Curve decisionali per valutazione di modelli di previsione
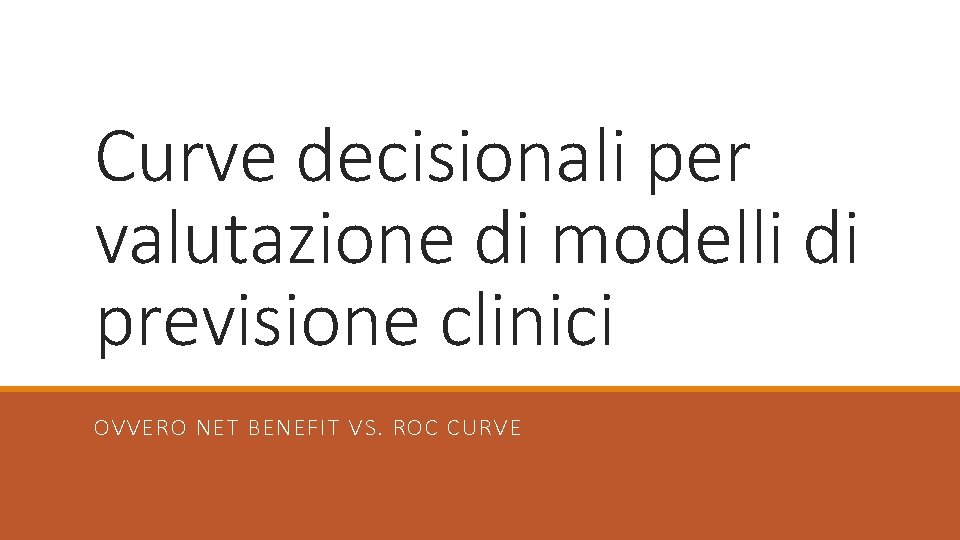
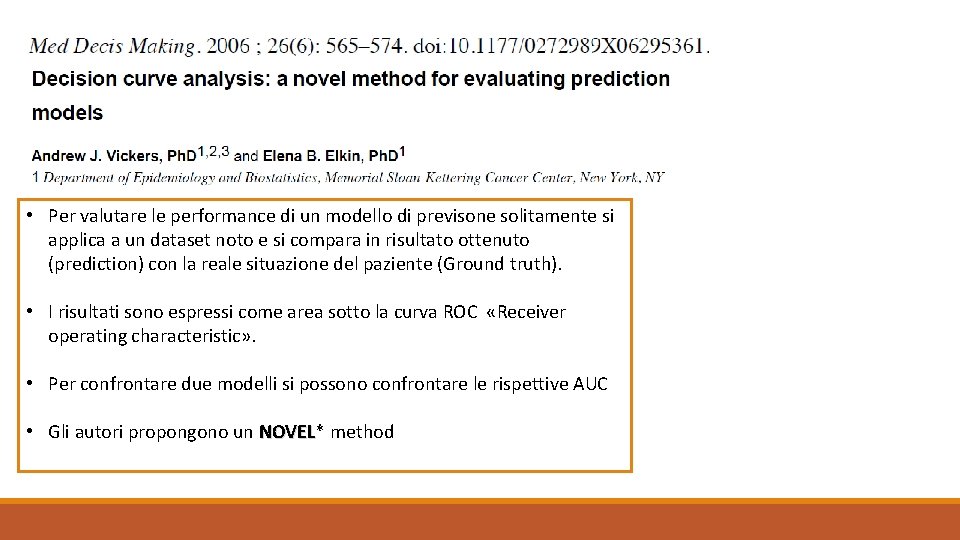
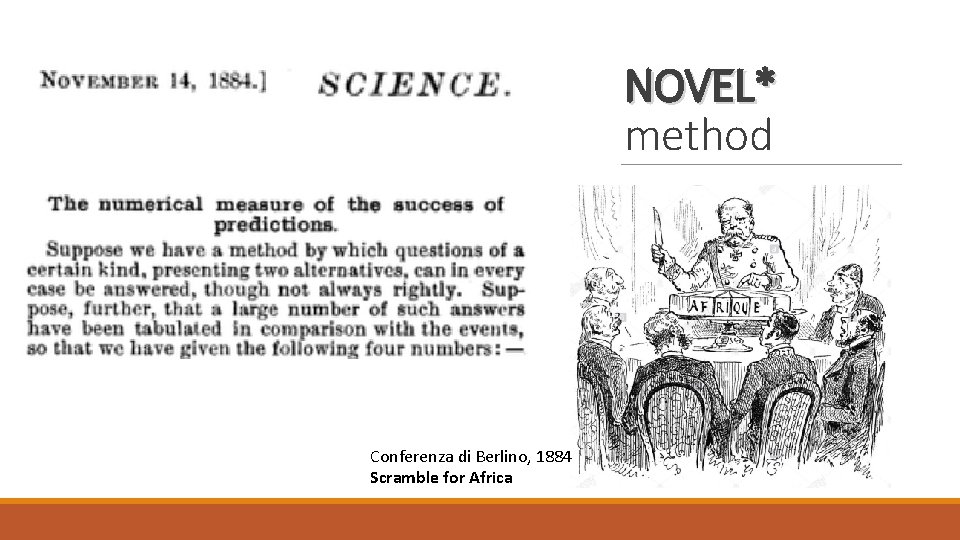
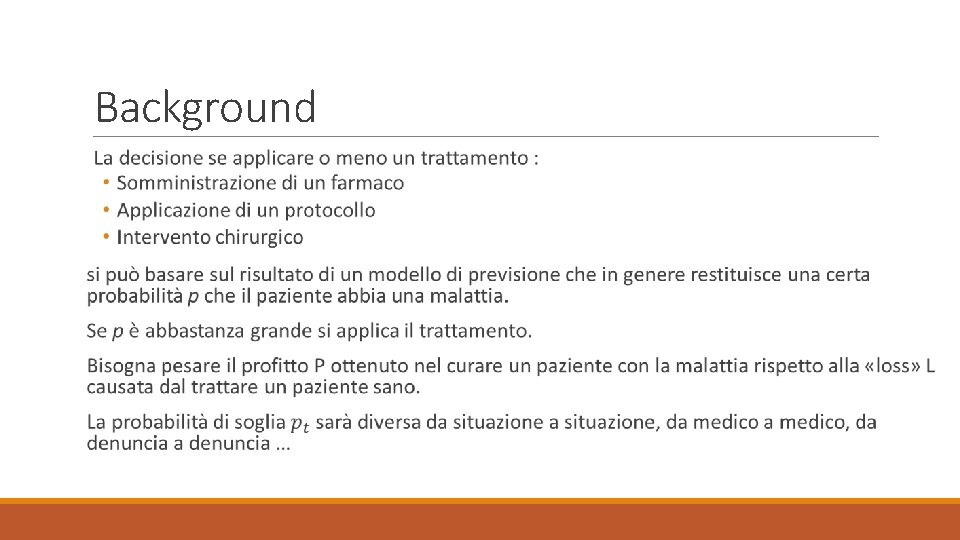
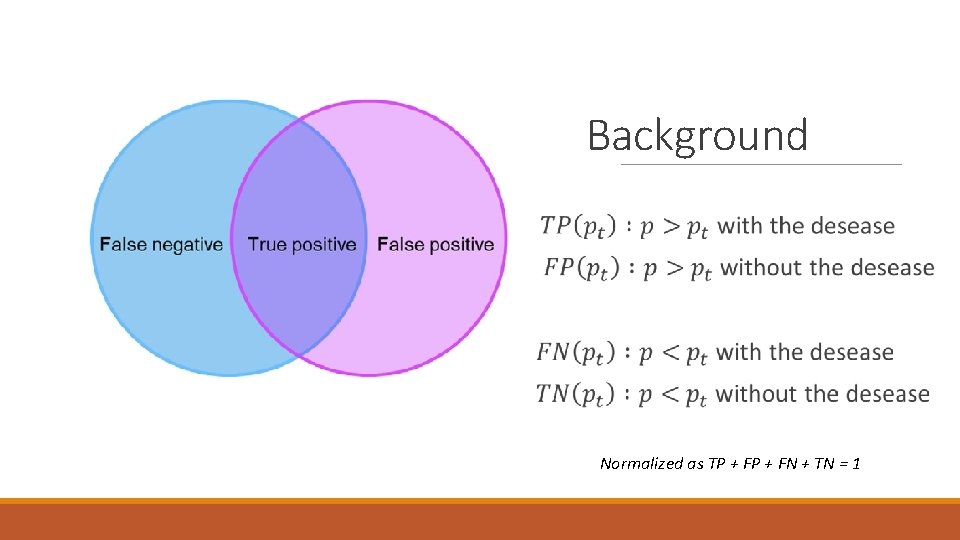
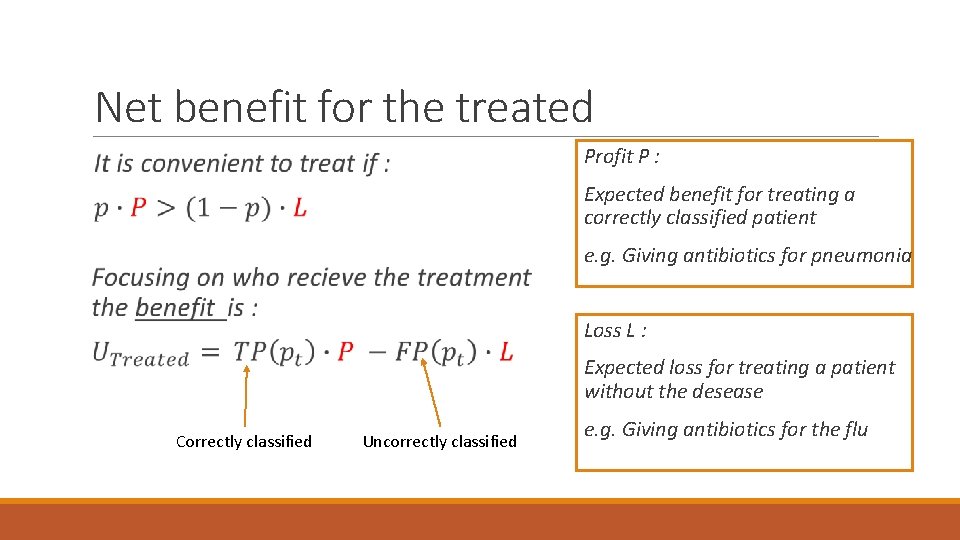
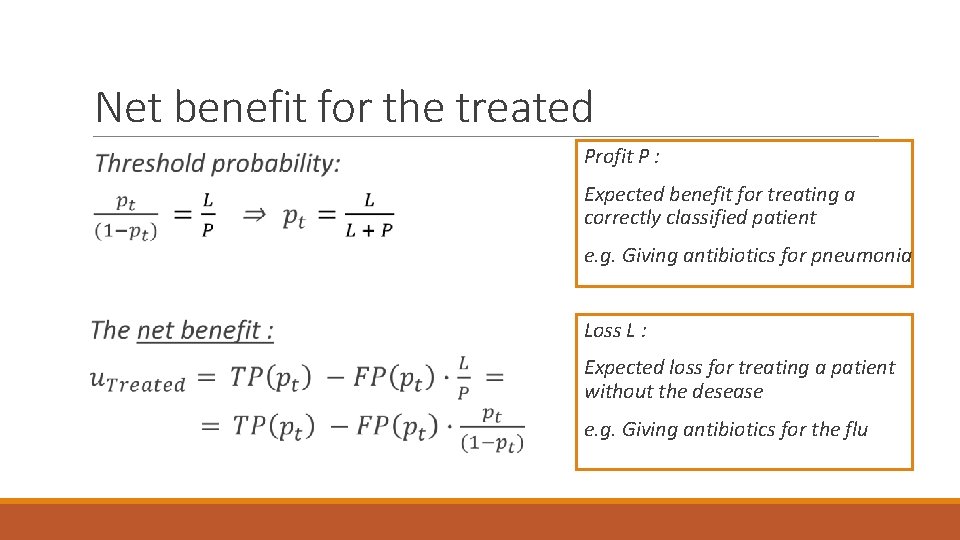
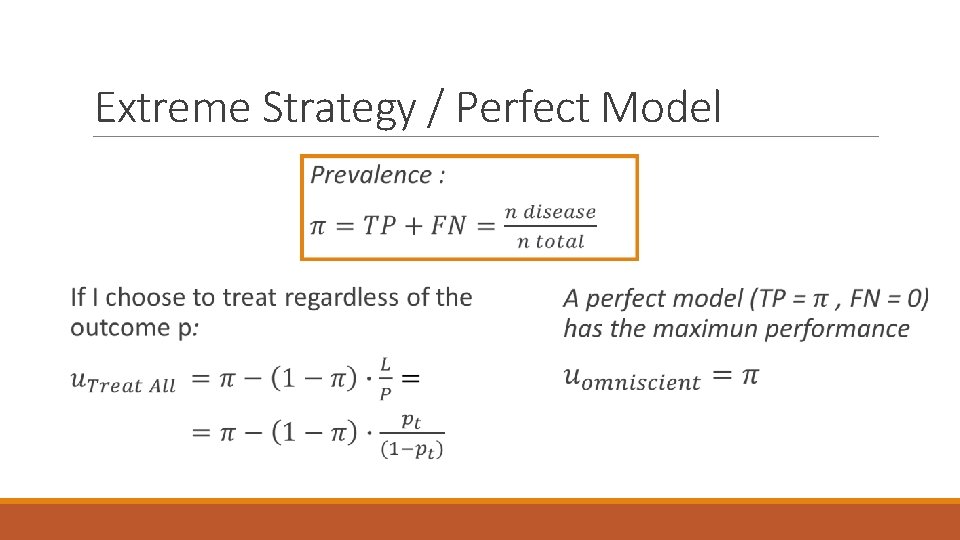
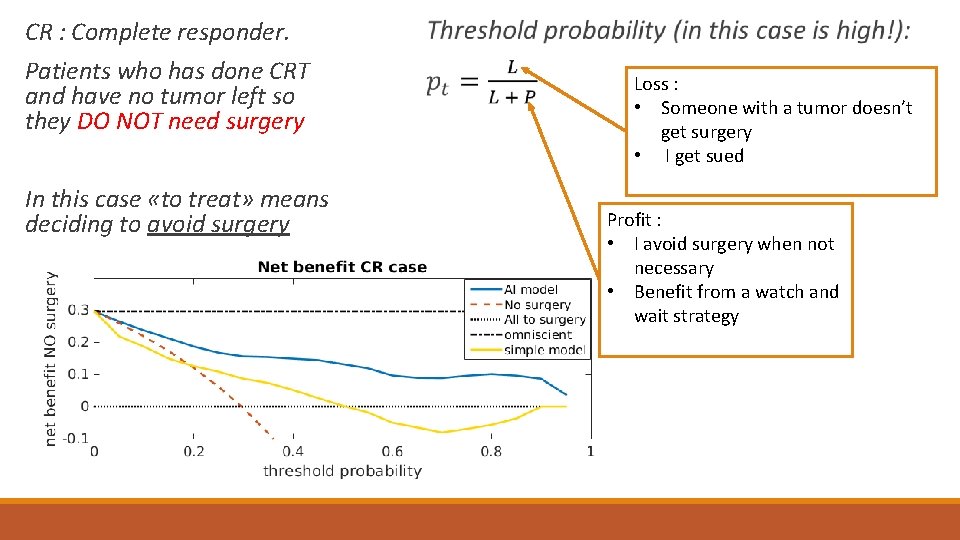
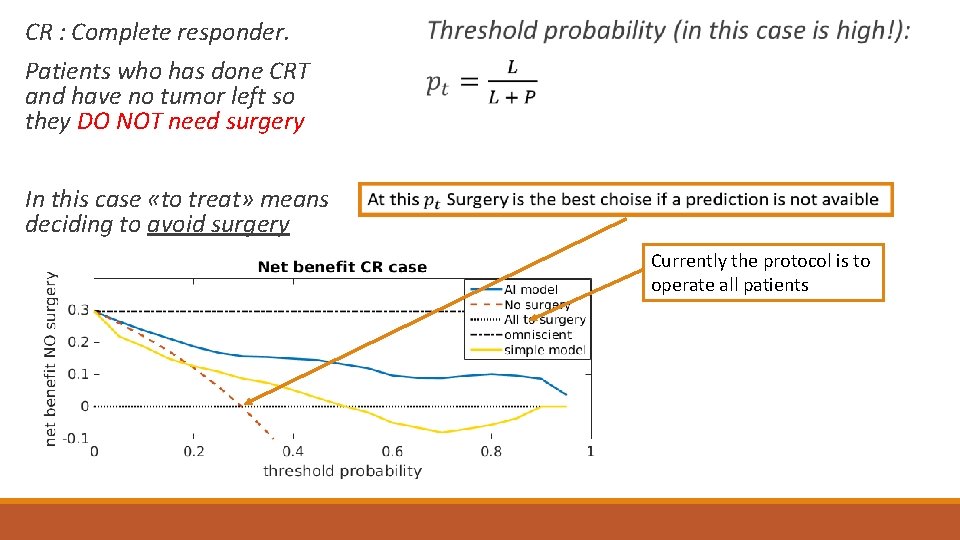
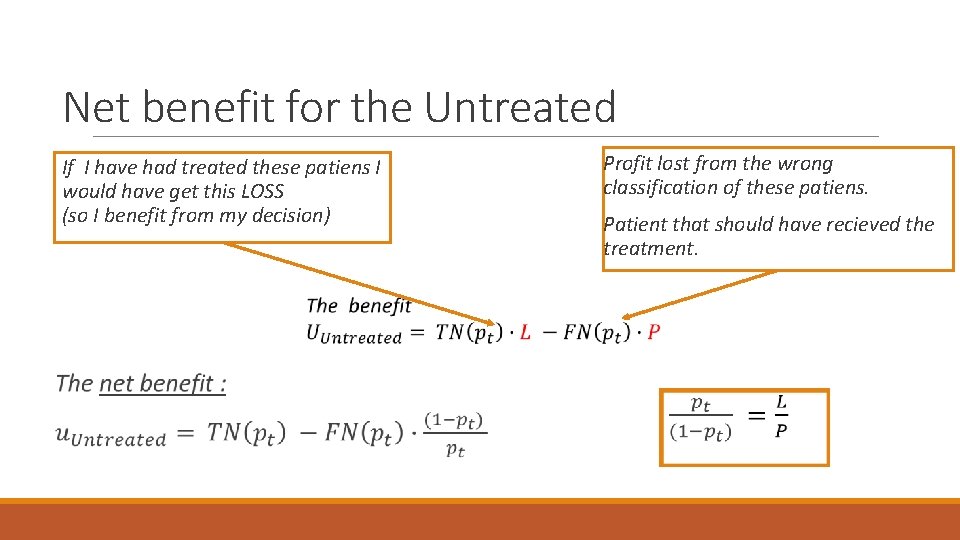
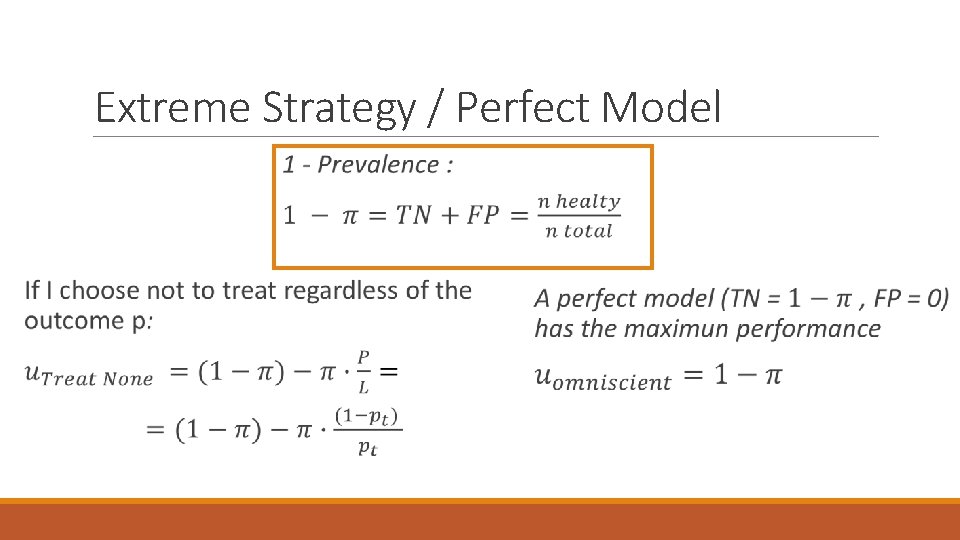
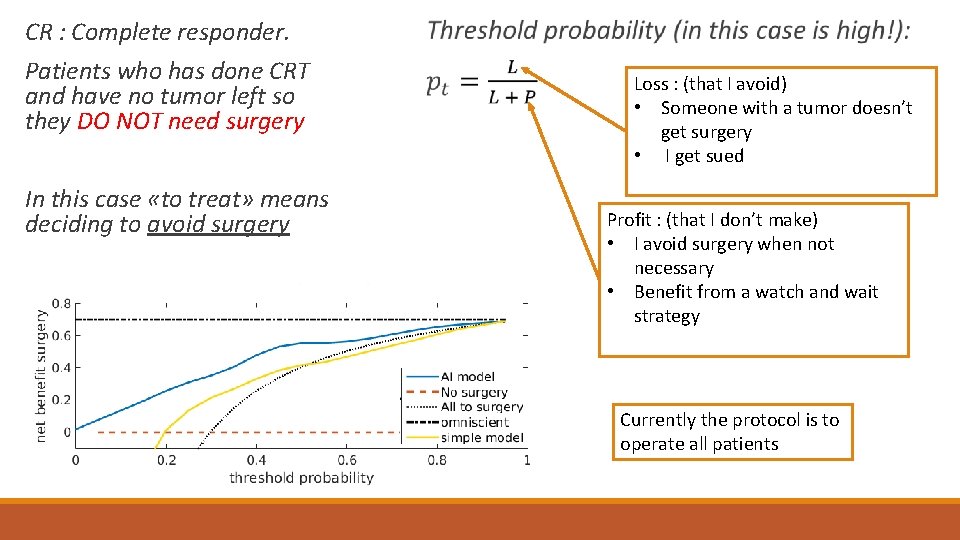
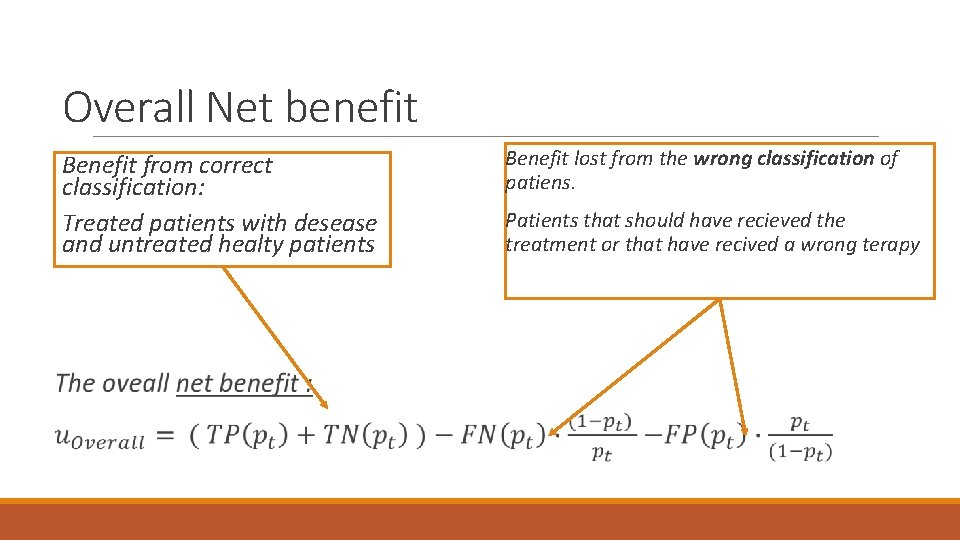
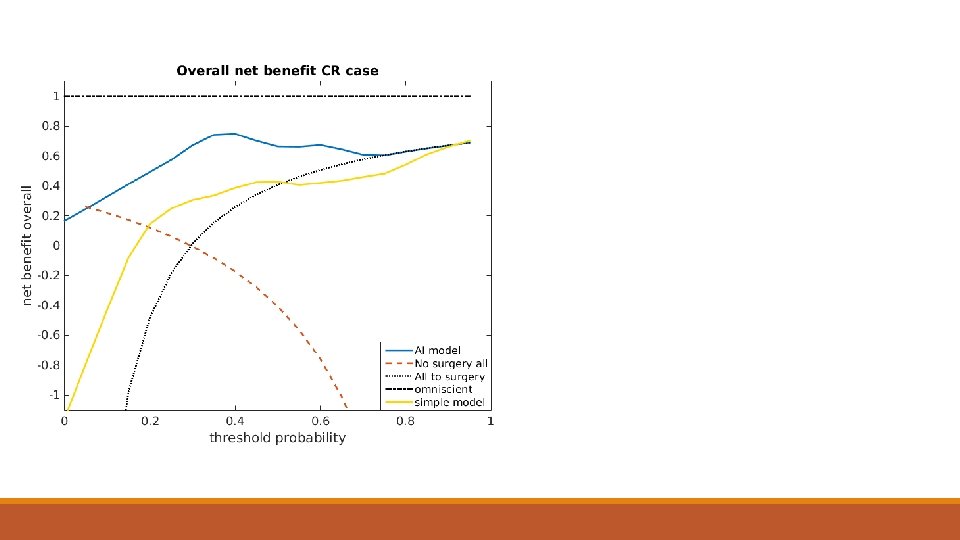
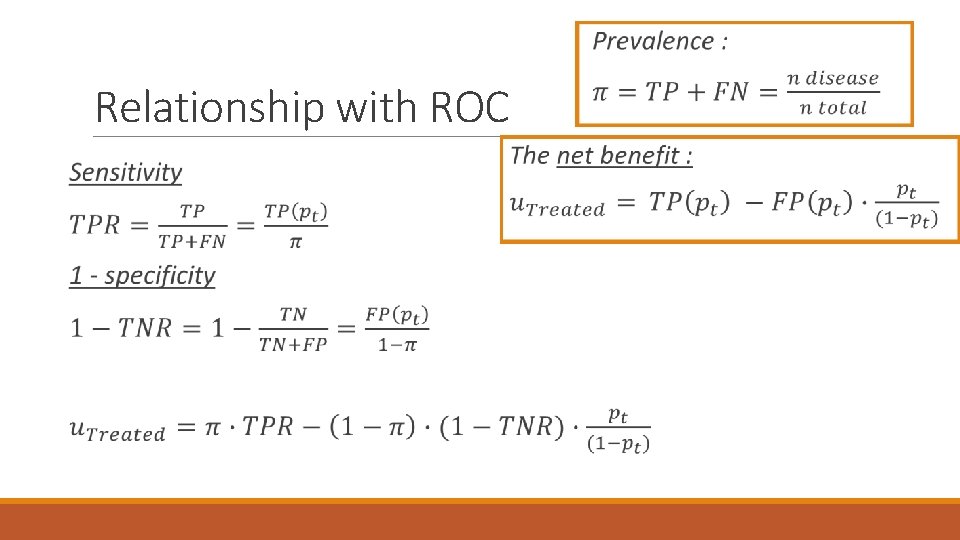
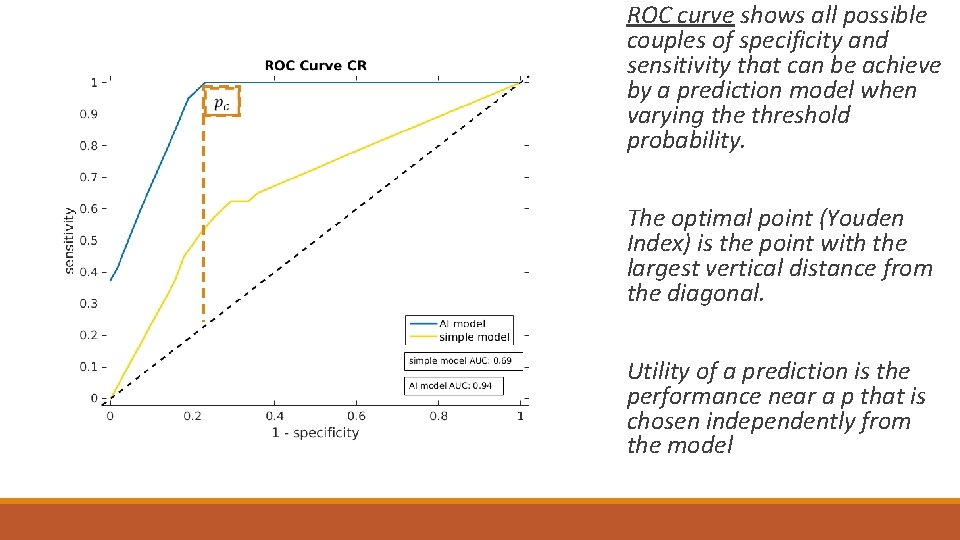
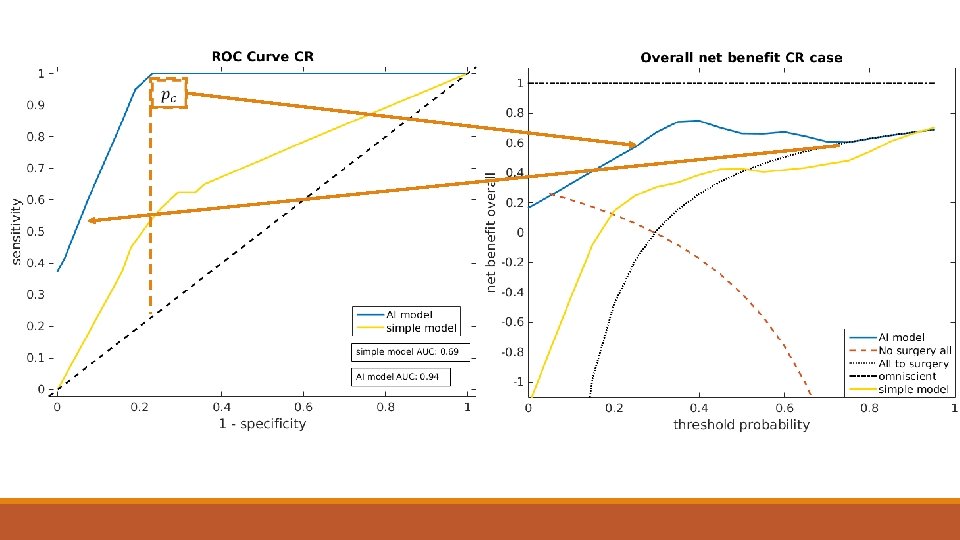
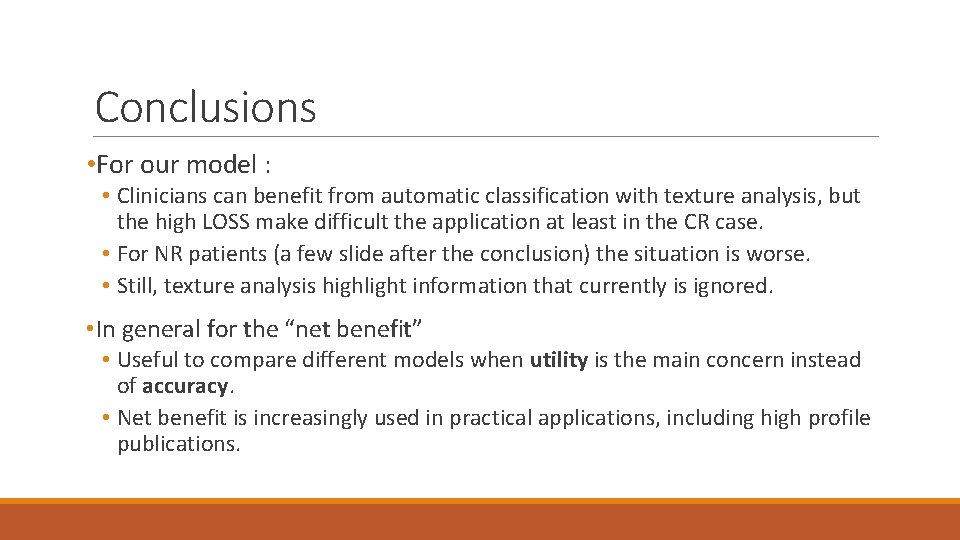
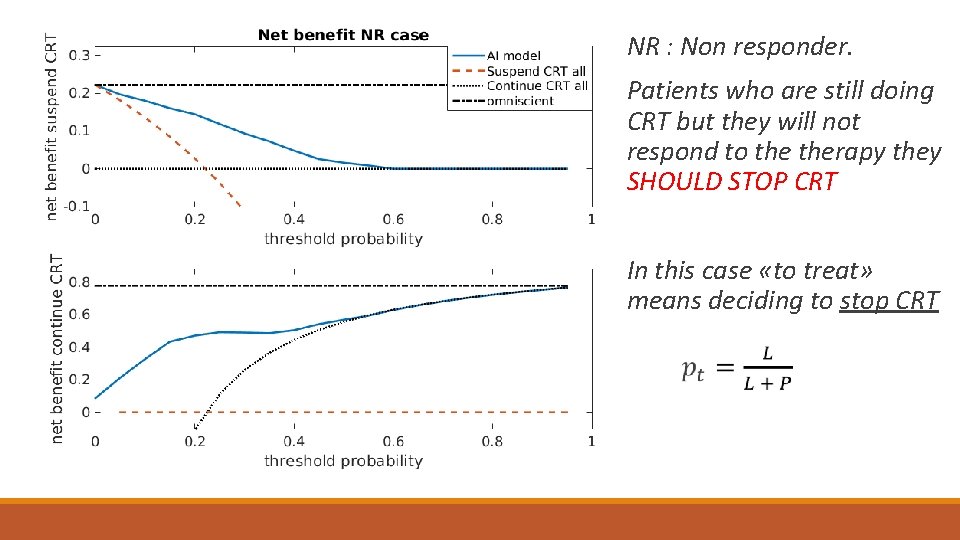
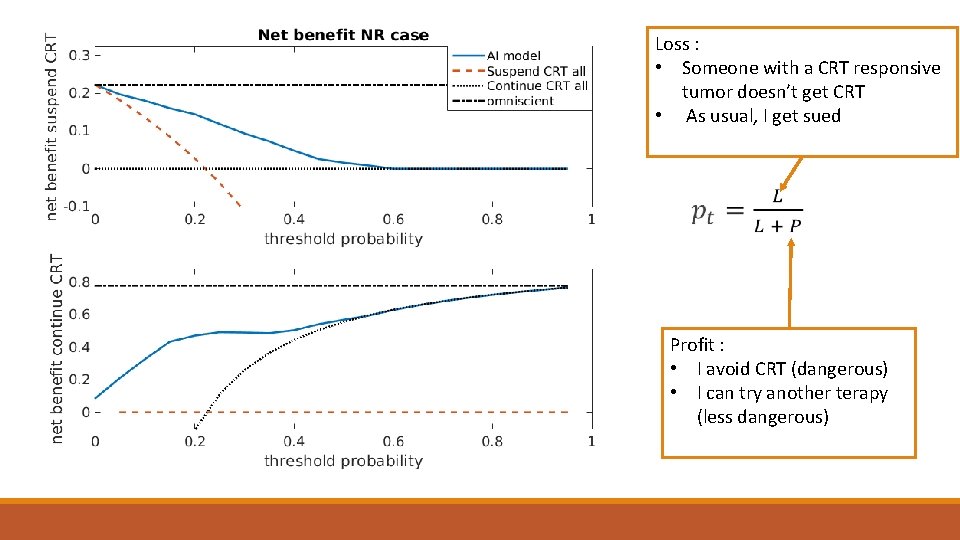
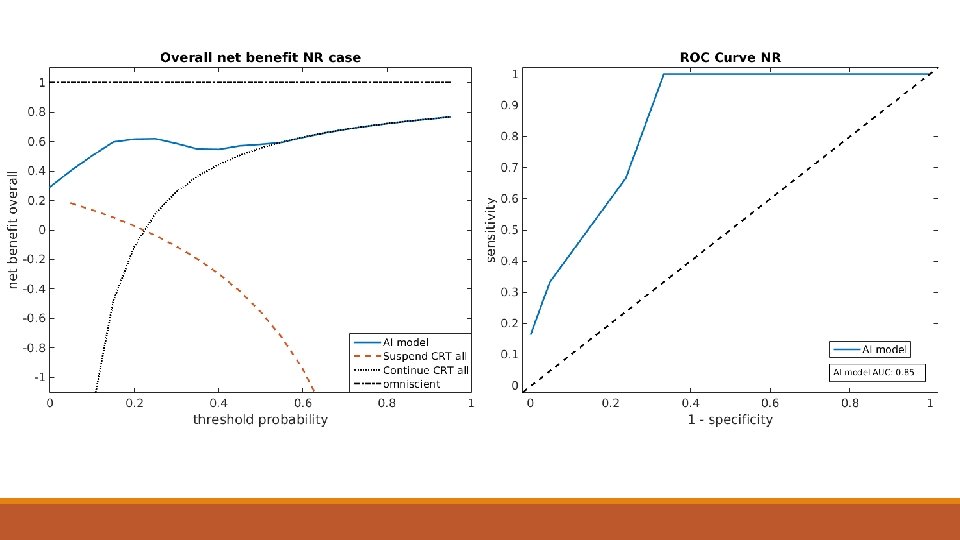
- Slides: 22
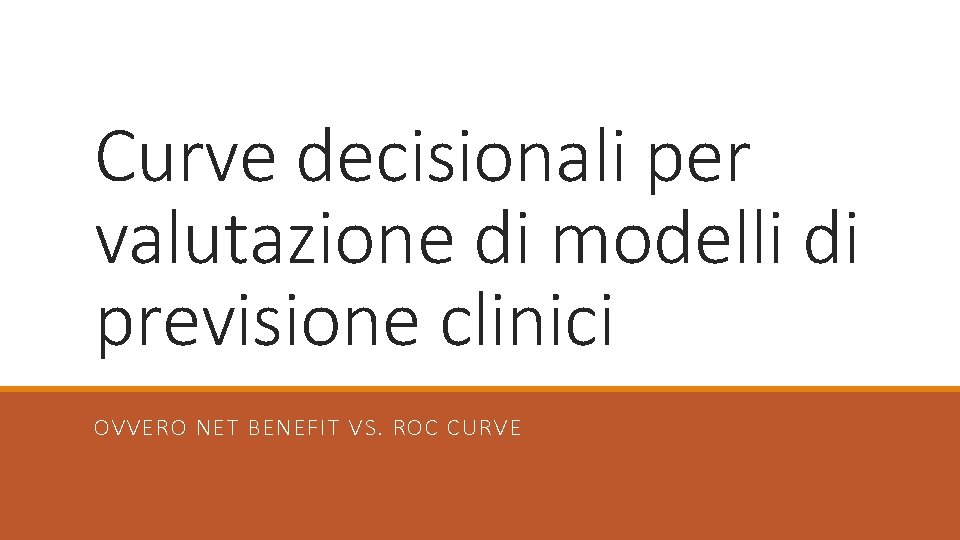
Curve decisionali per valutazione di modelli di previsione clinici OVVERO NET BENEFIT VS. ROC CURVE
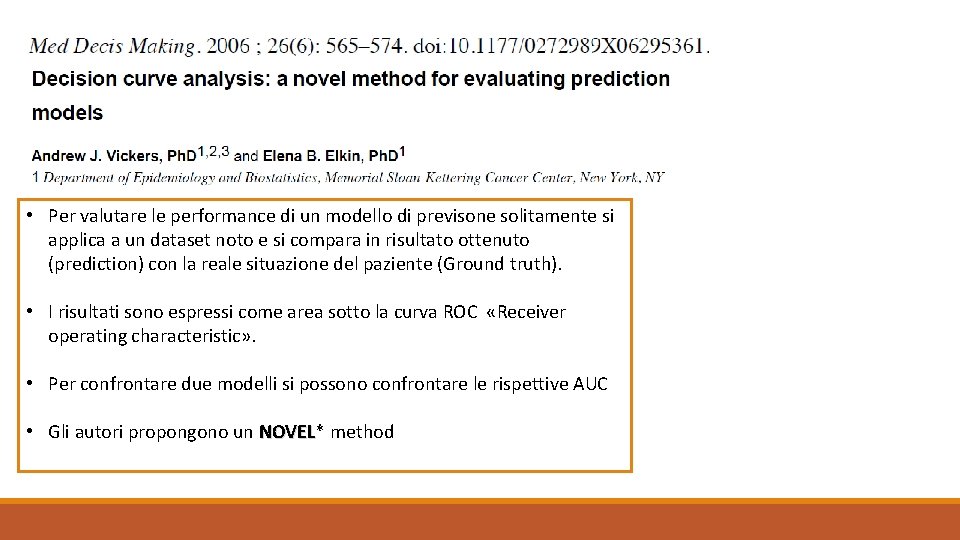
• Per valutare le performance di un modello di previsone solitamente si applica a un dataset noto e si compara in risultato ottenuto (prediction) con la reale situazione del paziente (Ground truth). • I risultati sono espressi come area sotto la curva ROC «Receiver operating characteristic» . • Per confrontare due modelli si possono confrontare le rispettive AUC • Gli autori propongono un NOVEL* NOVEL method
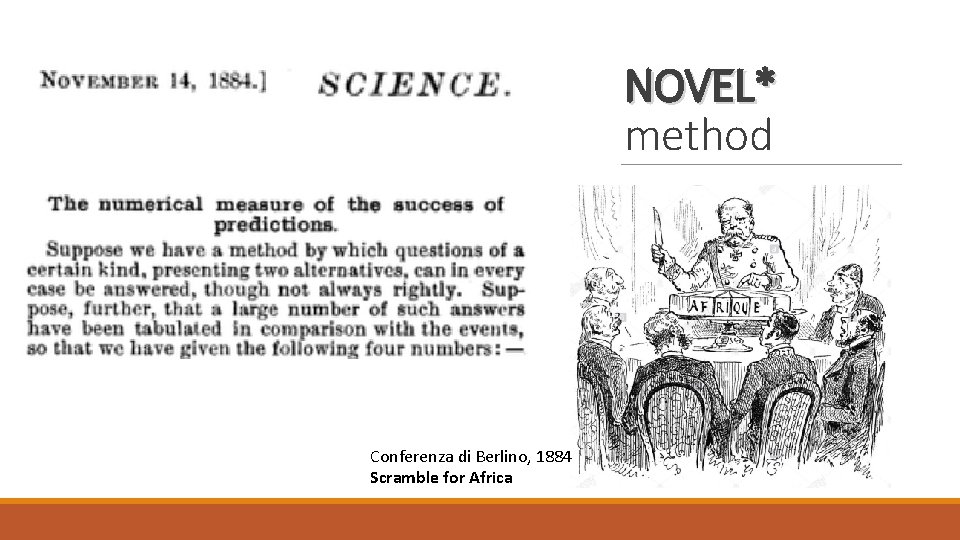
NOVEL* method Conferenza di Berlino, 1884 Scramble for Africa
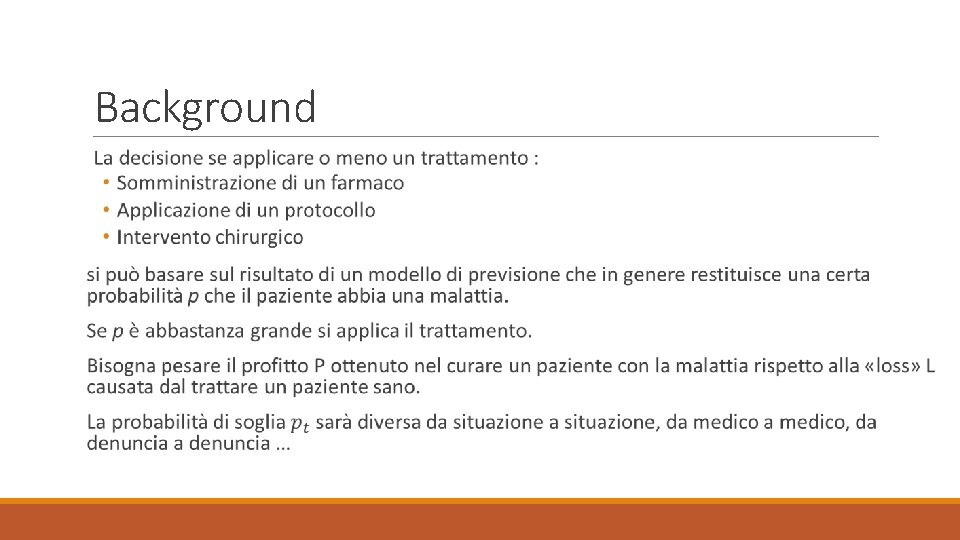
Background
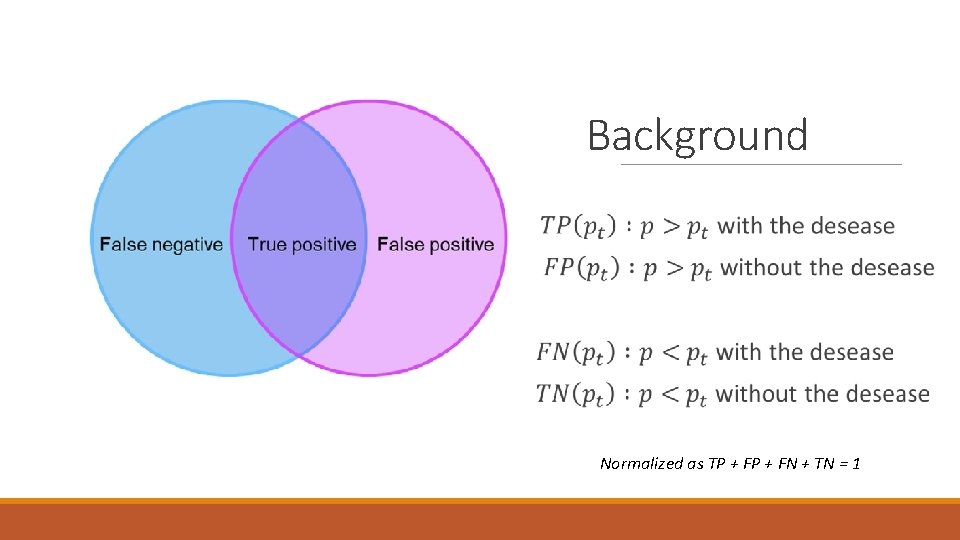
Background Normalized as TP + FN + TN = 1
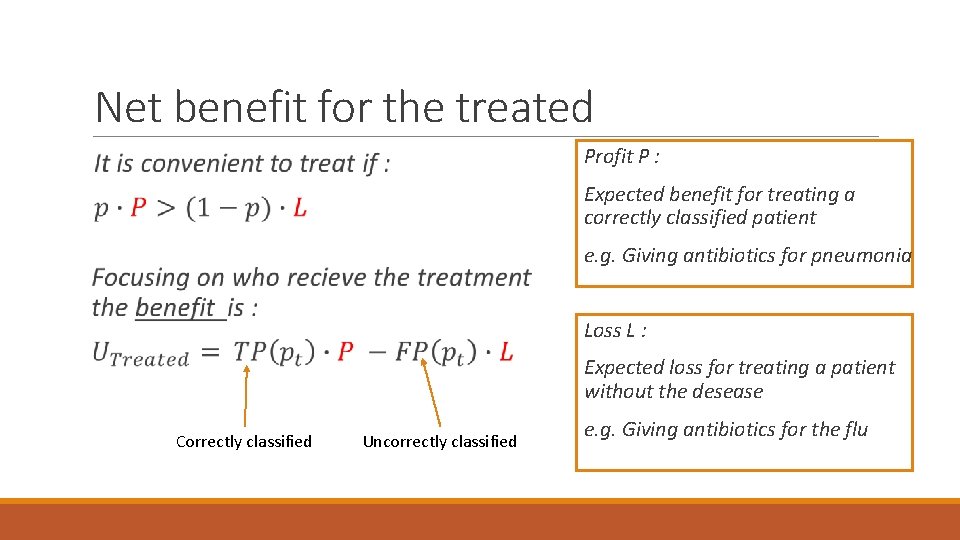
Net benefit for the treated Profit P : Expected benefit for treating a correctly classified patient e. g. Giving antibiotics for pneumonia Loss L : Expected loss for treating a patient without the desease Correctly classified Uncorrectly classified e. g. Giving antibiotics for the flu
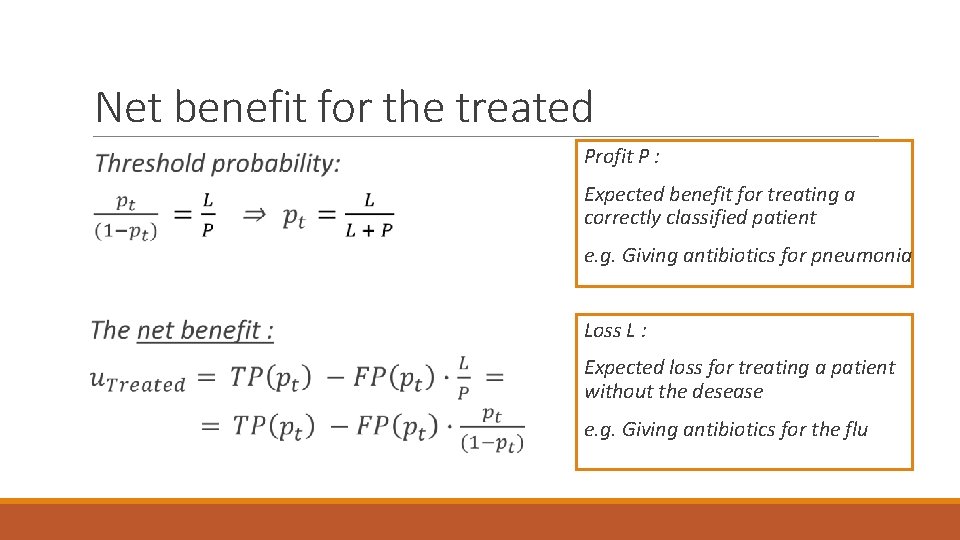
Net benefit for the treated Profit P : Expected benefit for treating a correctly classified patient e. g. Giving antibiotics for pneumonia Loss L : Expected loss for treating a patient without the desease e. g. Giving antibiotics for the flu
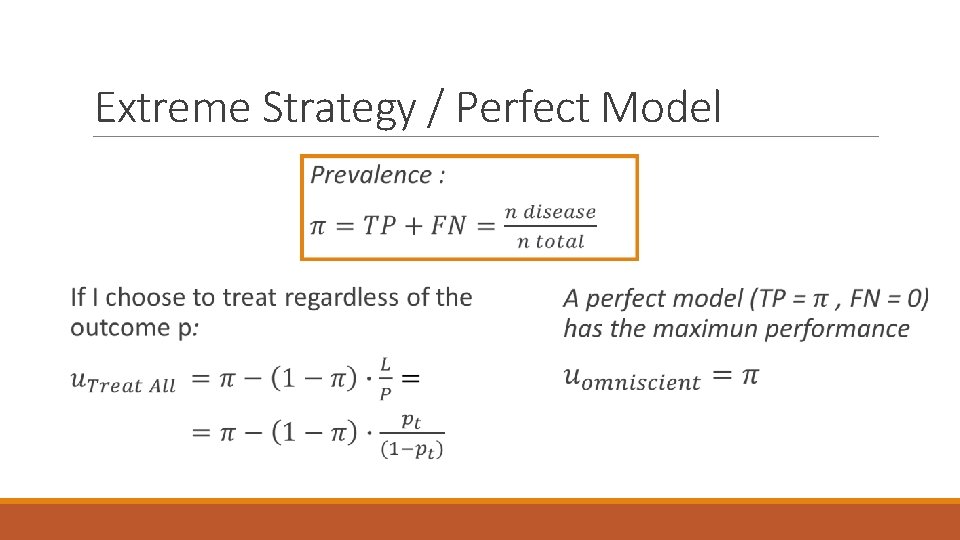
Extreme Strategy / Perfect Model
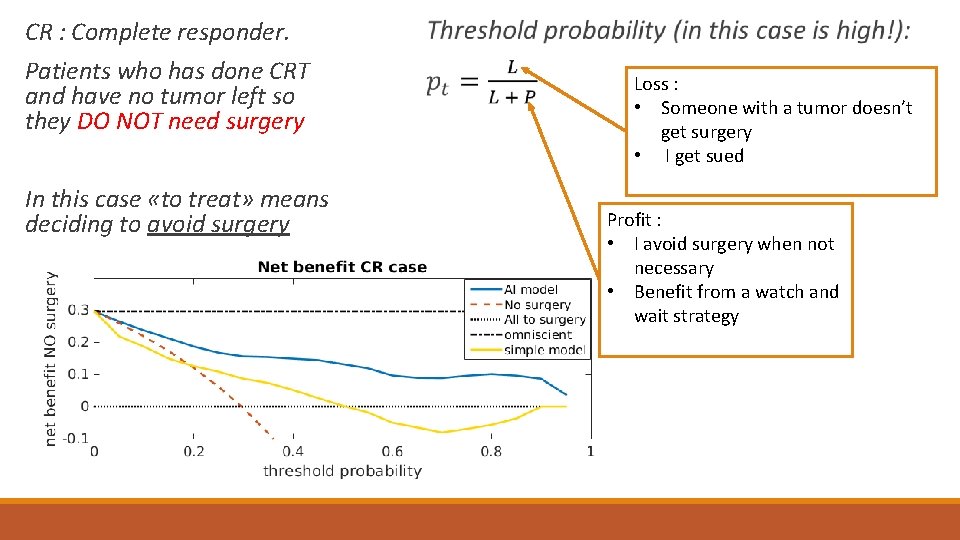
CR : Complete responder. Patients who has done CRT and have no tumor left so they DO NOT need surgery In this case «to treat» means deciding to avoid surgery Loss : • Someone with a tumor doesn’t get surgery • I get sued Profit : • I avoid surgery when not necessary • Benefit from a watch and wait strategy
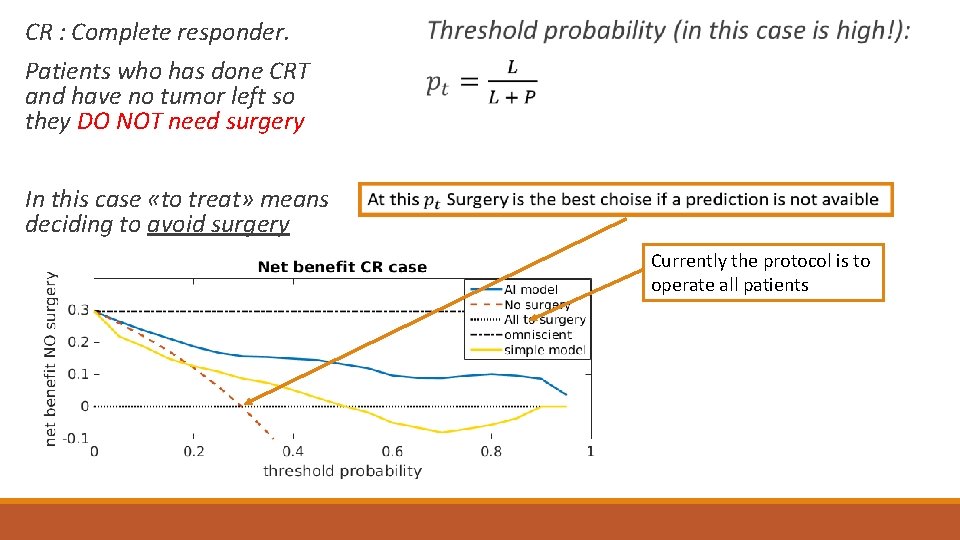
CR : Complete responder. Patients who has done CRT and have no tumor left so they DO NOT need surgery In this case «to treat» means deciding to avoid surgery Currently the protocol is to operate all patients
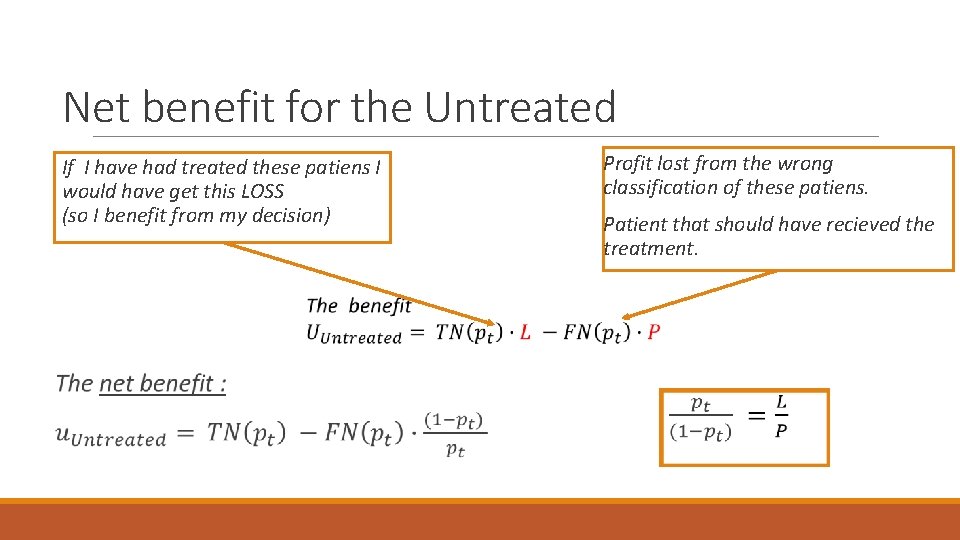
Net benefit for the Untreated If I have had treated these patiens I would have get this LOSS (so I benefit from my decision) Profit lost from the wrong classification of these patiens. Patient that should have recieved the treatment.
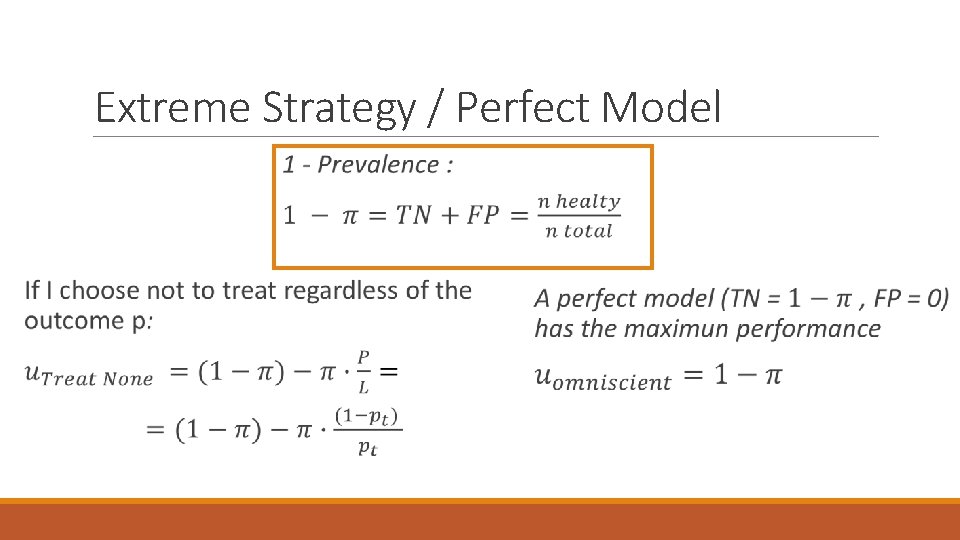
Extreme Strategy / Perfect Model
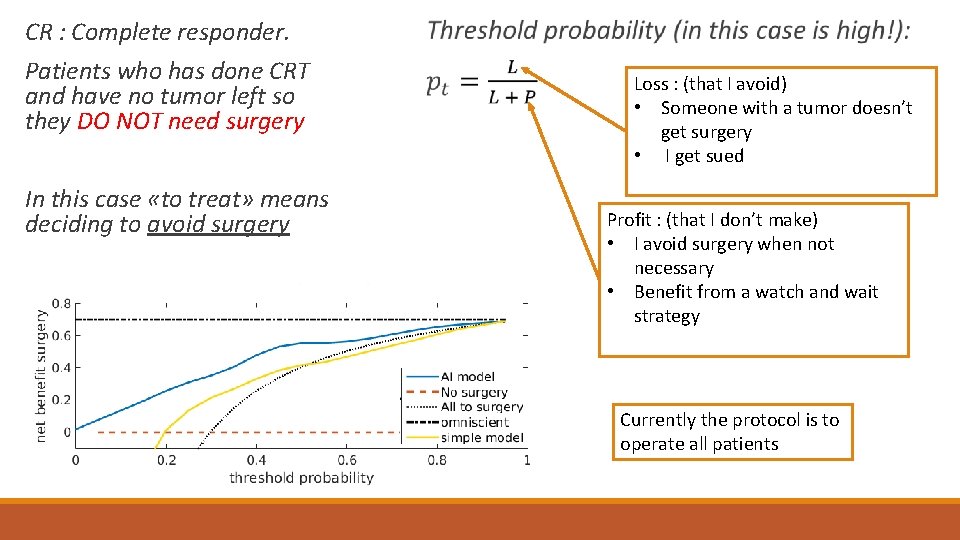
CR : Complete responder. Patients who has done CRT and have no tumor left so they DO NOT need surgery In this case «to treat» means deciding to avoid surgery Loss : (that I avoid) • Someone with a tumor doesn’t get surgery • I get sued Profit : (that I don’t make) • I avoid surgery when not necessary • Benefit from a watch and wait strategy Currently the protocol is to operate all patients
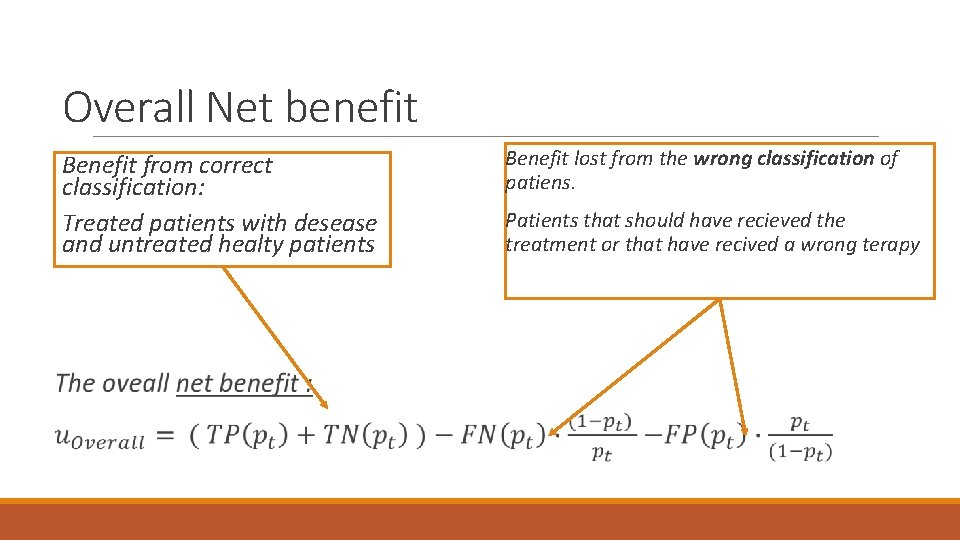
Overall Net benefit Benefit from correct classification: Treated patients with desease and untreated healty patients Benefit lost from the wrong classification of patiens. Patients that should have recieved the treatment or that have recived a wrong terapy
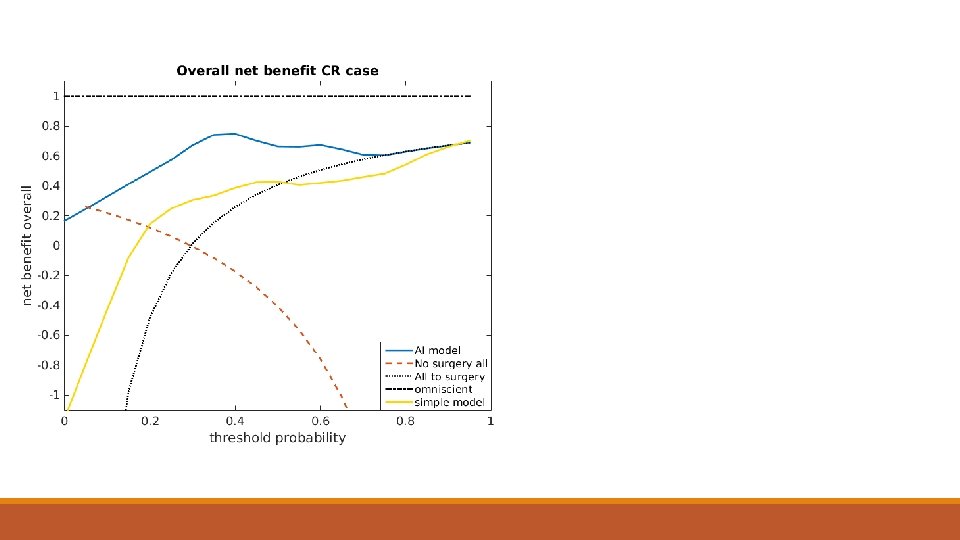
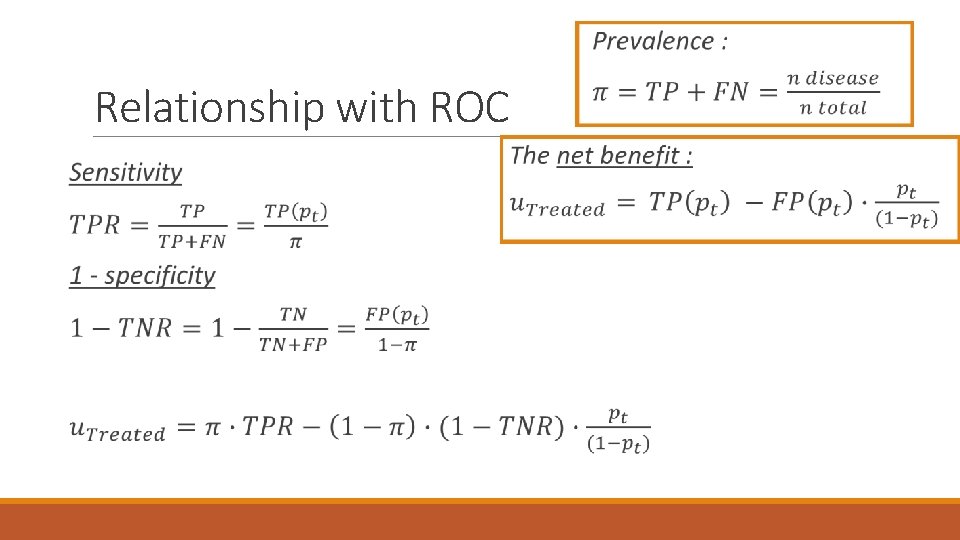
Relationship with ROC
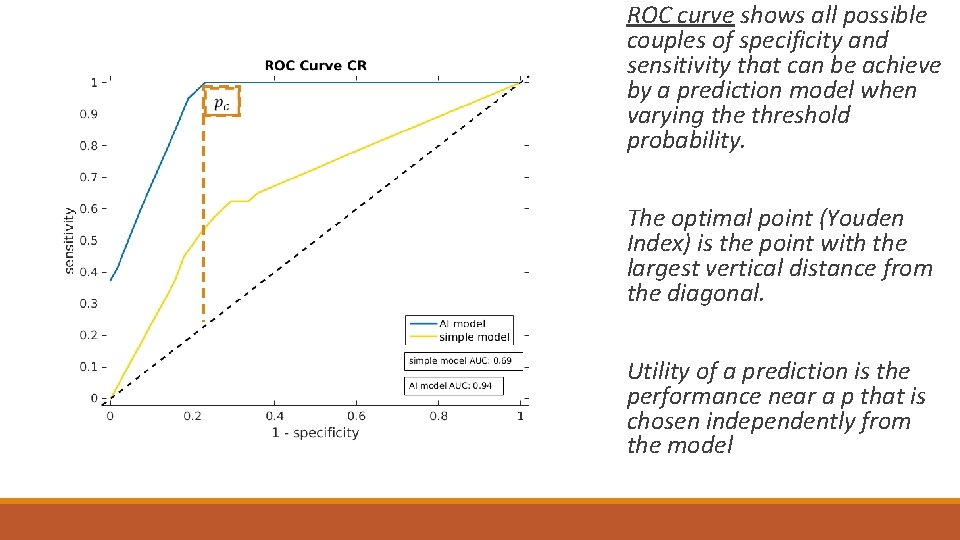
ROC curve shows all possible couples of specificity and sensitivity that can be achieve by a prediction model when varying the threshold probability. The optimal point (Youden Index) is the point with the largest vertical distance from the diagonal. Utility of a prediction is the performance near a p that is chosen independently from the model
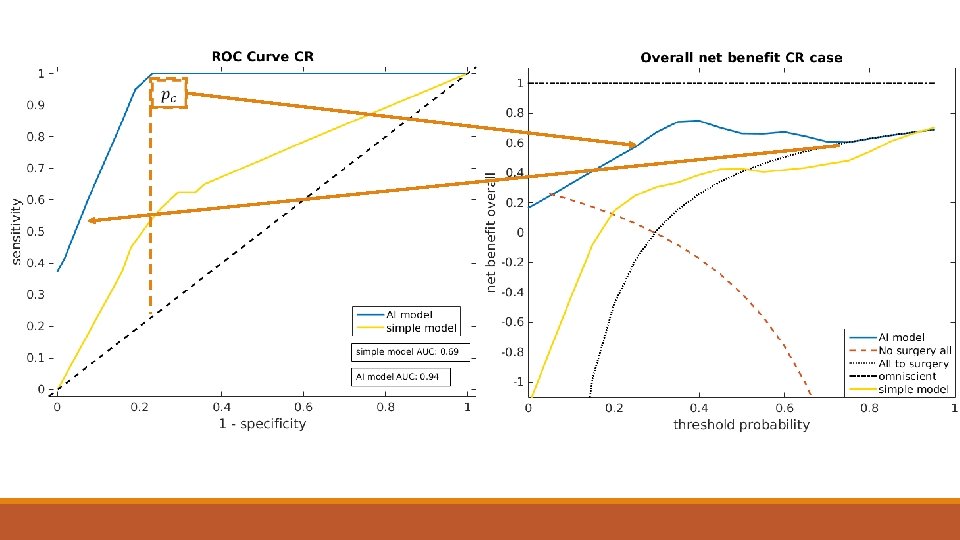
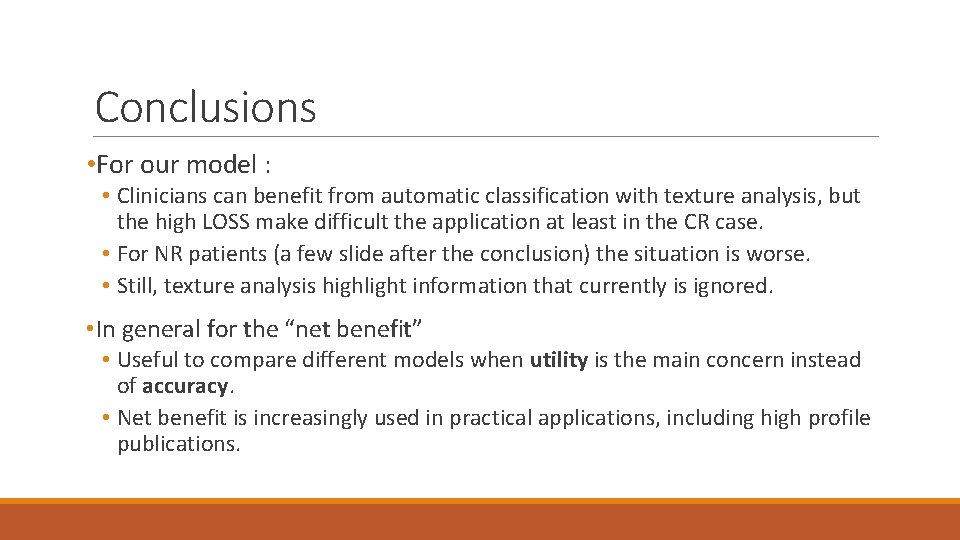
Conclusions • For our model : • Clinicians can benefit from automatic classification with texture analysis, but the high LOSS make difficult the application at least in the CR case. • For NR patients (a few slide after the conclusion) the situation is worse. • Still, texture analysis highlight information that currently is ignored. • In general for the “net benefit” • Useful to compare different models when utility is the main concern instead of accuracy. • Net benefit is increasingly used in practical applications, including high profile publications.
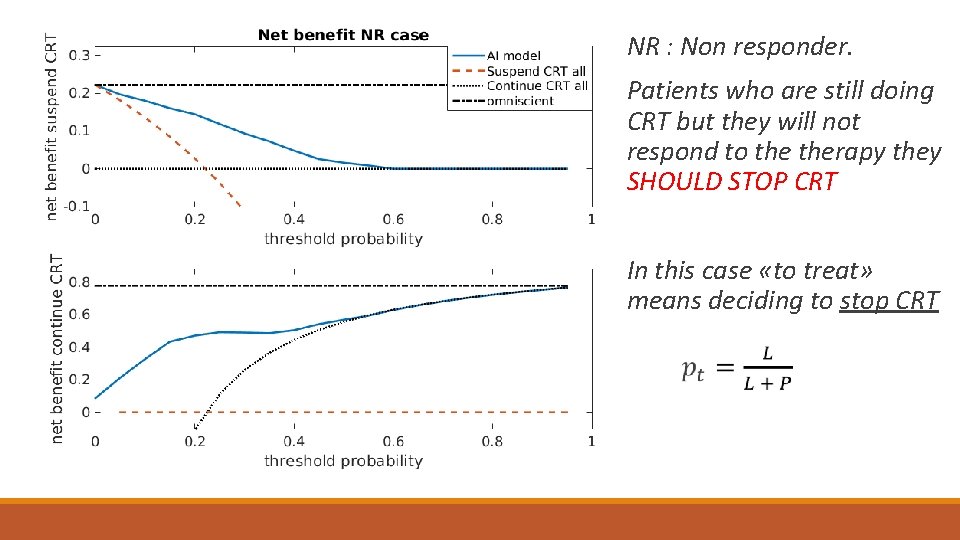
NR : Non responder. Patients who are still doing CRT but they will not respond to therapy they SHOULD STOP CRT In this case «to treat» means deciding to stop CRT
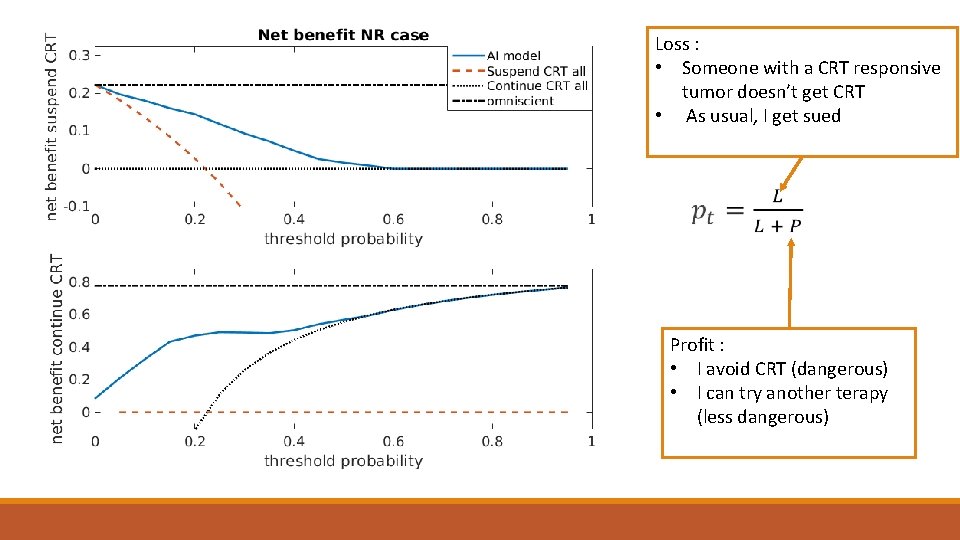
Loss : • Someone with a CRT responsive tumor doesn’t get CRT • As usual, I get sued Profit : • I avoid CRT (dangerous) • I can try another terapy (less dangerous)
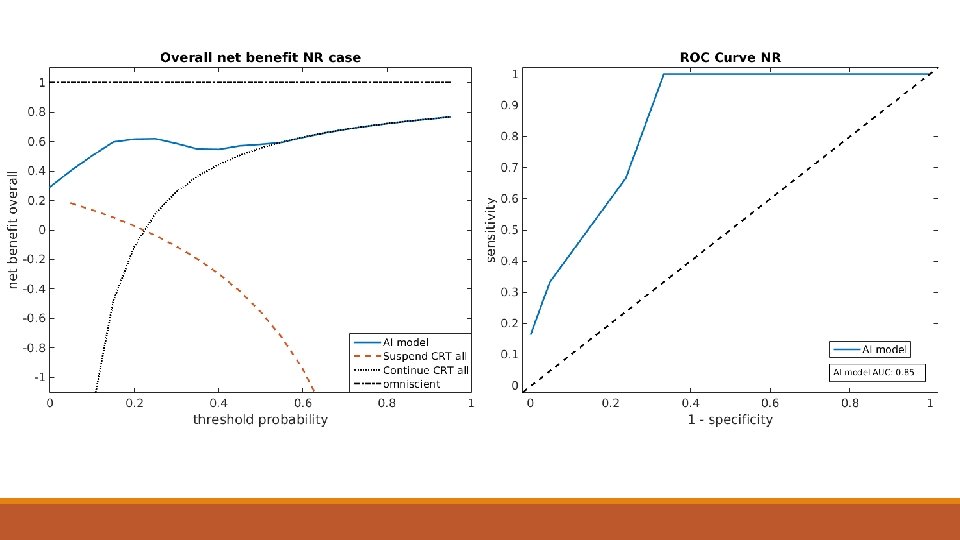
Modelli decisionali
Eurismi decisionali
Quafes
Griglia di valutazione per alunni stranieri
Tabella voti scuola media
Kounin glasser jones
Differenza tra primary nursing e case manager
Modelli base lapbook punti cardinali
Modelli di apprendimento
Mio nonno era un ciliegio schede didattiche
Organigramma divisionale esempio
Modelli di gestione delle risorse umane
Modelli di programmazione lineare
Da mendel ai modelli di ereditarietà
John dalton modello atomico
Evoluzione modelli atomici
Modelli deterministici e probabilistici psicologia
Hyperbolic and sigmoidal curve
S curve and j curve
Unilateral balanced occlusion adalah
Biotic potential and environmental resistance
Kruta ir kruta braska ir braska
Per capita vs per stirpes