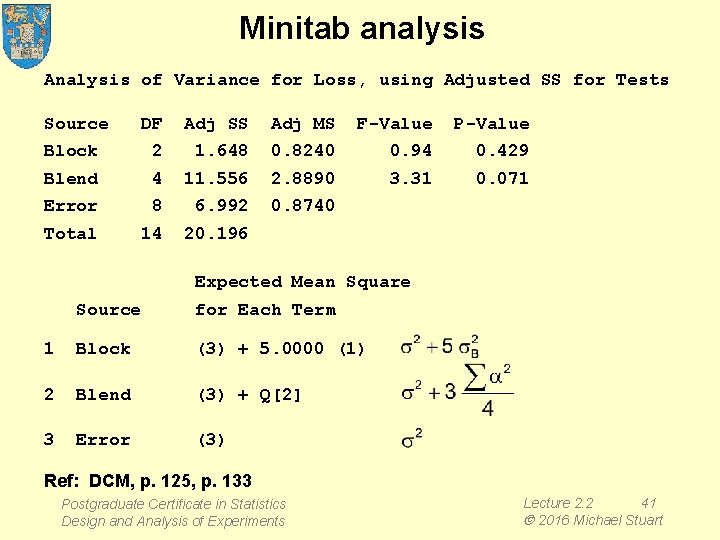
Minitab analysis Analysis of Variance for Loss, using Adjusted SS for Tests Source DF Adj SS Adj MS F-Value P-Value Block 2 1. 648 0. 8240 0. 94 0. 429 Blend 4 11. 556 2. 8890 3. 31 0. 071 Error 8 6. 992 0. 8740 Total 14 20. 196 Expected Mean Square Source for Each Term 1 Block (3) + 5. 0000 (1) 2 Blend (3) + Q[2] 3 Error (3) Ref: DCM, p. 125, p. 133 Postgraduate Certificate in Statistics Design and Analysis of Experiments Lecture 2. 2 41 2016 Michael Stuart
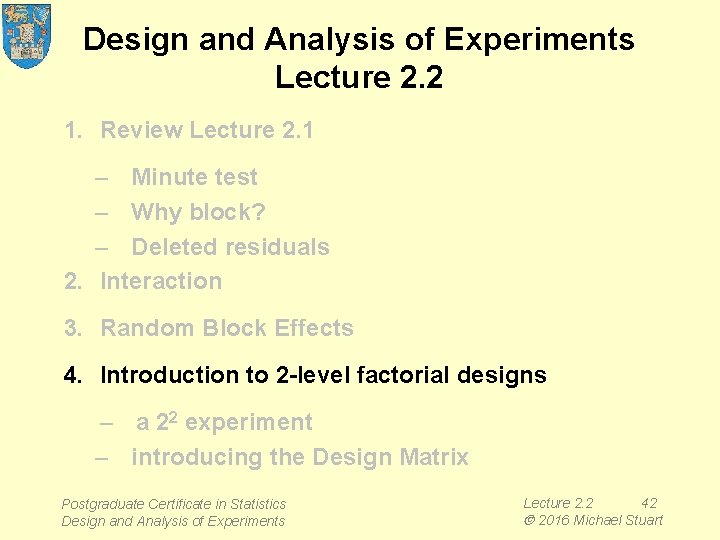
Design and Analysis of Experiments Lecture 2. 2 1. Review Lecture 2. 1 – Minute test – Why block? – Deleted residuals 2. Interaction 3. Random Block Effects 4. Introduction to 2 -level factorial designs – a 22 experiment – introducing the Design Matrix Postgraduate Certificate in Statistics Design and Analysis of Experiments Lecture 2. 2 42 2016 Michael Stuart
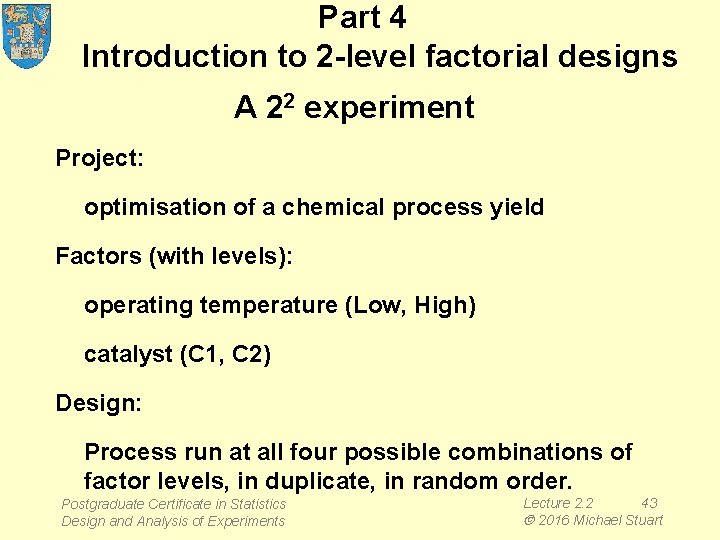
Part 4 Introduction to 2 -level factorial designs A 22 experiment Project: optimisation of a chemical process yield Factors (with levels): operating temperature (Low, High) catalyst (C 1, C 2) Design: Process run at all four possible combinations of factor levels, in duplicate, in random order. Postgraduate Certificate in Statistics Design and Analysis of Experiments Lecture 2. 2 43 2016 Michael Stuart
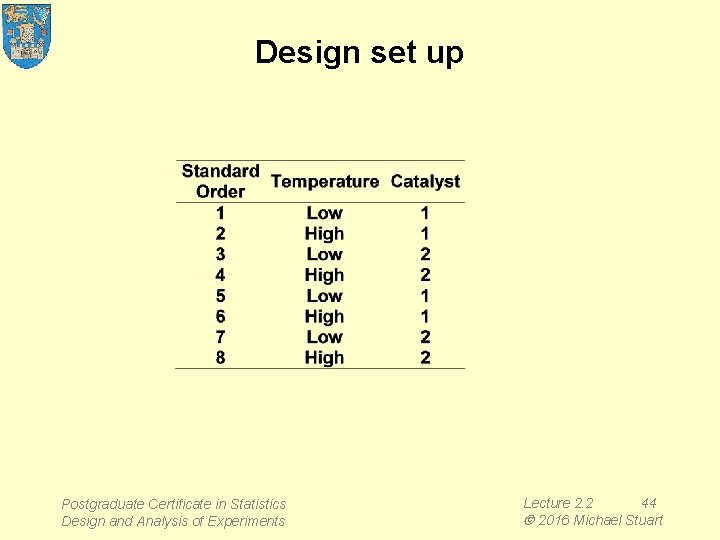
Design set up Postgraduate Certificate in Statistics Design and Analysis of Experiments Lecture 2. 2 44 2016 Michael Stuart
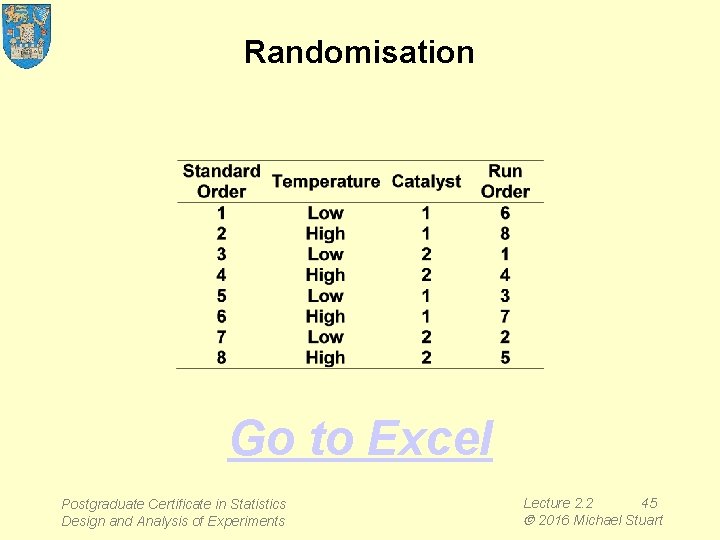
Randomisation Go to Excel Postgraduate Certificate in Statistics Design and Analysis of Experiments Lecture 2. 2 45 2016 Michael Stuart
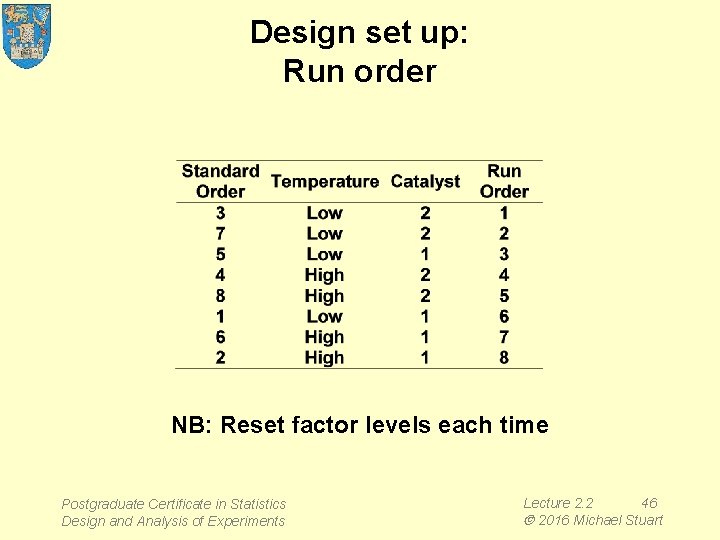
Design set up: Run order NB: Reset factor levels each time Postgraduate Certificate in Statistics Design and Analysis of Experiments Lecture 2. 2 46 2016 Michael Stuart
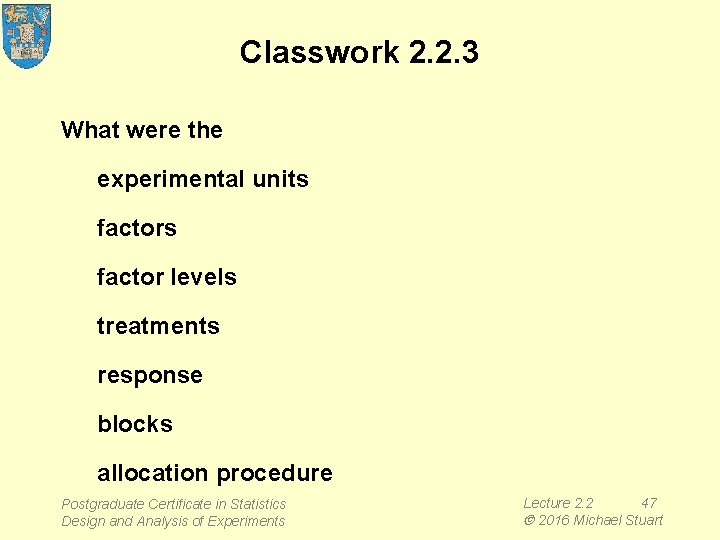
Classwork 2. 2. 3 What were the experimental units factor levels treatments response blocks allocation procedure Postgraduate Certificate in Statistics Design and Analysis of Experiments Lecture 2. 2 47 2016 Michael Stuart
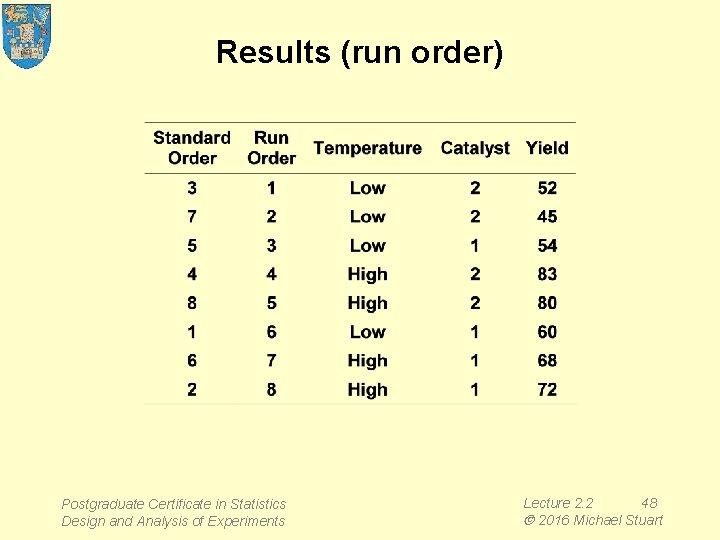
Results (run order) Postgraduate Certificate in Statistics Design and Analysis of Experiments Lecture 2. 2 48 2016 Michael Stuart
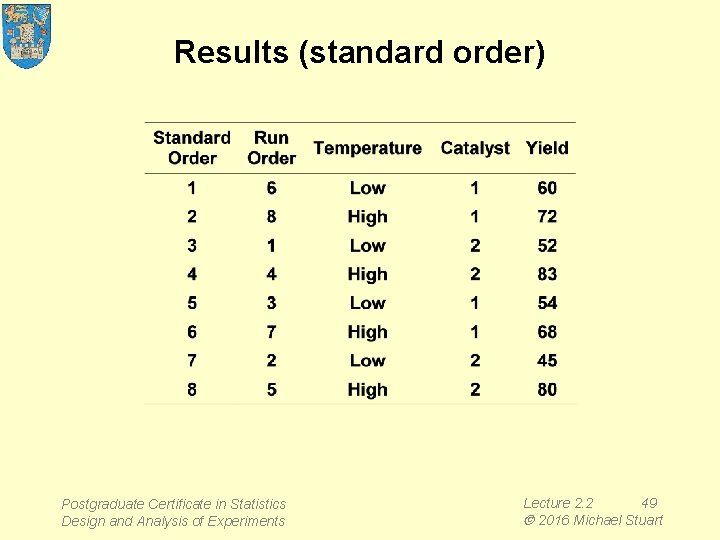
Results (standard order) Postgraduate Certificate in Statistics Design and Analysis of Experiments Lecture 2. 2 49 2016 Michael Stuart
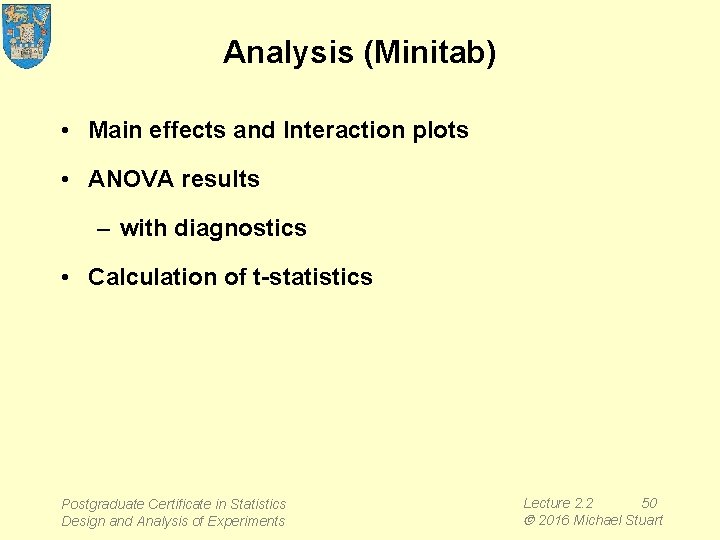
Analysis (Minitab) • Main effects and Interaction plots • ANOVA results – with diagnostics • Calculation of t-statistics Postgraduate Certificate in Statistics Design and Analysis of Experiments Lecture 2. 2 50 2016 Michael Stuart
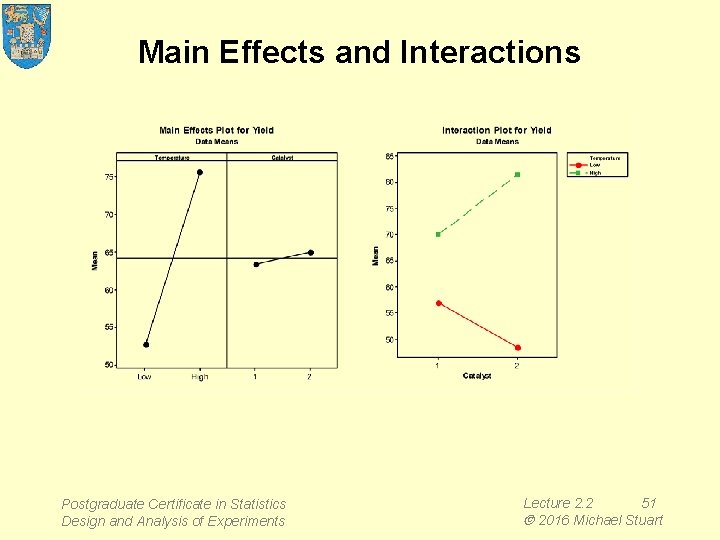
Main Effects and Interactions Postgraduate Certificate in Statistics Design and Analysis of Experiments Lecture 2. 2 51 2016 Michael Stuart
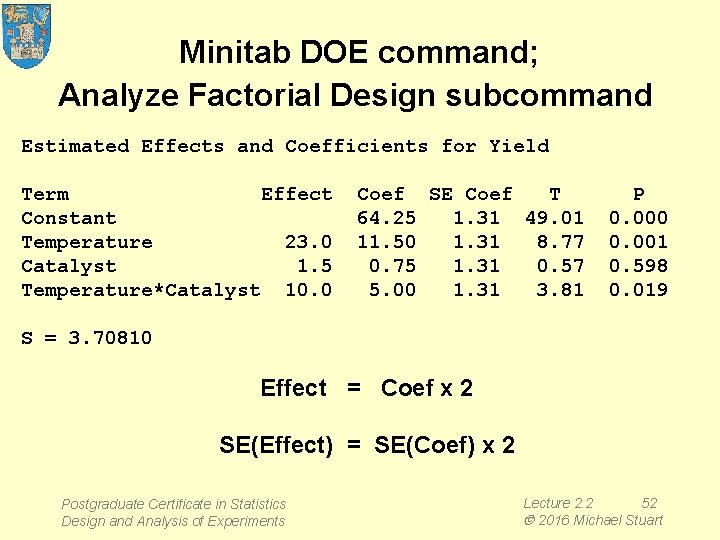
Minitab DOE command; Analyze Factorial Design subcommand Estimated Effects and Coefficients for Yield Term Effect Constant Temperature 23. 0 Catalyst 1. 5 Temperature*Catalyst 10. 0 Coef SE Coef T 64. 25 1. 31 49. 01 11. 50 1. 31 8. 77 0. 75 1. 31 0. 57 5. 00 1. 31 3. 81 P 0. 000 0. 001 0. 598 0. 019 S = 3. 70810 Effect = Coef x 2 SE(Effect) = SE(Coef) x 2 Postgraduate Certificate in Statistics Design and Analysis of Experiments Lecture 2. 2 52 2016 Michael Stuart
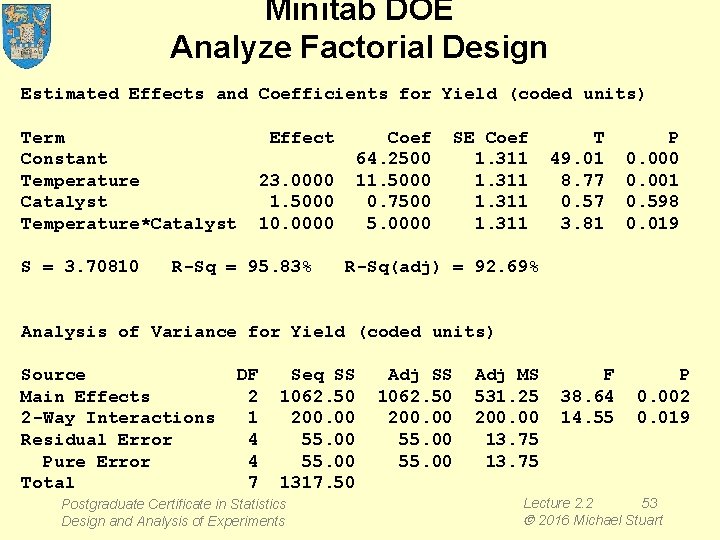
Minitab DOE Analyze Factorial Design Estimated Effects and Coefficients for Yield (coded units) Term Constant Temperature Catalyst Temperature*Catalyst S = 3. 70810 Effect Coef 64. 2500 11. 5000 0. 7500 5. 0000 23. 0000 1. 5000 10. 0000 R-Sq = 95. 83% SE Coef 1. 311 T 49. 01 8. 77 0. 57 3. 81 P 0. 000 0. 001 0. 598 0. 019 R-Sq(adj) = 92. 69% Analysis of Variance for Yield (coded units) Source Main Effects 2 -Way Interactions Residual Error Pure Error Total DF 2 1 4 4 7 Seq SS 1062. 50 200. 00 55. 00 1317. 50 Postgraduate Certificate in Statistics Design and Analysis of Experiments Adj SS 1062. 50 200. 00 55. 00 Adj MS 531. 25 200. 00 13. 75 F 38. 64 14. 55 P 0. 002 0. 019 Lecture 2. 2 53 2016 Michael Stuart
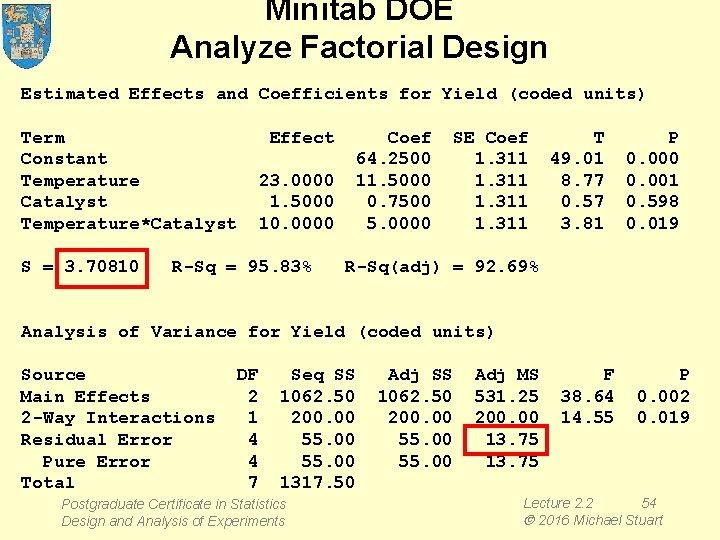
Minitab DOE Analyze Factorial Design Estimated Effects and Coefficients for Yield (coded units) Term Constant Temperature Catalyst Temperature*Catalyst S = 3. 70810 Effect Coef 64. 2500 11. 5000 0. 7500 5. 0000 23. 0000 1. 5000 10. 0000 R-Sq = 95. 83% SE Coef 1. 311 T 49. 01 8. 77 0. 57 3. 81 P 0. 000 0. 001 0. 598 0. 019 R-Sq(adj) = 92. 69% Analysis of Variance for Yield (coded units) Source Main Effects 2 -Way Interactions Residual Error Pure Error Total DF 2 1 4 4 7 Seq SS 1062. 50 200. 00 55. 00 1317. 50 Postgraduate Certificate in Statistics Design and Analysis of Experiments Adj SS 1062. 50 200. 00 55. 00 Adj MS 531. 25 200. 00 13. 75 F 38. 64 14. 55 P 0. 002 0. 019 Lecture 2. 2 54 2016 Michael Stuart
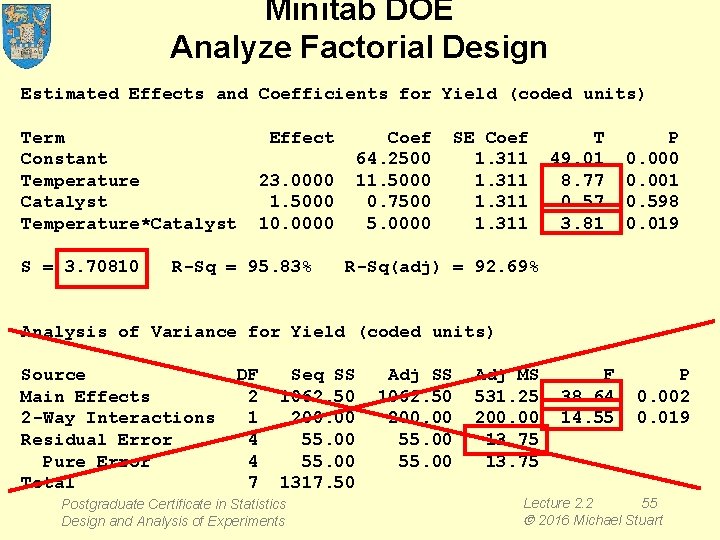
Minitab DOE Analyze Factorial Design Estimated Effects and Coefficients for Yield (coded units) Term Constant Temperature Catalyst Temperature*Catalyst S = 3. 70810 Effect Coef 64. 2500 11. 5000 0. 7500 5. 0000 23. 0000 1. 5000 10. 0000 R-Sq = 95. 83% SE Coef 1. 311 T 49. 01 8. 77 0. 57 3. 81 P 0. 000 0. 001 0. 598 0. 019 R-Sq(adj) = 92. 69% Analysis of Variance for Yield (coded units) Source Main Effects 2 -Way Interactions Residual Error Pure Error Total DF 2 1 4 4 7 Seq SS 1062. 50 200. 00 55. 00 1317. 50 Postgraduate Certificate in Statistics Design and Analysis of Experiments Adj SS 1062. 50 200. 00 55. 00 Adj MS 531. 25 200. 00 13. 75 F 38. 64 14. 55 P 0. 002 0. 019 Lecture 2. 2 55 2016 Michael Stuart
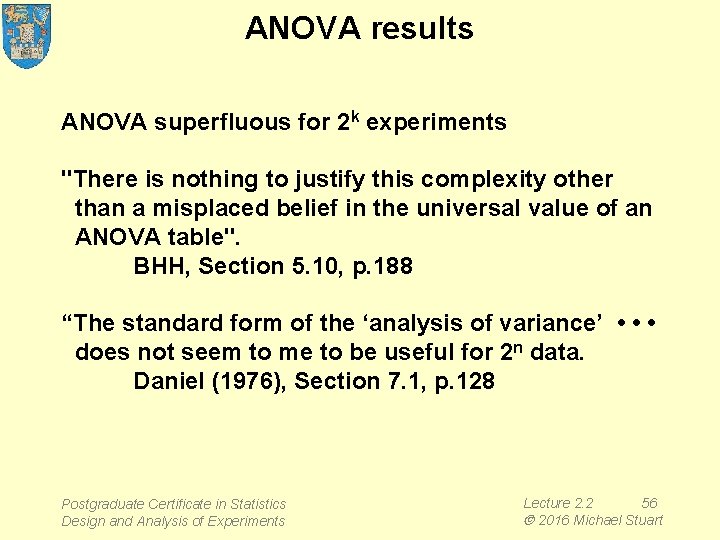
ANOVA results ANOVA superfluous for 2 k experiments "There is nothing to justify this complexity other than a misplaced belief in the universal value of an ANOVA table". BHH, Section 5. 10, p. 188 “The standard form of the ‘analysis of variance’ • • • does not seem to me to be useful for 2 n data. Daniel (1976), Section 7. 1, p. 128 Postgraduate Certificate in Statistics Design and Analysis of Experiments Lecture 2. 2 56 2016 Michael Stuart
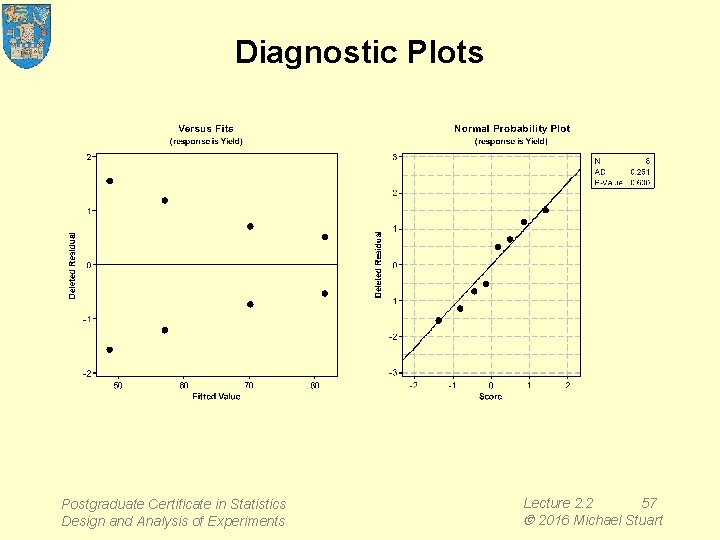
Diagnostic Plots Postgraduate Certificate in Statistics Design and Analysis of Experiments Lecture 2. 2 57 2016 Michael Stuart
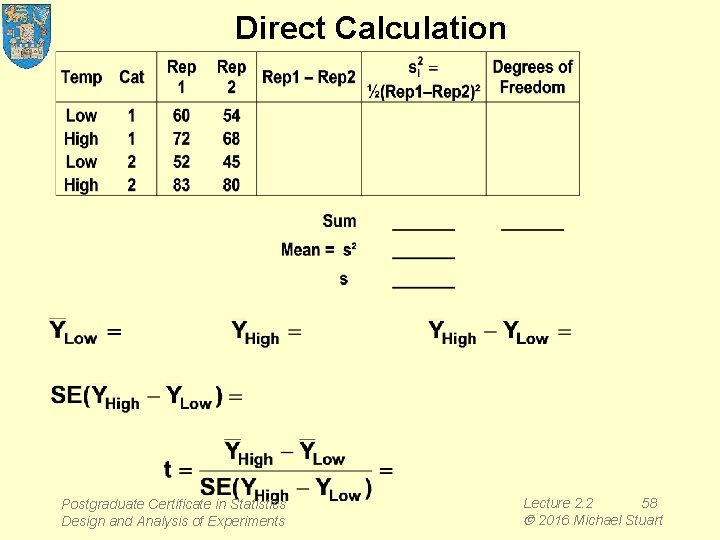
Direct Calculation Postgraduate Certificate in Statistics Design and Analysis of Experiments Lecture 2. 2 58 2016 Michael Stuart
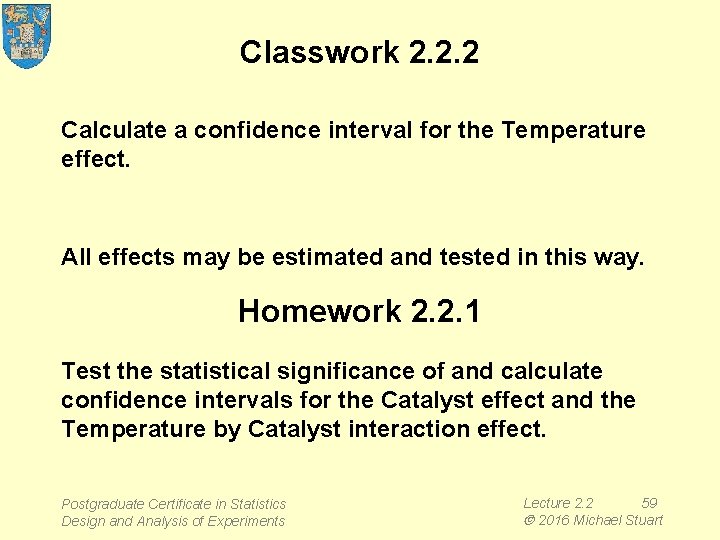
Classwork 2. 2. 2 Calculate a confidence interval for the Temperature effect. All effects may be estimated and tested in this way. Homework 2. 2. 1 Test the statistical significance of and calculate confidence intervals for the Catalyst effect and the Temperature by Catalyst interaction effect. Postgraduate Certificate in Statistics Design and Analysis of Experiments Lecture 2. 2 59 2016 Michael Stuart
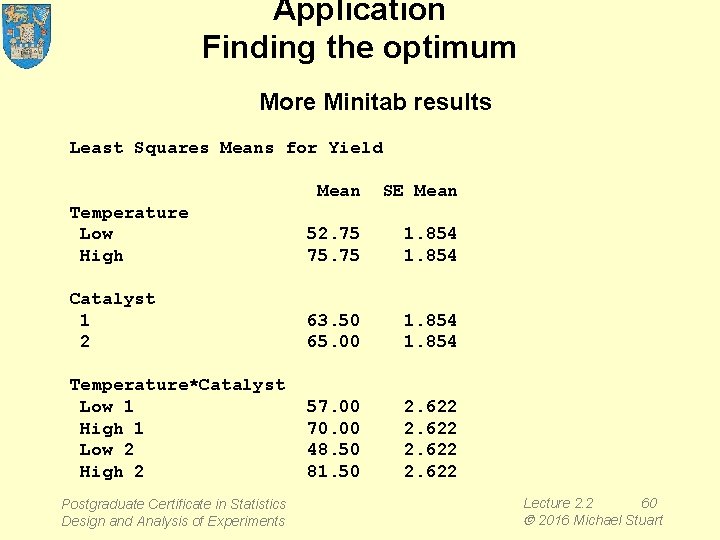
Application Finding the optimum More Minitab results Least Squares Means for Yield Mean SE Mean Temperature Low High 52. 75 75. 75 1. 854 Catalyst 1 2 63. 50 65. 00 1. 854 Temperature*Catalyst Low 1 High 1 Low 2 High 2 57. 00 70. 00 48. 50 81. 50 2. 622 Postgraduate Certificate in Statistics Design and Analysis of Experiments Lecture 2. 2 60 2016 Michael Stuart
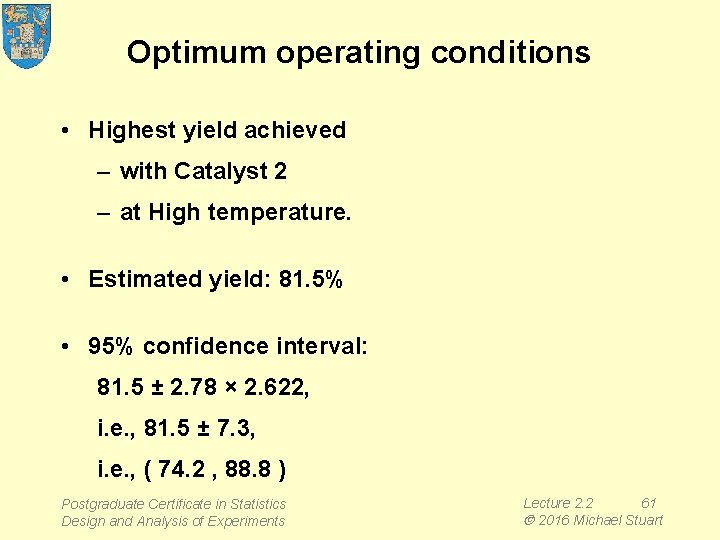
Optimum operating conditions • Highest yield achieved – with Catalyst 2 – at High temperature. • Estimated yield: 81. 5% • 95% confidence interval: 81. 5 ± 2. 78 × 2. 622, i. e. , 81. 5 ± 7. 3, i. e. , ( 74. 2 , 88. 8 ) Postgraduate Certificate in Statistics Design and Analysis of Experiments Lecture 2. 2 61 2016 Michael Stuart
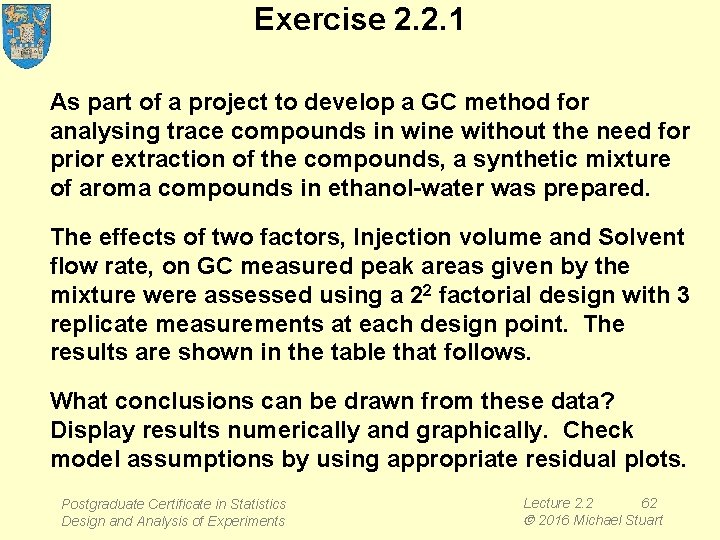
Exercise 2. 2. 1 As part of a project to develop a GC method for analysing trace compounds in wine without the need for prior extraction of the compounds, a synthetic mixture of aroma compounds in ethanol-water was prepared. The effects of two factors, Injection volume and Solvent flow rate, on GC measured peak areas given by the mixture were assessed using a 22 factorial design with 3 replicate measurements at each design point. The results are shown in the table that follows. What conclusions can be drawn from these data? Display results numerically and graphically. Check model assumptions by using appropriate residual plots. Postgraduate Certificate in Statistics Design and Analysis of Experiments Lecture 2. 2 62 2016 Michael Stuart
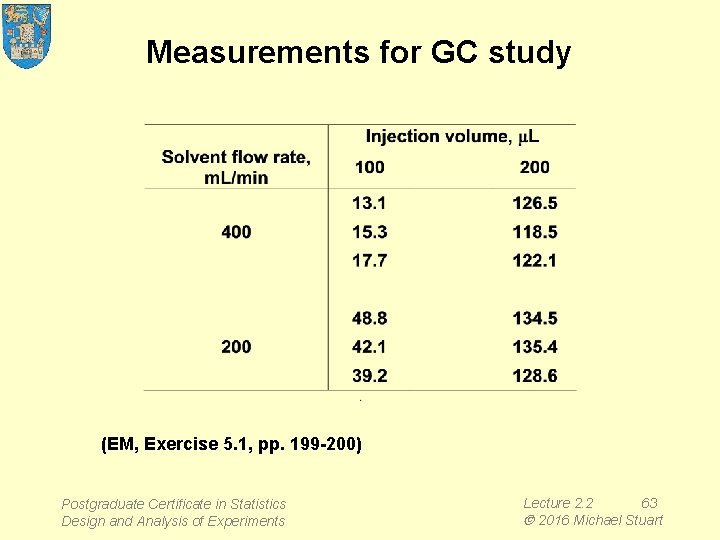
Measurements for GC study (EM, Exercise 5. 1, pp. 199 -200) Postgraduate Certificate in Statistics Design and Analysis of Experiments Lecture 2. 2 63 2016 Michael Stuart
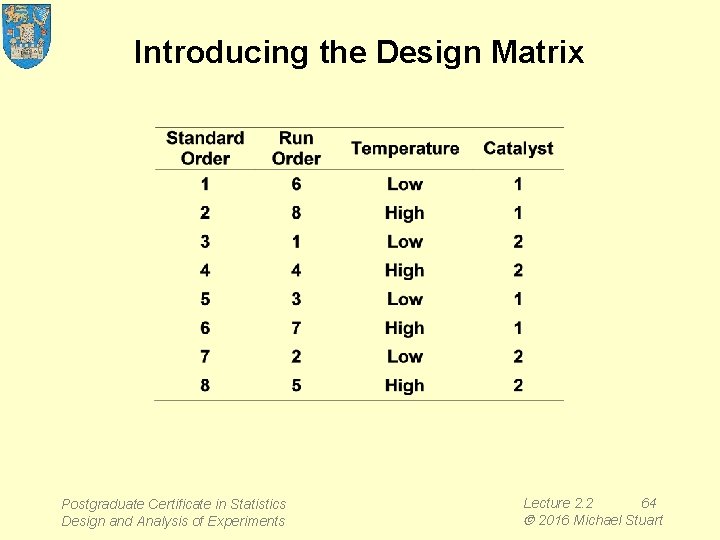
Introducing the Design Matrix Postgraduate Certificate in Statistics Design and Analysis of Experiments Lecture 2. 2 64 2016 Michael Stuart
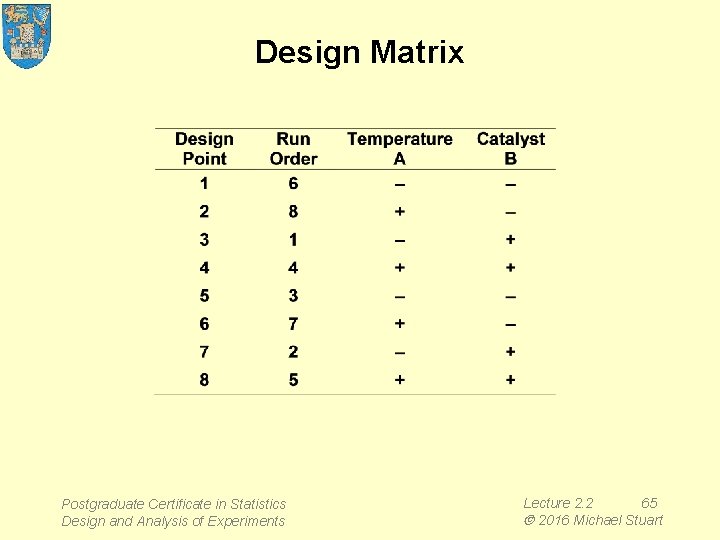
Design Matrix Postgraduate Certificate in Statistics Design and Analysis of Experiments Lecture 2. 2 65 2016 Michael Stuart
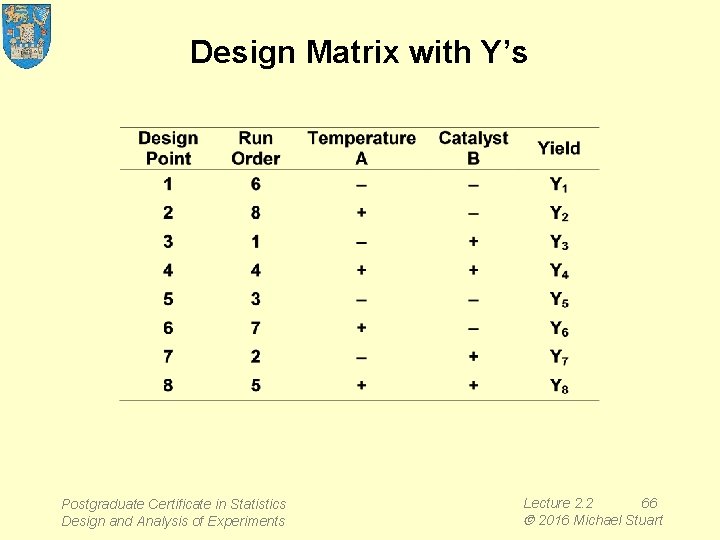
Design Matrix with Y’s Postgraduate Certificate in Statistics Design and Analysis of Experiments Lecture 2. 2 66 2016 Michael Stuart
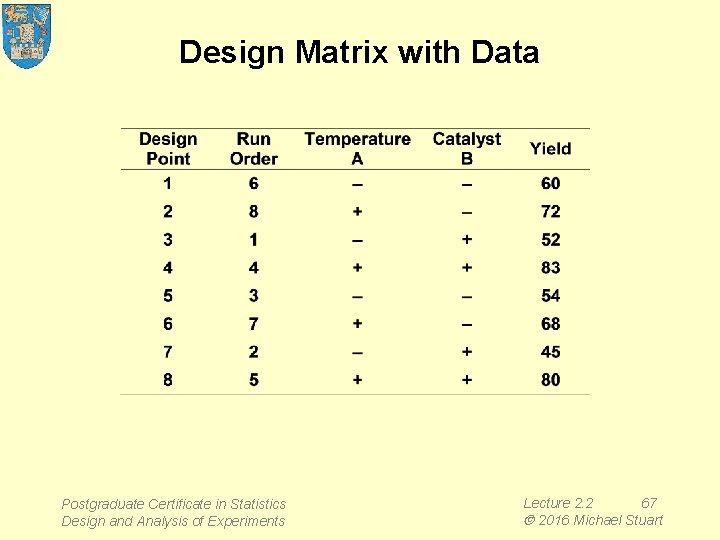
Design Matrix with Data Postgraduate Certificate in Statistics Design and Analysis of Experiments Lecture 2. 2 67 2016 Michael Stuart
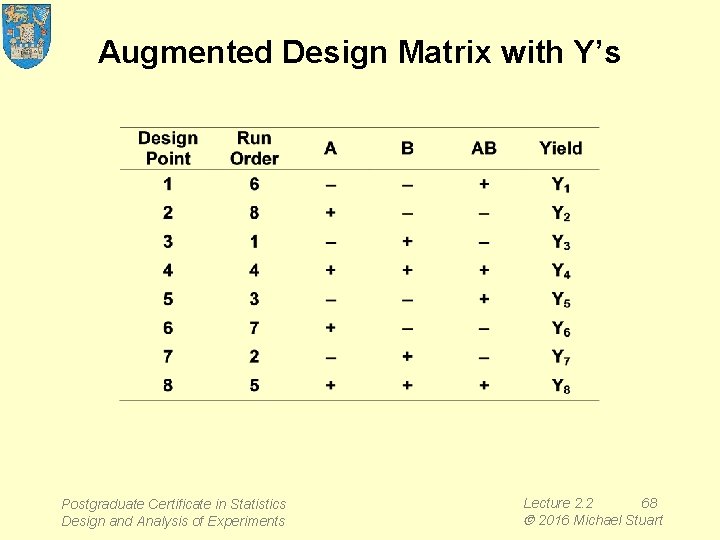
Augmented Design Matrix with Y’s Postgraduate Certificate in Statistics Design and Analysis of Experiments Lecture 2. 2 68 2016 Michael Stuart
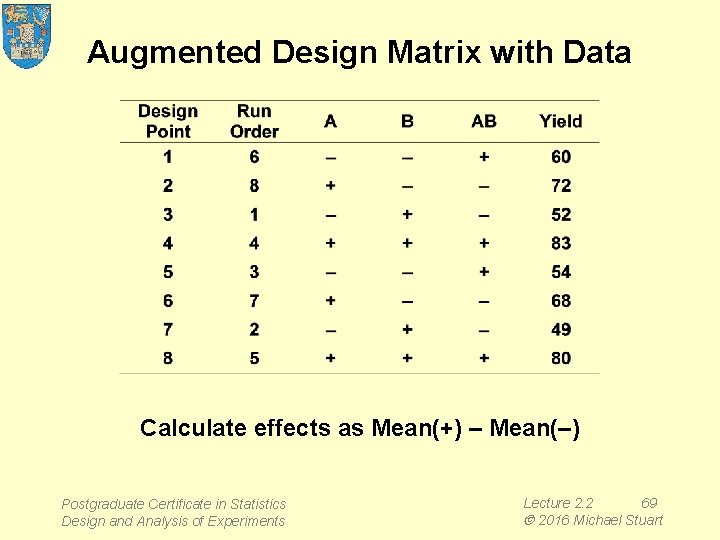
Augmented Design Matrix with Data Calculate effects as Mean(+) – Mean(–) Postgraduate Certificate in Statistics Design and Analysis of Experiments Lecture 2. 2 69 2016 Michael Stuart
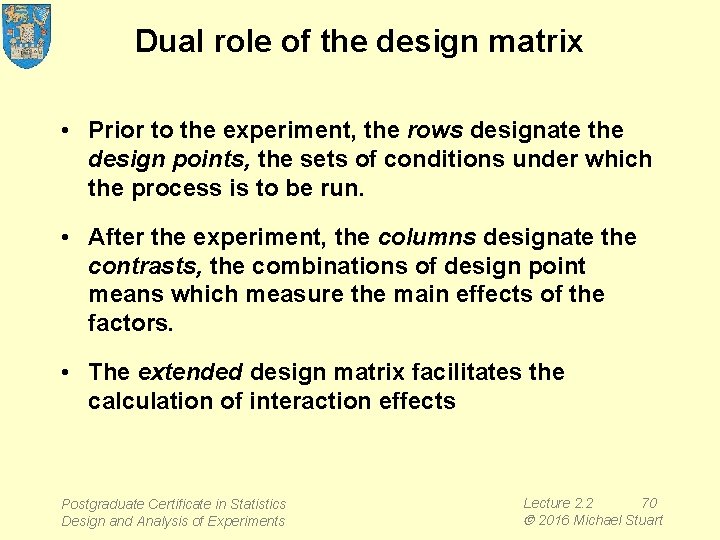
Dual role of the design matrix • Prior to the experiment, the rows designate the design points, the sets of conditions under which the process is to be run. • After the experiment, the columns designate the contrasts, the combinations of design point means which measure the main effects of the factors. • The extended design matrix facilitates the calculation of interaction effects Postgraduate Certificate in Statistics Design and Analysis of Experiments Lecture 2. 2 70 2016 Michael Stuart
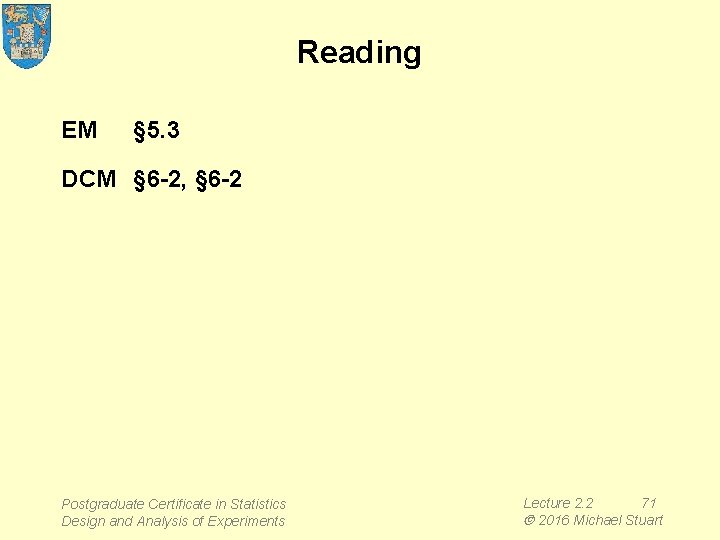
Reading EM § 5. 3 DCM § 6 -2, § 6 -2 Postgraduate Certificate in Statistics Design and Analysis of Experiments Lecture 2. 2 71 2016 Michael Stuart
Ddo vulnerability
01:640:244 lecture notes - lecture 15: plat, idah, farad
Design of experiments doe
Doe in jmp
Full factorial design
Design of experiments pmp example
Design of experiments presentation
Taguchi design of experiments
Pmp stakeholder engagement plan
Design of experiments
Six sigma betekenis
Design of experiments
Input system design
Biased and unbiased samples
What are surveys experiments or observation
2k factorial experiments and fractions
Chapter 13 experiments and observational studies
Miller and urey's experiments attempted to demonstrate
Experiments counting rules and assigning probabilities
Pros and cons of animal research
User interface design in system analysis and design
Dialogue design
Structured design tools
Fact finding and analysis
Organizational feasibility in system analysis and design
Eurocode lap lengths
Elemen urban design
Principles of design in interior design ppt
Lecture hall acoustic design
Game design lecture
Computer-aided drug design lecture notes
Cmos vlsi design lecture notes
Exploratory data analysis lecture notes
Sensitivity analysis lecture notes
Factor analysis lecture notes
Analysis of algorithms lecture notes
Streak plate
Power system analysis lecture notes
Http://earthobservatory.nasa.gov/experiments/biome/
Test your hypothesis
Coordination of secondary circular reactions
Engineering projects vs standard experiments
Science experiments for highschool
Eyewitness testimony video experiments
Computer science experiments
Czech experiments
Frederick griffith 1928
In his transformation experiments what did griffith observe
Merit and demerit of standard deviation
Examples of binomial experiments
Virtual labs iit kharagpur
George a miller ap psychology
Barbara herbert and daphne goodship
Construction leaving cert portfolio
Core flooding experiments
Bank wiring experiment
Experiments in goodness
Colorimetry involve
Counting rule for multiple-step experiments
Abnormal psychology experiments
Why is “replication key” psychology experiments?
True or false hot glass looks the same as cold glass.
Survey vs observational study
Pvt sample
American experiments chapter 2
Why are controlled experiments sometimes impossible
Google analz
Split brain experiments
Integrated science lab experiments
Viking experiments
Checklist for performing laboratory experiments
Risk assessment for science experiments