Deep Knowledge Tracing Chris Piech Jonathan Spencer Jonathan
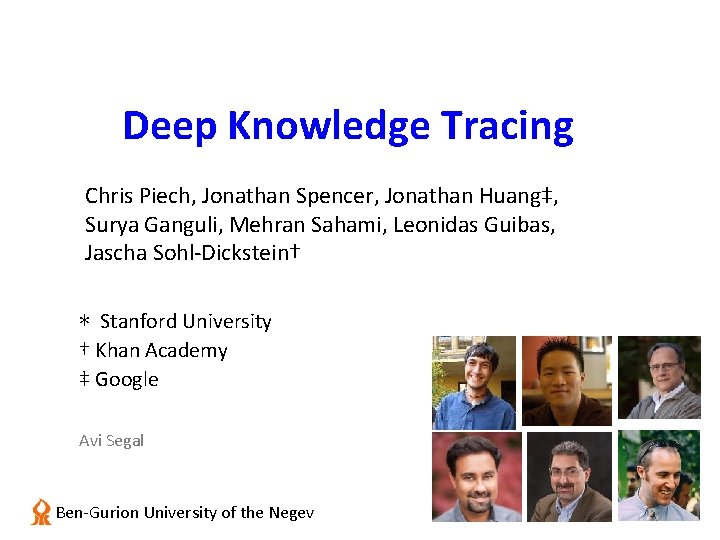
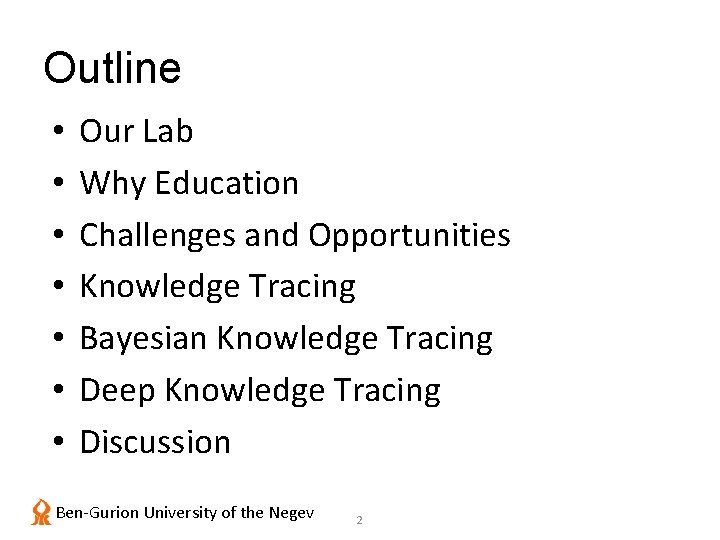
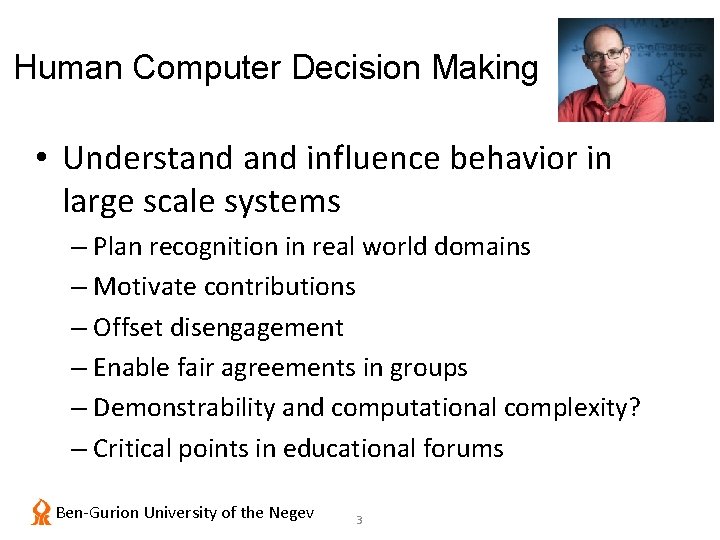
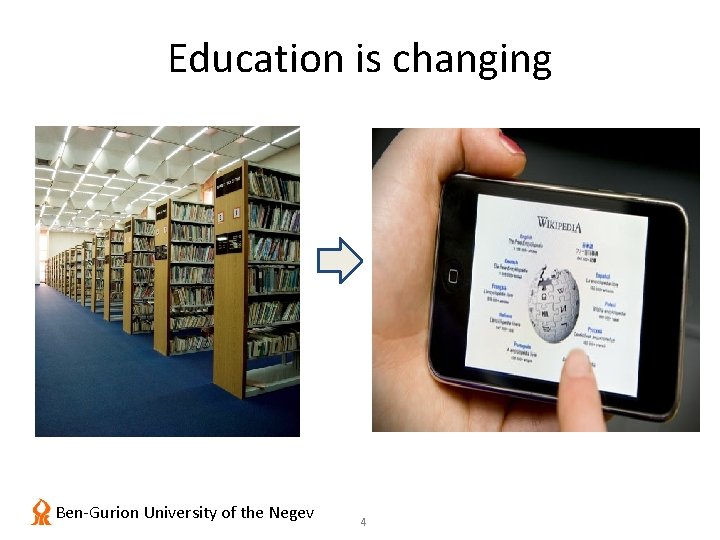
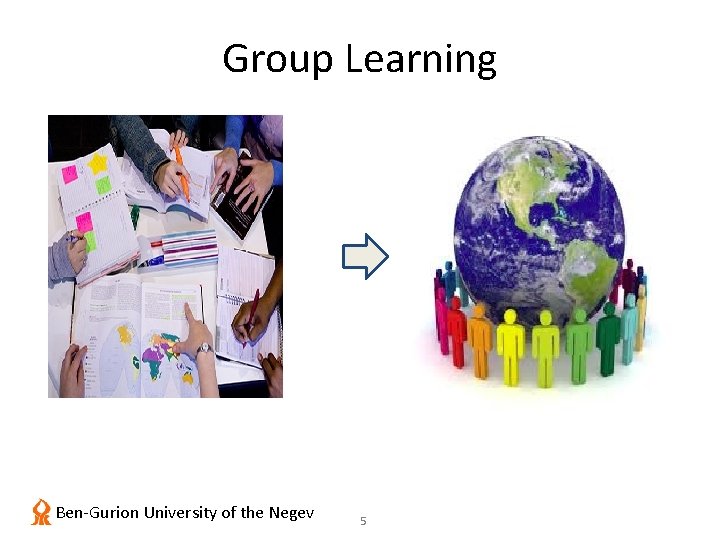
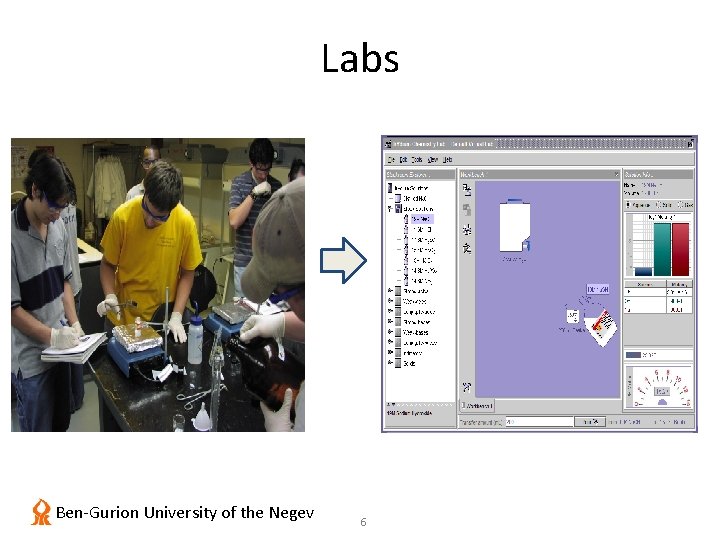
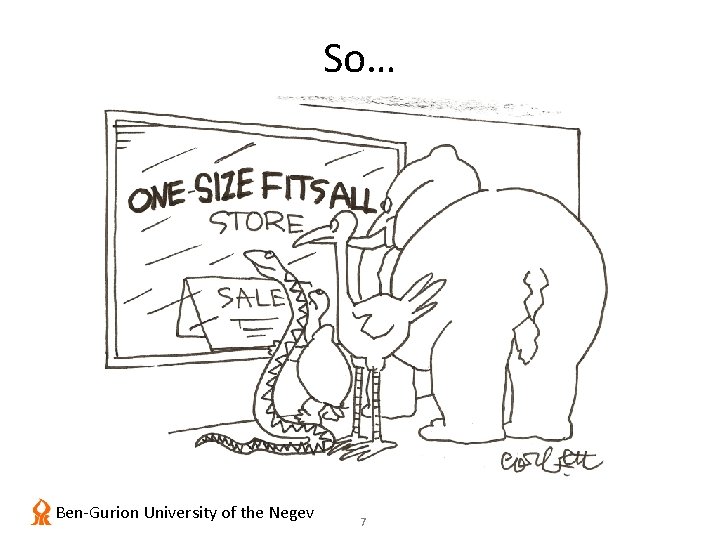
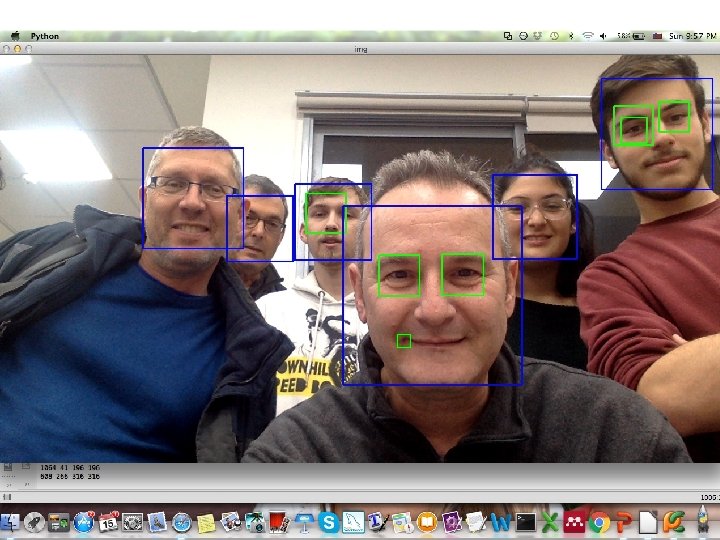
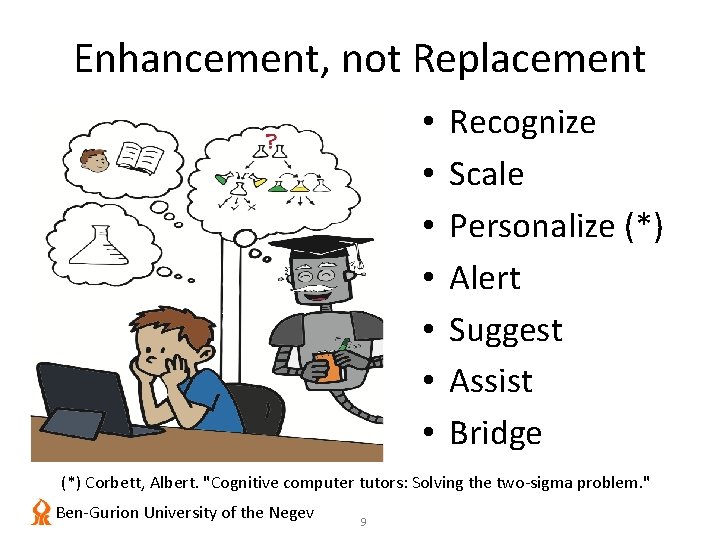
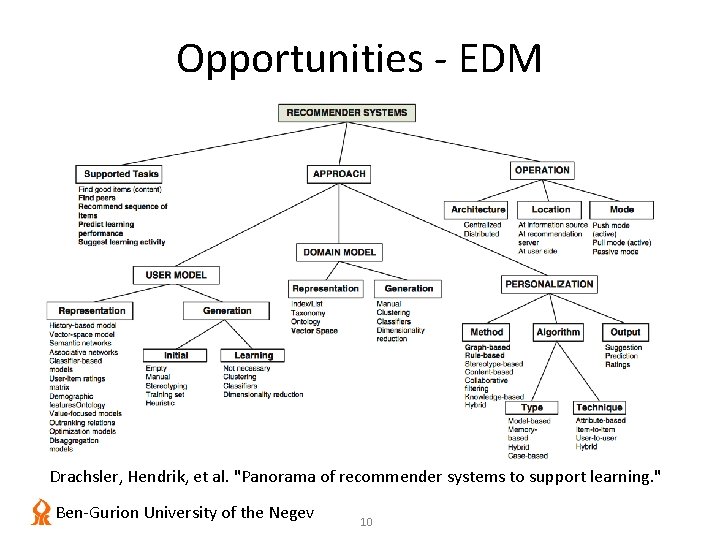
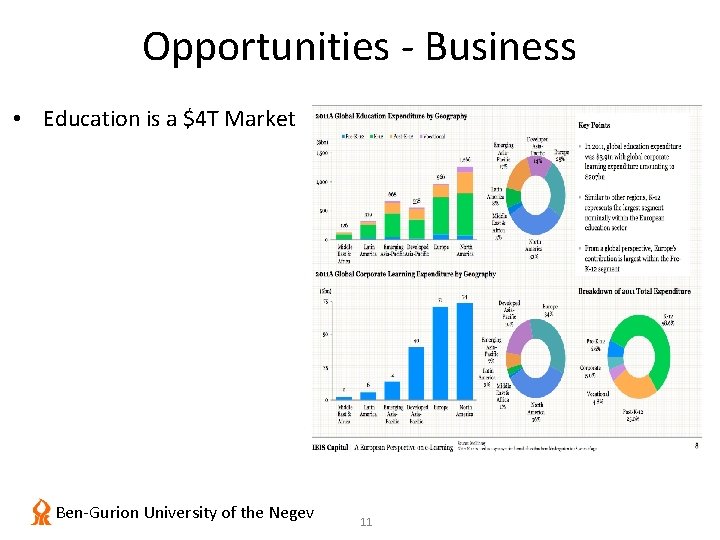
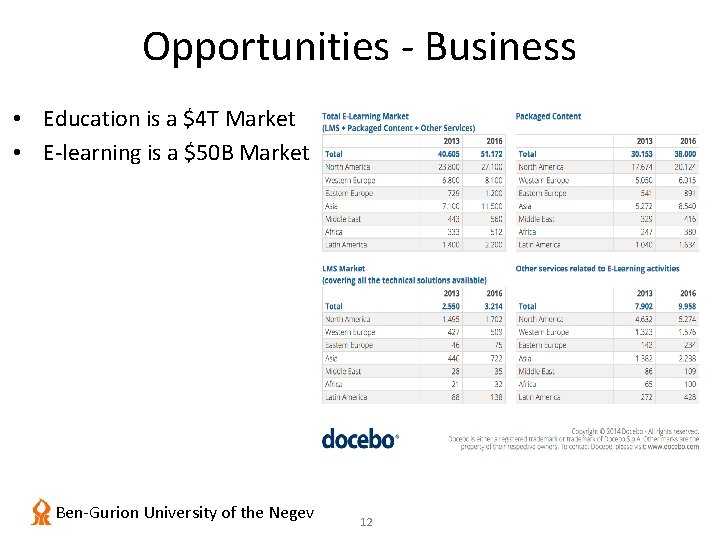
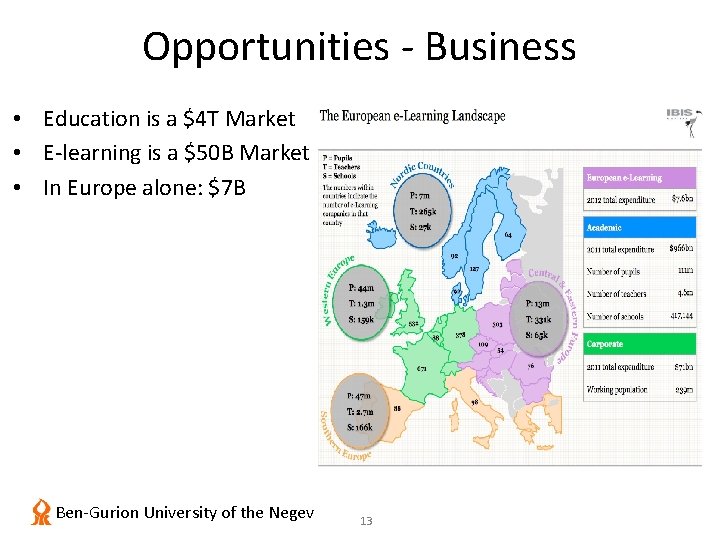
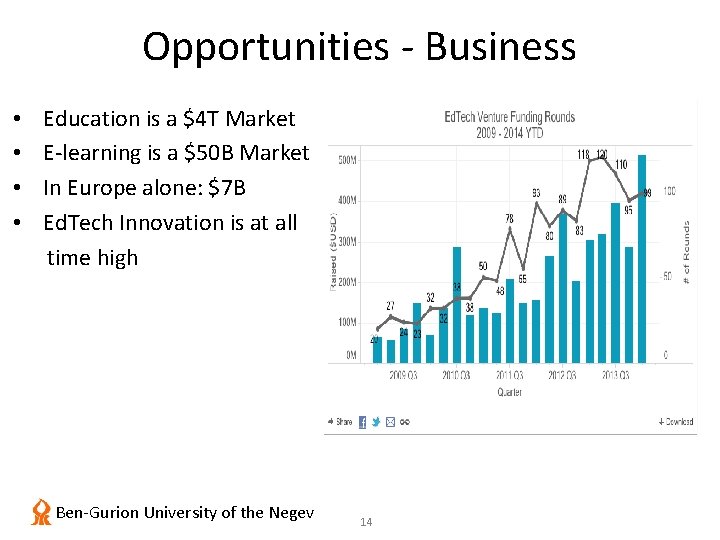
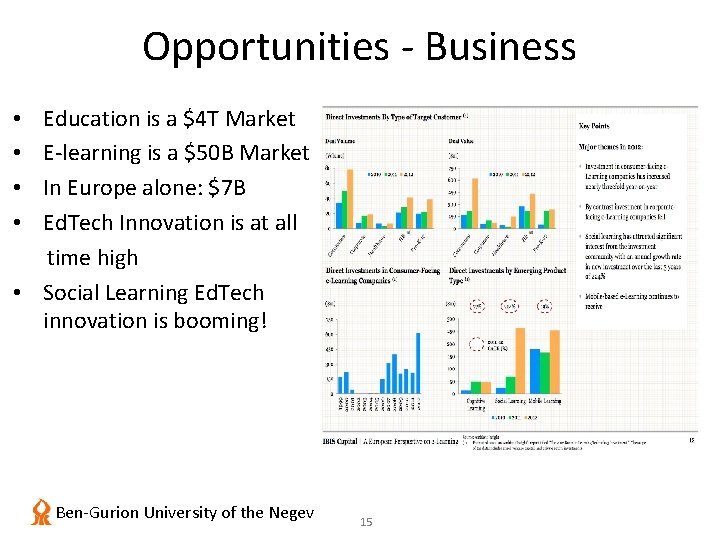
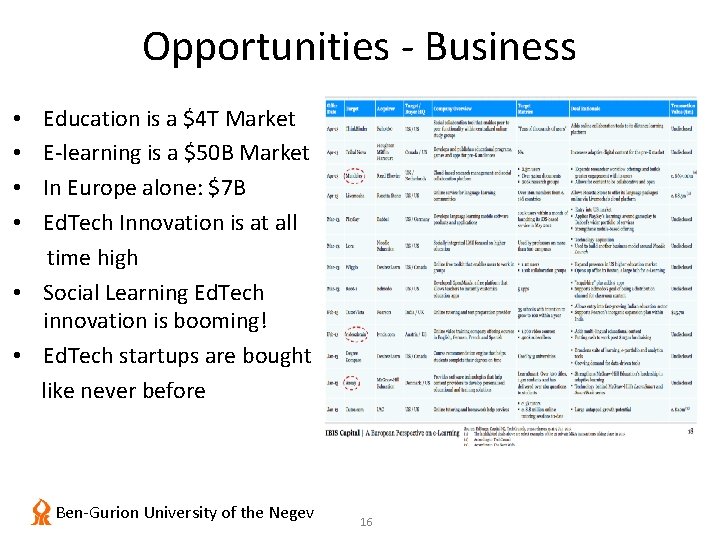
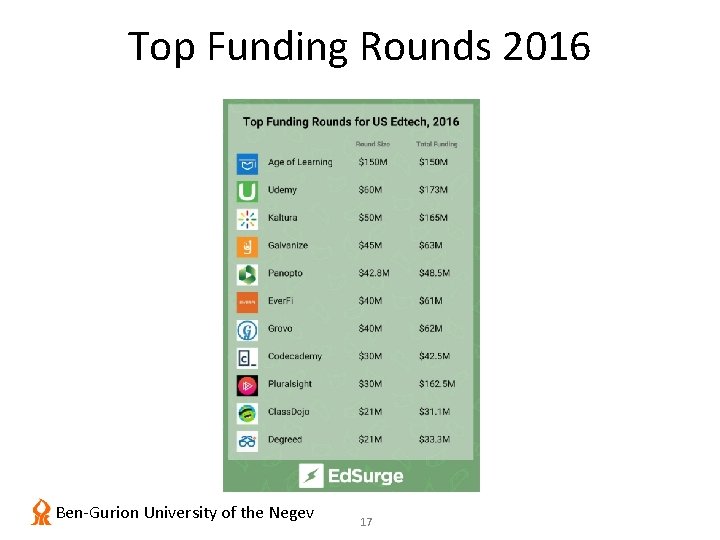
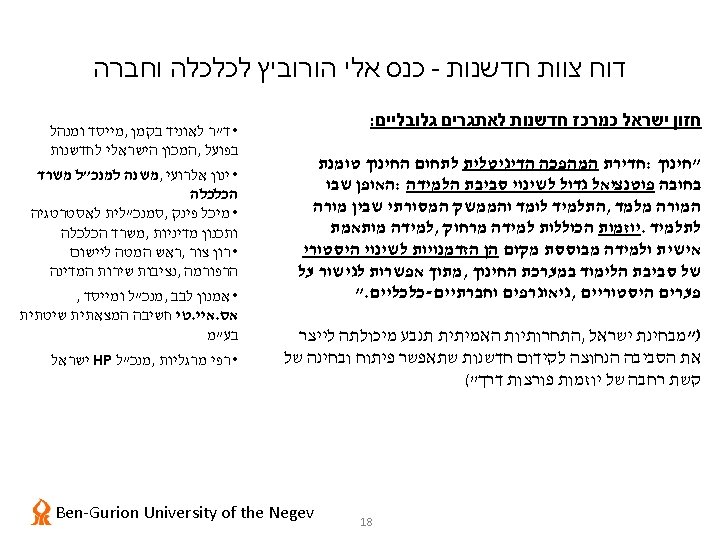
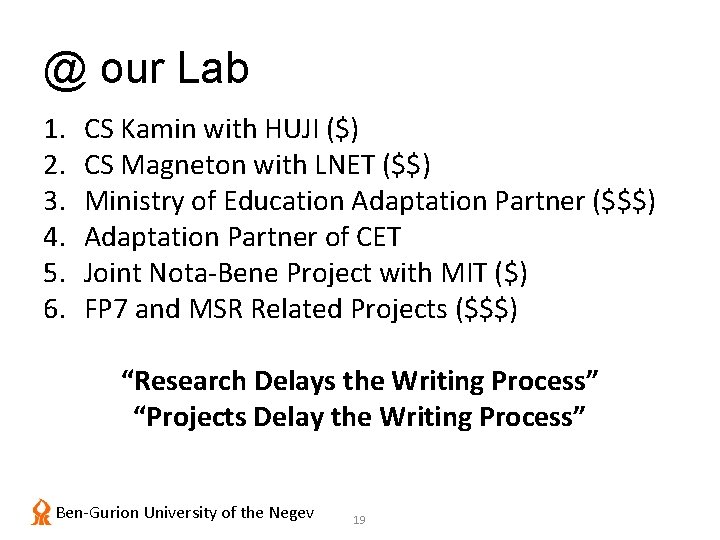
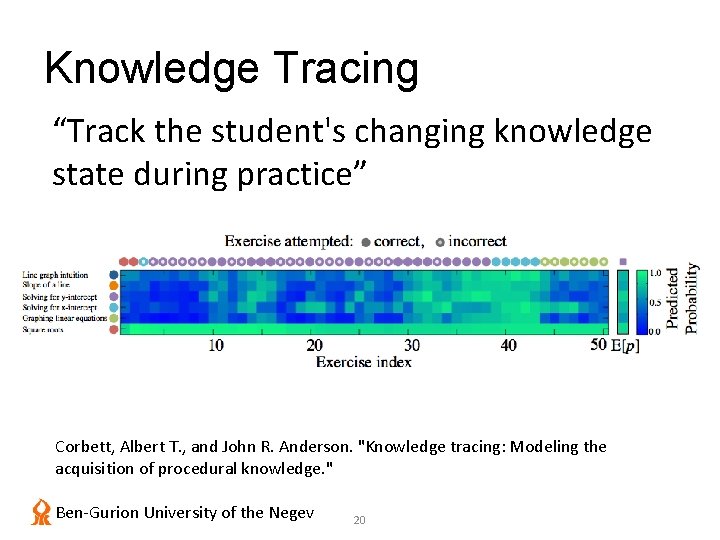
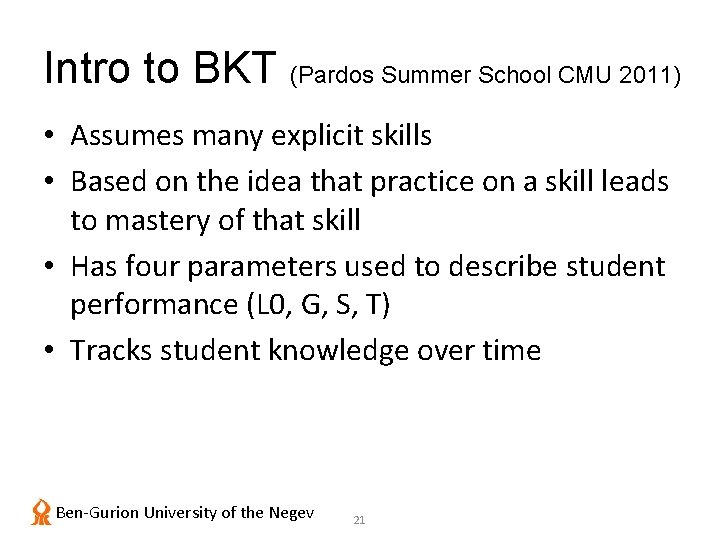
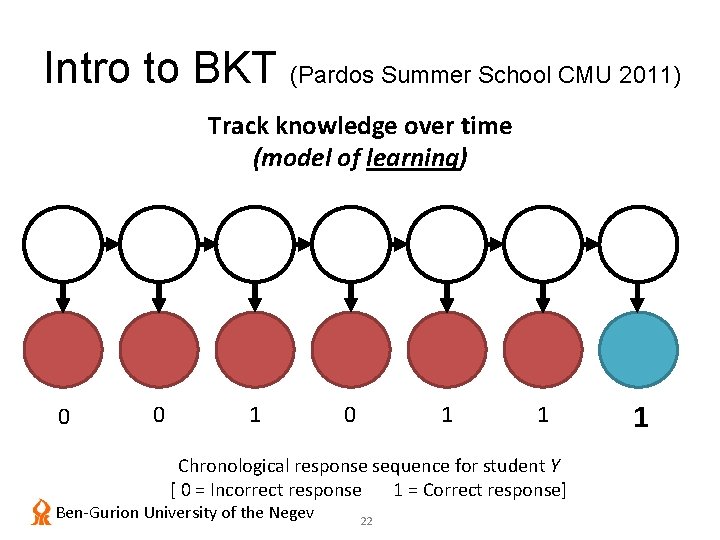
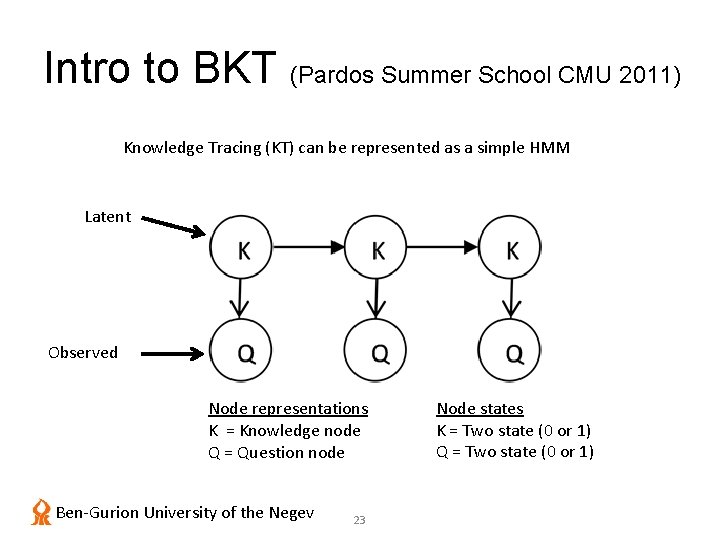
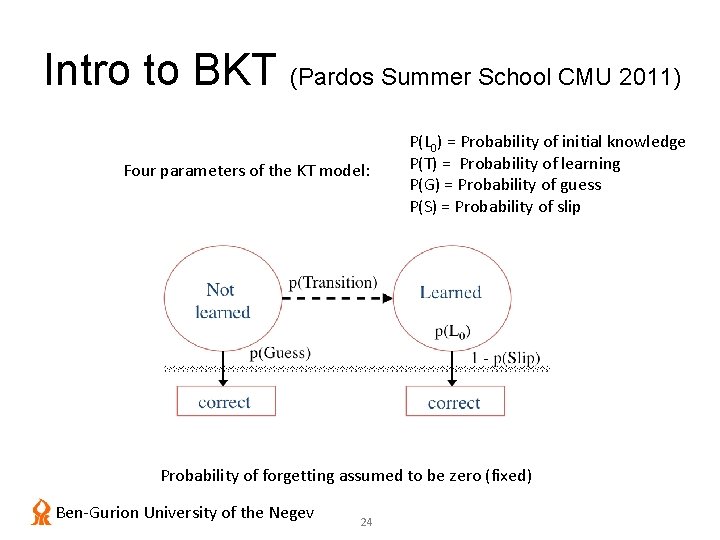
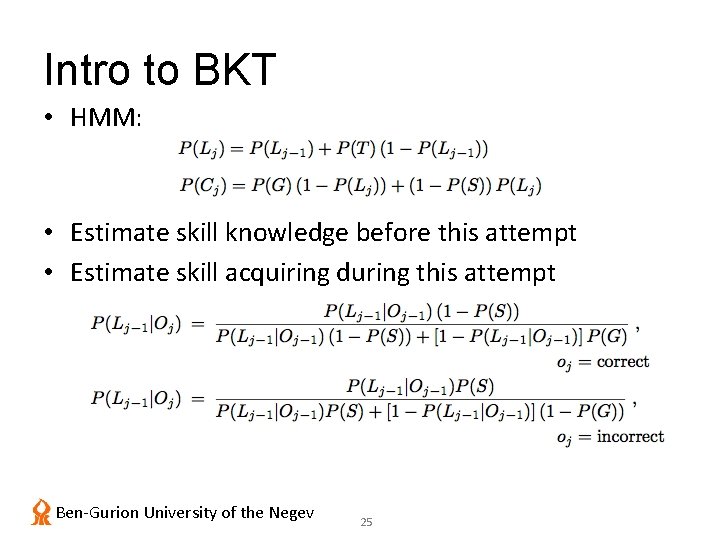
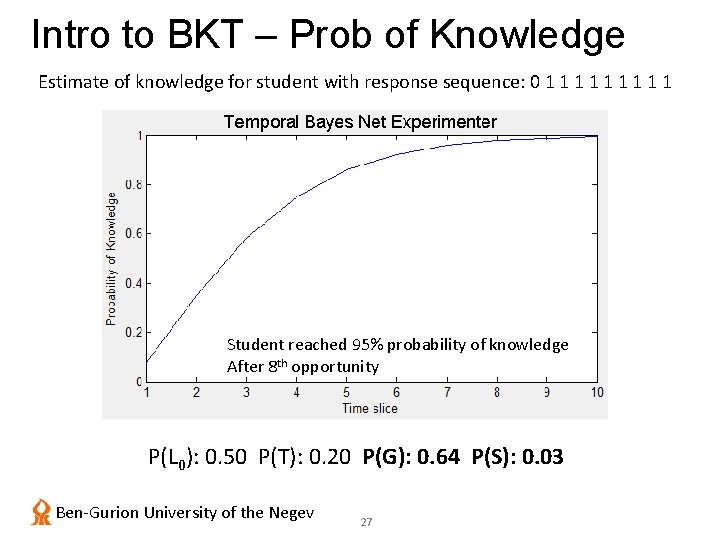
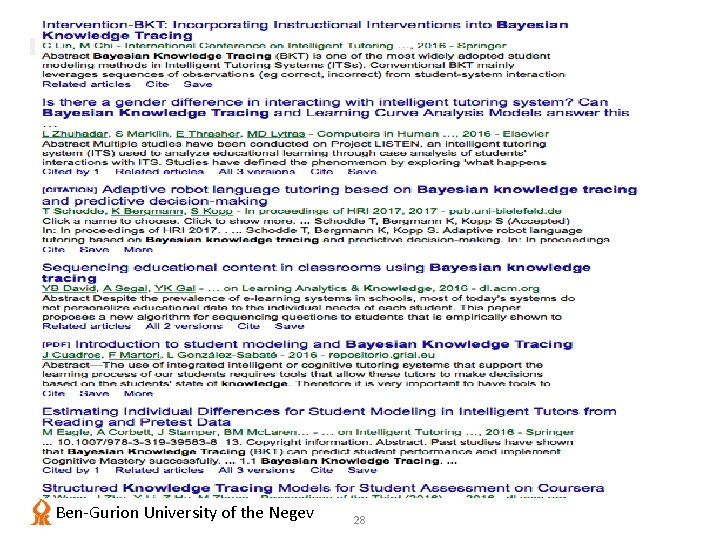
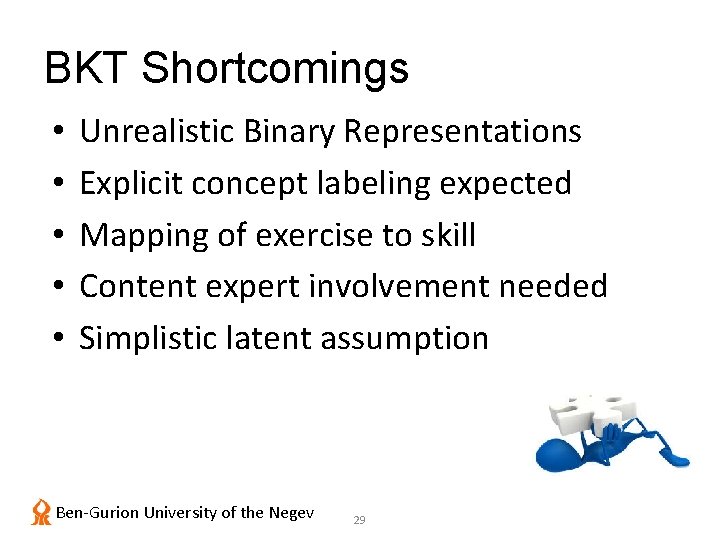
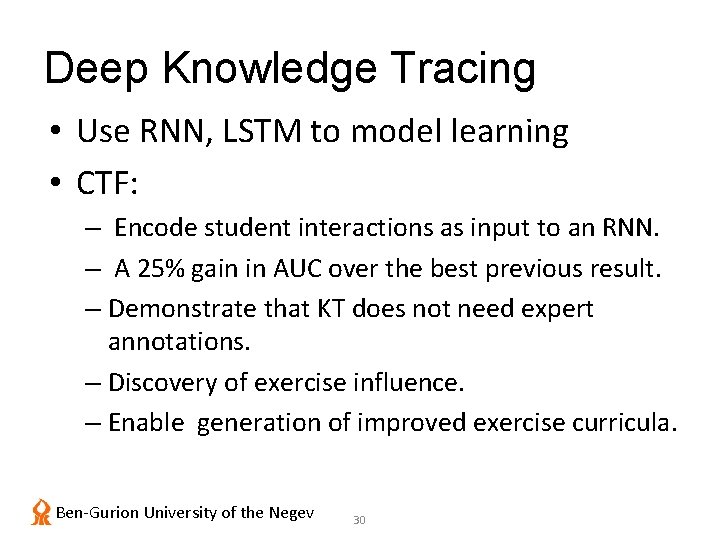
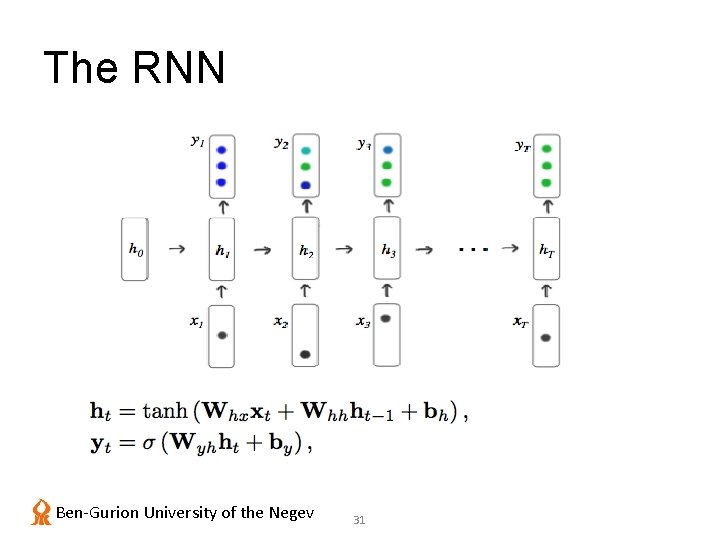
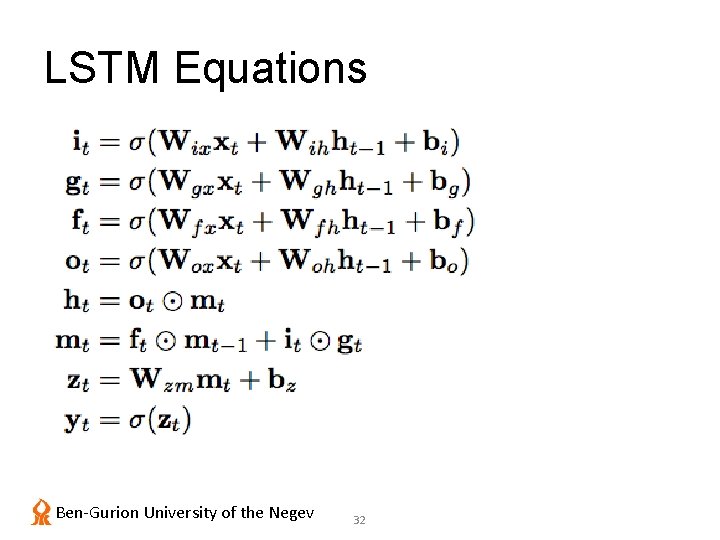
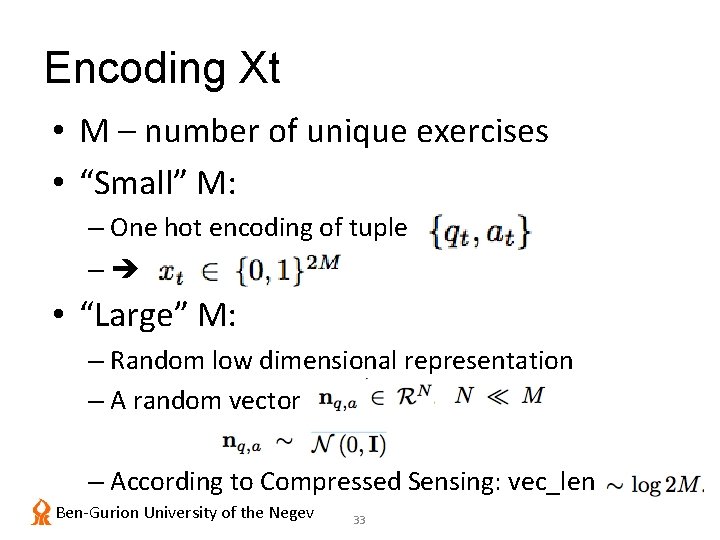
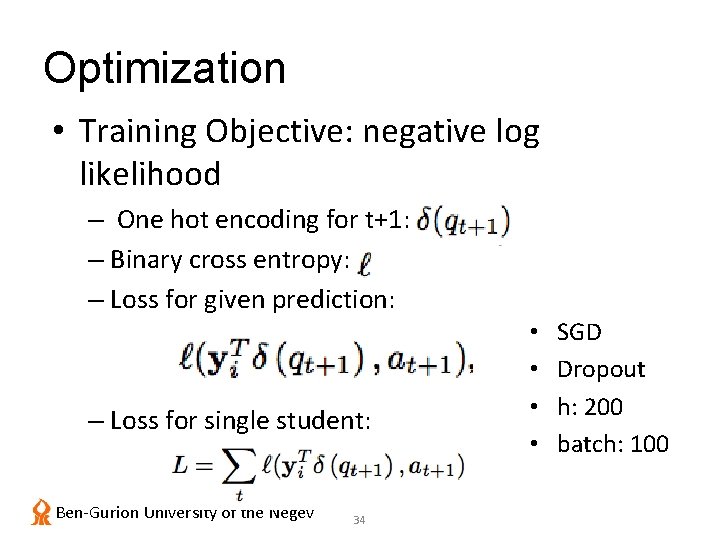
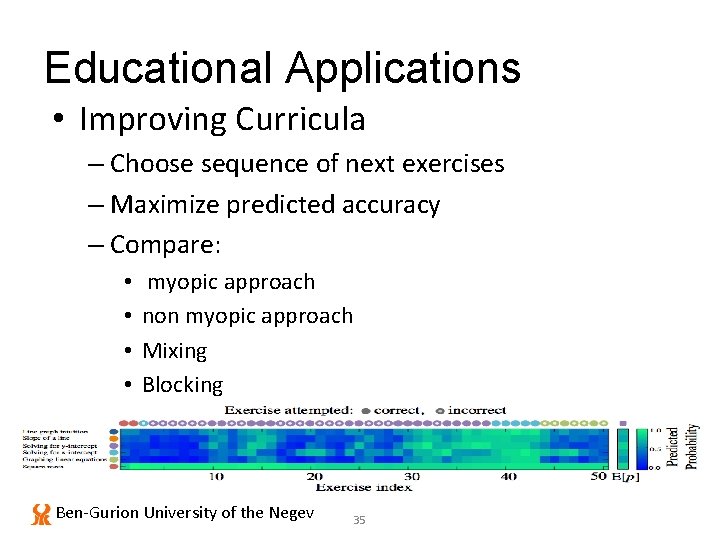
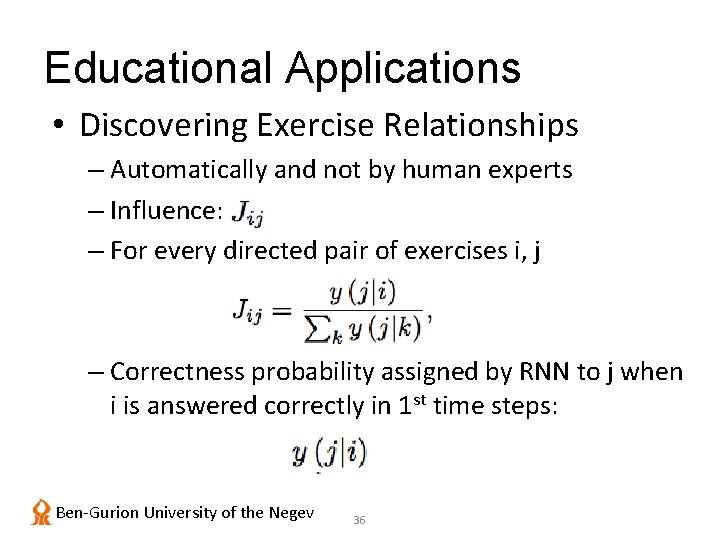
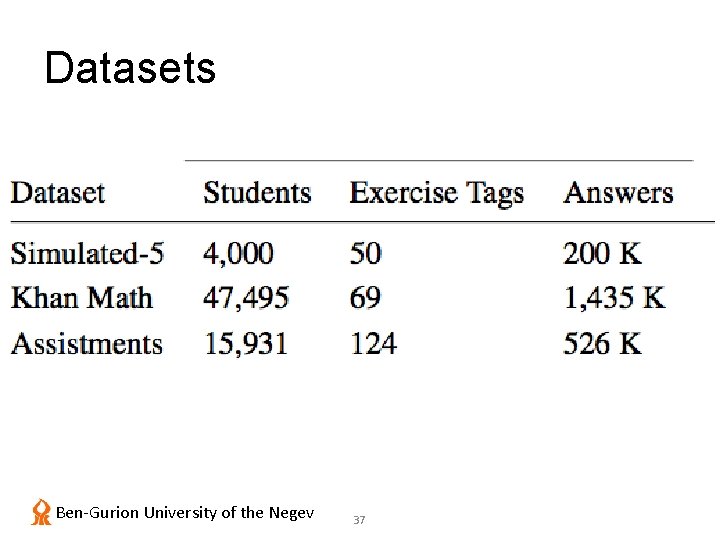
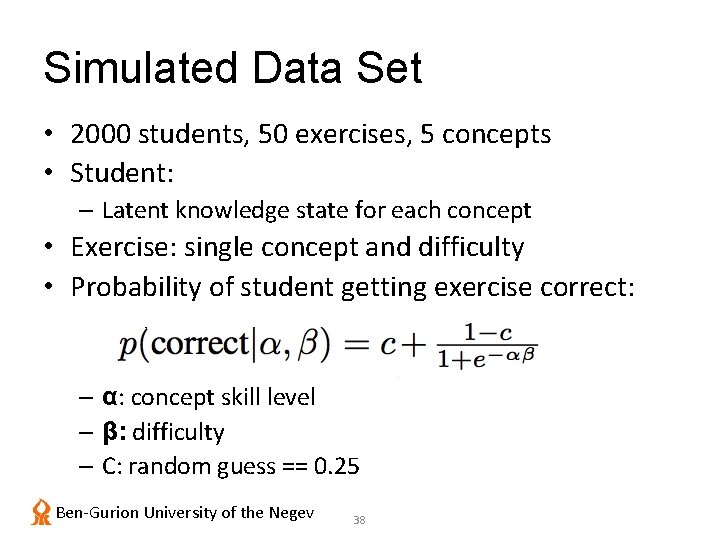
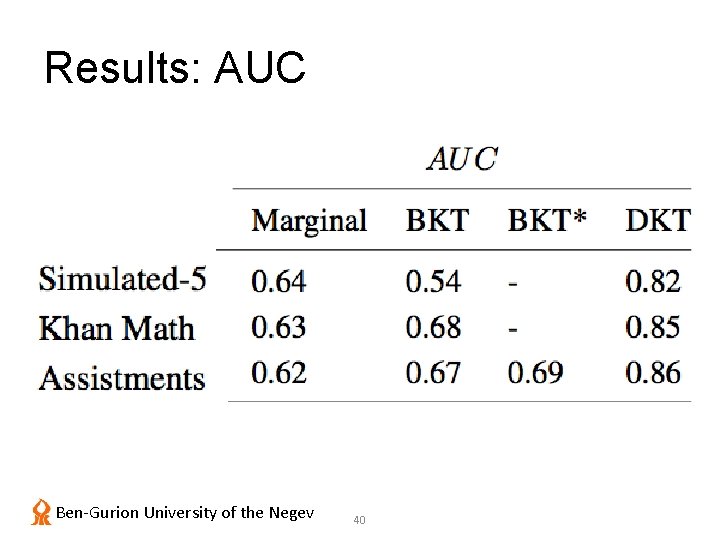
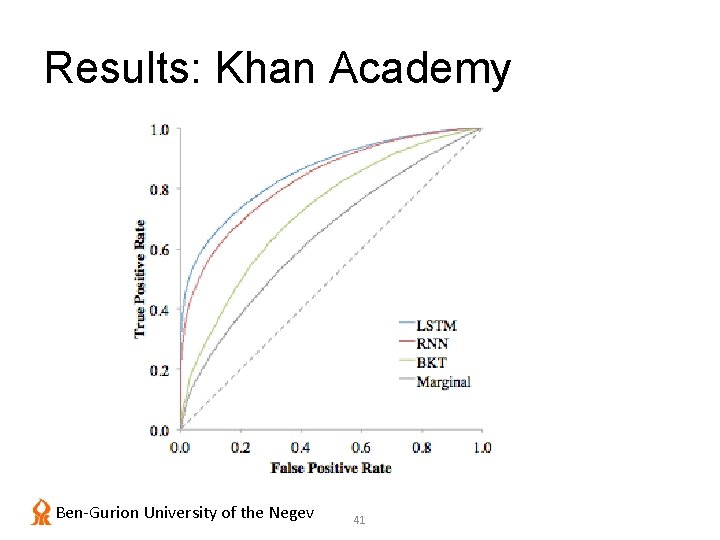
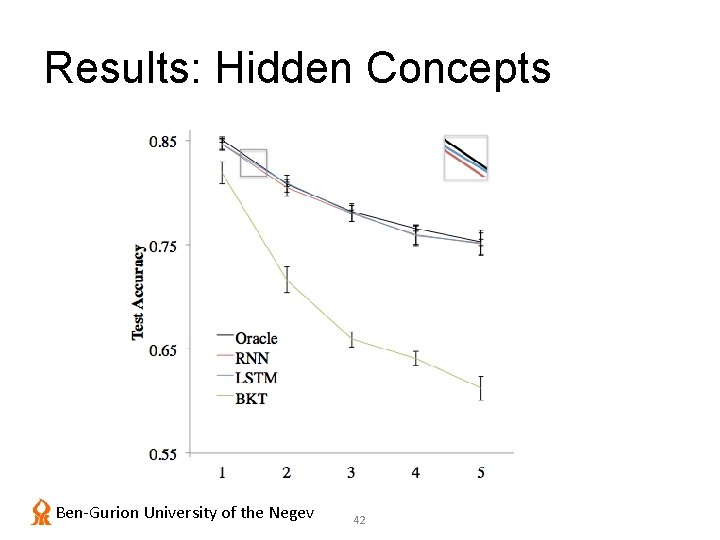
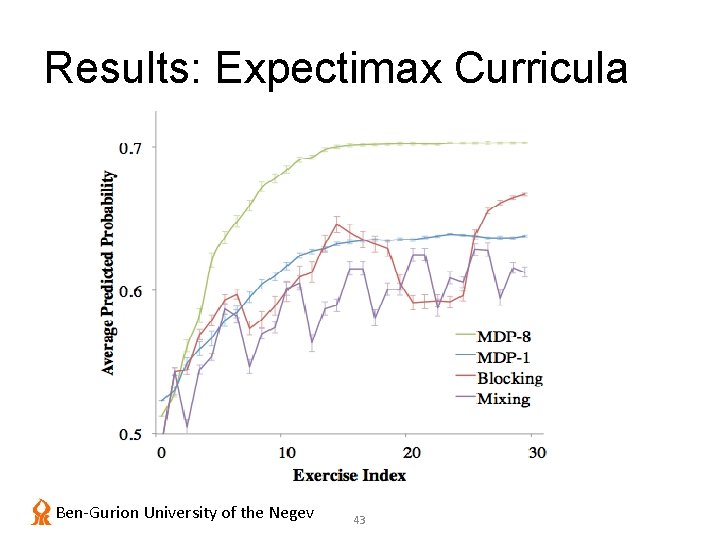
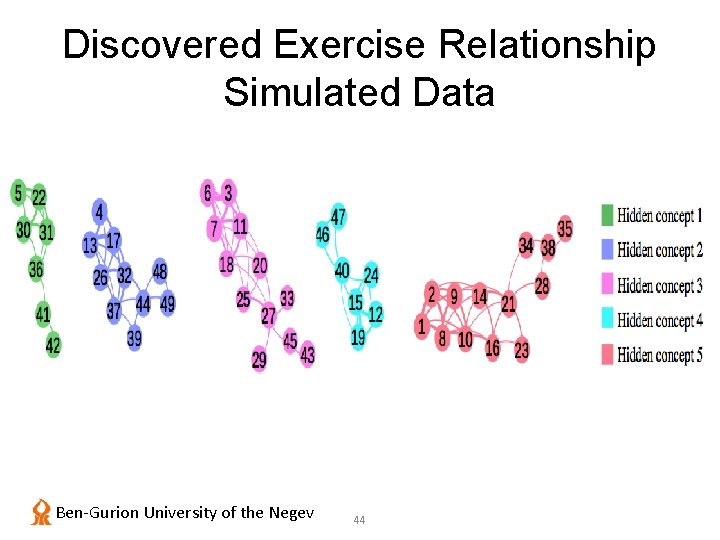
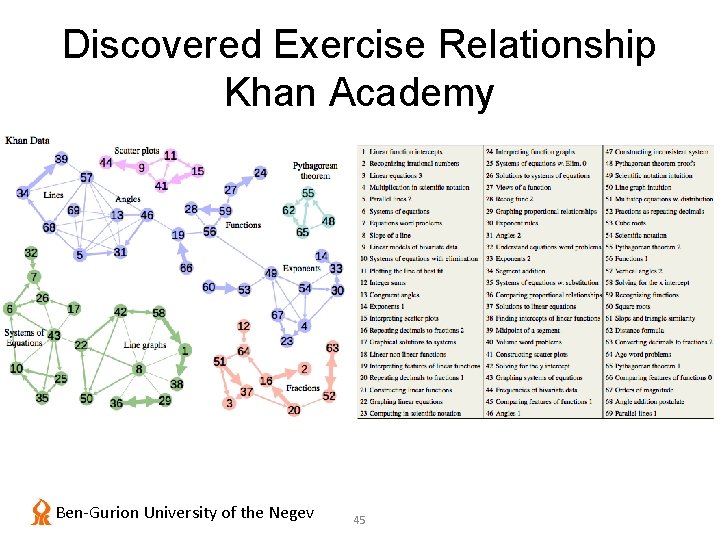
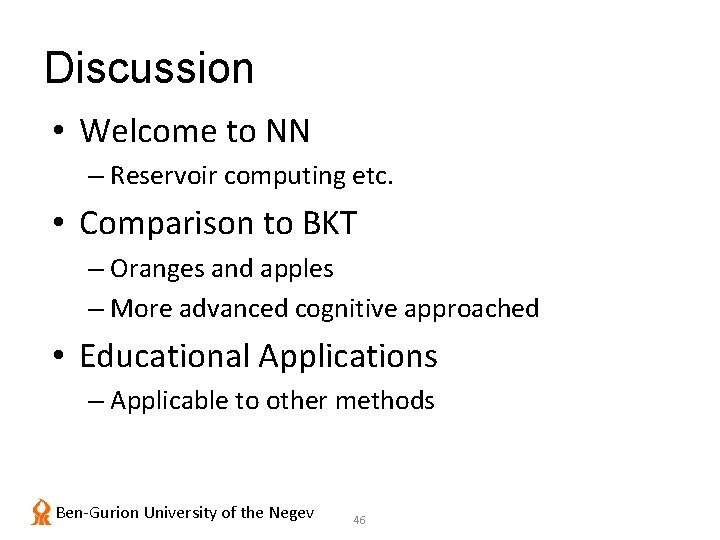
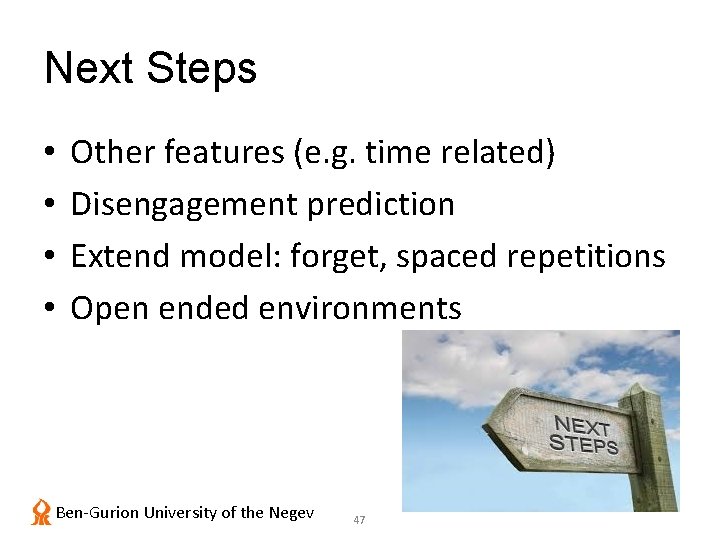
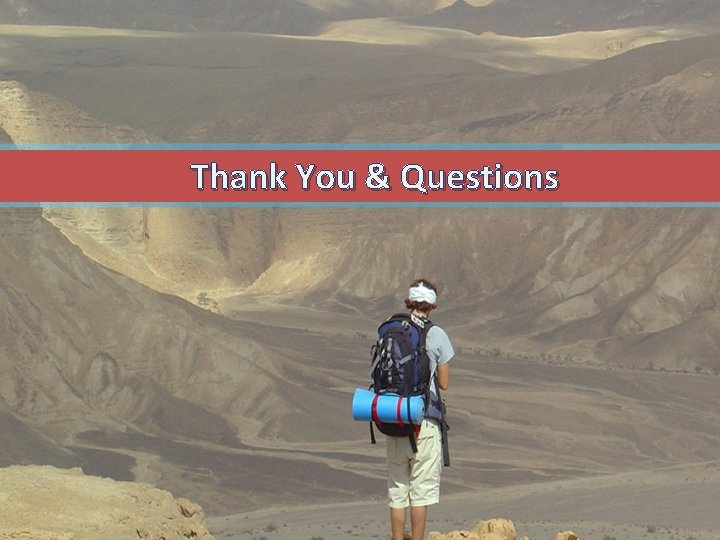
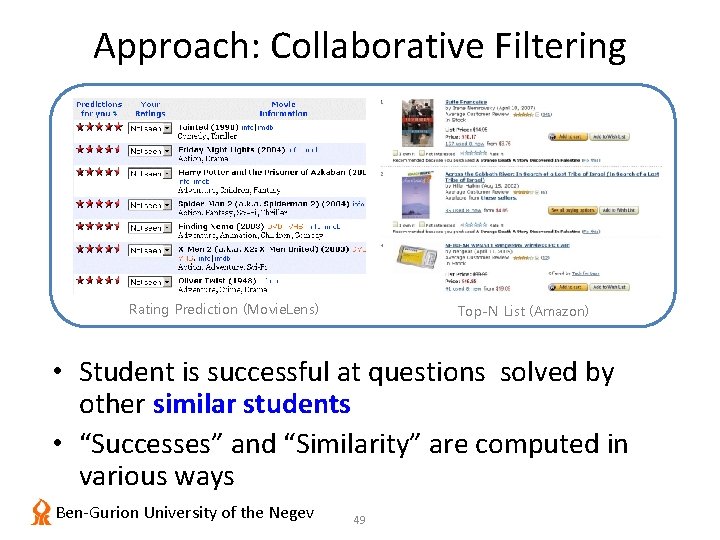
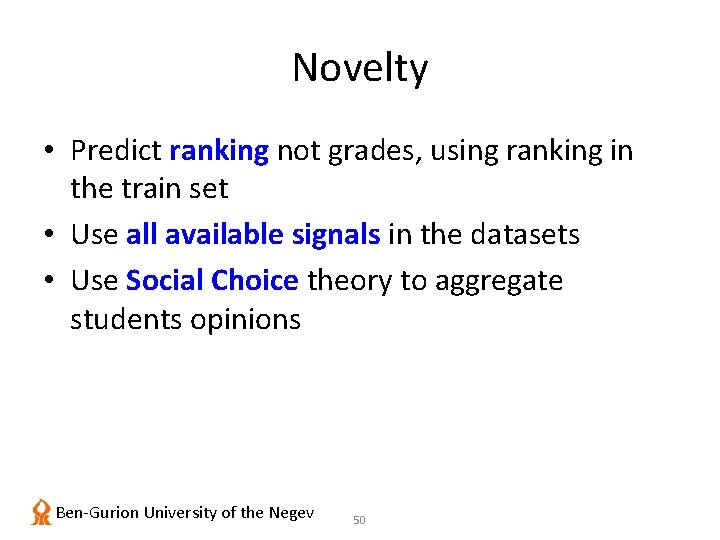
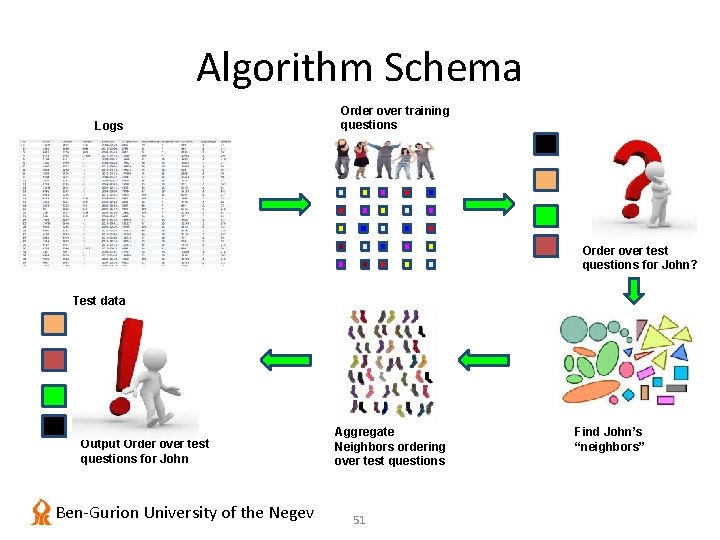
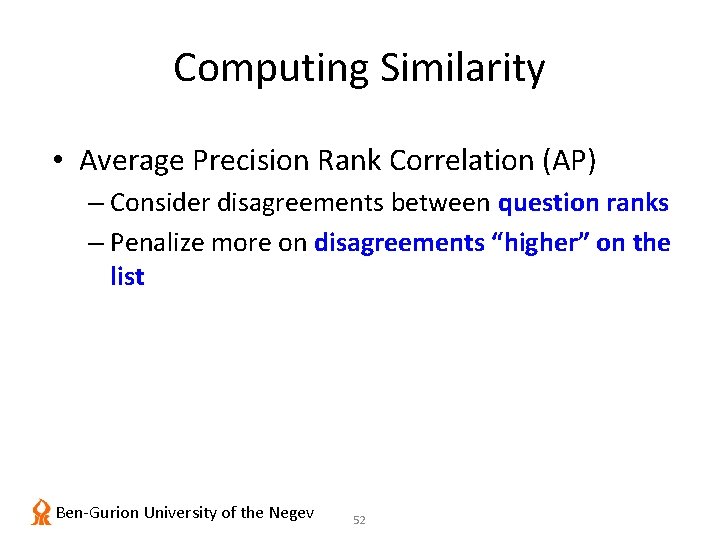
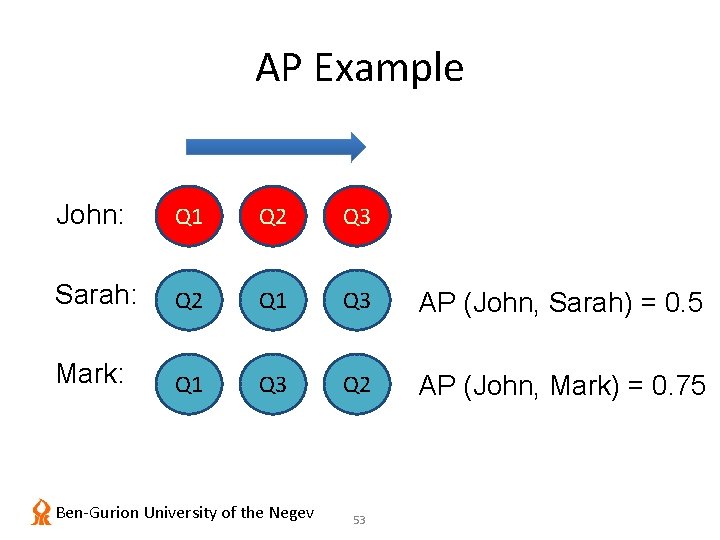
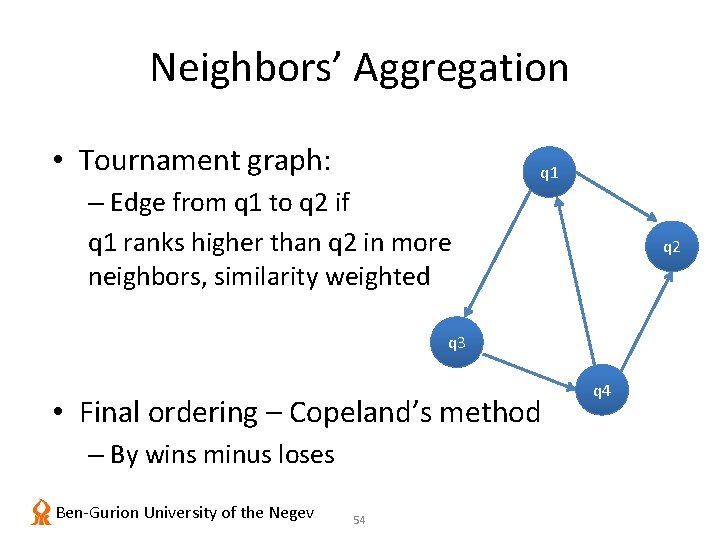
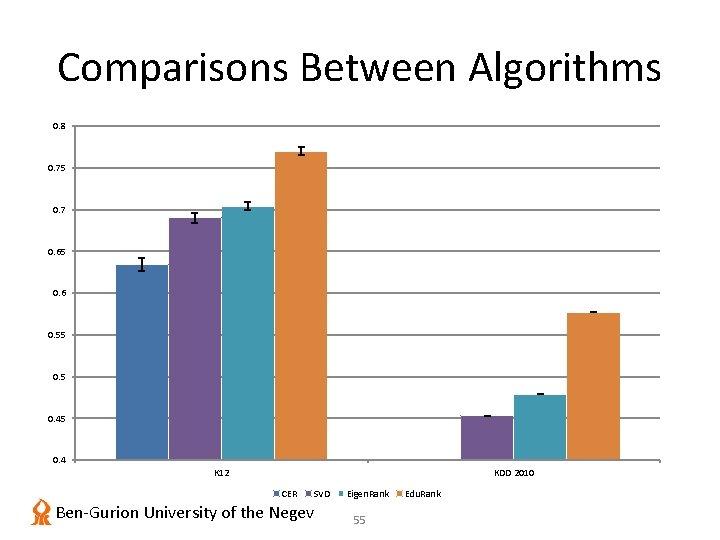
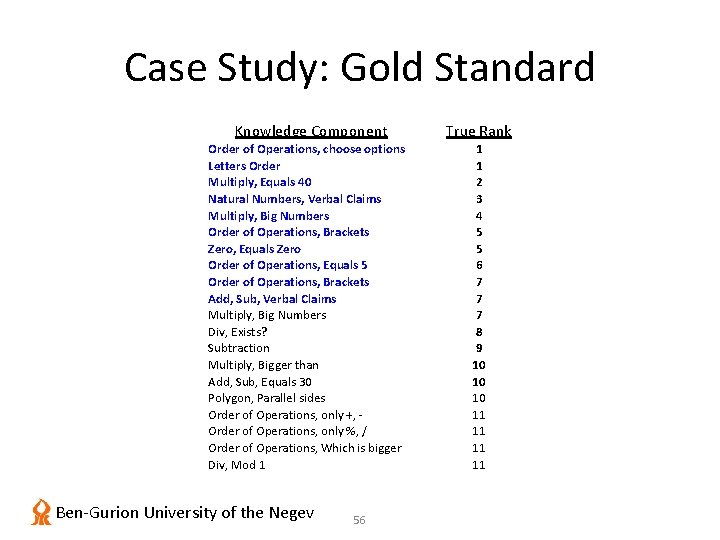
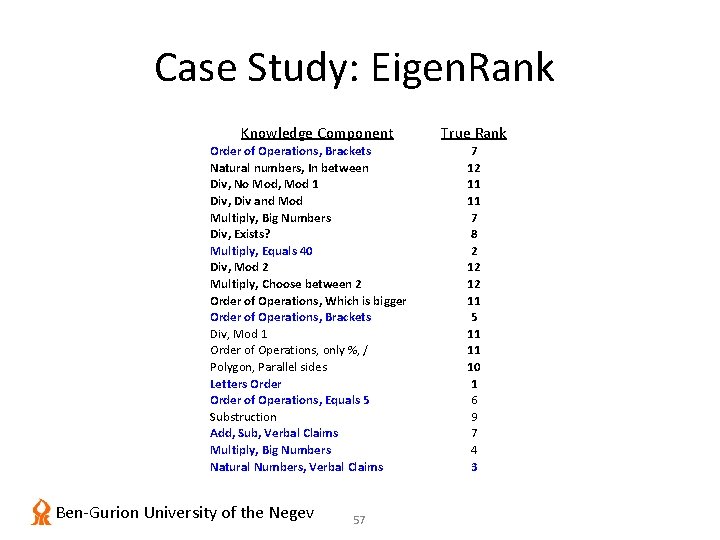
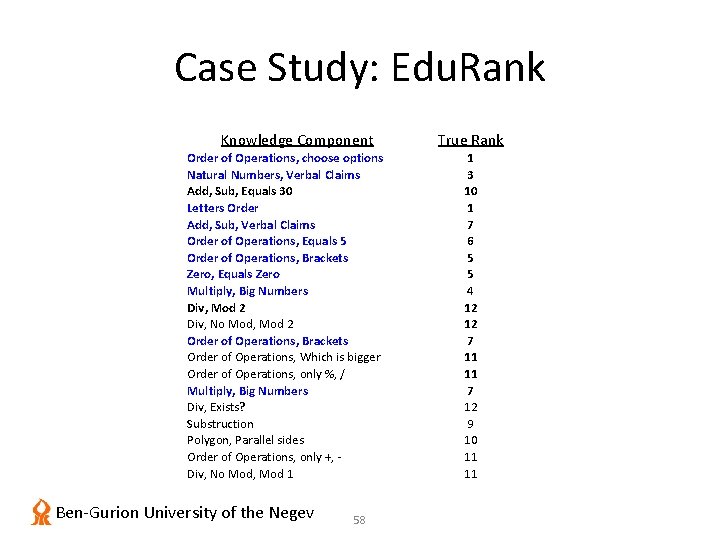
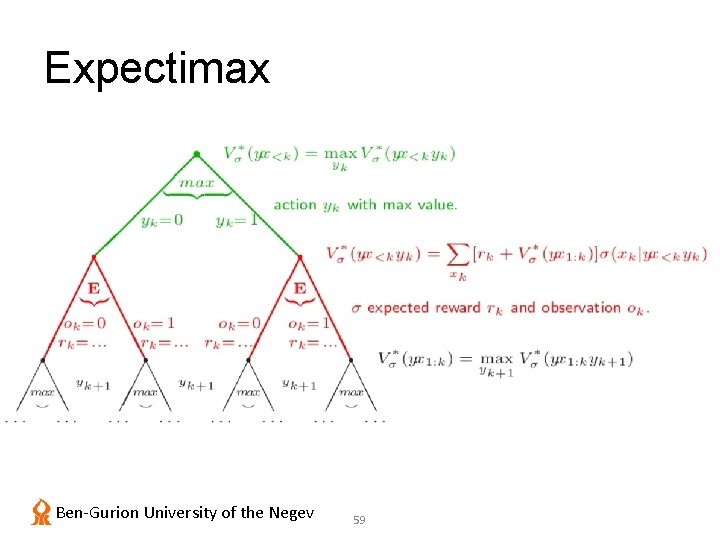
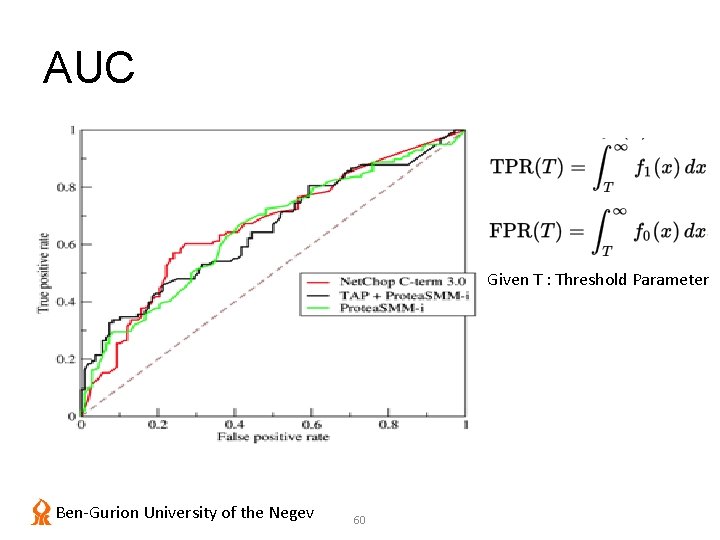
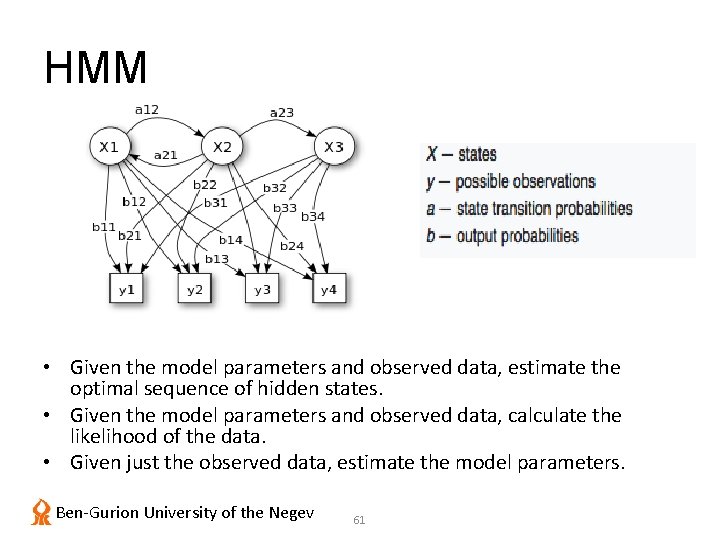
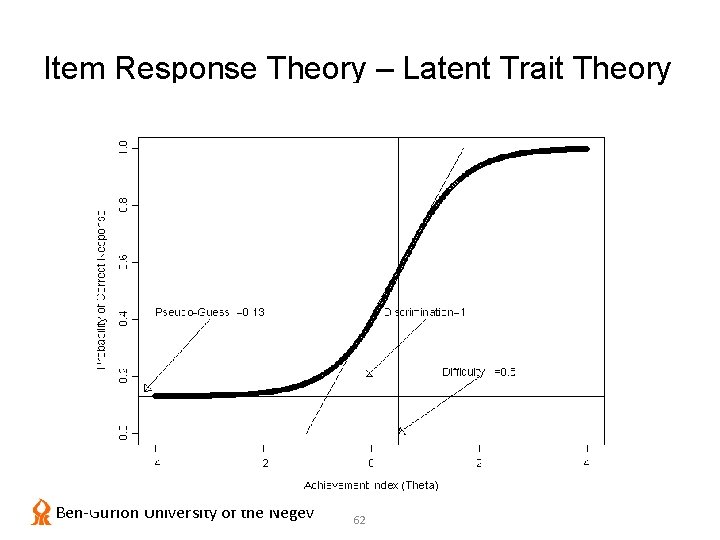
- Slides: 60
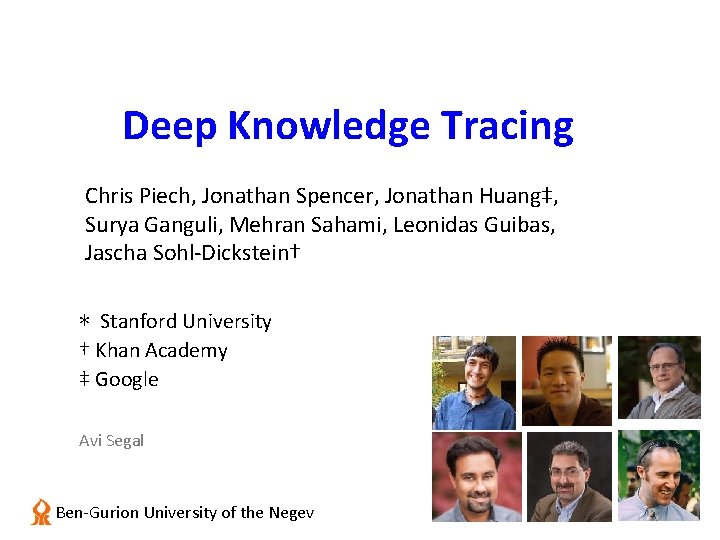
Deep Knowledge Tracing Chris Piech, Jonathan Spencer, Jonathan Huang‡, Surya Ganguli, Mehran Sahami, Leonidas Guibas, Jascha Sohl-Dickstein† ∗ Stanford University † Khan Academy ‡ Google Avi Segal Ben-Gurion University of the Negev
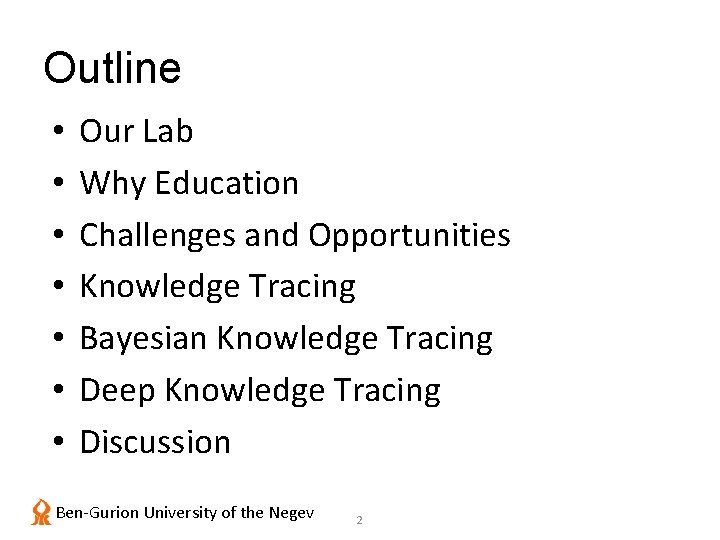
Outline • • Our Lab Why Education Challenges and Opportunities Knowledge Tracing Bayesian Knowledge Tracing Deep Knowledge Tracing Discussion Ben-Gurion University of the Negev 2
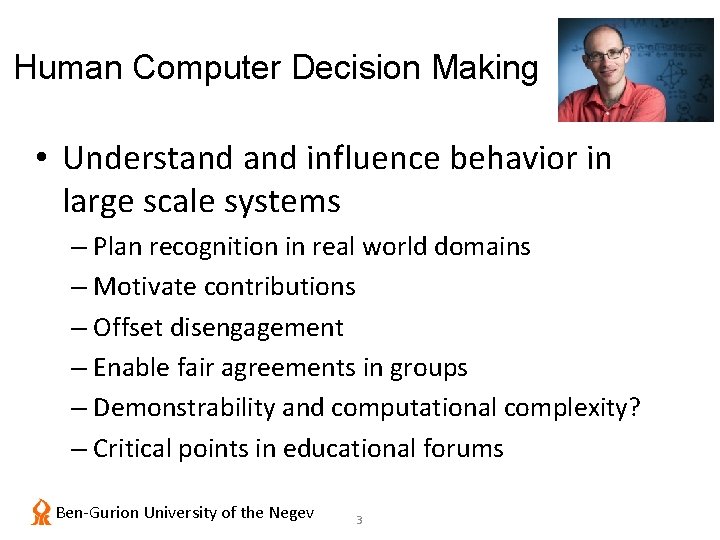
Human Computer Decision Making • Understand influence behavior in large scale systems – Plan recognition in real world domains – Motivate contributions – Offset disengagement – Enable fair agreements in groups – Demonstrability and computational complexity? – Critical points in educational forums Ben-Gurion University of the Negev 3
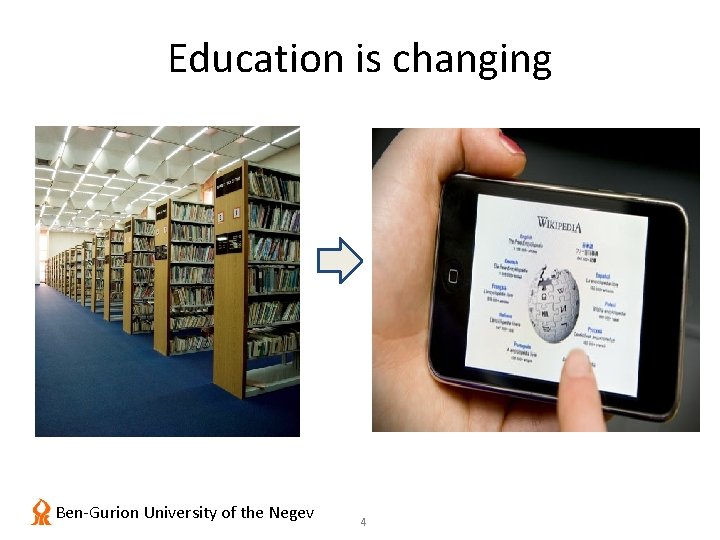
Education is changing Ben-Gurion University of the Negev 4
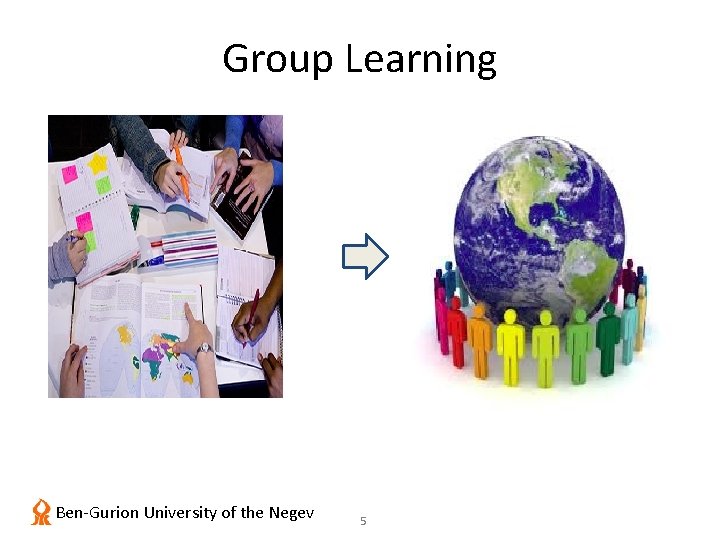
Group Learning Ben-Gurion University of the Negev 5
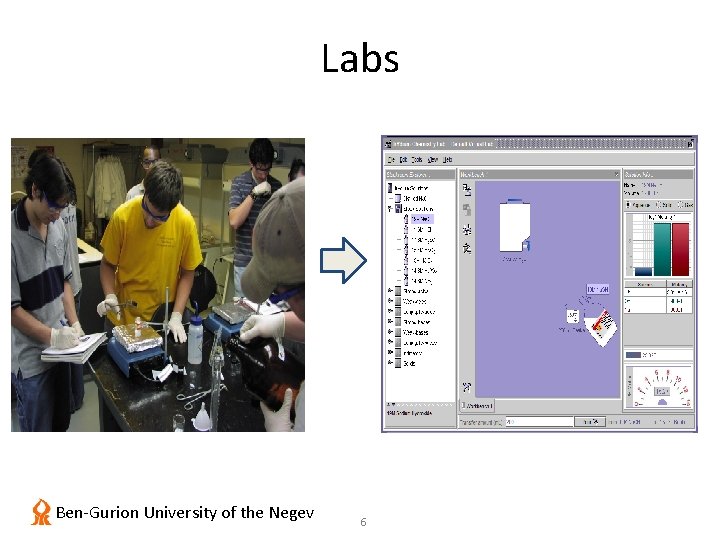
Labs Ben-Gurion University of the Negev 6
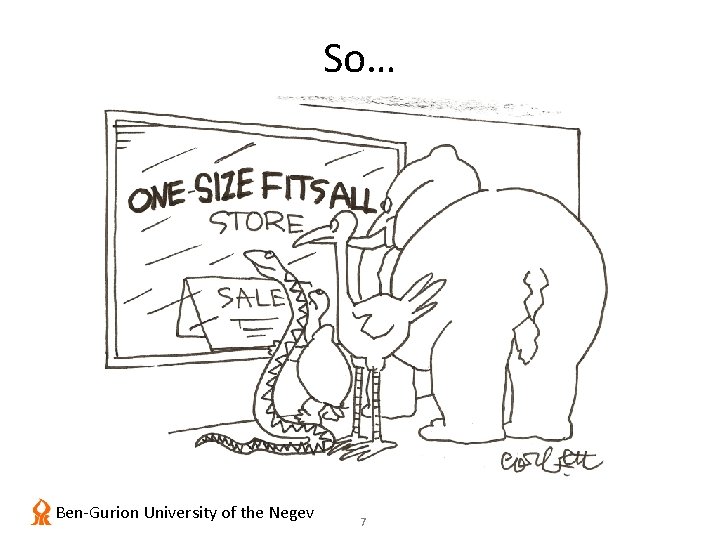
So… Ben-Gurion University of the Negev 7
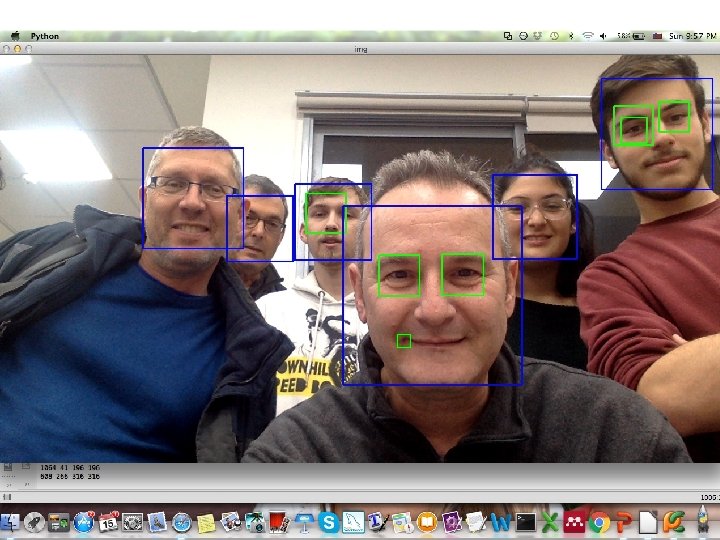
Ben-Gurion University of the Negev 8
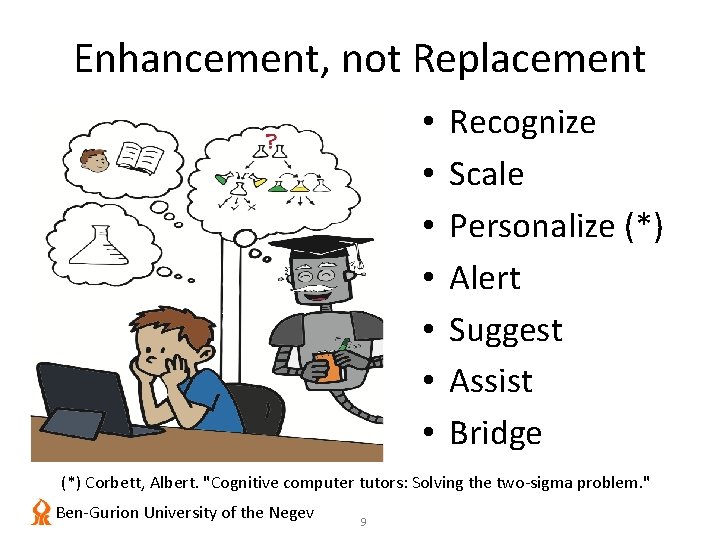
Enhancement, not Replacement • • Recognize Scale Personalize (*) Alert Suggest Assist Bridge (*) Corbett, Albert. "Cognitive computer tutors: Solving the two-sigma problem. " Ben-Gurion University of the Negev 9
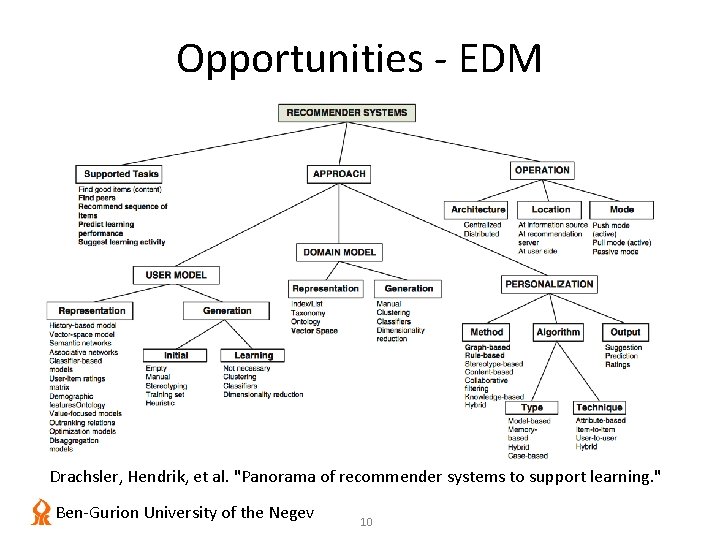
Opportunities - EDM Drachsler, Hendrik, et al. "Panorama of recommender systems to support learning. " Ben-Gurion University of the Negev 10
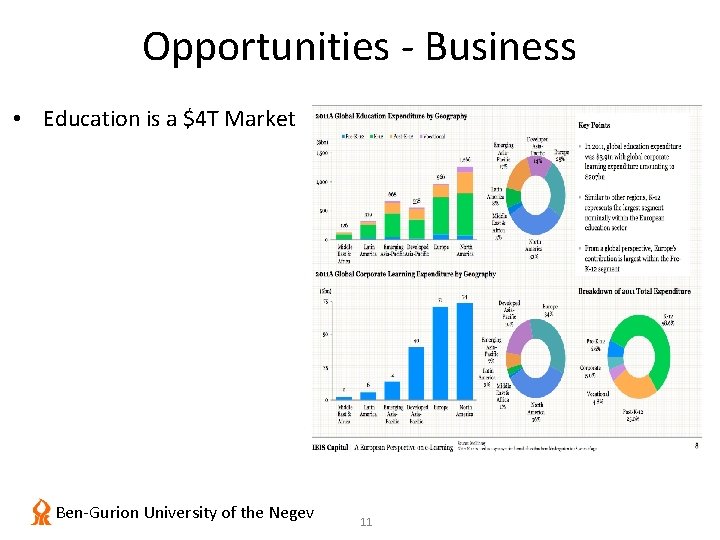
Opportunities - Business • Education is a $4 T Market Ben-Gurion University of the Negev 11
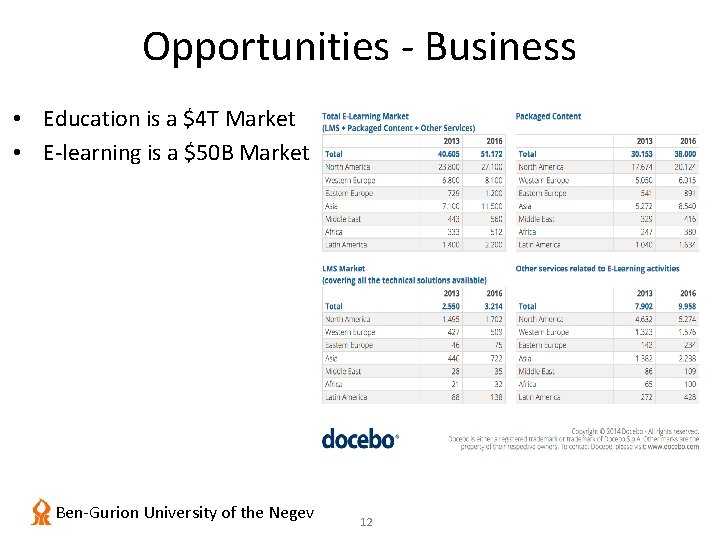
Opportunities - Business • Education is a $4 T Market • E-learning is a $50 B Market Ben-Gurion University of the Negev 12
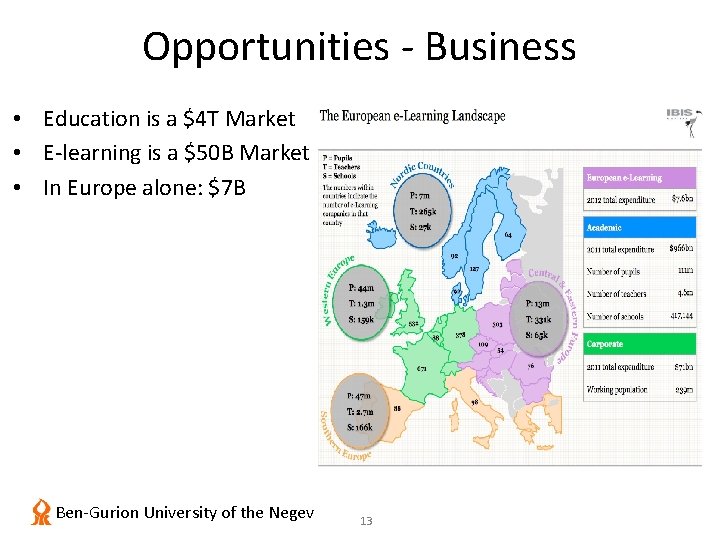
Opportunities - Business • Education is a $4 T Market • E-learning is a $50 B Market • In Europe alone: $7 B Ben-Gurion University of the Negev 13
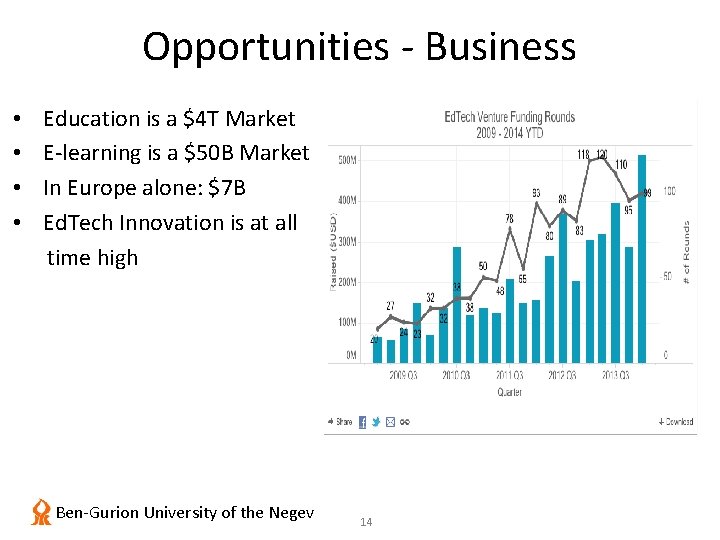
Opportunities - Business • • Education is a $4 T Market E-learning is a $50 B Market In Europe alone: $7 B Ed. Tech Innovation is at all time high Ben-Gurion University of the Negev 14
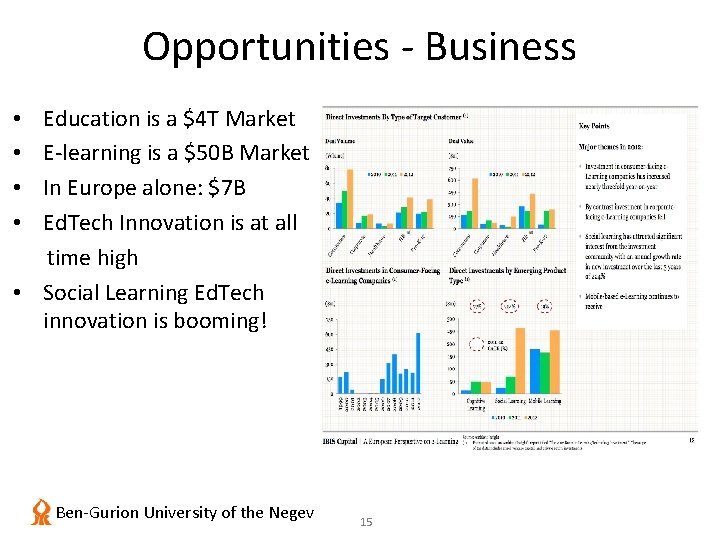
Opportunities - Business Education is a $4 T Market E-learning is a $50 B Market In Europe alone: $7 B Ed. Tech Innovation is at all time high • Social Learning Ed. Tech innovation is booming! • • Ben-Gurion University of the Negev 15
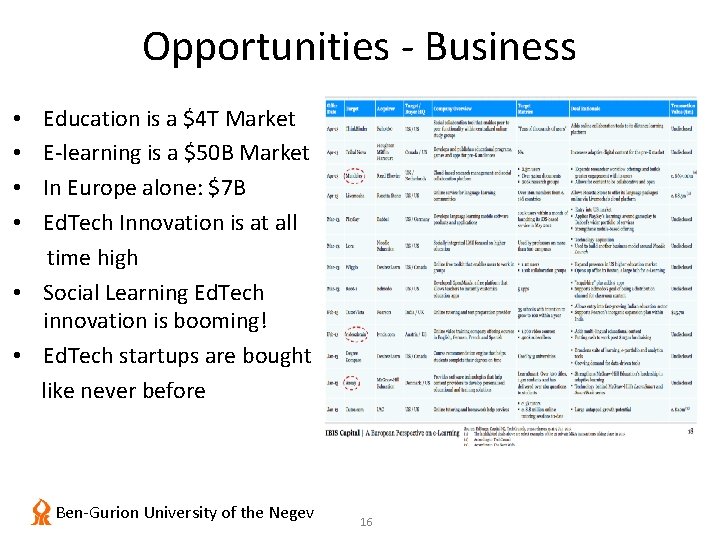
Opportunities - Business Education is a $4 T Market E-learning is a $50 B Market In Europe alone: $7 B Ed. Tech Innovation is at all time high • Social Learning Ed. Tech innovation is booming! • Ed. Tech startups are bought like never before • • Ben-Gurion University of the Negev 16
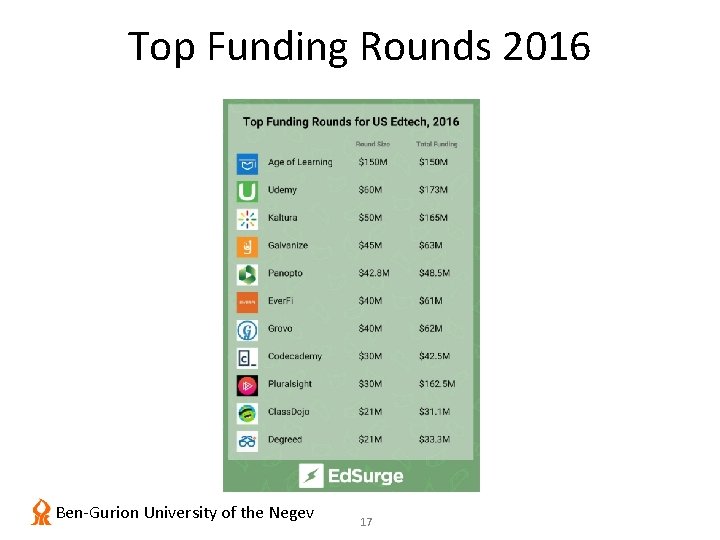
Top Funding Rounds 2016 Ben-Gurion University of the Negev 17
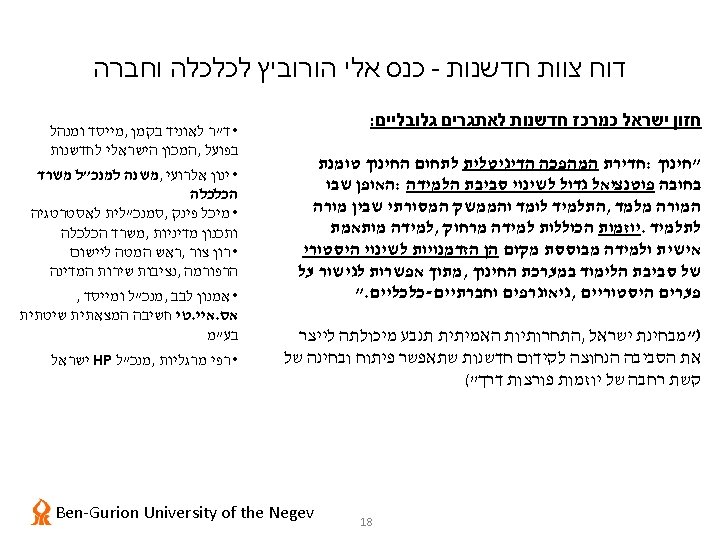
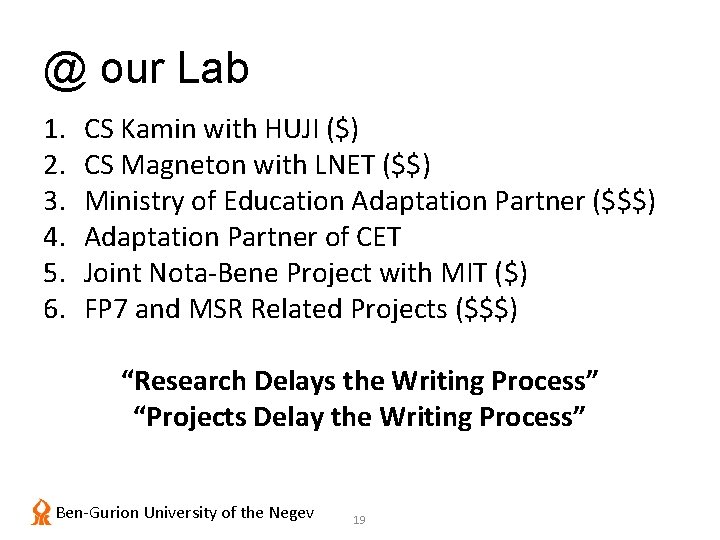
@ our Lab 1. 2. 3. 4. 5. 6. CS Kamin with HUJI ($) CS Magneton with LNET ($$) Ministry of Education Adaptation Partner ($$$) Adaptation Partner of CET Joint Nota-Bene Project with MIT ($) FP 7 and MSR Related Projects ($$$) “Research Delays the Writing Process” “Projects Delay the Writing Process” Ben-Gurion University of the Negev 19
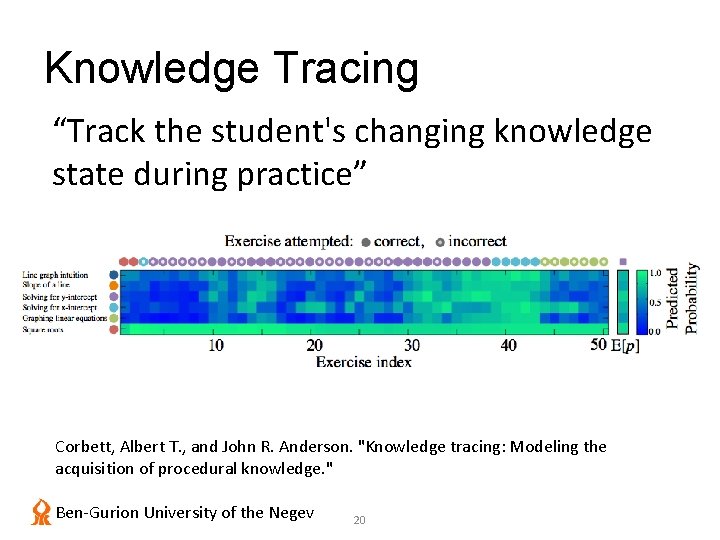
Knowledge Tracing “Track the student's changing knowledge state during practice” Corbett, Albert T. , and John R. Anderson. "Knowledge tracing: Modeling the acquisition of procedural knowledge. " Ben-Gurion University of the Negev 20
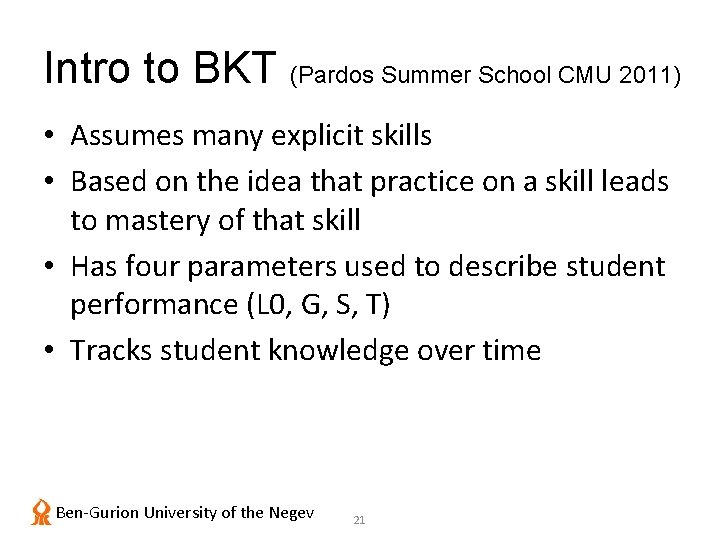
Intro to BKT (Pardos Summer School CMU 2011) • Assumes many explicit skills • Based on the idea that practice on a skill leads to mastery of that skill • Has four parameters used to describe student performance (L 0, G, S, T) • Tracks student knowledge over time Ben-Gurion University of the Negev 21
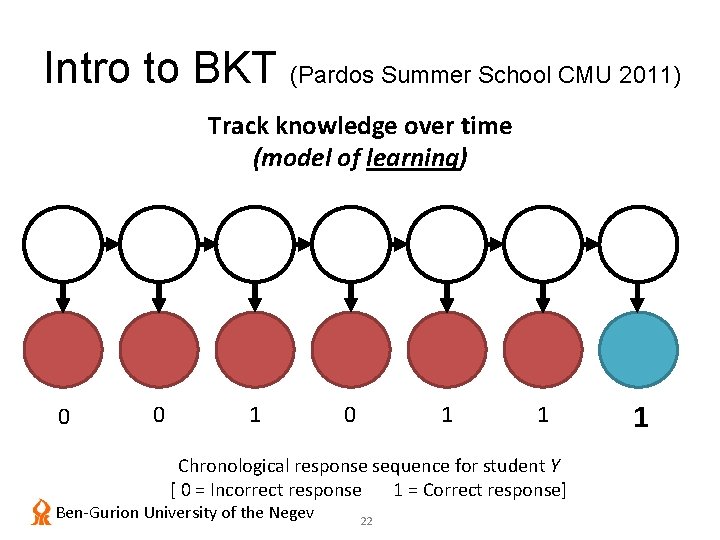
Intro to BKT (Pardos Summer School CMU 2011) Track knowledge over time (model of learning) 0 0 1 1 Chronological response sequence for student Y [ 0 = Incorrect response 1 = Correct response] Ben-Gurion University of the Negev 22 1
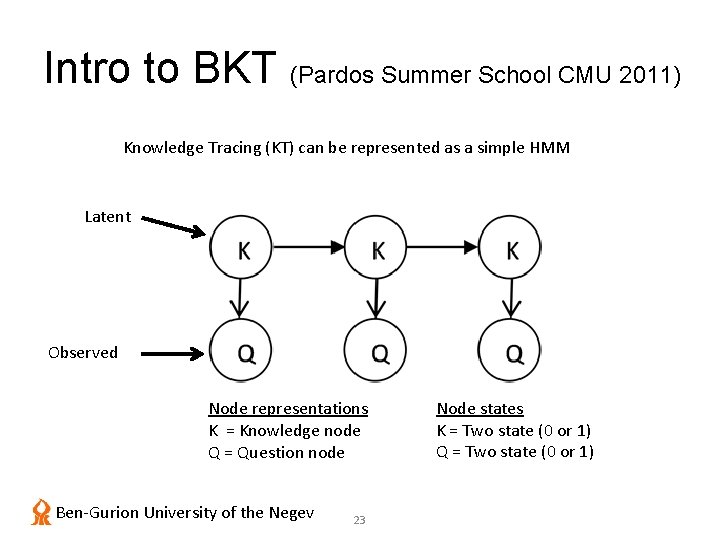
Intro to BKT (Pardos Summer School CMU 2011) Knowledge Tracing (KT) can be represented as a simple HMM Latent Observed Node representations K = Knowledge node Q = Question node Ben-Gurion University of the Negev 23 Node states K = Two state (0 or 1) Q = Two state (0 or 1)
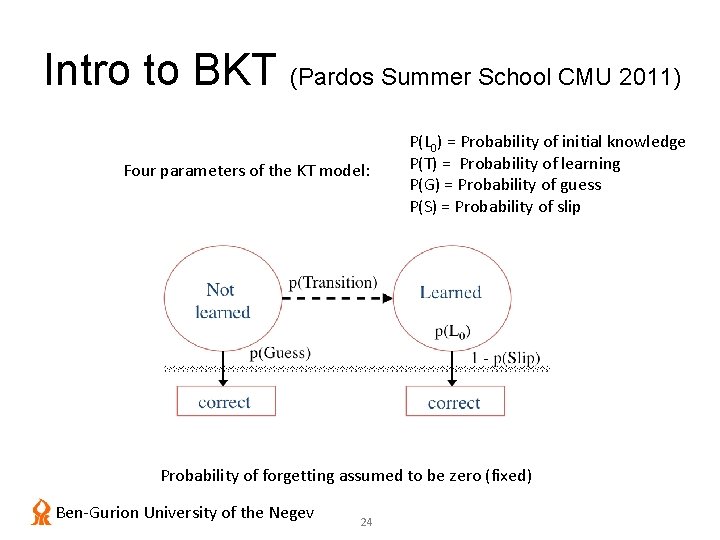
Intro to BKT (Pardos Summer School CMU 2011) Four parameters of the KT model: P(L 0) = Probability of initial knowledge P(T) = Probability of learning P(G) = Probability of guess P(S) = Probability of slip Probability of forgetting assumed to be zero (fixed) Ben-Gurion University of the Negev 24
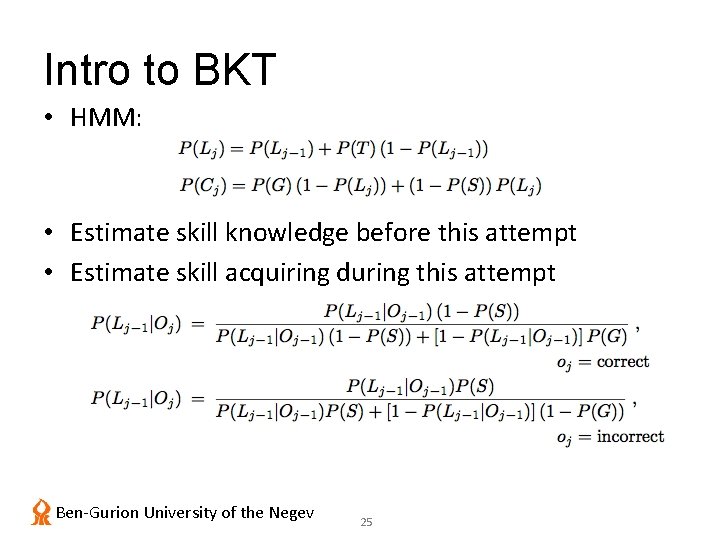
Intro to BKT • HMM: • Estimate skill knowledge before this attempt • Estimate skill acquiring during this attempt Ben-Gurion University of the Negev 25
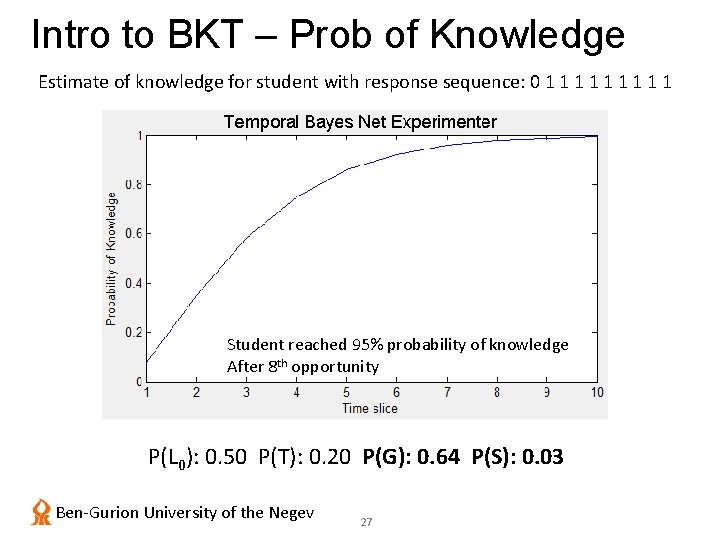
Intro to BKT – Prob of Knowledge Estimate of knowledge for student with response sequence: 0 1 1 1 1 1 Student reached 95% probability of knowledge After 8 th opportunity P(L 0): 0. 50 P(T): 0. 20 P(G): 0. 64 P(S): 0. 03 Ben-Gurion University of the Negev 27
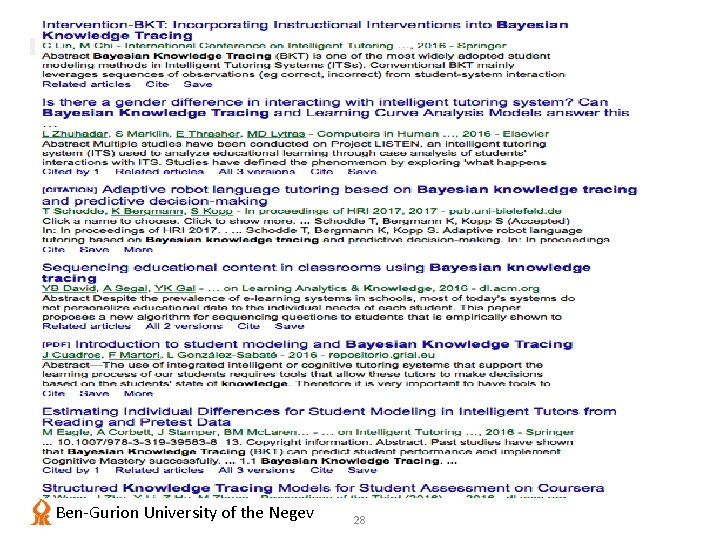
Ben-Gurion University of the Negev 28
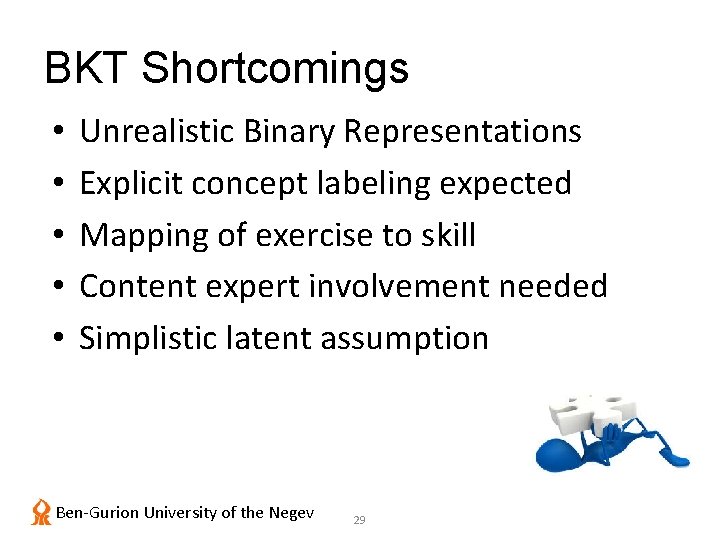
BKT Shortcomings • • • Unrealistic Binary Representations Explicit concept labeling expected Mapping of exercise to skill Content expert involvement needed Simplistic latent assumption Ben-Gurion University of the Negev 29
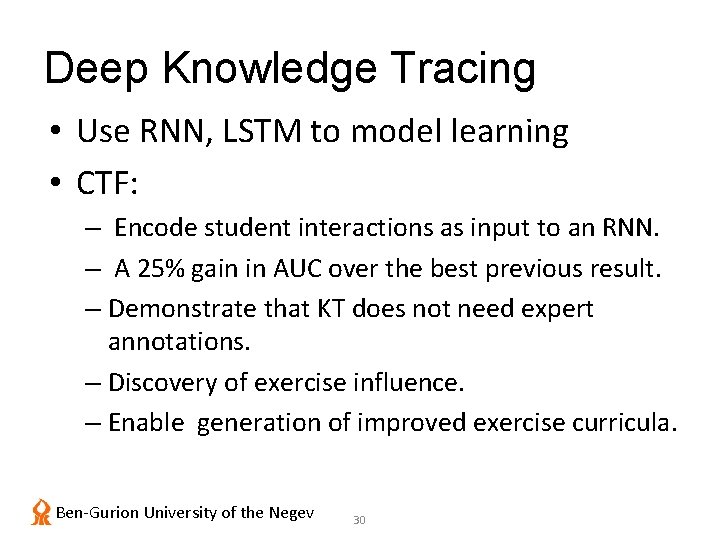
Deep Knowledge Tracing • Use RNN, LSTM to model learning • CTF: – Encode student interactions as input to an RNN. – A 25% gain in AUC over the best previous result. – Demonstrate that KT does not need expert annotations. – Discovery of exercise influence. – Enable generation of improved exercise curricula. Ben-Gurion University of the Negev 30
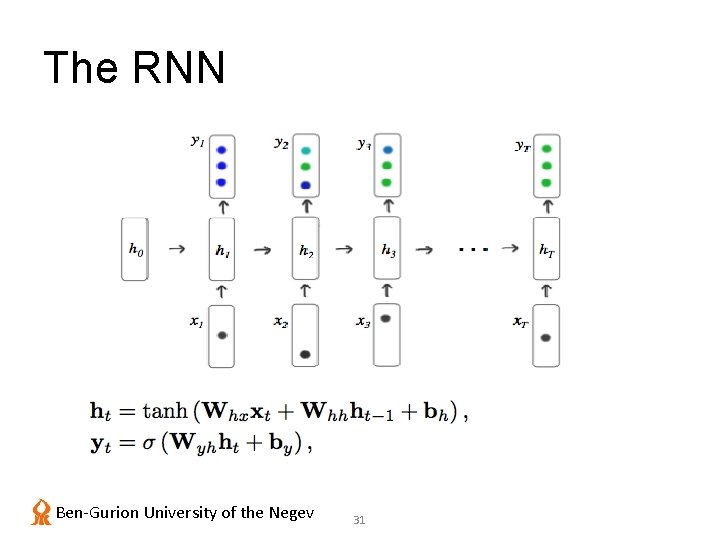
The RNN Ben-Gurion University of the Negev 31
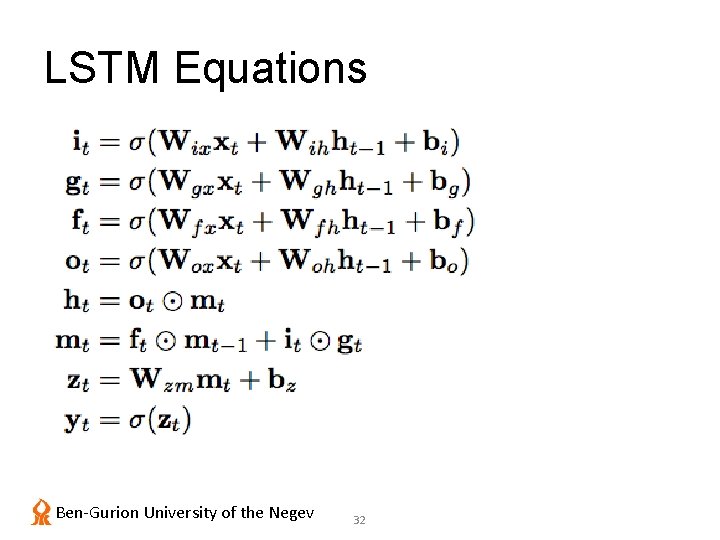
LSTM Equations Ben-Gurion University of the Negev 32
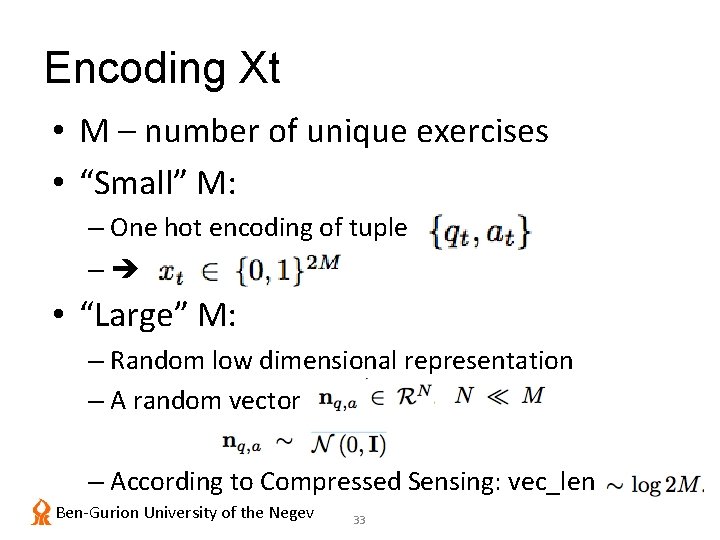
Encoding Xt • M – number of unique exercises • “Small” M: – One hot encoding of tuple – • “Large” M: – Random low dimensional representation – A random vector – According to Compressed Sensing: vec_len Ben-Gurion University of the Negev 33
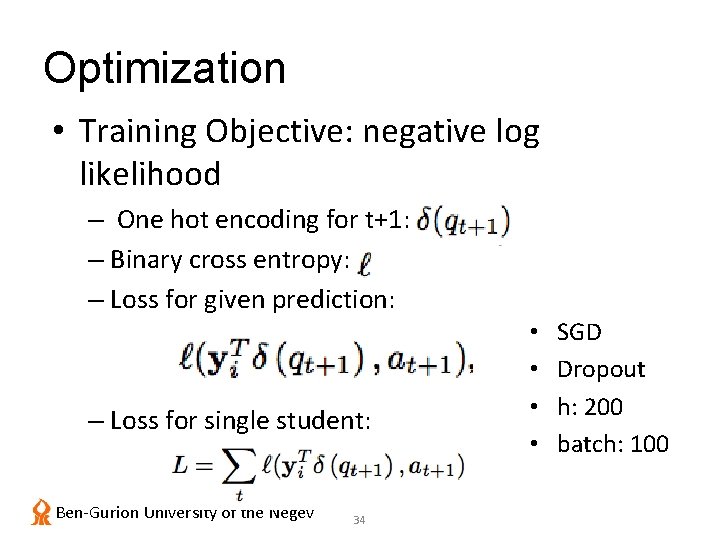
Optimization • Training Objective: negative log likelihood – One hot encoding for t+1: – Binary cross entropy: – Loss for given prediction: – Loss for single student: Ben-Gurion University of the Negev 34 • • SGD Dropout h: 200 batch: 100
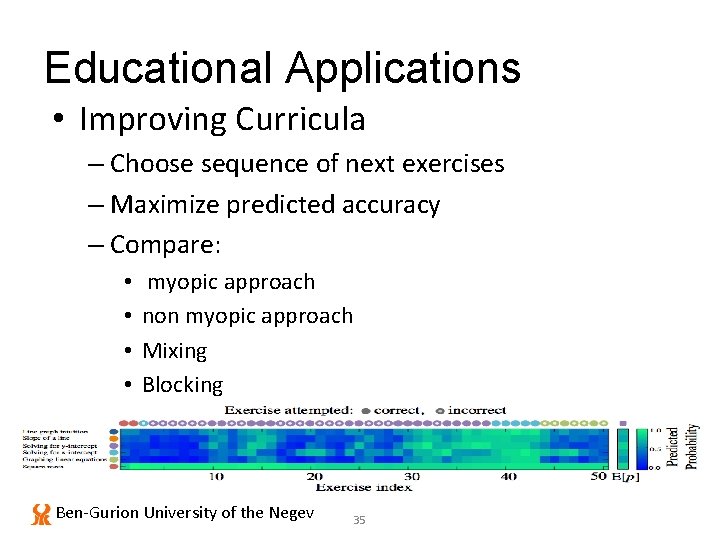
Educational Applications • Improving Curricula – Choose sequence of next exercises – Maximize predicted accuracy – Compare: • • myopic approach non myopic approach Mixing Blocking Ben-Gurion University of the Negev 35
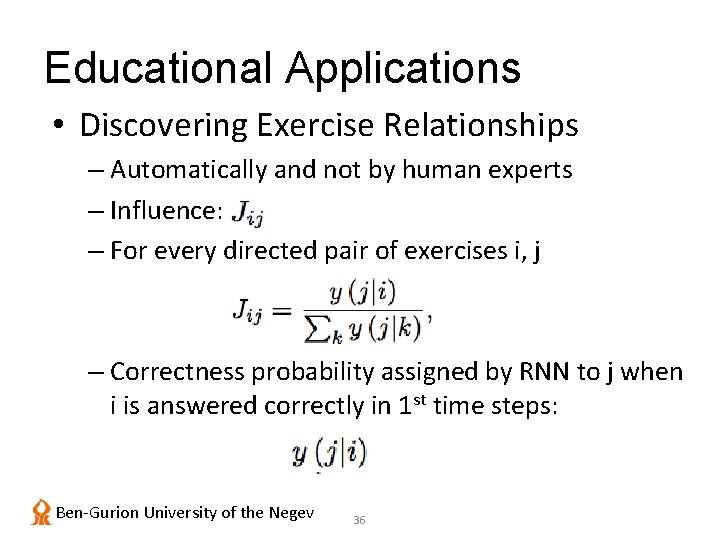
Educational Applications • Discovering Exercise Relationships – Automatically and not by human experts – Influence: – For every directed pair of exercises i, j – Correctness probability assigned by RNN to j when i is answered correctly in 1 st time steps: Ben-Gurion University of the Negev 36
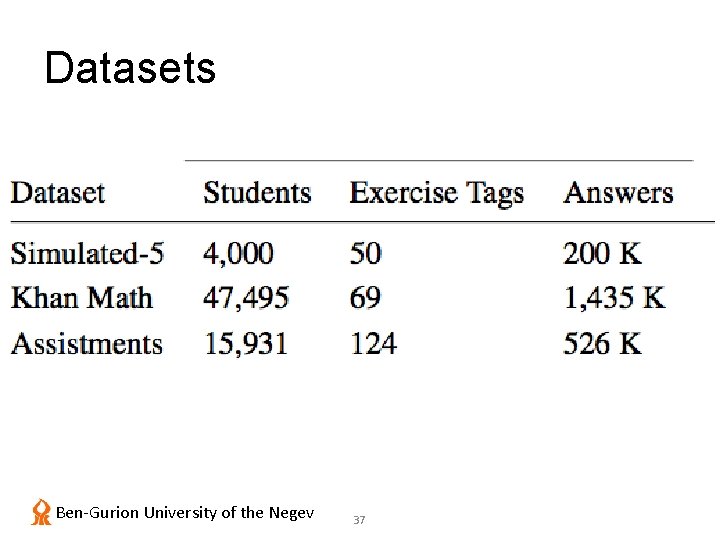
Datasets Ben-Gurion University of the Negev 37
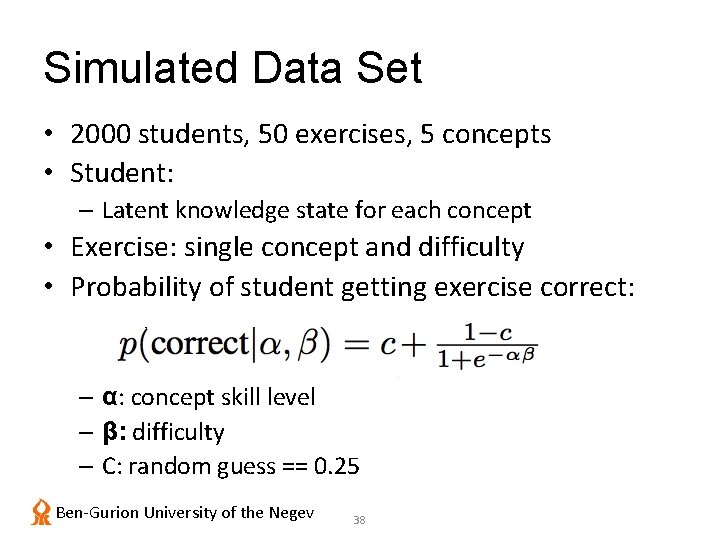
Simulated Data Set • 2000 students, 50 exercises, 5 concepts • Student: – Latent knowledge state for each concept • Exercise: single concept and difficulty • Probability of student getting exercise correct: – α: concept skill level – β: difficulty – C: random guess == 0. 25 Ben-Gurion University of the Negev 38
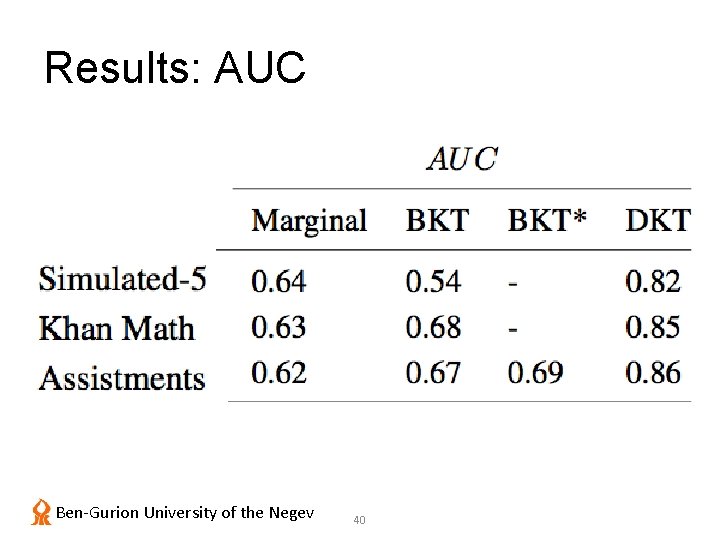
Results: AUC Ben-Gurion University of the Negev 40
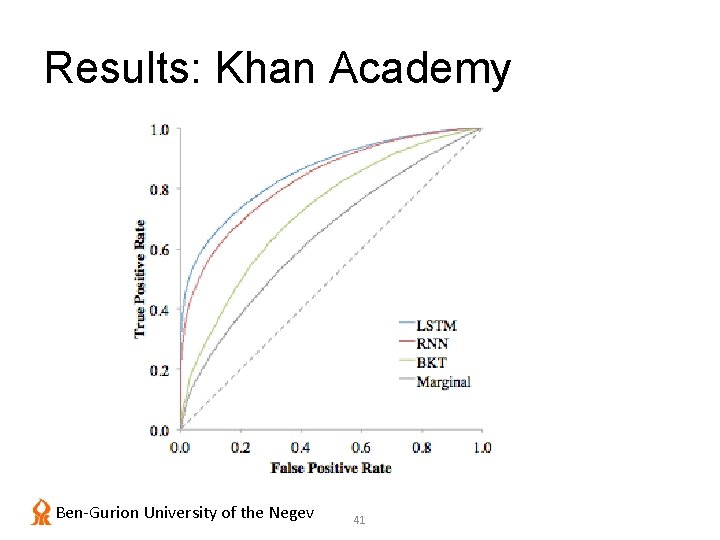
Results: Khan Academy Ben-Gurion University of the Negev 41
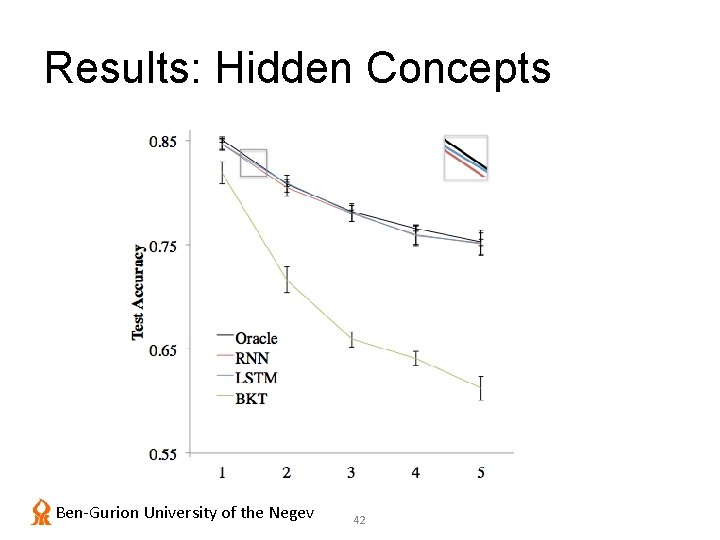
Results: Hidden Concepts Ben-Gurion University of the Negev 42
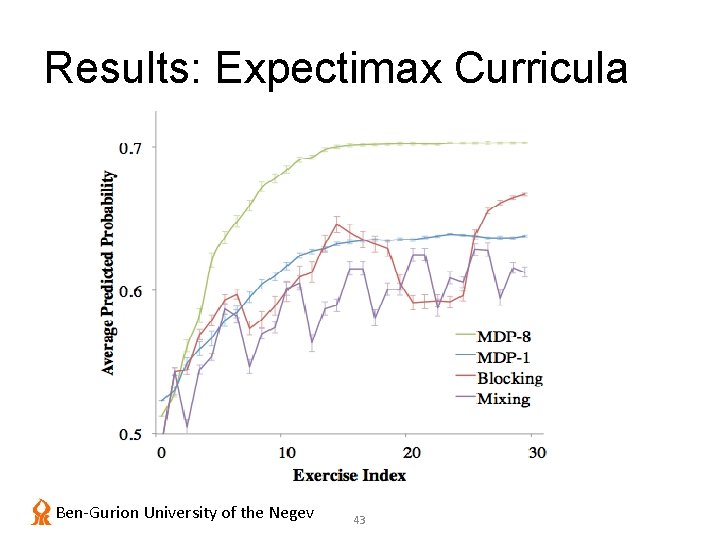
Results: Expectimax Curricula Ben-Gurion University of the Negev 43
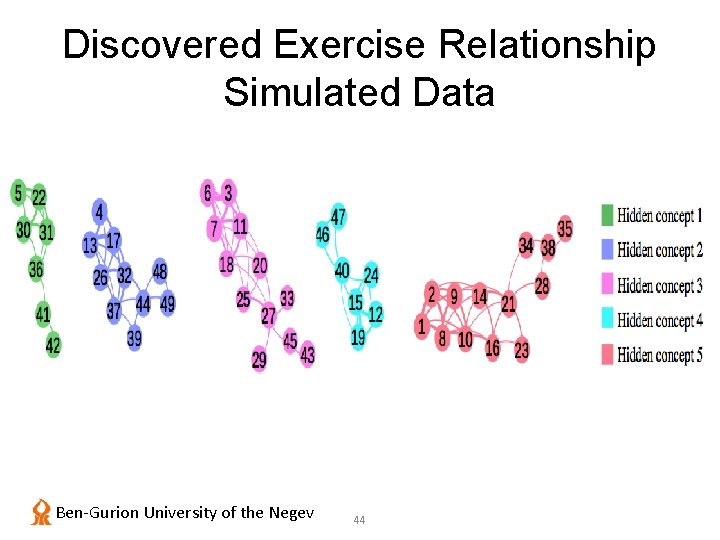
Discovered Exercise Relationship Simulated Data Ben-Gurion University of the Negev 44
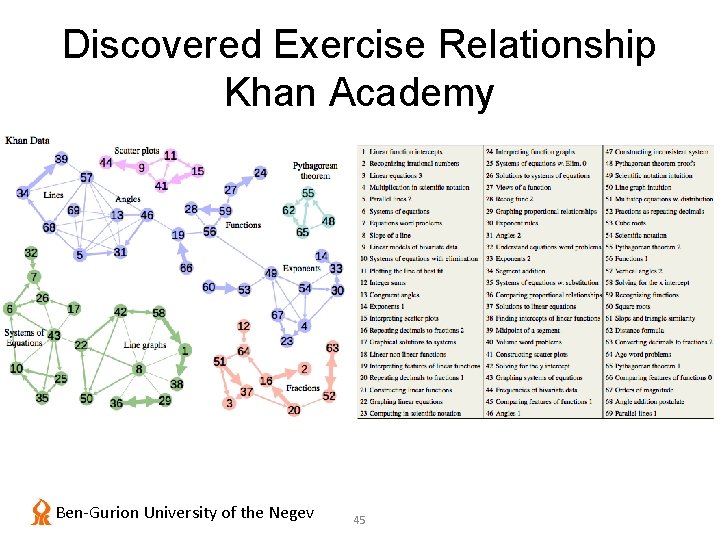
Discovered Exercise Relationship Khan Academy Ben-Gurion University of the Negev 45
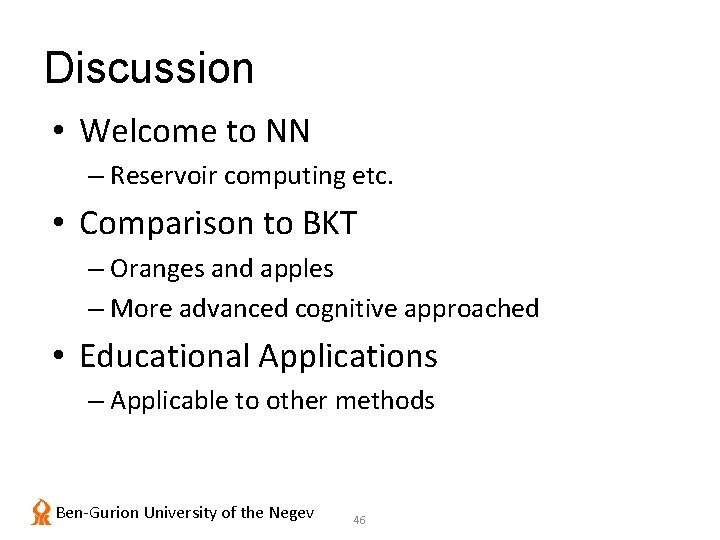
Discussion • Welcome to NN – Reservoir computing etc. • Comparison to BKT – Oranges and apples – More advanced cognitive approached • Educational Applications – Applicable to other methods Ben-Gurion University of the Negev 46
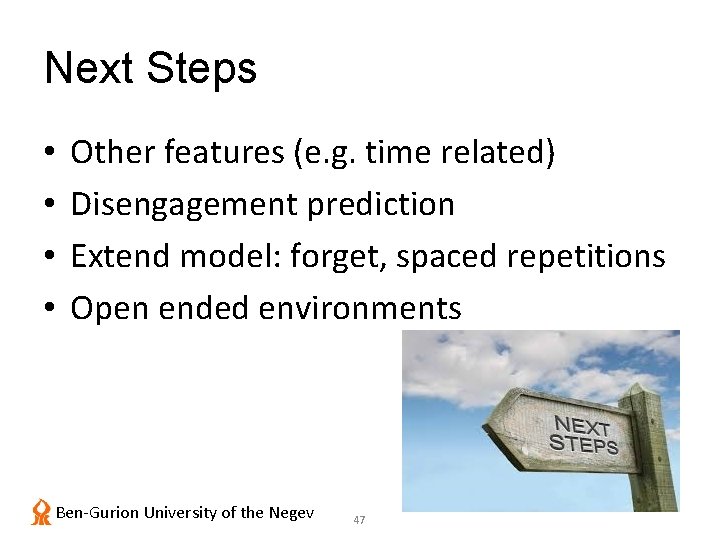
Next Steps • • Other features (e. g. time related) Disengagement prediction Extend model: forget, spaced repetitions Open ended environments Ben-Gurion University of the Negev 47
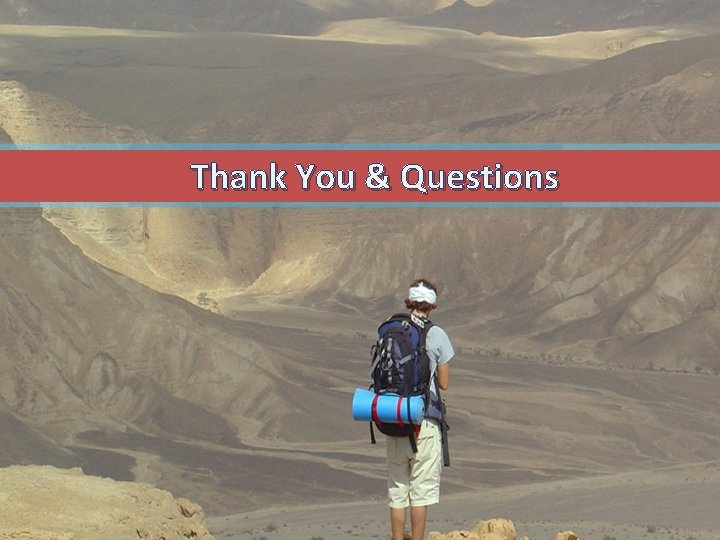
Thank You & Questions Ben-Gurion University of the Negev
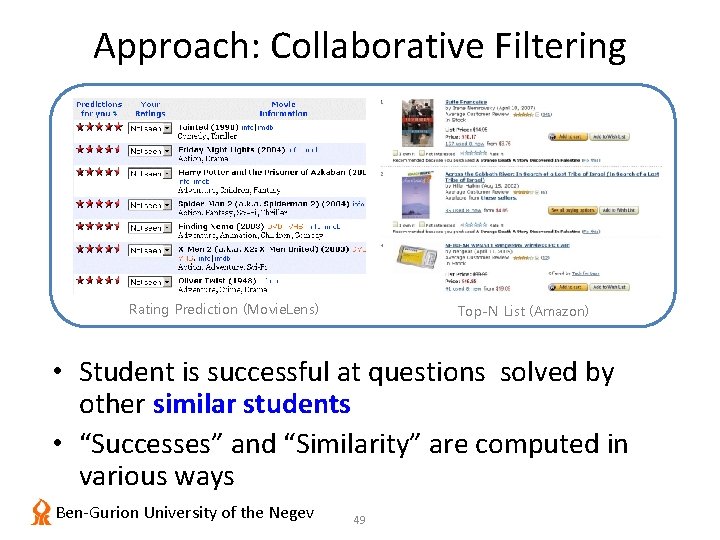
Approach: Collaborative Filtering Rating Prediction (Movie. Lens) Top-N List (Amazon) • Student is successful at questions solved by other similar students • “Successes” and “Similarity” are computed in various ways Ben-Gurion University of the Negev 49
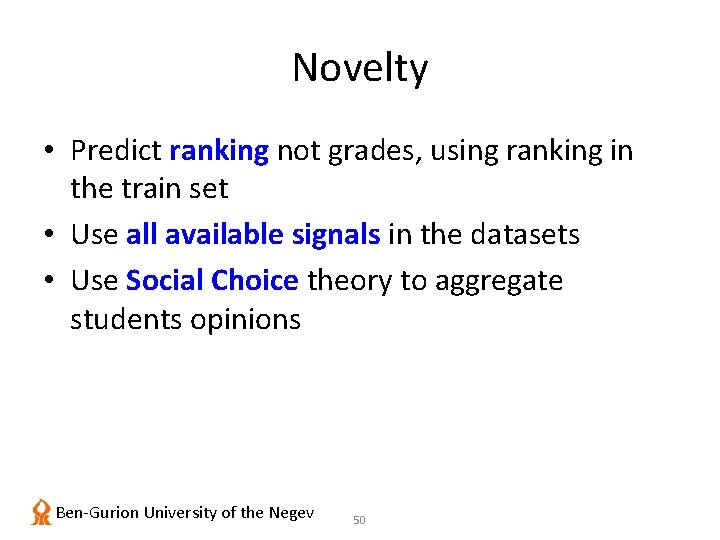
Novelty • Predict ranking not grades, using ranking in the train set • Use all available signals in the datasets • Use Social Choice theory to aggregate students opinions Ben-Gurion University of the Negev 50
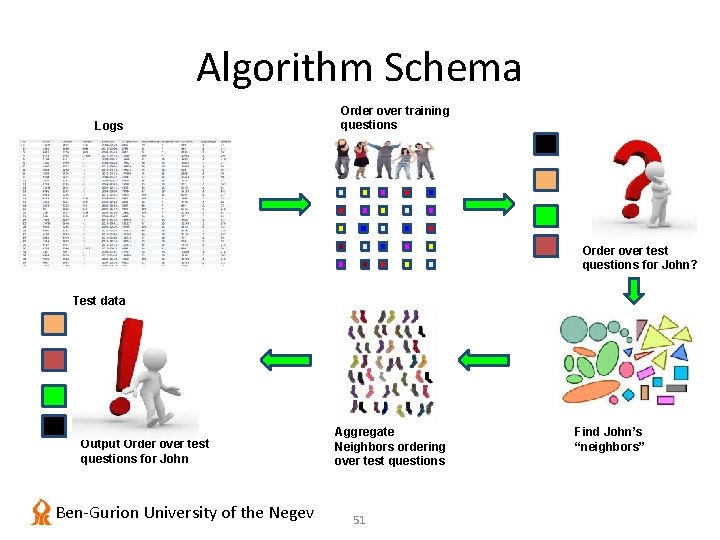
Algorithm Schema Logs Order over training questions Order over test questions for John? Test data Output Order over test questions for John Ben-Gurion University of the Negev Aggregate Neighbors ordering over test questions 51 Find John’s “neighbors”
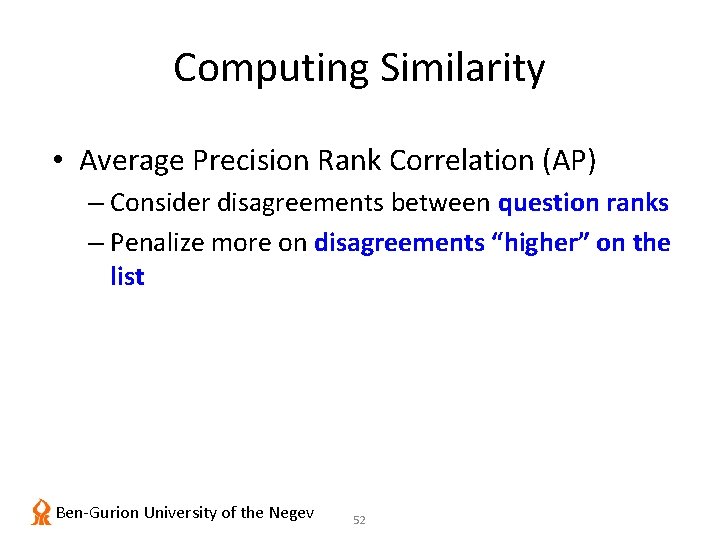
Computing Similarity • Average Precision Rank Correlation (AP) – Consider disagreements between question ranks – Penalize more on disagreements “higher” on the list Ben-Gurion University of the Negev 52
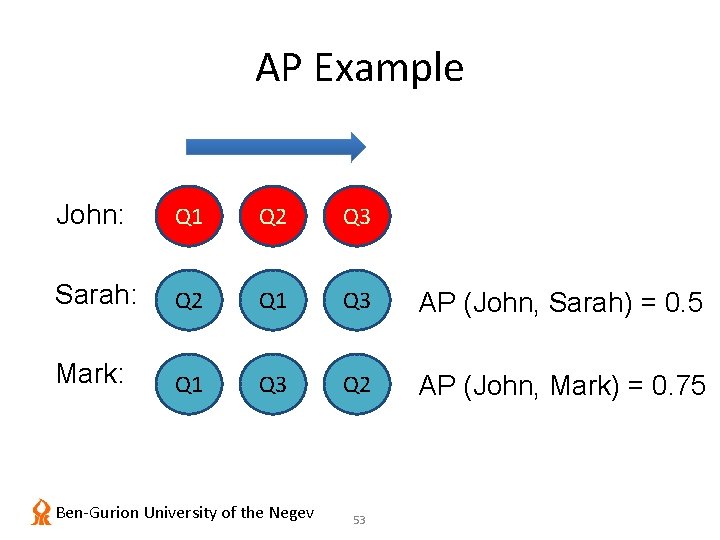
AP Example John: Q 1 Q 2 Q 3 Sarah: Q 2 Q 1 Q 3 AP (John, Sarah) = 0. 5 Mark: Q 1 Q 3 Q 2 AP (John, Mark) = 0. 75 Ben-Gurion University of the Negev 53
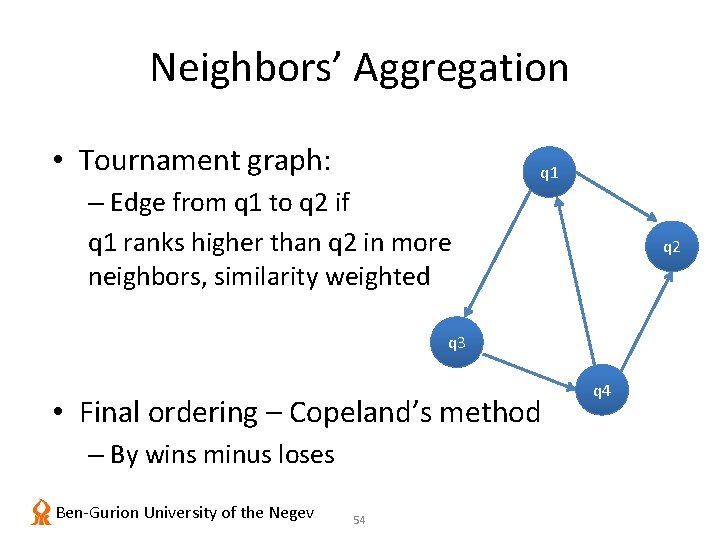
Neighbors’ Aggregation • Tournament graph: q 1 – Edge from q 1 to q 2 if q 1 ranks higher than q 2 in more neighbors, similarity weighted q 2 q 3 • Final ordering – Copeland’s method – By wins minus loses Ben-Gurion University of the Negev 54 q 4
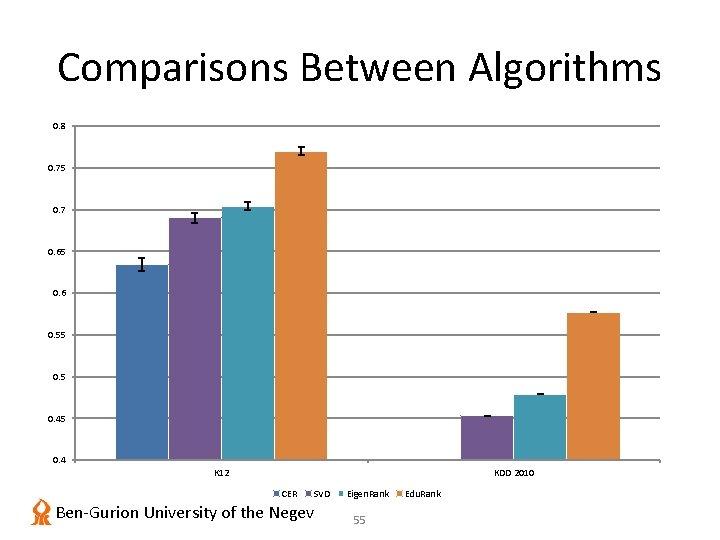
Comparisons Between Algorithms 0. 8 0. 75 0. 7 0. 65 0. 6 0. 55 0. 45 0. 4 K 12 KDD 2010 CER SVD Ben-Gurion University of the Negev Eigen. Rank 55 Edu. Rank
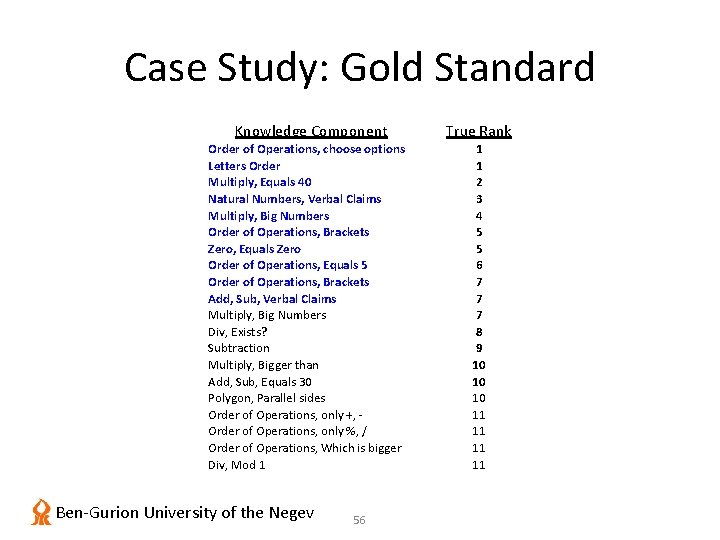
Case Study: Gold Standard Knowledge Component Order of Operations, choose options Letters Order Multiply, Equals 40 Natural Numbers, Verbal Claims Multiply, Big Numbers Order of Operations, Brackets Zero, Equals Zero Order of Operations, Equals 5 Order of Operations, Brackets Add, Sub, Verbal Claims Multiply, Big Numbers Div, Exists? Subtraction Multiply, Bigger than Add, Sub, Equals 30 Polygon, Parallel sides Order of Operations, only +, - Order of Operations, only %, / Order of Operations, Which is bigger Div, Mod 1 Ben-Gurion University of the Negev 56 True Rank 1 1 2 3 4 5 5 6 7 7 7 8 9 10 10 10 11 11
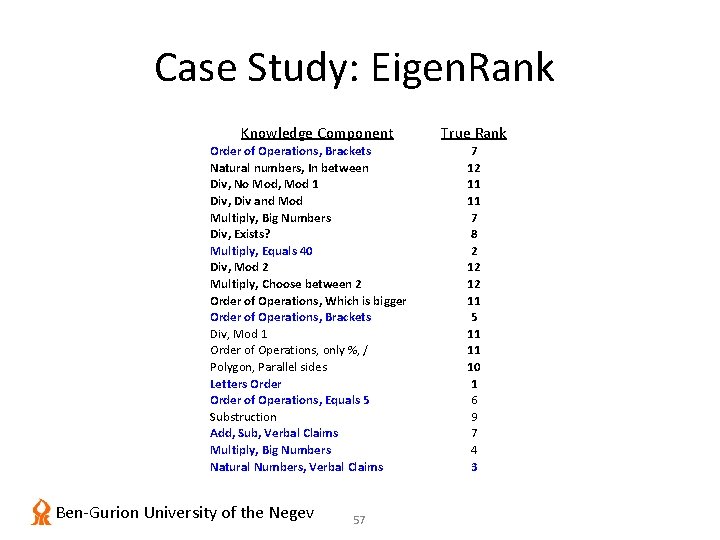
Case Study: Eigen. Rank Knowledge Component Order of Operations, Brackets Natural numbers, In between Div, No Mod, Mod 1 Div, Div and Mod Multiply, Big Numbers Div, Exists? Multiply, Equals 40 Div, Mod 2 Multiply, Choose between 2 Order of Operations, Which is bigger Order of Operations, Brackets Div, Mod 1 Order of Operations, only %, / Polygon, Parallel sides Letters Order of Operations, Equals 5 Substruction Add, Sub, Verbal Claims Multiply, Big Numbers Natural Numbers, Verbal Claims Ben-Gurion University of the Negev 57 True Rank 7 12 11 11 7 8 2 12 12 11 5 11 11 10 1 6 9 7 4 3
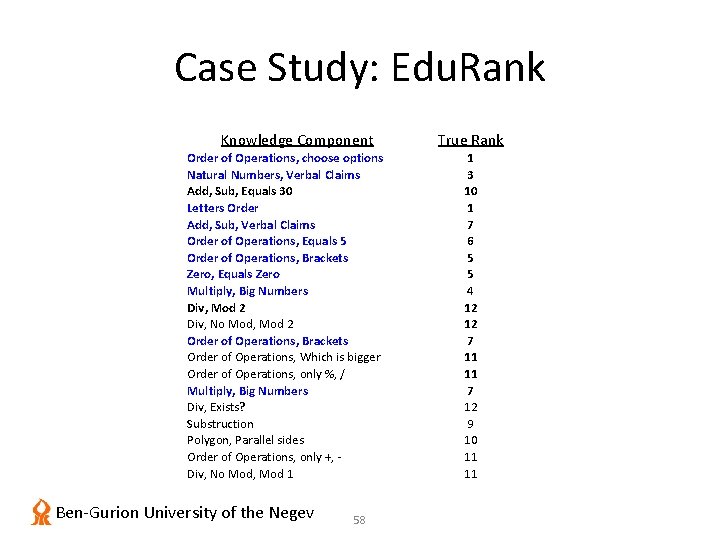
Case Study: Edu. Rank Knowledge Component Order of Operations, choose options Natural Numbers, Verbal Claims Add, Sub, Equals 30 Letters Order Add, Sub, Verbal Claims Order of Operations, Equals 5 Order of Operations, Brackets Zero, Equals Zero Multiply, Big Numbers Div, Mod 2 Div, No Mod, Mod 2 Order of Operations, Brackets Order of Operations, Which is bigger Order of Operations, only %, / Multiply, Big Numbers Div, Exists? Substruction Polygon, Parallel sides Order of Operations, only +, - Div, No Mod, Mod 1 Ben-Gurion University of the Negev 58 True Rank 1 3 10 1 7 6 5 5 4 12 12 7 11 11 7 12 9 10 11 11
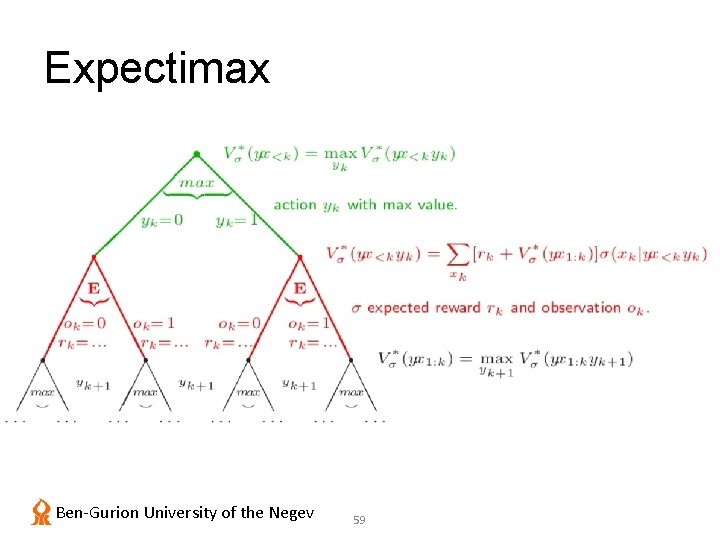
Expectimax Ben-Gurion University of the Negev 59
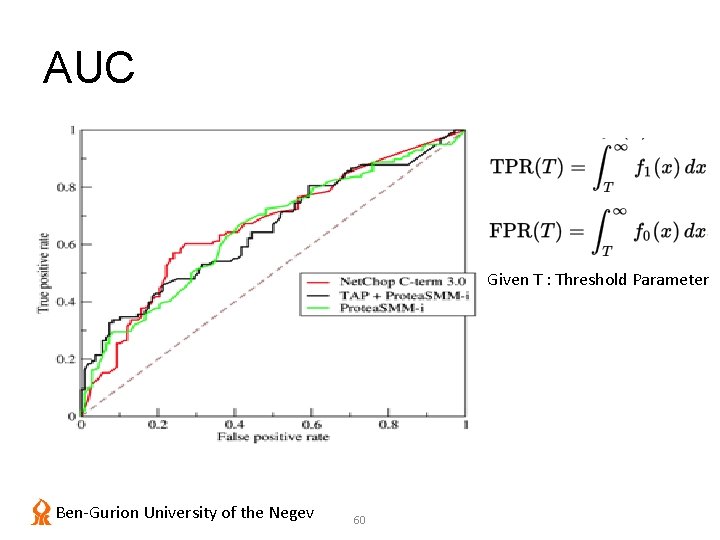
AUC Given T : Threshold Parameter Ben-Gurion University of the Negev 60
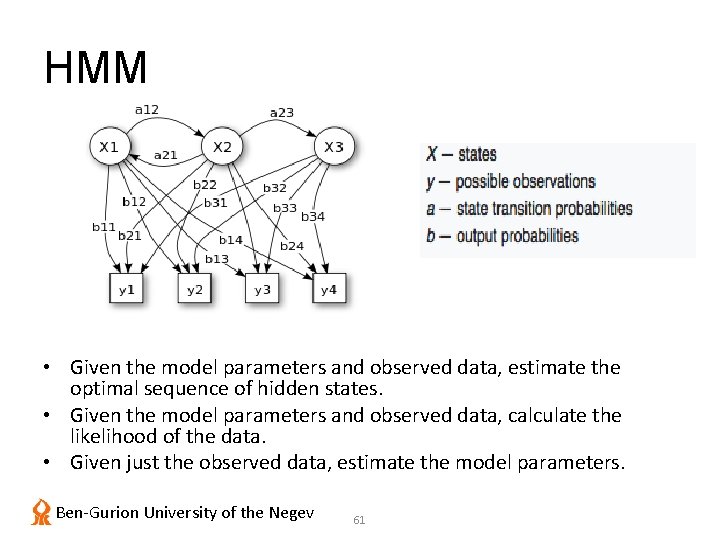
HMM • Given the model parameters and observed data, estimate the optimal sequence of hidden states. • Given the model parameters and observed data, calculate the likelihood of the data. • Given just the observed data, estimate the model parameters. Ben-Gurion University of the Negev 61
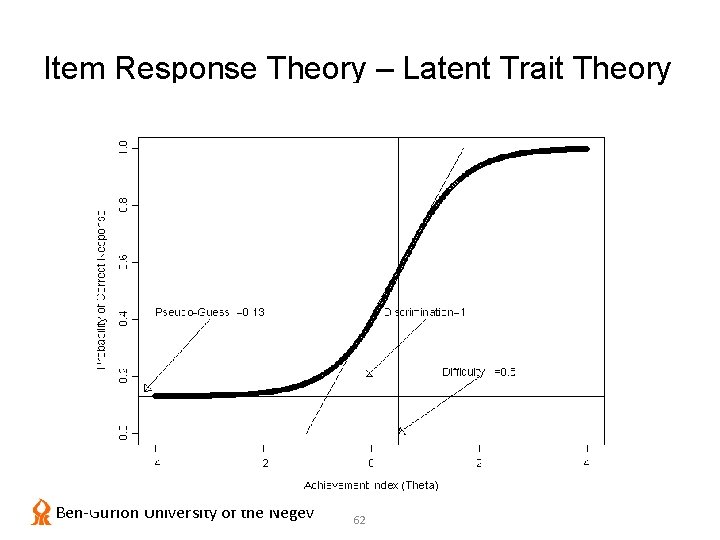
Item Response Theory – Latent Trait Theory Ben-Gurion University of the Negev 62
Chris piech stanford
Chris piech stanford
Spencer 1993
Spencer and spencer 1993
Iceberg della competenza
Iceberg model competency
Modello iceberg di spencer e spencer
Matrice delle competenze
Contoh shallow knowledge dan deep knowledge
Karolina piech
Karolina piech
深哉深哉耶穌的愛
Deep asleep deep asleep it lies
Deep forest: towards an alternative to deep neural networks
Chris jonathan
Knowledge claim
Knowledge creation and knowledge architecture
"the knowledge society" "the knowledge society" or tks
Personal and shared knowledge
Aposteriori
Knowledge shared is knowledge squared meaning
Street knowledge vs book knowledge
Knowledge shared is knowledge multiplied meaning
Al fayed
Rosa spencer
Martin luthor king
John spencer diana
Robert spencer manchester
Spencer wu
Militarisml
Jt spencer death
Aprendizaje cooperativo spencer kagan
Taylorsville elementary school
El estado teológico
Marks&spencer czechy
Herbert spencer social darwinism
Edie mccredie bus
Hannah chaplin charles spencer chaplin sr.
Princess diana family tree
Sociology major
Spencers burrowing frog
What is antineutrino
Spencer kagan
Mark spencer martain
Marks and spencer competitors analysis
Spencer macquarrie
Tim henn spencer stuart
The spencer optics company produces an inexpensive
Teoria de spencer
Spencer-oatey h. (2012) what is culture
M. lyle spencer
Spencer capital holdings
Herbert spencer theory
Spencer muse
Spencer rugaber
Percy spencer invented the microwave
Spencer
Dr lisa spencer
Iceberg freud
Psychology: principles in practice answers
Spencer e barbosa