Ariel Caticha on Information and Entropy July 8
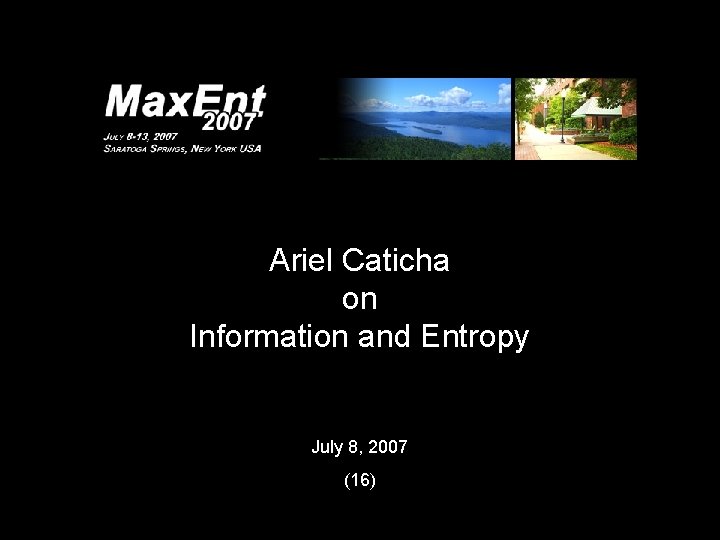
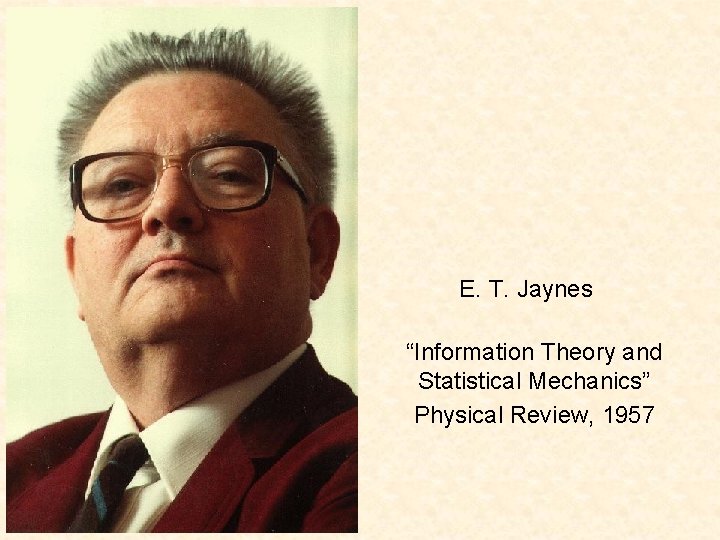
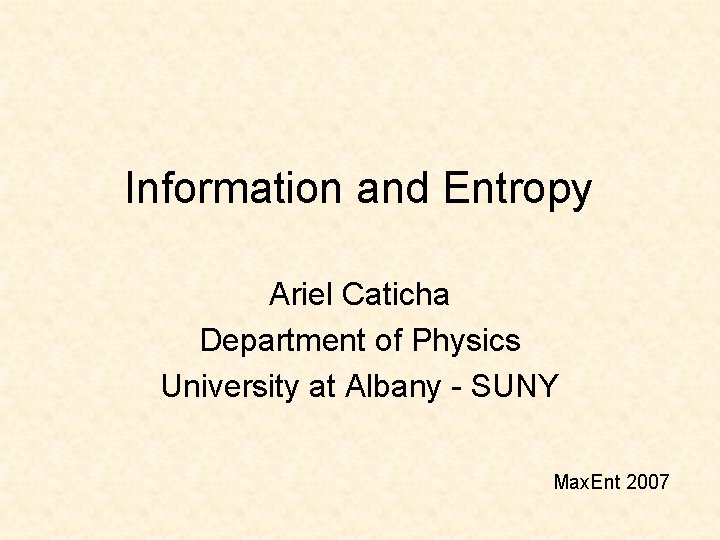
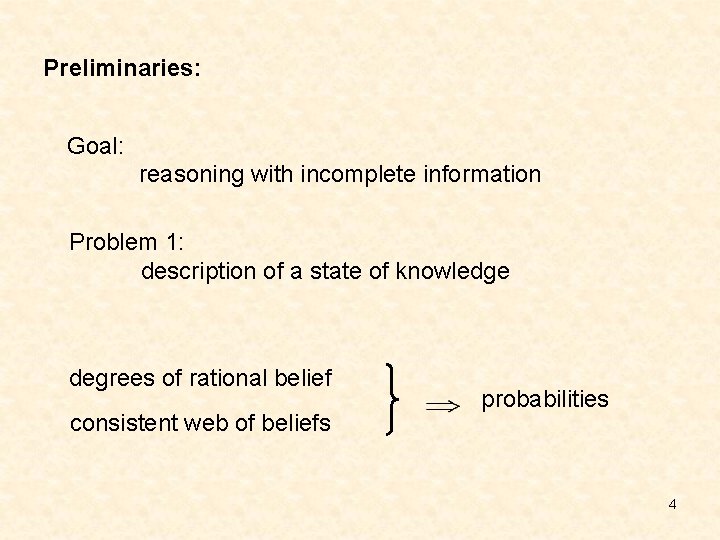
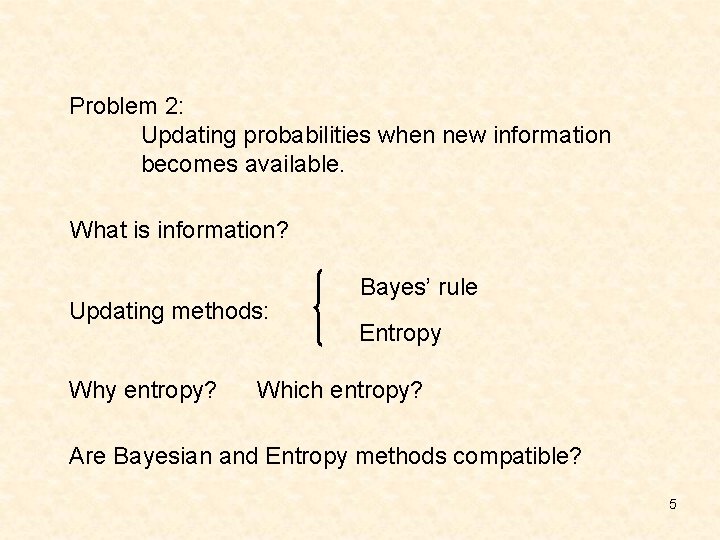
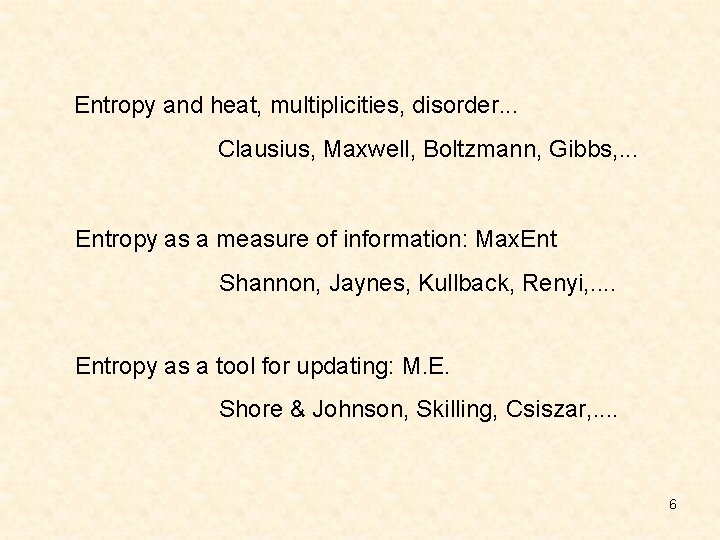
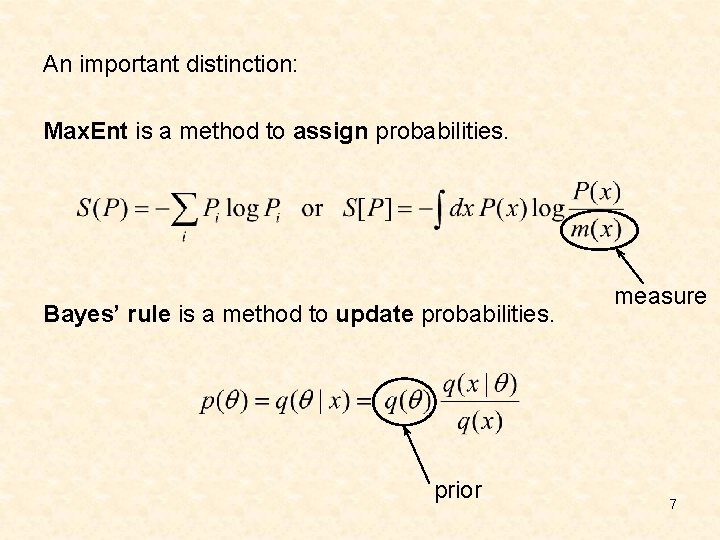
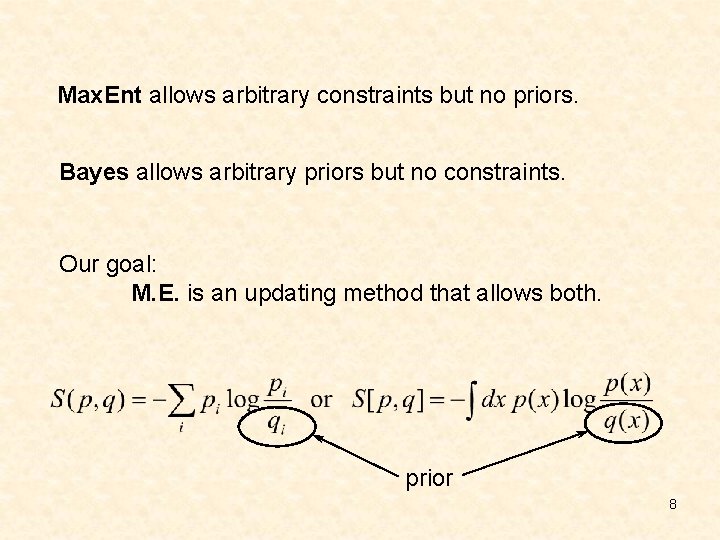
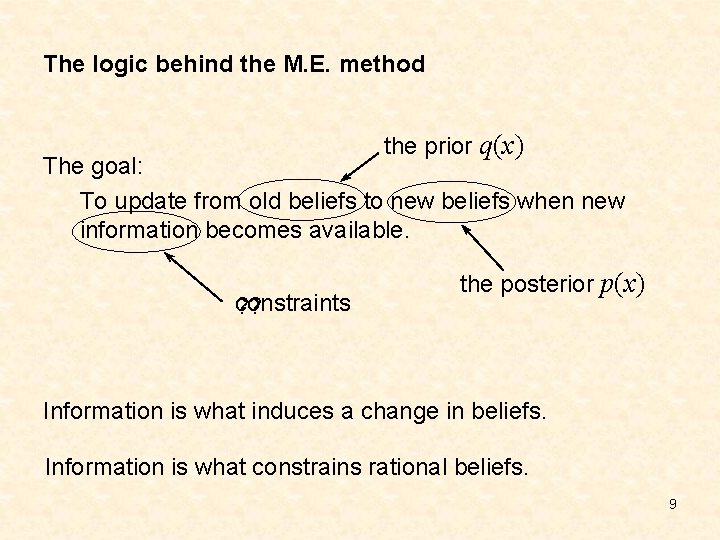
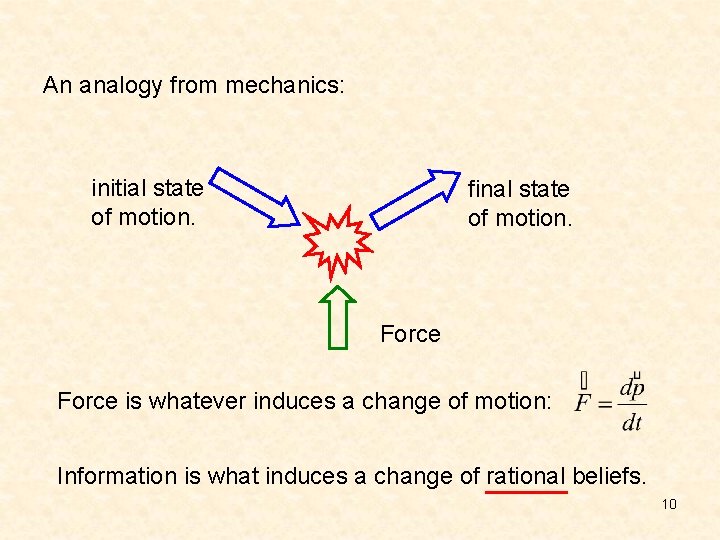
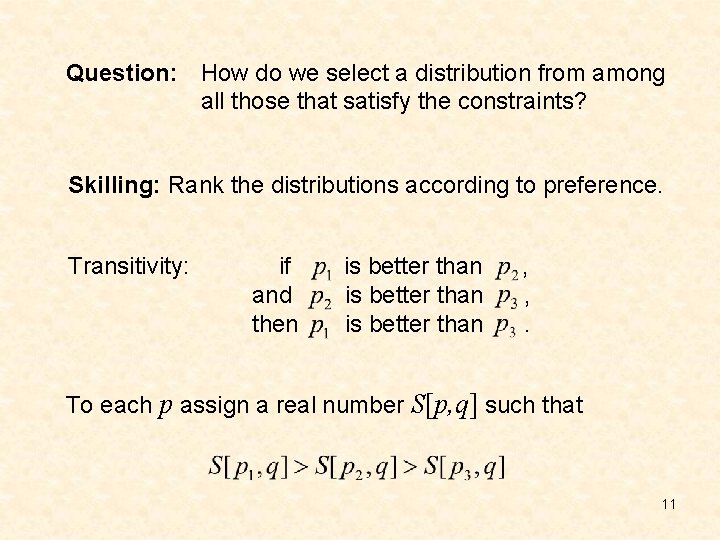
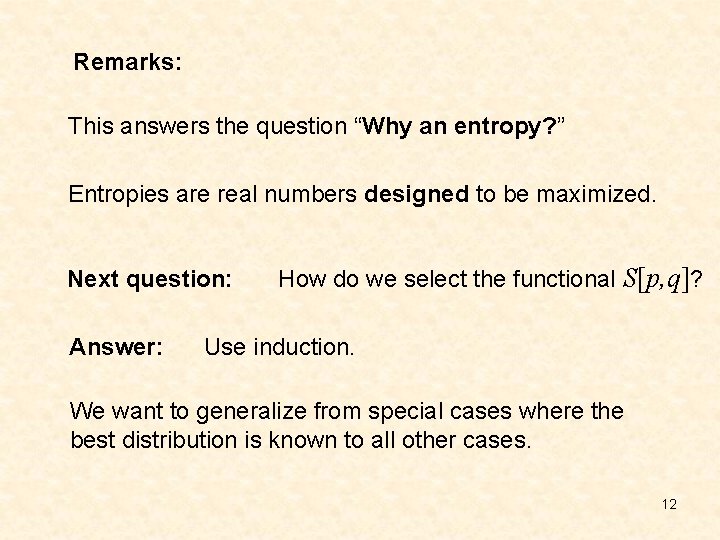
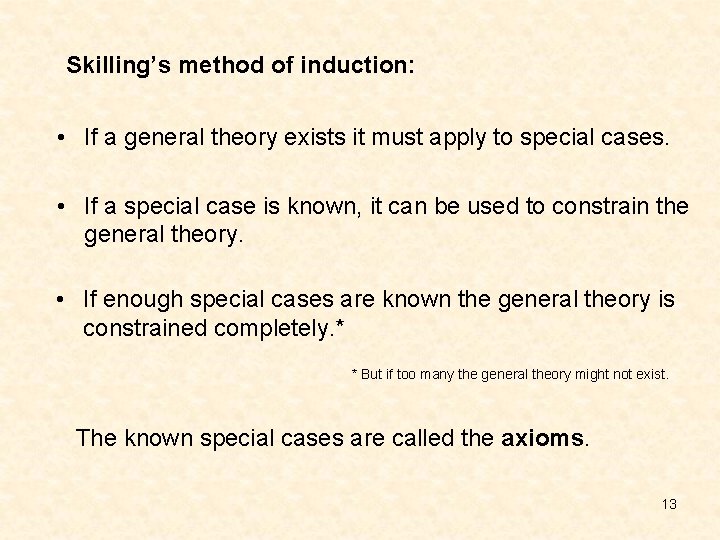
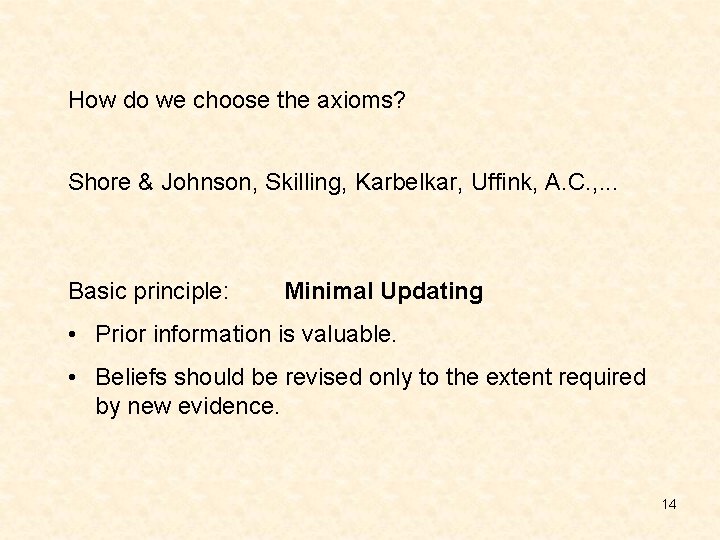
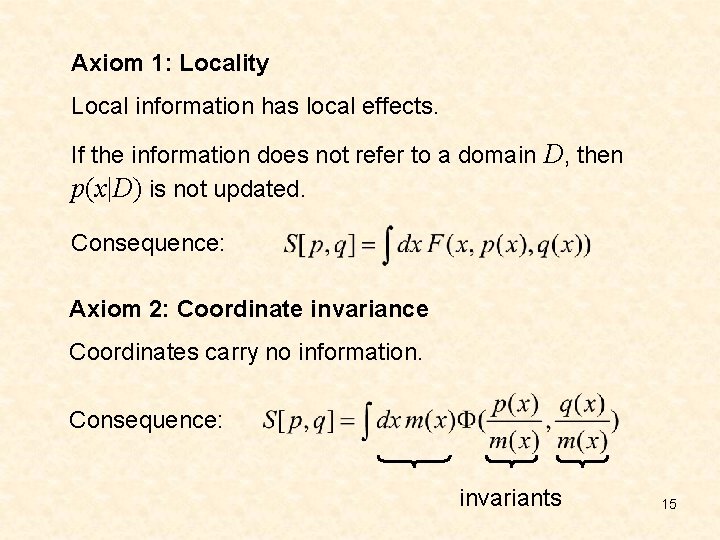
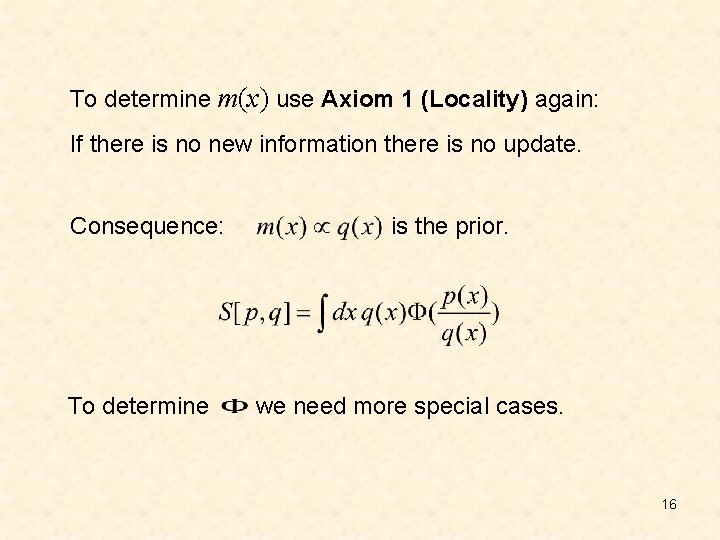
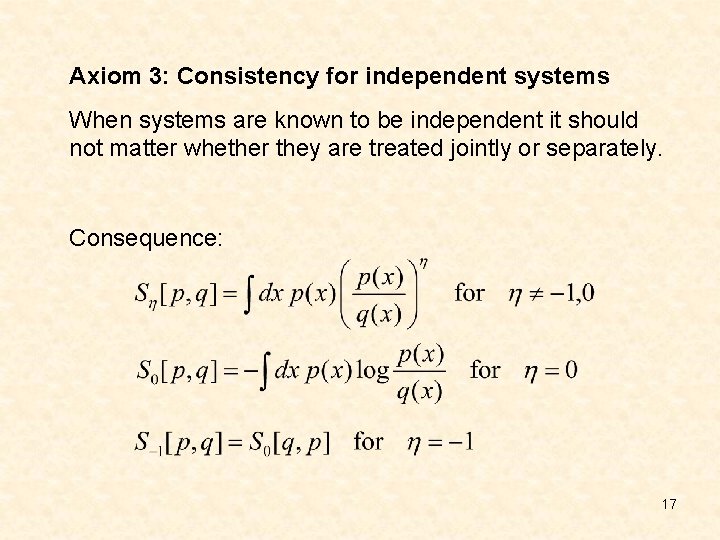
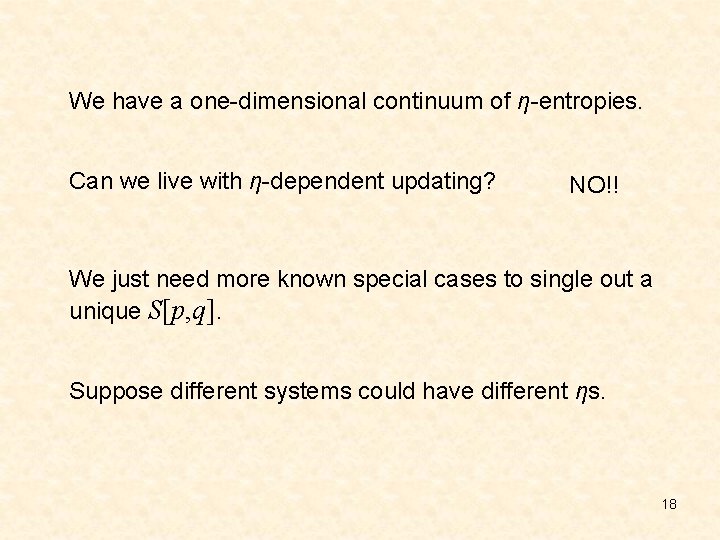
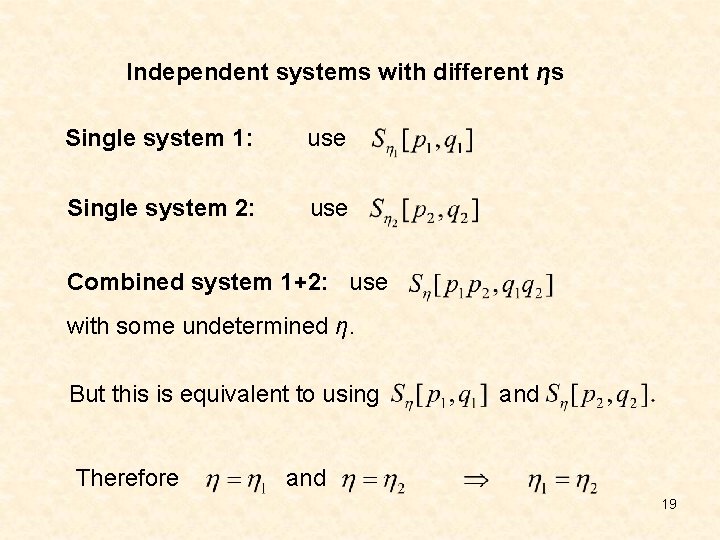
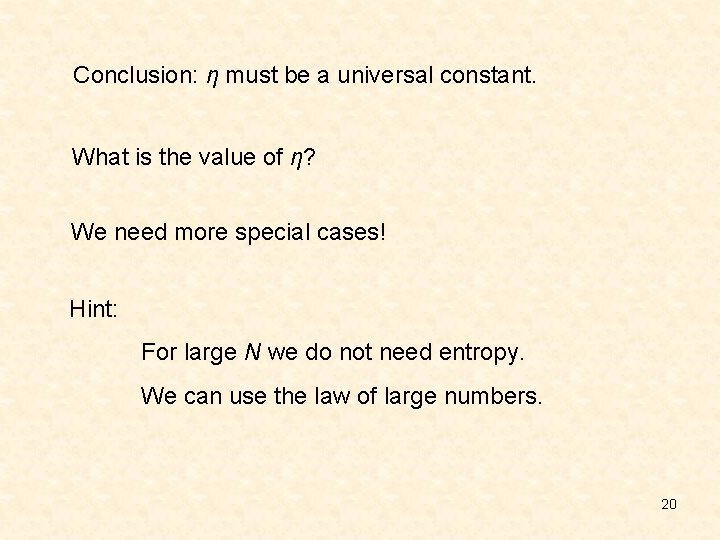
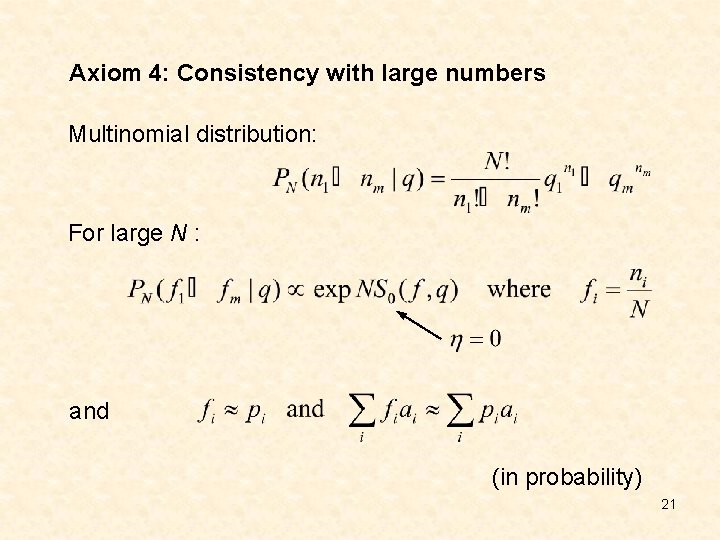
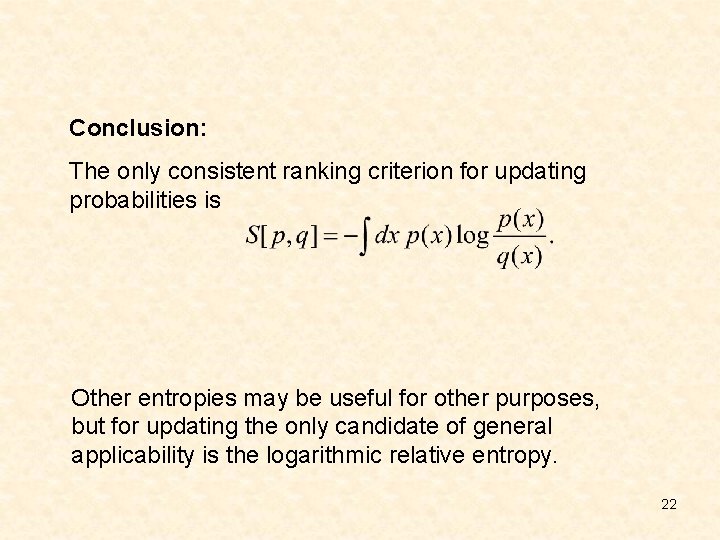
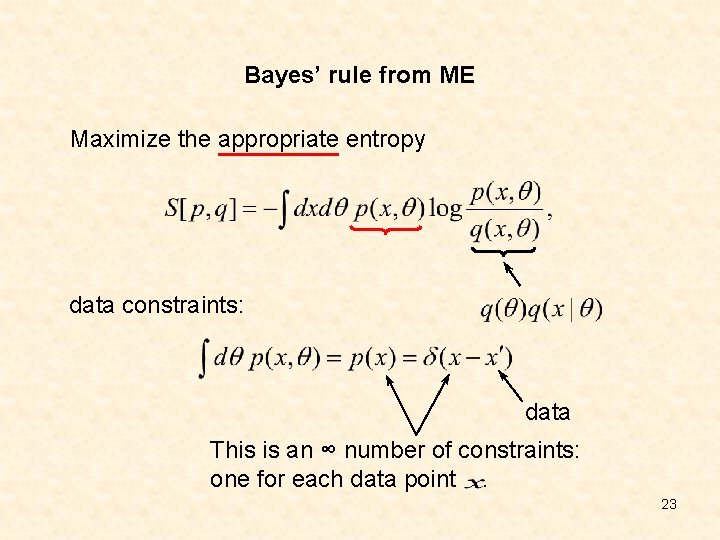
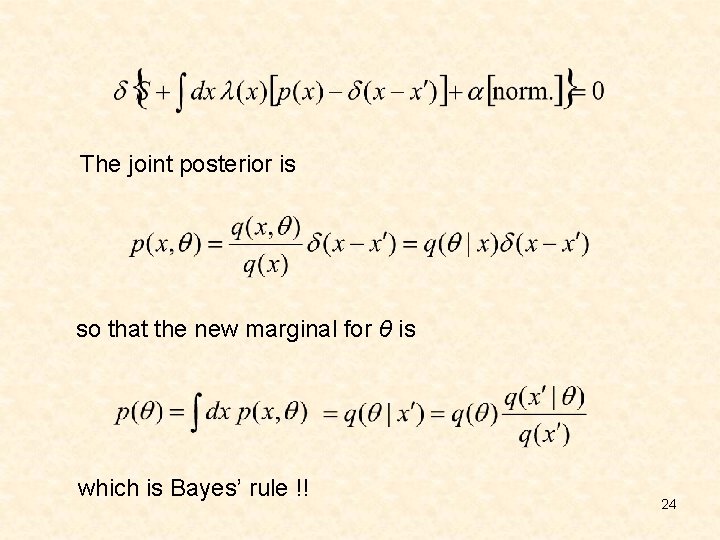
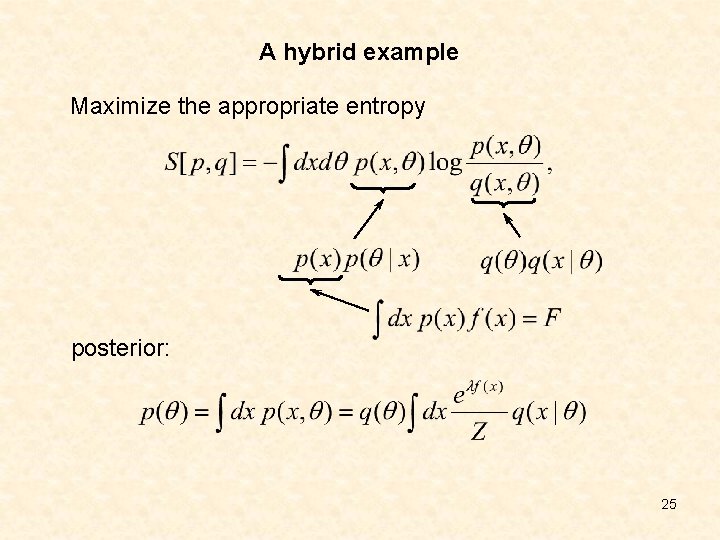
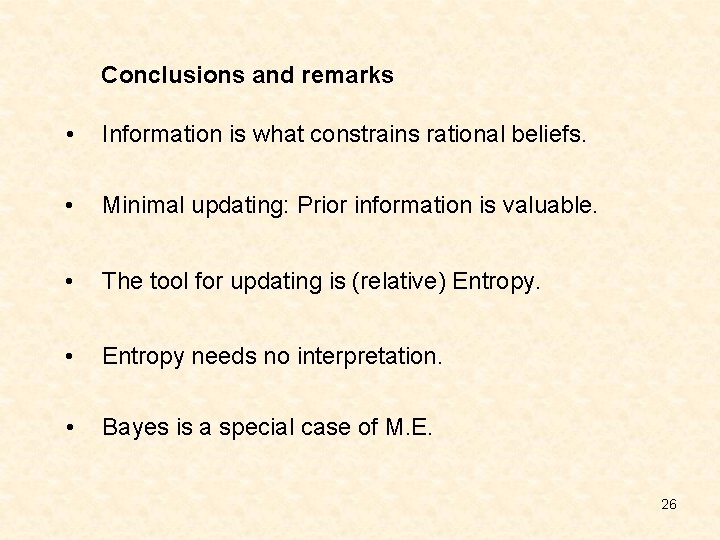
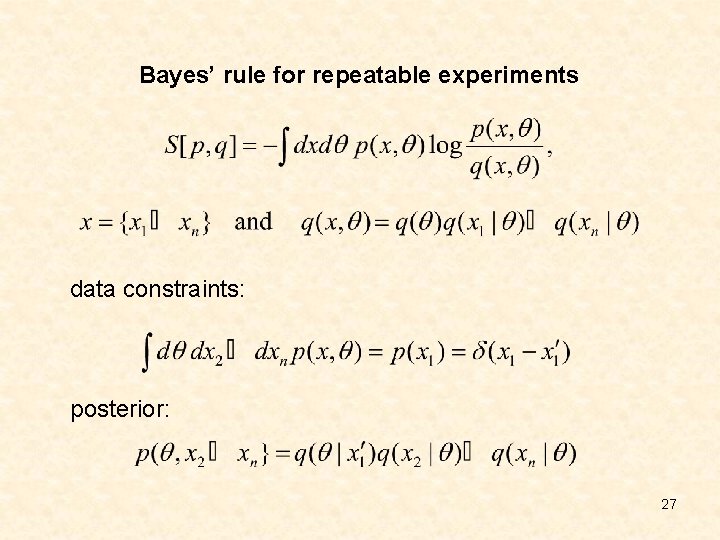
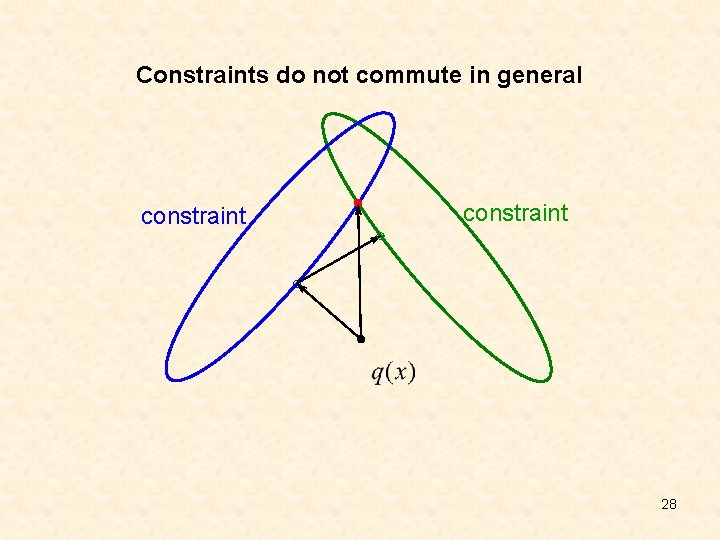
- Slides: 28
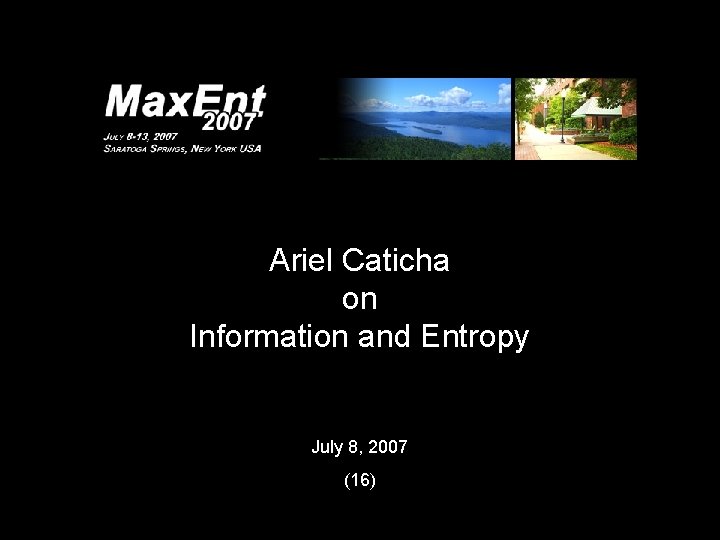
Ariel Caticha on Information and Entropy July 8, 2007 (16)
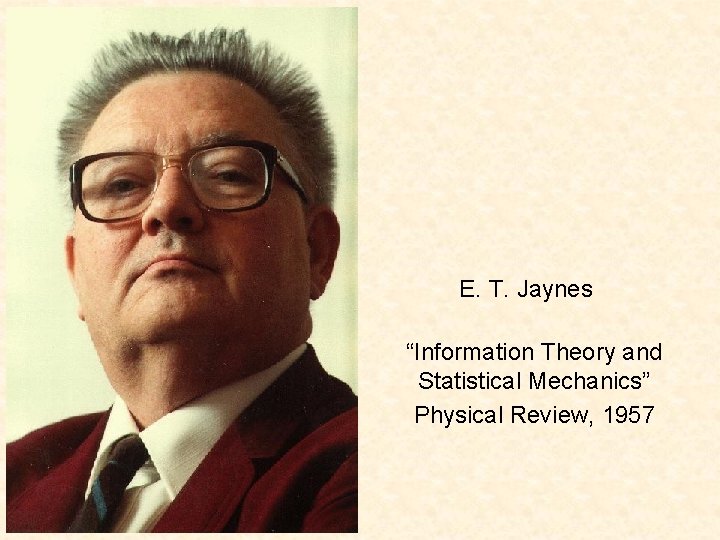
E. T. Jaynes “Information Theory and Statistical Mechanics” Physical Review, 1957
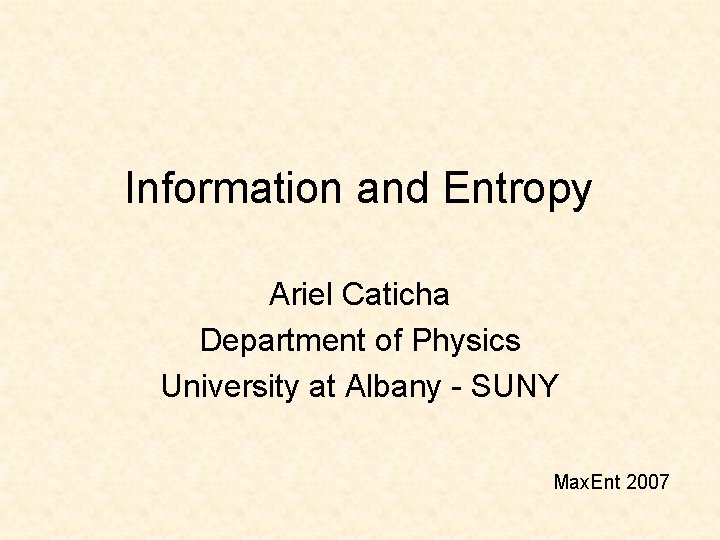
Information and Entropy Ariel Caticha Department of Physics University at Albany - SUNY Max. Ent 2007
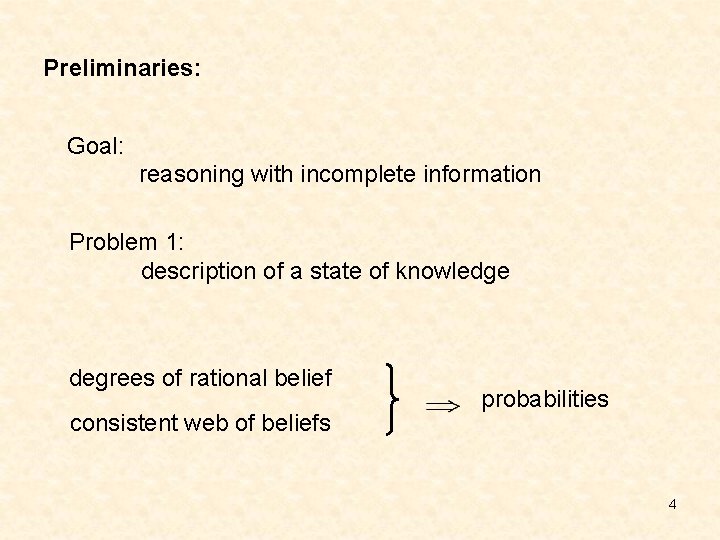
Preliminaries: Goal: reasoning with incomplete information Problem 1: description of a state of knowledge degrees of rational belief consistent web of beliefs probabilities 4
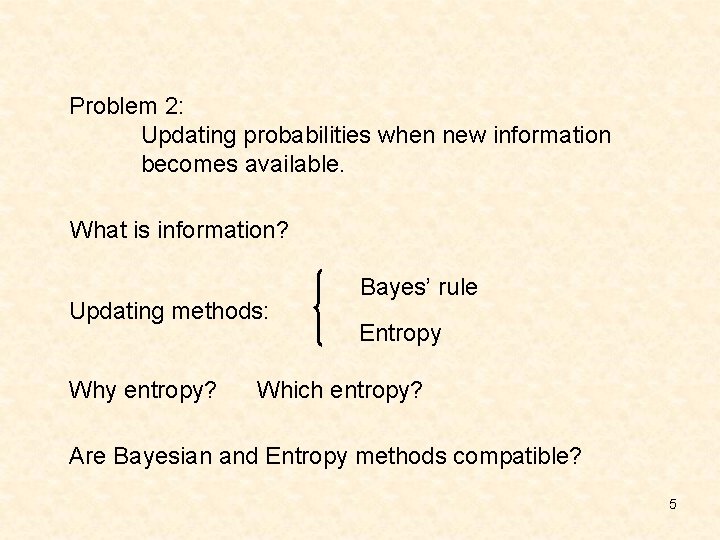
Problem 2: Updating probabilities when new information becomes available. What is information? Updating methods: Why entropy? Bayes’ rule Entropy Which entropy? Are Bayesian and Entropy methods compatible? 5
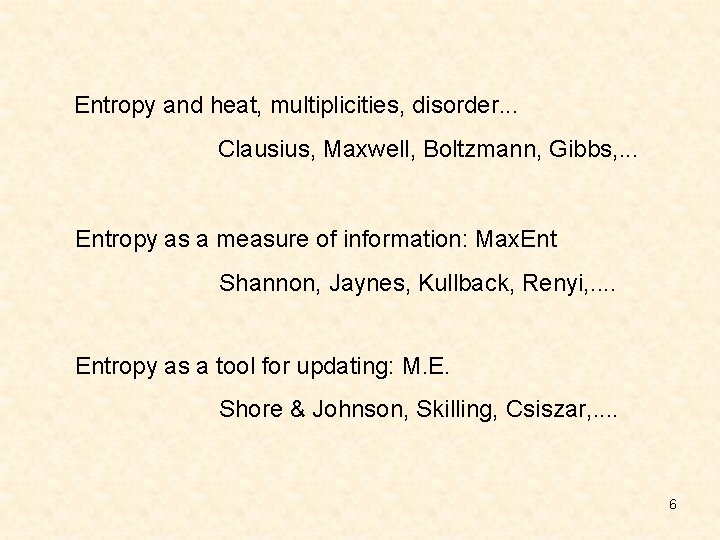
Entropy and heat, multiplicities, disorder. . . Clausius, Maxwell, Boltzmann, Gibbs, . . . Entropy as a measure of information: Max. Ent Shannon, Jaynes, Kullback, Renyi, . . Entropy as a tool for updating: M. E. Shore & Johnson, Skilling, Csiszar, . . 6
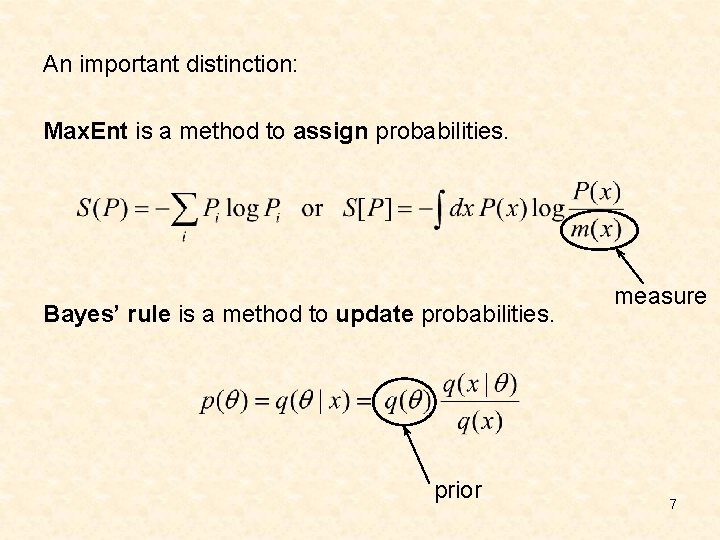
An important distinction: Max. Ent is a method to assign probabilities. Bayes’ rule is a method to update probabilities. prior measure 7
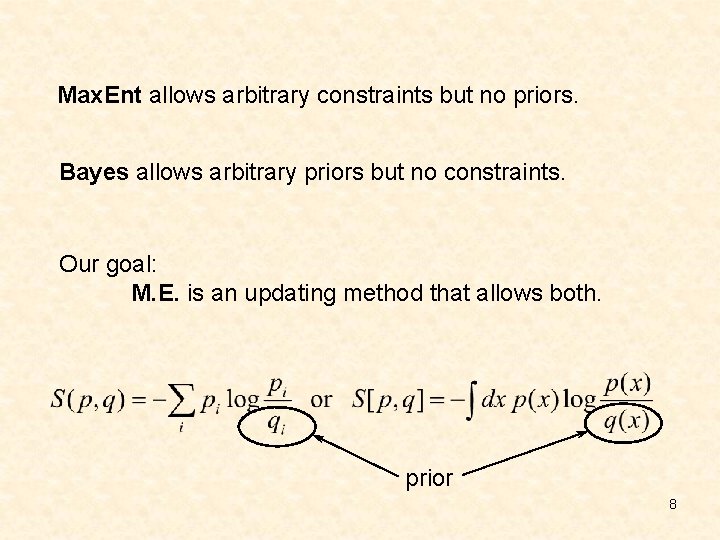
Max. Ent allows arbitrary constraints but no priors. Bayes allows arbitrary priors but no constraints. Our goal: M. E. is an updating method that allows both. prior 8
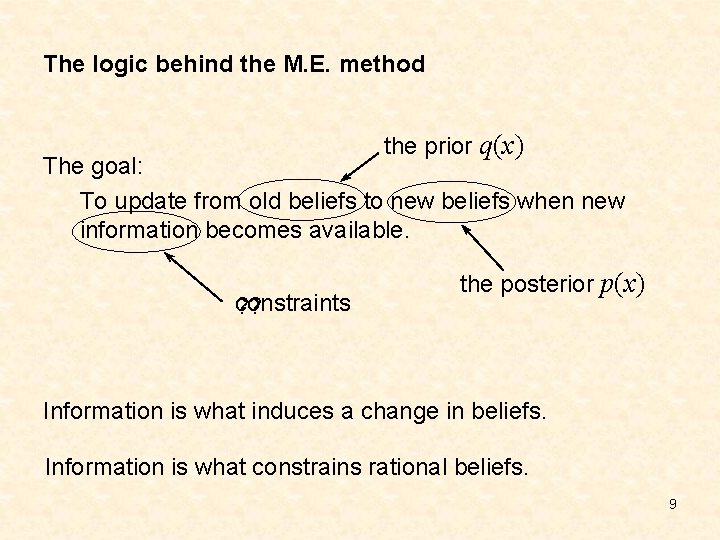
The logic behind the M. E. method the prior q(x) The goal: To update from old beliefs to new beliefs when new information becomes available. constraints ? ? the posterior p(x) Information is what induces a change in beliefs. Information is what constrains rational beliefs. 9
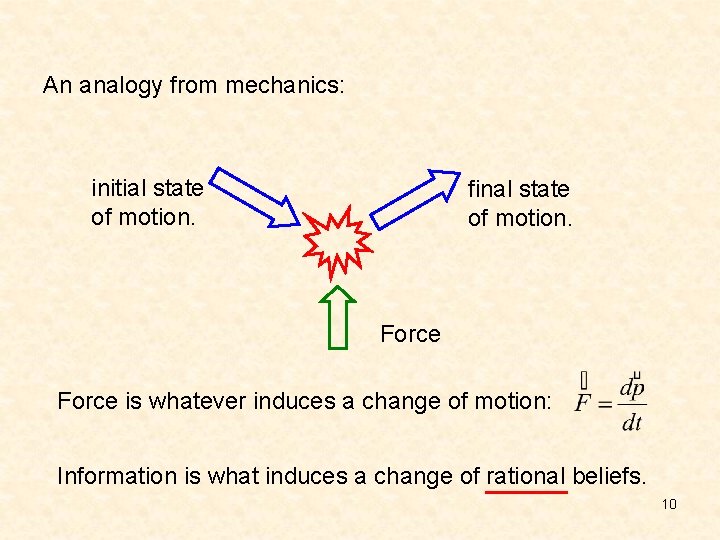
An analogy from mechanics: initial state of motion. final state of motion. Force is whatever induces a change of motion: Information is what induces a change of rational beliefs. 10
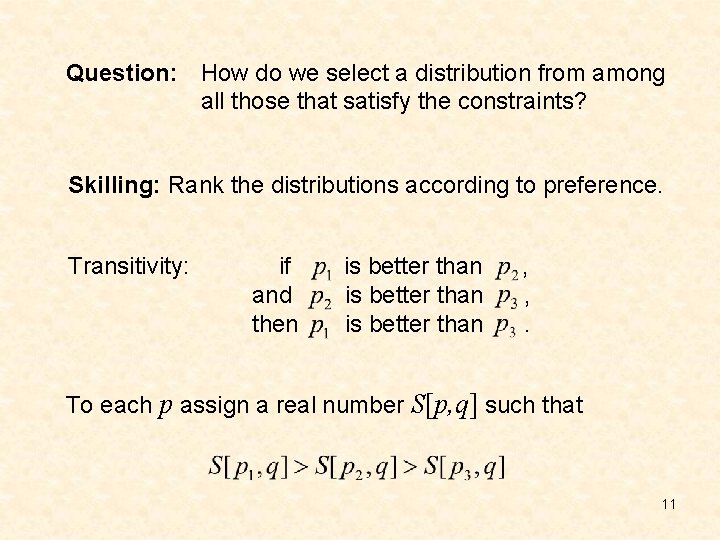
Question: How do we select a distribution from among all those that satisfy the constraints? Skilling: Rank the distributions according to preference. Transitivity: if and then is better than , , . To each p assign a real number S[p, q] such that 11
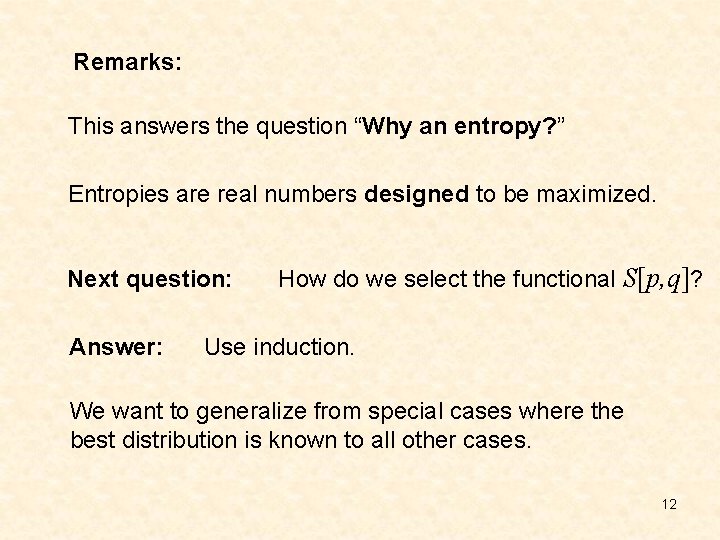
Remarks: This answers the question “Why an entropy? ” Entropies are real numbers designed to be maximized. Next question: Answer: How do we select the functional S[p, q]? Use induction. We want to generalize from special cases where the best distribution is known to all other cases. 12
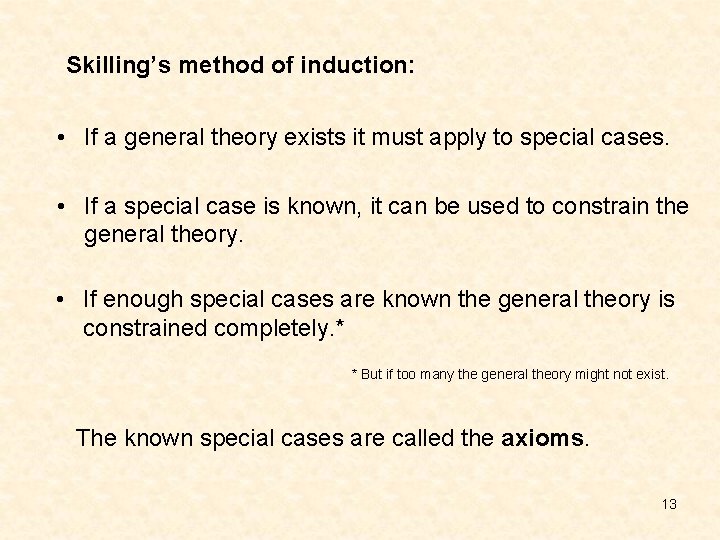
Skilling’s method of induction: • If a general theory exists it must apply to special cases. • If a special case is known, it can be used to constrain the general theory. • If enough special cases are known the general theory is constrained completely. * * But if too many the general theory might not exist. The known special cases are called the axioms. 13
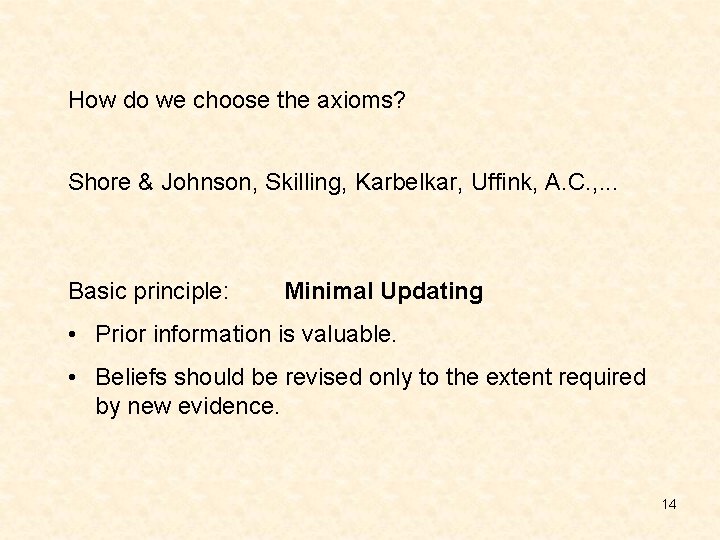
How do we choose the axioms? Shore & Johnson, Skilling, Karbelkar, Uffink, A. C. , . . . Basic principle: Minimal Updating • Prior information is valuable. • Beliefs should be revised only to the extent required by new evidence. 14
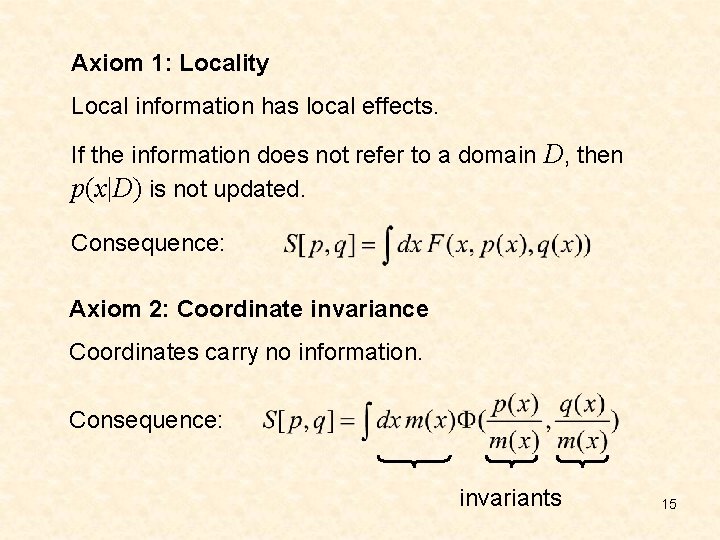
Axiom 1: Locality Local information has local effects. If the information does not refer to a domain D, then p(x|D) is not updated. Consequence: Axiom 2: Coordinate invariance Coordinates carry no information. Consequence: invariants 15
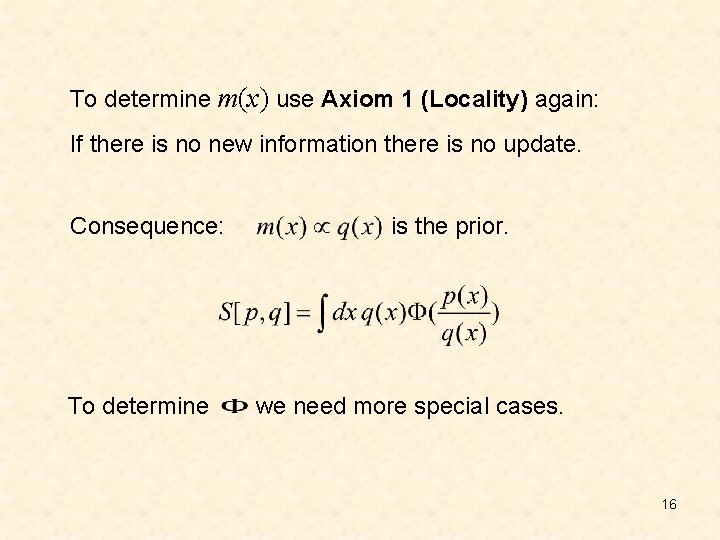
To determine m(x) use Axiom 1 (Locality) again: If there is no new information there is no update. Consequence: To determine is the prior. we need more special cases. 16
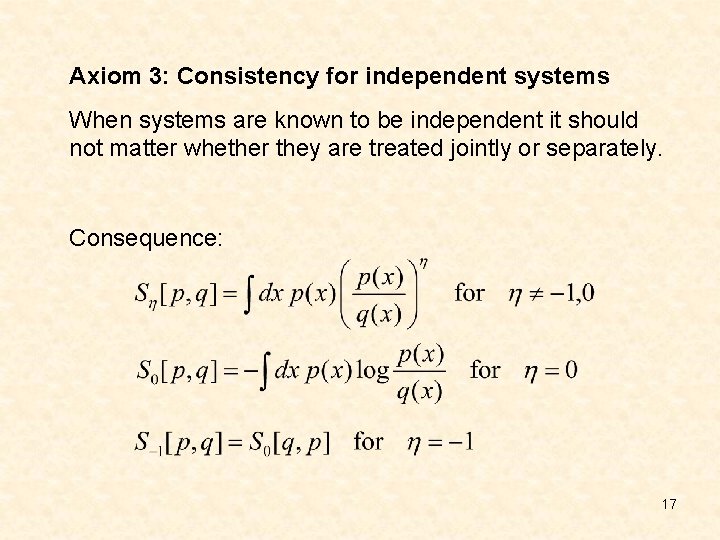
Axiom 3: Consistency for independent systems When systems are known to be independent it should not matter whether they are treated jointly or separately. Consequence: 17
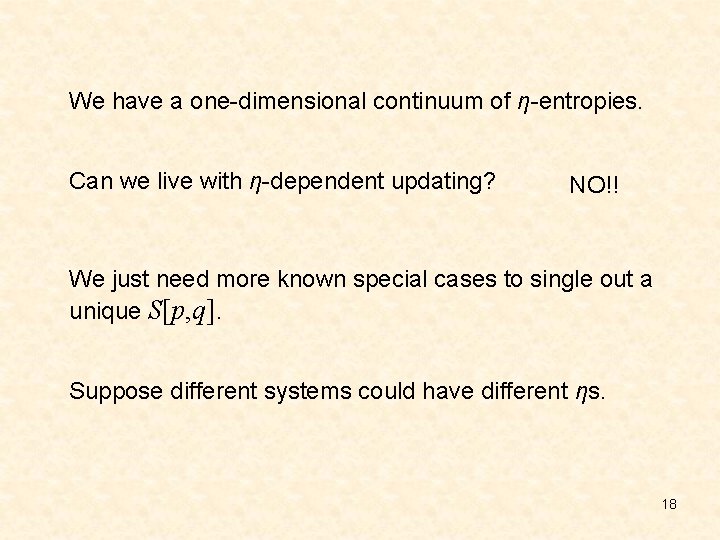
We have a one-dimensional continuum of η-entropies. Can we live with η-dependent updating? NO!! We just need more known special cases to single out a unique S[p, q]. Suppose different systems could have different ηs. 18
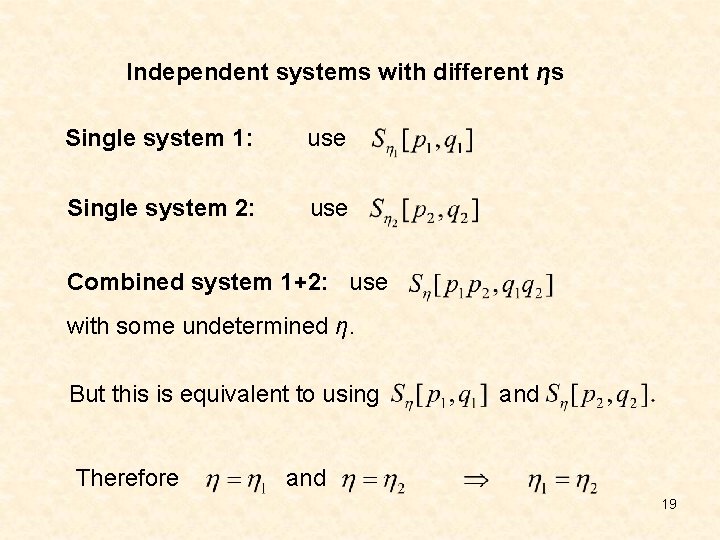
Independent systems with different ηs Single system 1: use Single system 2: use Combined system 1+2: use with some undetermined η. But this is equivalent to using Therefore and 19
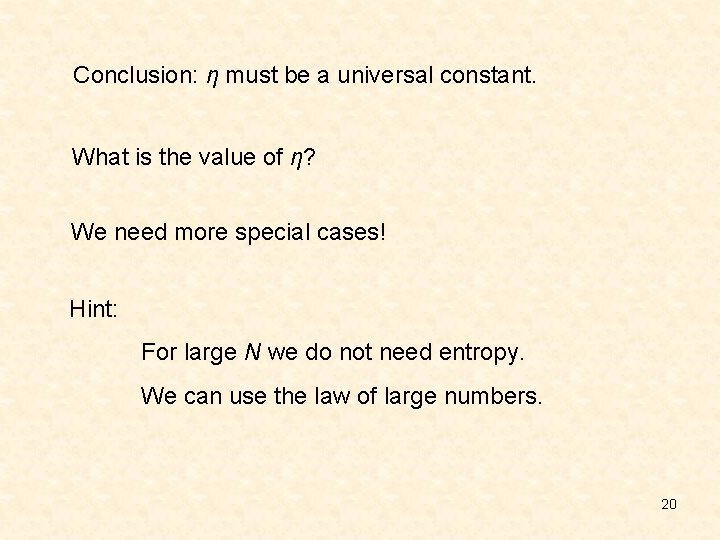
Conclusion: η must be a universal constant. What is the value of η? We need more special cases! Hint: For large N we do not need entropy. We can use the law of large numbers. 20
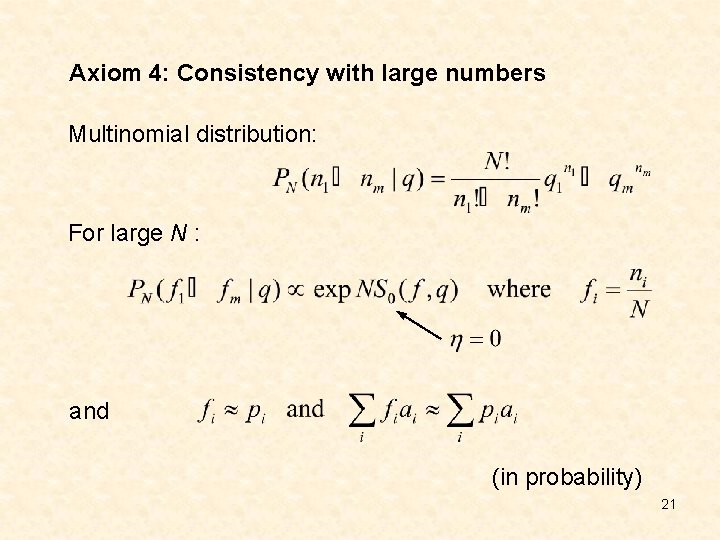
Axiom 4: Consistency with large numbers Multinomial distribution: For large N : and (in probability) 21
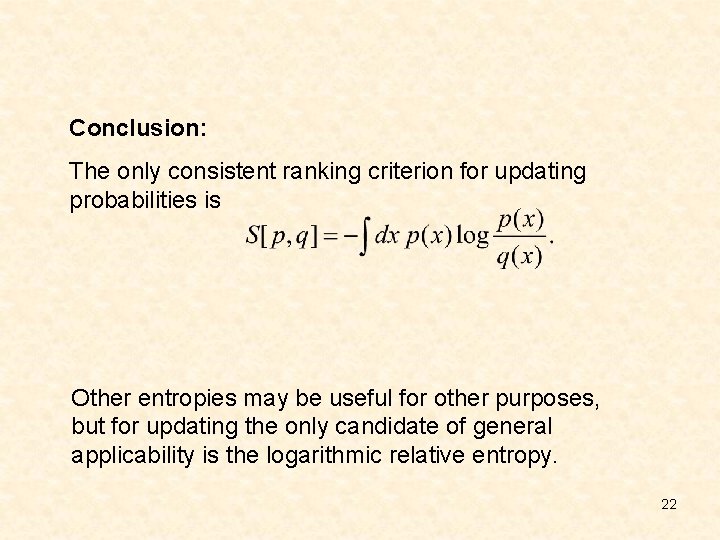
Conclusion: The only consistent ranking criterion for updating probabilities is Other entropies may be useful for other purposes, but for updating the only candidate of general applicability is the logarithmic relative entropy. 22
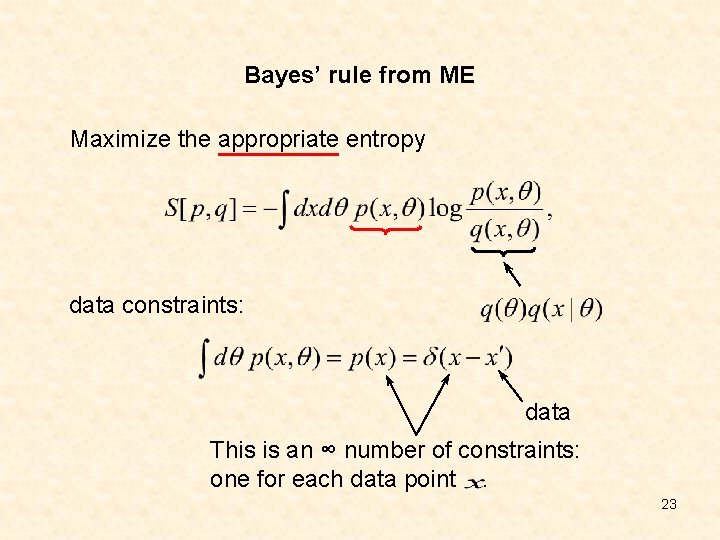
Bayes’ rule from ME Maximize the appropriate entropy data constraints: data This is an ∞ number of constraints: one for each data point. 23
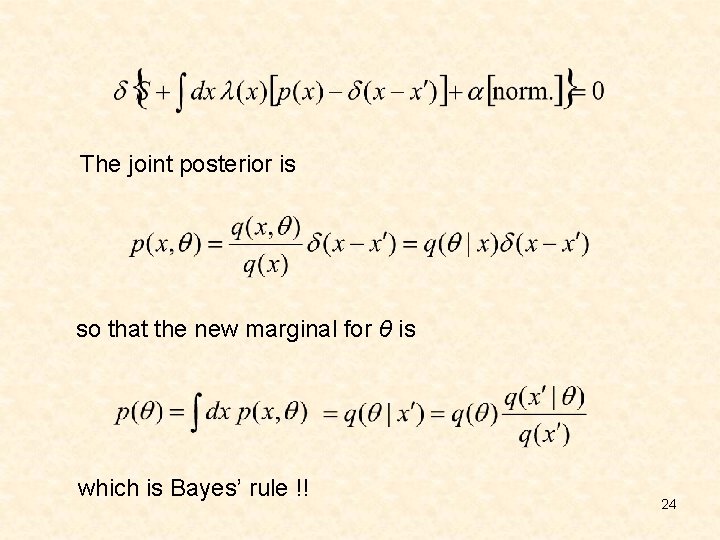
The joint posterior is so that the new marginal for θ is which is Bayes’ rule !! 24
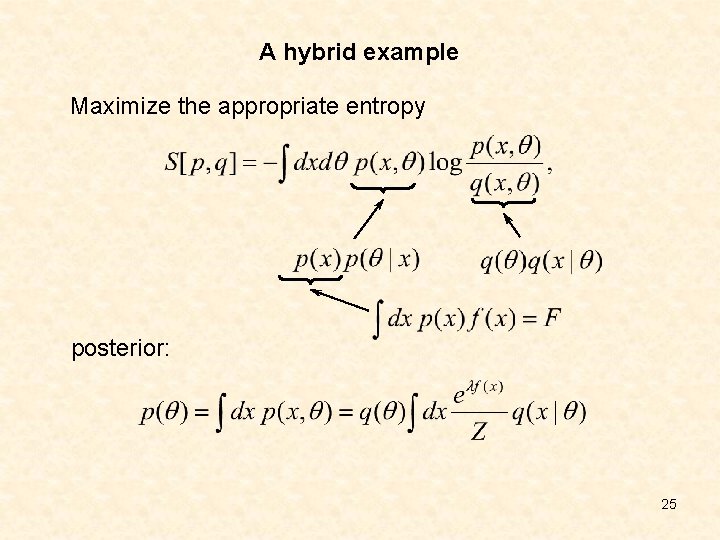
A hybrid example Maximize the appropriate entropy posterior: 25
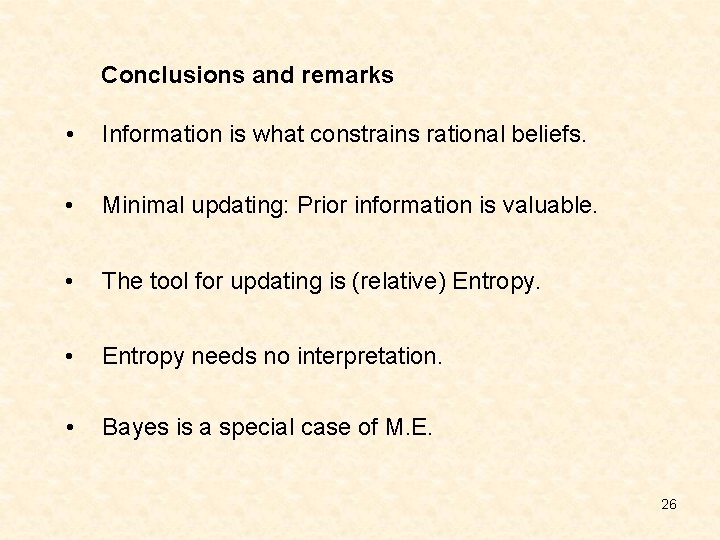
Conclusions and remarks • Information is what constrains rational beliefs. • Minimal updating: Prior information is valuable. • The tool for updating is (relative) Entropy. • Entropy needs no interpretation. • Bayes is a special case of M. E. 26
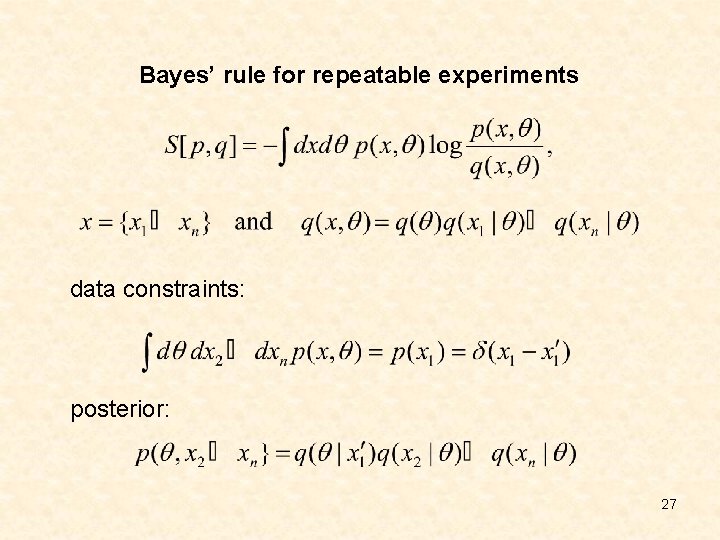
Bayes’ rule for repeatable experiments data constraints: posterior: 27
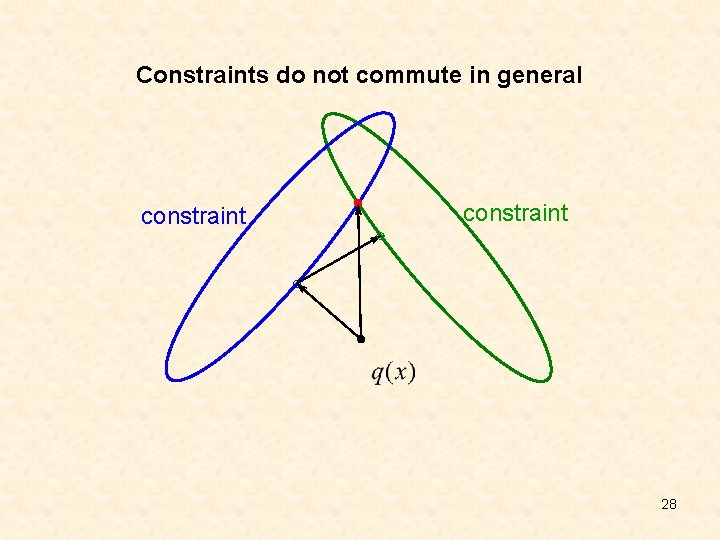
Constraints do not commute in general constraint 28
Ariel caticha
Entropy in information theory
April may june july
Bag of little bootstraps
Carlos sarraute
Sharon
Dr ariel stein
Ariel schwartzman
Ariel carroll
Scienze internazionali e istituzioni europee unimi
Ariel castro background
Itay ariel
Caliban ariel
Fernando ariel martinez mendoza
Qué significa ariel
Rusalka ariel
Ciclul de viata al produsului ariel
Itay ariel
Qué significa ariel
Healthcare sector analysis
Ariel sharom
Arielle charette
Ariel przybyłowicz
Ariel y eric en el lago
Ariel 1928
Ariel velazquez
Ariel
Ariel eizenberg
Ariel felner