STATS 730 Lecture 1 1022020 730 lecture 1
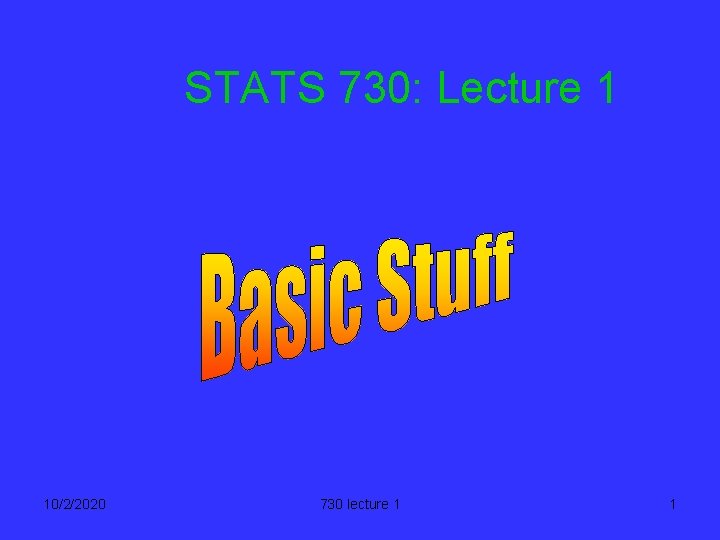
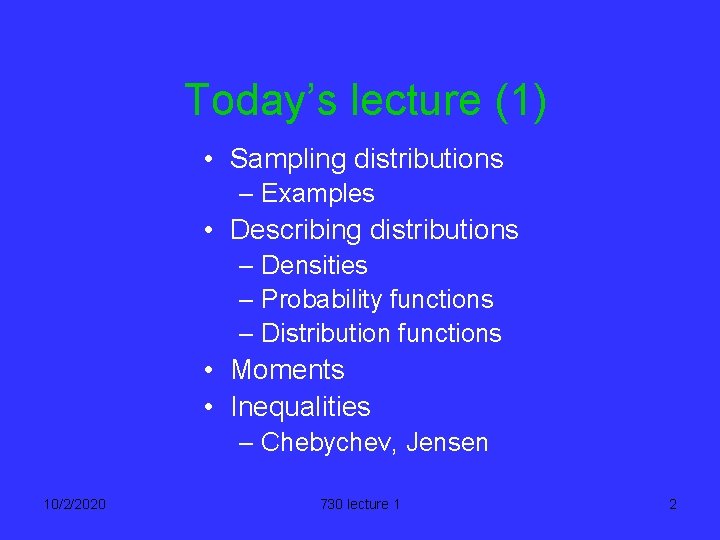
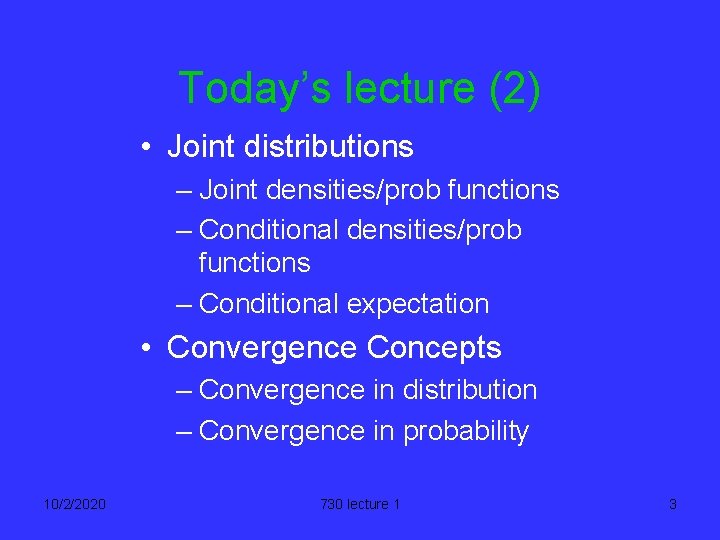
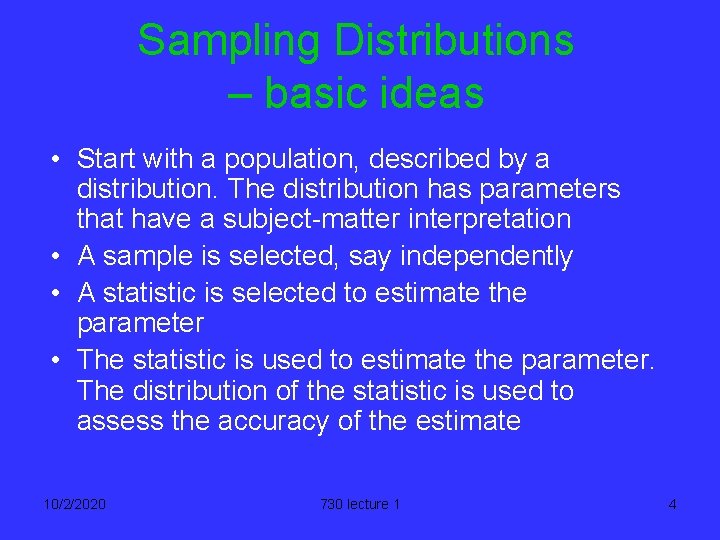
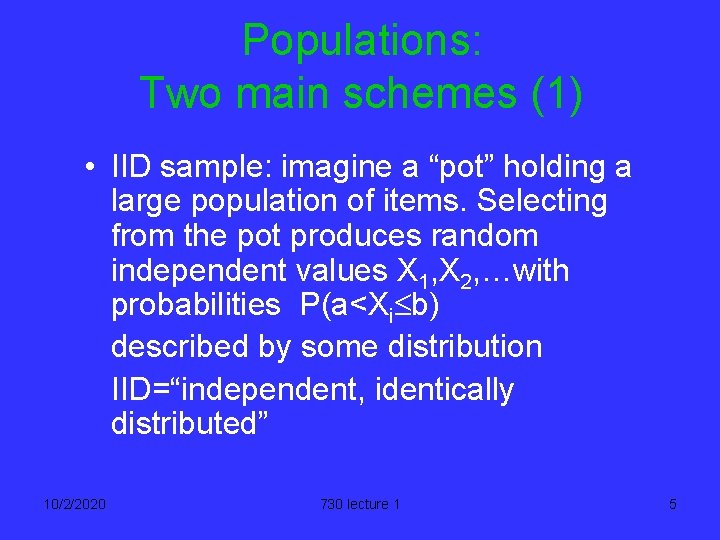
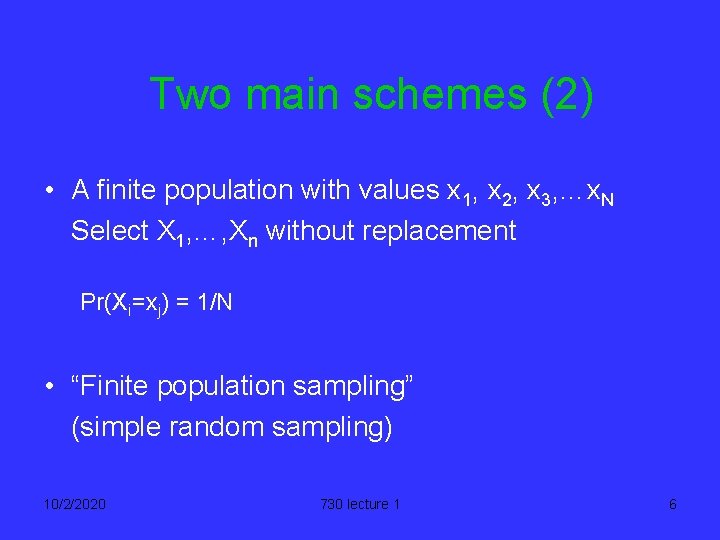
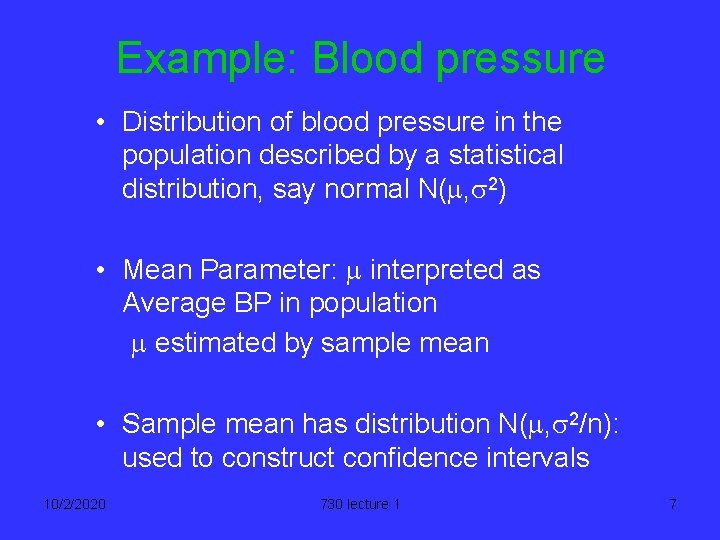
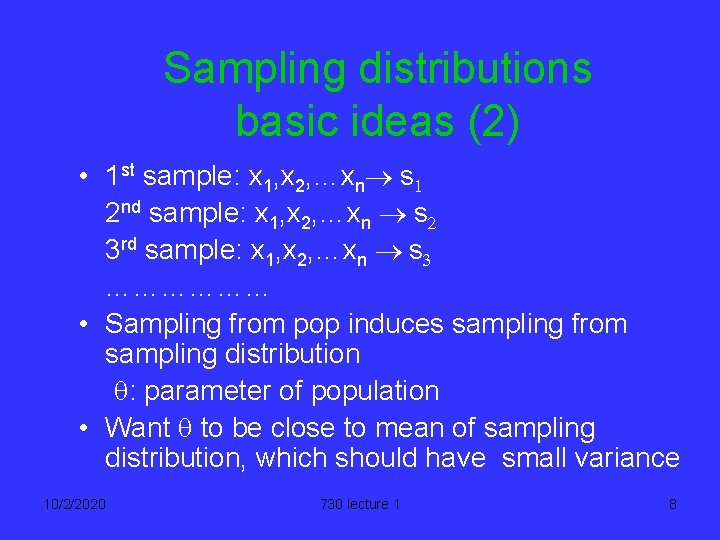
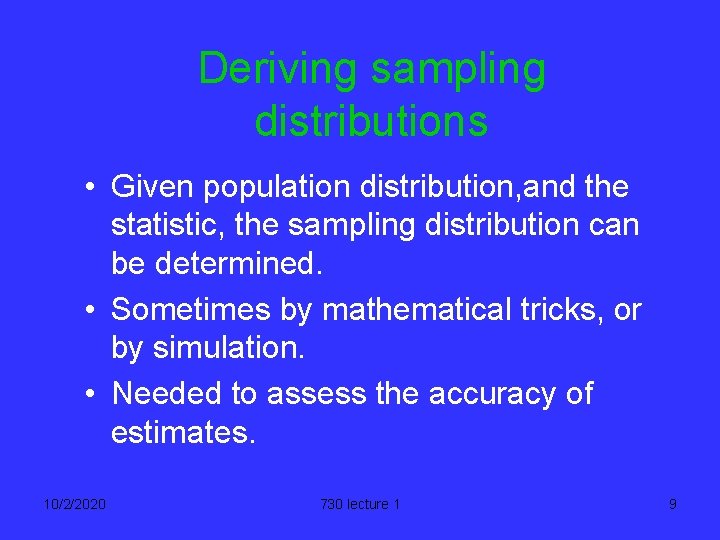
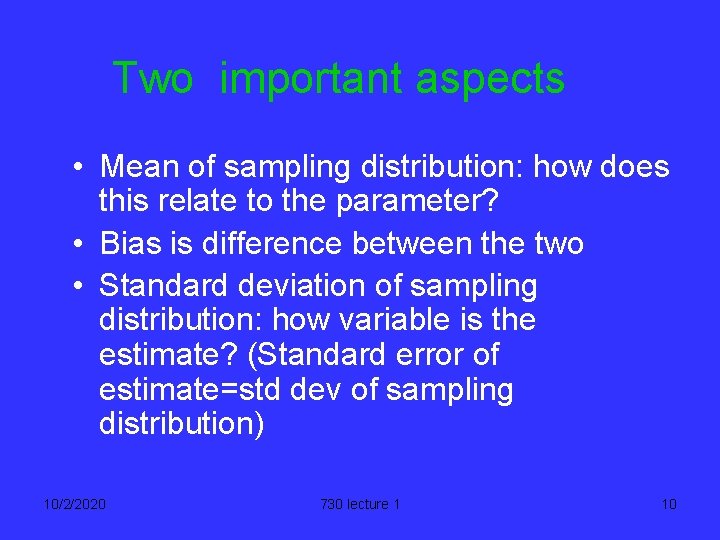
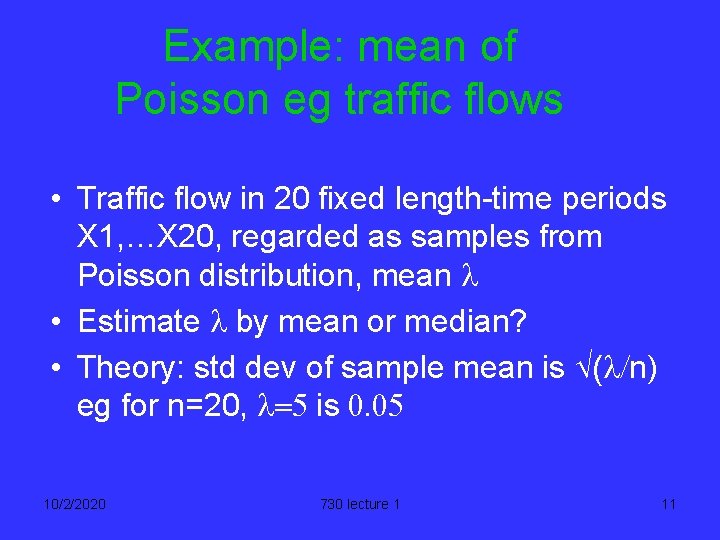
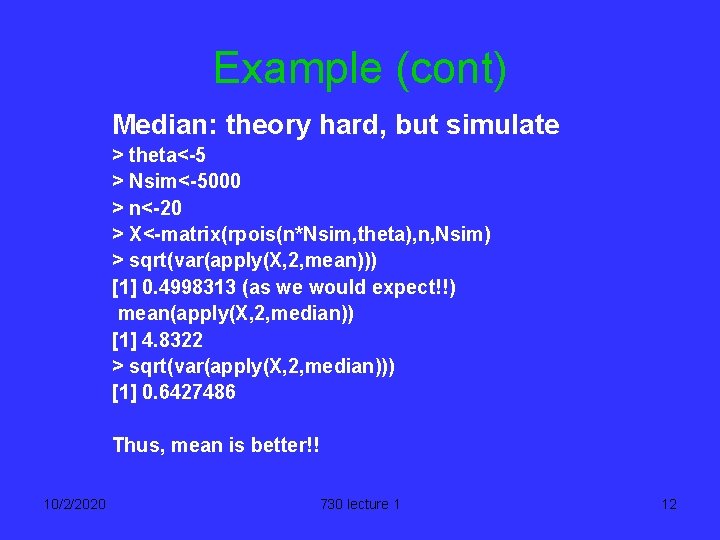
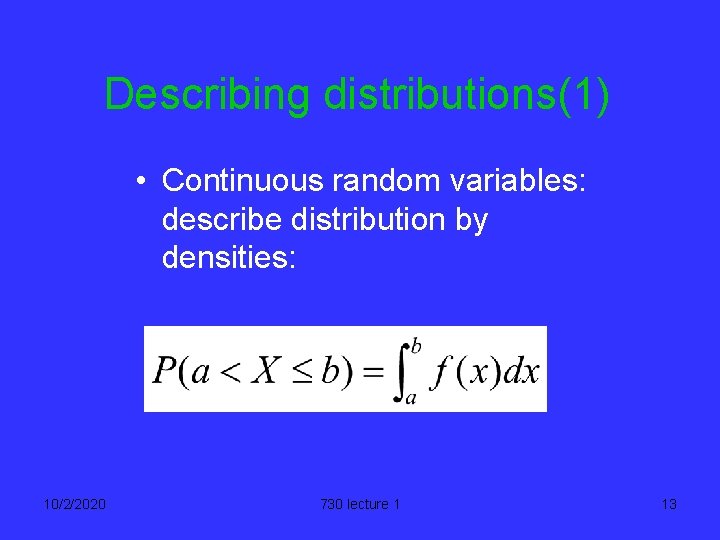
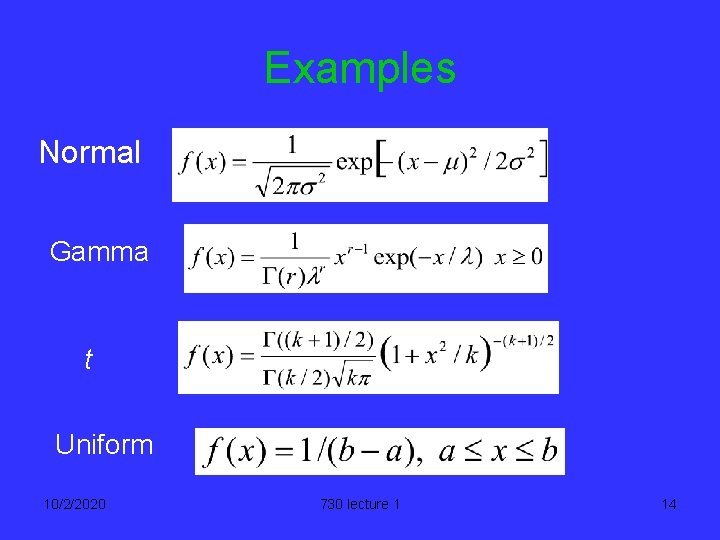
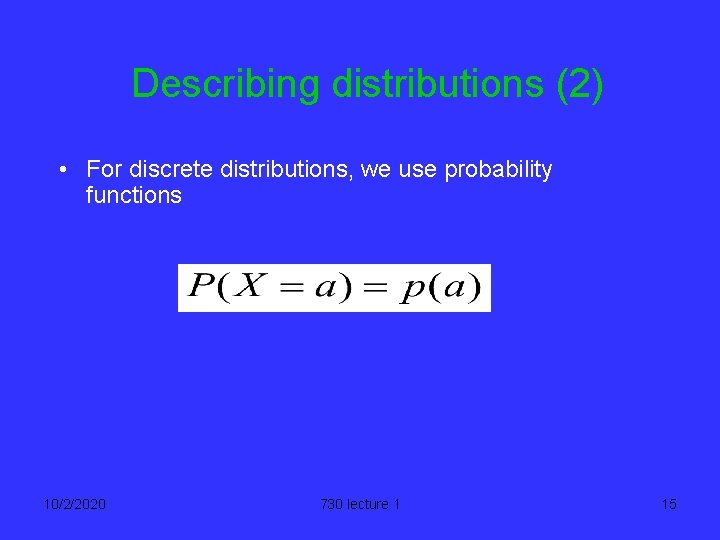
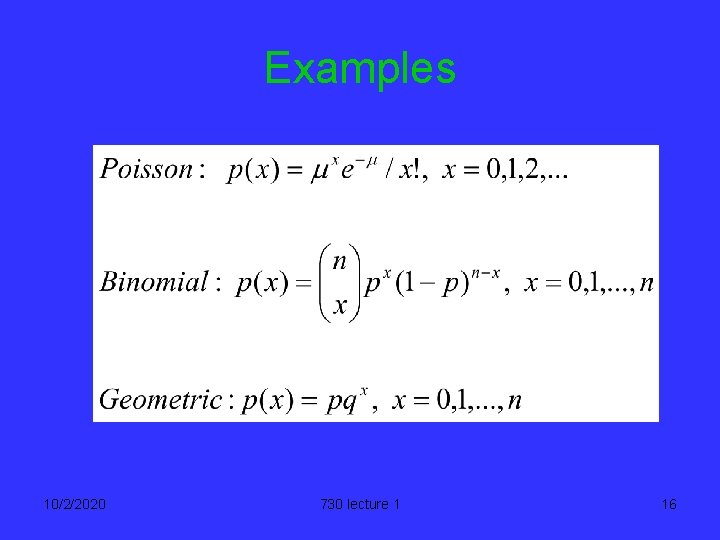
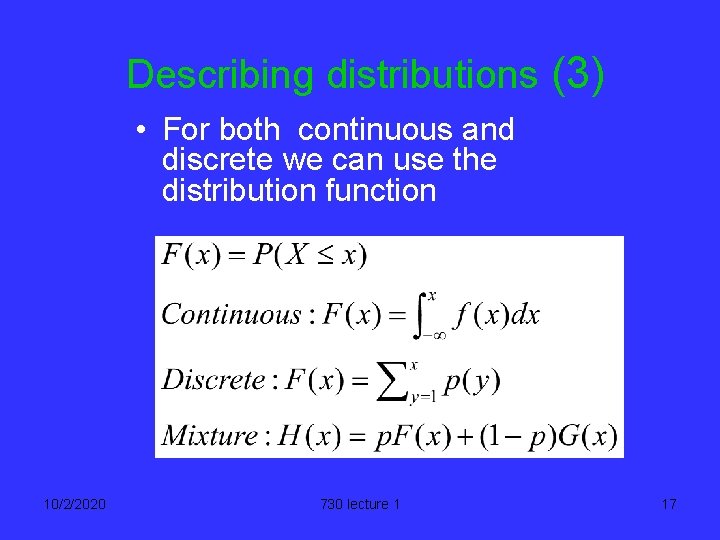
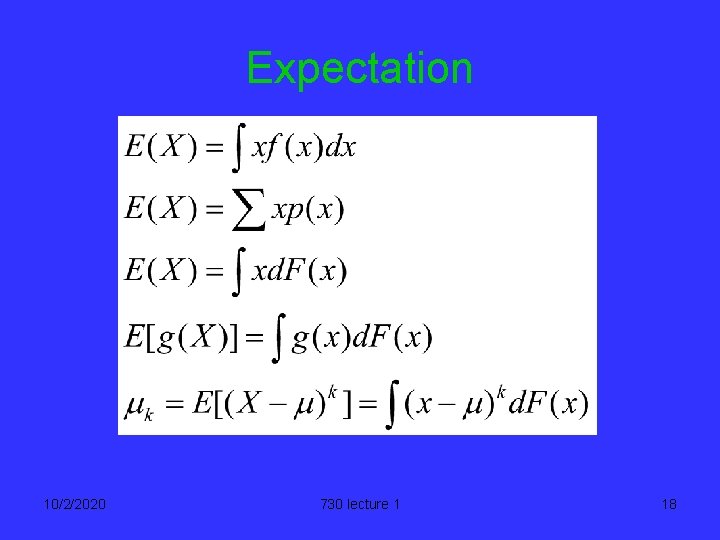
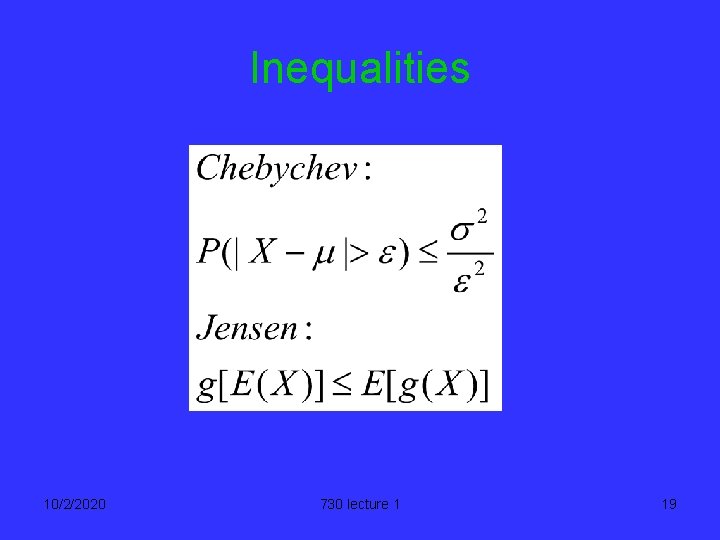
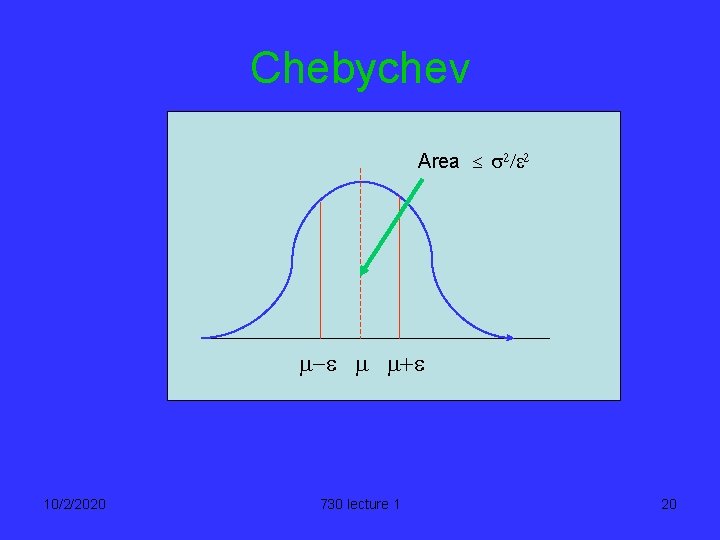
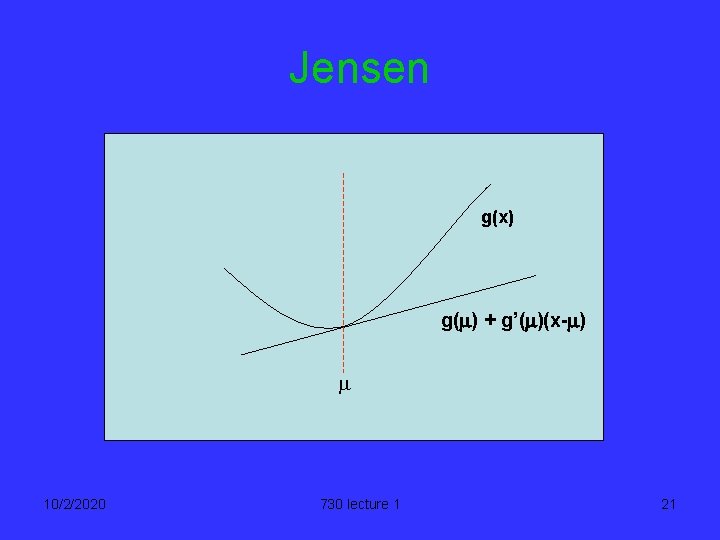
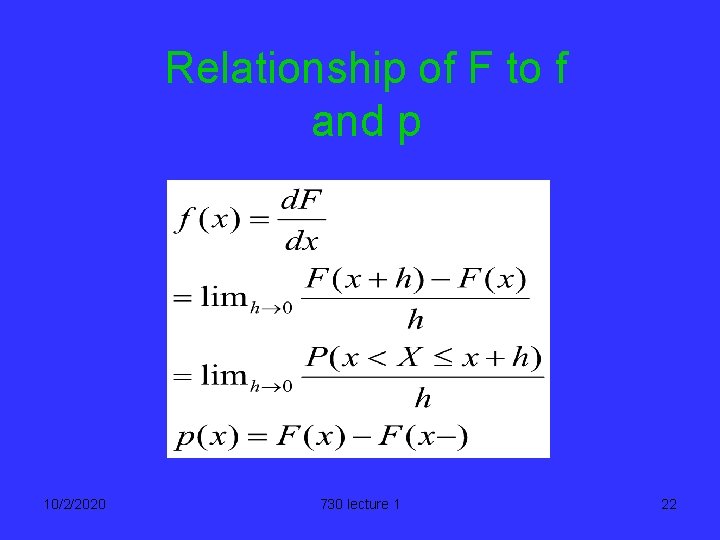
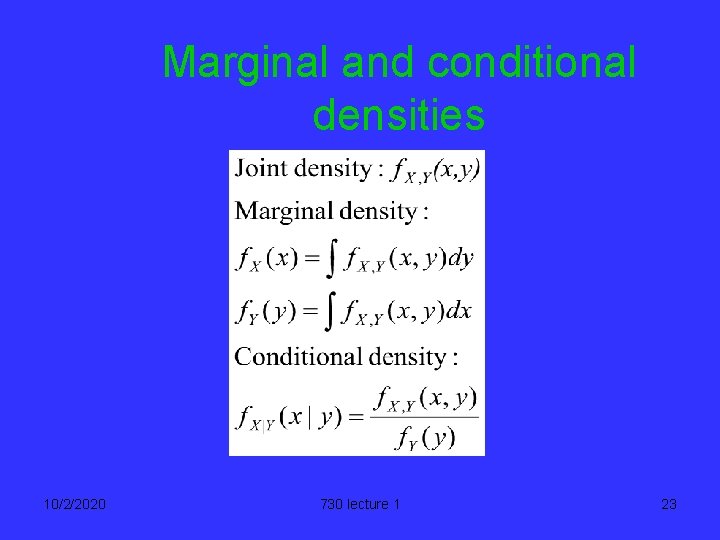
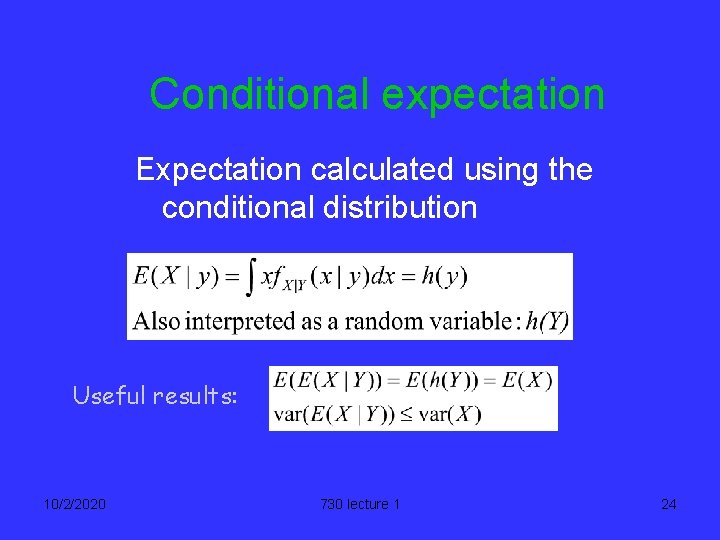
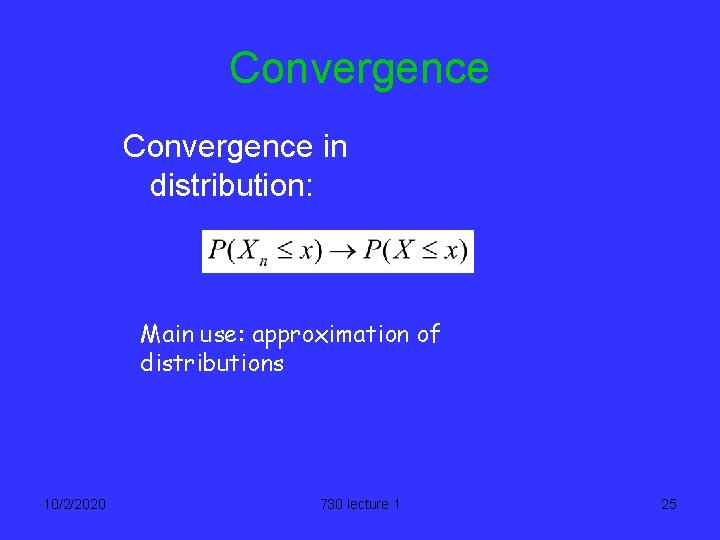
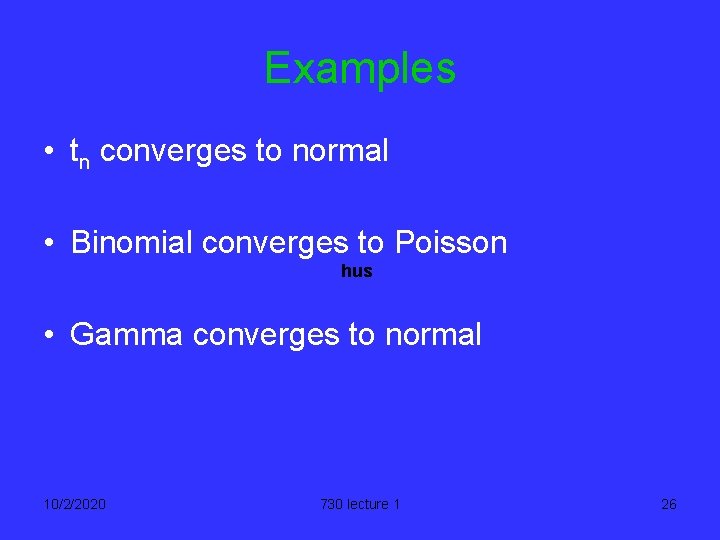
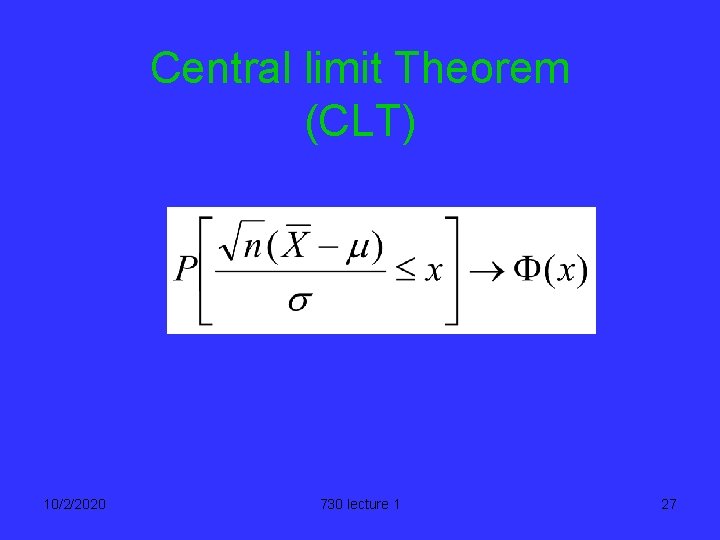
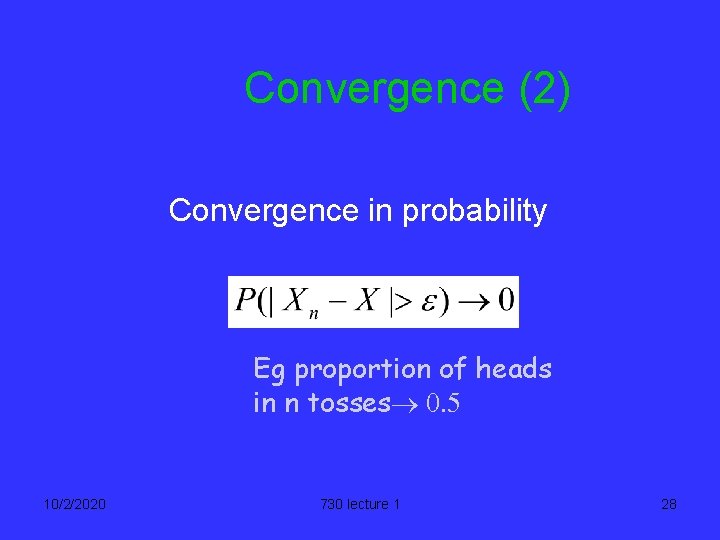
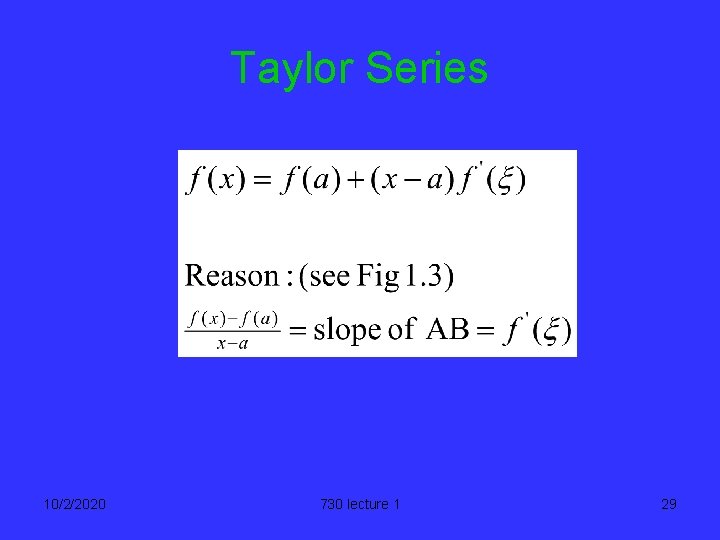
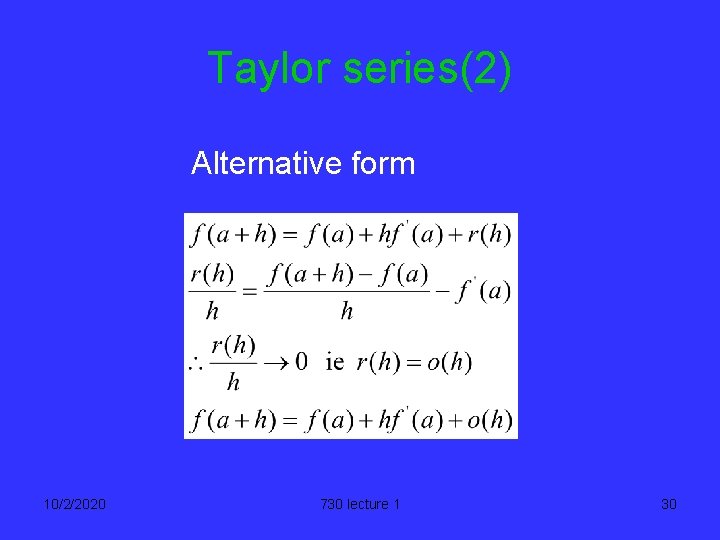
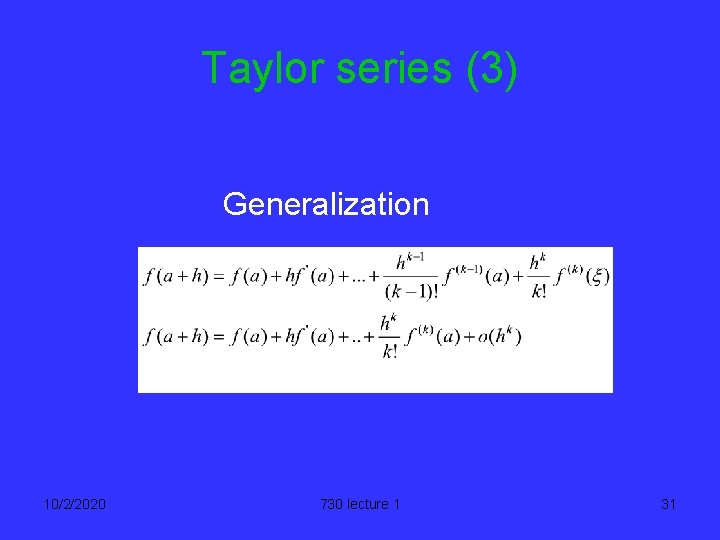
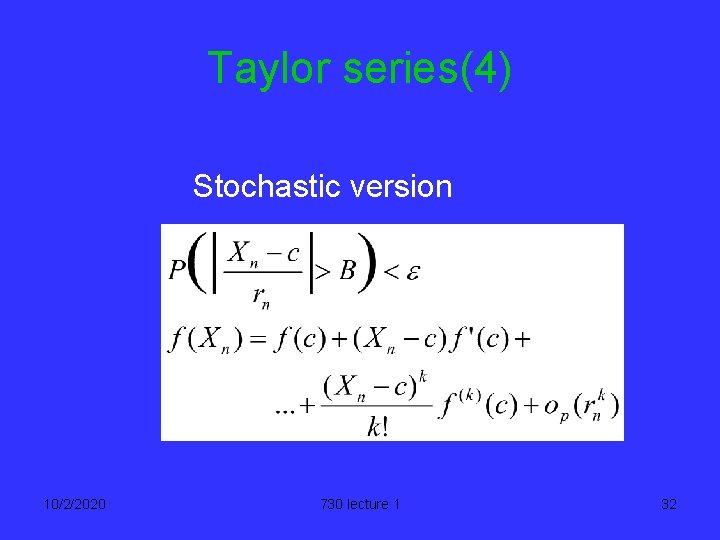
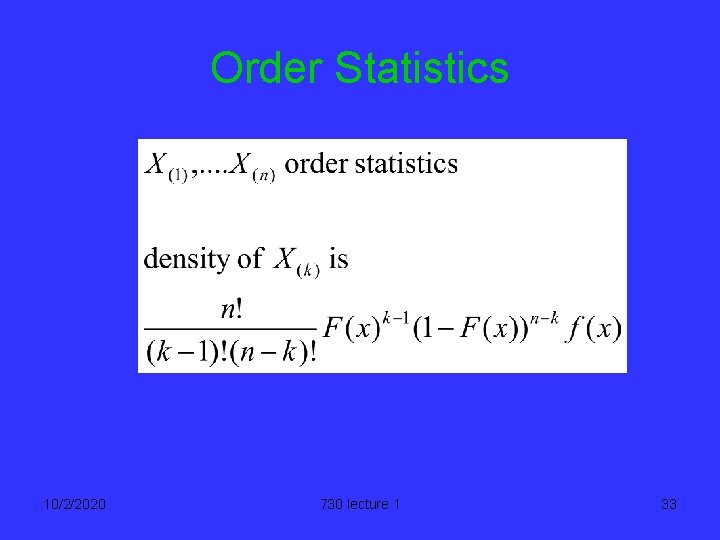
- Slides: 33
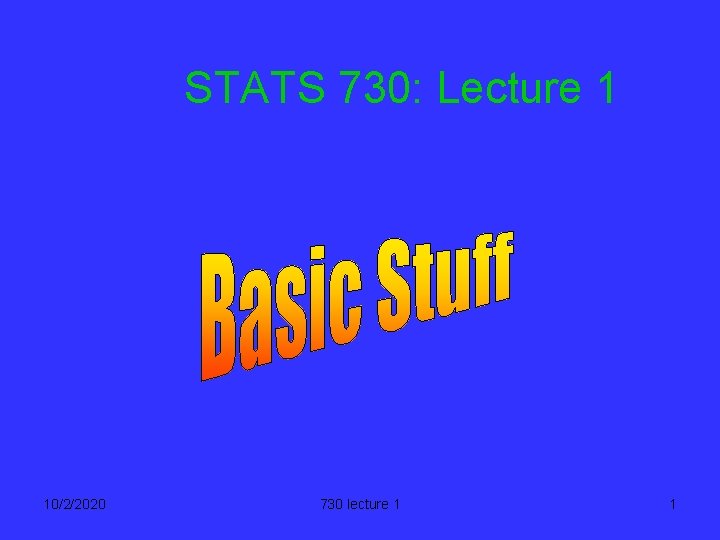
STATS 730: Lecture 1 10/2/2020 730 lecture 1 1
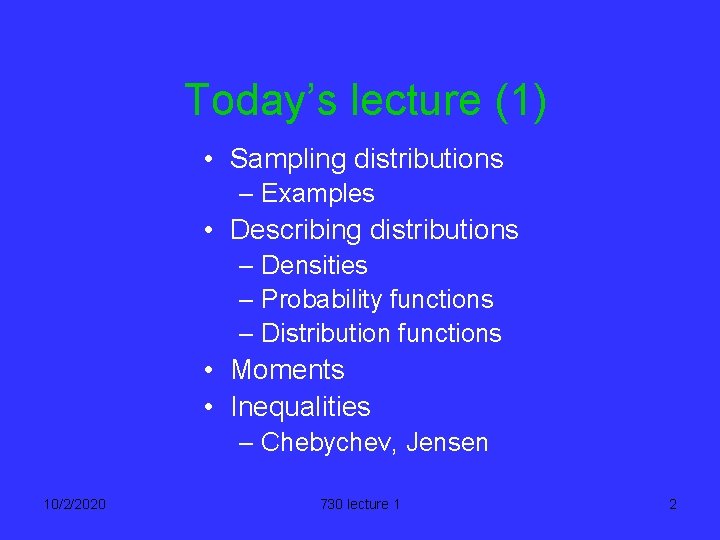
Today’s lecture (1) • Sampling distributions – Examples • Describing distributions – Densities – Probability functions – Distribution functions • Moments • Inequalities – Chebychev, Jensen 10/2/2020 730 lecture 1 2
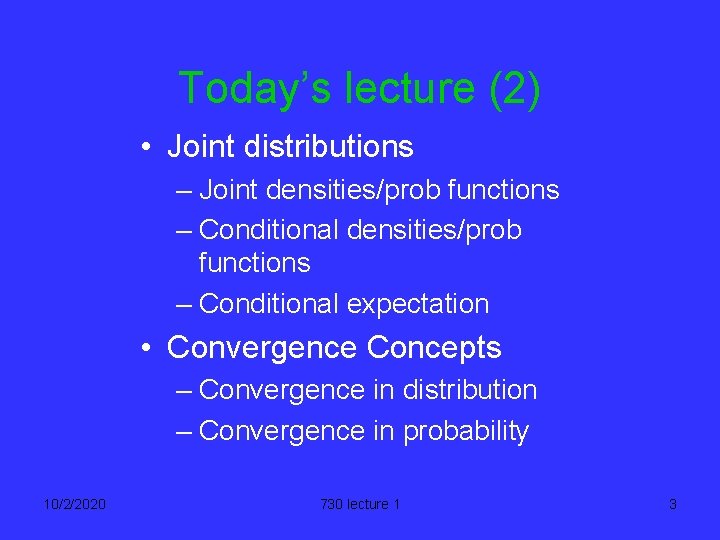
Today’s lecture (2) • Joint distributions – Joint densities/prob functions – Conditional expectation • Convergence Concepts – Convergence in distribution – Convergence in probability 10/2/2020 730 lecture 1 3
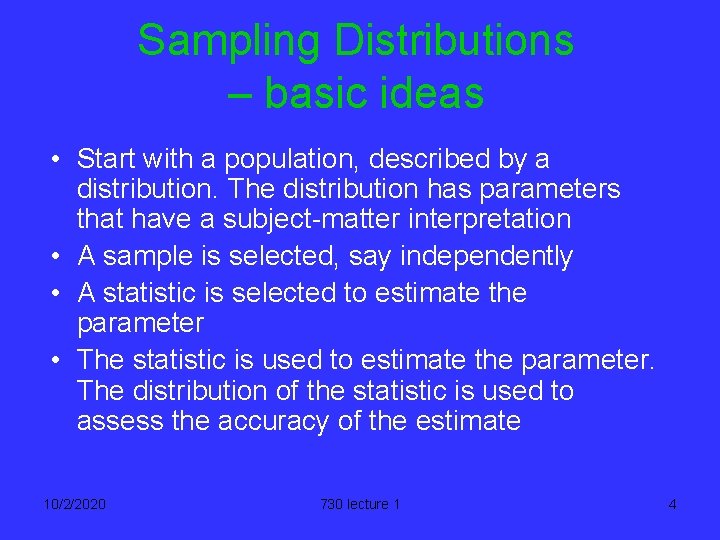
Sampling Distributions – basic ideas • Start with a population, described by a distribution. The distribution has parameters that have a subject-matter interpretation • A sample is selected, say independently • A statistic is selected to estimate the parameter • The statistic is used to estimate the parameter. The distribution of the statistic is used to assess the accuracy of the estimate 10/2/2020 730 lecture 1 4
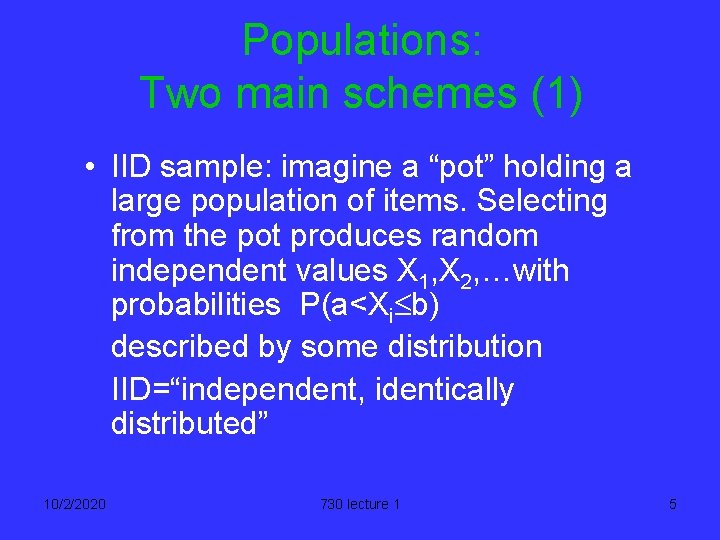
Populations: Two main schemes (1) • IID sample: imagine a “pot” holding a large population of items. Selecting from the pot produces random independent values X 1, X 2, …with probabilities P(a<Xi£b) described by some distribution IID=“independent, identically distributed” 10/2/2020 730 lecture 1 5
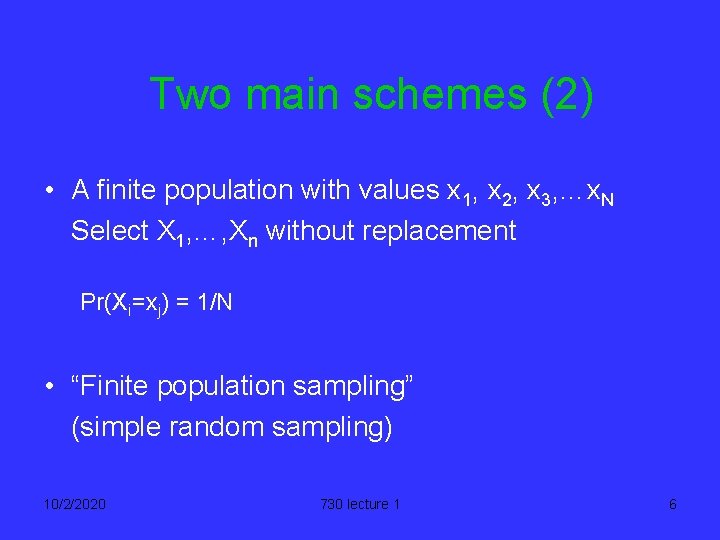
Two main schemes (2) • A finite population with values x 1, x 2, x 3, …x. N Select X 1, …, Xn without replacement Pr(Xi=xj) = 1/N • “Finite population sampling” (simple random sampling) 10/2/2020 730 lecture 1 6
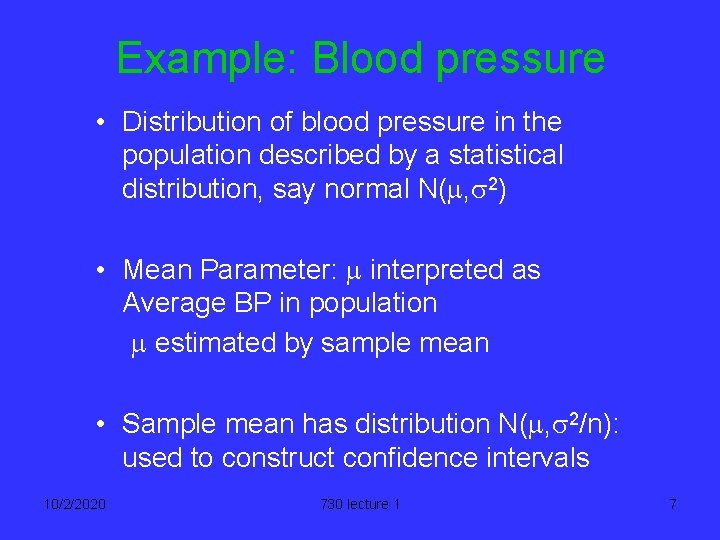
Example: Blood pressure • Distribution of blood pressure in the population described by a statistical distribution, say normal N(m, s 2) • Mean Parameter: m interpreted as Average BP in population m estimated by sample mean • Sample mean has distribution N(m, s 2/n): used to construct confidence intervals 10/2/2020 730 lecture 1 7
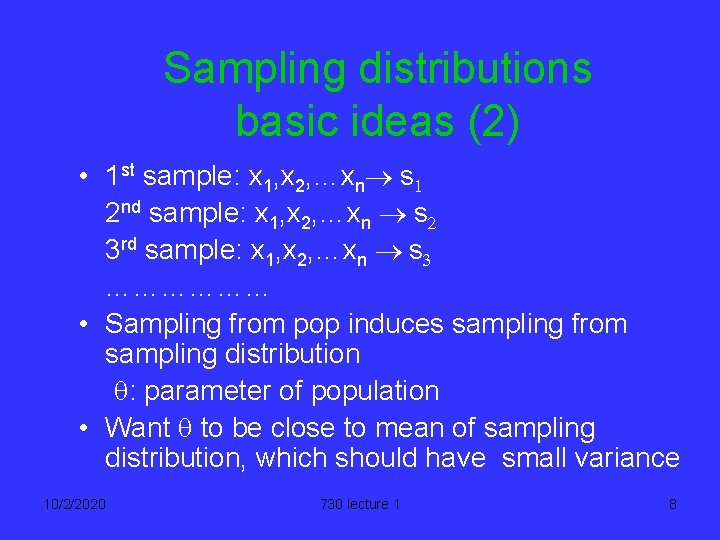
Sampling distributions basic ideas (2) • 1 st sample: x 1, x 2, …xn® s 1 2 nd sample: x 1, x 2, …xn ® s 2 3 rd sample: x 1, x 2, …xn ® s 3 ……………… • Sampling from pop induces sampling from sampling distribution q: parameter of population • Want q to be close to mean of sampling distribution, which should have small variance 10/2/2020 730 lecture 1 8
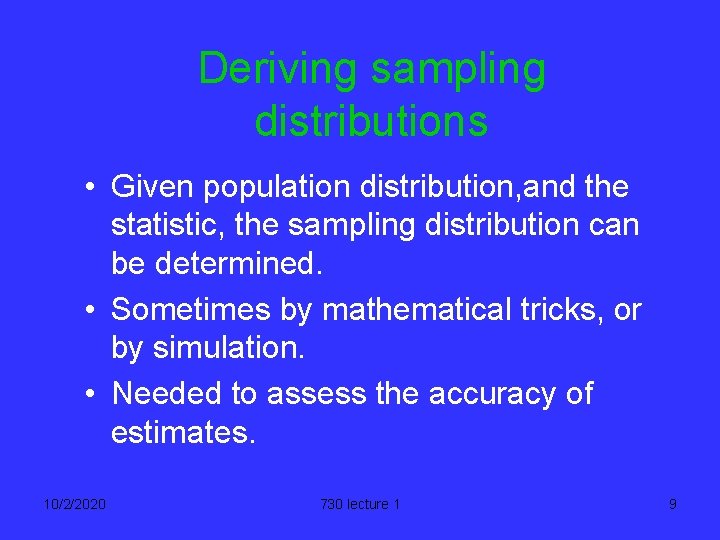
Deriving sampling distributions • Given population distribution, and the statistic, the sampling distribution can be determined. • Sometimes by mathematical tricks, or by simulation. • Needed to assess the accuracy of estimates. 10/2/2020 730 lecture 1 9
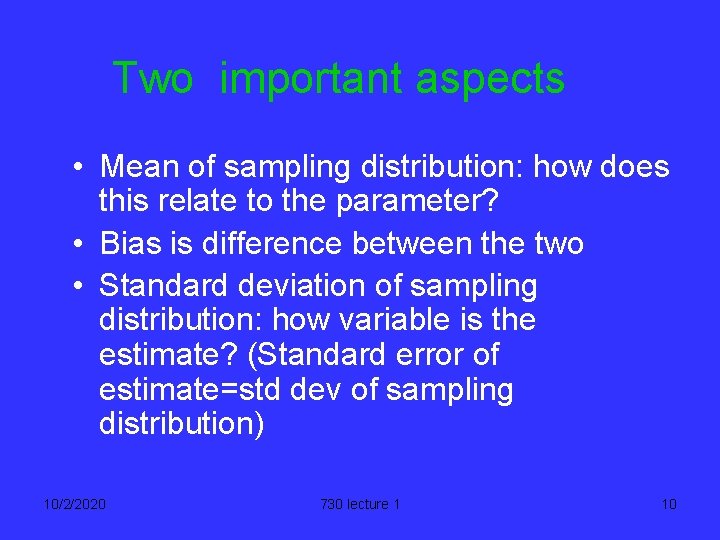
Two important aspects • Mean of sampling distribution: how does this relate to the parameter? • Bias is difference between the two • Standard deviation of sampling distribution: how variable is the estimate? (Standard error of estimate=std dev of sampling distribution) 10/2/2020 730 lecture 1 10
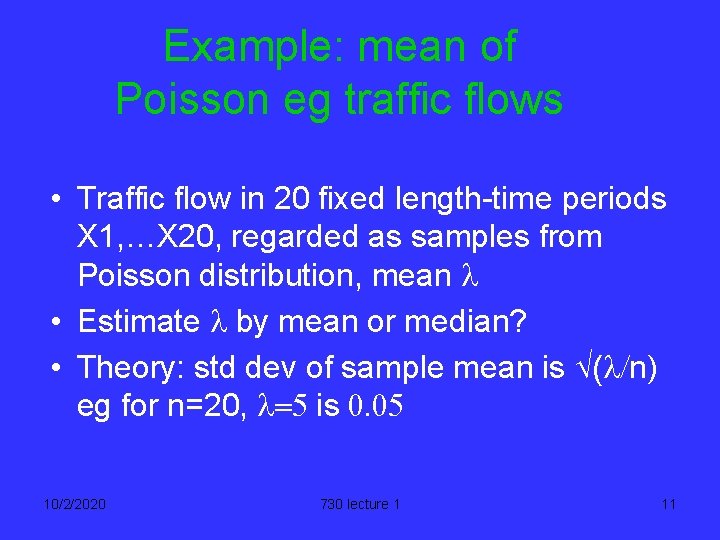
Example: mean of Poisson eg traffic flows • Traffic flow in 20 fixed length-time periods X 1, …X 20, regarded as samples from Poisson distribution, mean l • Estimate l by mean or median? • Theory: std dev of sample mean is Ö(l/n) eg for n=20, l=5 is 0. 05 10/2/2020 730 lecture 1 11
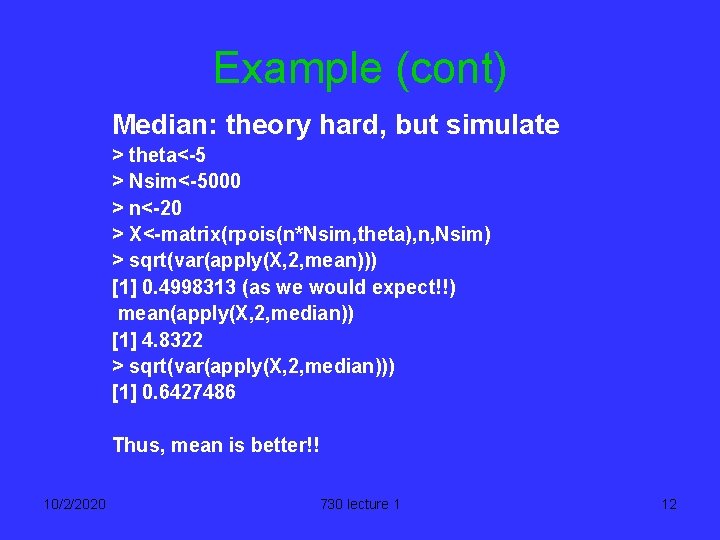
Example (cont) Median: theory hard, but simulate > theta<-5 > Nsim<-5000 > n<-20 > X<-matrix(rpois(n*Nsim, theta), n, Nsim) > sqrt(var(apply(X, 2, mean))) [1] 0. 4998313 (as we would expect!!) mean(apply(X, 2, median)) [1] 4. 8322 > sqrt(var(apply(X, 2, median))) [1] 0. 6427486 Thus, mean is better!! 10/2/2020 730 lecture 1 12
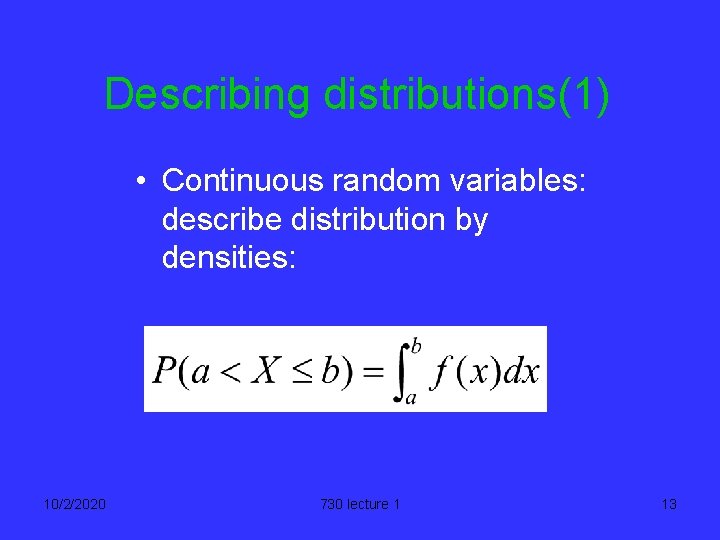
Describing distributions(1) • Continuous random variables: describe distribution by densities: 10/2/2020 730 lecture 1 13
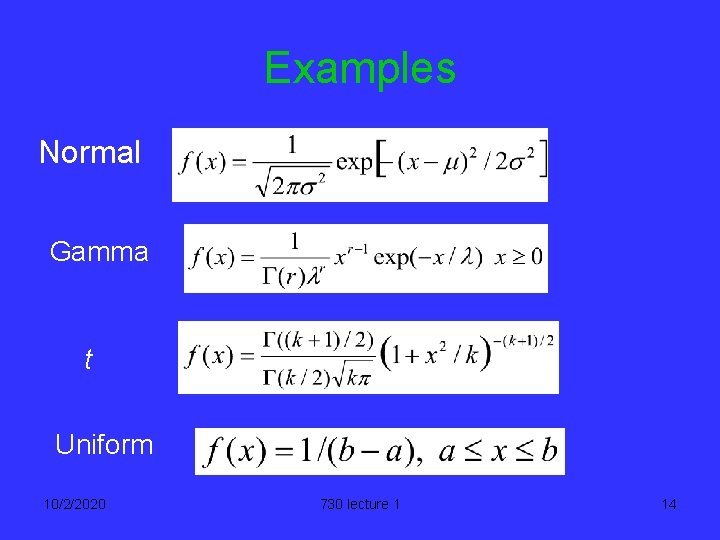
Examples Normal Gamma t Uniform 10/2/2020 730 lecture 1 14
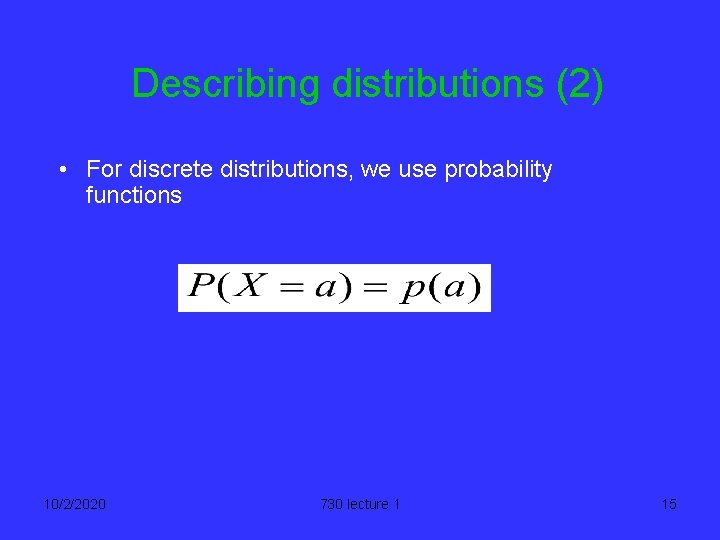
Describing distributions (2) • For discrete distributions, we use probability functions 10/2/2020 730 lecture 1 15
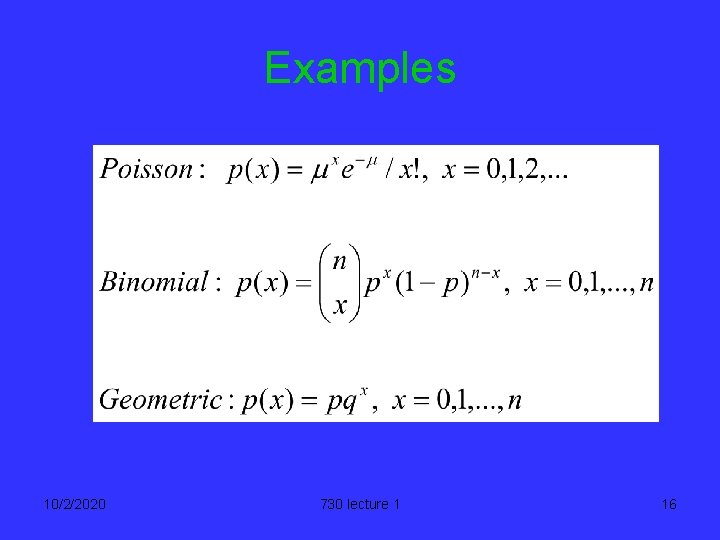
Examples 10/2/2020 730 lecture 1 16
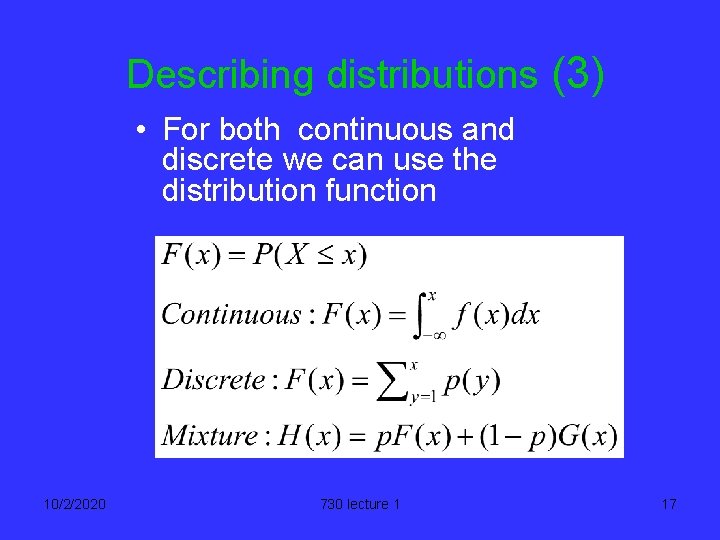
Describing distributions (3) • For both continuous and discrete we can use the distribution function 10/2/2020 730 lecture 1 17
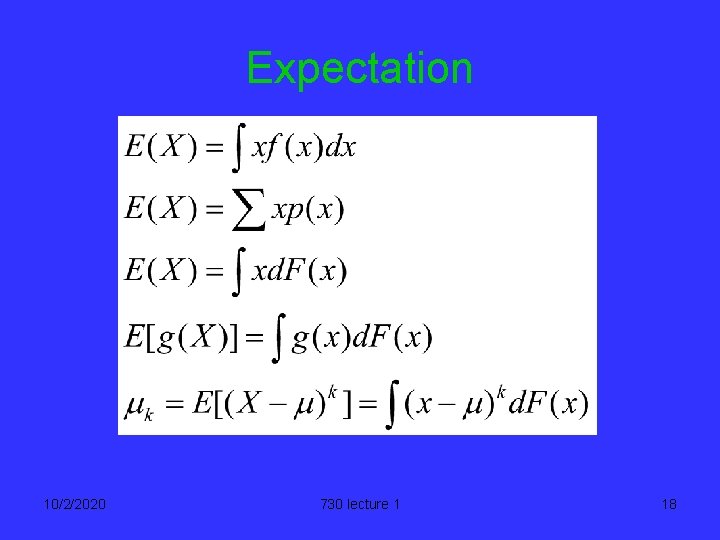
Expectation 10/2/2020 730 lecture 1 18
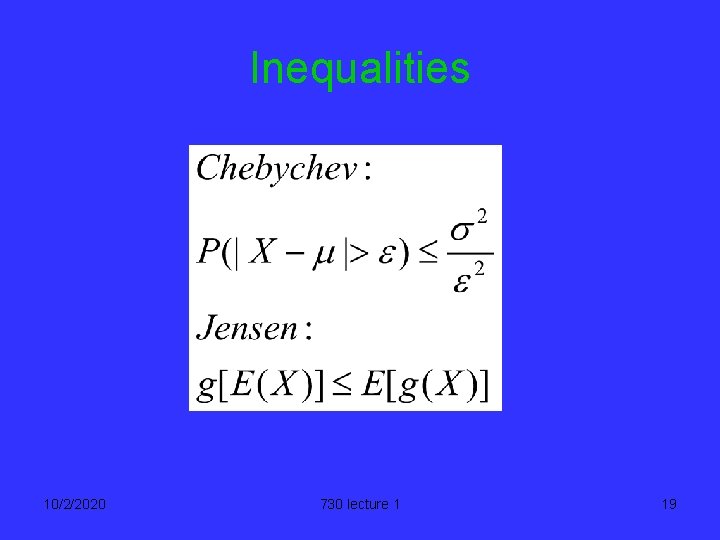
Inequalities 10/2/2020 730 lecture 1 19
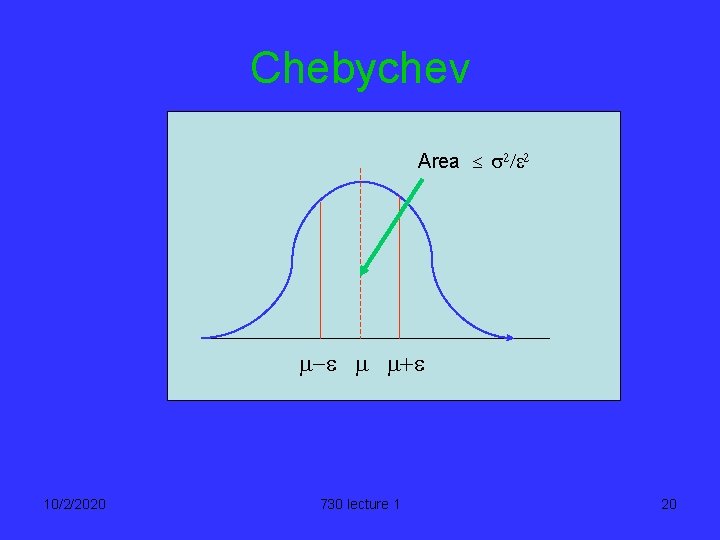
Chebychev Area £ s 2/e 2 m-e m m+e 10/2/2020 730 lecture 1 20
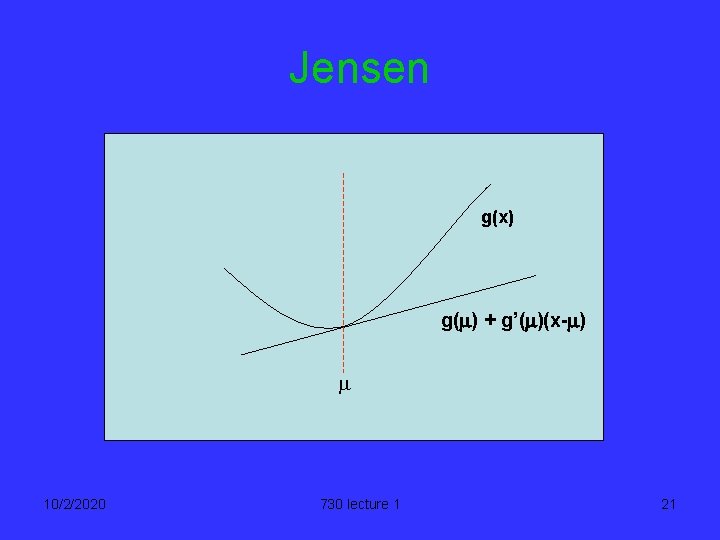
Jensen g(x) g(m) + g’(m)(x-m) m 10/2/2020 730 lecture 1 21
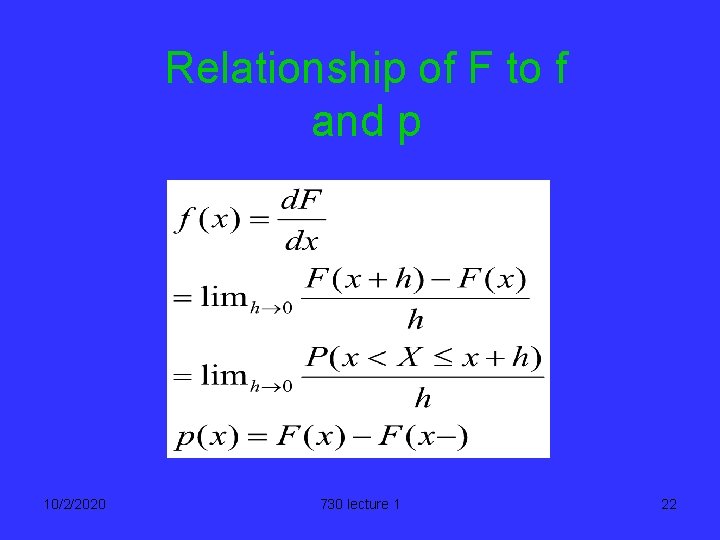
Relationship of F to f and p 10/2/2020 730 lecture 1 22
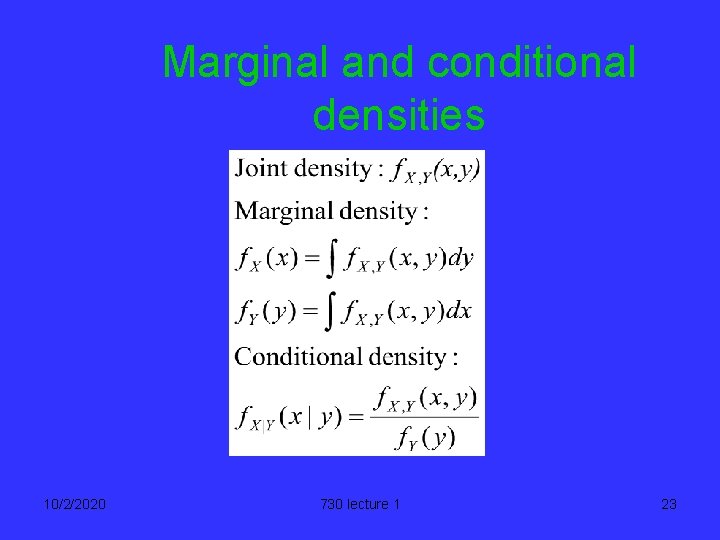
Marginal and conditional densities 10/2/2020 730 lecture 1 23
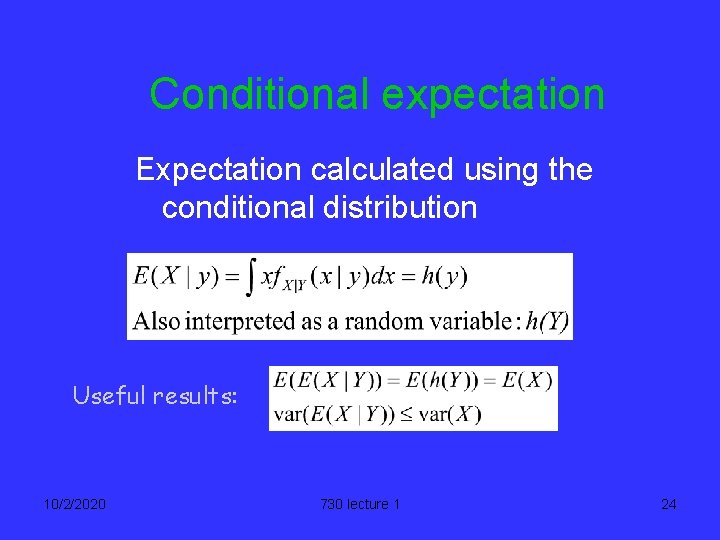
Conditional expectation Expectation calculated using the conditional distribution Useful results: 10/2/2020 730 lecture 1 24
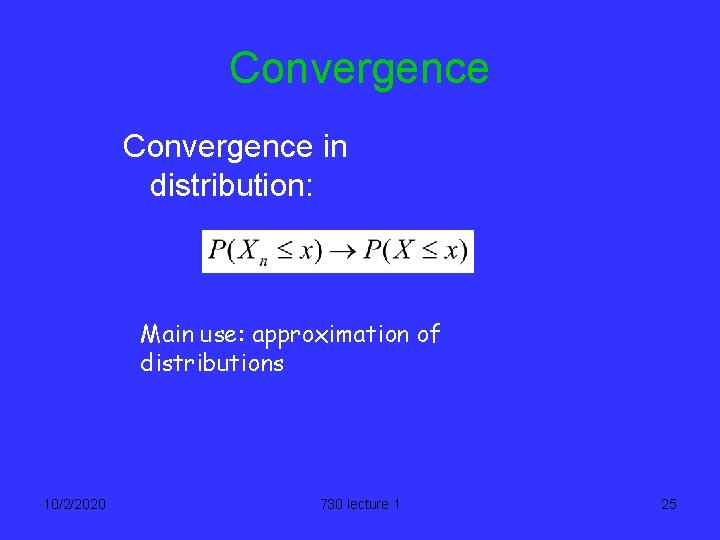
Convergence in distribution: Main use: approximation of distributions 10/2/2020 730 lecture 1 25
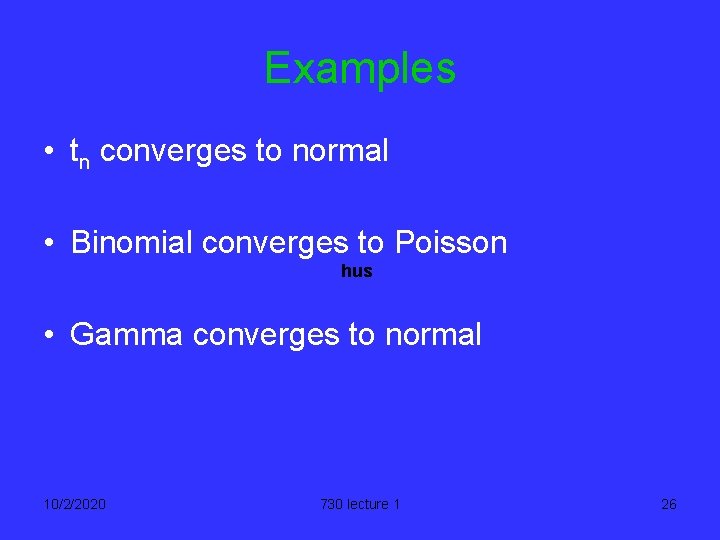
Examples • tn converges to normal • Binomial converges to Poisson hus • Gamma converges to normal 10/2/2020 730 lecture 1 26
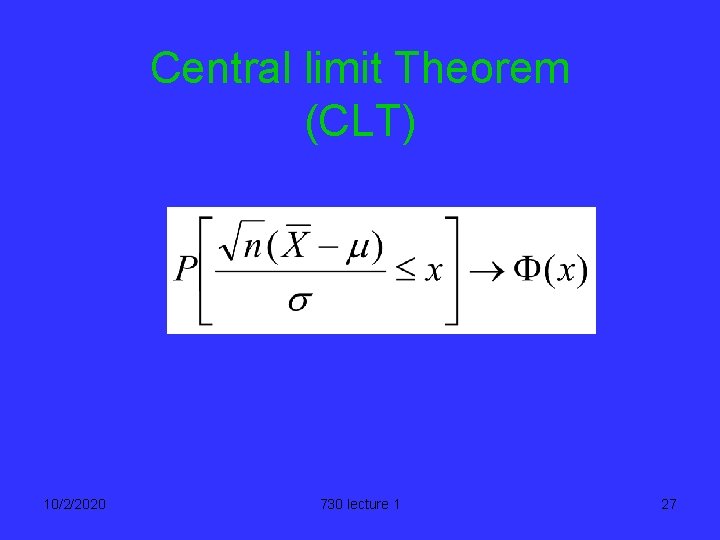
Central limit Theorem (CLT) 10/2/2020 730 lecture 1 27
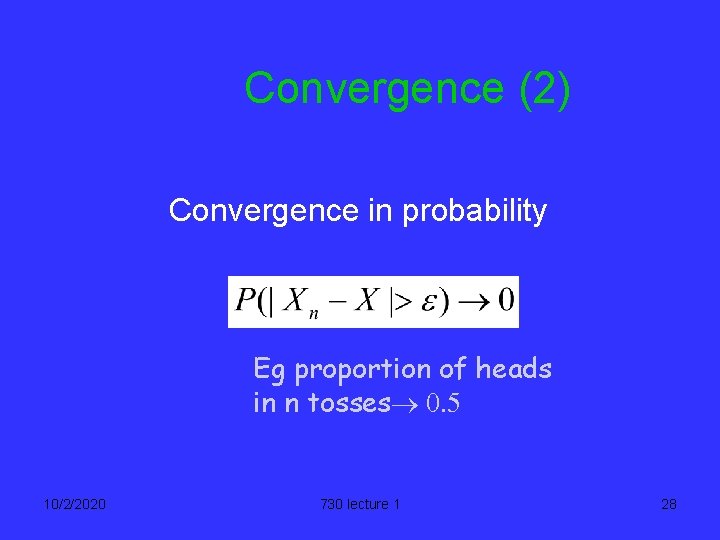
Convergence (2) Convergence in probability Eg proportion of heads in n tosses® 0. 5 10/2/2020 730 lecture 1 28
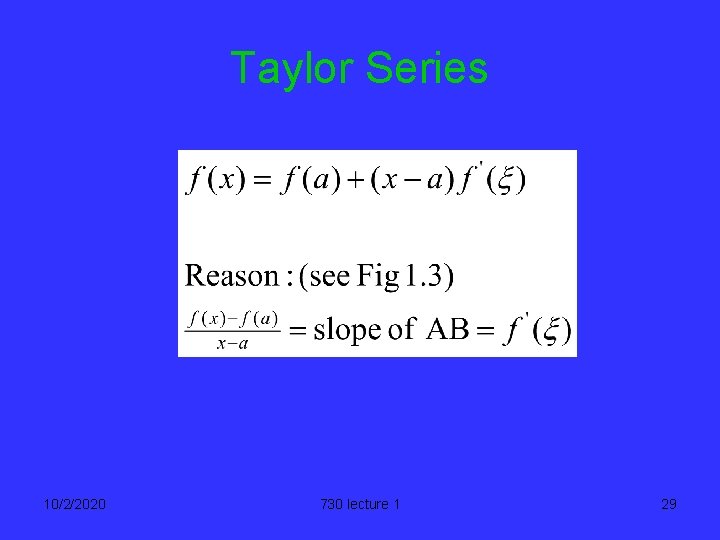
Taylor Series 10/2/2020 730 lecture 1 29
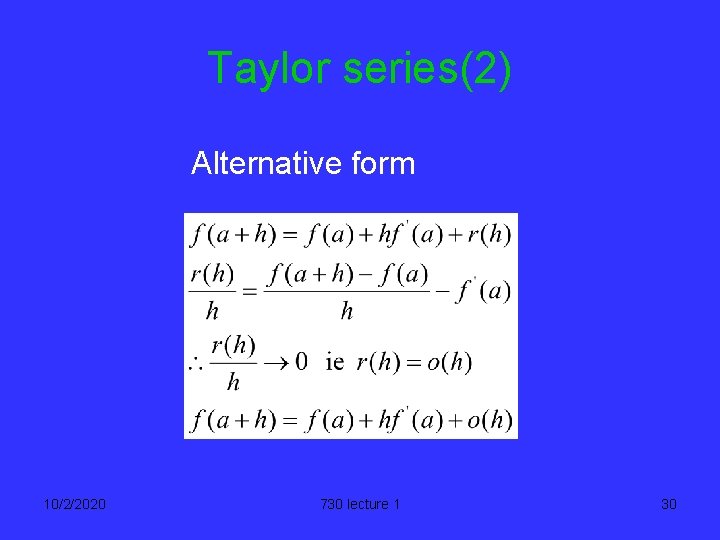
Taylor series(2) Alternative form 10/2/2020 730 lecture 1 30
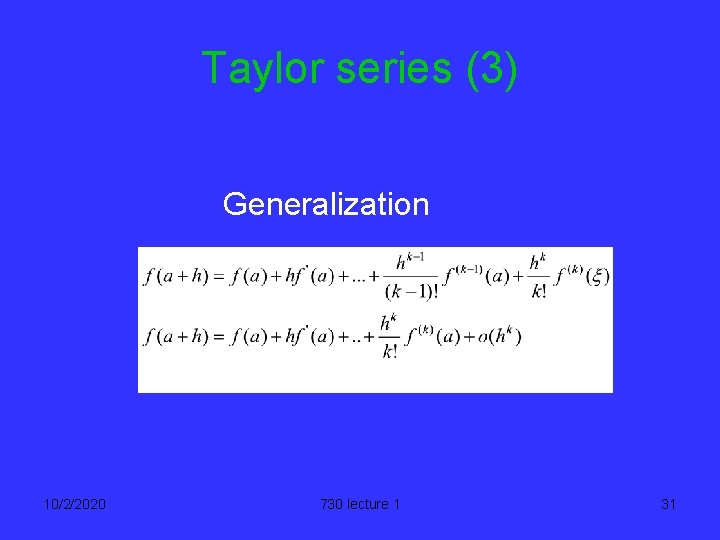
Taylor series (3) Generalization 10/2/2020 730 lecture 1 31
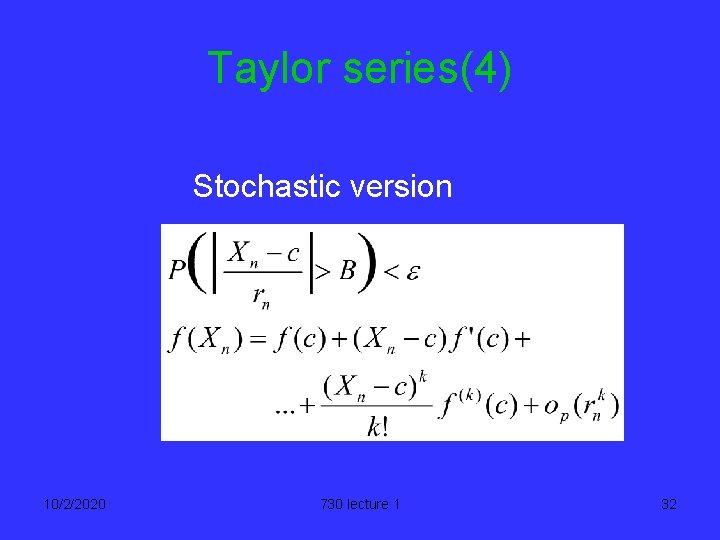
Taylor series(4) Stochastic version 10/2/2020 730 lecture 1 32
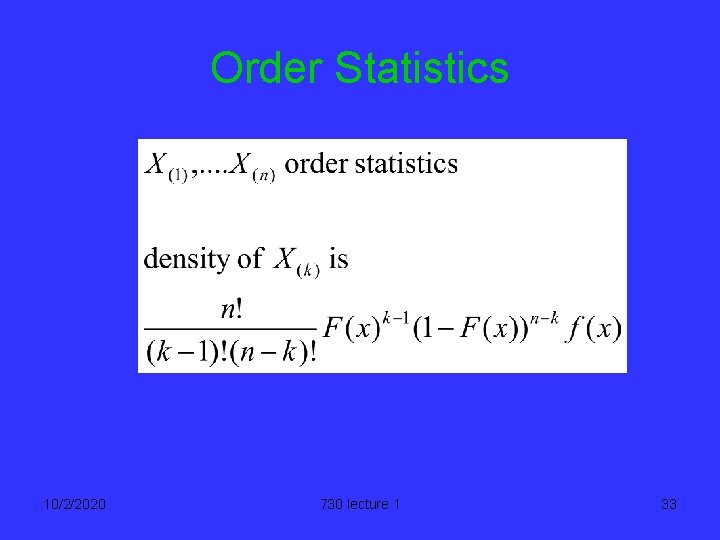
Order Statistics 10/2/2020 730 lecture 1 33
Ieee 730
730-170
Sps 730
Uniformity
53 en yakın onluğa yuvarlama
Ars 28-730
01:640:244 lecture notes - lecture 15: plat, idah, farad
Statsmodels.stats.weightstats.ztest
Questioning stance
Stats canada
Freesurfer color lut
Nonfiction signposts contrasts and contradictions
Example of testing hypothesis
Riak cs stats
Combined standard deviation formula
Statistics symbols
#sessions with conversions
Ap stats chapter 16
Ohio university stats
Mobile commerce stats
Stats general addition rule
Numbers and stats
Conditions for regression inference
Statistics variance
What is statistics
My stat
Statistics for beginners
Ogive curve
Chapter 21 comparing two proportions
Stats
Parameter example statistics
Stats p hat
Stats table psychology
Mrs daniels ap stats