Systematical veto analysis using all monitor signals Koji
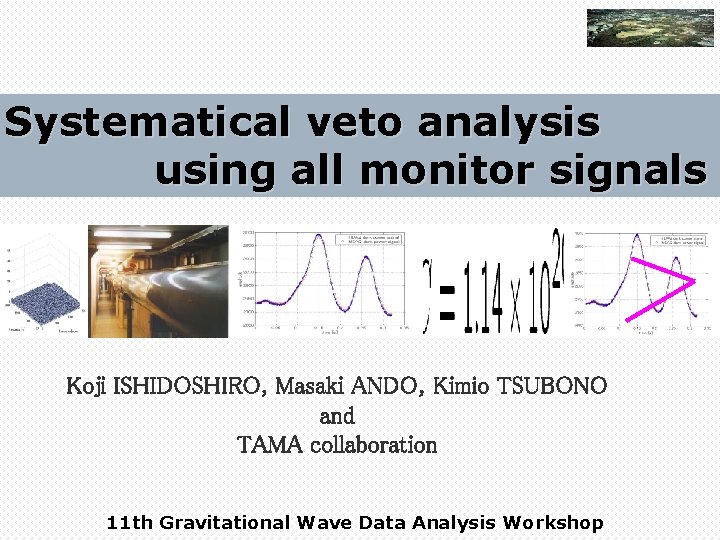
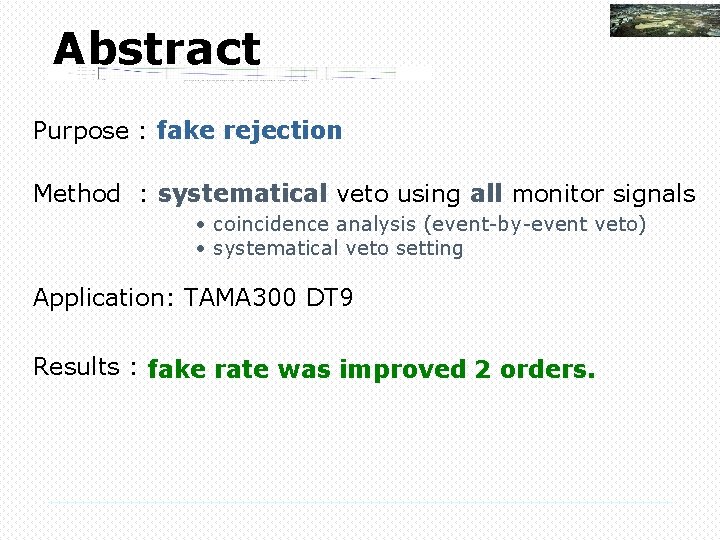
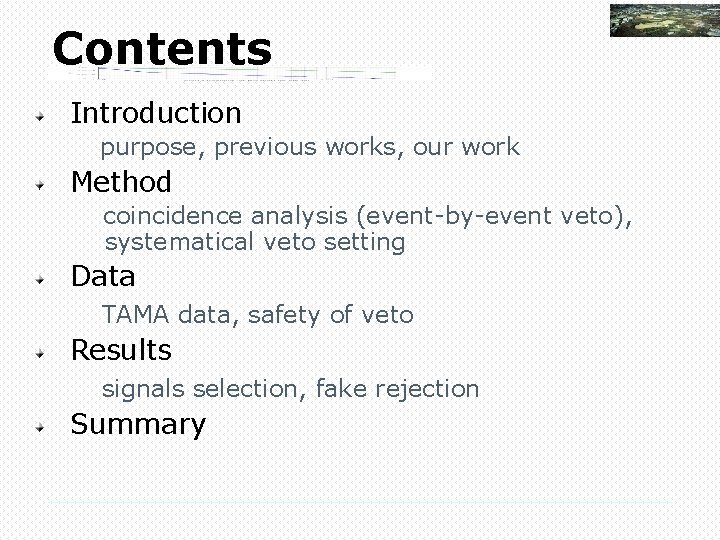
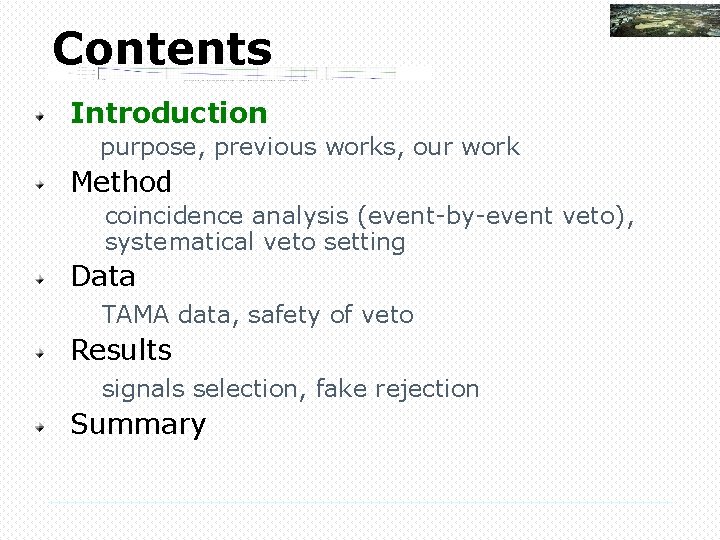
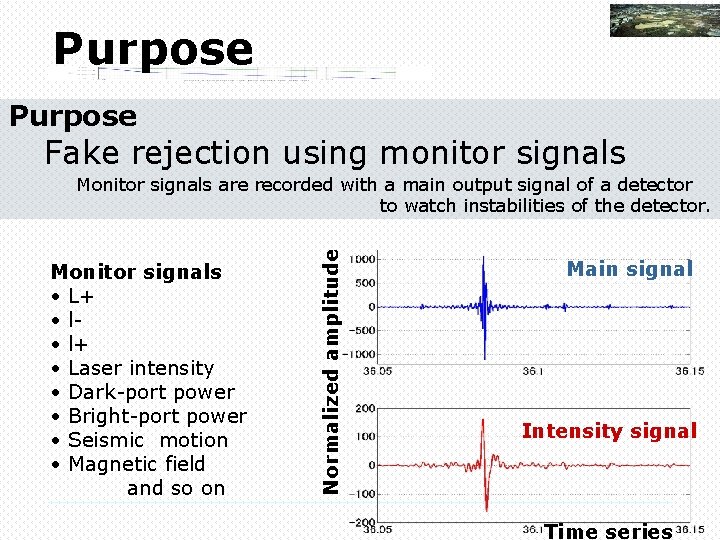
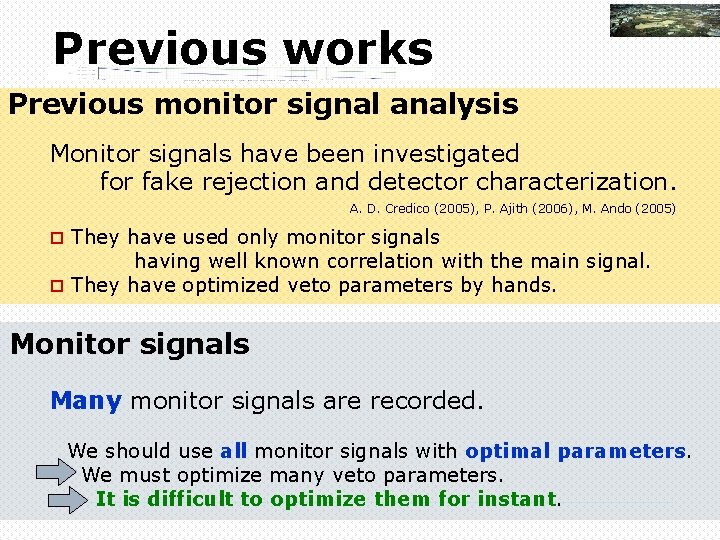
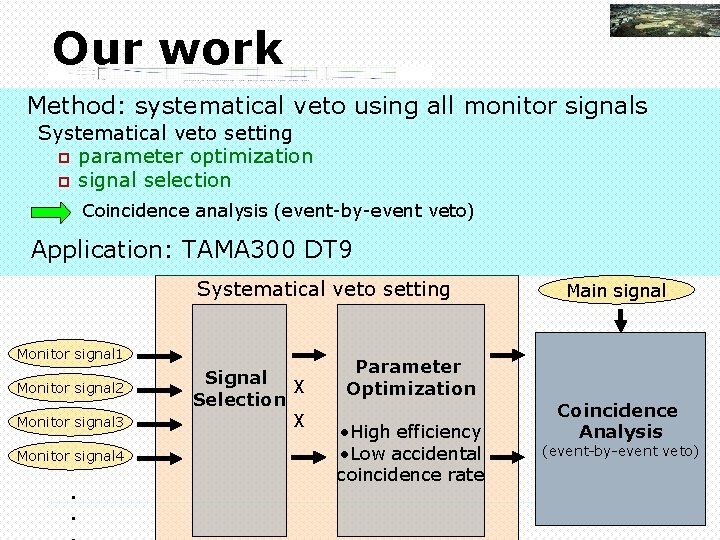
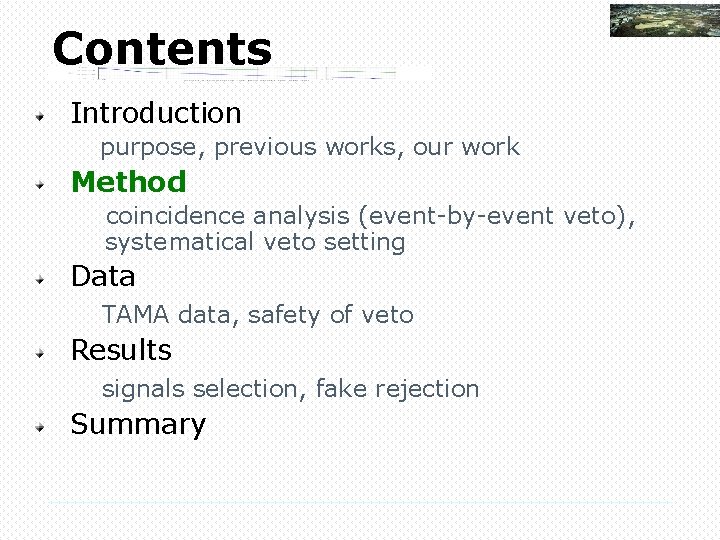
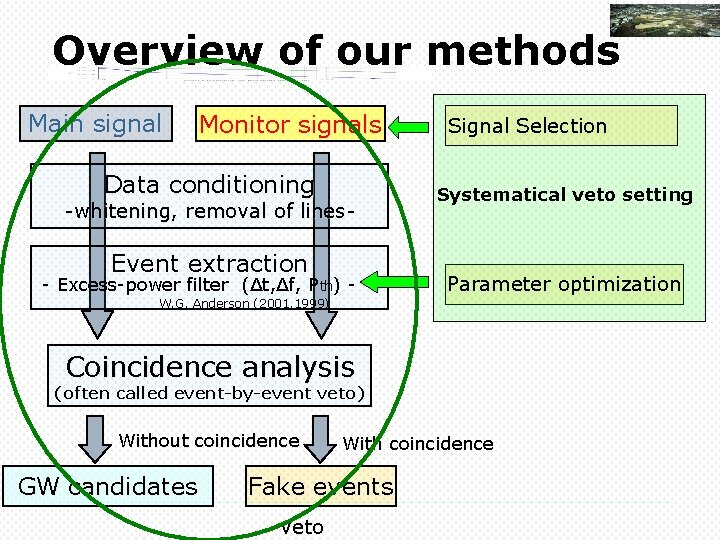
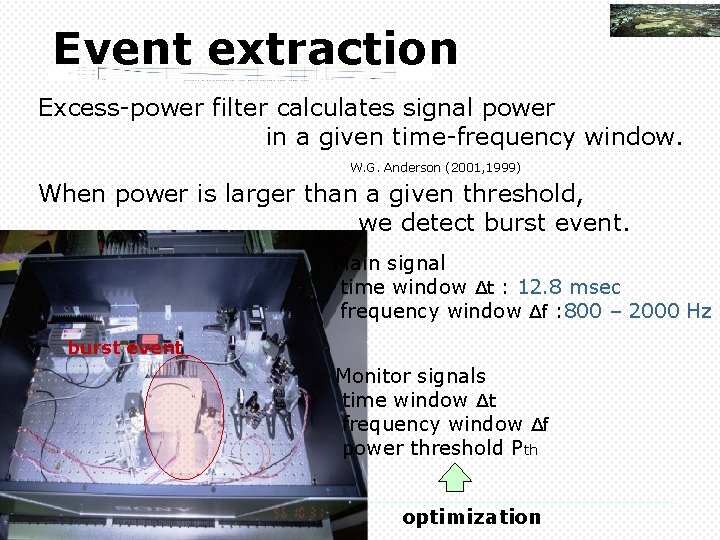
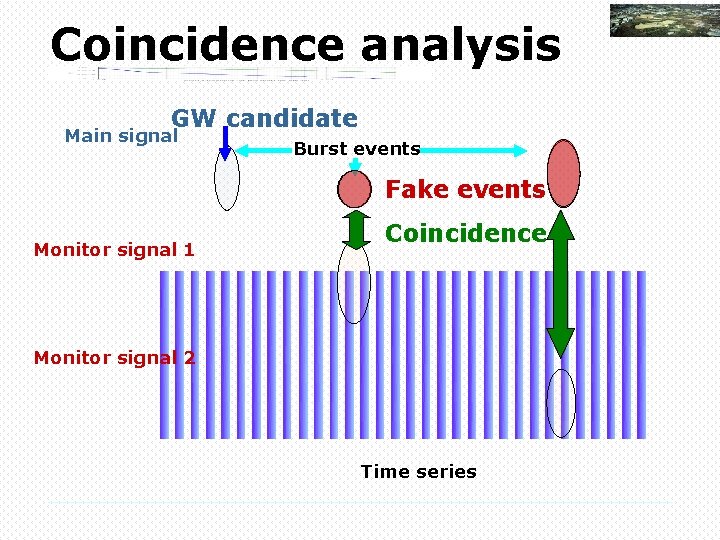
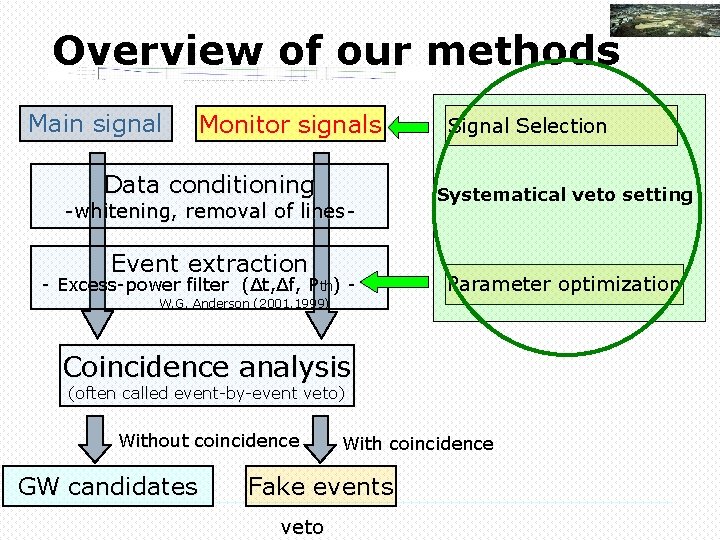
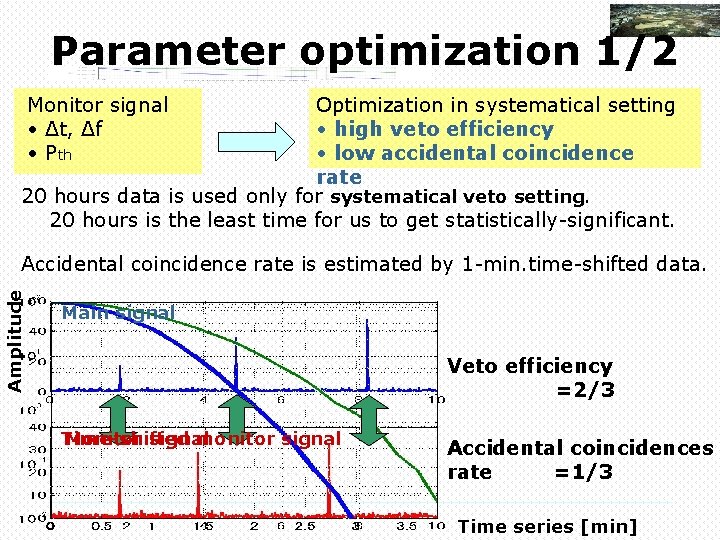
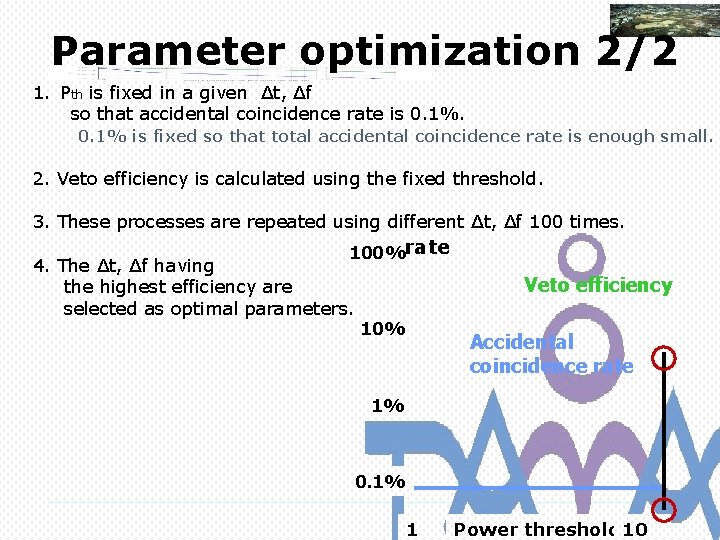
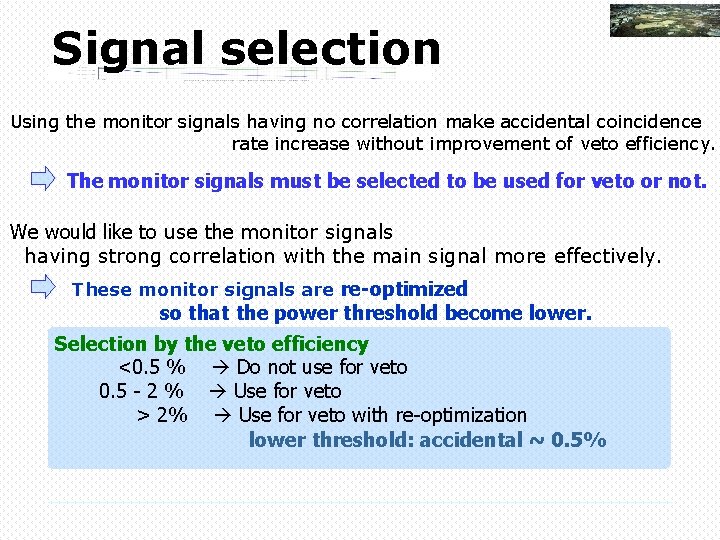
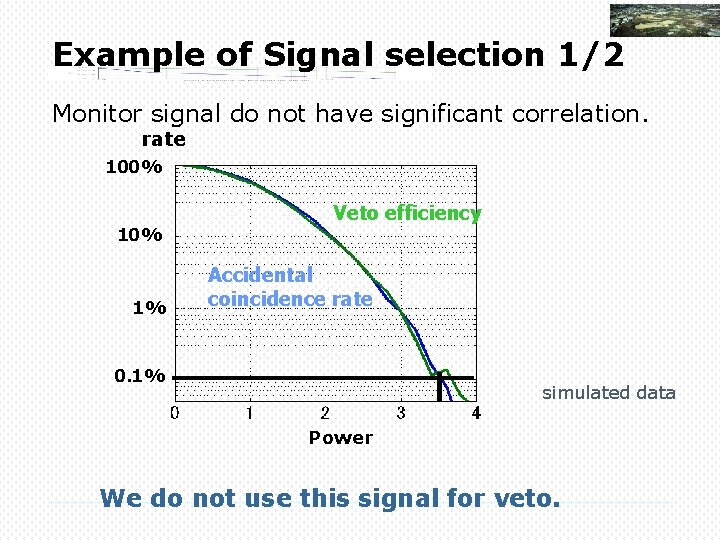
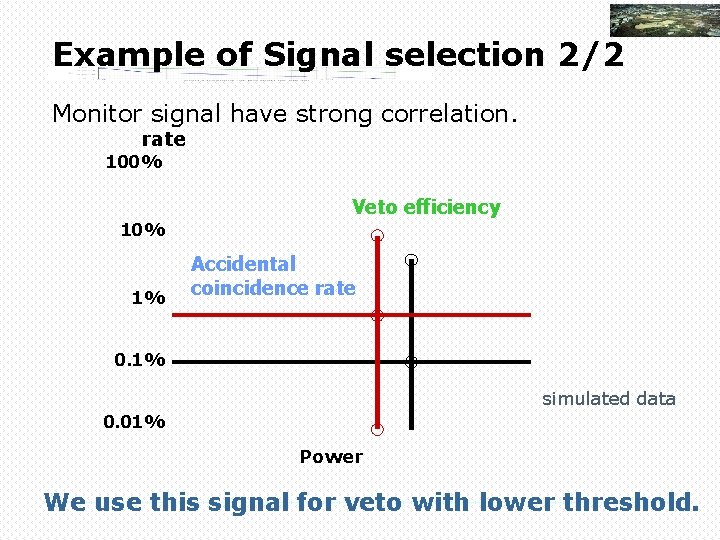
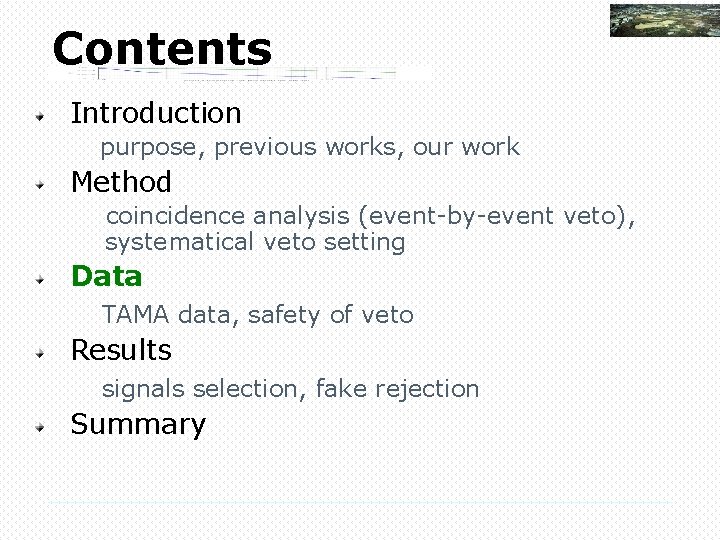
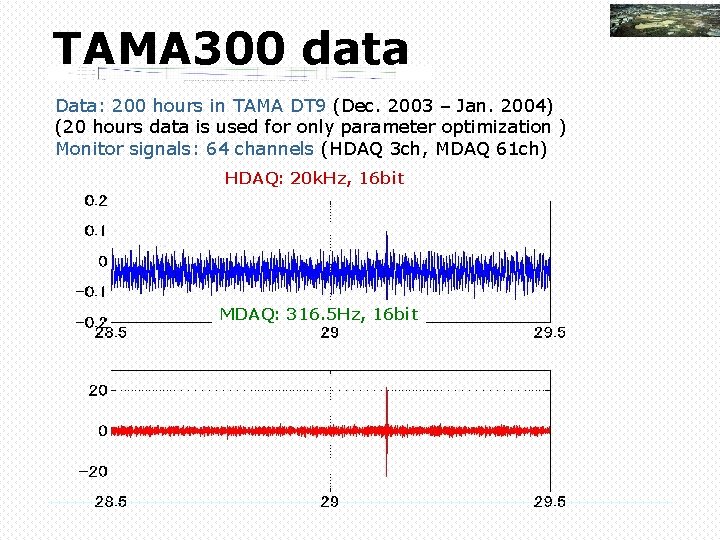
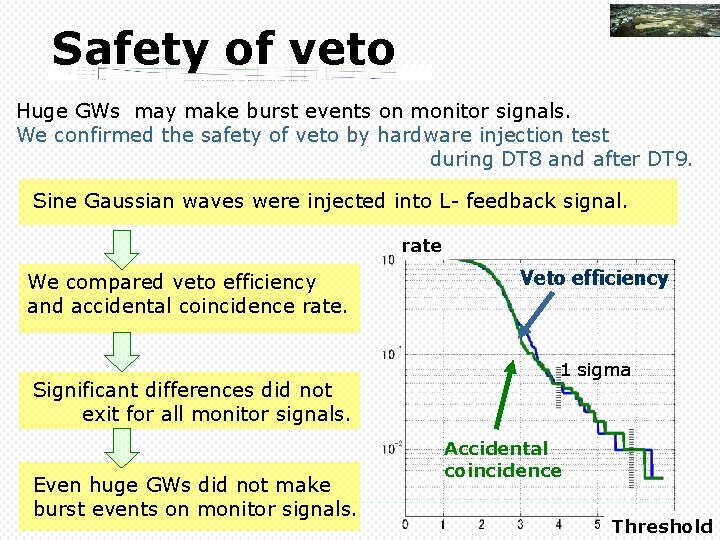
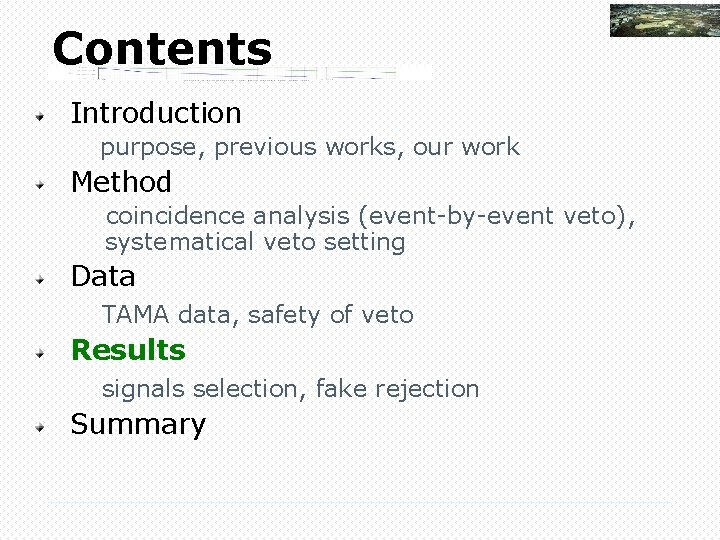
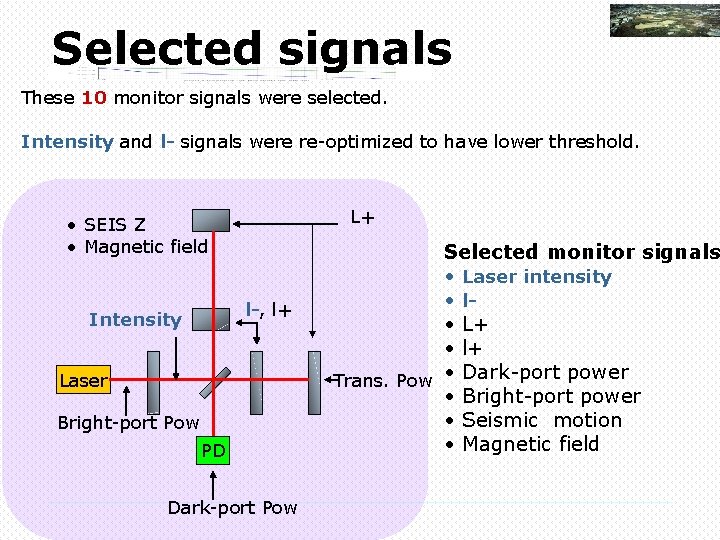
![Fake rate [Hz] Fake rate was improved 2 orders @ hrss = 10 -18. Fake rate [Hz] Fake rate was improved 2 orders @ hrss = 10 -18.](https://slidetodoc.com/presentation_image/7dd79cd221c55354e69529c8bed8ddcb/image-23.jpg)
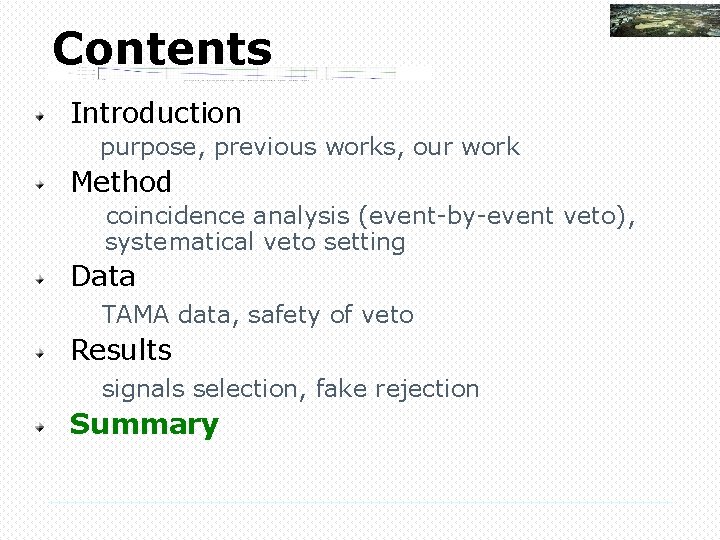
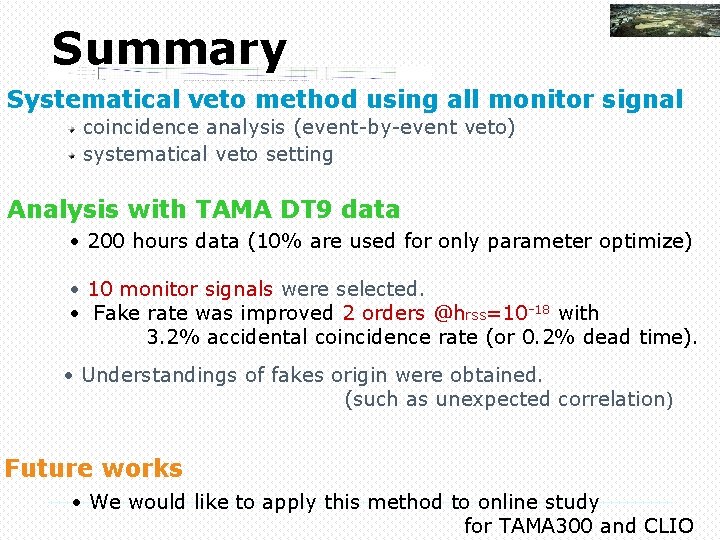
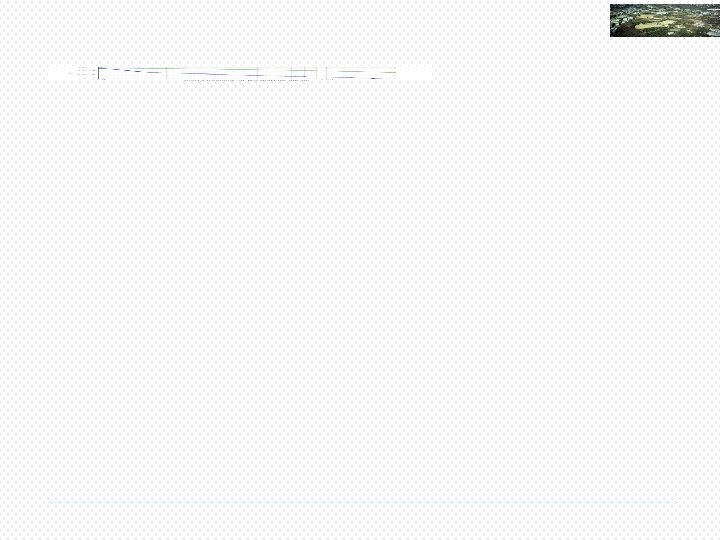
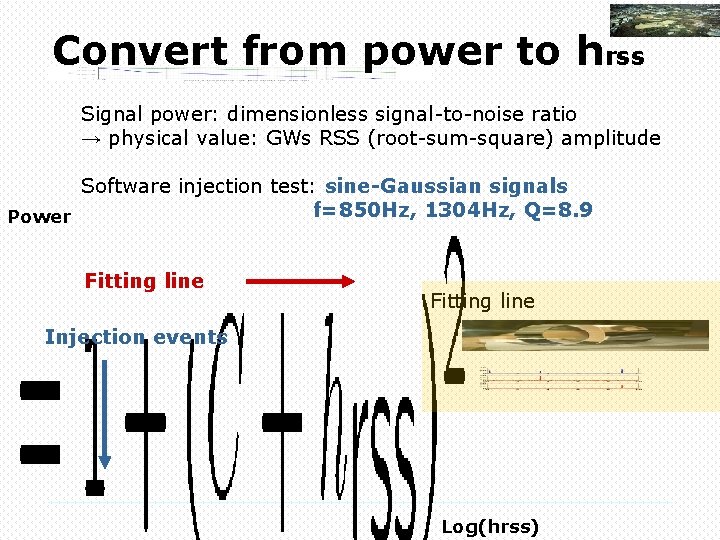
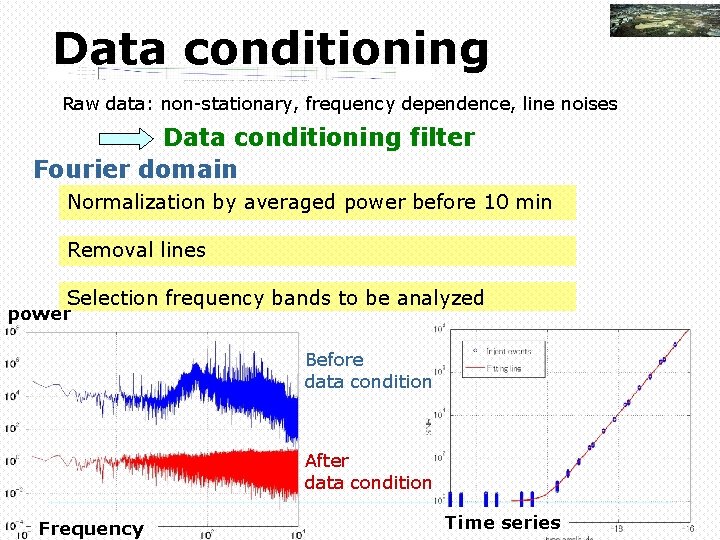
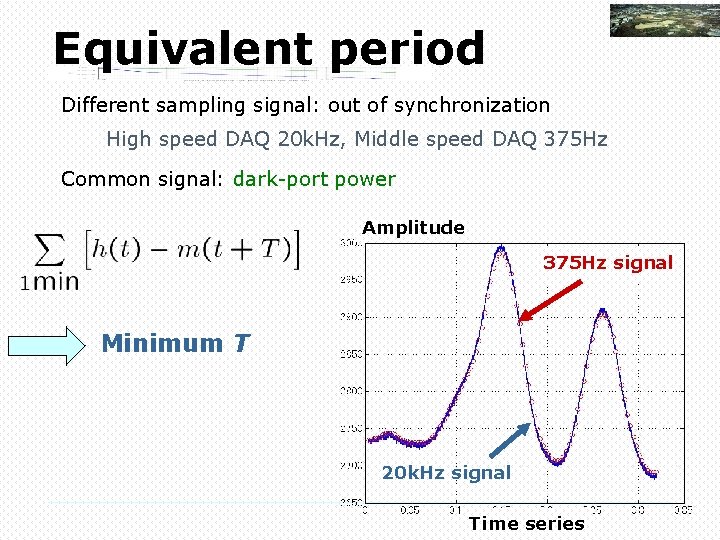
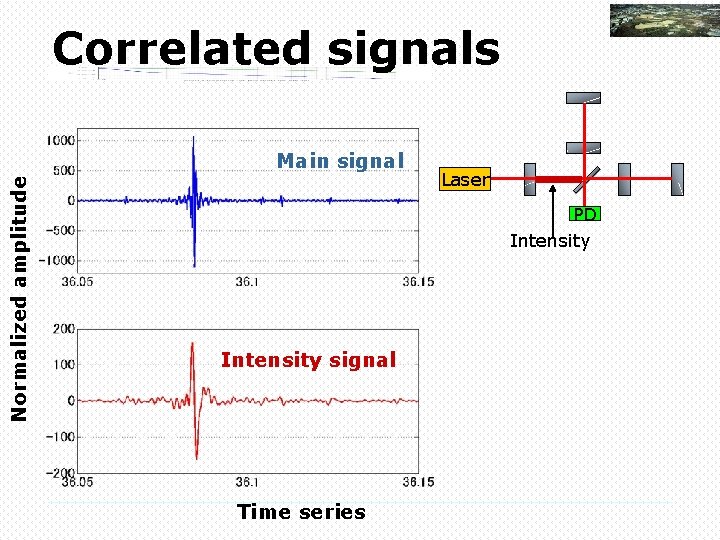
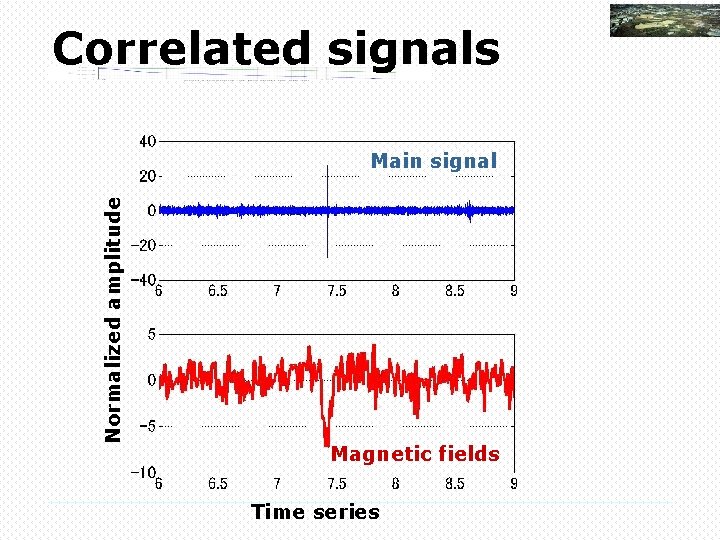
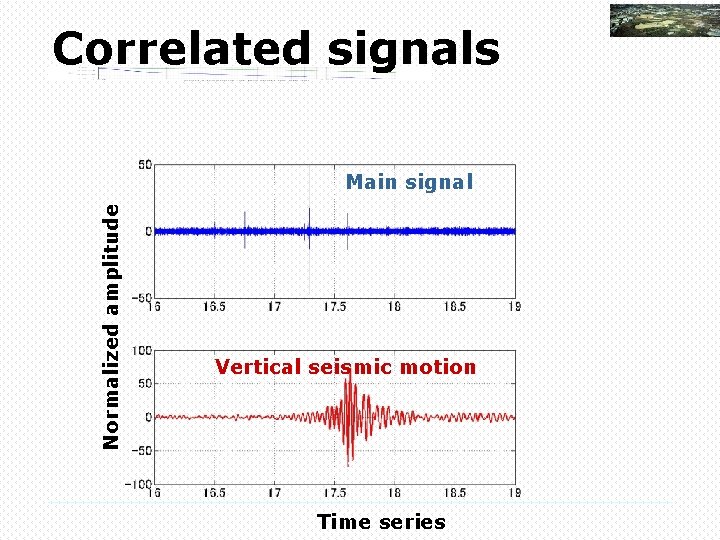
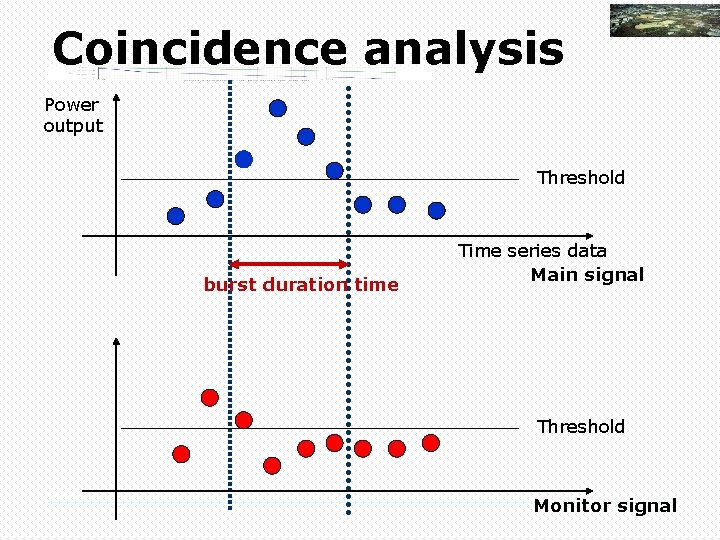
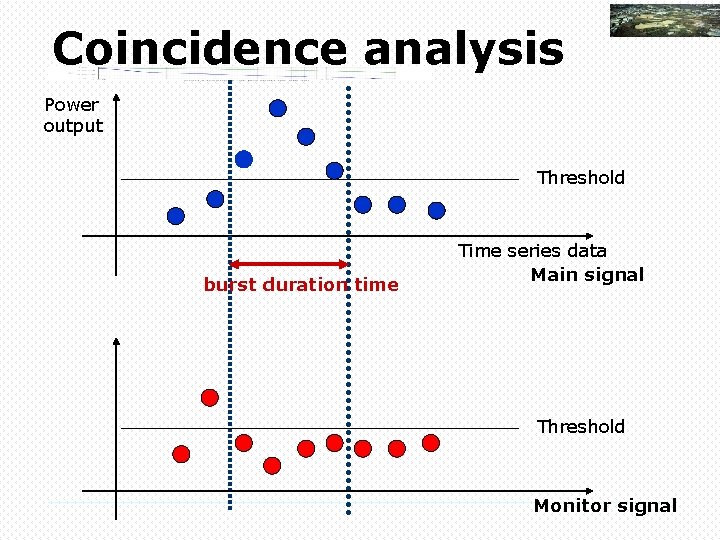
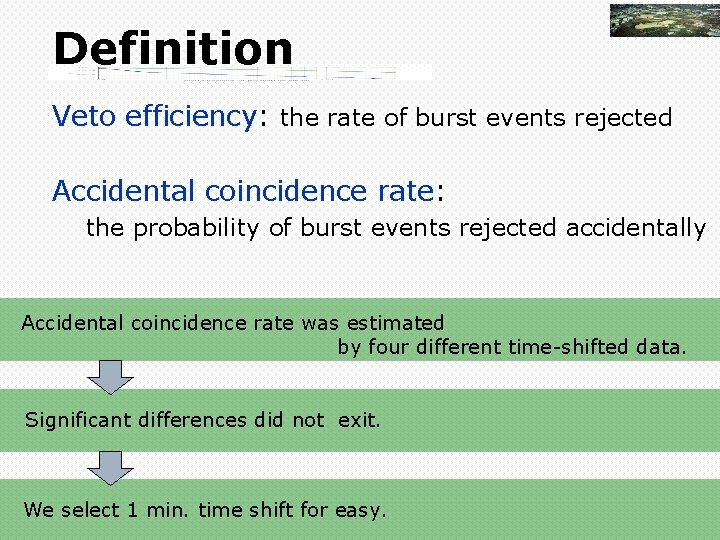
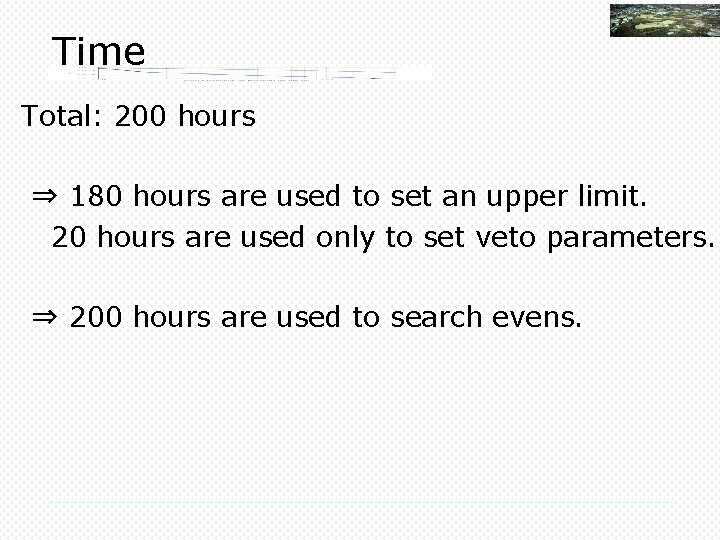
- Slides: 36
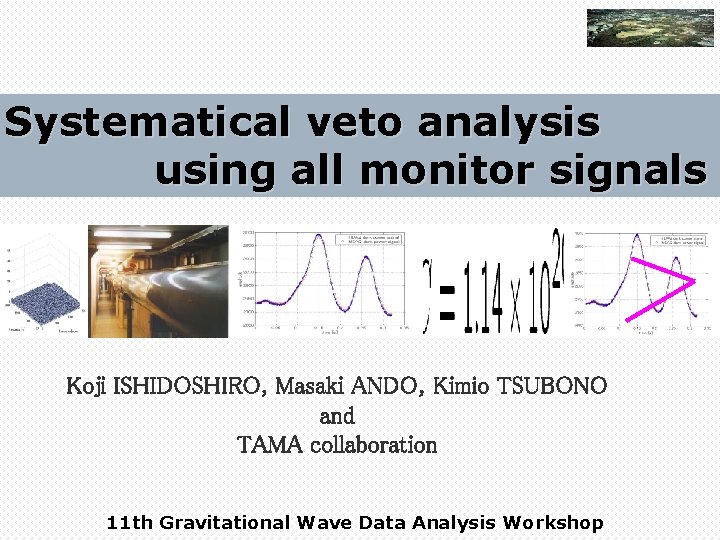
Systematical veto analysis using all monitor signals Koji ISHIDOSHIRO, Masaki ANDO, Kimio TSUBONO and TAMA collaboration 11 th Gravitational Wave Data Analysis Workshop
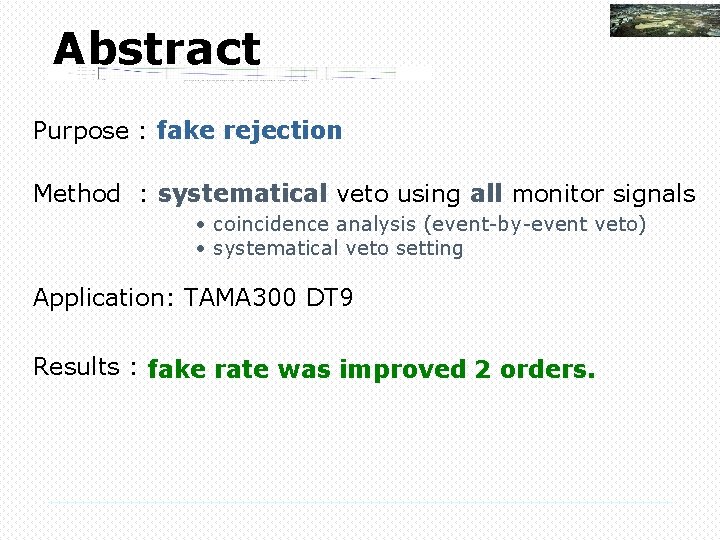
Abstract Purpose : fake rejection Method : systematical veto using all monitor signals • coincidence analysis (event-by-event veto) • systematical veto setting Application: TAMA 300 DT 9 Results : fake rate was improved 2 orders.
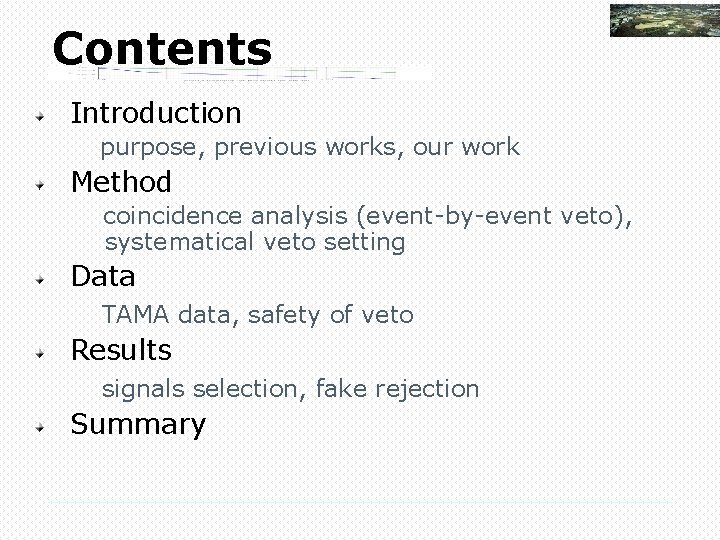
Contents Introduction purpose, previous works, our work Method coincidence analysis (event-by-event veto), systematical veto setting Data TAMA data, safety of veto Results signals selection, fake rejection Summary
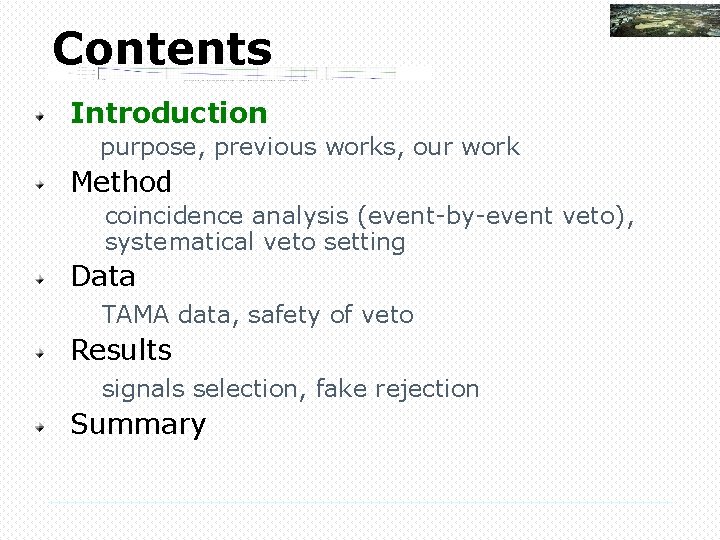
Contents Introduction purpose, previous works, our work Method coincidence analysis (event-by-event veto), systematical veto setting Data TAMA data, safety of veto Results signals selection, fake rejection Summary
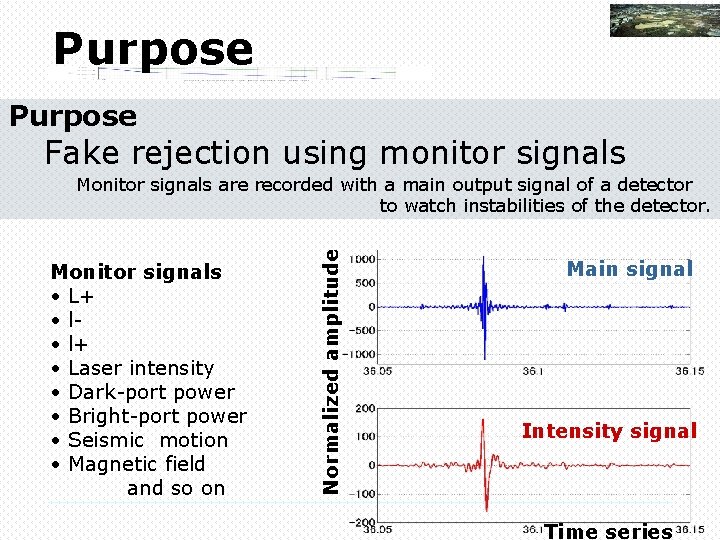
Purpose Fake rejection using monitor signals Monitor signals • L+ • l+ • Laser intensity • Dark-port power • Bright-port power • Seismic motion • Magnetic field and so on Normalized amplitude Monitor signals are recorded with a main output signal of a detector to watch instabilities of the detector. Main signal Intensity signal Time series
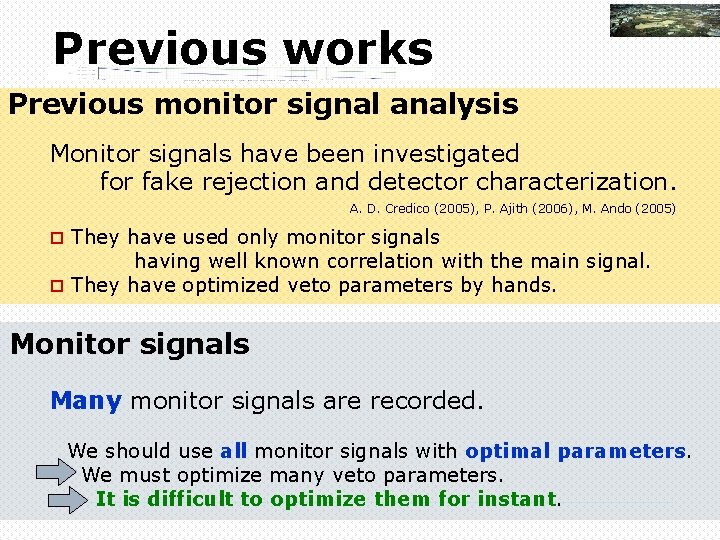
Previous works Previous monitor signal analysis Monitor signals have been investigated for fake rejection and detector characterization. A. D. Credico (2005), P. Ajith (2006), M. Ando (2005) They have used only monitor signals having well known correlation with the main signal. p They have optimized veto parameters by hands. p Monitor signals Many monitor signals are recorded. We should use all monitor signals with optimal parameters. We must optimize many veto parameters. It is difficult to optimize them for instant.
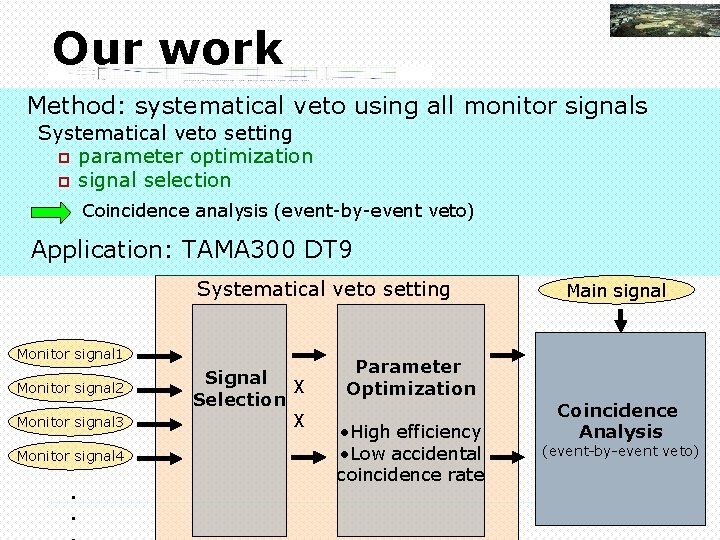
Our work Method: systematical veto using all monitor signals Systematical veto setting p parameter optimization p signal selection Coincidence analysis (event-by-event veto) Application: TAMA 300 DT 9 Systematical veto setting Monitor signal 1 Monitor signal 2 Monitor signal 3 Monitor signal 4 ・ ・ Signal X Selection X Parameter Optimization • High efficiency • Low accidental coincidence rate Main signal Coincidence Analysis (event-by-event veto)
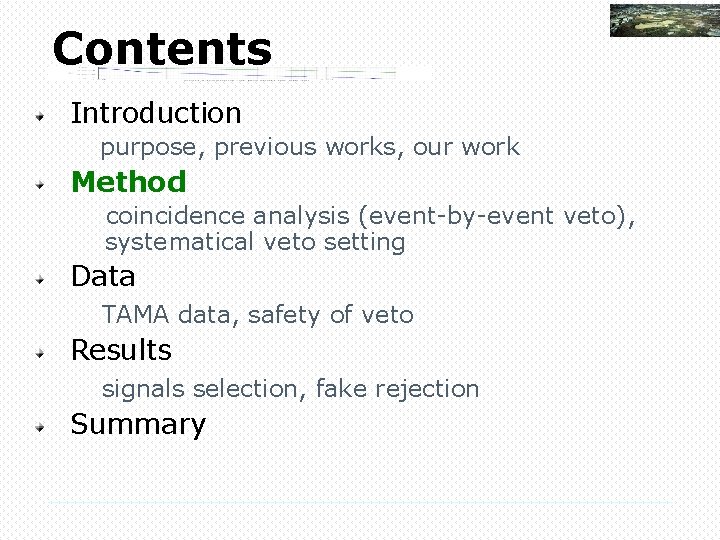
Contents Introduction purpose, previous works, our work Method coincidence analysis (event-by-event veto), systematical veto setting Data TAMA data, safety of veto Results signals selection, fake rejection Summary
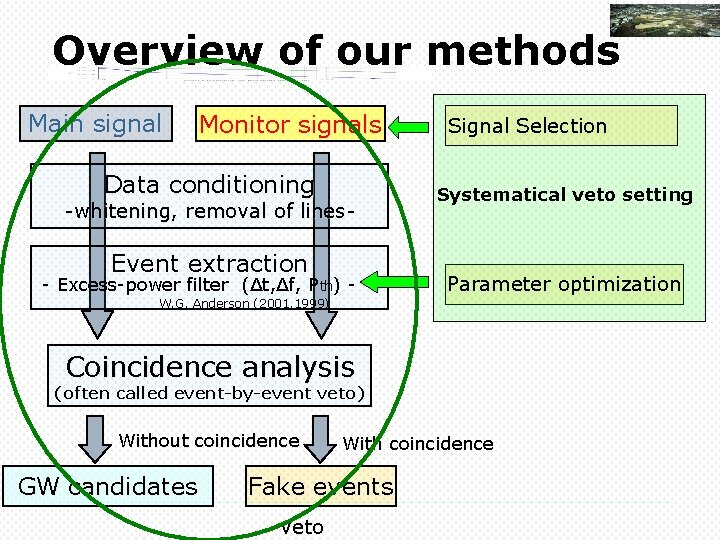
Overview of our methods Main signal Monitor signals Data conditioning -whitening, removal of lines- Event extraction - Excess-power filter (Δt, Δf, Pth) - Signal Selection Systematical veto setting Parameter optimization W. G. Anderson (2001, 1999) Coincidence analysis (often called event-by-event veto) Without coincidence GW candidates With coincidence Fake events veto
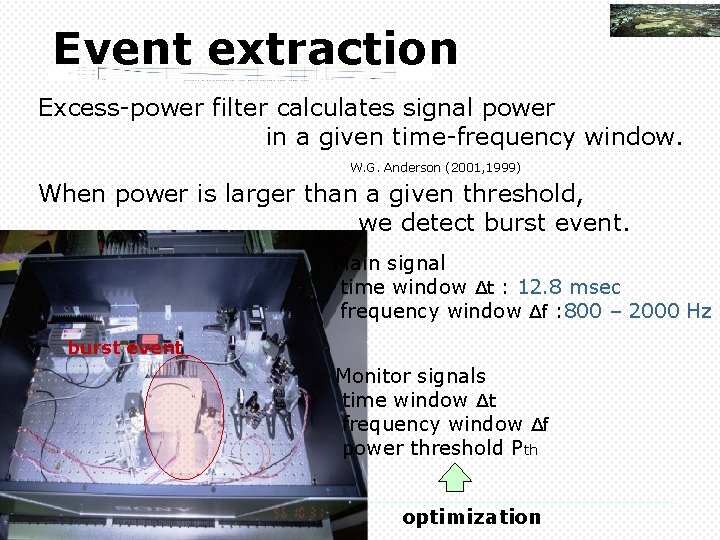
Event extraction Excess-power filter calculates signal power in a given time-frequency window. W. G. Anderson (2001, 1999) When power is larger than a given threshold, we detect burst event. Main signal time window Δt : 12. 8 msec frequency window Δf : 800 – 2000 Hz burst event Monitor signals time window Δt frequency window Δf power threshold Pth optimization
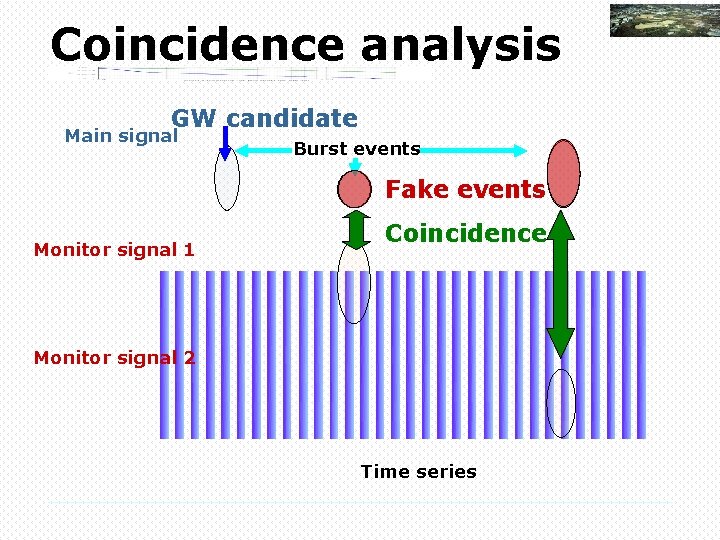
Coincidence analysis GW candidate Main signal Burst events Fake events Monitor signal 1 Coincidence Monitor signal 2 Time series
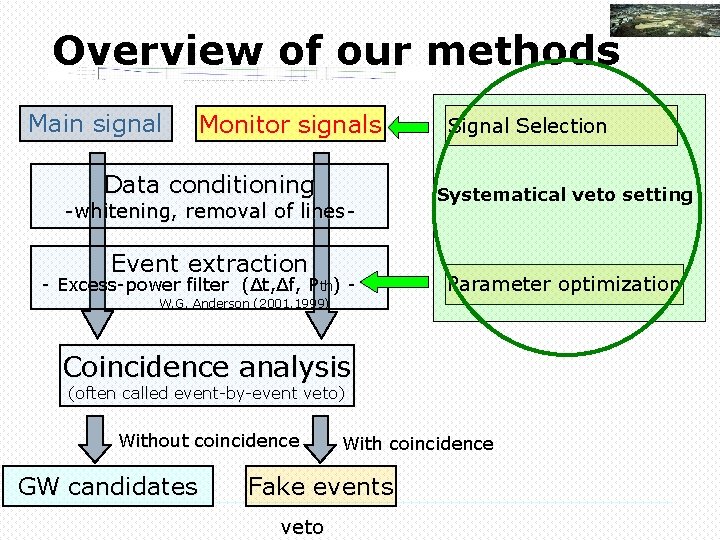
Overview of our methods Main signal Monitor signals Data conditioning -whitening, removal of lines- Event extraction - Excess-power filter (Δt, Δf, Pth) - Signal Selection Systematical veto setting Parameter optimization W. G. Anderson (2001, 1999) Coincidence analysis (often called event-by-event veto) Without coincidence GW candidates With coincidence Fake events veto
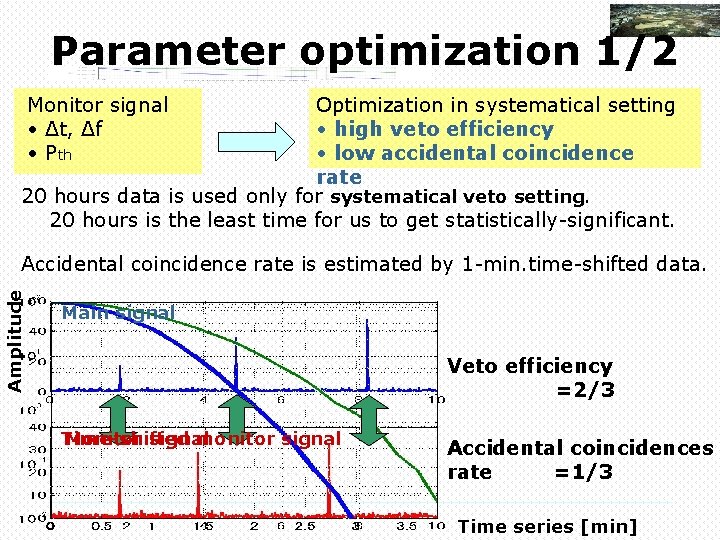
Parameter optimization 1/2 Monitor signal • Δt, Δf • Pth Optimization in systematical setting • high veto efficiency • low accidental coincidence rate 20 hours data is used only for systematical veto setting. 20 hours is the least time for us to get statistically-significant. Amplitude Accidental coincidence rate is estimated by 1 -min. time-shifted data. Main signal Veto efficiency =2/3 Time-shifted Monitor signal monitor signal Accidental coincidences rate =1/3 Time series [min]
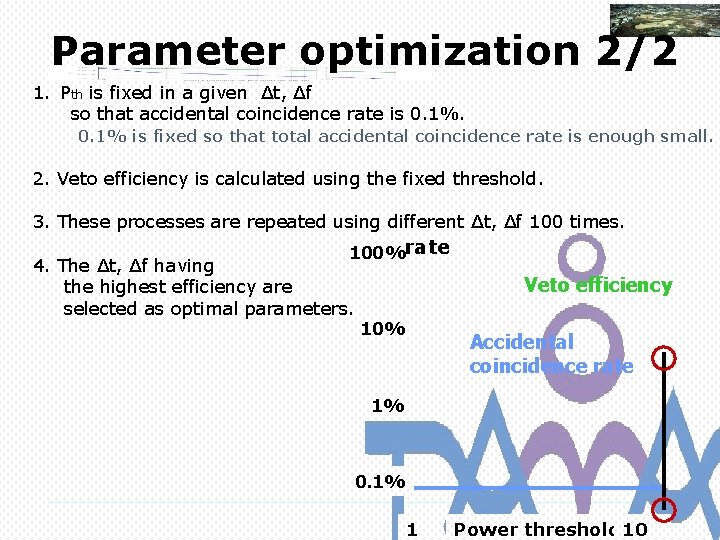
Parameter optimization 2/2 1. Pth is fixed in a given Δt, Δf so that accidental coincidence rate is 0. 1% is fixed so that total accidental coincidence rate is enough small. 2. Veto efficiency is calculated using the fixed threshold. 3. These processes are repeated using different Δt, Δf 100 times. 100%rate 4. The Δt, Δf having the highest efficiency are selected as optimal parameters. Veto efficiency 10% Accidental coincidence rate 1% 0. 1% 1 Power threshold 10
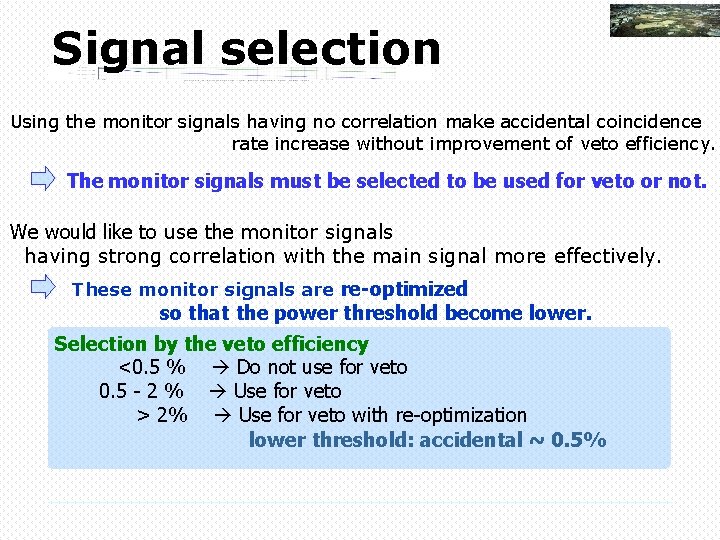
Signal selection Using the monitor signals having no correlation make accidental coincidence rate increase without improvement of veto efficiency. The monitor signals must be selected to be used for veto or not. We would like to use the monitor signals having strong correlation with the main signal more effectively. These monitor signals are re-optimized so that the power threshold become lower. Selection by the veto efficiency <0. 5 % Do not use for veto 0. 5 - 2 % Use for veto > 2% Use for veto with re-optimization lower threshold: accidental ~ 0. 5%
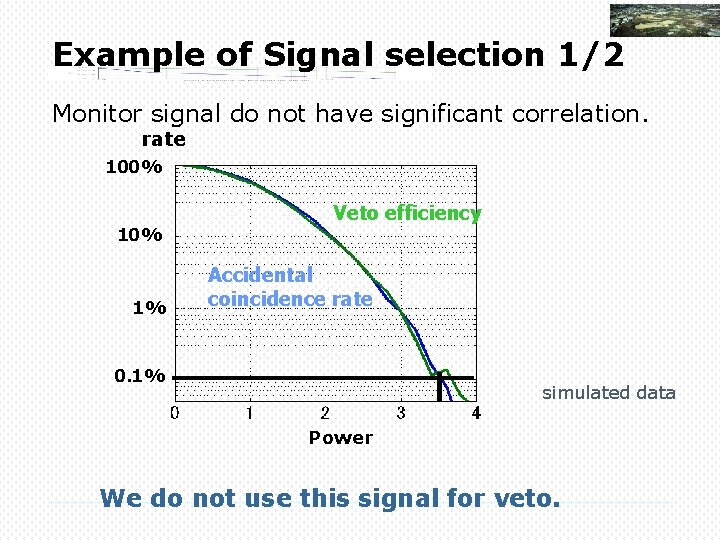
Example of Signal selection 1/2 Monitor signal do not have significant correlation. rate 100% 1% Veto efficiency Accidental coincidence rate 0. 1% simulated data Power We do not use this signal for veto.
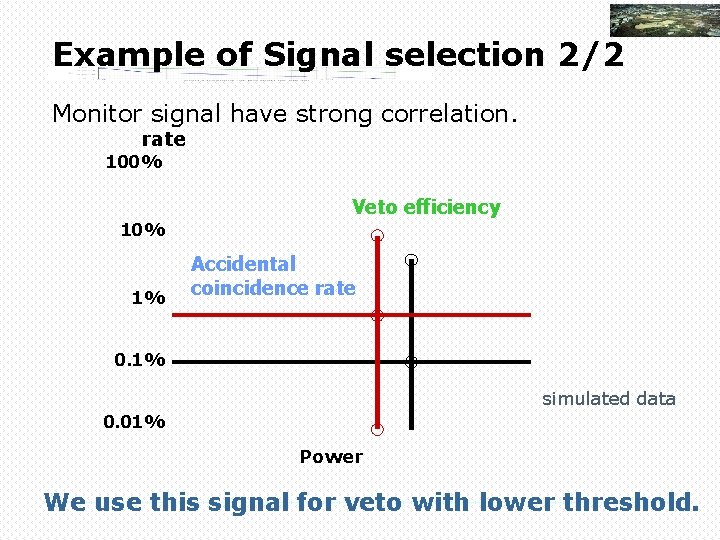
Example of Signal selection 2/2 Monitor signal have strong correlation. rate 100% 1% Veto efficiency Accidental coincidence rate 0. 1% simulated data 0. 01% Power We use this signal for veto with lower threshold.
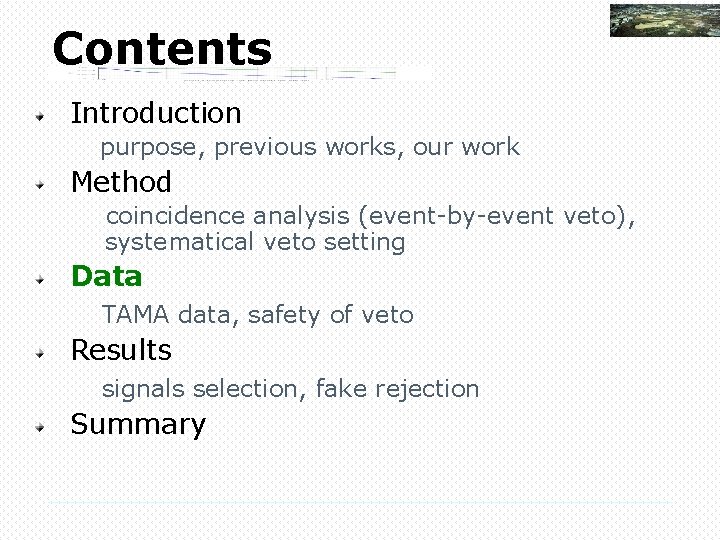
Contents Introduction purpose, previous works, our work Method coincidence analysis (event-by-event veto), systematical veto setting Data TAMA data, safety of veto Results signals selection, fake rejection Summary
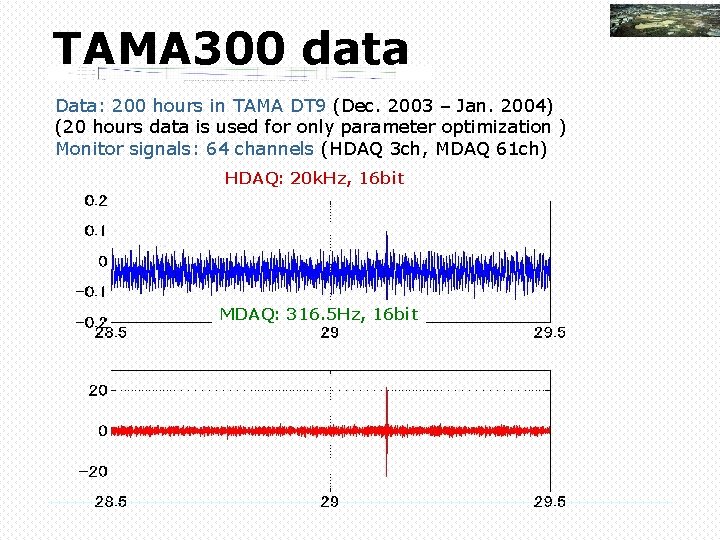
TAMA 300 data Data: 200 hours in TAMA DT 9 (Dec. 2003 – Jan. 2004) (20 hours data is used for only parameter optimization ) Monitor signals: 64 channels (HDAQ 3 ch, MDAQ 61 ch) HDAQ: 20 k. Hz, 16 bit MDAQ: 316. 5 Hz, 16 bit
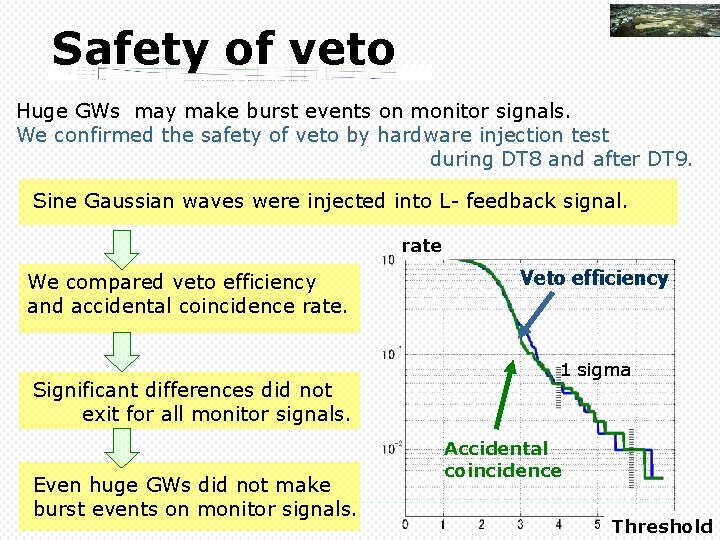
Safety of veto Huge GWs may make burst events on monitor signals. We confirmed the safety of veto by hardware injection test during DT 8 and after DT 9. Sine Gaussian waves were injected into L- feedback signal. rate We compared veto efficiency and accidental coincidence rate. Significant differences did not exit for all monitor signals. Even huge GWs did not make burst events on monitor signals. Veto efficiency 1 sigma Accidental coincidence Threshold
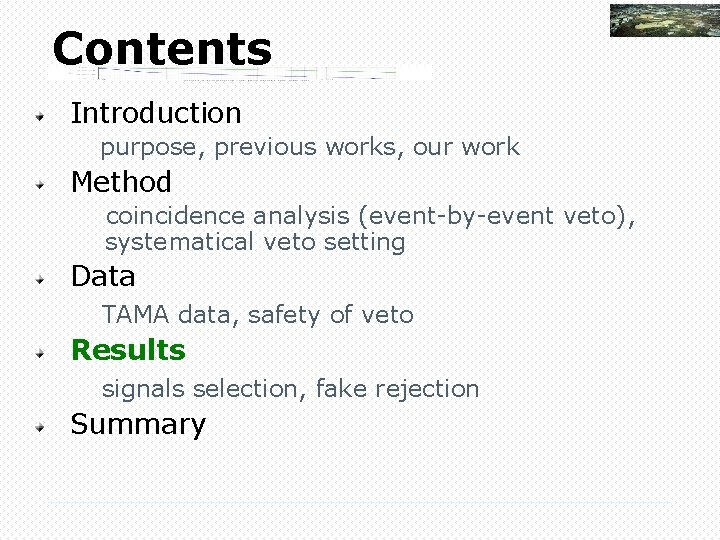
Contents Introduction purpose, previous works, our work Method coincidence analysis (event-by-event veto), systematical veto setting Data TAMA data, safety of veto Results signals selection, fake rejection Summary
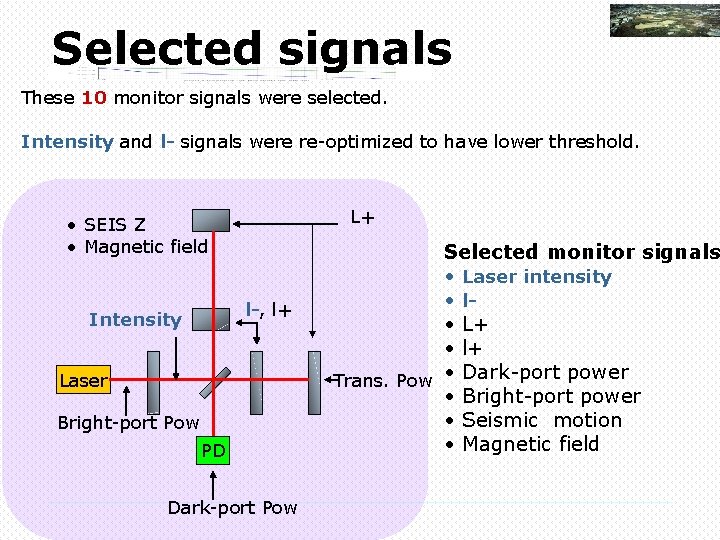
Selected signals These 10 monitor signals were selected. Intensity and l- signals were re-optimized to have lower threshold. L+ • SEIS Z • Magnetic field l-, l+ Intensity Laser Bright-port Pow PD Dark-port Pow Selected monitor signals • Laser intensity • l • L+ • l+ Trans. Pow • Dark-port power • Bright-port power • Seismic motion • Magnetic field
![Fake rate Hz Fake rate was improved 2 orders hrss 10 18 Fake rate [Hz] Fake rate was improved 2 orders @ hrss = 10 -18.](https://slidetodoc.com/presentation_image/7dd79cd221c55354e69529c8bed8ddcb/image-23.jpg)
Fake rate [Hz] Fake rate was improved 2 orders @ hrss = 10 -18. Maximum amplitude of fakes was improved by 1/4. Without Accidental coincidence rate 3. 2% veto Dead time 0. 2% Power threshold With veto 1/100 1/4 hrss threshold Software injection test hrss threshold
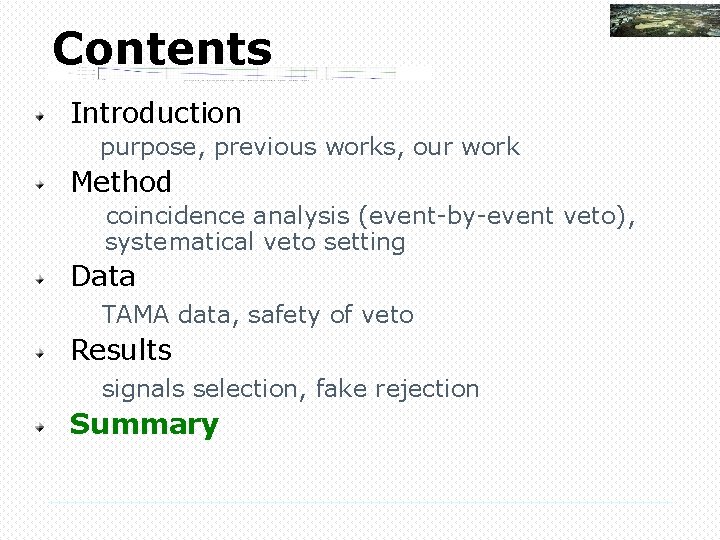
Contents Introduction purpose, previous works, our work Method coincidence analysis (event-by-event veto), systematical veto setting Data TAMA data, safety of veto Results signals selection, fake rejection Summary
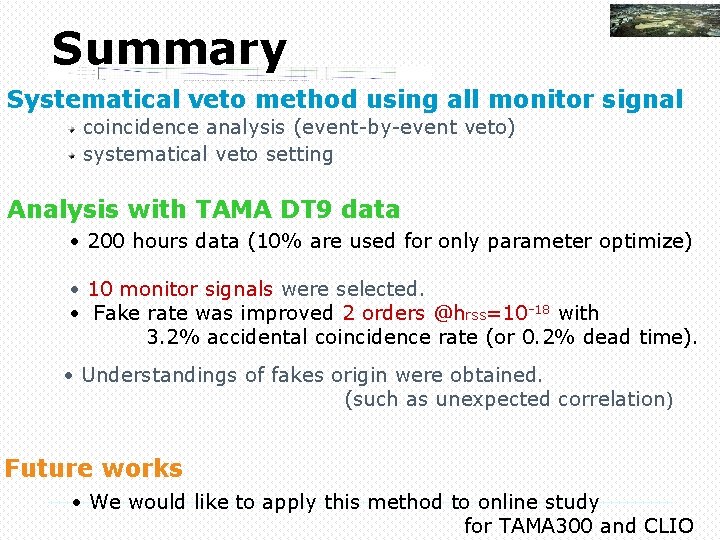
Summary Systematical veto method using all monitor signal coincidence analysis (event-by-event veto) systematical veto setting Analysis with TAMA DT 9 data • 200 hours data (10% are used for only parameter optimize) • 10 monitor signals were selected. • Fake rate was improved 2 orders @hrss=10 -18 with 3. 2% accidental coincidence rate (or 0. 2% dead time). • Understandings of fakes origin were obtained. (such as unexpected correlation ) Future works • We would like to apply this method to online study for TAMA 300 and CLIO
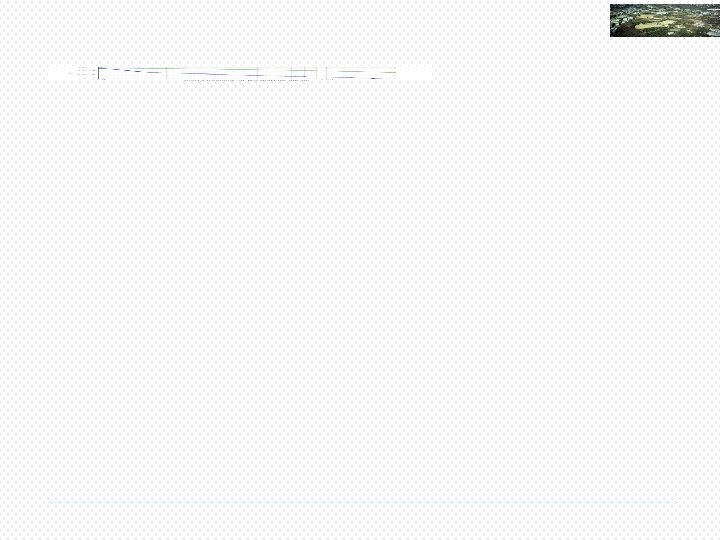
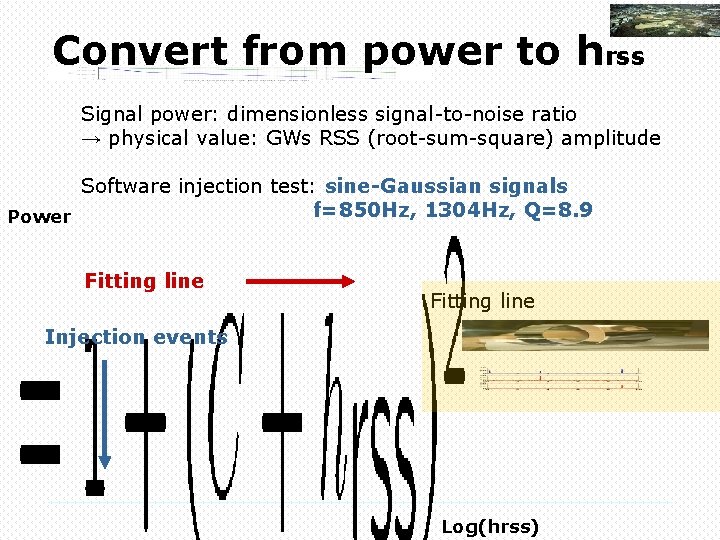
Convert from power to hrss Signal power: dimensionless signal-to-noise ratio → physical value: GWs RSS (root-sum-square) amplitude Software injection test: sine-Gaussian signals f=850 Hz, 1304 Hz, Q=8. 9 Power Fitting line Injection events Log(hrss)
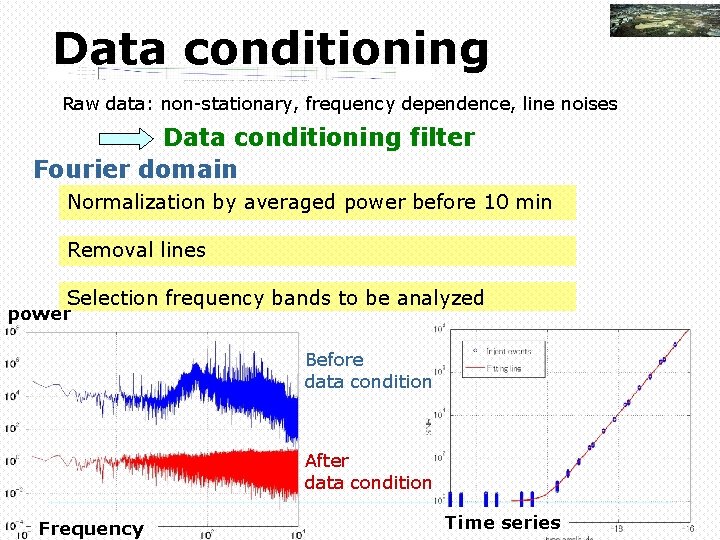
Data conditioning Raw data: non-stationary, frequency dependence, line noises Data conditioning filter Fourier domain Normalization by averaged power before 10 min Removal lines Selection frequency bands to be analyzed power Before data condition After data condition Frequency Time series
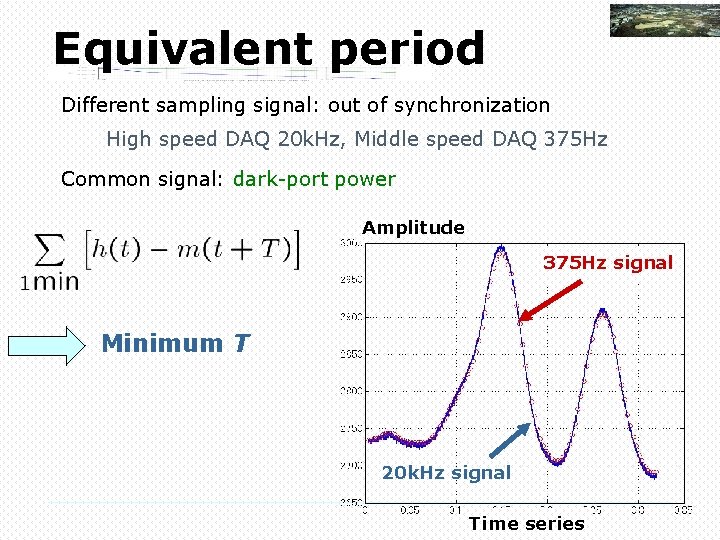
Equivalent period Different sampling signal: out of synchronization High speed DAQ 20 k. Hz, Middle speed DAQ 375 Hz Common signal: dark-port power Amplitude 375 Hz signal Minimum T 20 k. Hz signal Time series
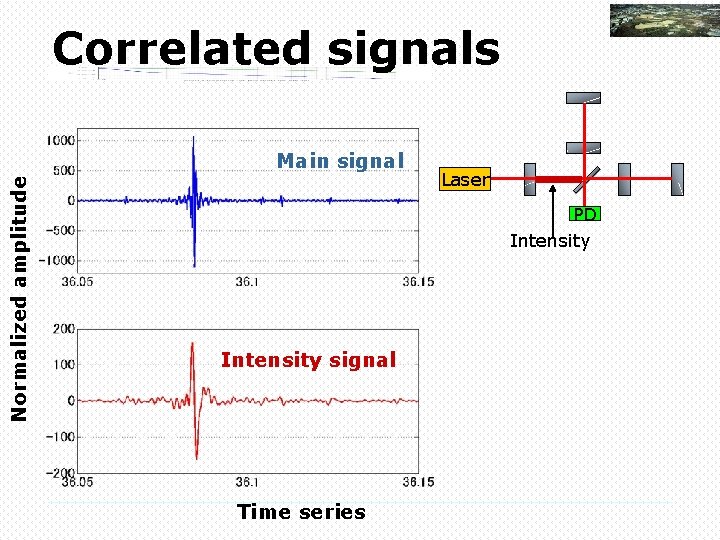
Correlated signals Normalized amplitude Main signal Laser PD Intensity signal Time series
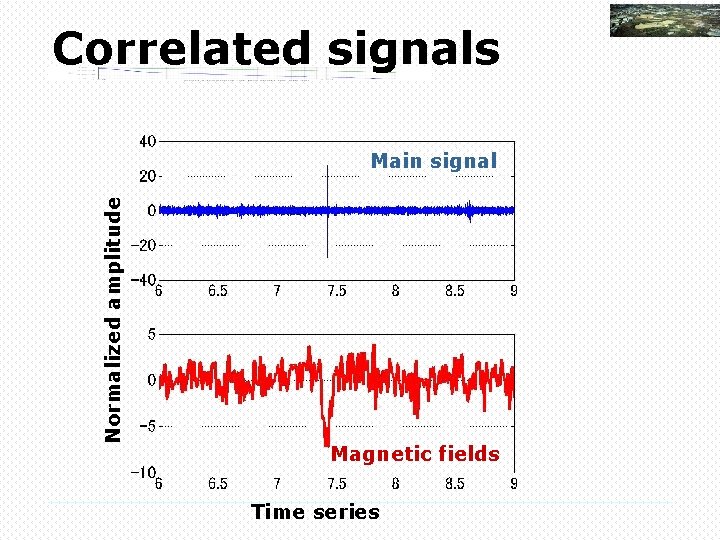
Correlated signals Normalized amplitude Main signal Magnetic fields Time series
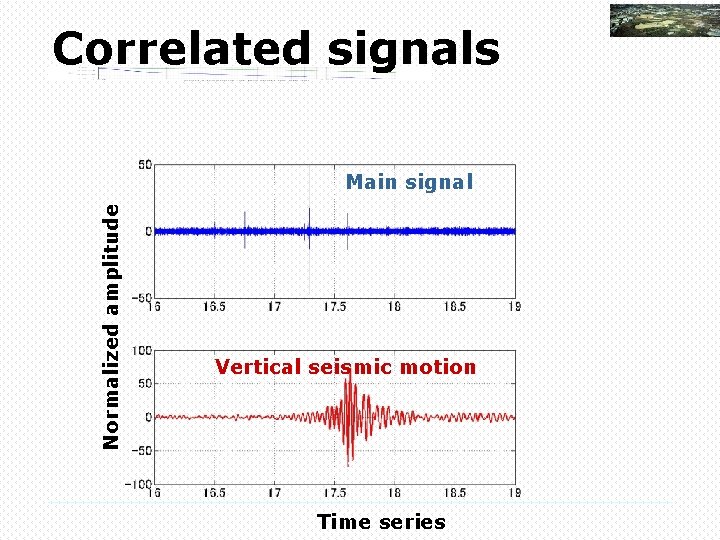
Correlated signals Normalized amplitude Main signal Vertical seismic motion Time series
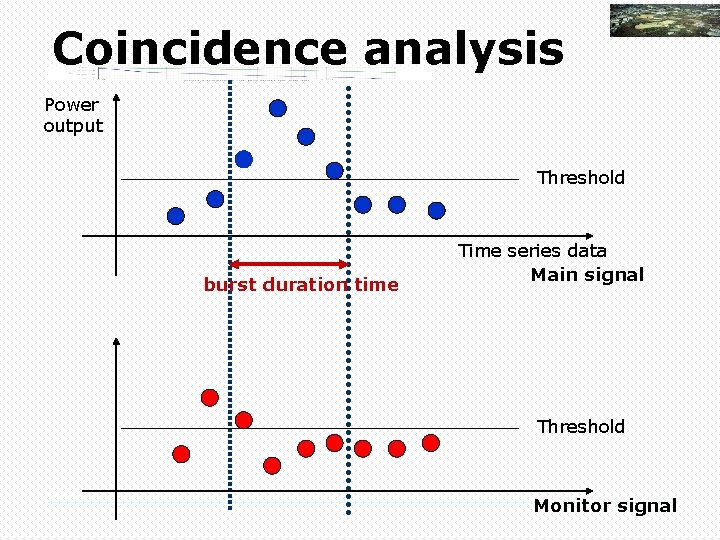
Coincidence analysis Power output Threshold burst duration time Time series data Main signal Threshold Monitor signal
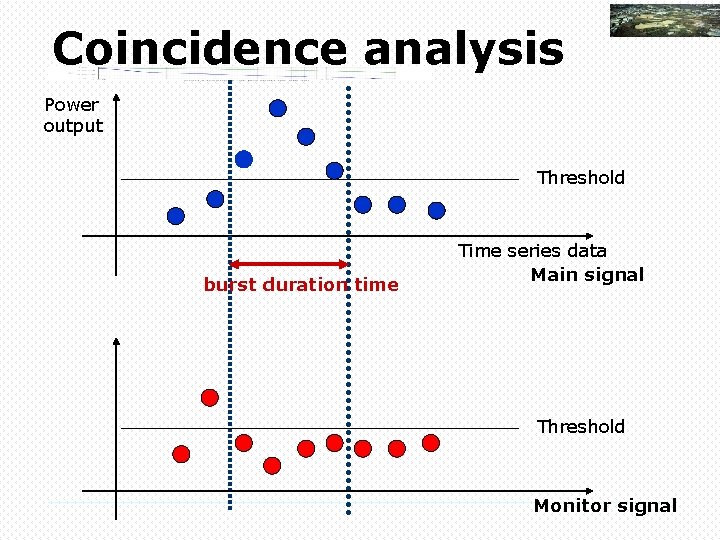
Coincidence analysis Power output Threshold burst duration time Time series data Main signal Threshold Monitor signal
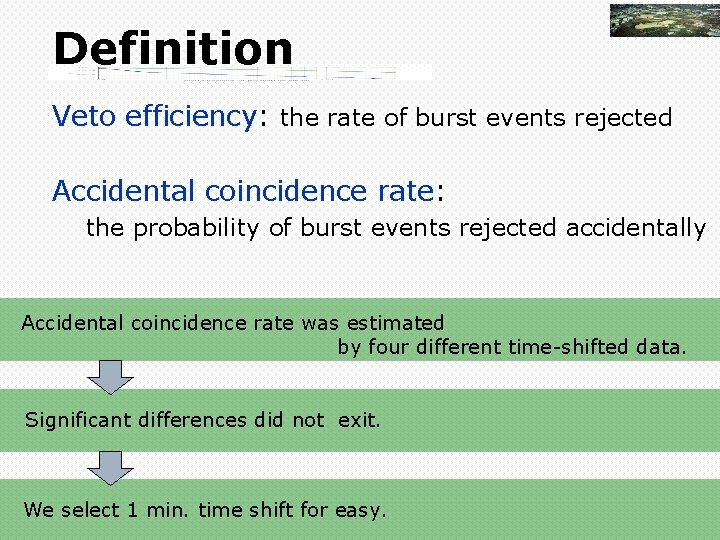
Definition Veto efficiency: the rate of burst events rejected Accidental coincidence rate: the probability of burst events rejected accidentally Accidental coincidence rate was estimated by four different time-shifted data. Significant differences did not exit. We select 1 min. time shift for easy.
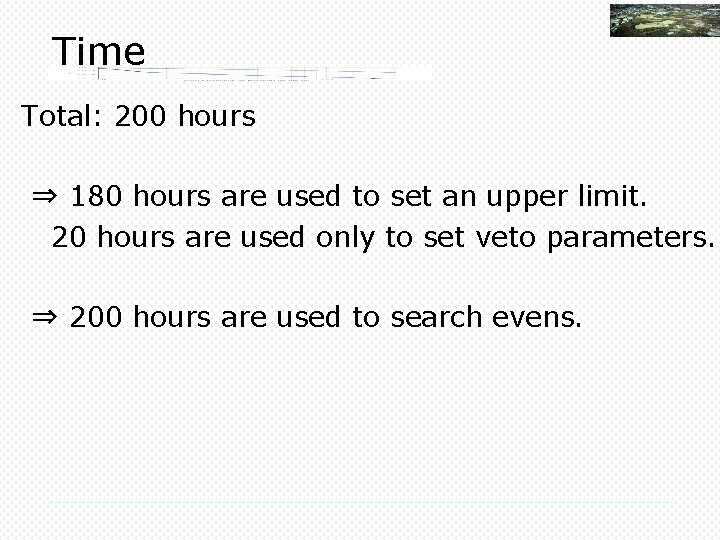
Time Total: 200 hours ⇒ 180 hours are used to set an upper limit. 20 hours are used only to set veto parameters. ⇒ 200 hours are used to search evens.
Maysville veto
A council that advised rome's leaders
Veto a law
Communicative signals and informative signals
Communicative and informative signals
Communicative signals and informative signals
Name a line containing point a
Team to serve hand signal in volleyball
Fourier analysis of discrete time signals
System.collections.generics
Dtfd switch
Dfd symbols are referenced by using all
What is data and process modeling
Using a single marketing strategy to reach all customers.
Programi koji se mogu koristiti bez ograničenja i naknade
što je umanjenik
Prirodni brojevi su
Duchamp akt
Reljef u gorskoj hrvatskoj
Razlomci brojnik i nazivnik
Neposredni sljedbenik
Predmet iz proslosti
Unutarnji procesi oblikovanja reljefa
Kanal koji spaja atlantik i pacifik
лимени дувачки инструменти
Koji su dijelovi fabule
Tvorba aorista
Vrste internet servisa
Online servisi i usluge
Inke prezentacija
Aleluja onom koji uskrsnu
Naselja gorske hrvatske
Sva geometrijska tela
Akt koji silazi niz stepenice
Filmski rodovi
Na kom kontinentu je dubai
Ukupan broj dijagonala